- 1Department of Economics, Rovira i Virgili University, Reus, Spain
- 2Facultad de Comunicación y Ciencias Sociales, Universidad San Jorge, Zaragoza, Spain
- 3Research Group on Statistics, Economic Evaluation and Health (GRAEES), Reus, Spain
- 4Research Center on Economics and Sustainability (ECO-SOS), Reus, Spain
Inequalities in the equipment and use of information and communications technology (ICT) in Spanish households can lead to users being unable to access certain information or to carry out certain procedures. Accessibility to ICT is considered a social determinant of health (SDOH) because it can generate inequalities in access to information and in managing access to health services. In the face of a chronic illness such as diabetes mellitus (DM)—for which a comprehensive approach is complex and its complications have a direct impact on current healthcare systems—all the resources that patients may have are welcome. We aimed to analyze hospitalizations and amputations as direct consequences of DM among the autonomous communities of Spain (ACS) in 2019, along with socioeconomic factors related to health, including inequalities in access to ICT between territories, as well as citizens' interest in online information searches about DM. We used different databases such as that of the Ministerio de Sanidad (Spain's health ministry), Ministerio de Asuntos Económicos y transformación (Ministry of Economic Affairs and Digital Transformation), Google Trends (GT), and the Instituto Nacional de Estadística (Spain's national institute of statistics). We examined the data with R software. We employed a geolocation approach and performed multivariate analysis (specifically factor analysis of mixed data [FAMD]) to evaluate the aggregate interest in health information related to DM in different regions of Spain grounded in online search behavior. The use of FAMD allowed us to adjust the techniques of principal component analysis (PCA) and multiple correspondence analysis (MCA) to detect differences between the direct consequences of DM, citizen's interest in this non-communicable disease, and socioeconomic factors and inequalities in access to ICT in aggregate form between the country's different ACS. The results show how SDOH, such as poverty and education level, are related to the ACS with the highest number of homes that cite the cost of connection or equipment as the reason for not having ICT at home. These regions also have a greater number of hospitalizations due to DM. Given that in Spain, there are certain differences in accessibility in terms of the cost to households, in the case of DM, we take this issue into account from the standpoint of an integral approach by health policies.
Introduction
The equipment and use of information and communications technology as a social determinant of health
The social determinants of health (SDOH) are essential for achieving good public health. They are framed in an environment where political, social, and economic forces interact and where people are born, grow, live, work, and age. Surveillance in the field of public health is therefore crucial. Surveillance encompasses not only the systematic, continuous collection of data on the population's health; information analysis and interpretation are also critical to plan, implement, and evaluate public health actions (1–4). According to (5), both digital literacy and Internet connectivity can be understood as “super [SDOH]” because they include all other social determinants related to health. In this regard, there are remarkable inequalities in the world. According to (6), between 2005 and 2019, the number of internet users grew by 10% on average. In 2019, the global penetration rate increased over 53%, and Europe had the highest internet usage rates. In 2019, the vast majority of the world's population lived within reach of a mobile network and, in developed countries, about 87% of the population used the internet.
Organisation for Economic Co-operation and Development OECD, (7) stresses that access to and use of information and communications technology (ICT) translates into real social benefits. In OECD countries, the population that has come to use the internet has grown by 30 percentage points over the last decade. In most member states, almost all young people between the ages of 16 and 24 use the internet on a daily basis. However, among individuals between 55 and 74 years old, the median stands at 55%, with very considerable differences depending on the country analyzed (8).
The use of ICT by all people—including fundamental human rights such as privacy and ensuring the ethical use of data—must prevail. Although there may be a risk of fostering inequality and prejudice between groups who have access to data and know how to use them, and those who do not, the discrepancies that may arise and that could end up hindering access to ICT must be avoided (9). Although exclusion related to the information society can take place passively (due to social and cultural environmental conditions) or actively (for reasons external to the individual, whether governmental or political), some authors consider that although access to digital resources is more costly for people without incomes, when they have access to ICT and are going to use it, they may be more interested in it. Hence, not having access to ICT does not imply social exclusion, but not having ICT means that the situation of exclusion is exacerbated for the population without access to it. Given this scenario, it is vital to highlight the role of the “digital divide” and to distinguish between the impact of “access” and “use” of ICT (10, 11).
Health care services and digital health technologies
The emergence of electronic health (eHealth) marks a breakthrough as it represents support for citizens' health related to (among other aspects) healthcare services, education, and surveillance (12). Currently, eHealth is implemented in different governments as well as public administrations, and embodies one more step toward so-called electronic government, since the use of eHealth, in some countries, denotes support for universal health coverage (13–15).
While digital health technologies aim to promote the efficiency of healthcare delivery by offering better medical services to citizens (both in the public and private sectors), their application might not always be homogeneous (16, 17).
Health informatics interventions are designed to improve the quality of health and the safety of healthcare, even though they might lead to inequalities and might not benefit the most disadvantaged people (18). There may be a relationship between an individual's social status, the probability of contracting a disease, and his/her life expectancy; in this context, social inequalities could end up affecting people (19). Faced with this reality, the citizen's experience is essential in terms of patient-centered health care delivery and involvement (20).
Diabetes mellitus in the equipment and use of ICT
Diabetes mellitus (DM) is a serious chronic disease (CD) that is on the rise (21–23). Regardless of the patient's age, good care in the context of this CD is not easy, and has to be approached with the help of ongoing medical care and multifactorial interventions that do not simply focus on glycemic control (24, 25). To achieve this, there is a need to reduce (as much as possible) the risk of developing complications associated with DM through good self-management (26). Additional factors to consider include a proper diet, supervised physical exercise, healthy behaviors, and control of indicators such as blood pressure, lipid values, and thrombotic control (27, 28). A lot of sacrifice is required and will only be attained if the patient has a lot of discipline, support from his/her environment, and seeks help from professionals to establish guidelines to avoid dreaded consequences, such as the need to be hospitalized due to diabetic decompensation, or limb amputations with all the emotional and economic implications that they entail (29–34).
Ongoing digitization in DM management offers new opportunities for patients, their environment, and healthcare professionals. Improvements have had a particular impact on patients' glycemic control, as well as enhancing patient autonomy and quality of life (35). The (36) uses the term “diabetes technology” to describe the hardware, devices, and software that people use to manage DM, ranging from controlling blood glucose levels to lifestyle. Previously, DM technology consisted of insulin administered by various devices, as well as monitoring using a meter or a continuous glucose monitor. Currently, hybrid devices have been available that not only monitor glucose; they can also administer insulin, and some of them have built-in software that assists the patient and others in his/her environment in terms of diabetic control (22, 37).
