- Illinois Natural History Survey, Prairie Research Institute, University of Illinois at Urbana-Champaign, Champaign, IL, United States
The interplay among invasive alien vectors and the species assemblage of native potential vectors in areas of range expansion may affect the dynamics of pathogen transmission. In this study we investigate how Aedes albopictus, an invasive mosquito of considerable public health concern fits within mosquito communities at the edge of its range of distribution. This was addressed using a 2-year field survey of mosquitoes in south-eastern Illinois. We found that Ae. albopictus was more broadly distributed in this region than previously realized, with new occurrence records for nine counties. Abundance of this species varied strongly and peaked in locations of low-intermediate overall mosquito species richness. This differed from overall mosquito abundance, as well as abundance of another important vector, Cx. pipiens, for which the abundance-richness relationships were best described with power functions. Metacommunity analyses revealed that mosquito communities showed a non-random distribution with a Clementsian gradient, which suggests a pattern whereby distinct species assemblages are associated with specific habitats or environmental conditions. Land use was a significant underlying factor shaping mosquito community structure and species assemblages. Multivariate analyses showed that while Ae. canadensis and Cx. pipiens complex mosquitoes were associated with high and low proportions of wetlands in the environment, respectively, Ae. albopictus was most strongly associated with urban settlements. This work sheds light on landscape-level processes, such as niche differentiation driven by urban and agricultural development, structuring mosquito communities. We suggest that mosquito community assessments across habitats be incorporated as part of a One Health vector surveillance approach to aid in the goal of prediction and prevention of new and (re-)emerging vector-borne diseases.
Introduction
The worldwide range expansion over recent decades of mosquito vectors of arthropod-borne viruses (arboviruses), such as Aedes albopictus and Ae. japonicus, poses a serious concern for public health (1, 2). The introduction and expansion of these container-breeding species can alter the epidemiology of arboviral diseases caused by native or introduced viruses and the interactions with other vectors (3). Examples include the recent outbreaks of chikungunya virus (Chikungunya virus, CHKV) in Italy and La Reunion (4, 5), and Zika virus (Zika virus genus Flavivirus, ZIKV) in Central Africa (6). In the United States, autochthonous transmission has been recorded for both these viruses (7–9). The spread of ZIKV through Central and South America and the morbidity associated with this virus (10, 11) intensified the public concerns about invasive mosquitoes and pathogens transmitted by them (10). In addition, these invasive alien mosquito species may co-occur with native known vectors serving as augmentative vector populations, ultimately increasing the incidence of arboviral diseases. For example, mixed populations of the native Ae. triseriatus and the exotic Ae. albopictus both contribute to the transmission of La Crosse virus (La Crosse orthobunyavirus, LACV), which has been the major cause of arboviral encephalitis among children in the United States (12–14).
Mosquito and arbovirus surveillance programs are a valuable tool to track viruses and define the risks for emerging diseases or increased virulence and persistence (15), and can benefit from being designed around an approach that targets the mosquito community as a whole (16, 17), including alien invasive species. However, the prevailing paradigm has historically been focused on single species of interest (18–20), posing challenges to implementing such programs (21). To develop comprehensive insight into the ways invasive mosquito vectors may affect vector-borne disease transmission patterns in newly invaded areas, it is important to not only understand the patterns and processes related to the distribution and abundance of invasive species, but to understand how they fit within or alter the broader mosquito community in newly invaded areas. One example of this relates to the paradigm of bridge vectors, species that due to their broader host utilization are unlikely to act as primary vectors driving transmission, but can play an important role in spreading pathogens between host species and increasing exposure in dead-end hosts such as humans (22). In the case of West Nile virus (genus Flavivirus, WNV), for instance, it has been suggested that in certain areas Ae. albopictus may play such a role (although this may be tempered by their apparent low feeding rate on avian species) (23), and understanding the extent to which they overlap within the landscape with primary vectors is an important component to understanding the risk (for humans) associated with their spread. Likewise, if competition occurs between possible vector species, understanding the consequences for co-occurrence is important in understanding how an invasive mosquito like Ae. albopictus may affect transmission of arboviruses such as WNV. Larval competition between Ae. albopictus and Cx. pipiens, for instance, has been documented and evidence suggests that Ae. albopictus is likely to outcompete Cx. pipiens in areas where they overlap and share larval development sites (7, 24–26). Additionally, a pressing question that was laid bare by the Zika epidemic was how an emerging arbovirus, once introduced into a new range, would be transmitted and which species of vectors would be involved in its transmission, leading to statistical predictive associations between vectors and viruses (27). Such novel associations between pathogens, hosts and vectors rely in part on the community ecology of vectors and understanding how invasive mosquitoes affect species assemblages (and thereby host-vector-pathogen networks) in different landscape contexts is thus likely to be an important component of predicting future risk.
The link between landscape composition and how it affects mosquito species assemblages and in turn the transmission intensity of vector-borne pathogens is an area of active study (28–30). A number of studies have explored the composition of mosquito communities in different habitats, for instance focusing on differences in species composition among different environments such as forest, field, or ecotone environments, or along urbanization gradients (18, 31–33). Relatively few studies however have assessed how invasive species such as Ae. albopictus take up residence within species assemblages and the landscape (32). Additionally, although we have studies that describe how mosquito species assemblages vary between different types of landscapes, there remains a lack of understanding regarding how (e.g., due to which processes) mosquito communities are assembled and in what manner they shift with changes in the landscape. Such questions require species distribution patterns to be addressed using a metacommunity framework which can statistically distinguish various possible distribution patterns, and can offer insight into the environmental components and processes that are primarily driving species assemblage structure among sites (34, 35). It has been suggested that processes involving entire communities at larger spatial scale may influence the dynamics of infectious diseases (36). Recently, Suzán et al. (37) proposed a conceptual model linking observed metacommunity structure with the distribution of potential and actual vectors or hosts across the landscape. According to this model, human modified landscapes will drive higher risk of emergence of diseases by favoring certain communities. They also hypothesized that nested metacommunities may support higher rates of transmission and pathogen prevalence across landscapes by supporting more competent vectors and related species. On the contrary, antinested structures (e.g., Clementsian), may interrupt pathogen transmission along a landscape gradient by filtering specific communities which share similar phylogenetic and ecological characteristics which determine vectorial capacity (37, 38). Understanding such patterns and processes in detail may aid in understanding how range expansion of an invasive vector might affect vector-borne disease transmission.
In response to concerns related to potential introduction of ZIKV into the United States, and a lack of systematic surveillance of Aedes species in Illinois prior to 2016, we initiated a 2-year Aedes albopictus surveillance project with the Illinois Department of Public Health. The objective of this project was to improve understanding of the current distribution and abundance of this invasive species in Illinois, at the current northward edge of this species' range in the U.S. (39).
Using mosquito surveillance data collected during this 2-year project (2016–2017) in south-eastern Illinois, we characterized mosquito community composition and diversity to improve understanding of the potential risk posed by invasive species in Illinois and spreading of potential vectors. We also assessed the underlying processes shaping the metacommunity structure. Specifically, we tested the hypothesis that mosquito assemblages are shaped by shared responses to a gradient of landscape-level variables defining the major boundaries among metacommunities.
Materials and Methods
Study Sites and Landscape Variables
A 2-year surveillance survey was conducted in 18 communities (hereafter locations: Albion, Benton, Cairo, Champaign, Charleston, Danville, Effingham, Fairfield, Lawrenceville, Marion, Marshall, Mattoon, Mt. Carmel, Mt. Vernon, Paris, Robinson, Salem, Tuscola) distributed in 17 counties in south-eastern Illinois. The locations were preselected with the intent to sample mosquito populations from a balance of counties from which Ae. albopictus either had or had not previously been reported, while including a diversity in the proportion of rural or semi-rural and urban habitats, which are the dominant landscape units in Illinois, and other natural habitat types. In each location, three sampling sites were selected in coordination with local public health districts. To characterize the habitat of each of the sampling sites, we made use of the Illinois Gap Analysis Program Land Cover Classification, 1999–2000 dataset (40). We used a 1 km buffer around each sampling site using the “gBuffer” function, and within this buffer calculated the proportion of land cover classified as either agricultural land, forested land, urban land, or wetland using the sp, rgdal and rgeos packages in R (41).
