- 1Escola Nacional de Saúde Pública Sergio Arouca, Oswaldo Cruz Foundation (Fiocruz), Rio de Janeiro, Brazil
- 2Instituto de Saúde Coletiva, Universidade Federal do Estado do Rio de Janeiro, Rio de Janeiro, Brazil
- 3Instituto Nacional de Infectologia Evandro Chagas, Oswaldo Cruz Foundation (Fiocruz), Rio de Janeiro, Brazil
- 4Centro de Desenvolvimento Tecnológico em Saúde, Oswaldo Cruz Foundation (Fiocruz), Rio de Janeiro, Brazil
- 5Department of Psychiatry and Behavioural Neurosciences, McMaster University, Hamilton, ON, Canada
- 6Bipolar Disorder Program, Laboratory of Molecular Psychiatry, Instituto Nacional de Ciência e Tecnologia Translacional em Medicina, Hospital de Clínicas de Porto Alegre, Porto Alegre, Brazil
- 7Department of Psychiatry, Universidade Federal do Rio Grande do Sul, Porto Alegre, Brazil
- 8Teaching Unit of Psychiatry and Psychological Medicine, Department of Medicine, University of Valencia, CIBERSAM, Valencia, Spain
- 9Institute of Scientific and Technological Communication and Information in Health, Oswaldo Cruz Foundation (Fiocruz), Rio de Janeiro, Brazil
Background: Lifestyle Medicine (LM) aims to address six main behavioral domains: diet/nutrition, substance use (SU), physical activity (PA), social relationships, stress management, and sleep. Digital Health Interventions (DHIs) have been used to improve these domains. However, there is no consensus on how to measure lifestyle and its intermediate outcomes aside from measuring each behavior separately. We aimed to describe (1) the most frequent lifestyle domains addressed by DHIs, (2) the most frequent outcomes used to measure lifestyle changes, and (3) the most frequent DHI delivery methods.
Methods: We followed the Preferred Reporting Items for Systematic Reviews and Meta-Analyses (PRISMA-ScR) Extension for Scoping Reviews. A literature search was conducted using MEDLINE, Cochrane Library, EMBASE, and Web of Science for publications since 2010. We included systematic reviews and meta-analyses of clinical trials using DHI to promote health, behavioral, or lifestyle change.
Results: Overall, 954 records were identified, and 72 systematic reviews were included. Of those, 35 conducted meta-analyses, 58 addressed diet/nutrition, and 60 focused on PA. Only one systematic review evaluated all six lifestyle domains simultaneously; 1 systematic review evaluated five lifestyle domains; 5 systematic reviews evaluated 4 lifestyle domains; 14 systematic reviews evaluated 3 lifestyle domains; and the remaining 52 systematic reviews evaluated only one or two domains. The most frequently evaluated domains were diet/nutrition and PA. The most frequent DHI delivery methods were smartphone apps and websites.
Discussion: The concept of lifestyle is still unclear and fragmented, making it hard to evaluate the complex interconnections of unhealthy behaviors, and their impact on health. Clarifying this concept, refining its operationalization, and defining the reporting guidelines should be considered as the current research priorities. DHIs have the potential to improve lifestyle at primary, secondary, and tertiary levels of prevention—but most of them are targeting clinical populations. Although important advances have been made to evaluate DHIs, some of their characteristics, such as the rate at which they become obsolete, will require innovative research designs to evaluate long-term outcomes in health.
Introduction
According to the latest Global Burden of Disease Study, the drivers of increased Disability-adjusted life years (DALYs) from 1990 to 2019 include six health problems that mostly affect adults older than 50 years (such as ischemic heart disease and diabetes) and four that mostly affect individuals from 10 to 49 years (such as depressive disorders) (1). In 2019, eight of the top 10 risk factors for death and disability were behavioral or metabolic problems [such as high systolic blood pressure, smoking, high fasting plasma glucose, high body-mass index, high low-density lipoprotein (LDL) cholesterol, and alcohol use] (2). Such figures, in addition to the extensive literature on the matter (3–10), emphasize the importance of addressing unhealthy behaviors to prevent morbidity and mortality.
Lifestyle Medicine (LM) profits from evidence-based strategies to prevent and treat the progression of chronic diseases and improve quality of life (11). As per the American College of Lifestyle Medicine (12), the LM foundation is established over six main domains: diet, physical activity (PA), avoiding substance use (SU), sleep, social relationships, and stress management. Thus, LM aims toward a comprehensive change in unhealthy behaviors. Unhealthy behaviors tend to cluster and present an additive effect on decreased survival time without disability and earlier mortality (5, 8, 10, 13). However, most epidemiological studies do not include all the lifestyle domains considered in LM when evaluating health outcomes. Instead, unhealthy behaviors have been considered as independent risk factors. The multidimensional evaluation of lifestyle is becoming more frequent, either by using lifestyle indexes (5, 10) or including multiple lifestyle domains and testing their interaction effects (9). In this sense, our research group recently developed and validated a scale for the multidimensional evaluation of lifestyle (14–17).
Digital health interventions (DHIs) may be understood as interventions delivered via digital technologies (such as apps, digital platforms, and wearables) to improve the health of individuals (18–21). DHIs have increasingly been used to promote behavior change and a healthier lifestyle. For instance, a study conducted in Australia (June 2018–July 2018) searched for digital apps, using the words “health” and “well-being” (in the Australian iTunes and Google Play), and retrieved 2,12,352 related apps (22). In addition, DHIs have been advocated as a way to increase access to health, such as mental health (23), due to their relatively low cost and ease of scalability. However, the debate on how DHIs must be evaluated to ensure their quality and efficacy is far from over (24, 25). Due to the velocity and dynamic changes of DHIs, some argue that the gold standard of medical evaluation—randomized clinical trials (RCT)—would not be appropriate to DHIs assessment. Such velocity may also jeopardize the broader evaluation of effectiveness—which is usually made through systematic reviews and meta-analyses. Whenever there are multiple systematic reviews on the same topic it may be necessary to conduct a meta-review (i.e., the systematic review of systematic reviews) (26). Moreover, if the field is highly heterogeneous and/or wide, scoping meta-reviews may be necessary to summarize concepts, theories, sources, and knowledge gaps.
An important step in the advancement of DHI evaluation was the publication of the Evidence Standards Framework for Digital Health Technologies (27), in the United Kingdom (UK). Although developed to “demonstrate (DHIs) value in the UK health and social care system,” it may be useful for guiding other countries, especially those presenting universal healthcare systems. Regarding digital interventions for preventing unhealthy lifestyles, the guideline recommends a minimum of high-quality observational or quasi-experimental studies demonstrating relevant outcomes. The determination of relevant outcomes in LM, however, is not simple. In 2017, a meta-review on DHIs for cardiometabolic events pointed to the heterogeneity in study measures, such as DHI modalities, populations, and outcomes. Such heterogeneity precluded the formation of strong conclusions regarding the effectiveness of the evaluated interventions (28). In a meta-review evaluating the effect of DHIs on mental health, studies focusing on symptoms of anxiety or depression presented positive small/medium effect sizes (29). However, mental health symptoms are also heterogeneous and may not always represent a clinical psychiatric diagnosis, which could jeopardize future attempts to reproduce the findings.
Besides the outcomes, control groups also may represent a challenge. For instance, a meta-review evaluating DHIs for weight loss concluded that the interventions were more effective than minimal treatment but less effective than face-to-face interventions (30). Also, it is possible that some lifestyle domains, such as PA and diet, are of greater interest for addressing and evaluating DHIs due to the easily measurable outcomes associated with these domains. This hypothesis may make it even harder to evaluate lifestyle as a multidimensional construct, since easily measurable outcomes may lead to an unbalance in the field, with higher representation of lifestyle domains from which there is more available evidence.
Given the huge heterogeneity of DHIs and the specific challenges related to lifestyle research, in this article, we aimed to (1) identify the most frequent lifestyle domains evaluated (diet, PA, SU, sleep, social relationships, and stress management—as proposed by the American College of Lifestyle Medicine (12), and most common combinations of them); (2) describe the most frequent outcomes used to measure lifestyle changes in each domain or in multidimensional evaluations; and (3) to identify the most frequent delivery methods. Answering these questions and revealing research gaps is a crucial step for designing, implementing, and evaluating DHI in order for these interventions to have clinical and public health relevance.
Methods
The methodology followed Arksey and O'Malley's framework for scoping reviews (31) and the Preferred Reporting Items for Systematic Reviews and Meta-Analyses Extension for Scoping Reviews (PRISMA-ScR) (32). A scoping review is defined as a type of research synthesis that aims to map the literature on a particular topic or research area to identify key concepts, research gaps, and sources of evidence to inform practice, policymaking, and research.