Thus, awakening interest and encouraging the involvement of patients (young and old) and others in their environment will raise awareness of this reality. The technology developed in recent decades can be of great help to patients, others in their environment, and health professionals in order to provide patients with resources to face their CD (38–40). New innovations include the Móvil electronic device (MED) (41–50).
Special emphasis should also be placed on the fact that on the internet, users can exchange information with other people, as well as discuss any queries they may have in relation to DM. To this end, users must have the appropriate technological equipment (e.g., suitable hardware and software, an internet connection, etc.). Since the most widely used search engine is Google, it is possible to use Google Trends (GT) to monitor what the population can find out about DM through this search engine (51).
Diabetes mellitus and the impact of digital competency on healthcare in Spain
As discussed above, accessibility to ICT is considered an SDOH; inequality in access to information for the population, as well as managing access to health services, are critical. Given the need to establish digital governance, digital competence is one of the factors that can have the greatest impact on social inequality (52).
Furthermore, the importance of the educational structure in explaining proper use of the internet should be emphasized (53). According to (54), studies carried out in Spain on the determinants of the use of e-Government found that digital skills and confidence in the internet could have an impact on the use of e-Government. This also suggests that digital skills are affected by citizens' resources, economic and education level, age, and gender. The same study noted that trust in the internet was conditioned (among other factors) by the concern of being the object of advertising. Extrapolating these findings to health issues in Spain, within the context of ongoing improvement, the Quality Plan for the National Health System of Spain (2006–2010) included issues such as protection, health promotion, and prevention. These actions are in line with the principles established in the Tallinn Charter, signed in 2008 by the Spanish government. Within this framework, we carried out the present study to reduce health inequality. The abovementioned actions are framed within the general policies of Spain's Ministry of Health and Social Policy, and one of those actions relies on ICT to improve care provided to the Spanish population (55).
From the standpoint of worrisome data related to inequality, death, prevalence, and cost in Spain (56), a CD such as DM must be seriously considered. Previous referrals to the need for patient care must always be present, because poor care related to DM can have serious consequences for the patient's health, as well as for economic costs (56–61). Unfortunately, two of the sequelae of this poorly controlled illness are hospitalizations (62–64) and amputations (65–68).
We aimed to analyze the impact of inadequate diabetic control as direct consequences of DM among the autonomous communities of Spain (ACS), along with socioeconomic factors related to health, including inequalities in access to ICT as an SDOH (between territories, as well as citizens' interest in online information searches about DM. Hence, we aimed to scrutinize hospitalizations and amputations as direct consequences of DM in ACS, as well as their relationship with socioeconomic factors of health, including inequality in access to ICT throughout the ACS and the population's behavior toward online information searches about DM in 2019 (before pre-pandemic trends).
This research paper is structured as follows: Section 2 “materials and methods” explains the steps to set up the database as well as the methodology and software used. Section 3 “Results” shows the results of the research. Section 4 “Discussion” shows the relevant findings of the present work.
Materials and methods
We performed our study in the following steps:
(1) Database design and sources searched
In order to build a database, we needed to check different sources.
(a) Government sources
(a1) Spain health ministry (69).
- A profile of the Spanish population: Education level and poverty. We coded these variables as “education” and “poverty.”
- Health data related to hospitalizations and amputations caused by DM. We coded these variables as “hospital” and “amputation.”
- Public health spending managed by the ACS per inhabitant. We coded this variable as “healthcare.”
(a2) Ministry of Economic Affairs and Digital Transformation (State Secretariat for Digitalization and Artificial Intelligence Department) (70). We extracted information related to the percentage of broadband coverage through different connection technologies and speeds (Table 1).
- Asymmetric Digital Subscriber Line (“ADSL ≥ 2 Mbps” and “ADSL ≥ 10 Mbps”). We coded these variables as “adsl.2 mb” and “adsl.10 mb.”
- Very High-Rate Digital Subscriber Line (VDSL). We coded this variable as “vdsl.”
- Hybrid Fiber Coaxial (HFC). We coded this variable as “hfc.”
- Fiber to the Home (FTTH). We coded this variable as “ftth.”
- Wireless ≥ 30 Mbps. We coded this variable as “in.30.”
- Universal Mobile Telecommunications System (UMTS) with High-Speed Packet Access (HSPA). “umts.”
- Long-Term Evolution (LTE) 4G. We coded this variable as “lte.”
- Speech per coverage ≥30 Mbps. We coded this variable as “sp30 mb.”
- Speech per coverage ≥100 Mbps. We coded this variable as “sp100 mb.”
- Number of households. We extracted this information from (71) since it contains data on the number of households for 2019.
(b) Instituto Nacional de Estadística (71)
Using the data provided by Instituto Nacional de Estadística, it was feasible to set up the database based on the information related to these four areas:
(b1) Area of ACS per square kilometer. We coded this variable as “housing_density.”
We extracted the information in sections b2, b3, and b4 from the “Survey on Equipment and Use of [ICT] in Households, 2019.” The population analyzed was between 16 and 74 years of age.
(b2) ICT product equipment in homes.
The internet access of primary households by ACS and type of connection. We considered the number of households and those with broadband and narrowband connections. We coded these variables as “broadband” and “narrowband.”
(b3) Internet services used for specific reasons according to the type of service and by ACS. This relates to citizens who searched for information about health issues. We coded this variable as “health.info.pop.”
(b4) The main reasons for which main homes do not have internet access by ACS.
Reasons included the cost of having hardware (we coded this variable as “hardware.cost”) or the cost of the connection (we coded this variable as “connect.cost”).
(c) Google Trends
The internet is a good environment for understanding individuals' concerns and needs. GT is a very good tool to monitor citizens' interests, parameterizing the information collected through the search engine's users (51). In this way, GT collected data in 2019 through users who searched for the term “diabetes mellitus,” which shaped the respective proportion through the relative search volume (RSV). The information obtained by the GT has been used as a subrogate of online health information seeking behavior. We parameterized the respective normalization from 0 to 100 and matched it to the highest proportion of the searched term. We coded this variable as “hits.dm.”
(2) Methodology and software
(a) Methodology
To identify patterns to carry out the current research, it became necessary to set up the database, relying on the sources indicated above. This involved the following steps:
(a1) We created the corresponding projection on different maps of Spain (see Appendix 1).
(a2) We used a multivariate method to treat the variables. We took into account that the variables extracted were both quantitative and qualitative. As such, we used factorial analysis of mixed data (FAMD) to classify the data (72, 73).
(b) Software
We used several free R software libraries to achieve the abovementioned outputs (72–74). We used FactoMineR package and FactoExtra package for FAMD. To create the maps included in this article and in the appendices (Appendix 1 and Figure 4), we employed several libraries, including the tmaptools package, the maptools package, the tmap package, the rgdal package, the tidyverse package, the sf package, the raster package, the rworldxtra package, the leaflet package, and the spdep package.
