- 1Department of Industrial Design, Design Academy, Sichuan Fine Arts Institute, Chongqing, China
- 2Department of Digital Media Art, Design Academy, Sichuan Fine Arts Institute, Chongqing, China
New types of artificial intelligence products are gradually transferring to voice interaction modes with the demand for intelligent products expanding from communication to recognizing users' emotions and instantaneous feedback. At present, affective acoustic models are constructed through deep learning and abstracted into a mathematical model, making computers learn from data and equipping them with prediction abilities. Although this method can result in accurate predictions, it has a limitation in that it lacks explanatory capability; there is an urgent need for an empirical study of the connection between acoustic features and psychology as the theoretical basis for the adjustment of model parameters. Accordingly, this study focuses on exploring the differences between seven major “acoustic features” and their physical characteristics during voice interaction with the recognition and expression of “gender” and “emotional states of the pleasure-arousal-dominance (PAD) model.” In this study, 31 females and 31 males aged between 21 and 60 were invited using the stratified random sampling method for the audio recording of different emotions. Subsequently, parameter values of acoustic features were extracted using Praat voice software. Finally, parameter values were analyzed using a Two-way ANOVA, mixed-design analysis in SPSS software. Results show that gender and emotional states of the PAD model vary among seven major acoustic features. Moreover, their difference values and rankings also vary. The research conclusions lay a theoretical foundation for AI emotional voice interaction and solve deep learning's current dilemma in emotional recognition and parameter optimization of the emotional synthesis model due to the lack of explanatory power.
Introduction
Nowadays, the core technologies of artificial intelligence (AI) are becoming increasingly mature. People face a new bottleneck in giving the “emotional temperature of humans” to a cold, intelligent device (Yonck, 2017). The conversational voice-user interface (VUI) is the most natural and instinctive interactive mode for humans. Recently, natural language processing (NLP) has improved significantly due to the development of deep learning (DL) technology. The VUI demands of the new type of intelligent products transform communication to include emotional listening and feedback of users (Hirschberg and Manning, 2015; Dale, 2016; Chkroun and Azaria, 2019; Harper, 2019; Nguyen et al., 2019; Guo et al., 2020; Hildebrand et al., 2020). Giving computers similar emotional mechanisms and emotional intelligence concepts as humans is becoming increasingly critical in the information and cognitive sciences. The goal of “affective computing” is to endow computers with abilities of understanding and generating affective characteristics. Finally, the computer can become intimate with the nature and makeup of vivid interactions, like people. This involves interdisciplinary study in the areas of psychology, sociology, information science, and physiology (Picard, 2003, 2010) and is becoming a hot spot of laboratory research in academic and industrial circles (Bänziger et al., 2015; Özseven, 2018). Although VUI has considerable potential, effective semantic and emotional communication not only requires the subtle understanding of the physics and psychology of voice signals but also needs a method of extracting and analyzing voice features from human voice data (Picard, 2003; Guo et al., 2020; Hildebrand et al., 2020).
Affective computing is crucial to implementing man–machine emotional interactions through intelligent products (Picard, 2010; Dale, 2016). In the past, many studies of emotional voice recognition and synthesis have been reported. Nevertheless, they mainly establish acoustic models and systems based on information science. Abundant voice data have been input into the DL core of AI and several affective factors of acoustic features summarized from the 3-D pleasure-arousal-dominance (PAD) emotional state model on a “continuous dimension.” A mathematical model was constructed and abstracted using mathematical knowledge and computer algorithms. Subsequently, the computer was able to learn from the data and make predictions by combining training data and its large-scale operation capability (Ribeiro et al., 2016; Rukavina et al., 2016; Kratzwald et al., 2018; Vempala and Russo, 2018; Badshah et al., 2019; Heracleous and Yoneyama, 2019; Guo et al., 2020). Although these practices can gain accurate prediction results quickly, they do not provide an understanding of where the results come from (e.g., black box) and lack explanatory ability (Kim et al., 2016; Ribeiro et al., 2016; Murdoch et al., 2019; Molnar, 2020). As a result, understanding how to adjust the model parameters is a problem that has yet to be solved, requiring an urgent empirical study of the connection between acoustic features and psychology as the theoretical basis for adjustment of model parameters (Ribeiro et al., 2016; Skerry-Ryan et al., 2018; Evans et al., 2019; Molnar, 2020). Research into voice rhythms from the cognitive psychology perspective has mainly focused on fundamental frequency, sound intensity, voice length, and other features (Juslin and Scherer, 2005). Emotional classifications are described quantitatively, which is different from the “continuous dimension” in existing intelligent systems. None of these studies yields 3-D coordinates through transformation to provide affection matching.
As a result of these shortcomings, an empirical study on the correlation between information enabling the emotional evaluation of acoustic features concerning emotional voice state and psychology is required in AI emotional voice interaction using a PAD model, which is the theoretical basis for adjustment of model parameters (Ribeiro et al., 2016; Skerry-Ryan et al., 2018; Evans et al., 2019; Molnar, 2020). Different average speech characteristics between males and females in human conversations have been reported in most studies (Childers and Wu, 1991; Feldstein et al., 1993). Furthermore, males and females show different emotional expressions. This study connected emotional states and voice features of male and female users through cross informatics and cognitive psychology from the voice interaction application scenes of intelligent products. Hence, this study focuses on the influences of “gender” and “emotions” on the “physical features of voices” in human–computer interactions as well as the quantitative expressions of the “physical features of voices.” The research conclusions lay a theoretical foundation for AI emotional voice interaction and solve DL's current dilemma in emotional recognition and parameter optimization of the emotional synthesis model due to lack of explanatory powers.
Literature Review
Studies on Emotions and Classification
According to research within psychology and the neurosciences, there is extensive interaction between the emotions and cognition of humans (Osuna et al., 2020), displaying behavioral and psychological features (Fiebig et al., 2020) that have a profound impact on the expression, tone, and posture behavior of people in daily life (Scherer, 2003; Ivanović et al., 2015; Poria et al., 2017). In the past 20 decades, studies on emotions have increased significantly (Wang et al., 2020). At present, there are two mainstream affective description modes. One is to make a qualitative description of an emotional classification using adjectives from the perspective of “discrete dimensions,” such as the six basic emotion categories proposed by Ekman and Oster (1979). The other is to describe the consequence determined by common affective factors of a “continuous dimension.” The emotional states can be characterized and divided by quantitative emotional coordinates on different dimensions (Sloman, 1999; Bitouk et al., 2010; Chauhan et al., 2011; Harmon-Jones et al., 2016; Badshah et al., 2019). Specifically, 1-D space focuses on positive or negative emotional classification, and 2-D spatial emotional states are generally expressed by two coordinates, such as peace–excitement and happiness–sadness. The 3-D space is proposed by Schlosberg (1954), Osgood (1966), Izard (1991), Wundt and Wozniak (1998), and Dai et al. (2015), respectively.
Quantitative measurement of emotions is a requirement of affective computing (Dai et al., 2015). Because three-dimensional space is easy to compute, computational models of emotion (CMEs) in the current AI system adopt the continuous dimension; the most used is the PAD model proposed by Mehrabian and Russell in 1994. The PAD model hypothesizes that users have three emotional states according to the situation stimulus, including pleasure, arousal, and dominance. These 3-D axes act as an emotional generation mechanism (Mehrabian and Russell, 1974; Wang et al., 2020). For example, emotions are divided into eight states with eight blocks of 3-D negative (–) and positive (+) combinations in the three dimensions as seen in Table 1 (Mehrabian, 1996b).
As a CME, PAD can distinguish different emotional states effectively (Russell, 1980; Gao et al., 2016) and break from the traditional tag-description method. As one of the relatively mature emotional models (Mehrabian and Russell, 1974; Mehrabian, 1996a; Gunes et al., 2011; Jia et al., 2011; Chen and Long, 2013; Gao et al., 2016; Osuna et al., 2020; Wang et al., 2020), the PAD model measures the mapping relationship between emotional states and typical emotions by “distance” to some extent, thus transforming the analytical studies of discrete emotional voices into quantitative studies of emotional voices (Mehrabian and Russell, 1974; Mehrabian, 1996a; Gunes et al., 2011; Jia et al., 2011; Chen and Long, 2013; Gao et al., 2016; Osuna et al., 2020; Wang et al., 2020). It has been extensively applied in information processing, emotional computing, and man–machine interaction (Dai et al., 2015; Weiguo and Hongman, 2019). PAD is beneficial for establishing an external stimulus emotional calculation model to realize emotional responses during personalized man–machine interaction (Weiguo and Hongman, 2019).
Affective Computing and Emotions in Voice Interaction
Voice signals are the most natural method of communication for people (Weninger et al., 2013). On the one hand, voice signals contain the verbal content to be transmitted. On the other hand, rhythms in the vocalizations contain rich emotional indicators (Murray and Arnott, 1993; Gao et al., 2016; Noroozi et al., 2018; Skerry-Ryan et al., 2018). Each emotional state has unique acoustic features (Scherer et al., 1991; Weninger et al., 2013; Liu et al., 2018). For example, various prosodic features, including different tones, velocity, and volume, can express the speaker's different emotional states (Apple et al., 1979; Trouvain and Barry, 2000; Chen et al., 2012; Yanushevskaya et al., 2013).
