- 1 Experimental Psychology, Helmholtz Institute, Universiteit Utrecht, Utrecht, Netherlands
- 2 Faculty of Human Movement Sciences, Vrije Universiteit, Amsterdam, Netherlands
Evidence for contextual effects is widespread in visual perception. Although this suggests that contextual effects are the result of a generic property of the visual system, current explanations are limited to the domain in which they occur. In this paper we propose a more general mechanism of global influences on the perception of slant. We review empirical data and evaluate proposed explanations of contextual biases. By assessing not only a model about three-dimensional slant perception but also evaluating more generic mechanisms of contextual modulation, we show that surround suppression of neural responses explains the major phenomena in the empirical data on contextual biases. Moreover, contextual biases may be part of a mechanism of grouping and segmentation.
Introduction
Human observers perceive three-dimensional (3D) forms from two-dimensional projections on the retinas. Vision science has largely unraveled how the visual system achieves this task by combining information from different depth cues such as binocular disparity, motion, and perspective. However, perception of a surface is influenced not only by information within the surface but also by information in its spatial context. Although naive observers probably only notice this when assembling a color pallet for their daily outfit, perception of a visual stimulus is influenced by adjacent stimuli. A straight line may appear tilted when presented on an oriented grating (Figure 1A), a gray patch may appear lighter on dark surface (Figure 1B) and a stationary stimulus may appear moving leftward in a rapidly rightward moving surround. Perhaps even less apparent to the naive observer, these contextual biases also occur in the perception of 3D shape. When a central slanted surface is flanked by a larger surface, perceived slant depends on the flanking surface’s slant. In contrast biases, relative differences with adjacent stimuli are perceptually enhanced. This causes a moderately slanted surface to appear more slanted when it is presented between two surfaces with shallower slant and less slanted when the adjacent surfaces have a steeper slant. In assimilation biases, perception of a central stimulus assimilates to surrounding stimuli, which causes a surfaces slant to appear more like the slant of adjacent stimuli (Figure 1C).
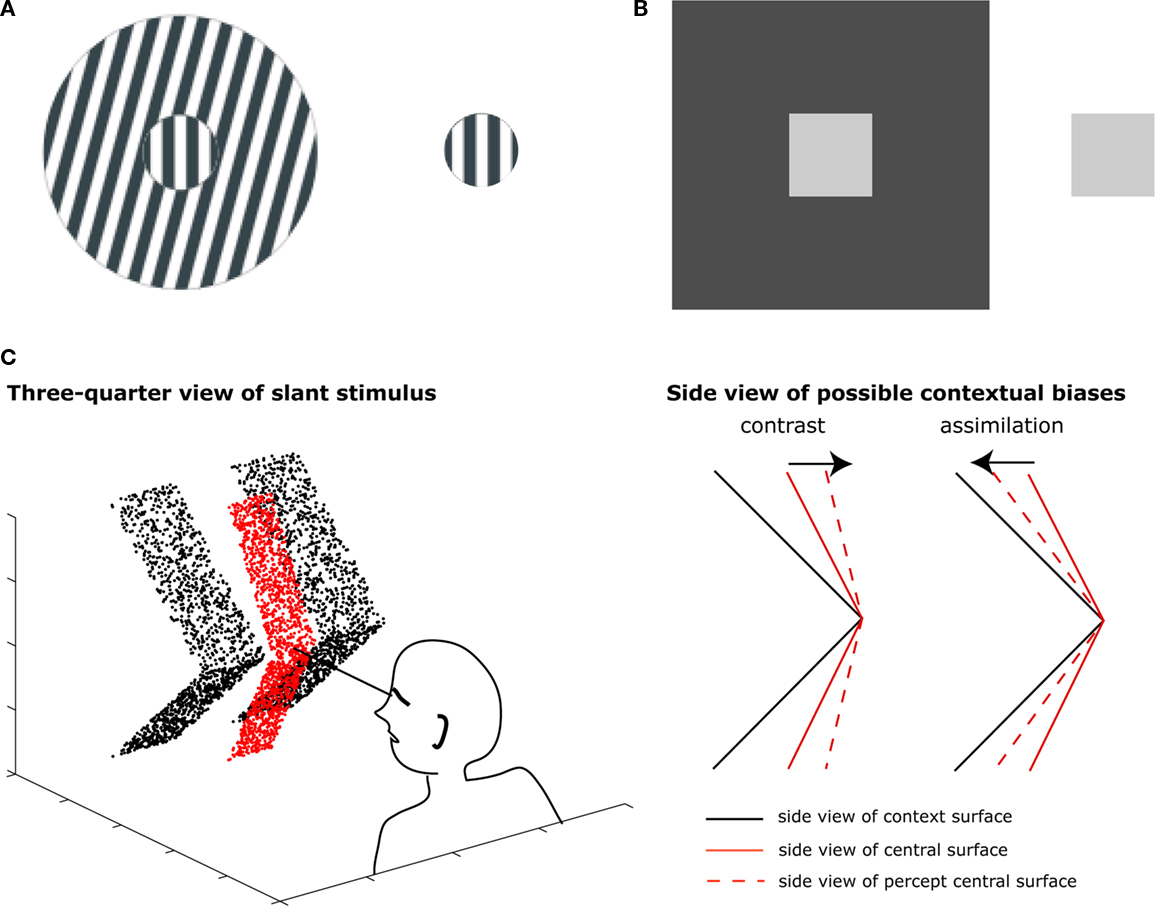
Figure 1. (A) Tilt illusion. The vertical grating appears leftward tilted in the rightward tilted surround. (B) Color contrast. The gray patch appears lighter when presented on the darker surface. (C) Side view of stimulus used to induce slant contrast (van der Kooij and Te Pas, 2009a,b, 2010) and cartoon illustration of contrast and assimilation biases that may occur in this stimulus.