Mosquito Collection and Identification
Mosquitoes were sampled using a BG-sentinel 2 trap to which a proprietary lure was added as an additional cue (Biogents, Regensburg, Germany). Dry ice was added to the traps in order to sample a broader proportion of the mosquito community. Three BG-sentinel traps were set for an approximately 24-h period every 2-weeks in each location between July and October in 2016 and 2017. The samples were transported to the laboratory in a cool box containing dry ice and were subsequently identified to species on a chill table using taxonomic key provided by (42). In cases where morphological identification was not possible with confidence (e.g., due to loss of identifying characters), specimens were identified to genus only. For instance, morphological identification of possible Cx. pipiens, hybrids with Cx. quinquefasciatus, as well as Cx. restuans, typically being recorded as Culex spp. This group of species includes taxa that are known as vectors of pathogens such as Plasmodium spp. (avian malaria), Dirofilaria spp. (filarioid helminths), and viruses such as Usutu, Sindbis, St. Louis encephalitis virus and WNV (43). Because our analyses of the mosquito communities use distinct species as variables, the occurrence of morphologically similar or cryptic species has to be taken into account. Therefore, to further clarify the genetic diversity and structure of these individuals, a subsample of 492 individuals (representing the relative proportion of the total individuals collected in each site) was selected for molecular analyses.
DNA Extraction, PCR Amplification, and Sequencing
Total DNA was extracted from a single mosquito leg of each specimen using the Phire Tissue Direct PCR master mix kit (Thermo Fisher Scientific, Waltham, MA). The fragment of mitochondrial cytochrome C oxidase (COI) gene was amplified using the primer pair MTFN (5'-GGA TTT GGA AAT TGA TTA GTT CCT T-3') and MTRN (5'-AAA AAT TTT AAT TCC AGT TGG AAC AGC-3') (44). The amplicons were sequenced by the Keck Center at the University of Illinois at Urbana-Champaign. Each sequence read was BLAST searched at GenBank and the mosquito identification was selected if the sequence match was at least 97%. Sequences were edited by removing Ns at each end using a custom perl script and purging long successive Ns in the middle manually. To identify the samples, we performed TBLASTN searches against the standard nucleotide databases in NCBI under default settings except that only the top 10 hits were shown. Identification was confirmed only for those sequences with an identity value ≥ 97% and a matching length (query length * coverage) ≥ 600 bp compared with the reference sequences. Sequences matching more than three different species in the top 10 hits all showed low identity values therefore were excluded from further analyses. Sequences successfully identified as Cx. pipiens or matching both Cx. pipiens and Cx. quinquefasciatus but with low identity values or match length were aligned using PASTA v1.8.5 (45) under default settings. The alignment was checked and trimmed manually.
Haplotype Analyses of Culex pipiens Complex
In order to analyze the genetic diversity, the 492 individuals of Cx. pipiens complex were grouped based on their location of collection and the haplotype analysis was carried out. The DnaSP6 program was used to identify haplotype diversity (46). All population genetic parameters, including the number of polymorphic (segregating) sites, haplotype number (Hap), haplotype diversity (Hd), nucleotide diversity (n), and neutrality statistic information, such as Tajima's D, were calculated. The NETWORK ver. 4.6.1.2 program (www.fluxus-engineering.com) was used to construct a median-joining haplotype network (47) with maximum parsimony calculation. Relationships among the haplotypes were depicted with PopART (Population Analysis with Reticulate Trees) v. 1.7 (http://popart.otago.ac.nz) (48).
Statistical Analysis of Community of Culicidae
We first analyzed the functional forms describing the relationship between species richness (total number of species collected from a given location) and mosquito abundance per trap night of Ae. albopictus, Culex pipiens, and all mosquito species jointly. Specifically, we used the following functions: power (y = a*xb), logistic (), ricker (y = a*x*e(−b*x)), Hollling type 3 (), shepherd (), and a linear function (y = a*x). These functions were chosen to represent a wide range of commonly encountered functional forms, and not necessarily related to the original reason they were developed. Abundance was modeled using a Poisson distribution and parameter values with 95% confidence intervals were obtained through maximum likelihood estimation using the “mle2” function from the bbmle package in R (49).
The metacommunity dataset includes 54 sampling sites each representing a local community of mosquitoes. We tested the hypothesis that mosquito metacommunity was non-random and the structure was assessed using the framework (EMS, Elements of Metacommunity Structure framework) proposed by (34) and (35). Three metrics were calculated: coherence (degree to which a pattern can be collapsed into a single dimension, ordination axis, or the significance of modularity, nestedness or randomness shown by the metacommunity), turnover (calculated if positive coherence is detected and is the turnover in species composition along a dimension or ordination axis. It measures the number of species replacements), and boundary clumping (which takes into account how the edges of species range boundaries are distributed along a dimension or an ordination axis). The metrics were calculated using the equiprobable rows and columns (“r00” method) and fixed–proportional null model (“r1” method, i.e., preserves the site (row) frequencies, but uses column marginal frequencies as probabilities of selecting species) and 1,000 simulations in the R package metacom (50). Differences in the coherence and turnover (z-values) between groups of sites based on landscape variables were tested using a Kruskal-Wallis test.
The sampling sites were classified based on their landscape similarity, the Silhouette Coefficient method was used to define the number of groups and K-means algorithm technique to divide the sampling sites into given K groups with nearest mean. For each landscape category (agriculture, forested, wetland and urban), between-group comparisons were performed using 2-tailed t-test or Yuen-Welch's test, as appropriate. The spatial autocorrelation of landscape variables was evaluated using the Moran's I coefficient (51) based on spatial weights matrix, by testing the null hypothesis that there is a random distribution of the landscape types in the whole study area. The significance was performed utilizing the Monte-Carlo permutation test after 999 permutations. If the MC observed value is greater than that expected by chance then similar landscape values are near to each other across space (positive spatial autocorrelation), while a significant negative value of MC indicates dissimilarity among neighboring values (negative spatial autocorrelation). Moran's I was computed using “moran.randtest” function in adespatial package.
The k-mean clustering algorithm was applied to the landscape matrix to segregate the sampling sites into k groups, with each of them being allocated in the closest group in the distance to its center (52). To define the parameter of optimal number of groups, we first calculated the silhouette score using “fviz_nbclust” and “kmeans” functions in stats and factoextra R packages. Once the clusters were calculated and each sampling site assigned, the Shapiro-Wilk test and F test statistic were applied to check if the variables were normally distributed and the homogeneity of variances. We tested the significance of the difference between groups for each variable using a Welch's t-test or Yuen's trimmed mean test (53) if homogeneity of variances or normality is violated.
Using the community species matrix, we calculated the sampling site scores derived from the primary reciprocal averaging axis (50, 54). These scores can be interpreted as a latent environmental gradient along which species distributions are structured. The non-parametric Spearman's rank correlation coefficient was used to test the association between sampling site scores and landscape values of each variable. To evaluate the variables most strongly associated with the variance of the observed communities, we applied redundancy analysis (RDA, a multiple linear regression model that accounts for multispecies response data and multiple explanatory variables) on the Hellinger-transformed abundance data and after removing singletons (species collected in 1 sampling site).
Finally, permutational multivariate analysis of variance (PERMANOVA); (55) with 999 permutations was used to test the effect of landscape units (agriculture, forested, wetlands and urban) on Culicidae relative abundance using the function “adonis” in the package vegan. The multivariate homogeneity of group dispersions (PERMDISP) was tested using the function “betadisper”.
All statistical analyses were conducted in R version 4.0.5 (41).
An overview of the data analyses is presented in Figure 1 and the location of sampling sites in Figure 2.