The review included the following key phases: (a) formulating a research question, (b) Population, Intervention, Comparator, Outcome, Study Design (PICOS) acronym and eligibility criteria definition (c) identifying relevant studies, (d) study selection, (e) data extraction, and (f) collating, summarizing, and reporting the results.
Research Question
The main research question was, “What are we measuring when we evaluate digital interventions for improving lifestyle?” Specifically, we aimed to answer the following questions: “What are the most frequent lifestyle domains addressed by DHI?,” “What are the most frequent outcomes used to measure lifestyle changes?,” and “What are the most frequent DHI delivery methods?”
Inclusion and Exclusion Criteria
We included systematic reviews or meta-analyses of RCTs that assessed the effectiveness of DHI focusing on any of the lifestyle domains proposed by the American College of Lifestyle Medicine (i.e., diet, SU, PA, social relationships, sleep, and stress management). Those reviews had to include adults (equal or above 18 years) either from the general population, patients, or at-risk population. DHIs could be delivered by smartphone apps, computer or tablet, digital games, digital platforms, monitoring devices, social media, websites, SMS, and/or e-mail. There were no restrictions regarding the control group. Reviews were included if they were published after 2010 (following the widespread use of smartphones) and if published in English, Portuguese, Spanish, or French.
Exclusion criteria were systematic reviews of observational or qualitative studies, study protocols, types of publications different from full articles, such as congress abstract, letters, or comments to the editor. Studies that include only children, adolescents, or pregnant women were excluded because outcomes evaluating the effectiveness of DHI may not be applied to adults (such as the growth curve or early childbirth). Studies including only interventions delivered by SMS were also excluded.
Information Sources and Search Strategies
The search was carried out in September 2020 and updated in October 2021 across four electronic databases: MEDLINE through PubMed, Cochrane Library, EMBASE, and Web of Science. Construction of the search strategy was written using controlled vocabulary terms, specific to the databases; main descriptors were: “lifestyle,” “e-health,” “m-health,” and other similar terms, such as “systematic review” and “clinical trials.” Supplementary Material 1 presents the strategies for each bibliographic database. Additionally, the reference lists of the selected articles were manually scrutinized for other studies that could have been lost in the electronic search.
Study Selection
After the removal of duplicated references, titles and abstracts were screened according to inclusion criteria. Full texts of articles meeting the inclusion criteria were retrieved and checked for their eligibility through complete reading. The process of screening citations and selecting articles was carried out independently by two reviewers, and the discrepancies were resolved by consensus or decided by a third researcher. A table containing the list of excluded studies along with the reasons for their exclusion was prepared (Supplementary Material 1). The Rayyan QCRI web application (https://rayyan.qcri.org/) (33) and Mendeley® were used to screen and manage the references, respectively; both are open-access.
Data Extraction
Data extraction was conducted independently by two reviewers using a pre-pilot spreadsheet in the Excel program. The developed spreadsheet was tested by the study team in a small sample of papers (n = 2) and calibrated before use. Discordances in the extracted data were resolved by consensus. Data extracted from each study are presented in Table 1.
Data Analysis
The data were compiled into a single Excel 2010 spreadsheet for validation and coding. To avoid frequent issues related to papers using different terminology, before the analysis, all string data were reviewed. Synonyms were merged in unique terms decided by consensus with the reviewers. Descriptive statistics were performed analyzing the frequency of each lifestyle domain, the outcomes, and the delivery methods. In constructing the graphs, we used the software R v.4.0.5, the library Rgraphviz and its dependencies (34).
Results
Overall, 953 records were identified by searching the databases, and 1 record was additionally found through the manual search. Title and abstract screenings resulted in 152 included systematic reviews or meta-analyses reporting on the effectiveness of DHIs focusing on one or more of the lifestyle domains evaluated (i.e., diet, SU, PA, social relationships, sleep, and stress management) among adults. A total of 72 studies were included after full-text assessment, of which 35 conducted meta-analyses (Figure 1). All of them were published in English.
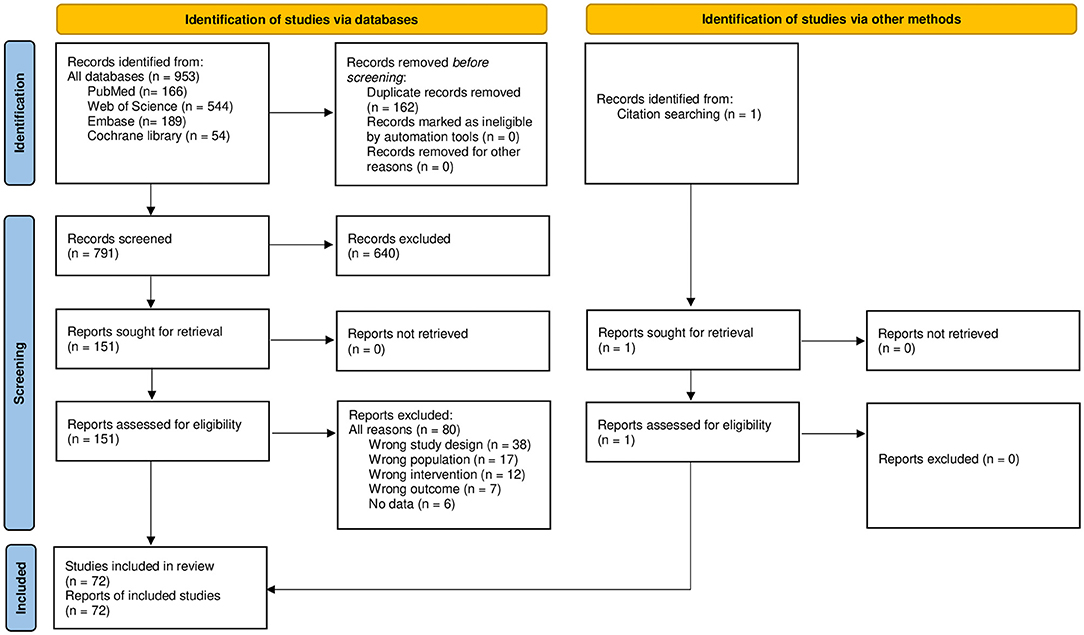
Figure 1. PRISMA 2020 flow diagram. PRISMA, the Preferred Reporting Items for Systematic Reviews and Meta-Analyses.
The systematic reviews included data ranging from a total of 2 (35) to 117 (36) RCTs. Overall, the sample size of the included RCTs ranged between 6 (37) and 69,219 (38) participants. Unfortunately, some systematic reviews did not report the size of the included RCTs. Most DHIs were compared to more than one alternative strategies. Table 2 shows the summary of systematic reviews included.
Of the 72 included reviews, only one evaluated all six lifestyle domains (i.e., diet, PA, SU, social relationships, sleep, and stress management) (100). In this study, the authors evaluated 14 web-based lifestyle programs designed to improve brain health among healthy individuals. A meta-analysis was performed including three RCTs. It showed a small-to-medium positive effect but high heterogeneity.
One systematic review evaluated five lifestyle domains (diet, PA, SU, social relationships, and sleep) (93). The effects of eHealth cardiac rehabilitation on health outcomes were evaluated in 15 trials, and meta-analyses were performed with data from 11 studies. Results indicated that interventions were effective in engaging patients into an active lifestyle, improving quality of life (QoL), and decreasing re-hospitalization. The authors also highlighted that empowerment components and tele-monitoring were crucial to the success of the interventions.
Five systematic reviews evaluated 4 lifestyle domains (59, 62, 74–76): all of them evaluated PA and stress management, 4 evaluated diet, 3 evaluated SU, 2 evaluated social relationships, and 1 evaluated restorative sleep. Most of the studies (n = 4) were related to cardiovascular disease (CVD), and 3 of them performed meta-analyses (59, 62, 76). All meta-analyses showed that DHIs are promising methods for managing CVDs.
Fourteen systematic reviews evaluated 3 lifestyle domains and all of them included diet and PA. Nine evaluated SU, 3 evaluated stress management, and 1 evaluated sleep. Only five conducted meta-analyses. These meta-analyses concluded that there is insufficient evidence on the effectiveness of DHI for reducing overall CVD (78). DHI significantly improved physical activity, HbA1c levels, body weight, empowerment, QoL. DHIs were effective, particularly in the short-term, to decrease the number of drinks consumed/week, DHIs may improve healthy behaviors (i.e., PA and healthy diet) but did not appear to reduce unhealthy behaviors (i.e., smoking, alcohol, and unhealthy diet) (39).
The remaining 52 systematic reviews evaluated only one or two domains. Fifty-one evaluated either diet, PA, or their combination (24 presented meta-analyses) and one evaluated DHI for smoking cessation.