Results
We had to find the density of households in each ACS (“housing_density”). This variable is obtained by dividing the number of households (ACS) by the surface area (in km2) (ACS).
From these data, we extracted the quartile corresponding to this ratio for the ACS among the Spanish regions analyzed. This variable represents the quartile that denotes the density of the number of households between surface area (in km2) in relation to Spain as a whole. The “housing_density” variable characterizes each region according to the population of households and its dispersion in the region as an approximation of the pressure of the demand for technological coverage existing in each ACS.
We obtained a variable from the broadband coverage data. This qualitative variable represents the group to which the ACS belongs according to the percentage of broadband technology implementation and coverage speed in the region. Note that we coded this variable as “TICR.” Please, be aware that it refers to the existing technological coverage in 2019 for each of the ACS: ADSL ≥ 2 Mbp, ADSL ≥ 10 Mbps, VDSL, HFC, FTTH, wireless ≥ 30 Mbps, UMTS with HSPA, LTE, sp30 mb and sp100 mb.
Using FAMD, we computed a qualitative variable (TICR) that described five possible clusters to which an autonomous region belonged according to the characteristics studied. The results generated by applying FAMD to obtain the TICR variable are shown below.
Supplementary Figure S1 (see Appendix 2) presents the sedimentation graph with the five dimensions, into which we grouped the variables of broadband technology coverage and the existing connection speed in each ACS (see Table 2).
Supplementary Figures S2–S7 (see Appendix 2) outline the variables grouped according to their contribution to each dimension.
Table 3 displays the outcomes of the contributions of the variables analyzed in each dimension. Dimension 1 groups coverage by LTE broadband technologies and connection speed above 100 Mbps (Supplementary Figure S2, see Appendix 2). Dimension 2 groups broadband coverage technologies by ADSL ≥10 Mbps, ADSL ≥ 2 Mbps, VDSL, and FTTH (Supplementary Figure S3, see Appendix 2).
Dimension 3 includes HFC broadband coverage technologies and connection speeds ≥30 Mbps (Supplementary Figure S4, see Appendix 2). Dimension 4 groups wireless technologies >30 Mbps and UMTS with HSPA (Supplementary Figure S5, see Appendix 2).
Lastly, Dimension 5 is reserved for the qualitative variable “housing_density.”
Supplementary Figure S6 (see Appendix 2) shows the data obtained for the density of households per km2 in each ACS, and the map factor of the distribution of this variable, “housing_density,” as a qualitative variable. Correlation circle of the quantitative variables is shown in Supplementary Figure S7 (see Appendix 2).
Figure 1 represents the distribution of the ACS analyzed in the map factor. In addition, each region has been brought together according to its category in the “housing_density” variable. We can see that the ACS with the lowest density of households per km2 are those with the highest percentage of ADSL ≥10 MBps and VDSL coverage technologies. By contrast, those with a higher density of households are the ones with the greatest percentage of FTTH broadband technology coverage and connection speeds above 100 Mbps.
Supplementary Figure S8 (see Appendix 2) and Figures 2, 3 represents the distribution of the ACS according to Dimension 1 (LTE coverage technologies with connection speeds higher than 100 Mbps) and Dimension 3 (LTE broadband coverage and connection speeds higher than 30 Mbps).
Finally, we obtained the grouping of the ACS into five clusters (Figure 1) using FAMD.
- Cluster 1 (c1) groups the following ACS: Castilla y León (CL), Castilla La Mancha (CL), Aragón (AR), and Extremadura (EX).
- Cluster 2 (c2) groups the following regions: La Rioja (RI), Andalucía (AN), and Navarra (NC).
- Cluster 3 (c3) groups the following regions: Galicia (GA), Asturias (AS), and the Canary Islands (CN).
- Cluster 4 (c4) groups the following regions: Cantabria (CB), the Balearic Islands (IB), Murcia (MC), Catalonia (CT), and Valencia.
- Cluster 5 (c5) groups the following regions: Madrid (MD) and Basque Country (PV).
Appendix 1 and Figure 4 plots, geographically, the clusters of the ACS according to variables “TICR”, “amputation”, “hospital”, “healthcare”, “broadband”, “narrowband”, “education”, “hits.dm”, “health.info.pop”, “povertry”, “hardware.cost” and “connect.cost”. Boxplots of each of these variables are brought together in Appendix 3. We used TICR as categorical variable in the second phase of this research jointly with variables related to DM, SDOH for all the ACS in Spain.
Table 4 summarizes the descriptive statistics of the variables related to DM, such as the number of hospitalizations (“hospital”) and amputations (“amputation”). On the other hand, the database has been filled in with variables related to the SDOH, such as education level and poverty. In addition, an analysis of the technological determinants of these regions was needed, such as the type of connection (broadband or narrowband) and the economic reasons for not having internet access at home, either due to the cost of the computer equipment to connect (“hardware.cost”) or the connection cost (“connet.cost”). Degree of broadband coverage for each type of technology and its connection speed (TICR) has been analyzed. It also included the use of the internet (aggregated for the ACS as a whole) for searches on health-related information in general (“health.info.pop”), and the volume of online searches for the keyword “diabetes mellitus” (“hits.dm”). Finally, we included the overall health spending per patient managed by each ACS.
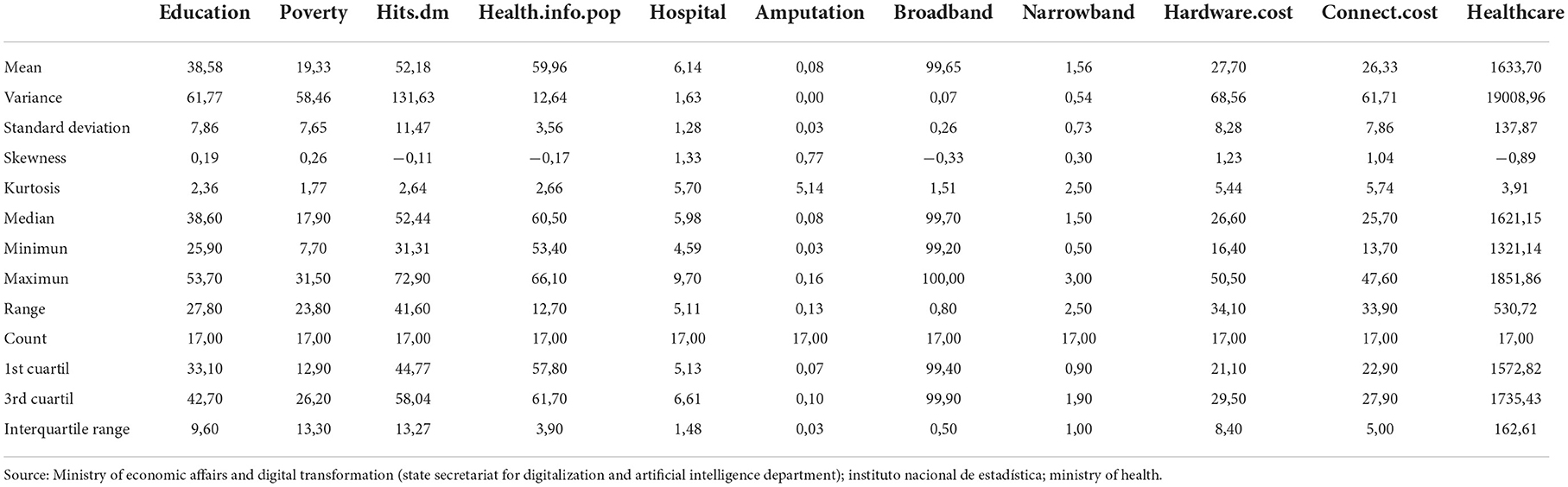
Table 4. Summary of the DM variables, determinants of health, health information searches, type of connection, and reasons for non–use of the internet at home.