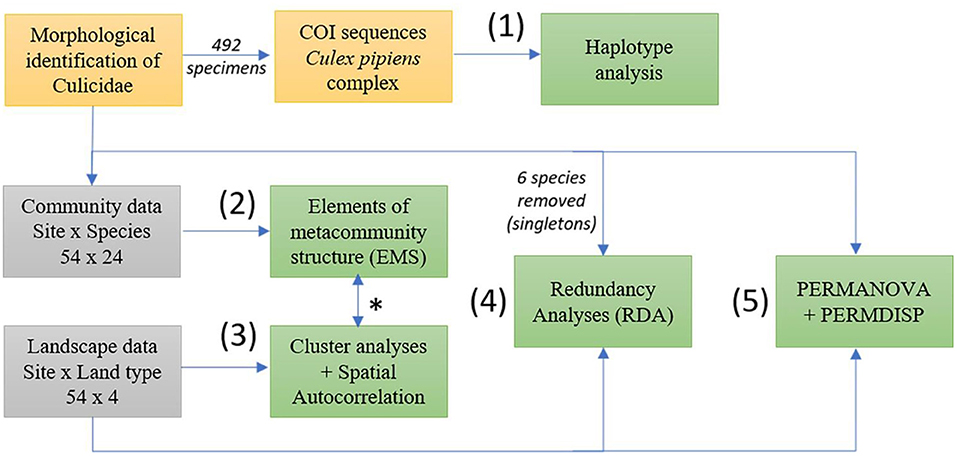
Figure 1. Scheme of the statistical analyses (boxes in green) used in this study. Mosquito and landscape data were collected in 54 sampling sites in south-eastern Illinois in 2016 and 2017. Individuals of Culicidae were identified using morphological characters and a subsample of 492 specimens belonging to Culex pipiens complex were further analyzed molecularly (boxes in yellow box), haplotype network analysis was applied to evaluate their genetic distance (1). Community data (box in gray) were used to identify metacommunity properties emerging from the observed structure (2). Landscape data (box in gray) informed cluster analyses, verify spatial autocorrelation of sites (3) and the correlation with sampling site scores from community data (*). Redundancy analysis with forward selection was performed to select the most important environmental variables that explain variation in the community matrices (4). PERMANOVA and PERMDISP were used to compare the variance and homogeneity of environmental and community distances between communities collected in different landscape units (5).
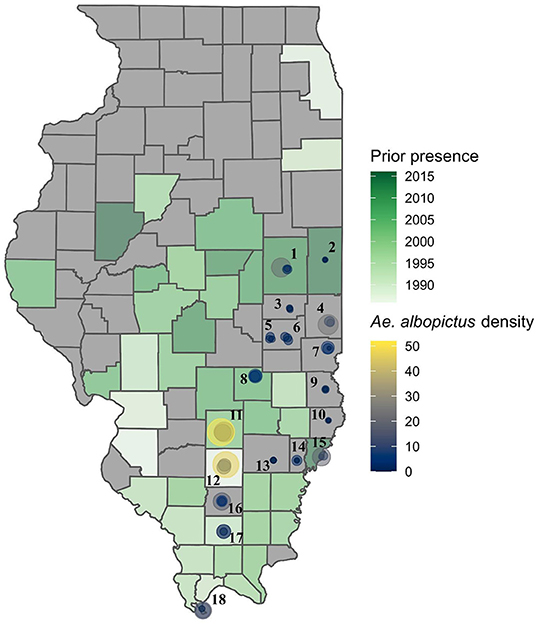
Figure 2. Sites within southern and eastern Illinois where mosquito surveys were performed in 2016 and 2017, first year of recorded occurrence of Aedes albopictus by county, and the mean density per trap per night for the sampling sites. Numbered sites: 1-Champaign; 2-Danville; 3-Tuscola; 4-Paris; 5-Mattoon; 6-Charleston; 7-Marshall; 8-Effingham; 9-Robinson; 10-Lawrenceville; 11-Salem; 12-Mt. Vernon; 13-Fairfield; 14-Albion; 15-Mt. Carmel; 16-Benton; 17-Marion; 18-Cairo.
Results
Species Composition, Abundance and Haplotypes
During the 2-years of surveys of known and potential vectors of arboviruses in Illinois a total of 24 species of Culicidae belonging to the genera Aedes (9 species), Anopheles (4), Culex (at least 3 species), Orthopodomyia (1), Psorophora (5), Toxorhynchites (1), and Uranotaenia (1) were collected (Supplementary Table 1). Four species (Aedes albopictus, Ae. canadensis, Anopheles punctipennis, and Ae. vexans) and the Cx. pipiens complex were present in most of the sites and most abundant (Supplementary Table 1, Supplementary Figure 1). Notably, Ae. albopictus was recorded from each county in which we sampled, including nine counties for which no prior occurrence record of this species existed (Figure 2), suggesting a wider patterns of spread and establishment of this invasive species throughout southern and central Illinois than previously realized.
For both specific vectors of interest (Ae. albopictus and Culex spp.) and mosquito species combined there were large differences in average abundance between sampling sites. To understand how these differences relate to overall species richness, a measure of biodiversity, we explored these relations by fitting varying functional forms to the data. For total mosquito (all species combined) abundance, this relationship was best described by a power function, suggesting that in areas with more diverse mosquito communities, the overall abundance of mosquitoes is also greater (Figure 3).
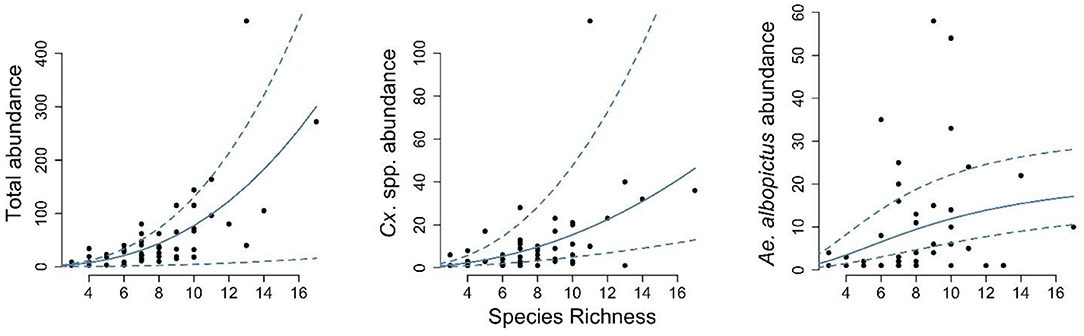
Figure 3. The relation between mosquito species richness and mosquito abundance. For each sampling site, the species richness over the entire season is compared the total mosquito abundance (left panel), the abundance of Culex pipiens complex (middle panel), and the abundance of Aedes albopictus (right panel). The solid blue lines represent the best fit, estimated using maximum likelihood, and the dashed lines the associated 95% confidence interval.
The same functional form gave the best fit for the relation between species richness and Culex spp. (i.e., Cx. pipiens complex / Cx. restuans) abundance specifically. Greater numbers of Cx. pipiens were found in more diverse trap locations, and the relation between these factors was well-described by a similar power function. This indicates that mosquito species richness in an area could predict Cx. pipiens complex abundance. For Ae. albopictus, however, a different pattern was found, with the highest levels of Ae. albopictus abundance being associated with locations with moderate levels of species richness, and a Holling type III gave the best fit to these data (Figure 3).
A total of 492 individuals in the Cx. pipiens complex, collected in 2016 and 2017, were subjected to molecular analyses. The sequences were processed in BLASTN (GenBank) and showed an identity value higher than 97% and a matching length (query length × coverage) longer than 600 bp compared with the reference sequences belonging to species in Cx. pipiens complex. The final alignment of COI sequences represents 492 samples and contains 686 nucleotide positions. However, the best tree of the maximum likelihood analysis is not informative for the identification of the samples due to the overall low branch support values (tree not shown). Therefore, we further performed haplotype analyses. The statistical parsimony network calculated with TCS recovered one single network with 7 haplotypes (Ha1: 455 specimens, Ha2: 37, Ha3: 2, Ha4-Ha7: 1, Supplementary Table 2) which vary in six substitutions (Supplementary Figure 2). Haplotype diversity (Hd = 0.162 ± 0.022), nucleotide diversity (n = 0.0004), negative Tajima's D (−1.53) suggested bottleneck or a selective sweep. The most dominant mitochondrial COI haplotype in the analyzed material was Ha1, shared by 455 specimens of 492. Four haplotypes had one specimen only.
Community of Culicidae and Landscape Variables
The community of Culicidae in central-south Illinois showed significant positive coherence, suggesting it is not well described by random or checkerboard processes. Additionally, turnover of species assemblages was significantly positive, and there was significant positive boundary clumping (Table 1). A pattern with these combinations of traits can be described as a Clementsian metacommunity, which indicates that the environmental drivers of community composition led to discrete groups of species which replace each other in a clumped or groupwise fashion (34). Because such patterns are often associated with landscape and ecotonal effects, rather than smooth gradients (e.g., related to temperature or elevation), we focused on land use for the remainder of the analysis.
