- 1Department of Economics, Rovira i Virgili University, Reus, Spain
- 2Facultad de Comunicación y Ciencias Sociales, Universidad San Jorge, Zaragoza, Spain
- 3Research Group on Statistics, Economic Evaluation and Health (GRAEES), Reus, Spain
- 4Research Center on Economics and Sustainability (ECO-SOS), Reus, Spain
Diabetes mellitus (DM) is a serious non-communicable disease (NCD) and relies on the patient being aware of their condition, proactive, and having adequate medical care. European countries healthcare models are aware of the impact of these variables. This study evaluates the impact of online health information seeking behavior (OHISB) during World Diabetes Mellitus Day (WDMD) in European countries from 2014 to 2019 by grouping countries according to the changes in citizens' search behavior, diabetes mellitus prevalence, the existence of National Health Plans (NHP), and their respective healthcare systems. We extracted data from Global Burden of Disease, Google Trends (GT), Public Health European Commission, European Coalition for Diabetes, and the Spanish Ministry of Health. First, we used the broken-line models to analyze significant changes in search trends (GT) in European Union member countries in the 30-day intervals before and after the WDMD (November 14) from 2014 to 2019. Then the results obtained were used in the second phase to group these countries by factor analysis of mixed data (FAMD) using the prevalence of DM, the existence of NHP, and health models in each country. The calculations were processed using R software (gtrendsR, segmented, Factoextra, and FactoMineR). We established changes in search trends before and after WDMD, highlighting unevenness among European countries. However, significant changes were mostly observed among countries with NHP. These changes in search trends, in addition to being significant, were reiterated over time and occurred especially in countries belonging to the Beveridge Model (Portugal, Spain, and Sweden) and with NHPs in place. Greater awareness of diabetes mellitus among the population and continuous improvements in NHP can improve the patients' quality of life, thus impacting in disease management and healthcare expenditure.
Introduction
One of Europe's most serious chronic metabolic disorders is diabetes mellitus (DM), and it is on the rise (1). DM affected approximately 463 million people in 2019 and is predicted to affect 578 million by 2030 and 700 million by 2045. However, it was also observed that its prevalence is higher in urban than rural areas (2). Worldwide, an estimated 19.3% of the population between 65 and 99 years live with this non-communicable disease (NCD) (3). Therefore, to achieve good control of this NCD, regardless of its etiological classification, it is necessary to follow exhaustively the guidelines provided by health professionals to have a good quality of life (4–6). Patient prescriptions should always be adapted to the reality of each case and include guidelines related to hypoglycemic medication and healthy lifestyles ranging from a balanced diet to regular physical exercise (7).
Patients and their environment must be aware of the critical nature of DM. In case of poor management, it can have serious consequences that can affect the body in the short- (e.g., most frequently severe hypoglycemia and hyperglycemia) or medium/long-term (e.g., diabetic nephropathy, diabetic neuropathy, diabetic retinopathy, etc.), which can be very serious and can cause death (8–10).
Health systems are the first lines of care for their citizens' health. Although the structure of these systems differs according to the models to which the European countries being analyzed belong, the underlying goal of them is to provide good care to the population in order to look after their health (11–15). There are numerous frameworks that could be used to categorize countries of the European Union into healthcare system typologies (16–20). One of them [(18), p. PE114], defines “Beveridge” Systems, “Bismarck” Systems and Mixed Systems. Amongst other differences between these models, the Bismarck Model finances healthcare through compulsory social security contributions by employees and employers, while the Beveridge model was financed through public taxes. Further, while the Beveridge model is referred to as National Health System, those under the Bismarck model is also referred as Social Health Insurance System. The Mixed model is a different categorization. It's also referred as the Private Health Insurance System.
According to Gaeta et al. [(18), p. E114], these are the European countries following each healthcare system: Bismarck model, Belgium, Czech Republic, Estonia, France, Germany, Hungary, Lithuania, Luxembourg, Netherlands, Poland, Romania, Slovakia, and Slovenia; Beveridge model, Cyprus, Denmark, Finland, Ireland, Italy, Latvia, Malta, Portugal, Spain, Sweden, and United Kingdom; Mixed model: Austria, Bulgaria, Croatia and Greece.
The governments of different countries, public and private organizations, researchers, and healthcare providers are aware of the concerning rise in the number of cases of DM and the related work carried out jointly by the different international organizations. Therefore, they have promoted public policies that have boosted prevention campaigns aimed at the population, encouraging diabetes education, early detection, and promoting healthy lifestyles. These actions aim to raise public awareness of this condition and enable patients to adequately manage the acute symptoms of their chronic condition (1, 2, 21, 22). Additionally, the National Health Plans (NHP) have been established recently, including initiatives targeted toward DM (23, 24). According to a past study (23), the European countries of Austria, Bulgaria, Croatia, Czechia, Denmark, Finland, Greece, Hungary, Ireland, Italy, Netherlands, Poland, Portugal, Romania, Slovakia, Slovenia, Spain, and Sweden would have implemented NHPs. On the other hand, Belgium, Cyprus, Estonia, France, Germany, Latvia, Lithuania, Luxembourg, Malta, and United Kingdom would not have implemented NHPs or would be in the process of implementation.