What Are the Most Frequent Lifestyle Domains Addressed by DHI?
Figure 2 shows that the most evaluated domains are Diet/Nutrition (n = 58) and PA (n = 60), while sleep and social relationships are the least evaluated. Interventions targeting clinical populations were the most frequent (n = 32).
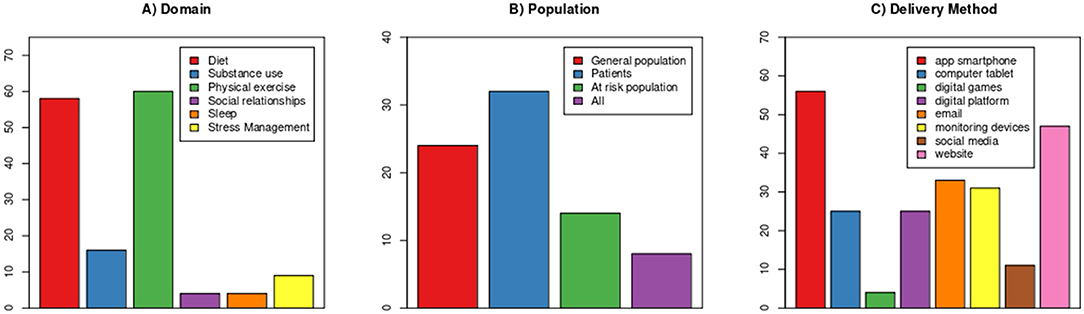
Figure 2. Frequency of systematic reviews that evaluated: (A) each lifestyle domain (physical activity, diet/nutrition, substance use, social relationships, sleep, and stress management); (B) each population; and (C) each delivery method.
What Are the Most Frequent Outcomes Used to Measure Lifestyle Changes?
Figure 3 shows the outcome groups (i.e., objective measures, self-reported measures, biochemical exams, and clinical conditions) and the description of the outcomes, by their frequency. Considering all the groups, objective measures were the most frequently reviewed, in particular, weight, body mass index (BMI), minutes of physical activity (either per day or week), and steps count. The second group was self-reported measures, specifically using scales/questionnaires to evaluate physical activity and dietary intake. Among biochemical measures, the most frequently reviewed were blood lipids (50, 57, 59, 62, 76, 78, 93, 99) and measures related to DM [such a glucose and glycated hemoglobin (39, 42, 51, 78, 79, 84, 104)]. Finally, regarding clinical conditions, CVD/events (41, 52, 59, 62, 80–82, 92, 93, 95, 96) and psychiatric disorders [depression (46, 80, 81, 84, 87, 92, 93) and anxiety (59, 80, 81, 84, 92, 93)] were the most common. Some outcomes, such as smoking cessation, were reviewed either as objective measures (94) or self-reported (39, 79, 80, 83, 93–97, 105).
What Are the Most Frequent DHI Delivery Methods?
The most frequent delivery methods of DHIs were apps (n = 47) and websites (n = 42), followed by e-mails and monitoring devices (Figure 2). Notably, from the 72 studies included, 62 evaluated the effectiveness of more than one delivery method.
Discussion
To our knowledge, this is the first scoping review mapping the most frequent lifestyle domains and outcomes addressed by DHIs. Up to September 2021, we observed that only one systematic review simultaneously evaluated the six lifestyle domains proposed by the American College of Lifestyle Medicine (12). The majority of the reviews targeted diet and physical activity. Objective measures, such as weight and BMI, were the most frequently observed outcomes, followed by self-reported questionnaires. DHIs were delivered using different technologies, with smartphone apps and websites being the most common.
Lifestyle is a developing research theme, which has exponentially attracted interest during the COVID-19 pandemic. Researchers around the world are evaluating the delayed effect of unhealthy behaviors during the pandemic over the population health (i.e., increased incidence of chronic diseases and mental health problems after the changes in lifestyle). Overall, studies evaluated only one or a couple of behaviors that may have changed during this period (106, 107). Such finding is convergent with our results, showing that most reviews evaluated a single behavior/domain or a combination of diet and PA. This approach is valuable for estimating prevalence and specific targets for public policies, as well as increasing our understanding of each specific domain. However, at the individual level, it disregards that a healthy lifestyle represents a complex balance among multiple behaviors and that the development of chronic diseases results from the combination of different risk factors (i.e., genetic, lifestyle, and environmental) (108). LM, relying on six main domains, may be a step forward to address those behaviors, their complex interactions, and interconnections—especially if a multidimensional approach is applied. From a research perspective, the main priority should be to clarify the concept of lifestyle, and how to operationalize it. In this sense, guidelines and reporting guidelines are still missing and could be of great value to inform further studies.
From the 72 systematic reviews included in our study, 58 addressed diet/nutrition, and 60 evaluated PA. Diet and PA are fundamental features of a healthy lifestyle and well-known risk factors for chronic diseases (1, 11, 109). Given this importance, it is not a surprise that the most frequent domains and outcomes evaluated in the literature were related to them. Additional factors that may explain the frequency of DHIs addressing diet/PA include their importance for clinical populations, and the availability of objective outcomes to be measured (such as weight, BMI, and biochemical measures). Still, the high heterogeneity of those outcomes, delivery methods, and control groups precluded most of the systematic reviews to perform meta-analyses on the effectiveness of the DHI. Beyond the consensus on those aspects, an upcoming challenge is if and how hard outcomes (mortality or stroke for example) will ever be potential targets for DHIs. Hard outcomes take a longer follow-up time to be observed, while DHIs are fast-changing and volatile. It is possible we will need “adaptive” interventions, with real-time evaluation and modifications guided by short-term outcomes. Although methodological and ethical issues will be raised, creating new short-lived interventions will hardly be cost-effective and/or sustainable in the long run.
Regarding SU, LM literature concentrates on the use of tobacco and alcohol which, at the population level, present the highest impact on health. Overall, 7.7 million deaths were attributable to smoking in 2019, with smoking being the major cause of death among men in the world (110). Since 2015, it is recommended that clinicians screen all adults for cigarette smoking and prescribe some behavioral and pharmacotherapy therapy for smokers (unless there is some medical contraindication) (111). In 2016, there were 2.4 billion current drinkers globally, and 2.8 million deaths were attributed to alcohol (112). The burden of disease related to alcohol use may be seen as a result of binge drinking and alcohol use disorders, which usually require different treatment/prevention approaches. Given such figures, it is remarkable that we found only sixteen reviews addressing DHI to decrease SU (mostly to reduce smoking). It is possible that researchers do not recognize the importance of these substances or do not have sufficient training to provide evidence-based interventions. In fact, alcohol use disorders are among the least diagnosed medical conditions in clinical practice (113), and alcohol remains the blind spot of global health (114). In addition, as in the case of diet and PA, most reviews evaluating SU were conducted among clinical or at-risk populations, which may represent the most severe and least responsive individuals. Nevertheless, a recent review of systematic reviews found that DHIs have a small but positive effect to decrease alcohol and tobacco use among the general population (115). Such results are encouraging in the sense that DHI, together with public policies, may represent a step forward in the primary prevention of more than 200 diseases caused by smoking and/or alcohol use. One important aspect to evolve in this endeavor is to rigorously evaluate the severity of the SU disorder to determine who is going to benefit and which components of the DHIs are effective.
Stress management and social relationships were among the least evaluated lifestyle domains in our study. Compared to diet and PA, social relationships and stress management present less evidence of the effect on hard outcomes such as mortality. Furthermore, it is difficult to find reliable objective measures/outcomes to be used in DHI. Innovative digital methods to address this gap are necessary and these areas could benefit from passive sensing data obtained via smartphones and wearable devices, in the same way, step count is used to evaluating PA. Digital phenotyping through these tools allows quantifying the biological stress response in real time (116). In experimental conditions, algorithms developed based on physiological proxies of the autonomic nervous system activity (e.g., heart rate, body temperature, skin temperature, and conductance) have demonstrated a high accuracy to detect stress (117). Moreover, smartphone-based episodic audio recordings allow analyses of voice and speech features that may be potential vocal markers of stress (118, 119). Phone usage and the number of calls, texts, and interactions in online social media can be used as proxies of social contact or interaction (116, 120–122), while global positioning system (GPS)-based mobility and conversations captured by smartphone microphones were used to estimate social activity and loneliness in individuals with schizophrenia (123). Social support, however, is more complex than social contacts. To also account for the quality of social relationships, calls to close contacts with strong ties (such as family members and close friends) have been evaluated (124). Moreover, preliminary evidence suggests that passive audio collection on smartphones combined with machine learning techniques helped to identify auditory stimuli from the social environment of adolescents and young mothers that can signal social support (125).