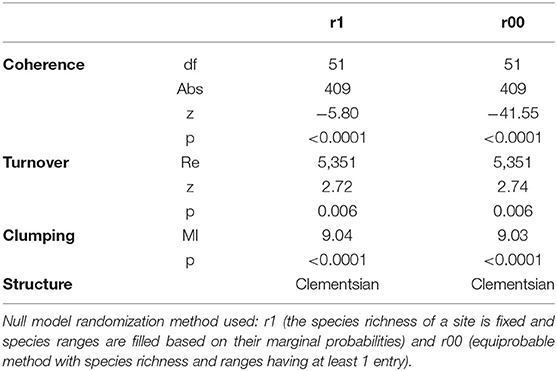
Table 1. Elements of metacommunity structure (EMS) data for mosquitoes (Culicidae) collected in south-eastern Illinois.
We identified and characterized 2 main clusters of sampling sites with distinct landscape composition. The average silhouette metric peaked at two clusters and the k-means classification regrouped 21 (group 1) and 33 (group 2) sampling sites. Interestingly, almost all sampling sites belonging to the same location grouped together except for Salem-16 (in group 1), Charleston-7 (in group 2), Paris-36 (in group 2), Marshall-39 (in group 1), and Mt_Carmel-47 (in group 1) denoting a high degree of landscape variability among closest sampling sites (Figure 4). Group 1 included 33 sampling sites and was characterized by urban settlements, group 2 (21 sampling sites) was dominated by agricultural settlements (mainly annual crops, such as corn and soybean).
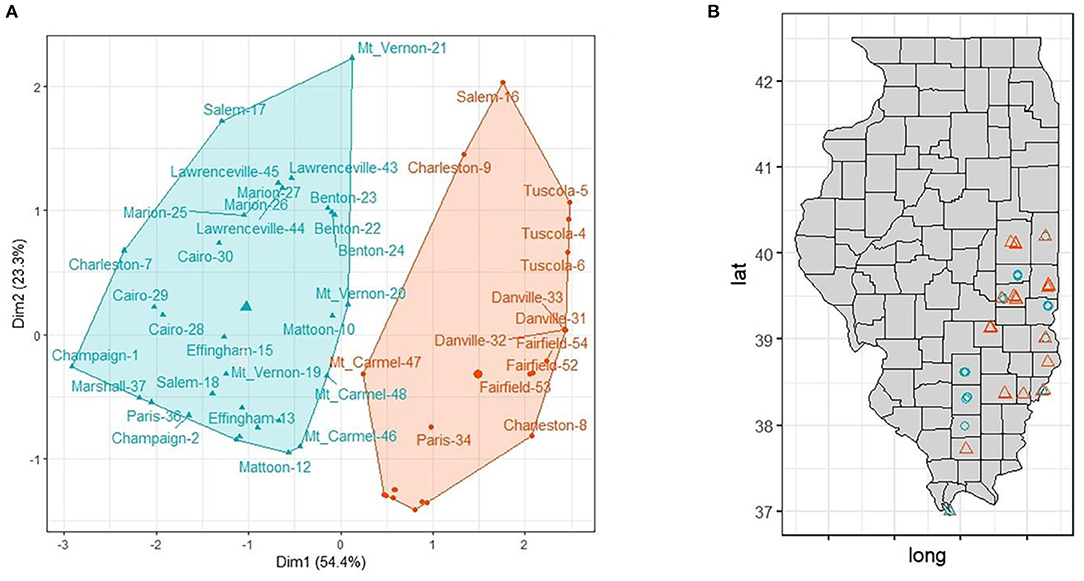
Figure 4. (A) Cluster analysis separated sampling sites in two main groups based on landscape variables (agriculture, wetlands, forested and urban). (B) Distribution of the of sampling sites in Illinois. Group 1 (n = 21): orange triangle; Group 2 (n = 33): blue circle.
The comparison between the two groups showed that there is a significant difference among all variables (agriculture, urban and forested; p < 0.0001) except for wetland (p = 0.77) (Table 2).
Spatial autocorrelation of each landscape variable was investigated using Moran's I and comparisons against null simulations. Overall, a significant positive spatial autocorrelation (Moran's I varying from 0.52 to 0.71, p < 0.001) was observed revealing that each landscape unit type was more clustered on the landscape than would be expected by chance (Supplementary Table 3).
When considering the correlation between sampling site scores (which summarize the mosquito community in each site) and each landscape variable there was a moderate positive correlation with agriculture and forested variables (rho = 0.26, p = 0.059 and rho = 0.27, p =0.049) and negative correlation with urban and wetlands (rho = −0.26, p = 0.056 and rho= −0.20, p = 0.141). To evaluate these associations in the multivariate space, we further investigated the effect of landscape variables using RDA.
The full RDA model for landscape variables was significant for the community composition of culicids (p = 0.02) explaining about 14% of the observed variation. The first constrained axis (RDA1) explains 7% of the variance, while the second (RDA2) explains 6%. The community of culicids across the different sampling sites were characterized by 4 species or group of species: Ae. canadensis, Cx. pipiens complex, Ae. albopictus, Ae. vexans. The first two are associated with the first RDA axis (−0.39 and 0.27 species scores, respectively) which is highly correlated with proportion of wetlands in the surrounding landscape. Higher values of wetlands favor presence and abundance of Ae. canadensis, whereas lower values favor Cx. pipiens complex. The second RDA axis showed positive relation between Ae. albopictus and higher values of urban settlements and between Ae. vexans and agriculture (0.32 and −0.28 species scores) (Figure 5).
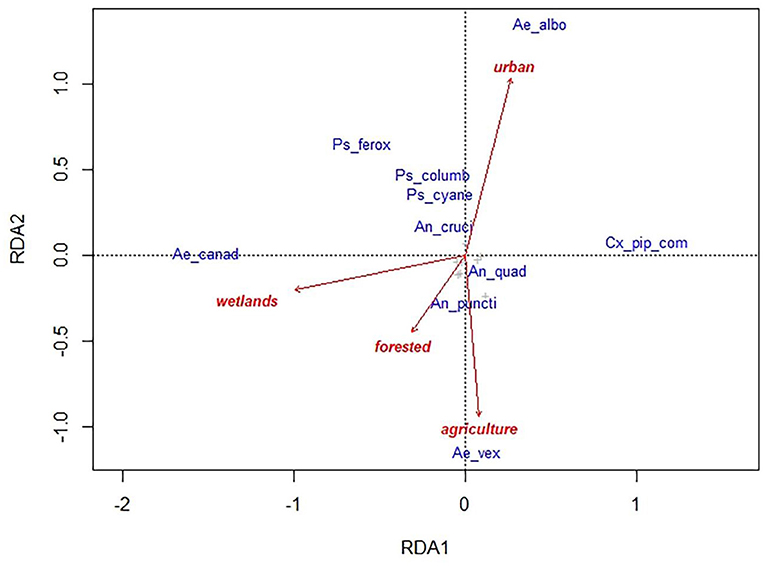
Figure 5. Redundancy analyses (RDA) ordination diagram for culicid species (in blue) and landscape variables (arrows in red) data collected in 54 sampling sites in Illinois. The full model explained 14% of the total variance, the first two constrained axis explained 7% (RDA1) and 6% (RDA2). Ae_canad, Aedes canadensis; Ae_albo, Ae. albopictus; Cx_pip_com, Culex pipiens complex; Ae_vex, Ae. vexans; Ps_ferox, Psorophora ferox; Ps_columb, Ps. columbie; Ps_cyane, Ps. cyanescens; An_cruci, Anopheles crucians; An_quad, An. quadrimaculatus; An_puncti, An. punctipennis.
The three factors: sampling sites, collecting period (4 months from July to October) and landscape, were considered altogether in the same permutational model (PERMANOVA). The community composition (a total of 24 species excluding singletons) of culicids was significantly affected by collecting period (p = 0.001) and landscape (p = 0.005), while the interaction between factors was not significant (Table 3). Analyses of the homogeneity of variance (PERMDISP) showed significant differences in community dispersion among farthest sampling sites (p = 0.019; Table 3). The difference between collecting periods (all combinations between months) and between landscape cluster groups (1 and 2) was not significant (Table 3). Because one of the assumptions for PERMANOVA, i.e., homogeneous dispersion, is fulfilled for periods and landscape, we can infer that the effect of these two variables on communities of culicids is reliable and not an artifact of heterogeneous dispersions.