Table 5 outlines the correlations between the abovementioned variables.
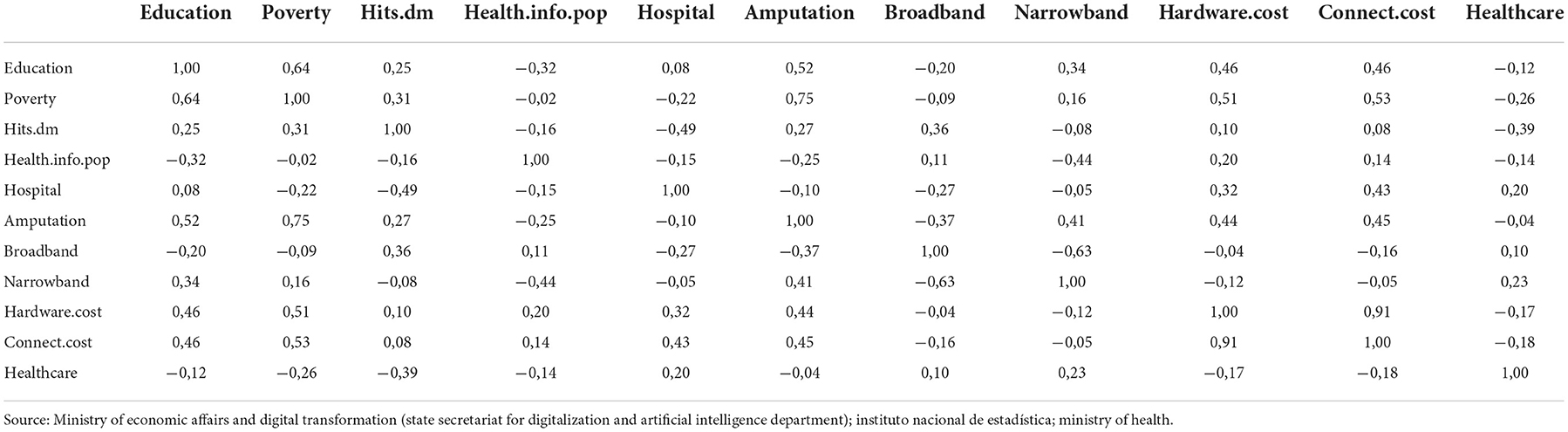
Table 5. Correlations between variables “education”, “poverty”, “hits.dm”, “health.info.pop”, “hospital”, “amputation”, “broadband”, “narrowband”, “hardware.cost”, “connect.cost”, “healthcare”.
In reference to Figure 5, the first two dimensions explain 44.85% of the cumulative variance percent. Dimension 1 (Table 6 and Figure 5) explains 25.86% of the variance, clustering the variables of “education,” “poverty,” “amputation,” and “connect.cost.” Dimension 1 (Supplementary Figure S9, see Appendix 2) summarizes (by its level of contribution to this dimension) the number of amputations caused by DM, along with the socioeconomic factors linked to the level of education and poverty as SDOH, as well as the economic reasons given for not having an internet connection at home (Supplementary Figures S9–S14, see Appendix 2).
Dimension 2 explains 18.98% of the variance percent (Supplementary Figure S10, see Appendix 2) clustering the variables “narrowband,” “hardware.cost,” and “broadband” related to the types of internet connection speed in people's homes and the economic reasons for not using the internet at home in relation to the cost of computer equipment.
Dimension 3 explains 15.63% of the variance percent (Supplementary Figure S11, see Appendix 2) shows the variables related to the volume of online searches for the term “diabetes mellitus” (RSV), together with the number of hospitalizations caused by DM. Dimension 4 (Supplementary Figure S12, see Appendix 2) represents the variable “health.info.pop,” which relates to people's use of the internet to search for information on health-related topics in general.
Lastly, Dimension 5 includes the variables of healthcare spending per patient managed by the ACS (healthcare), and the variable that categorizes each region analyzed according to its level of broadband coverage by technology and connection speed (TICR). See Supplementary Figure S13 (Appendix 2).
Figure 6 represents the distribution of the ACS in the map factor and in which, in turn, each region is categorized according to the value obtained in the TICR variable. Supplementary Figure S14 (see Appendix 2) plots the circle of correlations of the quantitative variables, which allowed us to check the clusters between variables, as well as their level of contribution to the corresponding dimensions.
Thus, the results obtained previously in the correlation coefficients in Table 5 show these variables related to amputations as a consequence of DM (“amputation”), together with SDOH such as poverty, education level (“education”), and internet connection cost (“connect.cost”). Figure 6 represents ACS with the highest level of amputations caused by DM, with regions that in turn have socioeconomic conditions of greater poverty, lower levels of education, and a higher share of the population whose reason for not having internet access for individual use derives from economic reasons due to the cost of the connection. Regarding Dimension 2 (Supplementary Figure S14, see Appendix 2) and as mentioned before (according to Table 5), the variables related to the proportion of the population that has a narrowband vs. broadband internet connection don't have internet at home due to the economic cost of computer equipment.
In Figure 6, it's highlighted that the existence of a greater number of amputations caused by DM, coincides with more severe socioeconomic conditions (poverty and education level). A greater amount of the population is unable to have an internet connection and computer equipment for economic reasons. It should be noted that there is a coincidence of higher numbers of amputations, higher poverty, and lower education level. We also identified a higher share of the population that is using narrowband for an internet connection. In other words, the areas with the lowest levels of broadband coverage and connection speed (coinciding with the highest share of the population reporting the use of narrowband for an internet connection) are also areas where there are higher poverty rates and lower education levels and, in turn, more economic difficulties in accessing the internet. However, these regions are also characterized, compared to the rest, by higher numbers of amputations due to DM.