Huttar (1968) further demonstrates that prosodic features of voice play an important role in emotions and suggests simulating these features (e.g., tone, velocity, and volume) in the interface by using artificial voices to express the emotional states of the speaker (Sauter et al., 2010). Subsequently, Professor Picard proposed affective computing (Picard, 2000) and attempted to endow computers with a similar affective mechanism to intelligently understand human emotions in man–machine interactions and, thus, realize effective interactions between an artificial voice and users. It is necessary to gain a subtle understanding of voices using an interdisciplinary approach, including physics and psychology, to understand how to extract and analyze phonetic features (Schwark, 2015; Guo et al., 2020). In addition to the automatic speech recognition (ASR) and text-to-speech (TTS) found in artificial speech, the process involves the emotional analysis of users (Tucker and Jones, 1991; Guo et al., 2020; Hildebrand et al., 2020). In Figure 1, the relationship between artificial acoustic waves and emotional states and the role of artificial acoustic waves in the voice interaction systems of intelligent products are reviewed. Specifically, a user's current emotional state in the PAD model is identified through affective computing according to emotional acoustic features in voice interactions. The user receives responses in an empathic voice expression of the computer in the AI product.
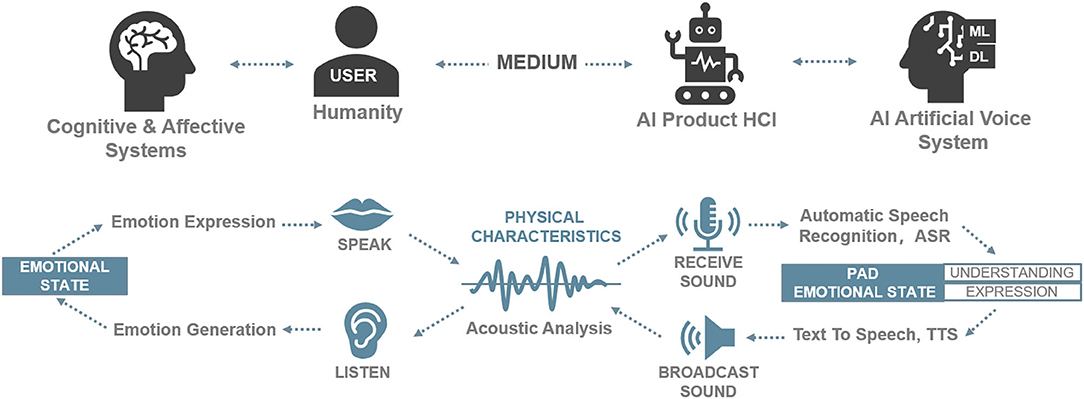
Figure 1. The relationship between artificial acoustic waves and emotional states in the voice interaction systems of intelligent products. Source: Drawn by the authors.
A Dimensional Framework of the Acoustic Features of Emotions
From a physiological perspective, loosening and contracting the vocal cords leads to rhythm changes in the voice, indicating emotions (Johar, 2016). From the perspective of psychology, relevant studies have proved that prosodic features of voices, such as basic frequency, velocity, and volume, are closely related to any emotional states (Williams and Stevens, 1972; Bachorowski, 1999; Kwon et al., 2003; Audibert et al., 2006; Hammerschmidt and Jürgens, 2007; Sauter et al., 2010; Quinto et al., 2013; Łtowski, 2014; Johar, 2016; Dasgupta, 2017; Hildebrand et al., 2020; Kamiloglu et al., 2020). Murray and Arnott (1993) introduce the concept of utterances and people's emotions, finding three major aspects that influence voice parameters of emotional impacts: utterance timing, utterance pitch contour, and voice quality. Among them, utterance timing and utterance pitch contour are prosodic features. In the past, most studies focused on prosodic features. Although these parameters gave certain differences in emotional distinction, some studies also find disadvantages for intelligent products in judging the emotions of the speaker, including voice quality (spectrum) (Toivanen et al., 2006). Jurafsky and Martin (2014). Experts in both linguistics and computers point out that each acoustic wave can be described completely by the four dimensions of time, frequency, amplitude, and spectrum. Connections between these four dimensions of acoustic waves and emotions in relevant studies are summarized in Table 2.
The first dimension is time, determined by the duration of a vibration from the sound maker (Sueur, 2018; Wayland, 2018) and measured in seconds or milliseconds of acoustic waves. Previous studies explore the influence of gender on velocity. Some studies demonstrate that the velocity of males is higher than females (Feldstein et al., 1993; Verhoeven et al., 2004; Jacewicz et al., 2010); however, most studies on people who speak English find no differences between males and females (Robb et al., 2004; Sturm and Seery, 2007; Nip and Green, 2013). Velocity can indicate the emotional state of the speaker, generally with a high velocity in positive and negative emotional states (e.g., anger, fear, and happiness), but a low velocity in low-wakefulness states (Juslin and Laukka, 2003).
The second dimension is frequency, expressed by the number of vibrations of the acoustic wave per second (unit: Hz). The scale of this objective physical quantity corresponds to the fundamental frequency (Fo) of the vocal cord vibrations. Pitch is a subjective psychological quantity of sound, its value determined by the frequency of the acoustic waves (unit: Mel) (Juslin and Laukka, 2003; Colton et al., 2006). Pitch can represent different emotional states. The pitch is increased when a person is feeling anger, happiness, or fear and decreased when a person is sad or bored (Murray and Arnott, 1993; Johar, 2016). With respect to gender, the Fo of a male adult's voice is often lower than a female adult's voice (Mullennix et al., 1995; Pernet and Belin, 2012).
The third dimension is amplitude, which determines the intensity of sound (unit: dB). Loudness is the scale of a subjective psychological index of intensity and results from a subjective judgment of a pure tone (unit: phon) (Sueur, 2018; Wayland, 2018). Generally speaking, the loudness of people is about 70 dB (Awan, 1993; Brown et al., 1993). Higher loudness is generally believed to relate to greater dominant traits or aggressiveness (Scherer and Giles, 1979; Abelin and Allwood, 2000; Asutay and Västfjäll, 2012; Yanushevskaya et al., 2013); relatively low loudness indicates people are fearful, sad, or gentle (Johar, 2016). Additionally, males' intensity of sound is slightly higher than that of females (Awan, 1993; Brockmann et al., 2011).
The fourth dimension is spectrum, referring to the energy distribution of signals (e.g., voice) in the frequency domain; it is expressed in graphs by analyzing perturbations of acoustic waves or periodic features (Sueur, 2018). The degree of “sound instability” during the formation of voices has been summarized (Hildebrand et al., 2020), reflecting voice quality (Kamiloglu et al., 2020). Vocal jitter is a measure of the periodic variation in fundamental frequency, indicating uneven tones of the speaker. A nervous speaker has instability in the voice (high perturbations) and a quiet speaker has a steady and stable sound (low perturbation) (Farrús et al., 2007; Kamiloglu et al., 2020). Specifically, jitter percentage expresses each basic frequency period's irregularity, that is, the degree of frequency perturbation. It is the ratio between the fluctuations of the fundamental frequency and mean values. A high numerical value indicates that the tone quality is unstable. Shimmer percentage refers to differences in repeated amplitude changes, that is, the degree of amplitude perturbation. It describes the ratio of the mean amplitude variation and respective mean. A high numerical value of shimmer percentage indicates greater changes in sound volume. HNR reflects the ratio of periodic segments and noises in signals (unit: dB). Lower noise energy in voices reflects fewer components of noises and better sound quality (Baken and Orlikoff, 2000; Ferrand, 2007). Some studies have proved that gender has no significant influences on jitter percentage, shimmer percentage, or HNR (Wang and Huang, 2004; Awan, 2006; Brockmann et al., 2008; Ting et al., 2011).
Research Directions on Connections of Acoustic Features and Emotional States
Studies on the emotional rhythm of voice have pointed out that people's sounds, characterized by pitch, loudness or intensity, and velocity, transfer different emotional information to listeners (Sauter et al., 2010). During a conversation, emotions can be recognized from video clips as short as 60 ms (Pollack et al., 1960; Pell and Kotz, 2011; Schaerlaeken and Grandjean, 2018). The same words and phrases can be expressed differently through fluctuation of different emotional states (Dasgupta, 2017); for example, rumination is related to low velocity and an extended dwell time. Anger is generally related to the loudness of voice (Juslin and Laukka, 2003; Clark, 2005). Fear is related to variations in pitch (Juslin and Laukka, 2003; Clark, 2005). The affective computing team from MIT analyzed variations in acoustic parameters, such as fundamental frequency and duration, during different emotional states; their results show that acoustic features of affective sounds (e.g., happy, surprise, and anger) are similar with the sad acoustic feature being relatively obvious (Sloman, 1999). In brief, the formation of human spoken language involves the interaction of individual traits and emotional states, used as a communication means to understand voices. To recognize and extract information for voice analysis, it is necessary to measure voice quality properties (Johar, 2016; Schaerlaeken and Grandjean, 2018).