Patients can use online resources and platforms to promote their well-being and improve that of those around them (25–28). For example, they can use online resources when suffering from a disease, its complications, having an interest in taking care of their health, or to simply be informed and seek real-time answers (29, 30). It should be noted that healthcare providers and official sources can bring the most accurate information as they know the pathology concerned (31). However, suppose users choose to search through the current online resources offered through the Internet (as a public information provider). In that case, such interests of citizens can be monitored through search engine data. Google (32) is one of the relevant search engines, being the most widely used worldwide and having a large market share (33). In this regard, Google Trends (GT) (34) is becoming a tool that is raising special interest for probable forecasts and in understanding the interests of the population (35). Data provided by GT can be used as a “surrogate” of online health information seeking behavior (OHISB) and give useful information on search behaviors related to health or healthy habits (33, 36–38). Some research examines the association between data provided by internet sources and public health policies (39).
Within this framework and as evidenced in previous research papers (40–45), global days dedicated to raising public awareness of specific health issues can lead to Internet search by citizens and provide valuable information on search behaviors. In particular, under the initiative of the International Diabetes Federation and with the support of World Health Organization, November 14 was established as the world DM day (WDMD) (46). This initiative was an attempt to promote information related to the treatment, prevention, and timely diagnosis, as well as raise awareness of DM among the population. It should be emphasized that DM evokes serious impacts at both the human and economic dimensions. Therefore, based on this information and considering the impact of DM on the current healthcare systems, this original research analyzes the prevalence of this disease in Europe. We used the European healthcare system model (Bismarck, Beveridge and mixed models) of countries to monitor the interest raised in the European population on the WDMD and 30 days before and after it, using two different methodologies to reach the output.
This study evaluates the impact of World Diabetes Mellitus Day (WDMD) in European countries from 2014 to 2019, grouping countries according to the changes in the citizens' search behaviors by the prevalence of the disease, the existence of NHP, and their respective healthcare systems. The period of this study began in 2014, when a prior study analyzing the NHP in Europe (23) was published. The period under analysis extends to 2019, namely, before pre-pandemic trends.
This research paper is structured as follows: Section 2 (materials and methods) explains the steps to set up the Database (DB) as well as the methodology and software used. Section 3 (results) shows the results of the research, and Section 4 (discussion) discusses the relevant findings of the present work.
Materials and methods
The research methodology is structured in the following steps: DB approach and sources consulted, and Methodology and software used.
First step: Database approach and sources consulted
Prevalence of DM
Using the data provided by the Global Burden of Disease Collaborative Network-Global Burden of Disease Study (47), it was feasible to set up the database based on the information related to the prevalence of DM.
Monitoring of the population's interest
The Internet is an excellent environment to analyze the users' interests 30 days before and after November 14 (WDMD). The tool used in this case is GT on the European scale, used as an index of the population's interest related to DM. The information set by Relative Search Volumes (RSV) for the search term “Diabetes Mellitus” shows the proportion of queries for this centralized term in a specific time and region. Its standardization structured from 0 to 100 has been linked to the highest proportion of the searched term in each set year (34).
Official sources
The characteristics of healthcare systems in European Union countries have been analyzed based on different official sources from several organizations. The data have been retrieved from the “Public Health European Commission” (48), “Spanish Ministry of Health” (49), and “Health Care Systems in EU-28, National Health Care Service and Social Security System” (15).
European Coalition for Diabetes (EURADIA, FEND, International Diabetes Federation, and PCDE)
Information related to NHP existing in European countries has been compiled (23).
Second step: Methodology and software
Methodology
Two methods were combined to achieve the required outputs of this novel research. First, to process the previously generated databases related to monitoring and search trends, it was necessary to use the broken-line models (BLM). With them, it was possible to track the population's interest during the 30 days before and after the WDMD (50–54). Once this output was achieved and processed to obtain the monitoring data, multivariate analysis was used (55–57). In particular, the factorial analysis of mixed data (FAMD) was used to classify the quantitative and qualitative variables of this research. The combination of these methods provided a major advantage in processing, visualizing, and analyzing the data set.
Broken-line models
Regression analysis analyzes dependent variables as a linear function of explanatory variables. In this framework, the model's response is presented as a linear function of the explanatory variables (58). However, the relationship between the response and some explanatory variables may not be linear. BLM are known to be segmented, and are represented using two or more straight lines; these lines are then connected at unknown values between the response and explanatory variables (53, 54). Here, it can be observed how the effect on the expected response of the breakpoints changes sharply. When this happens, it is necessary to use additional, non-standard optimization techniques to estimate the models because, in the estimation of the parameters of the cut-off points, the log-likelihood differs by segments, and the conditions established in the classical models are no longer fulfilled (50–54). According to Muggeo (52, 53) a segmented relationship is modeled when μ = E[Y] and variable Z is i = 1,2,3… n then
Where (zi − ψ)+ = (zi − ψ) × I(zi > ψ) and I(·)
When a linear predictor is needed,
At each iteration, the breakpoint value has to be updated,
because a standard linear model must be fitted.
The Delta method for is obtained due to the standard error of when the algorithm converges.
Davies test (DT)
When breakpoint does not exist, the test for ψ is,
and there is no difference-in-slopes parameter. It is zero. β2 depends on a nuisance parameter, and ψ fades under H0. The DT (50) is useful for performing this hypothesis test. It must be noted that statistical tests such as Wald are not satisfied, and p-values are underestimated. Here, it must be pointed out that while DT is not suitable for selecting the number of the joinpoints, it is good for testing a breakpoint.