Figure 6 also shows ACS with lower amputation rates, but also with better socioeconomic conditions (poverty and education), together with lower rates of narrowband housing, fewer cases of not using the internet due to economic cost, and greater use of broadband. In addition, there is a coincidence of the ACS which, according to the TICR classification, belongs to clusters 2, 4 and 5. This implies that a greater percentage of the population has a broadband connection and speeds higher than 100 Mbps.
Next, we analyzed the relationship between the variables linked to DM (“hospital” and “amputation”), socioeconomic factors (“poverty” and “education”), types of internet connection speeds (“broadband” and “narrowband”), and the share of the population that uses the internet to search for information about health in general (“health.info.pop”) and DM in particular (“hits.dm”). According to Supplementary Figure S15 (see Appendix 2), there is no correlation of the hits.dm variable with socioeconomic factors, type of internet connection band, economic reasons for not using the internet, or the amputation rate. If we compare this relationship in Supplementary Figure S16 (see Appendix 2), we can also observe that the variable health.info.pop is not related to the health determinant variable (the amputation rate), to the type of connection, or to the reasons for using or not using the internet. However, as with the “hits.dm,” the variable for searching for information on the internet on health issues (“health.info.pop”) also has a negative relationship with the rate of hospitalizations caused by DM (“hospital”), although less than the variable referred to RSV on “hits.dm.” Figure 7, Supplementary Figure S17 shows that there isn't a TICR pattern in ACS (Supplementary Figures S16–S18, see Appendix 2).
Dimensions 3 and 4 (Supplementary Figure S18, see Appendix 2), variable “health.info.pop” is not correlated with “hits.dm” or with “hospital.” There isn't a TICR pattern in ACS in Figure 8.
Finally, we explored the distribution of the ACS according to dimensions 1 and 5. Based on the clusters obtained according to the correlations between the variables (Supplementary Figure S19, see Appendix 2), healthcare spending per patient managed by the ACS (“healthcare”) is not correlated with amputation rate (“amputation”) or with the social and economic conditioning factors of the regions (“poverty” and “education”), or with the economic reasons for not having an internet connection for individual use (“hardware.cost” and “connect.cost”). Figure 9 shows that there isn't a TICR pattern in ACS.
Discussion
The present study coincides with the conclusions of prior research, discussed above, related to inequality and the use of ICT (5, 17–19, 54). Likewise, we also agree with (53) on the importance of education and inequality in internet use, and with (10) approach to internet use. We agree with (2) on what kinds of inequalities can affect society.
Our results revealed a greater number of amputations in regions (ACS) with a higher rate of poverty and a lower level of education. These are regions with the highest number of individuals who have declared, according to official statistics, that they do not use the internet for economic reasons, especially because of connection costs. Among those who do use it, they state that the connections have been through narrowband. Furthermore, according to the variable obtained in this study, these ACS are regions with the greatest percentage of internet coverage among the set of technologies analyzed and at the lowest speed compared to the other regions.
On the other hand, ACS with fewer amputations have lower aggregate poverty rates, higher levels of education, and fewer individuals who report not using the internet for economic reasons. Moreover, with regard to the variable created, TICR, these are regions with greater internet coverage on average in comparison to the rest of the ACS among the set of technologies, and with higher connection speeds. Regarding the search for online information on health issues in general and DM in particular, we noted that people in ACS with a lower number of hospitalizations had a greater interest in searching for the term “diabetes mellitus,” and, unexpectedly, in regions with a higher number of hospitalizations due to DM. We did not detect any relationship between hospitalizations due to DM and use of the internet declared by users regarding searches on topics about health in general. In relation to online searches and internet coverage and connection speeds, the results were disparate, and we observed no pattern between the regions. This was the case with health care costs per patient and the variable TICR, with different relationships between the ACS.
This study on the effects of DM in Spain, related to the rates of amputations and hospitalizations as a consequence of DM, includes as a novelty the use of technological determinants of health. To this end, and as a contribution of this work, we obtained the TICR variable in the initial phase using FAMD. This variable classifies the ACS according to their percentage of broadband coverage based on different existing technologies, as well as connection speeds. Subsequently, this new qualitative variable that categorizes the Spanish regions according to their ICT infrastructure has been linked to variables related to the type of internet connection, the reason for using the internet to search for information on health in general and DM in particular, and economic reasons for not having internet access at home. This set of technological determinants of health has been tied to health-related socioeconomic variables (poverty and education level), in addition to health spending.
Please note that, as a limitation of this study, we extracted the sources (aggregated data) of the database from official institutions. Ceuta and Melilla (two Spanish ACS) were excluded from this study because some variables needed were not available. Furthermore, we extracted the data related to the monitoring of interest in DM through the internet via GT; this tool is linked to user searches on the Google search engine. Please, be aware that in this research, the results were not classified by population age ranges in patients with DM due to the technological variables used in this study. Data available did not show these demographic characteristics. In addition, the multivariate methodology applied has been useful for measuring and explaining the degree of relationships between the selected variables.
This research is propitious to open new lines of work, such as performing an exhaustive study of the same in each autonomous community, adding more variables related to the telecommunications sector. It would also be interesting to evaluate more sequelae resulting from DM and, for this purpose, to use microdata. The information generated would be useful for public policymakers and, in this way, helpful for generating proposals for citizens to promote the use of ICT, education, and ultimately contribute to the development of eHealth.
Data availability statement
Publicly available datasets were analyzed in this study. This data can be found here: Spain's Health Ministry, http://inclasns.msssi.es/main.html; Ministry of Economic Affairs and Digital Transformation, https://portal.mineco.gob.es/; Google Trends, https://trends.google.es; Spain's National Institute of Statistics, https://www.ine.es.
Author contributions
Conceptualization, formal analysis, investigation, and writing—original draft preparation: IBF. Methodology, resources, and data curation: IBF and FCV. Validation and supervision: IBF, FCV, MCL, and MJPL. Writing—review and editing: IBF, FCV, and MCL. Project administration: MCL and IBF. All authors have read and agreed to the published version of the manuscript.
Acknowledgments
We would like to thank Editage (www.editage.com) for English language editing.
Conflict of interest
The authors declare that the research was conducted in the absence of any commercial or financial relationships that could be construed as a potential conflict of interest.
Publisher's note
All claims expressed in this article are solely those of the authors and do not necessarily represent those of their affiliated organizations, or those of the publisher, the editors and the reviewers. Any product that may be evaluated in this article, or claim that may be made by its manufacturer, is not guaranteed or endorsed by the publisher.