To effectively establish an emotional identification and expression system, emotional identification and synthesis based on DL have considerable potential in human–machine interactions (Schuller and Schuller, 2021). Recognizing emotions through the automatic extraction of acoustic features and generating expressions through emotions are the main strategies for relevant research development. It has been proven that a generative adversarial network (GAN) can improve the machine's performance in emotional analysis tasks (Han et al., 2019). Additionally, people begin to think about transfer learning applications in relevant tasks and voice emotional computing modes (Schuller and Schuller, 2021).
Based on the above literature review, research can primarily presently be divided into two types. On the one hand, some studies based on information science strive to gain accurate emotional identification and natural voice expressions through DL. However, these studies lack the explanation for establishing a mathematical model (Ribeiro et al., 2016; Murdoch et al., 2019), thus resulting in the absence of a theoretical foundation for parameter optimization and adjustment. On the other hand, some studies are based on cognitive science and emotional states from the “discrete dimension.” Most of these studies use prosodic features only and have shortages in emotional identification and expression (Toivanen et al., 2006). Studies rarely use the PAD model's emotional states in the intelligent product VUI as the framework for incorporating acoustic features of the spectrum and gender impacts. Hence, interdisciplinary studies are needed to solve the black box problems caused by DL.
Methods
This study aims to connect humans' emotions and acoustic features from across information, acoustics, and psychology disciplines based on acoustic and cognitive psychology concepts.
Research Design
Both the purpose of this study and the literature review results have directed the current research to investigate the correlation of two independent variables, namely “gender” and “emotional state.” The emotional state, different from other emotional classification models, considers each emotion has sole coordinates in the PAD space, enabling different emotions to show acoustic features independently. Therefore, the PAD model uses the eight basic emotions for emotional classification and neutral emotions as the benchmark. The dependent variables are seven main features associated with emotional states in the four dimensions of emotional voice sound waves.
Subjects and Materials
A total of 31 male and 31 female respondents were recruited by the stratified random sampling mode. Respondents have clear cognition with the nine basic emotions of PAD and display explicit oral expression. This study focuses on vocalizations from voice signals, and verbalizations are not transmitted; therefore, the recording of voice data used neutral words and verbalizations transmitted by “” (Chinese). Because it is easy to induce and simulate emotional recordings that can express real and natural emotions to some extent, PPT was used to provide films as the emotional stimuli to induce and guide recording of the participant (Figure 2). The provided film was confirmed by three relevant experts and then predicted and modified to assure effective induction and prompts.
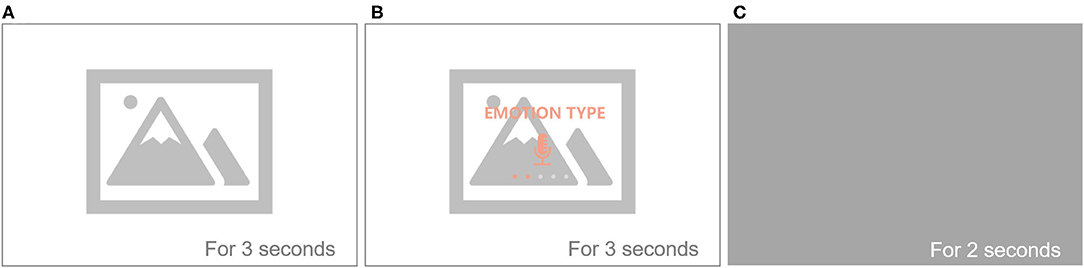
Figure 2. Emotional induction and guidance cases during voice recording of different emotions. (A) Emotional stimulus is induced. (B) Text to remind the emotion, and then record. Subsequently, (C) Interval shady, and then enter the next emotional stimulus to induce. The complete contents are shown in the Appendix: Supplementary Material.
Setting and Program of Experiments
Setup of experiments for data acquisition: An empirical study using laboratory experiments was carried out. All respondents engaged in the experiments, and voices were recorded in the same environment using the same settings. The input sound volume was fixed at 70 dB SP. The recording formula was mono channel; sampling frequency: 44.1 kHz; and resolution: 16 bits and WAV file. The relevant program is shown in Figures 3, 4.
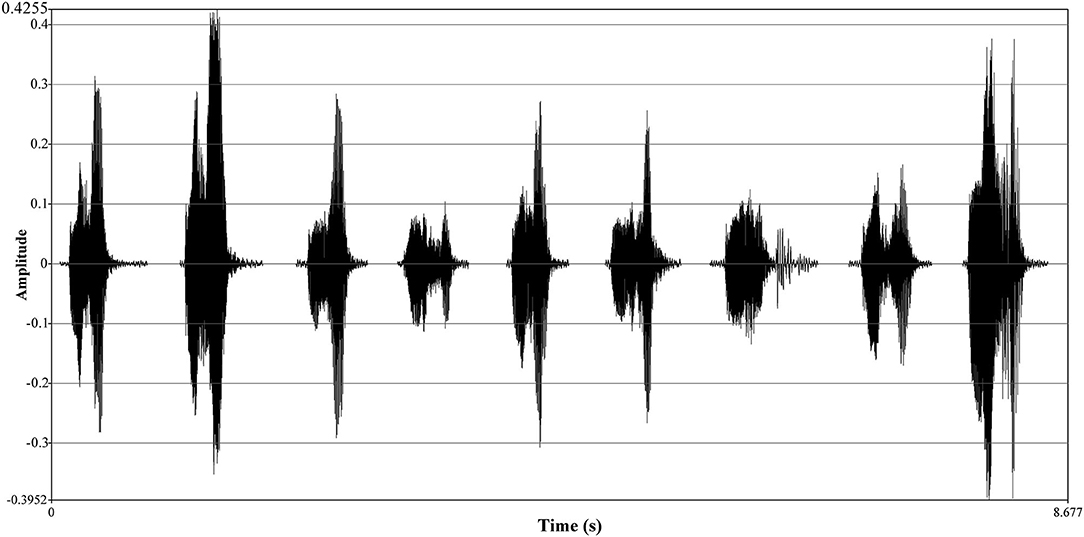
Figure 4. Oscillograph comparison of different emotional voices of respondents. The Y-axis of the oscillograph expresses time (unit: s). The X-axis, amplitude, has different units of expressions, either decibel (dB) or relative values; it ranges between [−1, 1] and can be expressed by a percentage or frequency value (Sueur, 2018; Wayland, 2018). From the left to the right, a respondent records nine emotions of “” from ID.1 to ID.9.
The audio recording process: First, selected respondents, in the closed experimental space without disturbance, were introduced to the experimental process and audition by the same prompts. Second, respondents wore a headset microphone in a closed space, and a provided laptop played the stimulus and prompted the film using Adobe Audition 2019. Respondents provided data of nine emotions: neutral, exuberant, bored, dependent, disdainful, relaxed, anxious, docile, and hostile. The content of the audio recordings from each respondent was then confirmed, and residual contents were preprocessed, including polishing and numbering. Finally, acoustic features were analyzed using the Praat 6.13 voice software (Figure 5).
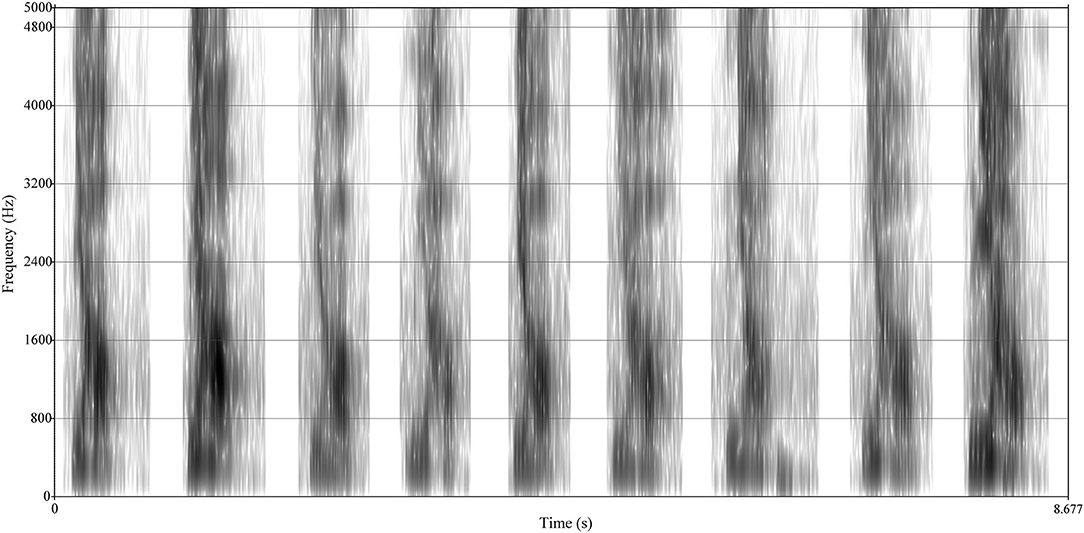
Figure 5. Comparison of spectrographs of respondents among different emotions. The Y-axis of the spectrograph is the same as the waveform and expresses the amplitude. The X-axis represents frequency (unit: Hz). The frequency spectrum is the variation of voice energy with frequency. In addition, different amplitudes (or loudness) were expressed by the color gradient of data points.