DT is framed in the range of Z when given the K fixed ordered values of breakpoints ψ1 < ψ2 < …ψk.
When the standard normal distribution ψk is fixed, there is a relevant K of the test statistic {S(ψk)}k = 1, …, K.
The one-side hypothesis test is,
and the respective alternative is,
Being that the maximum of the K statistic M = max{S(ψk)}k, Φ(·) is a function of standard normal,
{S(ψk)}k is total variation.
Factorial analysis of mixed data
As aforementioned, the database used included qualitative and quantitative variables. The FAMD technique was employed because it allows both types of variables to be used simultaneously, generating a space of smaller dimensions. The quantitative variables were normalized, and the qualitative variables were treated in a normalized data table to balance all the variables under study. Principal component analysis and multiple correspondence analysis (59, 60) were combined to visualize the differences and the similarities (distances) of the analyzed elements, thereby helping us find the correlation of the continuous variables.
According to Pagès [(60), p. 71], there are two types of relationships:
a) From RK toward R1.
Case 1) A quantitative variable:
Case 2) A category kq of variable q: Fs(kq) is the coordinate of the center of gravity of individuals with category (kq):
b) From RI toward RK
It is expressed as follows:
Software used
We used open-source software libraries to obtain the results based on the abovementioned methodologies (53, 56, 59–62); specifically, we used gtrendsR and Segmented package for the BLM and FactoMineR and FactoExtra package for FAMD.
Results
We obtained the results needed to develop this study with the DB created and previously mentioned techniques. Table 1 shows the variables related to the prevalence of DM and RSV from 2014–2019 for the European countries studied.
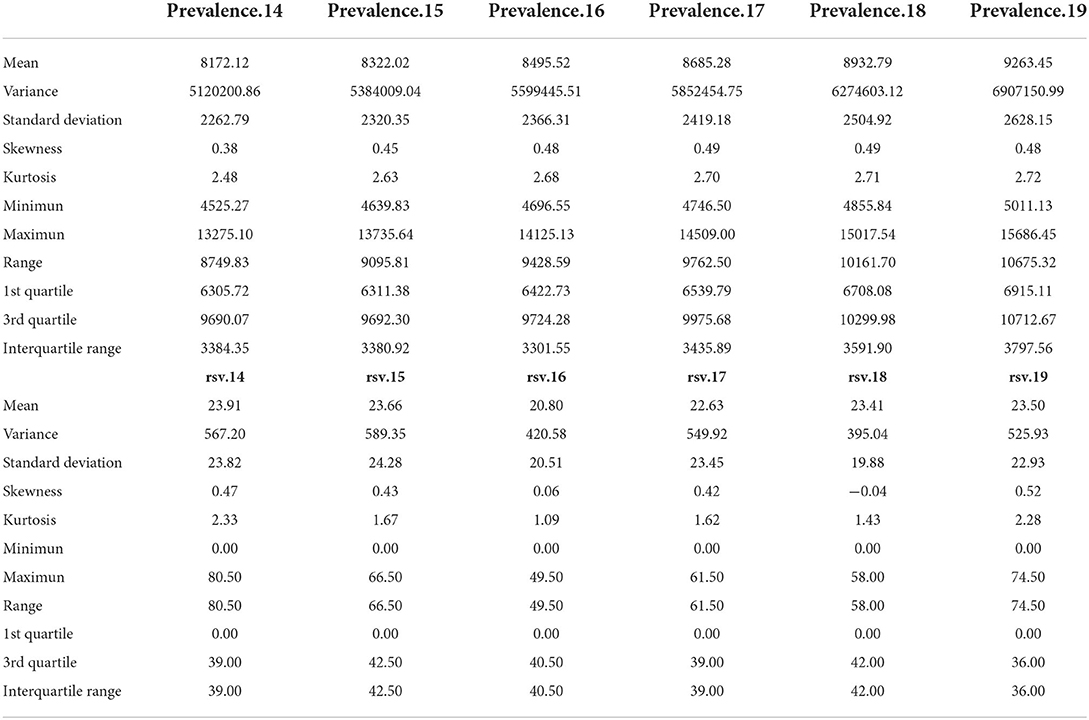
Table 1. Descriptive statistics for diabetes mellitus prevalence and relative search volumes from 2014–2019 for European countries.
The results of BLM applied in the different European countries are shown in Annex.
The analysis of monitoring achieved through the BLM during the 30 days before and after WDMD was related to the change in the search trend on the designated day in the different European countries during the analyzed period. This variable is denoted as “sig” (Figure 1).
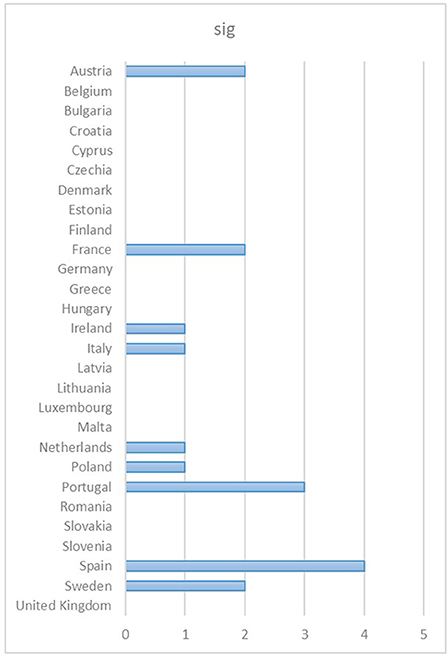
Figure 1. Changes in search trends on the designated day in the different European countries. Source: Compiled by the authors with data extracted from GT (2022).