In the same manner, sleep was evaluated in only three reviews. Sleep problems are a growing concern in global public health due to the severe consequences of poor sleep on cognition, emotion, the risk for serious medical conditions, and mortality (126). Particularly during the COVID-19 pandemic, sleep problems have been highly prevalent with ~40% of the general population reporting poor sleep quality (127). Sleep comprises of different areas, such as sleep onset latency, sleep restfulness, sleep disturbances, sleep schedule, efficiency, and daytime napping, among others (126, 128). Traditional, non-digital interventions to improve sleep quality are well-established and have been used to target specific problems, such as cognitive-behavioral therapy (CBT), relaxation and mindfulness therapy, stimulus control therapy, sleep hygiene education, and similar cognitive-behavioral interventions aiming to improve these areas (129, 130). DHIs for sleep improvements, such as digital CBT and digital sleep restriction therapy, have demonstrated potential as effective e-health interventions, however, further refinement and investigations of these delivery methods are required (131, 132).
Digital Health Interventions have emerged as promising methods to help patients and people at risk by providing immediate access to suggestions on improvement of nutritional and PA habits, education about their conditions, and medication adherence. The most frequent delivery method we found was the use of a smartphone, which has increased over the last decade following the widespread of this technology. The progressive advancement of efficient, accessible, and reliable technology has made it possible for various populations to have quick access to information and health. This progress has led to the exponential increase of the number of apps addressing lifestyle (22, 133), and the COVID-19 pandemic will likely lead to a further increase in those numbers. Beyond issues regarding costs (a considerable proportion of apps is paid, although it is hard to estimate the exact proportion) and accessibility (in populations with low cell phone coverage), adherence, usability, and long-term engagement remain to be improved. Co-design and user experience design are important ways to overcome these barriers, as well as behavioral sciences and health economics (134). As mentioned before, the proper scientific evaluation of DHI effectiveness is still to be determined but important steps, such as the publication of the NICE framework (27) and the WHO recommendations (21) have advanced the field. Importantly, while RCTs are the gold standard to evaluate DHIs, the CONSORT EHEALTH extension should be widely adopted to improve their quality, transparency, and reproducibility (135). In the same direction, systematic reviews and meta-analyses should also evaluate how closely are those guidelines being followed by authors. Another aspect that has not been adequately assessed in the systematic reviews is the possibility of harm, including confidentiality breaches. Although most people may believe DHIs present low-level harm, it is still necessary to report on that, and even bring to light harms that were not considered beforehand.
Our study has some limitations, such as the absence of data (e.g., the sample size of included RCTs) for some systematic reviews, and the complexity of study characteristics: different populations (general population and people at risk or living with specific diseases), interventions, delivery methods, and comparators. To properly answer our research question, the inclusion criteria were broad, including as many systematic reviews as possible, which limited synthesis and comparisons between studies. However, scoping reviews usually aim to provide an overview/map of the evidence instead of the result/answer to a particular question (136). Nevertheless, our review highlighted the following major gaps to be addressed regarding DHIs to improve lifestyle: (1) the concept of lifestyle is unclear and fragmented, including mostly one or two unhealthy behaviors; (2) lifestyle domains for which there are few objective outcomes to be measured (such as SU, social relationships, and stress management) are understudied; (3) there is a lack of assessment of hard/long-term outcomes; (4) although DHIs are valued by their high accessibility, most studies are not designed to include the general population; and (5) there is high heterogeneity on reporting the methods, such as outcomes, description of the interventions, and control groups.
The COVID-19 pandemic has shown the importance of connections and real-time data monitoring in health (137) and has consolidated the use of technology (such as tele-medicine) across the globe. DHIs will be increasingly important to improve lifestyle at primary, secondary, and tertiary prevention. However, to be cost-effective and sustainable, such interventions will need to be constantly monitored, adapted, and fully integrated into health systems. Integrating technologies and data are crucial to inform precision medicine, allowing algorithms to suggest the best interventions for each individual. LM and Public Health may profit from those advances to prevent chronic diseases.
Data Availability Statement
The original contributions presented in the study are included in the article/Supplementary Material, further inquiries can be directed to the corresponding author/s.
Author Contributions
RC and RD conceived the study. RC and CR searched the literature. RC, HP, CO, CR, MS, and RD reviewed the literature and extracted data. MR-A analyzed data. RC, MS, and RD wrote the first draft. VB-M and FK reviewed for important intellectual content. All authors contributed to the article and approved the submitted version.
Funding
RD would like to thank the Conselho Nacional de Desenvolvimento Científico e Tecnologico (CNPq #312543/2020-4) and Fundação de Amparo ‘a Pesquisa do Estado do Rio de Janeiro (FAPERJ E-26/203.154/2017).
Conflict of Interest
The authors declare that the research was conducted in the absence of any commercial or financial relationships that could be construed as a potential conflict of interest.
Publisher's Note
All claims expressed in this article are solely those of the authors and do not necessarily represent those of their affiliated organizations, or those of the publisher, the editors and the reviewers. Any product that may be evaluated in this article, or claim that may be made by its manufacturer, is not guaranteed or endorsed by the publisher.
Supplementary Material
The Supplementary Material for this article can be found online at: https://www.frontiersin.org/articles/10.3389/fpubh.2021.735624/full#supplementary-material
References
1. Abbafati C, Abbas KM, Abbasi-Kangevari M, Abd-Allah F, Abdelalim A, Abdollahi M, et al. Global burden of 369 diseases and injuries in 204 countries and territories, 1990–2019: a systematic analysis for the global burden of disease study 2019. Lancet. (2020) 396:1204–22. doi: 10.1016/S0140-6736(20)30925-9
2. Abbafati C, Abbas KM, Abbasi-Kangevari M, Abd-Allah F, Abdelalim A, Abdollahi M, et al. Global burden of 87 risk factors in 204 countries and territories, 1990–2019: a systematic analysis for the global burden of disease study 2019. Lancet. (2020) 396:1223–49. doi: 10.1016/S0140-6736(20)30752-2
3. Lippi G, Henry BM, Sanchis-Gomar F. Physical inactivity and cardiovascular disease at the time of coronavirus disease 2019 (COVID-19). Eur J Prev Cardiol. (2020) 27:906–8. doi: 10.1177/2047487320916823
4. Chen P, Mao L, Nassis GP, Harmer P, Ainsworth BE, Li F. Coronavirus disease (COVID-19): the need to maintain regular physical activity while taking precautions. J Sport Heal Sci. (2020) 9:103–4. doi: 10.1016/j.jshs.2020.02.001
5. Nyberg ST, Singh-Manoux A, Pentti J, Madsen IEH, Sabia S, Alfredsson L, et al. Association of healthy lifestyle with years lived without major chronic diseases. JAMA Intern Med. (2020) 180:760–8. doi: 10.1001/jamainternmed.2020.0618
6. Firth J, Solmi M, Wootton RE, Vancampfort D, Schuch FB, Hoare E, et al. A meta-review of “ lifestyle psychiatry ”: the role of exercise , smoking , diet and sleep in the prevention and treatment of mental disorders. World Psychiatry. (2020) 19:360–80. doi: 10.1002/wps.20773
7. Wu W, Xu W, Englund S, Shang Y, Pan K-Y, Rizzuto D. Can health behaviours prolong survival and compress the period of survival with the disability? A population-based cohort study. Age Ageing. (2021) 50:480–7. doi: 10.1093/ageing/afaa143
8. Lee DH, Nam JY, Kwon S, Keum NN, Lee JT, Shin MJ, et al. Lifestyle risk score and mortality in Korean adults: a population-based cohort study. Sci Rep. (2020) 10:1–8. doi: 10.1038/s41598-020-66742-y
9. Ruiz-Estigarribia L, Martínez-González MÁ, Díaz-Gutiérrez J, Gea A, Rico-Campà A, Bes-Rastrollo M. Lifestyle-Related factors and total mortality in a mediterranean prospective cohort. Am J Prev Med. (2020) 59:e59–67. doi: 10.1016/j.amepre.2020.01.032
10. Wu M, Wang J, Zhu Y, Lu J, Li D, Yu Z, et al. Impact of individual and combined lifestyle factors on mortality in china: a cohort study. Am J Prev Med. (2020) 59:461–8. doi: 10.1016/j.amepre.2020.01.029
12. American College of Lifestyle Medicine. What is Lifestyle Medicine? (2021). Available online at: https://lifestylemedicine.org/ACLM/About/What_is_Lifestyle_Medicine/ACLM/About/What_is_Lifestyle_Medicine_/Lifestyle_Medicine.aspx?hkey=26f3eb6b-8294-4a63-83de-35d429c3bb88 (accessed December 12, 2021).