Contrast and assimilation biases cause large discrepancies between perception and physical shape and therefore pose a challenge to understanding how we successfully interact with our environment. Imagine for instance placing a full glass on a table when we misestimate the tables slant relative to its surroundings. Despite their fundamental importance, contextual biases in 3D perception are not well understood (Gillam et al., 2007). Explanations of contextual biases in slant perception have mainly been sought in the observation that contextual surfaces provide relative information about shape (i.e., a surface is more slanted than the adjacent surface). However, none of the resulting hypotheses explains the full range of phenomena in contextual biases, as we will explain in the next paragraph.
More insight may be gained from the fact that contextual biases occur in the perception of a wide range of features including tilt (e.g., Wenderoth and Johnstone, 1988; Westheimer, 1990), motion (e.g., Tadin et al., 2006), size (Ebbinghaus illusion), curvature (e.g., Curran and Johnstone, 1996) and slant (e.g., Rogers and Graham, 1982), which suggests that these biases have a common mechanism. Especially biases in tilt perception have been well studied, and are commonly referred to as the tilt illusion. Psychophysical experiments have measured how tilt perception varies when a tilted stimulus is presented with surrounds of different tilt and have revealed a robust function of how biases depend on relative differences (e.g., O’Toole and Wenderoth, 1977; Westheimer, 1990; Solomon et al., 2004). On the neural level, physiological experiments have revealed how the amplitude of neural responses to tilt is modified by presenting another tilted stimulus in the surround of the receptive field (e.g., Sengpiel et al., 1997; Cavanaugh et al., 2002). Importantly, computational studies have been able to predict perceptual biases from physiological data showing a causal relation between the two phenomena (e.g., Clifford et al., 2000; Schwartz et al., 2009). Proposed mechanisms for the tilt illusion may also apply to contextual biases in 3D perception.
Contextual biases in slant perception have not been subject to detailed study of how biases vary as a function of slant differences with the surround, as most studies have measured biases with no more than two different contextual slants. Contextual biases in slant perception have been subject to very different types of study. Because these biases have been attributed mainly to the process of 3D estimation, psychophysical studies have focused on how biases vary with the available 3D information in the stimulus (van Ee et al., 1999; Sato and Howard, 2001; Poom et al., 2007; van der Kooij and Te Pas, 2009a). In this paper, we take a broader perspective and assemble data from different studies to reveal the basic properties of how contextual biases in slant perception vary as a function of slant differences with the surround. This way we can compare empirical data from the literature not only to theories of slant processing from a combination of depth cues but also to mechanisms that have been proposed for contextual biases in tilt perception and that are based on the processing of differences between stimuli. With this comparison, we show that taking such a broad perspective offers insight in the mechanisms and functionality of contextual biases in slant perception and are able to outline the important novel questions.
The typical experiment on contextual biases in slant perception, presents observers with a stereogram, which depicts a central surface with two larger adjacent surfaces of a different slant. In these stereograms, slant is defined by binocular disparity. Quantitative measures of slant perception can be derived in three ways. In a discrimination task, comparisons are made with a reference stimulus that is presented at an earlier or later time interval (van der Kooij and Te Pas, 2009a,b, 2010). In a matching task, the slant of a haptic comparison stimulus is adjusted to match a visual test stimulus (Sato and Howard, 2001; Gillam et al., 2007). In a nulling task, the slant of the test stimulus is adjusted until it is a perceptually fronto-parallel (van Ee et al., 1999; Sato and Howard, 2001; Poom et al., 2007). A comparison of contextual biases found in these studies shows that the method used does not significantly affect the results. Therefore we are able to compare data from different experiments to theories of contextual biases. We start with an explanation that is rooted in computational theories of slant processing from a combination of depth cues and next discuss a more general explanation that has been proposed for contextual biases in tilt perception.
Bayesian Estimation of Slant
Slant perception can be described in a Bayesian model of perceptual inference, which has successfully been used to explain a wide range of phenomena in visual perception. In A Bayesian model of slant perception, the visual system overcomes uncertainty by combining different slant signals from the environment, such as binocular disparity, motion, and perspective with an internal model of the probability to encounter a certain slant in the environment (prior). Combination occurs by taking a weighted average of slant signals. This means that when a slant signal, say binocular disparity, is unreliable, it will have less influence on perception and other slant signals, such as accommodation and perspective, as well as the prior, will have more effect. Such Bayesian models successfully describe how 3D slant is estimated from a combination of depth cues (e.g., Landy et al., 1995; Knill and Saunders, 2003). In the next paragraph, we address the issue whether these models are also applicable to the perception of slant in context.