The multivariate analysis (Figure 2) showed that dimension 1 and 2 of the sedimentation graph accounted for 73.15% of the accumulated variance, whereas dimensions 3 and 4 accounted for 9.13 and 6.79% of the variance, respectively.
Figure 3 shows the four dimensions grouping the study variables. Dimension 1 shows the prevalence of DM. Dimension 2 shows the RSV related to DM. Dimension 3 shows the European countries analyzed that have a NHP (DM plan & Healthcare models & sig). Please, be noted that we coded this variable as “DM plan” Finally, Dimension 4 shows the monitoring analysis achieved when the BLM methodology was used 30 days before and after WDMD, getting the “sig” variable (explained above).
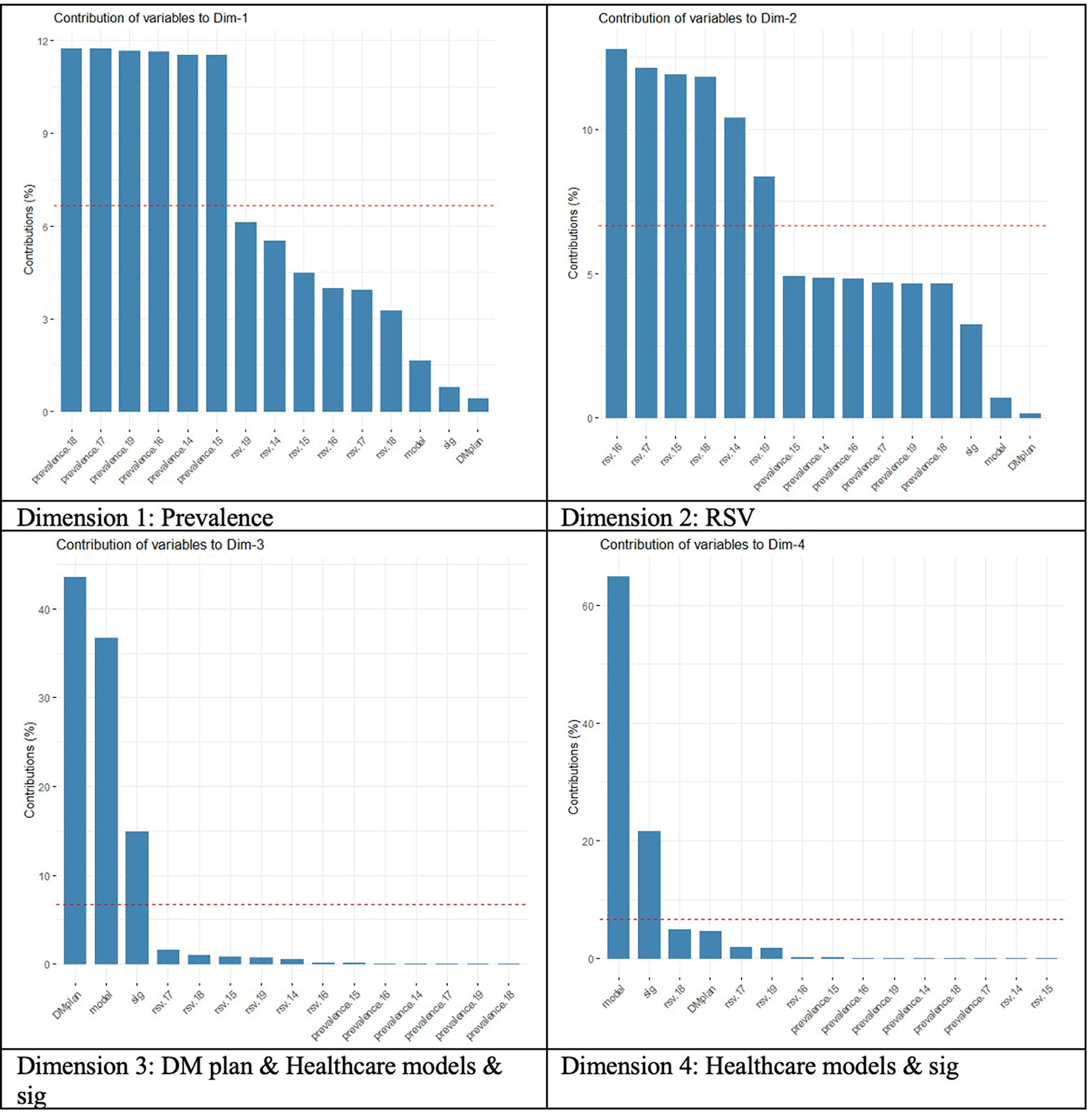
Figure 3. Contribution of variables to the principal dimensions (“Prevalence,” “RSV diabetes,” “DM day,” “DM plan, Healthcare models & Sig” and “Healthcare models & Sig”).
Likewise, the correlation circle (Figures 4, 5) shows the relationship between quantitative variables and their contribution to dimensions 1 and 2. There is no correlation between RSV and prevalence of DM, but there are high contributions to dimensions 1 and 2, respectively.
The correlation circle in Figure 6 shows the relationship between the quantitative variables and their contribution to the factor map in dimensions 1 and 3. Prevalence of DM was unrelated to searches in the WDMD.