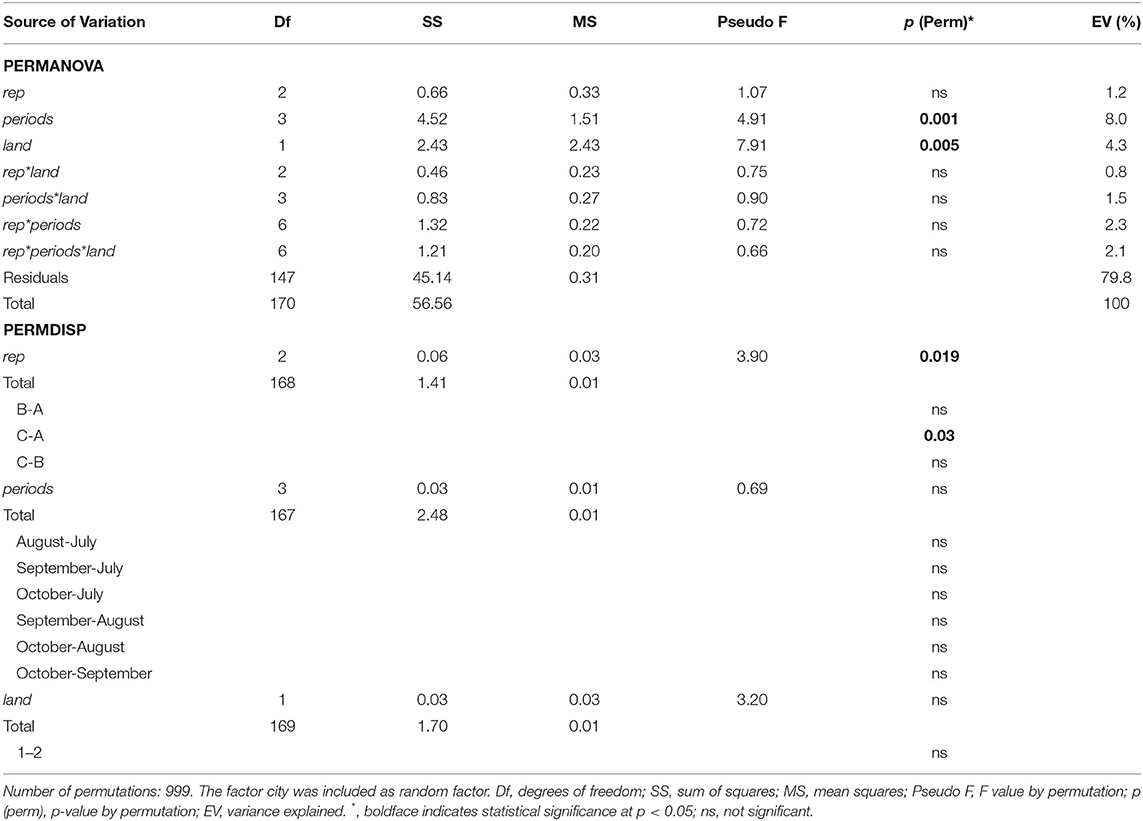
Table 3. PERMANOVA and PERMDISP analysis of the effect of between groups of farthest sampling sites (3 “rep” for each city), collecting period (4 “periods” - from July to October), and landscape (cluster group 1 and 2 “land”) on community composition of Culicidae collected in central-south Illinois in 2016–2017.
Discussion
There is a growing recognition for the need to anticipate, prepare for, and prevent emerging infectious diseases (EIDs) through action programs and initiatives such as those based on One Health approaches (56–58). The main thrust is to use a holistic method to consider the links among human, plant, animal health and the environment, with a focus on understanding how anthropogenic pressures on the environment (e.g., land use change or urbanization) shape the threat of EIDs. A recent formalized strategic protocol (DAMA, Document-Assess-Monitor-Act) to prevent EIDs proposed actionable information to anticipate outbreaks (59), and assessing the risk space requires the evaluation of the interfaces among landscape types (17). In the context of mosquito-borne diseases, information about the influence of landscape type on community composition and the prevalence of mosquito species of particular interest are of pivotal importance to predict emergence and spread of zoonotic diseases. The diversity and species composition of vector communities can play an important role in shaping the transmission intensity of vector-borne pathogens (30, 60, 61), yet our understanding of the environmental factors that shape mosquito communities is still relatively limited. This is particularly true when considering how invasive species fit within existing assemblages in areas of range expansion, yet such situations may be particularly important when considering potential emergence of novel or neglected pathogens.
To better understand the patterns and processes behind the overall mosquito metacommunity in our study, i.e., how species assemblages vary among sites, we employed the elements of metacommunity structure analysis framework. This suggests that these assemblages are Clementsian, i.e specific groups of species characterize discrete units of landscape types. One of the elements, coherence, which was significant and positive, suggests that these assemblages are distinctly non-random, and are not well-described as checkerboard patterns, which could occur if competitive interactions dominate. They are also characterized by a significant positive amount of turnover, which suggests that there are unique, differing assemblages throughout the landscape, rather than nested subsets (which would point toward, for instance, a process whereby a full community exists in pristine habitats, from which species are lost as the habitat degrades). Finally, the community is described as having clumped boundaries, suggesting that small groups of species change or are lost with changes in the environment. A gradual loss or turnover of species is typically associated with environmental gradients such as temperature or elevation as shown for other communities [e.g., (62)], which does not appear to be the main driver of the communities in this region. Instead, such clumped turnover can be associated with changes in land use and in particular with ecotonal landscapes, i.e., interfaces among habitat types (35). Due to this, as well as significant correlation between the ordinated site scores and different land use classes, our results further indicate metacommunities shaped by habitat preferences with species in each community showing similar responses to environmental gradients that replace each other across space. Ecotones have previously been associated with high abundance of Cx. tarsalis, and can be used by mosquitoes as flight paths and for host seeking (63, 64). Such interfaces between distinct land use types can thus both mark transitions between distinct habitats harboring their own communities, but also provide a unique habitat within which specific mosquito assemblages can be associated (18). The consideration of habitat interfaces and fragmentation (potentially associated with agricultural use or urban development) was not incorporated directly in this study but is a promising direction for future research.
Our PERMANOVA and RDA analyses highlighted that different species were most significantly associated with different proportions of land use. Aedes canadensis was associated with areas with a higher degree of land characterized as wetlands, while the Cx. pipiens complex was associated with environments with low levels of wetlands. Aedes vexans was most strongly associated with agricultural areas, while Ae. albopictus was most associated with urban or developed lands. The latter fits with habitat associations from single species evaluations [e.g., (65)].
Other studies that have investigated the effect of landscape on community composition of Culicidae have likewise found that landscape type affects the structure of mosquito communities (66). In the same study, a non-significant effect of sampling period was reported which was probably due to the climatic conditions of the subtropical zone. In our study the sampling periods have a significant effect suggesting the importance for climatic factors to be included in prediction models in areas where constraints such as temperature fluctuations may affect growth rates of populations.
It has been suggested that the type of discrete communities associated with a Clementsian metacommunity structure could limit spread of pathogens over the landscape due to a partitioning of competent reservoir hosts or vectors (37). For vector-borne diseases, this prediction remains to be tested and was beyond the scope of the current study. Limiting transmission to specific habitats or seasons is most likely to be relevant for vector-borne pathogen systems that rely on multiple amplifying and possible bridge vector species. Seasonal changes in metacommunity structure are also likely to be of importance, as they may further partition competent vectors, and the importance of bridge vector species in terms of risk of exposure depends on when their seasonal peak occurs in relation to that of amplifying vectors.
A limitation to the use of metacommunity analysis based on species (co-)occurrence is that variation in population size or abundance of different species is not considered directly. Two important vectors, Ae. albopictus, and Cx. pipiens, for instance, were broadly distributed, but the way species-specific abundance relies on the species assemblage in which they are embedded could provide additional important insights.