In theory, a Bayesian model can incorporate contextual effects by treating contextual surfaces as relative cues to slant, which are also weighted. Typically, the visual system is rather insensitive to scale and shear within a surface (Shipley and Hyson, 1972; Mitchison and Westheimer, 1984; Gillam et al., 1988; Stevens and Brookes, 1988; van Ee and Erkelens, 1996; van Ee et al., 1999) and is more sensitive to differences between surfaces (Gillam et al., 1984; van Ee and Erkelens, 1996). Therefore, relative information is considered the more reliable cue and, together with underestimation of absolute slant, this is thought to cause a contrast bias (van Ee and Erkelens, 1996; van Ee et al., 1999). Straightforward as this rationale may seem, it is unclear how the visual system combines absolute and relative information to slant. First, an absolute cue to slant specifies the orientation of a surface whereas a relative cue specifies the slant relative to a reference. Hence, averaging of the absolute and relative cue can only produce veridical perception after cues have been gaged to an appropriate standard (i.e., the mean slant in the stimulus). Gaging relative information to an inappropriate standard can cause biased perception. Second, standard cue combination rules (Ernst and Banks, 2002) are based on the assumption of uncorrelated variances. In the case of relative and absolute disparity cues, independence cannot be assumed. One computational study addresses this issue and shows that combination of cues with correlated variances leads to less reliable estimates then when combining uncorrelated cues (Oruç et al., 2003). However, the proposed model fitted the data of only some of the observers and the empirical part of this study does not allow for definitive conclusions. How absolute and relative information are combined remains an important open question.
One model of slant perception made an attempt to formalize how absolute and relative information are combined (van Ee et al., 1999). This model attributes contextual biases in slant perception to the laboratory setting where stereograms are presented on a flat computer screen, which introduces cue conflict: a single cue (say binocular disparity) signals a slanted surface whereas other cues such as accommodation and perspective signal a flat surface. Combination of slant by disparity and other cues results in an estimate of absolute slant that is shallower than disparity-defined slant. A “contrast” bias occurs because a relative disparity signal is gaged to an underestimation of the slant of the contextual surface.
Conflict between disparity and perspective cues may play an important role in contextual biases: in the van Ee et al. (1999) model, slant of the contextual surface is only underestimated due to combination of monocular flatness cues with slant cues from disparity. We call this explanation of contextual biases the “cue conflict hypothesis.” The cue conflict hypothesis is supported by the finding that contrast biases depend on an individuals use of perspective cues (Sato and Howard, 2001) and almost disappear for real surfaces (van Ee et al., 1999). However, in those studies, the effect of cue conflict may be confounded with an effect of reliability. Observers who rely more on monocular cues usually have poorer stereovision (Landy et al., 1995; Knill and Saunders, 2003; Hillis et al., 2004) and thus receive less reliable information from the stimuli. Reliability of the central and contextual stimulus affects the amplitude of contextual biases (van der Kooij and Te Pas, 2009b). Thus evidence that contrast biases depend on the reliability of available depth cues does not serve as compulsory support for the cue conflict hypothesis.
To recall, in a Bayesian model contextual biases occur by gaging relative cues to an inappropriate perceived contextual plane. Besides cue conflict, normalization towards a fronto-parallel background plane can cause underestimation of the contextual plane, which has been called the normalization hypothesis (Gillam et al., 1988, 2007; Sato and Howard, 2001; Gillam and Pianta, 2005). Gaging relative cues to an underestimation of contextual slant causes contrast biases when the contextual surface is more slanted than the central surface. However, when the contextual surface has a slant with the same sign as the contextual surface but is relatively less slanted, the model predicts an assimilation bias instead of a contrast bias (Figure 2). This prediction does not hold, as equivalent biases have been found when the contextual surface has a larger or smaller slant compared to the test surface (van der Kooij and Te Pas, 2009a,b). So, gaging relative cues to an underestimation of slant predicts a shift in slant estimates, not the contrast enhancement found in psychophysical experiments.
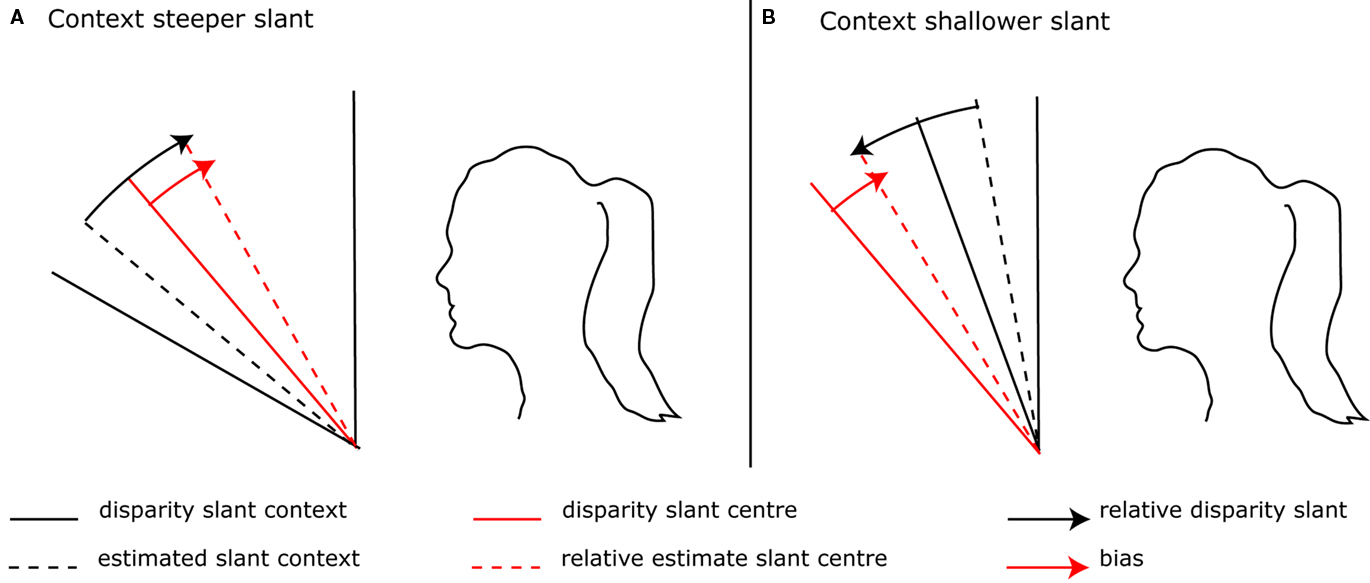
Figure 2. Gaging relative disparity to an underestimation of contextual slant. (A) When the context has a steeper slant than the central surface a contrast bias occurs. (B) But when the context has a shallower slant than the central surface, an assimilation instead of contrast bias occurs.