The correlation circle in Figure 7 shows the relationship between the variable's contribution to the factor map in dimensions 2 and 3.
Figures 8, 9 show the distribution of the countries between dimension 1 and dimension 2. It can be noted that countries with a NHP and with high searches for the term “diabetes mellitus” had a high and low prevalence of this disease.
Figure 10 shows the distribution of the countries in the factor map represented through dimension 1 and 3. Among countries with NHPs and higher prevalence of DM, many changes in internet search trends happened on WDMD.
The Figure 11 factor map is generated from dimension 2 and 3. Among countries with NHPs, higher searches for RSV imply a higher number of times of noticeable changes in internet searches on DM during the WDMD.
Consequently, changes in the search trend before and after the WDMD were uneven among European countries during the period analyzed. The most important changes were mostly observed among countries with NHPs. These noticeable changes in the search were reiterated over time and occurred especially in the countries belonging to the Beveridge model (Portugal, Sweden and Spain).
Figure 12 shows the classification obtained through the FAMD method of the European countries analyzed. The three clusters show the following coincidences:
Cluster 1 shows a comparatively low prevalence of DM, low RSV related to DM, half of the country samples with no NHP, and no considerable changes in internet search trends during the WDMD.
Cluster 2 shows, for the most part, a low prevalence of DM, a high RSV related to DM, countries with NHPs, and unevenly noticeable changes in internet search trends during the WDMD.
Cluster 3 shows a high prevalence of DM, a high RSV related to DM, and, for the most part, countries with NHPs. Rarely, they present significant changes in internet search trends during the WDMD.
Table 2 and Figure 13 shows, for each European country, the number of times that the search changes were significant and their respective clusters, the healthcare systems, and whether they have NHPs.
Therefore, the combination of the results using two methods (BLM and FAMD) leads to the following patterns: a high RSV related to DM and higher prevalence of DM was noted in countries with NHPs, which showed a greater number of considerable changes in search trends during the WDMD. These changes in OHISB were reiterated over time and happened mainly in countries with NHPs and belonging to the Beveridge Model (Portugal, Spain, and Sweden). Meanwhile, most countries belonging to the Bismarck model, with NHPs, and a high frequency of searches for DM showed a low prevalence of DM compared to the others. Therefore, they have not presented noticeable changes in search trends during the WDMD.
Discussion
Our research work demonstrates the potential of analyzing user activity collected through Google and GT. Our results concur with those of prior research (33, 36, 40, 43), and it emphasizes the importance of OHISB evaluation in current days for raising public awareness of specific health issues (37, 40–45). Furthermore, our study is novel as it evaluates the impact of OHISB on the WDMD considering selected European countries and depending on whether they have NPHs or not, their healthcare system model, and the prevalence of DM among the population. We have managed to extract the respective patterns framed between 2014 and 2019. We emphasize that the information related to the NHPs of each European country, used to classify them, was extracted from the (23), as shown in section 1 introduction of this study. Two groups were created: whether they were implemented or not (countries that were in the process of establishing the NPHs were classified as “not implemented” as they were in the process of doing so). Please, note that numerous frameworks could be used to categorize countries of the European Union into health system typologies. According to Gaeta et al. [(18), p. E114], we categorized countries into Social Health Insurance System (“Bismarck” model), National health services (“Beveridge” model), and mixed models. This research focused on the European population with access to the Internet and using Google as a search engine, so the limitation of the search data was framed in GT. Therefore, we agree with one prior study (33, 38) which argues that GT is a useful tool for research. These components and the methods presented in this study (BLM and FAMD) enriched the final output. This novel work shows how the combination of the two used methods can provide an advantage in processing, visualizing, and analyzing the data set of interest. For this study, proper statistical methods were used to process data. This DB analyzed variables related to the prevalence of DM, the NHPs, the healthcare systems, and OHISB during the WDMD. It highlighted how European countries were grouped according to the study's variables. The main contribution is the pattern observed, showing that countries with a matching NHP, for the most part, had populations with a greater OHISB related to DM. Additionally, the presence of a high and medium prevalence of DM in these countries coincided with a greater number of changes in search behavior during the WDMD. These contributions can be useful to public bodies to acquire more knowledge and public interest in DM.
Future research might focus on how these combined methods and web-based tools could be used to raise the population's awareness of DM. It can help improve decision-making of public stakeholders regarding the establishment of relevant actions for improving the quality of life of the population interested in this NCD and help those with the disease to deal with it. To this end, it would be worthwhile to focus on more patterns of variables related to DM within the context of socio-economic determinants of health using the methods shown in this research.
Data availability statement
Publicly available datasets were analyzed in this study. This data can be found here: Global Burden of Disease Collaborative Network. Global Burden of Disease Study 2019 (GBD 2019) Results. Seattle, United States: Institute for Health Metrics and Evaluation (IHME), 2020. https://vizhub.healthdata.org/gbd-results. Google Trends (2022): https://trends.google.es. European Coalition for Diabetes (IDF Europe, FEND, PCDE, and EURADIA. https://www.idf.org/our-network/regions-members/europe/publications-and-resources/56-diabetes-in-europe-policy-puzzle.html.