Supplementary material
The Supplementary Material for this article can be found online at: https://www.frontiersin.org/articles/10.3389/fpubh.2022.1033461/full#supplementary-material
References
1. Espelt A, Borrell C, Roskam AJ, Rodríguez-Sanz M, Stirbu I, Dalmau-Bueno A, et al. Socioeconomic inequalities in diabetes mellitus across Europe at the beginning of the 21st century. Diabetologia. (2008) 51:1971–9. doi: 10.1007/s00125-008-1146-1
2. Espelt A, Continente X, Domingo-Salvany A, Domínguez-Berjón MF, Fernández-Villa T, Monge S, et al. La vigilancia de los determinantes sociales de la salud. Gaceta Sanitaria. (2016) 30:38–44. doi: 10.1016/j.gaceta.2016.05.011
3. Islam MM. Social Determinants of health and related inequalities: confusion and implications. Front Public Health. (2019) 7:11. doi: 10.3389/fpubh.2019.00011
4. Jakab Z, Marmot M. Social determinants of health in Europe. Lancet. 2012) 379:103–5. doi: 10.1016/S0140-6736(11)61511-0
5. Sieck CJ, Sheon A, Ancker JS, Castek J, Callahan B, Siefer A. Digital inclusion as a social determinant of health. NPJ Digital Med. (2021) 4:52–52. doi: 10.1038/s41746-021-00413-8
6. International Telecommunication Union (2019). Measuring digital development facts and figures. International Telecommunication Union. Available online at: https://www.itu.int/en/ITU-D/Statistics/Pages/facts/default.aspx (accessed May 23, 2022).
7. Organisation for Economic Co-operation Development OECD. Guide to Measuring the Information Society. 2011, OECD Publishing. Available online at: https://www.oecd.org/digital/ieconomy/oecdguidetomeasuringtheinformationsociety2011.htm (accessed May 23, 2022).
8. Organisation for Economic Co-operation and Development. Measuring the Digital Transformation: A Roadmap for the Future. Paris: OECD Publishing. (2019)
9. Lee JW. Big data strategies for government, society and policy-making. J Asian Finance Economic and Business. (2020) 7:475–87. doi: 10.13106/jafeb.2020.vol7.no7.475
10. Van Winden W. The end of social exclusion? On information technology policy as a key to social inclusion in large European cities. Reg Stud. (2001) 35:861–77.
11. Katz JE, Rice RE. Social consequences of Internet use. Access, involvement, and interaction. Edu Inform. (2002) 20:277–9. doi: 10.7551/mitpress/6292.001.0001
12. World Health Organization Atlas of eHealth Country Profiles. The Use of eHealth in Support of Universal Health Coverage. (2016). Available online at: https://www.who.int/publications/i/item/9789241565219 (accessed May 23, 2022).
14. World Health Organization (2016). Global Diffusion of eHealth: Making Universal Health Coverage Achievable: Report of the Third Global Survey on eHealth. World Health Organization. Available online at: https://www.who.int/publications/i/item/9789241511780 (accessed May 23, 2022).
15. World Health Organization. Global Strategy on Digital Health 2020-2025. (2021). Retrieved from: https://www.who.int/publications/i/item/9789240020924 (accessed May 23, 2022).
16. Lewis T, Synowiec C, Lagomarsino G, Schweitzer J. E-health in low- and middle-income countries: findings from the Center for Health Market Innovations. Bull World Health Organ. (2012) 90:332–40.
17. Yao R, Zhang W, Evans R, Cao G, Rui T, Shen L. Inequities in Health Care Services Caused by the Adoption of Digital Health Technologies: Scoping Review. J Med Internet Res. (2022) 24:e34144–e34144. doi: 10.2196/34144
18. Veinot TC, Mitchell H, Ancker JS. Good intentions are not enough: how informatics interventions can worsen inequality. JAMIA. (2018) 25:1080–8. doi: 10.1093/jamia/ocy052
19. Matteucci I. Social determinants of health inequalities: moving toward a socio-constructivist model supported by information and communication technologies. Problemi di bioetica. (2015) 26:206–17. doi: 10.1080/11287462.2015.1101213
20. Windrum P, García-Goñi M, Coad H. The Impact of Patient-Centered versus Didactic Education Programs in Chronic Patients by Severity: The Case of Type 2 Diabetes Mellitus. Value in health. (2016) 19:353–62. doi: 10.1016/j.jval.2016.01.014
21. Lin X, Xu Y, Pan X, Xu J, Ding Y, Sun X, et al. Global, regional, and national burden and trend of diabetes in 195 countries and territories: an analysis from 1990 to 2025. Sci Rep. (2020) 10:14790–14790. doi: 10.1038/s41598-020-71908-9
22. American Diabetes Association Introduction: Standards of Medical Care in Diabetes−2022. Diabetes Care. (2022) 45 (Suppl_1): S1–S2. doi: 10.2337/dc22-Sint
23. American Diabetes Association Professional Practice Committee 2. Classification and Diagnosis of Diabetes: Standards of Medical Care in Diabetes−2022. Diabetes Care 1 January 2022 45 (Supplement_1): S17–S38. doi: 10.2337/dc22-S002
24. Lee, YJ, Yoo S, Yi S, et al. Trajectories in glycated hemoglobin and body mass index in children and adolescents with diabetes using the common data model. Sci Rep. (2021) 11:14614. doi: 10.1038/s41598-021-94194-5
25. Gomes MB, Tang F, Chen H, Cid-Ruzafa J, Fenici P, Khunti K, et al. Socioeconomic Factors Associated With Glycemic Measurement and Poor HbA1c Control in People With Type 2 Diabetes: the Global DISCOVER Study. Front Endocrinol. (2022) 13:831676. doi: 10.3389/fendo.2022.831676
26. Kowall B, Rathmann W, Stang A, Bongaerts B, Kuss O, Herder C, et al. Perceived risk of diabetes seriously underestimates actual diabetes risk: The KORA FF4 study. PLoS ONE. (2017) 12:e0171152–e0171152. doi: 10.1371/journal.pone.0171152
27. Richards SE, Wijeweera C, Wijeweera A. Lifestyle and socioeconomic determinants of diabetes: Evidence from country-level data. PLoS ONE. (2022) 17:e0270476. doi: 10.1371/journal.pone.0270476
28. Rohani H, Bidkhori M, Eslami AA, Sadeghi E, Sadeghi A. Psychological factors of healthful diet promotion among diabetics: an application of health action process approach. Electronic Physician. (2018) 10:6647–54. doi: 10.19082/6647
29. García-Goñi M, Hernández-Quevedo C, Nuño-Solinís R, Paolucci F. Pathways towards chronic care-focused healthcare systems: Evidence from Spain. Health Policy (Amsterdam). (2012) 108:236–45. doi: 10.1016/j.healthpol.2012.09.014
30. Hoffstad O, Mitra N, Walsh J, Margolis DJ. Diabetes, lower-extremity amputation, and death. Diabetes Care. (2015) 38:1852–7. doi: 10.2337/dc15-0536
31. Jeffcoate WJ, Van Houtum WH. Amputation as a marker of the quality of foot care in diabetes. Diabetologia. (2004) 47:2051–8. doi: 10.1007/s00125-004-1584-3
32. Jeffcoate W, Game F, Morbach S, Narres M, Van Acker K, Icks A. Assessing data on the incidence of lower limb amputation in diabetes. Diabetologia. (2021) 64:1442–6. doi: 10.1007/s00125-021-05440-4
33. Moxey PW, Gogalniceanu P, Hinchliffe RJ, Loftus IM, Jones KJ, Thompson MM, et al. Lower extremity amputations - a review of global variability in incidence. Diabetic Med. (2011) 28:1144–53. doi: 10.1111/j.1464-5491.2011.03279.x
34. Tchero H, Kangambega P, Lin L, Mukisi-Mukaza M, Brunet-Houdard S, Briatte C, et al. Cost of diabetic foot in France, Spain, Italy, Germany and United Kingdom: A systematic review. Annales d'endocrinol. (2018) 79:67–74. doi: 10.1016/j.ando.2017.11.005
35. Eberle C, Stichling S. Löhnert M. Diabetology 40: scoping review of novel insights and possibilities offered by digitalization. J Med Internet Res. (2021) 23:e23475. doi: 10.2196/23475
36. American Diabetes Association Professional Practice Committee. 7 diabetes technology: standards of medical care in diabetes-−2022. Diabetes Care. (2002) 45 (Suppl. 1):S97–112. doi: 10.2337/dc22-S007
37. World Health Organization. Improving diabetes outcomes for all, a hundred years on from the discovery of insulin: report of the Global Diabetes Summit. (2021). Available online at: https://www.who.int/publications/i/item/9789240038943 (accessed May 23, 2022).