Our analysis of mosquito abundance, i.e., all mosquito species combined, in relation to species richness reveals that it is well-described by a power function. Such a relationship could imply that environments with greater abundance of individual species result in a lower loss or local extinction (e.g., as a result of the habitat representing a larger continuous whole or placed in the landscape to be more receptive of introductions from nearby populations) of species and therefore a higher richness or could be the result of correlation with another factor (e.g., habitat productivity) (67). Interestingly, this relationship also held true for Cx. pipiens complex abundance, which increased according to a power function with overall species richness. For Ae. albopictus, abundance peaked at intermediate levels of species richness, suggesting that its population size is regulated through a different mechanism or that it thrives in distinct, more depauperate habitats. The latter has been reported elsewhere, for instance suggesting that suburban developments with age shift toward mosquito communities that are both dominated by Ae. albopictus and less diverse overall (32). Land cover disruption has been suggested as an important driver of the colonization of this species as well the biotic homogenization of the entire community of Culicidae (68). We were not able to test or differentiate between different processes that could lead to such outcomes [e.g., competitive displacement and competition between species (26, 69)], or factors that could affect the extent to which this occurs (e.g., seasonal shifting, microhabitat segregation).
The initial motivation for this study was the discovery and understanding the spatial spread, over other species of native Culicidae, of Ae. albopictus (Asian tiger mosquito), one of the most successful invasive species whose worldwide spread comes paired with significant concerns for human health (70). Our study revealed that Ae. albopictus was ubiquitously present in all communities in the current study, and notably was recorded in each county included in the surveillance effort, including nine for which prior to 2016 no occurrence record of this species in the state existed (39, 71) (Swanson, J., pers. comm.). These findings suggest either the continued expansion of this species' distribution throughout the state, or alternatively that it was already established but previously overlooked (e.g., due to use of traps that are less likely to pick up this species). We can infer that this species is now established throughout much of the southern half of the state. As Ae. albopictus is a highly competent vector for a wide range of arboviruses, the risk of arboviral outbreaks continues to pose a threat. West Nile virus is ubiquitous in Illinois and Ae. albopictus is, at least in the lab, a competent vector (72). It may also serve as a bridge vector for Eastern equine encephalitis virus (EEE) and serve as a vector for LACV (73). Understanding whether and how the expansion and increase of Ae. albopictus populations changes the landscape of arboviral transmission risk is thus an area in continuing need of research. Understanding the implications for vector control is likewise important. For instance, it was shown that co-occurring populations of Ae. triseriatus and Ae. albopictus cover a wider spatial and temporal range which makes targeted control measures more difficult (74).
Besides Ae. albopictus, the survey results indicated that at least 4 native species, Ae. vexans, Ae. canadensis, An. punctipennis and Cx. pipiens complex, were ubiquitously present in the surveyed communities. Although typically thought of as a nuisance biting mosquito, at least one study has shown that field-collected Ae. vexans are capable of transmitting Zika virus (75), and may also serve, in certain situations, as a bridge vector of WNV (22). As these species (and others, such as Ae. japonicus and Ae. triseriatus) can interact with Ae. albopictus, it will be important to understand whether their role in transmission of WNV, LACV, or other arboviruses, is altered as a result of their ecological interactions. This points to the importance of baseline information on likely co-occurrence of different species along a landscape gradient.
In our study, preferences for landscape types (Figure 5) showed that the four species have specific preferences for different habitats with partial ecological niche overlap. Similar results were obtained in other studies where anthropogenic habitats explained the presence of Cx. quinquefascaitus (76) and may be used as predictors to find WNV-positive mosquitoes (77).
A challenge in assessments of species assemblages relates to the existence of cryptic, co-existing species and hybrids. The Cx. pipiens complex in southern Illinois has previously been shown to fall within the region where Cx. pipiens and Cx. pipiens–Cx. quinquefasciatus hybrids co-occur (78). Individuals of the complex are very similar morphologically, and mitochondrial and nuclear markers are often used to characterize genetic diversity of cryptic species [e.g., (79)]. Additionally, another WNV vector present in this area, Cx. restuans, can be challenging to distinguish morphologically (80) from Cx. pipiens and are often (as we did here) reported as Culex spp., rather than as distinct species. To obtain insight into the genetic diversity within these samples, we sequenced a barcode region for a subset of specimens that were only identified to genus level. Specimens analyzed in this study showed a low genetic variability as noted in previous studies conducted in other countries (81, 82). Notably, all specimens analyzed in BLASTN database matched Cx. pipiens complex, suggesting that we may have not collected specimens of Cx. restuans or a very low presence within these samples. Whether this reflects the true composition in these environments, reflects the time of season of the collections (not starting until July) due to the earlier seasonal peak in Cx. restuans (83), or a bias related to the type of traps used is unclear. Our analysis identified one single haplotype that was dominant throughout the region, and a second, less common, haplotype (37 specimens out of 492) that was primarily found in the southernmost tip of the state, in Cairo, IL. We may speculate these specimens belong to Cx. quinquefasciatus as they match a haplotype identified as such in an earlier study, although further research evaluating the structure of this complex in Illinois in relation to land use is needed (84). One explanation for the dominance of one single haplotype could be related to the single sampling technique used or to the low coverage of natural habitats [e.g., (85)]. The reliance on BG Sentinel traps is also likely to have limited the species diversity in our samples by reducing the likelihood of sampling mosquito species that are not likely to be picked up in these traps (although the addition of dry ice as a CO2 source may have reduced this bias), and for future studies the use of multiple trap types to allow for sampling a broader diversity of the mosquito community is encouraged.
To conclude, an important invasive mosquito species, Ae. albopictus, is more widespread in Illinois than was previously realized. Using a number of analyses related to mosquito community ecology, we show that this species is particularly common in urban environments, and in areas with low to moderate species diversity. Questions that remain are for instance whether competition for resources in such urban environments negatively affects other mosquito species, and thereby shifts or changes the assemblage as this species invades. Information on species assemblages and how they change with land use inform potential risk assessments [e.g., (17)] for introductions of novel pathogens or (re-) emergence of diseases, and we recommend that such assessments of mosquito species assemblages and diversity be considered within One Health surveillance approaches.
Data Availability Statement
The dataset of the cytochrome c oxidase subunit I (COI) mitochondrial gene sequences and the original final sequence alignment used in the haplotype analyses for this study can be found in the Illinois Data Bank (DOI: 10.13012/B2IDB-5032907_V1). Other data presented in the study are included in the article/Supplementary Material.
Author Contributions
VT and CS drafted the paper, developed the data analysis, and led the writing of the manuscript. VT performed the statistical multivariate analyses and haplotype analyses. C-HK designed the field study. All authors critically reviewed, discussed, modified the manuscript, contributed to the article, and approved the submitted version.
Funding
This study was funded by the State of Illinois Used Tire Management and Emergency Public Health funds and through an agreement with the Illinois Department of Public Health. Points of view or opinions expressed in this document are those of the authors and do not necessarily represent the official position or policies of the Illinois Department of Public Health.
Conflict of Interest
The authors declare that the research was conducted in the absence of any commercial or financial relationships that could be construed as a potential conflict of interest.
Publisher's Note
All claims expressed in this article are solely those of the authors and do not necessarily represent those of their affiliated organizations, or those of the publisher, the editors and the reviewers. Any product that may be evaluated in this article, or claim that may be made by its manufacturer, is not guaranteed or endorsed by the publisher.
Acknowledgments
DaTeng Lin, Nadia Khan, Dhara Patel and Seth Yates provided technical assistance with collecting and processing of mosquitoes. We thank Andrew Park for discussions regarding metacommunity analysis, and are grateful to the three reviewers for their helpful comments.