Author contributions
Conceptualization, methodology, software, formal analysis, investigation, and writing—original draft preparation: IBF. Validation and supervision: IBF, FCV, MCL, and MJPL. Resources and data curation: IBF and FCV. Writing—review and editing: IBF, FCV, and MCL. Project administration: MCL and IBF. All authors have read and agreed to the published version of the manuscript.
Acknowledgments
We would like to thank Editage (www.editage.com) for English language editing.
Conflict of interest
The authors declare that the research was conducted in the absence of any commercial or financial relationships that could be construed as a potential conflict of interest.
Publisher's note
All claims expressed in this article are solely those of the authors and do not necessarily represent those of their affiliated organizations, or those of the publisher, the editors and the reviewers. Any product that may be evaluated in this article, or claim that may be made by its manufacturer, is not guaranteed or endorsed by the publisher.
Supplementary material
The Supplementary Material for this article can be found online at: https://www.frontiersin.org/articles/10.3389/fpubh.2022.1023404/full#supplementary-material
References
1. Word Health Organization. Noncommunicable diseases progress monitor (2020). Geneva: 2022. Licence: CC BY-NC-SA 3.0 IGO. Available online at: https://www.who.int/publications/i/item/9789240000490 (accessed June 06, 2022).
2. Saeedi P, Petersohn I, Salpea P, Malanda B, Karuranga S, Unwin N, et al. Global and regional diabetes prevalence estimates for 2019 and projections for 2030 and 2045: results from the international diabetes federation diabetes Atlas, 9th edition. Diabetes research and clinical practice. (2019) 157:107843. doi: 10.1016/j.diabres.2019.107843
3. Sinclair A, Saeedi P, Kaundal A, Karuranga S, Malanda B, Williams R. Diabetes and global ageing among 65–99-year-old adults: findings from the international diabetes federation diabetes Atlas, 9th edition. Diabetes Res Clin Pract. (2020) 162:108078–108078. doi: 10.1016/j.diabres.2020.108078
4. American Diabetes Association; 2. Classification and Diagnosis of Diabetes: Standards of Medical Care in Diabetes–2021. Diabetes Care. (2021) 44 (Supplement_1): S15–S33. doi: 10.2337/dc21-S002
5. Addendum. 2. Classification and Diagnosis of Diabetes: Standards of Medical Care in Diabetes–2021. Diabetes Care. (2021) 44(Suppl. 1):S15–S33. doi: 10.2337/dc21-ad09
6. Rathmann W. Screening und Epidemiologie des diabetes. Diabetologe. (2021) 17:494–500. doi: 10.1007/s11428-021-00774-4
7. Jones A, Vallis M, Cooke D, Pouwer F. Working together to promote diabetes control: a practical guide for diabetes health care providers in establishing a working alliance to achieve self-management support. J Diabetes Res. (2015) 2016:2830910–6. doi: 10.1155/2016/2830910
8. Glasheen WP, Renda A, Dong Y. Diabetes complications severity index (DCSI) – update and ICD-10 translation. J Diabetes Complications. (2017) 31:1007–13. doi: 10.1016/j.jdiacomp.2017.02.018
9. Swanson V, Maltinsky W. Motivational and behavior change approaches for improving diabetes management. Practical Diab Int. (2019) 36:121–5. doi: 10.1002/pdi.2229
10. Truong TH, Nguyen TT, Armor BL, Farley JR. Errors in the administration technique of insulin pen devices: a result of insufficient education. Diabetes therapy. (2017) 8:221–6. doi: 10.1007/s13300-017-0242-y
11. Balabanova D, Mills A, Conteh L, Akkazieva B, Banteyerga H, Dash U, et al. Good health at low cost 25 years on: lessons for the future of health systems strengthening. The Lancet (British edition). (2013) 381:2118–33. doi: 10.1016/S0140-6736(12)62000-5
12. Mills A. Health care systems in low- and middle-income countries. N Engl J Med. (2014) 370:552–7. doi: 10.1056/NEJMra1110897
13. Montagu D. The provision of private healthcare services in European countries: recent data and lessons for universal health coverage in other settings. Front Public Health. (2021) 9:636750–636750. doi: 10.3389/fpubh.2021.636750
14. Or Z, Cases C, Lisac M, Vrangbæk K, Winblad U, Bevan G. Are health problems systemic? Politics of access and choice under Beveridge and Bismarck systems. Health Econ Policy Law. (2010) 5:269–93. doi: 10.1017/S1744133110000034
15. Health Care Systems in EU-28. National Health Care Service and Social Security System (2021). Ministerio de Sanidad, Consumo y Bienestar Social. Secretaría General de Sanidad y Consumo. Available online at: http://www.sanidad-ue.es/en/sns-sss (accessed October 1, 2021).
16. Böhm K, Schmid A, Götze R, Landwehr C, Rothgang H. Five types of OECD healthcare systems: empirical results of a deductive classification. Health Policy. (2013) 113:258–69. doi: 10.1016/j.healthpol.2013.09.003
17. Ferreira PL, Tavares AI, Quintal C, Santana P, EU. health systems classification: a new proposal from EURO-healthy. BMC Health Serv Res. (2018) 18:511–511. doi: 10.1186/s12913-018-3323-3
18. Gaeta M, Campanella F, Capasso L, Schifino GM, Gentile L, Banfi G, et al. An overview of different health indicators used in the European health systems. J Prevent Med Hygiene. (2017) 58:114–20.