13. Loef M, Walach H. The combined effects of healthy lifestyle behaviors on all cause mortality: a systematic review and meta-analysis. Prev Med. (2012) 55:163–70. doi: 10.1016/j.ypmed.2012.06.017
14. Balanzá-Martínez, V Kapczinski F, de Azevedo Cardoso, T Atienza-Carbonell B, Rosa A, Mota J, et al. The assessment of lifestyle changes during the COVID-19 pandemic using a multidimensional scale. Rev Psiquiatr Salud Mentl. (2021) 14:16–26. doi: 10.1016/j.rpsm.2020.07.003
15. Giner-Murillo M, Atienza-Carbonell B, Cervera-Martínez J, Bobes-Bascarán T, Crespo-Facorro B, De Boni RB, et al. Lifestyle in undergraduate students and demographically matched controls during the COVID-19 pandemic in spain. Int J Environ Res Public Heal Artic Public Heal. (2021) 18:8133. doi: 10.3390/ijerph18158133
16. De Boni RB, Balanzá-Martínez V, Mota JC, Cardoso TDA, Ballester P, Atienza-Carbonell B, et al. Depression, anxiety, and lifestyle among essential workers: a web survey from brazil and spain during the COVID-19 pandemic. J Med Internet Res. (2020) 22:e22835. doi: 10.2196/22835
17. De Boni RB, Ribeiro-Alves M, Mota JC, Gomes M, Balanzá-Martínez V, Kapczinski F, et al. The cumulative effect of multiple dimensions of lifestyle on risky drinking during the Covid-19 pandemic. Prev Med. (2021) 150:106718. doi: 10.1016/j.ypmed.2021.106718
18. Murray E, Hekler EB, Professor A, Andersson G, Collins LM, Doherty A, et al. Evaluating digital health interventions: key questions and approaches. Am J Prev Med. (2016) 51:843–51. doi: 10.1016/j.amepre.2016.06.008
19. Michie S, Yardley L, West R, Patrick K, Greaves F. Developing and evaluating digital interventions to promote behavior change in health and health care: recommendations resulting from an international workshop. J Med Internet Res. (2017) 19:e232. doi: 10.2196/jmir.7126
21. WHO. WHO Guideline: Recommendations on Digital Interventions for Health System Strengthening. Geneva: World Health Organization (2019).
22. McKay FH, Wright A, Shill J, Stephens H, Uccellini M. Using health and well-being apps for behavior change: a systematic search and rating of apps. JMIR mHealth uHealth. (2019) 7:e11926. doi: 10.2196/11926
23. Patel V, Saxena S, Lund C, Thornicroft G, Baingana F, Bolton P, et al. The lancet commission on global mental health and sustainable development. Lancet. (2018) 392:1553–98. doi: 10.1016/S0140-6736(18)31612-X
24. The Lancet. Is digital medicine different? Lancet. (2018) 392:95. doi: 10.1016/S0140-6736(18)31562-9
25. Greaves F, Joshi I, Campbell M, Roberts S, Patel N, Powell J. What is an appropriate level of evidence for a digital health intervention? Lancet. (2018) 392:2665–7. doi: 10.1016/S0140-6736(18)33129-5
26. Aromataris E, Fernandez R, Godfrey CM, Holly C, Khalil H, Tungpunkom P. Summarizing systematic reviews: methodological development, conduct and reporting of an umbrella review approach. Int J Evid Based Healthc. (2015) 13:132–40. doi: 10.1097/XEB.0000000000000055
27. NICE (National Institute for Health Care Excellence). Evidence Standards Framework for Digital Health Technologies. (2021). Available online at: https://www.nice.org.uk/corporate/ecd7
28. O'Neil A, Cocker F, Rarau P, Baptista S, Cassimatis M, Taylor CB, et al. Using digital interventions to improve the cardiometabolic health of populations: ameta-review of reporting quality. J Am Med Informatics Assoc. (2017) 24:867–79. doi: 10.1093/jamia/ocw166
29. Lecomte T, Potvin S, Corbière M, Guay S, Samson C, Cloutier B, et al. Mobile apps for mental health issues: meta-review of meta-analyses. JMIR mHealth uHealth. (2020) 8:1–14. doi: 10.2196/17458
30. Sorgente A, Pietrabissa G, MauroManzoni G, Re F, Simpson S, Perona S, et al. Web-based interventions for weight loss or weight loss maintenance in overweight and obese people: a systematic review of systematic reviews. J Med Internet Res. (2017) 19:e229. doi: 10.2196/jmir.6972
31. Arksey H, O'Malley L. Scoping studies: towards a methodological framework. Int J Soc Res Methodol. (2005) 8:19–32. doi: 10.1080/1364557032000119616
32. Tricco AC, Lillie E, Zarin W, O'Brien KK, Colquhoun H, Levac D, et al. PRISMA extension for scoping reviews (PRISMA-ScR): checklist and explanation. Ann Intern Med. (2018) 169:467–73. doi: 10.7326/M18-0850
33. Ouzzani M, Hammady H, Fedorowicz Z, Elmagarmid A. Rayyan—a web and mobile app for systematic reviews. Syst Rev. (2016) 5:210. doi: 10.1186/s13643-016-0384-4
34. Hansen K, Gentry J, Long L, Gentleman R, Falcon S, Hahne F, et al. Rgraphviz: provides plotting capabilities for R graph objects. R Package. (2021). Available online at: https://www.bioconductor.org/packages/release/bioc/html/Rgraphviz.html (accessed December 14, 2021).
35. Daryabeygi-Khotbehsara R, Shariful Islam SM, Dunstan D, McVicar J, Abdelrazek M, Maddison R. Smartphone-based interventions to reduce sedentary behavior and promote physical activity using integrated dynamic models: systematic review. J Med Internet Res. (2021) 23:e26315. doi: 10.2196/26315
36. Mönninghoff A, Kramer JN, Hess AJ, Ismailova K, Teepe GW, Tudor Car L, et al. Long-term effectiveness of mhealth physical activity interventions: systematic review and meta-analysis of randomized controlled trials. J Med Internet Res. (2021) 23:e26699. doi: 10.2196/26699
37. Hardeman W, Houghton J, Lane K, Jones A, Naughton F. A systematic review of just-in-time adaptive interventions (JITAIs) to promote physical activity. Int J Behav Nutr Phys Act. (2019) 16:31. doi: 10.1186/s12966-019-0792-7
38. Muller AM, Alley S, Schoeppe S, Vandelanotte C. The effectiveness of e-& mHealth interventions to promote physical activity and healthy diets in developing countries: a systematic review. Int J Behav Nutr Phys Act. (2016) 13:109. doi: 10.1186/s12966-016-0434-2
39. Akinosun AS, Polson R, Diaz-Skeete Y, De Kock JH, Carragher L, Leslie S, et al. Digital technology interventions for risk factor modification in patients with cardiovascular disease: systematic review and meta-analysis. JMIR mHealth uHealth. (2021) 9:e21061. doi: 10.2196/21061
40. Allen JK, Stephens J, Patel A. Technology-assisted weight management interventions: systematic review of clinical trials. Telemed eHealth. (2014) 20:1103–20. doi: 10.1089/tmj.2014.0030
41. Beleigoli AM, Andrade AQ, Cançado AG, Paulo MNL, Diniz MDFH, Ribeiro AL. Web-based digital health interventions for weight loss and lifestyle habit changes in overweight and obese adults: systematic review and meta-analysis. J Med Internet Res. (2019) 21:e298. doi: 10.2196/jmir.9609
42. Beratarrechea A, Lee AG, Willner JM, Jahangir E, Ciapponi A, Rubinstein A. The impact of mobile health interventions on chronic disease outcomes in developing countries: a systematic review. Telemed eHealth. (2014) 20:75–82. doi: 10.1089/tmj.2012.0328
43. Bian RR, Piatt GA, Sen A, Plegue MA, De Michele ML, Hafez D, et al. The effect of technology-mediated diabetes prevention interventions on weight: a meta-analysis. J Med Internet Res. (2017) 19:e76. doi: 10.2196/jmir.4709
44. Bossen D, Veenhof C, Dekker J, De Bakker D. The effectiveness of self-guided web-based physical activity interventions among patients with a chronic disease: A systematic review. J Phys Act Heal. (2014) 11:665–77. doi: 10.1123/jpah.2012-0152
45. Brickwood KJ, Watson G, O'Brien J, Williams AD. Consumer-based wearable activity trackers increase physical activity participation: systematic review and meta-analysis. JMIR Mhealth Uhealth (2019) 7:e11819. doi: 10.2196/11819