Analysis of the spectrum was done using the calculation formulas of jitter percentage, shimmer percentage, and HNR as outlined below (Boersma, 1993; Fernandes et al., 2018; Sueur, 2018). Nine emotional voices were selected and analyzed by Praat, and characteristic parameter data of seven emotional voices were directly extracted.
In phonetics, jitter reflects the fast repeated changes of the fundamental frequency, and it primarily describes the variation amplitude of any fundamental frequency. As shown below,
Ti is the duration of the pitch period i (unit: ms), and N is the quantity of all pitch periods. Jitterabsolute calculates the absolute mean of differences between any two adjacent pitch periods. The mean period is calculated using
The jitter percentage is calculated using
The jitterabsolute is divided by the meanPeriod, deriving the ratio between perturbation of fundamental frequency and mean during the pronunciation.
Calculation of Shimmer Percentage
Shimmer percentage reflects changes of amplitude among different periods and is calculated using
The mean of amplitude changes between two adjacent periods is calculated from shimmerabsolute. The Shimmer% is the ratio between the mean variation of amplitudes and the average value.
Calculation of HNR
HNR refers to the ratio of the periodic and noise parts in speech signals, and it primarily reflects the hoarse degree of voices. The calculation used to determine HNR is explained below.
The autocorrelation function (r(x)) of the voice delay signal x is defined as
where s(t) is the stable time signal, and the function achieves the global maximum when x = 0. If the function has global maximum points at other moments in addition to x = 0, a period of T0 is assumed. For any positive integer (n), then
If no other global maximum points in addition to x = 0 are detected, then other local maximum points may exist, where
s(t) is defined as the periodic signal with a period of T0, and N(t) is a noise signal. At x = 0, the voice signal is r(0) = TH(0) + TN(0). As r(0) = rH(0) + rN(0), the following equations can be applied:
describes the size of the relative energy of periodic parts in the voice signals and its complementary set describes the size of the relative energy of noises in the voice signal. HNR can be further defined as
The function has a global maximum when τ = 0, where x(t) is a steady time signal and a global maximum when τ = 0.
Results
The extracted seven-feature data of different emotions of different genders were analyzed using SPSS V.26 to conduct a two-way ANOVA, mixed design. Gender was used as the independent variable, and emotional state was used as the dependent variable to understand the variation in seven acoustic features of different genders under different emotions.
General Conditions of Respondents
A total of 62 respondents, including 31 males and 31 females, were recruited. These participants can be grouped according to age: 21–30 years old: nine females and eight males; 31–40 years old: eight females and eight males; 41–50 years old: eight females and eight males; and 51–60 years old: six females and seven males.
Difference Test Analysis of the Acoustic Parameters
To show significant differences in acoustic features under different emotions and gender, the same respondents were repeatedly measured, testing the seven acoustic features of emotions. Results of the correlation analyses are shown below.
Velocity: relevant data of seconds per word are listed in Tables 3, 4.
The interaction tests for gender and emotional state (SS = 0.01; Df = 2.53; MS = 0.00; F = 0.25; P > 0.05) did not yield any significant results, i.e., participants' velocity in expressing the nine different emotions was not significantly correlated to gender.
Gender main effect: The influence of velocity on overall emotional states varies significantly between males and females (F = 2587.76, p < 0.05). The velocity (M = 0.33) of female respondents under different emotional states is significantly lower than that of males (M = 0.29).
State main effect: Velocity under different emotional states varies significantly for the overall factor, gender (F = 76.37, p < 0.05). According to the multiple comparison, the state anxious (M = 0.23) shows the highest velocity, followed by exuberant and hostile (M = 0.26), disdainful (M = 0.27), neutral (M = 0.28), docile (M = 0.31), relaxed (M = 0.32), dependent (M = 0.37), and bored (M = 0.5), successively.
Fo (Hz): The interaction test showed significant results for both gender and emotional state (SS = 72887.47; Df = 1.85; MS = 39437.31; F = 15.90; p < 0.05; ω2 = 0.21), i.e., participants' Fo (Hz) varied across gender and emotional state. Relevant data abstracts of mean pitch are listed in Table 5.
Gender simple main effect: Females show significantly different effects of Fo on emotional states (F = 111.30, p < 0.05), according to the results of post hoc comparisons: (1) > (4); (2) > (1)–(6), (9); (3) > (5); (4) > (5); (6) > (1), (3) (5); (7) > (1), (3)–(6), (9); (8)> (1), (3)–(6), (9); (9) > (1), (3)–(5). Males (F = 103.96, p < 0.05) also show differences, according to results of post hoc comparisons: (1) > (4); (2) > (1)–(6), (8), (9); (3) > (4); (5) > (1), (3), (4); (6) > (1), (3)–(5), (8)–(9); (7) > (1), (3)–(9); (8) > (1). (3)–(5), (9); (9) > (1). (3)–(5). These results demonstrate that ranks of emotional states are different between males and females.
State simple main effect: With respect to influences of Fo (Hz) on gender under different emotional states, F-values of neutral, exuberant, bored, dependent, relaxed, disdainful, anxious, docile, and hostile states are 198.83, 113.02, 147.32, 324.47, 49.67, 51.28, 43.98, 66.71, and 207.12, respectively (p < 0.05). According to the results of post hoc comparisons, females have a significantly higher Fo than males.
Fo SD: The interaction test was significant across gender and emotional state (SS = 13144.75; Df = 3.67; MS = 3586.29; F = 10.80; p < 0.05; ω2 = 0.15), i.e., participants' Fo SD varied across gender and emotional state. Relevant data abstracts of pitch variability are listed in Table 6.
Gender simple main effect: Females show significantly different effects of Fo SD on emotional states (F = 2.43, p > 0.05), according to the results of post hoc comparisons: (1) > (4)–(6); (2) > (1), (4)–(8); (3) > (1), (4)–(8); (6) > (4); (7) > (1), (5); (8) > (4)–(6); (9) > (1), (4)–(8). Males (F = 2.43, p > 0.05) show no significant differences.
State simple main effect: Concerning influences of Fo SD on gender under different emotional states, F values of exuberant, bored, dependent, and hostile states are 47.88, 92.90, and 9.52, respectively (p < 0.05). According to the results of post hoc comparisons, females give significantly higher values than males; however, males > females with respect to the dependent variable.
Intensity (dB): The interaction test was significant across gender and emotional state (SS = 7624.57; Df = 1.99; MS = 314.08; F = 9.25; p < 0.05; ω2 = 0.13), i.e., participants' intensity varied across gender and emotional state. Relevant data abstracts of mean-sones intensity are listed in Table 7.
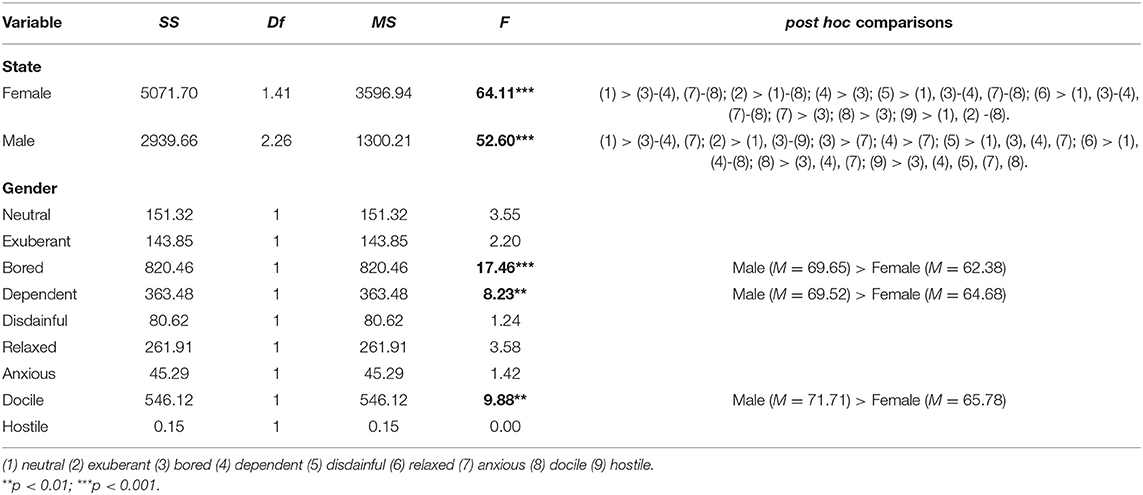
Table 7. Simple main effect test using mixed design of gender and emotional states on intensity (dB).