19. Hagenaars LL, Klazinga NS, Mueller M, Morgan DJ, Jeurissen PPT. How and why do countries differ in their governance and financing-related administrative expenditure in health care?: An analysis of OECD countries by health care system typology. Int J hHealth Plann Manag. (2018) 33:e263–78. doi: 10.1002/hpm.2458
20. Reibling N, Ariaans M, Wendt C. Worlds of healthcare: a healthcare system typology of OECD countries. Health Policy. (2019) 123:611–20. doi: 10.1016/j.healthpol.2019.05.001
21. Cho N, Shaw J, Karuranga S, Huang Y, da Rocha Fernandes J, Ohlrogge A, et al. IDF Diabetes atlas: global estimates of diabetes prevalence for 2017 and projections for 2045. Diabetes Res Clin Pract. (2018) 138:271–81. doi: 10.1016/j.diabres.2018.02.023
22. Dolley S. Big Data's Role in Precision Public Health. Front Public Health. (2018) 6:68. doi: 10.3389/fpubh.2018.00068
23. European Coalition for Diabetes. (2014). Diabetes in Europe policy puzzle: The state we are in. Available online at: https://www.idf.org/our-network/regions-members/europe/publications-and-resources/56-diabetes-in-europe-policy-puzzle.html (accessed October 1, 2021).
24. Müller G, Weser G, Schwarz PEH. The European perspective of diabetes prevention: The need for individualization of diabetes prevention. J Endocrinol Invest. (2014) 36:352–7. doi: 10.1007/BF03347104
25. Jamal A, Khan SA, AlHumud A, Al-Duhyyim A, Alrashed M, Bin Shabr F, et al. Association of online health information-seeking behavior and self-care activities among type 2 diabetic patients in Saudi Arabia. J Med Internet Res. (2015) 17:e196–e196. doi: 10.2196/jmir.4312
26. Longo DR, Schubert SL, Wright BA, LeMaster J, Williams CD, Clore JN. Health information seeking, receipt, and use in diabetes self-management. Ann Fam Med. (2010) 8:334–40. doi: 10.1370/afm.1115
27. Nuti SV, Wayda B, Ranasinghe I, Wang S, Dreyer RP, Chen SI, et al. The use of google trends in health care research: a systematic review. PLoS One. (2014) 9:e109583–e109583. doi: 10.1371/journal.pone.0109583
28. Thompson D, Callender C, Gonynor C, Cullen KW, Redondo MJ, Butler A, et al. Using relational agents to promote family communication around type 1 diabetes self-management in the diabetes family teamwork online intervention: longitudinal pilot study. J Med Internet Res. (2019) 21:e15318–e15318. doi: 10.2196/15318
29. Jellison SS, Bibens M, Checketts J, Vassar M. Using google trends to assess global public interest in osteoarthritis. Rheumatol Int. (2018) 38:2133–6. doi: 10.1007/s00296-018-4158-2
30. Mamykina L, Smaldone AM, Bakken SR. Adopting the sensemaking perspective for chronic disease self-management. J Biomed Inform. (2015) 56:406–17. doi: 10.1016/j.jbi.2015.06.006
31. Zarocostas J. How to fight an infodemic. The Lancet. (2020) 395:676–676. doi: 10.1016/S0140-6736(20)30461-X
32. Google (2022). Available online at: https://www.google.es/ (accessed December 1, 2021).
33. Cacciamani GE. Dell'Oglio P, Cocci A, Russo GI, De Castro Abreu A, Gill IS, et al. Asking “Dr Google” for a second opinion: the devil is in the details. Eu Urol Focus. (2021) 7:479–81. doi: 10.1016/j.euf.2019.10.011
34. Google Trends (2022). Available online at: https://trends.google.es (accessed December 1, 2021).
35. Choi H.,Varian H. Predicting the present with google trends. Economic Record. (2012) 88:2–9. doi: 10.1111/j.1475-4932.2012.00809.x
36. Kushwaha S, Khanna P, Jain R, Srivastava R. Determining the nutritional immunity information-seeking behavior during the COVID-19 pandemic in India: a google trends data analysis. Public Health Nutr. (2021) 24:5338–49. doi: 10.1017/S1368980021003232
37. Nishimura Y, Hagiya H, Keitoku K, Koyama T, Otsuka F. Impact of the world hand hygiene and global handwashing days on public awareness between 2016 and 2020: Google trends analysis. Am J Infect Control. (2022) 50:141–7. doi: 10.1016/j.ajic.2021.08.033
38. Rovetta A. Reliability of google trends: analysis of the limits and potential of web infoveillance during COVID-19 pandemic and for future research. Front Res Met Ana. (2021) 6:670226–670226. doi: 10.3389/frma.2021.670226
39. Lan R, Catherine JH, Chossegros C, Campana F, Vergnes JN, Had-Saïd M. Temporal association between the introduction of public health programs and interest in oral cancers on the internet in the European Union. Oral Oncol. (2021) 119:105250–105250. doi: 10.1016/j.oraloncology.2021.105250
40. Ajbar AA, Shepherd TATA, Robinson MM, Mallen CDCD, Prior JAJA. Using Google Trends to assess the impact of Global Public Health Days on online health information-seeking behavior in Arabian Peninsula. J Egyp Public Health Assoc. (2021) 96:63. doi: 10.1186/s42506-020-00063-w
41. Havelka EM, Mallen CD, Shepherd TA. Using Google Trends to assess the impact of global public health days on online health information seeking behavior in Central and South America. J Glob Health. (2020) 10:010403–010403. doi: 10.7189/jogh.10.010403
42. Lang-Illievich K, Lang J, Szilagyi IS, Ullrich T, Wagner-Skacel J, Repiská G, et al. The internet's interest in autism peaks in April: a google trends analysis. J Autism Develop Disord. (2022) 5:5614 doi: 10.1007/s10803-022-05614-y
43. Sebopelo LA, Bourcier AJ Dada OE, Adegboyega G, Nteranya DS, Kanmounye US. Retrospective review of google trends to gauge the popularity of global surgery worldwide: a cross-sectional study. Annals Med Surg. (2021) 71:102950–102950. doi: 10.1016/j.amsu.2021.102950
44. Silangcruz K, Nishimura Y, Czech T, Kimura N, Hagiya H, Koyama T, Otsuka F. Impact of the World inflammatory bowel disease day and Crohn's and colitis awareness week on population interest between 2016 and 2020: google trends analysis. JMIR Infodemiol. (2021) 1:e32856. doi: 10.2196/32856
45. Vernon E, Gottesman Z, Warren R. The value of health awareness days, weeks and months: a systematic review. Soc Sci Med. (1982) 268:113553. doi: 10.1016/j.socscimed.2020.113553
46. United Nations. Digital Library (2007): Resolution adopted by the General Assembly on 20 December 2006. Available online at: https://digitallibrary.un.org/record/589515?ln=en. (accessed October 1, 2021).