Finally, uncertainty plays an important role in Bayesian models, and they should be able to explain how perception depends on the reliability of information. When uncertainty is added to the central surface by randomly displacing the dots on the surface, contextual biases reverse direction from contrast to assimilation (van der Kooij and Te Pas, 2009b). This change from contrast to assimilation is not explained by modeling contextual surfaces as an extra cue to slant. Within a Bayesian framework, the change from contrast to assimilation can be explained by the prior, or the probability to encounter a certain slant. This prior is combined with slant signals from the environment and gains influence when information is unreliable. The change from contrast to assimilation would then occur because the influence of the prior, which causes assimilation, only becomes visible at a certain threshold. Specifically, a prior for spatial smoothness can cause an assimilation bias. However, the use of priors for global scene characteristics of 3D images is largely uninvestigated.
To conclude, for Bayesian frameworks to be applicable to contextual biases in slant perception, two important questions have to be answered experimentally. First, the combination of absolute and relative information to slant needs to be investigated more thoroughly. Second, measurements of natural scene statistics in 3D images may be able to provide insight in priors for 3D properties. However, Bayesian models of slant perception from a combination of depth cues may not apply to explaining contextual biases because the mechanism that causes contextual biases is not specific to 3D perception. Contextual biases in different depth cues (Te Pas et al., 2000) or features such as luminance, depth, color and motion (e.g., Anstis and Howard, 1978; Paffen et al., 2006) show commonalities and may have a common substrate. Contrast biases in the perception of slant, tilt (see Schwartz et al., 2007 for a review) and motion (Baker and Graf, 2010) depend on relative differences between the center and surround and appear to saturate at large differences. Furthermore, when the central stimulus is weak or unreliable, contextual biases in the perception of slant (van der Kooij and Te Pas, 2009a), tilt (Mareschal et al., 2010) and motion (Hanada, 2004) change from contrast to assimilation. These similarities lead us to investigate whether explanations of contextual biases in 2D features also apply to biases in 3D slant perception.
Surround Suppression
Contextual biases in 2D features are commonly explained by the observation that contextual stimuli affect not only perception of a central stimulus but also neural responses to this stimulus. In the phenomenon of “surround suppression,” the neural response to a stimulus presented on its receptive field is reduced when a similar stimulus is presented to in the surround of its receptive field. Surround suppression has been found throughout the cortex in areas ranging from the primary visual cortex (e.g., Carandini et al., 1997; Ringach, 2010), to area IT (Zoccolan et al., 2005), and area MT (Simoncelli and Heeger, 1998), which processes disparity and motion gradients, used for estimating slant (Xiao et al., 1997; Nguyenkim and DeAngelis, 2003).
Surround suppression of neural responses to similar stimuli has become a popular explanation for biases in the perception of tilt (e.g., Schwartz et al., 2009; Mareschal et al., 2010) and motion (e.g., Nakayama and Loomis, 1974; Paffen et al., 2004; Baker and Graf, 2010). Surround suppression has also been mentioned as a possible explanation for slant contrast biases (Anstis and Howard, 1978; Schumer and Ganz, 1979; Mitchison and Westheimer, 1984; Brookes and Stevens, 1989) but a computational explanation of how suppression causes contrast biases has only been put forth for biases in tilt and motion perception. We focus on mechanisms that have been proposed for tilt perception because tilt and slant are both orientations and are similar in feature space, although tilt does not require 3D processing. These proposed mechanisms rely on the concept that visual features are decoded from populations of neurons. Suppression of individual neurons responses to similar stimuli reduces their contribution to the population code, which may be derived by taking the maximal response or population vector (Gilbert and Wiesel, 1990; Jin et al., 2005; Schwartz et al., 2009). Because suppression removes similarities from this population code, it causes a contrast bias with the contextual stimuli.