Supplementary Material
The Supplementary Material for this article can be found online at: https://www.frontiersin.org/articles/10.3389/fpubh.2022.872812/full#supplementary-material
References
1. Lowe A-M, Forest-Bérard K, Trudel R, Lo E, Gamache P, Tandonnet M, et al. Mosquitoes know no borders: surveillance of potential introduction of Aedes species in Southern Québec, Canada. Pathog Basel Switz. (2021) 10:998. doi: 10.3390/pathogens10080998
2. Schaffner F, Medlock JM, Bortel WV. Public health significance of invasive mosquitoes in Europe. Clin Microbiol Infect. (2013) 19:685–92. doi: 10.1111/1469-0691.12189
3. Juliano SA, Lounibos LP. Ecology of invasive mosquitoes: effects on resident species and on human health. Ecol Lett. (2005) 8:558–74. doi: 10.1111/j.1461-0248.2005.00755.x
4. Ligon BL. Reemergence of an unusual disease: the chikungunya epidemic. Semin Pediatr Infect Dis. (2006) 17:99–104. doi: 10.1053/j.spid.2006.04.009
5. Charrel RN, de Lamballerie X, Raoult D. Chikungunya outbreaks–the globalization of vectorborne diseases. N Engl J Med. (2007) 356:769–71. doi: 10.1056/NEJMp078013
6. Grard G, Caron M, Mombo IM, Nkoghe D, Mboui Ondo S, Jiolle D, et al. Zika virus in Gabon (Central Africa)−2007: a new threat from Aedes albopictus? PLoS Negl Trop Dis. (2014) 8:e2681. doi: 10.1371/journal.pntd.0002681
7. Marini G, Guzzetta G, Rosà R, Merler S. First outbreak of Zika virus in the continental United States: a modelling analysis. Eurosurveillance. (2017) 22:30612. doi: 10.2807/1560-7917.ES.2017.22.37.30612
8. Lindsey NP, Staples JE, Fischer M. Chikungunya virus disease among travelers—United States, 2014–2016. Am J Trop Med Hyg. (2018) 98:192–7. doi: 10.4269/ajtmh.17-0668
9. Adams LE, Martin SW, Lindsey NP, Lehman JA, Rivera A, Kolsin J, et al. Epidemiology of dengue, chikungunya, and Zika virus disease in U.S. states and territories, 2017. Am J Trop Med Hyg. (2019) 101:884–90. doi: 10.4269/ajtmh.19-0309
10. Heymann DL, Hodgson A, Sall AA, Freedman DO, Staples JE, Althabe F, et al. Zika virus and microcephaly: why is this situation a PHEIC? Lancet. (2016) 387:719–21. doi: 10.1016/S0140-6736(16)00320-2
11. Mlakar J, Korva M, Tul N, Popović M, Poljšak-Prijatelj M, Mraz J, et al. Zika virus associated with microcephaly. N Engl J Med. (2016) 374:951–8. doi: 10.1056/NEJMoa1600651
12. McJunkin JE, de los Reyes EC, Irazuzta JE, Caceres MJ, Khan RR, Minnich LL, et al. La Crosse encephalitis in children. N Engl J Med. (2001) 344:801–7. doi: 10.1056/NEJM200103153441103
13. Leisnham PT, Juliano SA. Impacts of climate, land use, and biological invasion on the ecology of immature Aedes mosquitoes: implications for la Crosse emergence. Ecohealth. (2012) 9:217–28. doi: 10.1007/s10393-012-0773-7
14. Harris MC, Dotseth EJ, Jackson BT, Zink SD, Marek PE, Kramer LD, et al. La Crosse virus in Aedes japonicus japonicus mosquitoes in the Appalachian Region, United States. Emerg Infect Dis. (2015) 21:646–9. doi: 10.3201/eid2104.140734
15. Connelly CR. Continuation of mosquito surveillance and control during public health emergencies and natural disasters. Morb Mortal Wkly Rep. (2020) 69:938–40. doi: 10.15585/mmwr.mm6928a6
16. Zittra C, Vitecek S, Obwaller AG, Rossiter H, Eigner B, Zechmeister T, et al. Landscape structure affects distribution of potential disease vectors (Diptera: Culicidae). Parasit Vectors. (2017) 10:205. doi: 10.1186/s13071-017-2140-6
17. Trivellone V, Hoberg EP, Boeger WA, Brooks DR. Food security and emerging infectious disease: risk assessment and risk management. R Soc Open Sci. (2022) 1:1–8. doi: 10.1098/rsos.211687
18. Reiskind MH, Griffin RH, Janairo MS, Hopperstad KA. Mosquitoes of field and forest: the scale of habitat segregation in a diverse mosquito assemblage. Med Vet Entomol. (2017) 31:44–54. doi: 10.1111/mve.12193
19. Bradley DJ. Watson, Swellengrebel and species sanitation: environmental and ecological aspects. Parassitologia. (1994) 36:137–47.
20. Takken W, Snellen WB, Verhave JP, Knols BGJ, Atmosoedjono S. Environmental measures for malaria control in Indonesia. A historical review on species sanitation. Wageningen Agricultural University. Wageningen, Netherlands: Veenman Drukkers (1990). p. 16. Available online at: https://scholar.google.com/scholar_lookup?title=Environmental+measures+for+malaria+control+in+Indonesia.+A+historical+review+on+species+sanitation.&author=Takken%2C+W.&publication_year=1990 (accessed March 6, 2022).
21. Ruiz-Arrondo I, Hernández-Triana LM, Nikolova NI, Fooks AR, Oteo JA. Integrated approaches in support of taxonomic identification of mosquitoes (Diptera: Culicidae) in vector surveillance in Spain. Vector-Borne Zoonotic Dis. (2020) 20:831–42. doi: 10.1089/vbz.2020.2662
22. Kilpatrick AM, Kramer LD, Campbell SR, Alleyne EO, Dobson AP, Daszak P. West Nile virus risk assessment and the bridge vector paradigm. Emerg Infect Dis. (2005) 11:425–9. doi: 10.3201/eid1103.040364
23. Little EAH, Hutchinson ML, Price KJ, Marini A, Shepard JJ, Molaei G. Spatiotemporal distribution, abundance, and host interactions of two invasive vectors of arboviruses, Aedes albopictus and Aedes japonicus, in Pennsylvania, USA. Parasit Vectors. (2022) 15:36. doi: 10.1186/s13071-022-05151-8
24. Costanzo KS, Mormann K, Juliano SA. Asymmetrical Competition and Patterns of Abundance of Aedes albopictus and Culex pipiens (Diptera: Culicidae). J Med Entomol. (2005) 42:559–70. doi: 10.1603/0022-2585(2005)042<0559:ACAPOA>2.0.CO;2
25. Costanzo KS, Muturi EJ, Lampman RL, Alto BW. The effects of resource type and ratio on competition with Aedes albopictus and Culex pipiens (Diptera: Culicidae). J Med Entomol. (2011) 48:29–38. doi: 10.1603/ME10085
26. Leisnham PT, LaDeau SL, Saunders MEM, Villena OC. Condition-specific competitive effects of the invasive mosquito Aedes albopictus on the resident Culex pipiens among different urban container habitats may explain their coexistence in the field. Insects. (2021) 12:993. doi: 10.3390/insects12110993
27. Evans MV, Dallas TA, Han BA, Murdock CC, Drake JM. Data-driven identification of potential Zika virus vectors. Elife. (2017) 6:e22053. doi: 10.7554/eLife.22053
28. Gleiser RM, Zalazar LP. Distribution of mosquitoes in relation to urban landscape characteristics. Bull Entomol Res. (2010) 100:153–8. doi: 10.1017/S0007485309006919
29. Roche B, Rohani P, Dobson AP, Guégan J-F. The impact of community organization on vector-borne pathogens. Am Nat. (2013) 181:1–11. doi: 10.1086/668591
30. Spence Beaulieu MR, Federico JL, Reiskind MH. Mosquito diversity and dog heartworm prevalence in suburban areas. Parasit Vectors. (2020) 13:12. doi: 10.1186/s13071-019-3874-0
31. Gardner AM, Lampman RL, Muturi EJ. Land use patterns and the risk of West Nile virus transmission in central Illinois. Vector-Borne Zoonotic Dis. (2014) 14:338–45. doi: 10.1089/vbz.2013.1477
32. Beaulieu MRS, Hopperstad K, Dunn RR, Reiskind MH. Simplification of vector communities during suburban succession. PLoS ONE. (2019) 14:e0215485. doi: 10.1371/journal.pone.0215485
33. Wilke ABB, Vasquez C, Carvajal A, Moreno M, Fuller DO, Cardenas G, et al. Urbanization favors the proliferation of Aedes aegypti and Culex quiquefasciatus in urban areas of Miami-Dade County, Florida. Sci Rep. (2021) 11:22989. doi: 10.1038/s41598-021-02061-0
34. Leibold MA, Mikkelson GM. Coherence, species turnover, and boundary clumping: elements of meta-community structure. Oikos. (2002) 97:237–50. doi: 10.1034/j.1600-0706.2002.970210.x
35. Presley SJ, Higgins CL, Willig MR. A comprehensive framework for the evaluation of metacommunity structure. Oikos. (2010) 119:908–17. doi: 10.1111/j.1600-0706.2010.18544.x
36. Grenfell B, Harwood J. (Meta)population dynamics of infectious diseases. Trends Ecol Evol. (1997) 12:395–9. doi: 10.1016/S0169-5347(97)01174-9
37. Suzán G, García-Peña GE, Castro-Arellano I, Rico O, Rubio AV, Tolsá MJ, et al. Metacommunity and phylogenetic structure determine wildlife and zoonotic infectious disease patterns in time and space. Ecol Evol. (2015) 5:865–73. doi: 10.1002/ece3.1404
38. Brooks DR, McLennan DA. The Nature of Diversity: An Evolutionary Voyage of Discovery. Chicago, IL: University of Chicago Press (2002). p. 676. Available online at: https://press.uchicago.edu/ucp/books/book/chicago/N/bo3622396.html (accessed March 2, 2022).