38. Doyle-Delgado K, Chamberlain JJ. Use of diabetes-related applications and digital health tools by people with diabetes and their health care providers. Clin Diabetes. (2020) 38:449–61. doi: 10.2337/cd20-0046
39. Grunberger G, Sherr J, Allende M, Blevins T, Bode B, Handelsman Y, et al. American association of clinical endocrinology clinical practice guideline: the use of advanced technology in the management of persons with diabetes mellitus. Endocrine Pract. (2021) 27:505–37. doi: 10.1016/j.eprac.2021.04.008
40. Sim R, Lee SWH. Patient preference and satisfaction with the use of telemedicine for glycemic control in patients with type 2 diabetes: a review. Pat Prefer Adherence. (2021) 15:283–98. doi: 10.2147/PPA.S271449
41. Albanese-O'Neill A, Schatz DA, Thomas N, Bernhardt JM, Cook CL, Haller MJ, et al. Designing online and mobile diabetes education for fathers of children with type 1 diabetes: mixed methods study. JMIR Diabetes. (2019) 4:e13724.
42. Ruiz de., Adana M.S, Alhambra-Expósito M.R, Muñoz-Garach A, Gonzalez-Molero I, Colomo N, Torres-Barea I, R on behalf of the Diabetes Group of SAEDYN (Andalusian Society of Endocrinology, Diabetes, and Nutrition), randomized study to evaluate the impact of telemedicine care in patients with type 1 diabetes with multiple doses of insulin and suboptimal HbA1c in Andalusia (Spain): PLATEDIAN Study. Diabetes Care. (2020) 43:337–42. doi: 10.2337/dc19-0739
43. Fleming GA, Petrie JR, Bergenstal RM, Holl RW, Peters AL, Heinemann L. Diabetes digital app technology: benefits, challenges, and recommendations. A consensus report by the European Association for the Study of Diabetes (EASD) and the American Diabetes Association (ADA) Diabetes Technology Working Group. Diabetologia. (2019) 63:229–41. doi: 10.1007/s00125-019-05034-1
44. Imeri H, Desselle S, Hetemi D, Hoti K. Mobile electronic devices as means of facilitating patient activation and health professional empowerment related to information seeking on chronic conditions and medications: qualitative study. JMIR mHealth uHealth. (2021) 9:e26300. doi: 10.2196/26300
45. Iribarren SJ, Akande TO, Kamp KJ, Barry D, Kader YG, Suelzer E. Effectiveness of mobile apps to promote health and manage disease: systematic review and meta-analysis of randomized controlled trials. JMIR mHealth and uHealth. (2021) 9:e21563. doi: 10.2196/21563
46. Krishnan G, Selvam G. Factors influencing the download of mobile health apps: Content review-led regression analysis. Health Policy Technol. (2019) 8:356–64. doi: 10.1016/j.hlpt.2019.09.001
47. Liu Z, Wang C, Yang D, Luo S, Ding Y, Xu W, et al. High engagement in mobile peer support is associated with better glycemic control in type 1 diabetes: a real-world study. J Diabetes Investig. (2022) 16:13870. doi: 10.1111/jdi.13870
48. Martinez-Millana A, Jarones E, Fernandez-Llatas C, Hartvigsen G, Traver V. App features for type 1 diabetes support and patient empowerment: systematic literature review and benchmark comparison. JMIR mHealth and uHealth. (2018) 6:e12237. doi: 10.2196/12237
49. McGaugh SM, Edwards S, Wolpert H, Zaharieva DP, Gulati N, Riddell MC. The development of an exercise advisor app for type 1 diabetes: digitization facilitates more individualized guidance. J Diabetes Sci Technol. (2022) 16:760–3. doi: 10.1177/1932296820979811
50. Xie LF, Itzkovitz A, Roy-Fleming A, Da Costa D, Brazeau AS. Understanding Self-guided web-based educational interventions for patients with chronic health conditions: systematic review of intervention features and adherence. J Med Internet Res. (2020) 22:e18355. doi: 10.2196/18355