If contextual biases depend on surround suppression, as contextual biases in tilt perception do, this implies that surround suppression and contextual biases in slant perception depend similarly on information from the environment. Indeed, there are striking parallels between surround suppression of neural responses to tilt and contextual biases in slant perception. First, the two phenomena depend in a similar fashion on relative differences. Surround suppression is the largest when the contextual stimulus falls onto the suppressive surround of the receptive field coding for the central stimulus and wears off when the contextual stimulus approaches the facilitative center of the receptive field coding for the central stimulus or when the contextual slant falls of the central receptive field entirely (Cavanaugh et al., 2002). Consistently, contextual biases in the perception of tilt (e.g., Solomon et al., 2004) and motion (e.g., Baker and Graf, 2010) first increase and than saturate at larger center-surround differences (Figures 3A,B). For biases in tilt perception, contrast is abolished at very large angle differences. Such large angle differences between the center and context have not been measured for slant perception.
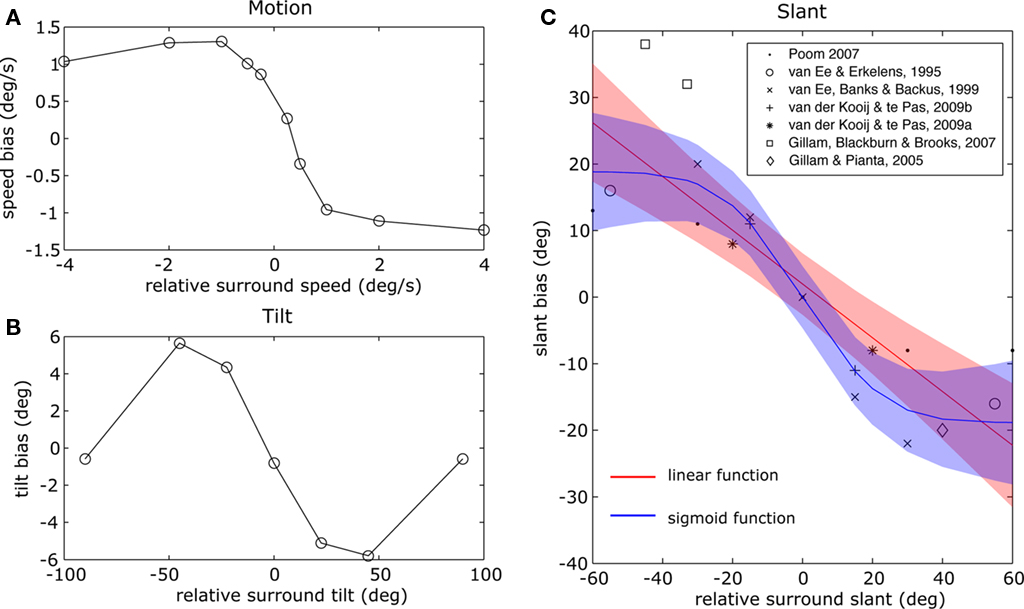
Figure 3. (A) Biases in speed perception replotted from Baker and Graf (2010). (B) Biases in tilt perception replotted from Solomon et al., 2004. Note how the angle differences for which contrast biases disappear or change to assimilation have not been measured for slant perception. (C) Slant contrast biases, measured in dfferent experiments plotted as a function of slant difference with the surround, fitted with a linear (red line) and sigmoid (blue line) function. The confidence interval for the prediction is plotted in transparent color. It can be observed that at large slant differences, the bias function saturates or decreases.
To reveal how contextual biases in slant perception vary with relative slant, we plotted contrast biases observed in different experiments as a function of the inducer slant minus the test slant (Figure 3C). We fitted two simple functions to the data: a linear function, which does not saturate at large differences and a sigmoid function, which does saturate at large differences. Our aim is not to fit a biologically plausible model but to reveal the basic properties of the contrast function. The sigmoid function explains more of the variance (X2 = 3.37) than the linear function (X2 = 5.09), which implies that contrast biases saturate at larger differences. Moreover, inspection of the graph shows that biases may even decrease at larger differences, as biases in tilt perception do.
Another important commonality between surround suppression and contextual biases in slant perception is that they both reverse in polarity when visual information is impoverished. Perceptual biases in slant perception reverse from contrast to assimilation with added noise (van der Kooij and Te Pas, 2009b) and surround suppression of neural responses to tilt reverses from suppression to facilitation, as measured by the spiking rate of macaque cortical neurons (Polat et al., 1998) and human BOLD responses (Tajima et al., 2010). Lowered contrast and decreased correlation (added noise) have analogous effects on surround suppression in the cat lateral geniculate nucleus (LGN) responses to luminance (Lesica et al., 2007). Thus, the shift from a contrast to assimilation bias in slant perception with added noise (van der Kooij and Te Pas, 2009b) may be caused by a shift from neural repulsion to facilitation, as has been proposed for contextual biases in tilt perception (Mareschal et al., 2010).
Similarities in how surround suppression and contextual biases in slant perception depend on relative differences and stimulus strength makes surround suppression a promising neural explanation for contextual biases in slant perception. This brings forth interesting novel questions. Psychophysical measurements will be able to reveal whether contextual biases and surround suppression depend in a similar way on stimulus contrast and whether contrast biases decrease at large angle differences, as biases in tilt perception do (Westheimer, 1990; Solomon et al., 2004). Physiological experiments can reveal how neural responses to slant are suppressed by neighboring stimuli. Finally, novel fMRI techniques allowing for population receptive field measurements (Dumoulin and Wandell, 2008), will be able show a causal link between biases on the neural and perceptual level in humans. Questions on the functionality of contextual biases, however, remain unanswered by the mechanistic explanation of surround suppression. In a final paragraph we discuss recent theories that attribute contextual biases in 2D perception to a mechanism of grouping and segmentation. If these theories apply to slant perception within spatial context, they may help uncover the functionality of contextual biases.