47. Global Burden of Disease Collaborative Network. Global Burden of Disease Study 2019 (GBD 2019) Results. Seattle, United States: Institute for Health Metrics and Evaluation (IHME), (2020). Available online at:: https://vizhub.healthdata.org/gbd-results (accessed July 18, 2021).
48. Public Health. European Comission (2021). Available online at: https://ec.europa.eu/health/index_en (accessed December 1, 2021).
49. Spanish Ministry of Health (2022). Available online at: https://www.sanidad.gob.es (accessed March 03, 2022).
50. Davies RB. Hypothesis testing when a nuisance parameter is present only under the alternative. Biometrika. (1987) 74:33–43. doi: 10.1093/biomet/74.1.33
51. Davies RB. Hypothesis testing when a nuisance parameter is present only under the alternative: linear model case. Biometrika. (2002) 89:484–9. doi: 10.1093/biomet/89.2.484
52. Muggeo VMR. Estimating regression models with unknown break-points. Stat Med. (2003) 22:3055–71. doi: 10.1002/sim.1545
53. Muggeo VM. Segmented: an R package to fit regression models with broken-line relationships. R News. (2008) 8:20–5.
54. Muggeo VMR. Interval estimation for the breakpoint in segmented regression: a smoothed score-based approach. Aust N Z J Stat. (2017) 59:311–22. doi: 10.1111/anzs.12200
55. Härdle WK, Simar L. Applied Multivariate Statistical Analysis. 5th ed. Cham: Springer International Publishing AG (2019). doi: 10.1007/978-3-030-26006-4
56. Lê S, Josse J, Husson F. FactoMineR : an R package for multivariate analysis. J Stat Softw. (2008) 25:1–18. doi: 10.18637/jss.v025.i01
57. Zelterman D. Applied Multivariate Statistics With R. Basel: Springer International Publishing (2015).
58. Atkinson AC, Riani M. Robust Diagnostic Regression Analysis. 2000. New York: Springer (2000). Print. Springer Texts in Statistics. doi: 10.1007/978-1-4612-1160-0
59. Pagès, J. Analyse factorielle de données mixtes. Revue de Statistique Appliquée. (2004) 52:93–111.
60. Pagès J. Multiple factor analysis by example using R. CRC Press. (2015) 5:7700. doi: 10.1201/b17700
61. Kassambara A. Practical guide to principal component methods in R: PCA, M (CA), FAMD, MFA, HCPC, factoextra. Sthda. (2017) 2:108–40.
62. R Development Core Team (2022). R: A Language and Environment for Statistical Computing. Vienna, Austria: R Foundation for Statistical Computing. Available online at: https://www.r-project.org
Keywords: diabetes mellitus, broken-line models, factor analysis of mixed data, Google trends, healthcare system
Citation: Bosch-Frigola I, Coca-Villalba F, Pérez-Lacasta MJ and Carles-Lavila M (2022) European national health plans and the monitoring of online searches for information on diabetes mellitus in different European healthcare systems. Front. Public Health 10:1023404. doi: 10.3389/fpubh.2022.1023404
Received: 19 August 2022; Accepted: 09 September 2022;
Published: 24 November 2022.
Edited by:
María Del Carmen Valls Martínez, University of Almeria, SpainReviewed by:
José Santos-Jaén, University of Murcia, SpainGema Fernández-Aviés, University of Castilla-La Mancha, Spain
Copyright © 2022 Bosch-Frigola, Coca-Villalba, Pérez-Lacasta and Carles-Lavila. This is an open-access article distributed under the terms of the Creative Commons Attribution License (CC BY). The use, distribution or reproduction in other forums is permitted, provided the original author(s) and the copyright owner(s) are credited and that the original publication in this journal is cited, in accordance with accepted academic practice. No use, distribution or reproduction is permitted which does not comply with these terms.
*Correspondence: Irene Bosch-Frigola, aWJvc2NoQHVzai5lcw==