Gender simple main effect: Both males and females show significantly different effects of intensity (dB) on emotional states: Females (F = 64.11, p < 0.05) and males (F = 52.60, p < 0.05). According to post hoc comparisons, results of females are (1) > (3)–(4), (7)–(8); (2) > (1)–(8); (4) > (3); (5) > (1), (3)–(4), (7)–(8); (6) > (1), (3)–(4), (7)–(8); (7) > (3); (8) > (3); (9) > (1), (2)–(8). Results of males are (1) > (3)–(4), (7); (2) > (1), (3)–(9); (3) > (7); (4) > (7); (5) > (1), (3), (4), (7); (6) > (1), (4)-(8); (8) > (3), (4), (7); (9) > (3), (4), (5), (7), (8). The results demonstrate that ranks of emotional states are different between males and females.
State simple main effect: Concerning influences of intensity (dB) on gender under different emotional states, F-values of bored, dependent, and docile are 17.46, 8.23 and 9.88, respectively (p < 0.05). According to the results of post hoc comparisons, males give significantly higher results than females.
Jitter%: The interaction test resulted in significant outcomes considering gender and emotional state (S = 230.33; Df = 2.60; MS = 88.67; F =32.05; p < 0.05; ω2 = 0.35), i.e., participants' Jitter% varied across gender and emotional state. Relevant data abstracts of the ratio between the fundamental frequency changes and the mean are listed in Table 8.
Gender simple main effect: With respect to Jitter% of males and females under different emotional states, females (F = 25.87, p < 0.05) and males (F = 37.01, p < 0.05) both have significant effects. According to post hoc comparisons, females show (1) > (2), (8); (3) > (2), (8); (4) > (2), (8); (5) > (2), (8)-(9); (6) > (1)–(5), (7)–(9); (7) > (1)–(5), (8)–(9). Males show (1) > (2)–(6), (9); (2) > (5)–(6), (9); (4) > (3)–(6), (9); (7) > (1)–(6), (8)–(9); (8) > (2)–(6), (9). These results demonstrate that ranks of emotional states are different between males and females.
State simple main effect: Concerning influences of Jitter% on gender under different emotional states, F-values of neutral, exuberant, bored, dependent, relaxed, anxious, and docile are 82.90, 63.04, 8.11, 14.52, 23.77, 35.51, and 65.22, respectively (p < 0.05). According to the results of post hoc comparisons, females > males for relaxed and males > females for the remaining six emotional states.
Shimmer%: The interaction test yielded significant results considering gender and emotional state (S = 1712.65; Df = 4.29; MS = 399.46; F = 49.4; p < 0.05; ω2 = 0.45), i.e., participants' Shimmer % varied across gender and emotional state. Relevant data abstracts of intensity perturbations are listed in Table 9.
Gender simple main effect: With respect to Shimmer% of males and females under different emotional states, females (F = 240.70, p < 0.05) and males (F = 241.26, p < 0.05) both have significant effects. According to post hoc comparisons, females show (1) > (2), (4), (8)–(9); (2) > (8)-(9); (3) > (2), (4), (8)-(9); (4) > (8)–(9); (5) > (1)–(4), (8)–(9); (6) > (1)–(4), (8)–(9); (7) > (1)–(4), (8)–(9); (8) > (9). Males show (1) > (2)–(9); (2) > (8)–(9); (3) > (2), (8)–(9); (4) > (2)–(3), (6), (8)–(9); (5) > (2), (6), (8)–(9); (6) > (8)–(9); (7) > (2)–(9); (8) > (9). These results demonstrate that ranks of emotional states are different between males and females.
State simple main effect: Concerning influences of Shimmer% on gender under different emotional states, F-values of neutral, exuberant, bored, dependent, disdainful, relaxed, anxious, docile, and hostile are 82.90, 63.04, 8.11, 14.52, 19.99, 23.77, 35.51, 65.22, and 7.58, respectively (p < 0.05). According to post hoc comparison results, females are significantly higher than males concerning disdainful and relaxed, which is the opposite of the remaining emotional states.
HNR: The interaction test yielded significant results considering gender and emotional state (SS = 1071.63; Df = 3.76; MS = 284.69; F = 37.42; p < 0.05; ω2 = 0.38), i.e., participants' HNR varied across gender and emotional state. Relative data abstracts of the ratio of periodic part and noise in signals are listed in Table 10.
Gender simple main effect: With respect to HNR of males and females under different emotional states, females (F = 45.87, p < 0.05) and males (F = 30.90, p < 0.05) both show a significant effect. According to post hoc comparisons, females show (1) > (3), (5)–(7); (2) > (1), (3)–(7); (3) > (6)–(7); (4) > (3), (5)–(7); (5) > (6)–(7); (8) > (1)–(7); (9) > (1), (3)–(7). Males show (2) > (1), (7); (3) > (1)–(2), (4)–(5), (7)–(9); (4) > (1); (5) > (1), (7); (6) > (1)–(2), (4), (7)–(9); (8) > (1)–(2), (7); (9) > (1)–(2), (7). These results demonstrate that ranks of emotional states are different between males and females.
State simple main effect: With respect to influences of HNR on gender under different emotional states, F-values of neutral, exuberant, bored, dependent, relaxed, docile, and hostile are 62.35, 40.59, 8.50, 18.12, 49.74, 22.60, and 15.43, respectively (p < 0.05). According to the results of post hoc comparisons, males give significantly higher values than females in terms of bored and relaxed although the opposite phenomenon is observed for the remaining five emotional states.
Discussion And Conclusions
This study focuses on physical quantities of acoustic features and their differences according to gender and the emotional states of the PAD model during emotion–voice interactions of AI. The study found significant differences in users' gender and emotional states of the PAD model with respect to seven major acoustic features: (1) With respect to gender and emotional states, Fo (Hz), Fo SD, intensity (dB), Jitter%, Shimmer%, and HNR have interactions, and velocity displays no interaction. (2) There are significant gender differences in terms of velocity of eight emotional states in PAD. Moreover, males show significantly higher velocity (M = 0.29) compared to females (M = 0.33). (3) Males show no significant differences in six of the acoustic features, except Fo SD. Looking at the gender simple main effect, there are significant gender differences in terms of degree and ranking of emotional states. Looking at the state simple main effect, Fo (Hz) shows significant differences among different emotional states. Fo SD is significantly different in terms of exuberant, bored, dependent, and hostile states. Intensity (dB) is significantly different with respect to bored, dependent, and docile states. There are significant differences in Jitter% in neutral, exuberant, bored, dependent, relaxed, anxious, and docile states. Shimmer% has significant differences. HNR presents significant differences in neutral, exuberant, bored, dependent, relaxed, docile, and hostile states. The above analyses found physical quantities of relevant parameters and rankings as shown in the results. Specifically, the voice-affective interaction of intelligent products was used as the preset scene. Therefore, the PAD model is different in terms of emotional classification from the emotional classification found in the literature review (Williams and Stevens, 1972; Johnstone and Scherer, 1999; Abelin and Allwood, 2000; Quinto et al., 2013; Bowman and Yamauchi, 2016; Dasgupta, 2017; Hildebrand et al., 2020). Moreover, some acoustic features are different, and it is impossible to compare directly. Directionality of classification is compared with research results, which has not been investigated in past empirical studies; however, there are significant differences in rhythms of different emotions. For gender, previous studies mainly found that men speak more quickly than women (Feldstein et al., 1993; Verhoeven et al., 2004; Jacewicz et al., 2010), but it has also been found that there is no significant difference between men and women (Robb et al., 2004; Sturm and Seery, 2007; Nip and Green, 2013). This study further compared expressions of emotional states and concluded that men speak more quickly than women.
We comprehensively explored the influence of eight emotional states of the PAD model and gender on affective recognition and expression of acoustic features (e.g., velocity, Fo, frequency spectra) in a systematic method. In terms of theoretical implications, the PAD model of intelligent products provides an emotional model that is different from previously used models. In emotional computing, the PAD model is conducive to understanding the influences of gender and emotional states on the connection between acoustic features and psychology in AI affective-voice interaction, including physical variables and their differences. This aids in understanding the acoustic features of affective recognition and expression. In terms of practical applications, in view of the development trends of intelligent products on the market, man–machine interaction will be popularized in intelligent-home life, travel, leisure, entertainment, education, and medicine in the future. This study will help to improve the affective-voice interaction scenes of intelligent products and connections between the emotional states and acoustic features of the speaker. The analysis of acoustic features under different emotions and genders provides an empirical foundation for adjusting the parameters of the affective-voice interaction mathematical models and offsets limitations of current deep learning acoustic models' “explanatory” power. The research results can provide a reference for the adjustment of model parameters during optimization of affective recognition and affective expression.