Grouping and Segmentation
On the functional level, surround suppression and consequent perceptual effects may be part of a mechanism of grouping and segmentation, which highlights differences at the borders between groups (e.g., Nakayama and Loomis, 1974; Zipser et al., 1996; Schwartz et al., 2009). In such a mechanism, responses to similar stimuli within a group are suppressed whereas responses to dissimilar stimuli on the borders between groups are not. We will focus on one recent computational model (Schwartz et al., 2009) that nicely predicts contextual biases in tilt perception. In this model, responses to similar stimuli from a common world source are reduced by a divisive normalization algorithm, where the response to the central stimulus is divided by the intensity of the contextual stimulus, and which has been widely applied to model cortical processing (e.g., Geisler and Albrecht, 1992; Heeger, 1992; Wilson and Humanski, 1993; Carandini et al., 1997). When target and context stimuli are held to originate from a different world source, the context is not included in the normalization pool of the target (Schwartz et al., 2009).
Whether two stimuli originate from a common source, and should be grouped, can be determined by taking advantage of the statistical dependency structure of natural images. For instance, the response of two neurons coding for similar tilt is often correlated whereas the response of two neurons coding for orthogonal tilt will be less correlated (Schwartz et al., 2009). Consistently, contrast is found for small angle differences, whereas assimilation is found for large angle differences (Westheimer, 1990; Schwartz et al., 2009). Interestingly, psychophysical data on contextual effects in other domains than tilt perception also show influences of the spatial correlation structure of the surround. Suppression of luminance is strongest when the second-order structure of the surround matches the structure of natural images (McDonald and Tadmor, 2006) and release from Vernier (horizontal offset) suppression occurs when the contextual stimuli group into a separate object (Herzog and Fahle, 2002; Sayim et al., 2010).
The hypothesis that contextual biases in slant perception are part of a mechanism of grouping and segmentation implies that contextual biases depend on global properties of a scene. Indeed, when a surface is presented within a string of 10 slanted surfaces, contextual biases depend not only on the slant of the adjacent surfaces but also on the slant distribution in the entire stimulus (van der Kooij and Te Pas, 2010). Furthermore, contextual biases depend not only on the slant of the central and contextual surface but also on the configuration in which they are presented (Gillam and Pianta, 2005; Gillam et al., 2007). Finally, bias size decreases with spatial separation (Gillam and Pianta, 2005; Poom et al., 2007). However, spatial proximity of the test and contextual surface not only affects grouping of the two surfaces, but also changes the disparity content of the stimulus.
Overall, empirical data on how contextual biases depend on global scene properties are sparse, but do support the idea that contextual biases depend on global context. The relation between contextual biases in slant perception and grouping is largely unexplored and the hypothesis that contextual biases are the result of a mechanism of grouping and segmentation brings forth fascinating novel questions on how perception of a surface depends on its spatial context. Contextual biases in slant perception may depend not only on the disparity content of a stimulus but also on unrelated surface features which determine grouping, such as color, texture, or common faith. Furthermore, contextual biases will depend on the spatial correlation structure of natural images. Psychophysical experiments testing such hypotheses and measurements of the orientation statistics of 3D images will be able reveal more on the functionality of contextual biases.
Conclusion
Bayesian models of slant perception from a combination of depth cues poorly explain contextual effects in slant perception. Before such a model can be used to quantify slant perception in complex scenes, questions on the combination of absolute and relative cues to slant will have to be answered. Also, natural scene statistics will have to be measured to elucidate the effect of prior experience with the environment.
At this point, more insight can be gained from explanations that have been proposed for contextual biases in other domains. Contextual biases in the perception of tilt (Gilbert and Wiesel, 1990; Schwartz et al., 2009; Mareschal et al., 2010) and motion (e.g., Nakayama and Loomis, 1974; Paffen et al., 2004; Baker and Graf, 2010) have been explained by surround suppression of neural responses to similar stimuli. Because surround suppression and contextual biases in slant perception depend in similar ways on relative differences and stimulus reliability, surround suppression is a promising mechanistic explanation for contextual biases in slant perception. On the functional level, contextual biases in slant perception may be part of a mechanism of grouping and segmentation, which operates on surround suppression (Schwartz et al., 2009). An exciting hypothesis, which is largely unexplored. Research on how contextual biases in slant perception depend on surround suppression or grouping may reveal novel ways in which the visual system deals with uncertainty in 3D information. The visual system may not only integrate visual information to increase reliability, as in Bayesian inference, but may also actively ignore redundant information within perceptual groups.
Conflict of Interest Statement
The authors declare that the research was conducted in the absence of any commercial or financial relationships that could be construed as a potential conflict of interest.
Acknowledgments
This work was supported by grant 40004196 of the Netherlands Organisation for Scientific Research (NWO) to Susan F. Te Pas. We thank Odelia Schwartz and Frans Verstraten for insightful discussion on the manuscript.
References
Anstis, S. M., and Howard, I. P. (1978). A Craik-O’Brien-Cornsweet illusion for visual depth. Vision Res. 18, 213–217.