46. Brørs G, Pettersen TR, Hansen TB, Fridlund B, Hølvold LB, Lund H, et al. Modes of e-Health delivery in secondary prevention programmes for patients with coronary artery disease: a systematic review. BMC Health Serv Res. (2019) 19:364. doi: 10.1186/s12913-019-4106-1
47. Cavero-Redondo I, Martinez-Vizcaino V, Fernandez-Rodriguez R, Saz-Lara A, Pascual-Morena C, Álvarez-Bueno C. Effect of behavioral weight management interventions using lifestyle mhealth self-monitoring on weight loss: a systematic review and meta-analysis. Nutrients. (2020) 12:1977. doi: 10.3390/nu12071977
48. Chea Tham SW, Stul KR, Fan Tigrassi M, Motel I. The efficacy of wearable activity tracking technology as part of a weight loss program: a systematic review. J Sports Med Phys Fitness. (2018) 58:534–48. doi: 10.23736/S0022-4707.17.07437-0
49. Christiansen PK, Skjøth MM, Rothmann MJ, Vinter CA, Lamont RF, Draborg E. Lifestyle interventions to maternal weight loss after birth: A systematic review. Syst Rev. (2019) 8:327. doi: 10.1186/s13643-019-1186-2
50. Cotter AP, Durant N, Agne AA, Cherrington AL. Internet interventions to support lifestyle modification for diabetes management: a systematic review of the evidence. J Diabetes Compl. (2014) 28:243–51. doi: 10.1016/j.jdiacomp.2013.07.003
51. Covolo L, Ceretti E, Moneda M, Castaldi S, Gelatti U. Does evidence support the use of mobile phone apps as a driver for promoting healthy lifestyles from a public health perspective? A systematic review of randomized control trials. Patient Educ Couns. (2017) 100:2231–43. doi: 10.1016/j.pec.2017.07.032
52. Devi R, Singh SJ, Powell J, Fulton EA, Igbinedion E, Rees K. Internet-based interventions for the secondary prevention of coronary heart disease. Cochrane database Syst Rev. (2015) 12:CD009386. doi: 10.1002/14651858.CD009386.pub2
53. Duan Y, Shang B, Liang W, Du G, Yang M, Rhodes RE. Effects of eHealth-based multiple health behavior change interventions on physical activity, healthy diet, and weight in people with noncommunicable diseases: systematic review and meta-analysis. J Med Internet Res. (2021) 23:1–13. doi: 10.2196/23786
54. Dutton GR, Laitner MH, Perri MG. Lifestyle interventions for cardiovascular disease risk reduction: a systematic review of the effects of diet composition, food provision, and treatment modality on weight loss. Curr Atheroscler Rep. (2014) 16:442. doi: 10.1007/s11883-014-0442-0
55. Fakih El Khoury C, Karavetian M, Halfens RJG, Crutzen R, Khoja L, Schols JMGA. The effects of dietary mobile apps on nutritional outcomes in adults with chronic diseases: a systematic review and meta-analysis. J Acad Nutr Diet. (2019) 119:626–51. doi: 10.1016/j.jand.2018.11.010
56. Haberlin C, O'Dwyer T, Mockler D, Moran J, O'Donnell DM, Broderick J. The use of eHealth to promote physical activity in cancer survivors: a systematic review. Support Care Cancer. (2018) 26:3323–36. doi: 10.1007/s00520-018-4305-z
57. Halldorsdottir H, Thoroddsen A, Ingadottir B. Impact of technology-based patient education on modifiable cardiovascular risk factors of people with coronary heart disease: a systematic review. Patient Educ Couns. (2020) 103:2018–28. doi: 10.1016/j.pec.2020.05.027
58. Hayba N, Partridge SR, Nour MM, Grech A, Allman Farinelli M. Effectiveness of lifestyle interventions for preventing harmful weight gain among young adults from lower socioeconomic status and ethnically diverse backgrounds: a systematic review. Obes Rev. (2018) 19:333–46. doi: 10.1111/obr.12641
59. Huang K, Liu W, He D, Huang B, Xiao D, Peng Y, et al. Telehealth interventions versus center-based cardiac rehabilitation of coronary artery disease: a systematic review and meta-analysis. Eur J Prev Cardiol. (2015) 22:959–71. doi: 10.1177/2047487314561168
60. Hutchesson MJ, Rollo ME, Krukowski R, Ells L, Harvey J, Morgan PJ, et al. EHealth interventions for the prevention and treatment of overweight and obesity in adults: a systematic review with meta-analysis. Diabetes Technol Ther. (2016) 18:S67. doi: 10.1111/obr.12268
61. Hwang N-K, Jung Y-J, Park J-S. Information and communications technology-based telehealth approach for occupational therapy interventions for cancer survivors: a systematic review. Healthcare. (2020) 8:355. doi: 10.3390/healthcare8040355
62. Jin K, Khonsari S, Gallagher R, Gallagher P, Clark AM, Freedman B, et al. Telehealth interventions for the secondary prevention of coronary heart disease: a systematic review and meta-analysis. Eur J Cardiovasc Nurs. (2019) 18:260–71. doi: 10.1177/1474515119826510
63. Joiner KL, Nam S, Whittemore R. Lifestyle interventions based on the diabetes prevention program delivered via eHealth: a systematic review and meta-analysis. Prev Med. (2017) 100:194–207. doi: 10.1016/j.ypmed.2017.04.033
64. Kelly JT, Reidlinger DP, Hoffmann TC, Campbell KL. Telehealth methods to deliver dietary interventions in adults with chronic disease: a systematic review and meta-analysis. Am J Clin Nutr. (2016) 104:1693–702. doi: 10.3945/ajcn.116.136333
65. Khoo S, Mohbin N, Ansari P, Al-Kitani M, Müller AM. Mhealth interventions to address physical activity and sedentary behavior in cancer survivors: a systematic review. Int J Environ Res Public Health. (2021) 18:5798. doi: 10.3390/ijerph18115798
66. Kim G, Lee J-S, Lee S-K. A technology-mediated interventional approach to the prevention of metabolic syndrome: a systematic review and meta-analysis. Int J Environ Res Public Health. (2021) 18:512. doi: 10.3390/ijerph18020512
67. Klassen KM, Douglass CH, Brennan L, Truby H, Lim MSC. Social media use for nutrition outcomes in young adults: a mixed-methods systematic review. Int J Behav Nutr Phys Act. (2018) 15:70. doi: 10.1186/s12966-018-0696-y
68. Kodama S, Saito K, Tanaka S, Horikawa C, Fujiwara K, Hirasawa R, et al. Effect of web-based lifestyle modification on weight control: a meta-analysis. Int J Obes. (2012) 36:675–85. doi: 10.1038/ijo.2011.121
69. Kuo CC, Su YJ, Lin CC. A systematic review and meta-analysis: effectiveness of internet empowerment-based self-management interventions on adults with metabolic diseases. J Adv Nurs. (2018) 74:1787–802. doi: 10.1111/jan.13574
70. Lee M, Lee H, Kim Y, Kim J, Cho M, Jang J, et al. Mobile app-based health promotion programs: a systematic review of the literature. Int J Environ Res Public Health. (2018) 15:2838. doi: 10.3390/ijerph15122838
71. Levine DM, Savarimuthu S, Squires A, Nicholson J, Jay M. Technology-assisted weight loss interventions in primary care: a systematic review. J Gen Intern Med. (2015) 30:107–17. doi: 10.1007/s11606-014-2987-6
72. Lewis ZH, Lyons EJ, Jarvis JM, Baillargeon J. Using an electronic activity monitor system as an intervention modality: a systematic review health behavior, health promotion and society. BMC Public Health. (2015) 15:585. doi: 10.1186/s12889-015-1947-3
73. Li C, Chen X, Bi X. Wearable activity trackers for promoting physical activity: a systematic meta-analytic review. Int J Med Inform. (2021) 152:104487. doi: 10.1016/j.ijmedinf.2021.104487
74. Lunde P, Nilsson BB, Bergland A, Kværner KJ, Bye A. The effectiveness of smartphone apps for lifestyle improvement in noncommunicable diseases: systematic review and meta-analyses. J Med Internet Res. (2018) 20:e162. doi: 10.2196/jmir.9751
75. Lyzwinski LN, Caffery L, Bambling M, Edirippulige S. A systematic review of electronic mindfulness-based therapeutic interventions for weight, weight-related behaviors, and psychological stress. Telemed eHealth. (2018) 24:173–84. doi: 10.1089/tmj.2017.0117
76. Ma Y, HY C, Cheng L, JWH S. The effectiveness of electronic health interventions on blood pressure control, self-care behavioural outcomes and psychosocial well-being in patients with hypertension: a systematic review and meta-analysis. Int J Nurs Stud. (2019) 92:27–46. doi: 10.1016/j.ijnurstu.2018.11.007