51. Google Trends (2022). Available online at: https://trends.google.es (accessed December 1, 2021).
52. Ebbers WE, Jansen MGM, van Deursen AJAM. Impact of the digital divide on e-government: Expanding from channel choice to channel usage. Gov Inf Q. (2016) 33:685–92. doi: 10.1016/j.giq.2016.08.007
53. Billon M, Crespo J, Lera-Lopez F. Do educational inequalities affect Internet use? An analysis for developed and developing countries. Telematics Inform. (2021) 58:101521. doi: 10.1016/j.tele.2020.101521
54. Robles JM, Torres-Albero C, Villarino G. Inequalities in digital welfare take-up: lessons from e-government in Spain. Policy Stud. (2022) 43:1096–111. doi: 10.1080/01442872.2021.1929916
55. Spanish Ministry of Health. Quality Plan for the National Health System of Spain. (2022). Available online at: https://www.sanidad.gob.es/organizacion/sns/planCalidadSNS/docs/InformePlanCalidad_ENG.pdf
56. Ruiz-Ramos M, Escolar-Pujolar A, Mayoral-Sánchez E, Corral-San Laureano F, Fernández-Fernández I. Diabetes Mellitus in Spain: death rates, prevalence, impact, costs and inequalities. Gaceta Sanitaria. (2006) 20:15–24. doi: 10.1157/13086022
57. Delgado-Velandia M, Gonzalez-Marrachelli V, Domingo-Relloso A, Galvez-Fernandez M, Grau-Perez M, Olmedo P, et al. Healthy lifestyle, metabolomics and incident type 2 diabetes in a population-based cohort from Spain. Int J Behav Nutr Phys Act. (2022) 19:8. doi: 10.1186/s12966-021-01219-3
58. Fernandez-Piciochi C, Martín-Saborido C, Bimbela-Pedrola JL, Sarria-Santamera A. The economic burden of anxiety and depression on the working age population with diabetes in Spain. Int J Health Plann Manage. (2022) 37:715–24. doi: 10.1002/hpm.3367
59. Larrañaga I, Arteagoitia JM, Rodriguez JL, Gonzalez F, Esnaola S, Piniés JA. Socio-economic inequalities in the prevalence of Type 2 diabetes, cardiovascular risk factors and chronic diabetic complications in the Basque Country, Spain. Diabetic Med. (2005) 22:1047–53. doi: 10.1111/j.1464-5491.2005.01598.x
60. Núñez M, Díaz S, Dilla T, Reviriego J, Pérez A. Epidemiology, Quality of Life, and Costs Associated with Hypoglycemia in Patients with Diabetes in Spain: A Systematic Literature Review. Diabetes Ther. (2019) 10:375–92. doi: 10.1007/s13300-019-0563-0
61. Osumili B, Artime E, Mitchell B., Rubio-de Santos M, Díaz-Cerezo S, Giménez M, et al. Cost of severe hypoglycemia and budget impact with nasal glucagon in patients with diabetes in Spain. Diabetes Ther. (2022) 13:775–94. doi: 10.1007/s13300-022-01238-8
62. Lopez-de-Andres A, Carrasco-Garrido P, Esteban-Hernandez J, Gil-de-Miguel Á, Jiménez-García R. Characteristics and hospitalization costs of patients with diabetes in Spain. Diabetes Res Clin Pract. (2010) 89:e2–4. doi: 10.1016/j.diabres.2010.03.026
63. Zapatero-Gaviria A, Gómez-Huelgas R, Canora-Lebrato J, Ena-Muñoz J, Romero-Sánchez M, Mendez-Bailón M, et al. Analysis of hospitalizations by cardiovascular disease in the population with diabetes in Spain. Revista clínica espanõla (English edition). (2019) 219:124–9. doi: 10.1016/j.rceng.2018.09.006
64. Lara-Rojas CM, Pérez-Belmonte LM, López-Carmona MD, Guijarro-Merino R, Bernal-López MR, Gómez-Huelgas R. National trends in diabetes mellitus hospitalization in Spain 1997–2010: analysis of over 5.4 millions of admissions. Eur J Intern Med. (2019) 60:83–9. doi: 10.1016/j.ejim.2018.04.005
65. Almaraz MC, González-Romero S, Bravo M, Caballero FF, Palomo MJ, Vallejo R, et al. Incidence of lower limb amputations in individuals with and without diabetes mellitus in Andalusia (Spain) from 1998 to 2006. Diabetes Res Clin Pract. (2011) 95:399–405. doi: 10.1016/j.diabres.2011.10.035
66. Lopez-de-Andres A, Jiménez-García R, Aragón-Sánchez J, Jiménez-Trujillo I, Hernández-Barrera V, Méndez-Bailón M, et al. National trends in incidence and outcomes in lower extremity amputations in people with and without diabetes in Spain, 2001–2012. Diabetes Res Clin Pract. (2015) 108:499–507. doi: 10.1016/j.diabres.2015.01.010
67. Lopez-de-Andres A, Jimenez-Garcia R, Hernández-Barrera V, de-Miguel-Diez J, de-Miguel-Yanes JM, Omaña-Palanco R, et al. Time trends (2001-2019) and sex differences in incidence and in-hospital mortality after lower extremity amputations among patients with type 1 diabetes in Spain. Cardiovascular Diabetol. (2022) 21:65–5. doi: 10.1186/s12933-022-01502-y
68. Rodríguez Pérez MDC, Chines C, Pedrero García AJ, Sousa D, Cuevas Fernández FJ, Marcelino-Rodríguez I, et al. Major amputations in type 2 diabetes between 2001 and 2015 in Spain: regional differences. BMC Public Health. (2020) 20:54–54. doi: 10.1186/s12889-019-8137-7
69. Spanish Ministry of Health. Available online at: http://inclasns.msssi.es/main.html (accessed July 22, 2022).
70. Spanish Ministry of Economic Affairs and Digital Transformation. Available online at: https://avancedigital.mineco.gob.es/banda-ancha/cobertura/Documents/Cobertura-BA-2019.pdf (accessed July 22, 2022).
71. Instituto Nacional de Estadística. Available online at: http://www.ine.es (accessed July 22, 2022).
72. Pagès, J. Analyse factorielle de données mixtes. Revue de Statistique Appliquée. (2004) 52:93–111.
Keywords: diabetes mellitus, factor analysis of mixed data, social determinants of health, economics, inequalities
Citation: Bosch-Frigola I, Coca-Villalba F, Pérez-Lacasta MJ and Carles-Lavila M (2023) Diabetes mellitus and inequalities in the equipment and use of information technologies as a socioeconomic determinant of health in Spain. Front. Public Health 10:1033461. doi: 10.3389/fpubh.2022.1033461
Received: 31 August 2022; Accepted: 14 October 2022;
Published: 09 January 2023.
Edited by:
María Del Carmen Valls Martínez, University of Almeria, SpainReviewed by:
Fernando Gimeno Arias, University of Murcia, SpainJosé-María Montero, University of Castilla-La Mancha, Spain
Copyright © 2023 Bosch-Frigola, Coca-Villalba, Pérez-Lacasta and Carles-Lavila. This is an open-access article distributed under the terms of the Creative Commons Attribution License (CC BY). The use, distribution or reproduction in other forums is permitted, provided the original author(s) and the copyright owner(s) are credited and that the original publication in this journal is cited, in accordance with accepted academic practice. No use, distribution or reproduction is permitted which does not comply with these terms.
*Correspondence: Irene Bosch-Frigola, aWJvc2NoQHVzai5lcw==