This study was designed for theoretical and practical application; however, the recorded voices only used Chinese materials. There may be some differences with different languages, which deserves particular attention for generalization of the results. Subsequent studies can further investigate correlations between emotional classification of PAD and voice rhythm of different genders in the PAD model to provide a theoretical basis and supplement shortages of deep learning. This study aims to strengthen emotional integration during man–machine interaction, allowing users and products to generate the empathy effect and, thus, expand the human–computer relationship and highlighting the value of products.
Data Availability Statement
The original contributions presented in the study are included in the article/Supplementary Material, further inquiries can be directed to the corresponding author/s.
Ethics Statement
Ethical review and approval was not required for the study on human participants in accordance with the local legislation and institutional requirements. Written informed consent for participation was not required for this study in accordance with the national legislation and the institutional requirements.
Author Contributions
K-LH: conceptualization and writing. K-LH and S-FD: methodology and formal analysis. K-LH and XL: investigation. S-FD and XL: resources. K-LH: organized the database and analyzed and interpreted the data.
Funding
This study was supported by the Science and Technology Research Program of Chongqing Municipal Education Commission (Grant No. KJZD-K201901001) and (Grant No. KJZD-M201801001).
Conflict of Interest
The authors declare that the research was conducted in the absence of any commercial or financial relationships that could be construed as a potential conflict of interest.
Supplementary Material
The Supplementary Material for this article can be found online at: https://www.frontiersin.org/articles/10.3389/fpsyg.2021.664925/full#supplementary-material
References
Abelin, A., and Allwood, J. (2000). “Cross linguistic interpretation of emotional prosody,” in ISCA Tutorial and Research Workshop (ITRW) on Speech and Emotion (Newcastle), 110–113.
Apple, W., Streeter, L. A., and Krauss, R. M. (1979). Effects of pitch and speech rate on personal attributions. J. Personal. Soc. Psychol. 37, 715–727. doi: 10.1037/0022-3514.37.5.715
Asutay, E., and Västfjäll, D. (2012). Perception of loudness is influenced by emotion. PLoS ONE 7:e38660. doi: 10.1371/journal.pone.0038660
Audibert, N., Vincent, D., Aubergé, V., and Rosec, O. (2006). “Expressive speech synthesis: evaluation of a voice quality centered coder on the different acoustic dimensions,” in Proc. Speech Prosody: Citeseer, 525–528.
Awan, S. N. (1993). Superimposition of speaking voice characteristics and phonetograms in untrained and trained vocal groups. J. Voice 7, 30–37. doi: 10.1016/S0892-1997(05)80109-2
Awan, S. N. (2006). The aging female voice: acoustic and respiratory data. Clin. Linguist. Phone. 20, 171–180. doi: 10.1080/02699200400026918
Bachorowski, J.-A. (1999). Vocal expression and perception of emotion. Curr. Dir. Psychol. Sci. 8, 53–57.
Badshah, A. M., Ahmad, J., Rahim, N., and Baik, S. W. (2019). “Speech emotion recognition from spectrograms with deep convolutional neural network,” in 2017 International Conference on Platform Technology and Service (PlatCon), 1–5. doi: 10.1109/PlatCon.2017.7883728
Baken, R. J., and Orlikoff, R. F. (2000). Clinical Measurement of Speech and Voice. San Diego, CA: Singular Thomson Learning.
Bänziger, T., Hosoya, G., and Scherer, K. R. (2015). Path models of vocal emotion communication. PLoS ONE 10:e0136675. doi: 10.1371/journal.pone.0136675
Bänziger, T., and Scherer, K. R. (2005). The role of intonation in emotional expressions. Speech Commun. 46, 252–267. doi: 10.1016/j.specom.2005.02.016
Bitouk, D., Verma, R., and Nenkova, A. (2010). Class-level spectral features for emotion recognition. Speech Commun. 52, 613–625. doi: 10.1016/j.specom.2010.02.010
Boersma, P. (1993). “Accurate short-term analysis of the fundamental frequency and the harmonics-to-noise ratio of a sampled sound,” in Proceedings of the Institute of Phonetic Sciences (Citeseer), 97–110.
Bowman, C., and Yamauchi, T. (2016). Perceiving categorical emotion in sound: the role of timbre. Psychomusicol. Music Mind Brain 26, 15–25. doi: 10.1037/pmu0000105
Brenner, M., Doherty, E. T., and Shipp, T. (1994). Speech measures indicating workload demand. Aviat. Space Environ. Med. 65, 21–26.
Brockmann, M., Drinnan, M. J., Storck, C., and Carding, P. N. (2011). Reliable Jitter and Shimmer measurements in voice clinics: the relevance of vowel, gender, vocal intensity, and fundamental frequency effects in a typical clinical task. J. Voice 25, 44–53. doi: 10.1016/j.jvoice.2009.07.002
Brockmann, M., Storck, C., Carding, P. N., and Drinnan, M. J. (2008). Voice loudness and gender effects on jitter and shimmer in healthy adults. J. Speech Lang. Hear. Res. 51, 1152–1160. doi: 10.1044/1092-4388(2008/06-0208)
Brown, W. S., Morris, R. J., Hicks, D. M., and Howell, E. (1993). Phonational profiles of female professional singers and nonsingers. J. Voice 7, 219–226. doi: 10.1016/S0892-1997(05)80330-3
Burgoon, J. K., Birk, T., and Pfau, M. (1990). Nonverbal behaviors, persuasion, and credibility. Hum. Commun. Res. 17, 140–169. doi: 10.1111/j.1468-2958.1990.tb00229.x
Chauhan, R., Yadav, J., Koolagudi, S. G., and Rao, K. S. (2011). “Text independent emotion recognition using spectral features,” in International Conference on Contemporary Computing, ed A.S.et al. (Springer). doi: 10.1007/978-3-642-22606-9_37
Chen, X., Yang, J., Gan, S., and Yang, Y. (2012). The contribution of sound intensity in vocal emotion perception: behavioral and electrophysiological evidence. PLoS ONE 7:e30278. doi: 10.1371/journal.pone.0030278
Chen, Y., and Long, R. (2013). Trainable emotional speech synthesis based on PAD. Pattern Recogn. Artif. Intell. 26, 1019–1025.
Childers, D. G., and Wu, K. (1991). Gender recognition from speech. Part II: Fine analysis. J. Acoust. Soc. Am. 90, 1841–1856. doi: 10.1121/1.401664
Chkroun, M., and Azaria, A. (2019). LIA: a virtual assistant that can be taught new commands by speech. Int. J. Hum. Comp. Interact. 35, 1596–1607. doi: 10.1080/10447318.2018.1557972
Colton, R. H., Casper, J. K., and Leonard, R. (2006). Understanding Voice Problems: A Physiological Perspective for Diagnosis and Treatment. Philadelphia, PA: Lippincott Williams & Wilkins.
Dai, W., Han, D., Dai, Y., and Xu, D. (2015). Emotion recognition and affective computing on vocal social media. Inf. Manag. 52, 777–788. doi: 10.1016/j.im.2015.02.003
Dale, R. (2016). The return of the chatbots. Nat. Lang. Eng. 22, 811–817. doi: 10.1017/S1351324916000243
Dasgupta, P. B. (2017). Detection and analysis of human emotions through voice and speech pattern processing. Int. J. Comput. Trends Technol. 52, 1–3. doi: 10.14445/22312803/IJCTT-V52P101
Ekman, P., and Oster, H. (1979). Facial expressions of emotion. Ann. Rev. Psychol. 30, 527–554. doi: 10.1146/annurev.ps.30.020179.002523
Evans, B. P., Xue, B., and Zhang, M. (2019). “What's inside the black-box? a genetic programming method for interpreting complex machine learning models,” in Proceedings of the Genetic and Evolutionary Computation Conference (Prague: Association for Computing Machinery).
Farrús, M., Hernando, J., and Ejarque, P. (2007). “Jitter and shimmer measurements for speaker recognition,” in Eighth Annual Conference of the International Speech Communication Association), 778–781.
Feldstein, S., Dohm, F.-A., and Crown, C. L. (1993). Gender as a mediator in the perception of speech rate. Bull. Psychon. Soc. 31, 521–524.
Fernandes, J., Teixeira, F., Guedes, V., Junior, A., and Teixeira, J. P. (2018). Harmonic to noise ratio measurement - selection of window and length. Proc. Comput. Sci. 138, 280–285. doi: 10.1016/j.procs.2018.10.040
Ferrand, C. T. (2007). Speech Science: An Integrated Approach to Theory and Clinical Practice. Boston, MA: Pearson/Allyn and Bacon.