77. McCarroll R, Eyles H, Ni Mhurchu C. Effectiveness of mobile health (mHealth) interventions for promoting healthy eating in adults: a systematic review. Prev Med. (2017) 105:156–68. doi: 10.1016/j.ypmed.2017.08.022
78. Merriel SWD, Andrews V, Salisbury C. Erratum for “Telehealth interventions for primary prevention of cardiovascular disease: a systematic review and meta-analysis”. Prev Med. (2014) 67:343. doi: 10.1016/j.ypmed.2014.07.012
79. Michaud TL, Ern J, Scoggins D, Su D. Assessing the impact of telemonitoring-facilitated lifestyle modifications on diabetes outcomes: a systematic review and meta-analysis. Telemed eHealth. (2021) 27:124–36. doi: 10.1089/tmj.2019.0319
80. Oosterveen E, Tzelepis F, Ashton L, Hutchesson MJ. A systematic review of eHealth behavioral interventions targeting smoking, nutrition, alcohol, physical activity and/or obesity for young adults. Prev Med. (2017) 99:197–206. doi: 10.1016/j.ypmed.2017.01.009
81. Palacios J, Lee GA, Duaso M, Clifton A, Norman IJ, Richards D, et al. Internet-Delivered Self-management Support for Improving Coronary Heart Disease and Self-management Y Related Outcomes A Systematic Review. J Cardiovasc Nurs. (2017) 32:9–23. doi: 10.1097/JCN.0000000000000392
82. Elnaggar A, Park VT, Lee SJ, Bender M, Siegmund LA, Park LG. Patients' use of social media for diabetes self-care: systematic review. J Med Internet Res. (2020) 22:e14209. doi: 10.2196/14209
83. Pfaeffli Dale L, Dobson R, Whittaker R, Maddison R. The effectiveness of mobile-health behaviour change interventions for cardiovascular disease self-management: a systematic review. Eur J Prev Cardiol. (2015) 23:801–17. doi: 10.1177/2047487315613462
84. Pradal-Cano L, Lozano-Ruiz C, Pereyra-Rodríguez JJ, Saigí-Rubió F, Bach-Faig A, Esquius L, et al. Using mobile applications to increase physical activity: a systematic review. Int J Environ Res Public Health. (2020) 17:1–16. doi: 10.3390/ijerph17218238
85. Podina IR, Fodor LA. Critical review and meta-analysis of multicomponent behavioral E-health interventions for weight loss. Heal Psychol. (2018) 37:501–15. doi: 10.1037/hea0000623
86. Rodriguez Rocha NP, Kim H. eHealth interventions for fruit and vegetable intake: a meta-analysis of effectiveness. Heal Educ Behav. (2019) 46:947–59. doi: 10.1177/1090198119859396
87. Ryan K, Dockray S, Linehan C. A systematic review of tailored eHealth interventions for weight loss. Digit Heal. (2019) 5:2055207619826685. doi: 10.1177/2055207619826685
88. Schoeppe S, Alley S, Van Lippevelde W, Bray NA, Williams SL, Duncan MJ, et al. Efficacy of interventions that use apps to improve diet, physical activity and sedentary behaviour: a systematic review. Int J Behav Nutr Phys Act. (2016) 13:127. doi: 10.1186/s12966-016-0454-y
89. Semper HM, Povey R, Clark-Carter D. A systematic review of the effectiveness of smartphone applications that encourage dietary self-regulatory strategies for weight loss in overweight and obese adults. Obes Rev. (2016) 17:895–906. doi: 10.1111/obr.12428
90. Seo DC, Niu J. Evaluation of internet-based interventions on waist circumference reduction: a meta-analysis. J Med Internet Res. (2015) 17:1–13. doi: 10.2196/jmir.3921
91. Short CE, James EL, Plotnikoff RC, Girgis A. Efficacy of tailored-print interventions to promote physical activity: a systematic review of randomised trials. Int J Behav Nutr Phys Act. (2011) 8:113. doi: 10.1186/1479-5868-8-113
92. Stevenson JK, Campbell ZC, Webster AC, Chow CK, Tong A, Craig JC, et al. Ehealth interventions for people with chronic kidney disease. Cochrane Database Syst Rev. (2019) 2019 8:CD012379. doi: 10.1002/14651858.CD012379.pub2
93. Su JJ, Yu DSF, Paguio JT. Effect of eHealth cardiac rehabilitation on health outcomes of coronary heart disease patients: a systematic review and meta-analysis. J Adv Nurs. (2020) 76:754–72. doi: 10.1111/jan.14272
94. Taylor G, Dalili M, Semwal M, Civljak M, Sheikh A, Car J. Internet-based interventions for smoking cessation (Review). Cochrane Database Syst Rev. (2017) 9:CD007078. doi: 10.1002/14651858.CD007078.pub5
95. Tighe SA, Ball K, Kensing F, Kayser L, Rawstorn JC, Maddison R. Toward a digital platform for the self-management of noncommunicable disease: systematic review of platform-like interventions. J Med Internet Res. (2020) 22. doi: 10.2196/16774
96. Tong HL, Quiroz JC, Kocaballi AB, Fat SCM, Dao KP, Gehringer H, et al. Personalized mobile technologies for lifestyle behavior change: a systematic review, meta-analysis, and meta-regression. Prev Med. (2021) 148:106532. doi: 10.1016/j.ypmed.2021.106532
97. Turan Kavradim S, Özer Z, Boz I. Effectiveness of telehealth interventions as a part of secondary prevention in coronary artery disease: a systematic review and meta-analysis. Scand J Caring Sci. (2020) 34:585–603. doi: 10.1111/scs.12785
98. Vegting IL, Schrijver EJM, Otten RHJ, Nanayakkara PWB. Internet programs targeting multiple lifestyle interventions in primary and secondary care are not superior to usual care alone in improving cardiovascular risk profile: a systematic review. Eur J Intern Med. (2014) 25:73–81. doi: 10.1016/j.ejim.2013.08.008
99. Villinger K, Wahl DR, Boeing H, Schupp HT, Renner B. The effectiveness of app-based mobile interventions on nutrition behaviours and nutrition-related health outcomes: a systematic review and meta-analysis. Obes Rev. (2019) 20:1465–84. doi: 10.1111/obr.12903
100. Wesselman LMP, Hooghiemstra AM, Schoonmade LJ, De Wit MCJ, Van Der Flier WM, Sikkes SAM. Web-based multidomain lifestyle programs for brain health: comprehensive overview and meta-analysis. J Med Internet Res. (2019) 21:1–17. doi: 10.2196/12104
101. Wieland S, Falzon L, Sciamanna C, Trudeau K, Folse S, Schwarts J, et al. Interactive computer-based interventions for weight loss or weight maintenance in overweight or obese people. Cochrane Database Syst Rev. (2012) 8:CD007675. doi: 10.1002/14651858.CD007675.pub2
102. Williams G, Hamm MP, Shulhan J, Vandermeer B, Hartling L. Social media interventions for diet and exercise behaviours: a systematic review and meta-analysis of randomised controlled trials. BMJ Open. (2014) 4:e003926. doi: 10.1136/bmjopen-2013-003926
103. Willmott TJ, Pang B, Rundle-Thiele S, Badejo A. Weight management in young adults: systematic review of electronic health intervention components and outcomes. J Med Internet Res. (2019) 21:e10265. doi: 10.2196/10265
104. Wu X, Guo X, Zhang Z. The efficacy of mobile phone apps for lifestyle modification in diabetes: systematic review and meta-analysis. JMIR Mhealth Uhealth. (2019) 7:e12297. doi: 10.2196/12297
105. Xu H, Long H. The effect of smartphone app–based interventions for patients with hypertension: systematic review and meta-analysis. JMIR mHealth uHealth. (2020) 8:e21759. doi: 10.2196/21759
106. Giuntella O, Hyde K, Saccardo S, Sadoff S. Lifestyle and mental health disruptions during COVID-19. Proc Natl Acad Sci USA. (2021) 118:e2016632118. doi: 10.1073/pnas.2016632118
107. Klemperer EM, West JC, Peasley-Miklus C, Villanti AC. Change in tobacco and electronic cigarette use and motivation to quit in response to COVID-19. Nicotine Tob Res. (2020) 22:1662–3. doi: 10.1093/ntr/ntaa072
108. Airhihenbuwa CO, Tseng T-S, Sutton VD, Price L. Global perspectives on improving chronic disease prevention and management in diverse settings. Prev Chronic Dis. (2021) 18:210055. doi: 10.5888/pcd18.210055