Baker, D. H., and Graf, E. W. (2010). Contextual effects in speed perception may occur at an early stage of processing. Vision Res. 50, 193–201.
Brookes, A., and Stevens, K. A. (1989). The analogy between stereo depth and brightness. Perception 18, 601–614.
Carandini, M., Heeger, D. J., and Movshon, A. (1997). Linearity and normalization in simple cells of macaque primary visual cortex. J. Neurosci. 17, 8621–8644.
Cavanaugh, J. R., Bair, W., and Movshon, A. (2002). Nature and interaction of signals from the receptive field center and surround in macaque v1 neurons. J. Neurophysiol. 88, 2530–2546.
Clifford, C., Wenderoth, P., and Spehar, B. (2000). A functional angle on some after-effects in cortical vision. Proc. Biol. Sci. 267, 1705–1710.
Curran, W., and Johnstone, A. (1996). Three-dimensional curvature contrast-geometric or brightness illusion? Vision Res. 34, 3641–3653.
Dumoulin, S. O., and Wandell, B. A. (2008). Population receptive field estimates in human visual cortex. Neuroimage 39, 647–660.
Ernst, M. O., and Banks, M. S. (2002). Humans integrate visual and haptic information in a statistically optimal fashion. Nature 415, 429–433.
Geisler, W. S., and Albrecht, D. G. (1992). Cortical neurons: isolation of contrast gain control. Vision Res. 32, 1409–1410.
Gilbert, C. D., and Wiesel, T. N. (1990). The influence of contextual stimuli on the orientation selectivity of cells in the primary visual cortex of the cat. Vision Res. 30, 1689–1701.
Gillam, B., Blackburn, S., and Brooks, K. (2007). Hinge versus twist: the effects of “reference surfaces” and discontinuities on stereoscopic slant perception. Perception 36, 596–616.
Gillam, B., Chambers, D., and Russo, T. (1988). Postfusional latency in stereoscopic slant perception and the primitives of stereopsis. J. Exp. Psychol. 14, 163–175.
Gillam, B., Flagg, T., and Finlay, D. (1984). Evidence for disparity change as the primary stimulus for stereoscopic processing. Percept. Psychophys. 36, 559–564.
Gillam, B., and Pianta, M. J. (2005). The effect of surface placement and surface overlap on stereo slant contrast enhancement. Vision Res. 45, 3083–3095.
Heeger, D. J. (1992). Normalization of cell responses in cat striate cortex. Vis. Neurosci. 9, 181–197.
Herzog, M. H., and Fahle, M. (2002). Effects of grouping in contextual modulation. Nature 415, 433–436.
Hillis, J. M., Watt, S. J., Landy, M. S., and Banks, M. S. (2004). Slant from texture and disparity cues: optimal cue combination. J. Vis. 4, 967–992.
Jin, D., Dragoi, V., Sur, M., and Seung, S. (2005). Tilt aftereffect and adaptation-induced changes in orientation tuning in visual cortex. J. Neurophysiol. 94, 2038–4050.
Knill, D. C., and Saunders, J. A. (2003). Do humans optimally integrate stereo and texture information for judgements of surface slant? Vision Res. 43, 2539–2558.
Landy, M. S., Maloney, L. T., Johnston, E. B., and Johnston, M. Y. (1995). Measurement and modeling of depth cue combination: in defense of weak fusion. Vision Res. 35, 389–412.
Lesica, N. A., Jianzhong, J., Weng, C., Yeh, C., Butts, D. A., Stanley, G. B., and Alonso, J. M. (2007). Adaptation to stimulus contrast and correlations during natural visual stimulation. Neuron 55, 479–491.
Mareschal, I., Morgan, M. J., and Solomon, J. A. (2010). Cortical distance determines whether flankers cause crowding or the tilt illusion. J. Vis. 10, 1–14.
McDonald, J. S., and Tadmor, Y. (2006). The perceived contrast of texture patches embedded in natural images. Vision Res. 46, 3098–3104.
Mitchison, G. J., and Westheimer, G. (1984). The perception of depth in simple figures. Vision Res. 24, 1063–1073.
Nakayama, K., and Loomis, J. M. (1974). Optical velocity patterns, velocity-sensitive neurons, and space perception: a hypothesis. Perception 3, 63–80.
Nguyenkim, J. D., and DeAngelis, G. C. (2003). Disparity-based coding of three-dimensional surface orientation by macaque middle temporal neurons. J. Neurosci. 23, 1717–1728.
Oruç, I., Maloney, L. T., and Landy, M. S. (2003). Weighted linear cue combination with possibly correlated error. Vision Res. 43, 2451–2468.
O’Toole, B., and Wenderoth, P. (1977). The tilt illusion: repulsion and attraction effects in the oblique meridian. Vision Res. 17, 367–374.
Paffen, C. L., Tadin, D., Te Pas, S. F., Blake, R., and Verstraten, F. A. (2006). Adaptive center-surround interactions in human vision during binocular rivalry. Vision Res. 46, 559–604.
Paffen, C. L., Te Pas, S. F., Kanai, R., van der Smagt, M. J., and Verstraten, F. A. (2004). Center-surround interactions in visual motion processing during binocular rivalry. Vision Res. 44, 1635–1639.