Fiebig, A., Jordan, P., and Moshona, C. C. (2020). Assessments of acoustic environments by emotions–the application of emotion theory in soundscape. Front. Psychol. 11:3261. doi: 10.3389/fpsyg.2020.573041
Gao, F., Sun, X., Wang, K., and Ren, F. (2016). “Chinese micro-blog sentiment analysis based on semantic features and PAD model,” in 2016 IEEE/ACIS 15th International Conference on Computer and Information Science (ICIS) (Okayama), 1–5. doi: 10.1109/ICIS.2016.7550903
Gunes, H., Schuller, B., Pantic, M., and Cowie, R. (2011). “Emotion representation, analysis and synthesis in continuous space: a survey,” in 2011 IEEE International Conference on Automatic Face and Gesture Recognition (FG) (Santa Barbara, CA,), 827–834. doi: 10.1109/FG.2011.5771357
Guo, F., Li, F., Lv, W., Liu, L., and Duffy, V. G. (2020). Bibliometric analysis of affective computing researches during 1999 2018. Int. J. Hum. Comp. Interact. 36, 801–814. doi: 10.1080/10447318.2019.1688985
Guyer, J. J., Fabrigar, L. R., and Vaughan-Johnston, T. I. (2019). Speech rate, intonation, and pitch: Investigating the bias and cue effects of vocal confidence on persuasion. Personal. Soc. Psychol. Bull. 45, 389–405. doi: 10.1177/0146167218787805
Hammerschmidt, K., and Jürgens, U. (2007). Acoustical correlates of affective prosody. J. Voice 21, 531–540. doi: 10.1016/j.jvoice.2006.03.002
Han, J., Zhang, Z., Cummins, N., and Schuller, B. (2019). Adversarial training in affective computing and sentiment analysis: recent advances and perspectives [review article]. IEEE Comput. Intell. Mag. 14, 68–81. doi: 10.1109/MCI.2019.2901088
Harmon-Jones, C., Bastian, B., and Harmon-Jones, E. (2016). The discrete emotions questionnaire: a new tool for measuring state self-reported emotions. PLoS ONE 11:e0159915. doi: 10.1371/journal.pone.0159915
Harper, R. H. R. (2019). The role of HCI in the age of AI. Int. J. Hum. Comp. Interact. 35, 1331–1344. doi: 10.1080/10447318.2019.1631527
Heracleous, P., and Yoneyama, A. (2019). A comprehensive study on bilingual and multilingual speech emotion recognition using a two-pass classification scheme. PLoS ONE 14:e0220386. doi: 10.1371/journal.pone.0220386
Hildebrand, C., Efthymiou, F., Busquet, F., Hampton, W. H., Hoffman, D. L., and Novak, T. P. (2020). Voice analytics in business research: Conceptual foundations, acoustic feature extraction, and applications. J. Bus. Res. 121, 364–374. doi: 10.1016/j.jbusres.2020.09.020
Hirschberg, J., and Manning, C. D. (2015). Advances in natural language processing. Science 349, 261–266. doi: 10.1126/science.aaa8685
Huttar, G. L. (1968). Relations between prosodic variables and emotions in normal American English utterances. J. Speech Hear. Res. 11, 481–487. doi: 10.1044/jshr.1103.481
Ivanović, M., Budimac, Z., Radovanović, M., Kurbalija, V., Dai, W., Bădică, C., et al. (2015). Emotional agents-state of the art and applications. Comput. Sci. Inf. Syst. 12, 1121–1148. doi: 10.2298/CSIS141026047I
Izard, C. E. (1991). The Psychology of Emotions. New York, NY: Plenum Press. doi: 10.1007/978-1-4899-0615-1
Jacewicz, E., Fox, R. A., and Wei, L. (2010). Between-speaker and within-speaker variation in speech tempo of American English. J. Acoust. Soc. Am. 128, 839–850. doi: 10.1121/1.3459842
Jacob, A. (2016). “Speech emotion recognition based on minimal voice quality features,” in 2016 International Conference on Communication and Signal Processing (ICCSP) (Melmaruvathur), 0886–0890. doi: 10.1109/ICCSP.2016.7754275
Jia, J., Zhang, S., Meng, F., Wang, Y., and Cai, L. (2011). Emotional audio-visual speech synthesis based on PAD. IEEE Trans. Audio Speech Lang. Process. 19, 570–582. doi: 10.1109/TASL.2010.2052246
Jiang, X., and Pell, M. D. (2017). The sound of confidence and doubt. Speech Commun. 88, 106–126. doi: 10.1016/j.specom.2017.01.011
Johar, S. (2016). “Psychology of voice,” in Emotion, Affect and Personality in Speech (Cham: Springer), 9–15.
Johnstone, T., and Scherer, K. R. (1999). “The effects of emotions on voice quality,” in Proceedings of the XIVth International Congress of Phonetic Sciences (Citeseer), 2029–2032.
Juslin, P. N., and Laukka, P. (2003). Communication of emotions in vocal expression and music performance: different channels, same code? Psychol. Bull. 129, 770–814. doi: 10.1037/0033-2909.129.5.770
Juslin, P. N., and Scherer, K. R. (2005). Vocal Expression of Affect. Oxford: Oxford University Press.
Kamiloglu, R. G., Fischer, A. H., and Sauter, D. A. (2020). Good vibrations: a review of vocal expressions of positive emotions. Psychon. Bull. Rev. 1–29. doi: 10.3758/s13423-019-01701-x
Kim, B., Khanna, R., and Koyejo, O. O. (2016). “Examples are not enough, learn to criticize! criticism for interpretability,” in Advances in Neural Information Processing Systems, 2280–2288.
Kratzwald, B., Ilić, S., Kraus, M., Feuerriegel, S., and Prendinger, H. (2018). Deep learning for affective computing: text-based emotion recognition in decision support. Decision Supp. Syst. 115, 24–35. doi: 10.1016/j.dss.2018.09.002
Kwon, O.-W., Chan, K., Hao, J., and Lee, T.-W. (2003). “Emotion recognition by speech signals,” in Eighth European Conference on Speech Communication and Technology (Geneva).
Li, X., Tao, J., Johnson, M. T., Soltis, J., Savage, A., Leong, K. M., et al. (2007). “Stress and emotion classification using Jitter and Shimmer Features,” in: 2007 IEEE International Conference on Acoustics, Speech and Signal Processing - ICASSP '07, IV-1081-IV-1084. doi: 10.1109/ICASSP.2007.367261
Liu, X., Xu, Y., Alter, K., and Tuomainen, J. (2018). Emotional connotations of musical instrument timbre in comparison with emotional speech prosody: evidence from acoustics and event-related potentials. Front. Psychol. 9:737. doi: 10.3389/fpsyg.2018.00737
Łtowski, T. (2014). Timbre, tone color, and sound quality: concepts and definitions. Arch. Acoust. 17, 17–30.
Mallory, E. B., and Miller, V. R. (1958). A possible basis for the association of voice characteristics and personality traits. Speech Monogr. 25, 255–260. doi: 10.1080/03637755809375240
Mehrabian, A. (1996a). Analysis of the big-five personality factors in terms of the PAD temperament model. Aust. J. Psychol. 48, 86–92. doi: 10.1080/00049539608259510
Mehrabian, A. (1996b). Pleasure-arousal-dominance: a general framework for describing and measuring individual differences in temperament. Curr. Psychol. 14, 261–292. doi: 10.1007/BF02686918
Mehrabian, A., and Russell, J. A. (1974). An Approach to Environmental Psychology. Cambridge, MA: The MIT Press.
Miller, N., Maruyama, G., Beaber, R. J., and Valone, K. (1976). Speed of speech and persuasion. J. Personal. Soc. Psychol. 34, 615–624. doi: 10.1037/0022-3514.34.4.615
Mohammadi, G., and Vinciarelli, A. (2012). Automatic personality perception: prediction of trait attribution based on prosodic features. IEEE Trans. Affect. Comput. 3, 273–284. doi: 10.1109/T-AFFC.2012.5
Mullennix, J. W., Johnson, K. A., Topcu-Durgun, M., and Farnsworth, L. M. (1995). The perceptual representation of voice gender. J. Acoust. Soc. Am. 98, 3080–3095. doi: 10.1121/1.413832
Murdoch, W. J., Singh, C., Kumbier, K., Abbasi-Asl, R., and Yu, B. (2019). Definitions, methods, and applications in interpretable machine learning. Proc. Natl. Acad. Sci. U.S.A. 116, 22071–22080. doi: 10.1073/pnas.1900654116
Murray, I. R., and Arnott, J. L. (1993). Toward the simulation of emotion in synthetic speech: A review of the literature on human vocal emotion. J. Acoust. Soc. Am. 93, 1097–1108. doi: 10.1121/1.405558
Nguyen, Q. N., Ta, A., and Prybutok, V. (2019). An integrated model of voice-user interface continuance intention: the gender effect. Int. J. Hum. Comp. Interact. 35, 1362–1377. doi: 10.1080/10447318.2018.1525023
Nip, I. S., and Green, J. R. (2013). Increases in cognitive and linguistic processing primarily account for increases in speaking rate with age. Child Dev. 84, 1324–1337. doi: 10.1111/cdev.12052
Noroozi, F., Marjanovic, M., Njegus, A., Escalera, S., and Anbarjafari, G. (2018). A study of language and classifier-independent feature analysis for vocal emotion recognition. arXiv [Preprint] arXiv:1811.08935.