110. Reitsma MB, Kendrick PJ, Ababneh E, Abbafati C, Abbasi-Kangevari M, Abdoli A, et al. Spatial, temporal, and demographic patterns in prevalence of smoking tobacco use and attributable disease burden in 204 countries and territories, 1990–2019: a systematic analysis from the global burden of disease study 2019. Lancet. (2021) 397:2337–60. doi: 10.1016/S0140-6736(21)01169-7
111. Siu AL. Behavioral and pharmacotherapy interventions for tobacco smoking cessation in adults, including pregnant women: U.S. Preventive services task force recommendation statement. Ann Intern Med. (2015) 163:622. doi: 10.7326/M15-2023
112. Griswold MG, Fullman N, Hawley C, Arian N, Zimsen SRM, Tymeson HD, et al. Alcohol use and burden for 195 countries and territories, 1990-2016: a systematic analysis for the global burden of disease study 2016. Lancet. (2018) 392:1015–35. doi: 10.1016/S0140-6736(18)31310-2
113. Mitchell AJ, Meader N, Bird V, Rizzo M. Clinical recognition and recording of alcohol disorders by clinicians in primary and secondary care: meta-analysis. Br J Psychiatry. (2012) 201:93–100. doi: 10.1192/bjp.bp.110.091199
114. Marten R, Amul GGH, Casswell S. Alcohol: global health's blind spot. Lancet Glob Heal. (2020) 8:e329–30. doi: 10.1016/S2214-109X(20)30008-5
115. Gold N, Yau A, Rigby B, Dyke C, Remfry EA, Chadborn T. Effectiveness of digital interventions for reducing behavioral risks of cardiovascular disease in nonclinical adult populations: systematic review of reviews. J Med Internet Res. (2021) 23:e19688. doi: 10.2196/19688
116. Goodday SM, Friend S. Unlocking stress and forecasting its consequences with digital technology. Npj Digit Med. (2019) 2:75. doi: 10.1038/s41746-019-0151-8
117. Hickey BA, Chalmers T, Newton P, Lin C-T, Sibbritt D, McLachlan CS, et al. Smart devices and wearable technologies to detect and monitor mental health conditions and stress: a systematic review. Sensors. (2021) 21:3461. doi: 10.3390/s21103461
118. Slavich GM, Taylor S, Picard RW. Stress measurement using speech: recent advancements, validation issues, and ethical and privacy considerations. Stress. (2019) 22:408–13. doi: 10.1080/10253890.2019.1584180
119. Sheikh M, Qassem M, Kyriacou PA. Wearable, environmental, and smartphone-based passive sensing for mental health monitoring. Front Digit Heal. (2021) 3:662811. doi: 10.3389/fdgth.2021.662811
120. Kyriazakos S, Pnevmatikakis A, Cesario A, Kostopoulou K, Boldrini L, Valentini V, et al. Discovering composite lifestyle biomarkers with artificial intelligence from clinical studies to enable smart ehealth and digital therapeutic services. Front Digit Heal. (2021) 3:648190. doi: 10.3389/fdgth.2021.648190
121. DaSilva AW, Huckins JF, Wang R, Wang W, Wagner DD, Campbell AT. Correlates of stress in the college environment uncovered by the application of penalized generalized estimating equations to mobile sensing data. JMIR mHealth uHealth. (2019) 7:e12084. doi: 10.2196/12084
122. MacLeod L, Suruliraj B, Gall D, Bessenyei K, Hamm S, Romkey I, et al. A mobile sensing app to monitor youth mental health: observational pilot study. JMIR mHealth uHealth. (2021) 9:e20638. doi: 10.2196/20638
123. Fulford D, Mote J, Gonzalez R, Abplanalp S, Zhang Y, Luckenbaugh J, et al. Smartphone sensing of social interactions in people with and without schizophrenia. J Psychiatr Res. (2021) 137:613–20. doi: 10.1016/j.jpsychires.2020.11.002
124. Doryab A, Villalba DK, Chikersal P, Dutcher JM, Tumminia M, Liu X, et al. Identifying behavioral phenotypes of loneliness and social isolation with passive sensing: statistical analysis, data mining and machine learning of smartphone and fitbit data. JMIR mHealth uHealth. (2019) 7:e13209. doi: 10.2196/13209
125. Poudyal A, van Heerden A, Hagaman A, Islam C, Thapa A, Maharjan SM, et al. What does social support sound like? Challenges and opportunities for using passive episodic audio collection to assess the social environment. Front Public Heal. (2021) 9:633606. doi: 10.3389/fpubh.2021.633606
126. Irish LA, Kline CE, Gunn HE, Buysse DJ, Hall MH. The role of sleep hygiene in promoting public health: a review of empirical evidence. Sleep Med Rev. (2015) 22:23–36. doi: 10.1016/j.smrv.2014.10.001
127. Jahrami H, BaHammam AS, Bragazzi NL, Saif Z, Faris M, Vitiello M V. Sleep problems during the COVID-19 pandemic by population: a systematic review and meta-analysis. J Clin Sleep Med. (2021) 17:299–313. doi: 10.5664/jcsm.8930
128. Knutson KL, Phelan J, Paskow MJ, Roach A, Whiton K, Langer G, et al. The national sleep foundation's sleep health index. Sleep Heal. (2017) 3:234–40. doi: 10.1016/j.sleh.2017.05.011
129. Blake MJ, Sheeber LB, Youssef GJ, Raniti MB, Allen NB. Systematic review and meta-analysis of adolescent cognitive–behavioral sleep interventions. Clin Child Fam Psychol Rev. (2017) 20:227–49. doi: 10.1007/s10567-017-0234-5
130. Sharma M, Andrade C. Behavioral interventions for insomnia: theory and practice. Indian J Psychiatry. (2012) 54:359. doi: 10.4103/0019-5545.104825
131. Erten-Uyumaz B, Overeem S, Feijs L, Rama MD, Hu J. A digital sleep restriction system for insomnia therapy based on sleep window shift negotiation. In: Proceedings of the 11th PErvasive Technologies Related to Assistive Environments Conference. New York, NY (2018). p. 58–61.
132. Luik AI, van der Zweerde T, van Straten A, Lancee J. Digital delivery of cognitive behavioral therapy for insomnia. Curr Psychiatry Rep. (2019) 21:50. doi: 10.1007/s11920-019-1041-0
133. Milne-Ives M, Lam C, De Cock C, Van Velthoven MH, Meinert E. Mobile apps for health behavior change in physical activity, diet, drug and alcohol use, and mental health: systematic review. JMIR mHealth uHealth. (2020) 8:e17046. doi: 10.2196/17046
134. Wendel S. Designing for Behavior Change: Applying Psychology and Behavioral Economics. 2nd ed. Sebastopol: O'Reilly (2020).
135. Eysenbach G, CONSORT-EHEALTH Group. CONSORT-EHEALTH: improving and standardizing evaluation reports of web-based and mobile health interventions. J Med Internet Res. (2011) 13:e126. doi: 10.2196/jmir.1923
136. Munn Z, Peters MDJ, Stern C, Tufanaru C, McArthur A, Aromataris E. Systematic review or scoping review? Guidance for authors when choosing between a systematic or scoping review approach. BMC Med Res Methodol. (2018) 18:143. doi: 10.1186/s12874-018-0611-x
Keywords: digital health interventions, lifestyle, diet, physical activity, substance use, stress management, social relationship, sleep
Citation: Castro R, Ribeiro-Alves M, Oliveira C, Romero CP, Perazzo H, Simjanoski M, Kapciznki F, Balanzá-Martínez V and De Boni RB (2022) What Are We Measuring When We Evaluate Digital Interventions for Improving Lifestyle? A Scoping Meta-Review. Front. Public Health 9:735624. doi: 10.3389/fpubh.2021.735624
Received: 02 July 2021; Accepted: 29 November 2021;
Published: 03 January 2022.
Edited by:
Brian W. Jack, Boston University, United StatesReviewed by:
Julia Mueller, University of Cambridge, United KingdomPatricia Marie Grace-Farfaglia, Sacred Heart University, United States
Katherine Gergen Barnett, Boston Medical Center, United States
Copyright © 2022 Castro, Ribeiro-Alves, Oliveira, Romero, Perazzo, Simjanoski, Kapciznki, Balanzá-Martínez and De Boni. This is an open-access article distributed under the terms of the Creative Commons Attribution License (CC BY). The use, distribution or reproduction in other forums is permitted, provided the original author(s) and the copyright owner(s) are credited and that the original publication in this journal is cited, in accordance with accepted academic practice. No use, distribution or reproduction is permitted which does not comply with these terms.
*Correspondence: Raquel B. De Boni, raquel.boni@icict.fiocruz.br