Polat, U., Mizobe, K., Pettet, M. W., Kasamatsu, T., and Norcia, A. M. (1998). Collinear stimuli regulate visual responses depending on cell’s contrast threshold. Nature 5, 580–584.
Poom, L., Olsson, H., and Borjesson, E. (2007). Dissociatons between slant-contrast and reversed slant-contrast. Vision Res. 47, 746–754.
Rogers, B. J., and Graham, M. E. (1982). Similarities between motion parallax and stereopsis in human depth perception. Vision Res. 22, 261–270.
Sato, M., and Howard, I. P. (2001). Effects of disparity-perspective cue conflict on depth contrast. Vision Res. 41, 415–426.
Sayim, B., Westheimer, G., and Herzog, M. H. (2010). Gestalt factors modulate basic spatial vision. Psychol. Sci. 21, 641–646.
Schumer, R., and Ganz, L. (1979). Independent stereoscopic channels for different extents of spatial pooling. Vision Res. 19, 1303–1314.
Schwartz, O., Hsu, A., and Dayan, P. (2007). Space and time in visual context. Nat. Rev. Neurosci. 8, 522–535.
Schwartz, O., Sejnowski, T., and Dayan, P. (2009). Perceptual organization in the tilt illusion. J. Vis. 9, 1–20.
Sengpiel, F., Sen, A., and Blakemore, C. (1997). Characteristics of surround inhibition in cat area 17. Exp. Brain Res. 116, 216–228.
Shipley, T., and Hyson, M. (1972). The stereoscopic sense of order-a classification of stereograms. Am. J. Optom. Arch. Am. Acad. Optom. 49, 83–96.
Simoncelli, E. P., and Heeger, D. J. (1998). A model of neural responses in visual area MT. Vision Res. 38, 743–761.
Solomon, J. A., Felisberti, F. M., and Morgan, M. J. (2004). Crowding and the tilt illusion: toward a unified account. J. Vis. 4, 500–508.
Stevens, K. A., and Brookes, A. (1988). Integrating stereopsis with monocular interpretations of planar surfaces. Vision Res. 28, 371–386.
Tadin, D., Lappin, J. S., and Blake, R. (2006). Fine temporal properties of center-surround interactions in motion revealed by reverse correlation. J. Neurosci. 26, 2614–2622.
Tajima, S., Watanabe, T., Imai, C., Ueno, K., Asamizuya, T., Sun, P., Tanaka, K., and Cheng, K. (2010). Opposing effects of contextual surround in human early visual cortex revealed by functional magnetic resonance imaging with continuously modulated visual stimuli. J. Neurosci. 30, 3264–3270.
Te Pas, S. F., Rogers, B. J., and Ledgeway, T. (2000). A curvature contrast effect for stereoscopically-defined surfaces. Curr. Psychol. Lett. 1, 117–126.
van der Kooij, K., and Te Pas, S. F. (2009a). Perception of 3D shape in context: contrast and assimilation. Vision Res. 49, 746–751.
van der Kooij, K., and Te Pas, S. F. (2009b). Uncertainty reveals surround modulation of shape. J. Vis. 9, 1–8.
van der Kooij, K., and Te Pas, S. F. (2010). Shape contrast: a global mechanism. Vision Res. 50, 2455–2459.
van Ee, R., Banks, M. S., and Backus, B. T. (1999). An analysis of binocular slant contrast. Perception 28, 1121–1145.
van Ee, R., and Erkelens, C. J. (1996). Anisotropy in Werner’s binocular depth-contrast effect. Vision Res. 36, 2253–2262.
Wenderoth, P., and Johnstone, S. (1988). The different mechanisms of the direct and indirect tilt illusions. Vision Res. 28, 301–312.
Westheimer, G. (1990). Simultaneous orientation contrast for lines in the human fovea. Vision Res. 30, 1913–1921.
Wilson, H. R., and Humanski, R. (1993). Spatial frequency adaptation and contrast gain control. Vision Res. 33, 1133–1149.
Xiao, D. K., Marcar, V. L., Raiguel, S. E., and Orban, G. A. (1997). Selectivity of macaque MT/V5 neurons for surface orientation in depth specified by motion. Eur. J. Neurosci. 9, 956–964.
Zipser, K., Lamme, V. A. F., and Schiller, P. H. (1996). Contextual modulation in primary visual cortex. J. Neurosci. 16, 7376–7389.
Keywords: 3D, slant contrast, long-range interaction, surround suppression, context
Citation: van der Kooij K and te Pas SF (2011) Perception of 3D slant out of the box. Front. Psychology 2:119. doi: 10.3389/fpsyg.2011.00119
Received: 23 November 2010; Accepted: 25 May 2011;
Published online: 06 June 2011.
Edited by:
Laurence T. Maloney, Stanford University, USAReviewed by:
Erich Graf, University of Southampton, UKSimon Barthelme, TU Berlin and Bernstein Center for Computational Neuroscience, Germany
Copyright: © 2011 van der Kooij and te Pas. This is an open-access article subject to a non-exclusive license between the authors and Frontiers Media SA, which permits use, distribution and reproduction in other forums, provided the original authors and source are credited and other Frontiers conditions are complied with.
*Correspondence: Katinka van der Kooij, Experimental Psychology Division, Helmholtz Institute, Universiteit Utrecht, Heidelberglaan 2, 3584 CS Utrecht, Netherlands. e-mail: katinkavanderkooij@gmail.com