Osgood, C. E. (1966). Dimensionality of the semantic space for communication via facial expressions. Scand. J. Psychol. 7, 1–30. doi: 10.1111/j.1467-9450.1966.tb01334.x
Osuna, E., Rodríguez, L.-F., Gutierrez-Garcia, J. O., and Castro, L. A. (2020). Development of computational models of emotions: a software engineering perspective. Cogn. Syst. Res. 60, 1–19. doi: 10.1016/j.cogsys.2019.11.001
Özseven, T. (2018). Investigation of the effect of spectrogram images and different texture analysis methods on speech emotion recognition. Appl. Acoustics 142, 70–77. doi: 10.1016/j.apacoust.2018.08.003
Pell, M. D., and Kotz, S. A. (2011). On the time course of vocal emotion recognition. PLoS ONE 6:e27256. doi: 10.1371/journal.pone.0027256
Pernet, C., and Belin, P. (2012). The role of pitch and timbre in voice gender categorization. Front. Psychol. 3:23. doi: 10.3389/fpsyg.2012.00023
Picard, R. W. (2003). Affective computing: challenges. Int. J. Hum. Comp. Stud. 59, 55–64. doi: 10.1016/S1071-5819(03)00052-1
Picard, R. W. (2010). Affective computing: from laughter to IEEE. IEEE Trans. Affect. Comput. 1, 11–17. doi: 10.1109/T-AFFC.2010.10
Pollack, I., Rubenstein, H., and Horowitz, A. (1960). Communication of verbal modes of expression. Lang. Speech 3, 121–130. doi: 10.1177/002383096000300301
Poria, S., Cambria, E., Bajpai, R., and Hussain, A. (2017). A review of affective computing: from unimodal analysis to multimodal fusion. Inf. Fusion 37, 98–125. doi: 10.1016/j.inffus.2017.02.003
Quinto, L., Thompson, W., and Keating, F. (2013). Emotional communication in speech and music: the role of melodic and rhythmic contrasts. Front. Psychol. 4:184. doi: 10.3389/fpsyg.2013.00184
Ray, G. B. (1986). Vocally cued personality prototypes: an implicit personality theory approach. Commun. Monogr. 53, 266–276. doi: 10.1080/03637758609376141
Ribeiro, M. T., Singh, S., and Guestrin, C. (2016). Model-agnostic interpretability of machine learning. arXiv [Preprint] arXiv:1606.05386.
Robb, M. P., Maclagan, M. A., and Chen, Y. (2004). Speaking rates of American and New Zealand varieties of English. Clin. Linguist. Phonet. 18, 1–15. doi: 10.1080/0269920031000105336
Rukavina, S., Gruss, S., Hoffmann, H., Tan, J.-W., Walter, S., and Traue, H. C. (2016). Affective computing and the impact of gender and age. PLoS ONE 11:e0150584. doi: 10.1371/journal.pone.0150584
Russell, J. A. (1980). A circumplex model of affect. J. Personal. Soc. Psychol. 39, 1161–1178. doi: 10.1037/h0077714
Sauter, D. A., Eisner, F., Calder, A. J., and Scott, S. K. (2010). Perceptual cues in nonverbal vocal expressions of emotion. Q. J. Exp. Psychol. 63, 2251–2272. doi: 10.1080/17470211003721642
Schaerlaeken, S., and Grandjean, D. (2018). Unfolding and dynamics of affect bursts decoding in humans. PLoS ONE 13:e0206216. doi: 10.1371/journal.pone.0206216
Scherer, K. R. (2003). Vocal communication of emotion: a review of research paradigms. Speech Commun. 40, 227–256. doi: 10.1016/S0167-6393(02)00084-5
Scherer, K. R., Banse, R., Wallbott, H. G., and Goldbeck, T. (1991). Vocal cues in emotion encoding and decoding. Motiv. Emot. 15, 123–148. doi: 10.1007/BF00995674
Scherer, K. R., and Giles, H. (1979). Social Markers in Speech. Cambridge: Cambridge University Press.
Schuller, D. M., and Schuller, B. W. (2021). A review on five recent and near-future developments in computational processing of emotion in the human voice. Emot. Rev. 13, 44–50. doi: 10.1177/1754073919898526
Schwark, J. D. (2015). Toward a taxonomy of affective computing. Int. J. Hum. Comp. Interact. 31, 761–768. doi: 10.1080/10447318.2015.1064638
Skerry-Ryan, R., Battenberg, E., Xiao, Y., Wang, Y., Stanton, D., Shor, J., et al. (2018). Towards end-to-end prosody transfer for expressive speech synthesis with tacotron. arXiv [Preprint] arXiv:1803.09047.
Sturm, J. A., and Seery, C. H. (2007). Speech and articulatory rates of school-age children in conversation and narrative contexts. Lang. Speech, Hear. Serv. Schools 38, 47–59. doi: 10.1044/0161-1461(2007/005)
Ting, H. N., Chia, S. Y., Abdul Hamid, B., and Mukari, S. Z.-M. S. (2011). Acoustic characteristics of vowels by normal Malaysian Malay young adults. J. Voice 25:e305–e309. doi: 10.1016/j.jvoice.2010.05.007
Toivanen, J., Waaramaa, T., Alku, P., Laukkanen, A.-M., Seppänen, T., Väyrynen, E., et al. (2006). Emotions in [a]: a perceptual and acoustic study. Logoped. Phoniatr. Vocol. 31, 43-48. doi: 10.1080/14015430500293926
Trouvain, J., and Barry, W. J. (2000). “The prosody of excitement in horse race commentaries,” in ISCA Tutorial and Research Workshop (ITRW) on Speech and Emotion (Newcastle), 86-91.
Tucker, P., and Jones, D. M. (1991). Voice as interface: an overview. Int. J. Hum.Comp. Interact. 3, 145–170. doi: 10.1080/10447319109526002
Tusing, K. J., and Dillard, J. P. (2000). The sounds of dominance. Vocal precursors of perceived dominance during interpersonal influence. Hum. Commun. Res. 26, 148–171. doi: 10.1111/j.1468-2958.2000.tb00754.x
Vempala, N. N., and Russo, F. A. (2018). Modeling music emotion judgments using machine learning methods. Front. Psychol. 8:2239. doi: 10.3389/fpsyg.2017.02239
Verhoeven, J., De Pauw, G., and Kloots, H. (2004). Speech rate in a pluricentric language: a comparison between Dutch in Belgium and the Netherlands. Lang. Speech 47, 297–308. doi: 10.1177/00238309040470030401
Wang, C.-C., and Huang, H.-T. (2004). Voice acoustic analysis of normal Taiwanese adults. J.-Chinese Med. Assoc. 67, 179–184.
Wang, Z., Ho, S.-B., and Cambria, E. (2020). A review of emotion sensing: categorization models and algorithms. Multimedia Tools Appl. doi: 10.1007/s11042-019-08328-z
Wayland, R. (2018). Phonetics: A Practical Introduction. Cambridge: Cambridge University Press. doi: 10.1017/9781108289849
Weiguo, W., and Hongman, L. (2019). Artificial emotion modeling in PAD emotional space and human-robot interactive experiment. J. Harbin Inst. Technol. 51, 29–37.
Weninger, F., Eyben, F., Schuller, B., Mortillaro, M., and Scherer, K. (2013). On the acoustics of emotion in audio: what speech, music, and sound have in common. Front. Psychol. 4:292. doi: 10.3389/fpsyg.2013.00292
Williams, C. E., and Stevens, K. N. (1972). Emotions and speech: some acoustical correlates. J. Acoust. Soc. Am. 52, 1238–1250. doi: 10.1121/1.1913238
Yanushevskaya, I., Gobl, C., and Ní Chasaide, A. (2013). Voice quality in affect cueing: does loudness matter? Front. Psychol. 4:335. doi: 10.3389/fpsyg.2013.00335
Keywords: voice-user interface (VUI), affective computing, acoustic features, emotion analysis, PAD model
Citation: Huang K-L, Duan S-F and Lyu X (2021) Affective Voice Interaction and Artificial Intelligence: A Research Study on the Acoustic Features of Gender and the Emotional States of the PAD Model. Front. Psychol. 12:664925. doi: 10.3389/fpsyg.2021.664925
Received: 06 February 2021; Accepted: 18 March 2021;
Published: 04 May 2021.
Edited by:
Pengjiang William Qian, Jiangnan University, ChinaCopyright © 2021 Huang, Duan and Lyu. This is an open-access article distributed under the terms of the Creative Commons Attribution License (CC BY). The use, distribution or reproduction in other forums is permitted, provided the original author(s) and the copyright owner(s) are credited and that the original publication in this journal is cited, in accordance with accepted academic practice. No use, distribution or reproduction is permitted which does not comply with these terms.
*Correspondence: Kuo-Liang Huang, shashi@scfai.edu.cn