39. Stone CM, Zuo Z, Li B, Ruiz M, Swanson J, Hunt J, et al. Spatial, temporal, and genetic invasion dynamics of Aedes albopictus (Diptera: Culicidae) in Illinois. J Med Entomol. (2020) 57:1488–500. doi: 10.1093/jme/tjaa047
40. Illinois Natural History Survey. Illinois Gap Analysis Program Land Cover Classification-Land & Water. Ill Dep Agric (2003). Available online at: https://www2.illinois.gov/sites/agr/Resources/LandWater/Pages/Illinois-Gap-Analysis-Program-Land-Cover-Classification.aspx (accessed February 7, 2022).
41. R Core Team. R: A language environment for statistical computing. Vienna, Austria: R Foundation for Statistical Computing (2020). Available online at: https://www.R-project.org/
42. Darsie RF, Ward RA. Identification and geographical distribution of the mosquitoes of North America, north of Mexico. Gainesville: University Press of Florida (2005).
43. Brugman V, Hernández-Triana L, Medlock J, Fooks A, Carpenter S, Johnson N. The role of Culex pipiens L (Diptera: Culicidae) in virus transmission in Europe. Int J Environ Res Public Health. (2018) 15:389. doi: 10.3390/ijerph15020389
44. Kumar NP, Rajavel AR, Natarajan R, Jambulingam P. DNA barcodes can distinguish species of Indian mosquitoes (Diptera: Culicidae). J Med Entomol. (2007) 44:01–7. doi: 10.1093/jmedent/41.5.01
45. Mirarab S, Nguyen N, Guo S, Wang L-S, Kim J, Warnow T, et al. Ultra-large multiple sequence alignment for nucleotide and amino-acid sequences. J Comput Biol. (2015) 22:377–86. doi: 10.1089/cmb.2014.0156
46. Rozas J, Ferrer-Mata A, Sánchez-DelBarrio JC, Guirao-Rico S, Librado P, Ramos-Onsins SE, et al. DnaSP 6: DNA sequence polymorphism analysis of large data sets. Mol Biol Evol. (2017) 34:3299–302. doi: 10.1093/molbev/msx248
47. Bandelt HJ, Forster P, Röhl A. Median-joining networks for inferring intraspecific phylogenies. Mol Biol Evol. (1999) 16:37–48. doi: 10.1093/oxfordjournals.molbev.a026036
48. Leigh JW, Bryant D. popart : full-feature software for haplotype network construction. Methods Ecol Evol. (2015) 6:1110–6. doi: 10.1111/2041-210X.12410
49. Bolker B. Ecological Models and Data in R. Princeton University Press (2008). p. 408. doi: 10.1515/9781400840908
50. Dallas T. metacom: an R package for the analysis of metacommunity structure. Ecography. (2014) 37:402–5. doi: 10.1111/j.1600-0587.2013.00695.x
51. Moran PAP. Notes on continuous stochastic phenomena. Biometrika. (1950) 37:17–23. doi: 10.1093/biomet/37.1-2.17
52. Zwitch R. Clustering Search Keywords Using K-Means Clustering (2013). Available online at: https://www.r-bloggers.com/2013/09/clustering-search-keywords-using-k-means-clustering/ (accessed January 30, 2022).
53. Yuen KK. The two-sample trimmed t for unequal population variances. Biometrika. (1974) 61:165–70. doi: 10.1093/biomet/61.1.165
54. Gauch HG, Whittaker RH, Wentworth TR. A comparative study of reciprocal averaging and other ordination techniques. J Ecol. (1977) 65:157–74. doi: 10.2307/2259071
55. Anderson MJ. A new method for non-parametric multivariate analysis of variance: non-parametric MANOVA for ecology. Austral Ecol. (2001) 26:32–46. doi: 10.1111/j.1442-9993.2001.01070.pp.x
56. PREDICT Consortium, Kelly TR, Machalaba C, Karesh WB, Crook PZ, Gilardi K, et al. Implementing One Health approaches to confront emerging and re-emerging zoonotic disease threats: lessons from PREDICT. One Health Outlook. (2020) 2:1. doi: 10.1186/s42522-019-0007-9
57. Ellwanger JH, Veiga ABG da, Kaminski V de L, Valverde-Villegas JM, Freitas AWQ de, Chies JAB. Control and prevention of infectious diseases from a One Health perspective. Genet Mol Biol. (2021) 44:e20200256. doi: 10.1590/1678-4685-gmb-2020-0256
58. Reaser JK, Hunt BE, Ruiz-Aravena M, Tabor GM, Patz JA, Becker D, et al. Fostering landscape immunity to protect human health: a science-based rationale for shifting conservation policy paradigms. Conserv Lett. (2022). e12869. doi: 10.1111/conl.12869
59. Brooks DR, Hoberg EP, Boeger WA. The Stockholm Paradigm: Climate Change and Emerging Disease. Chicago: University of Chicago Press (2019). p. 423.
60. Park AW, Cleveland CA, Dallas TA, Corn JL. Vector species richness increases haemorrhagic disease prevalence through functional diversity modulating the duration of seasonal transmission. Parasitology. (2016) 143:874–9. doi: 10.1017/S0031182015000578
61. Hoi AG, Gilbert B, Mideo N. Deconstructing the impact of malaria vector diversity on disease risk. Am Nat. (2020) 196:E61–70. doi: 10.1086/710005
62. Willig MR, Presley SJ, Bloch CP, Castro-Arellano I, Cisneros LM, Higgins CL, et al. Tropical metacommunities along elevational gradients: effects of forest type and other environmental factors. Oikos. (2011) 120:1497–508. doi: 10.1111/j.1600-0706.2011.19218.x
63. Lothrop HD, Reisen WK. Landscape affects the host-seeking patterns of Culex tarsalis (Diptera: Culicidae) in the coachella valley of California. J Med Entomol. (2001) 38:325–32. doi: 10.1603/0022-2585-38.2.325
64. Reisen WK. Landscape epidemiology of vector-borne diseases. Annu Rev Entomol. (2010) 55:461–83. doi: 10.1146/annurev-ento-112408-085419
65. Kache PA, Eastwood G, Collins-Palmer K, Katz M, Falco RC, Bajwa WI, et al. Environmental determinants of Aedes albopictus abundance at a northern limit of its range in the United States. Am J Trop Med Hyg. (2020) 102:436–47. doi: 10.4269/ajtmh.19-0244
66. Giordano BV, Cruz A, Pérez-Ramos DW, Ramos MM, Tavares Y, Caragata EP. Mosquito communities vary across landscape and vertical strata in Indian River County, Florida. Pathogens. (2021) 10:1575. doi: 10.3390/pathogens10121575
67. Yee DA, Juliano SA. Abundance matters: a field experiment testing the more individuals hypothesis for richness–productivity relationships. Oecologia. (2007) 153:153–62. doi: 10.1007/s00442-007-0707-1
68. Wilke ABB, Benelli G, Beier JC. Beyond frontiers: on invasive alien mosquito species in America and Europe. PLoS Negl Trop Dis. (2020) 14:e0007864. doi: 10.1371/journal.pntd.0007864
69. Marini G, Guzzetta G, Baldacchino F, Arnoldi D, Montarsi F, Capelli G, et al. The effect of interspecific competition on the temporal dynamics of Aedes albopictus and Culex pipiens. Parasit Vectors. (2017) 10:102. doi: 10.1186/s13071-017-2041-8
70. Bonizzoni M, Gasperi G, Chen X, James AA. The invasive mosquito species Aedes albopictus: current knowledge and future perspectives. Trends Parasitol. (2013) 29:460–8. doi: 10.1016/j.pt.2013.07.003
71. Kraemer MUG, Sinka ME, Duda KA, Mylne A, Shearer FM, Brady OJ, et al. The global compendium of Aedes aegypti and Ae. albopictus occurrence. Sci Data. (2015) 2:150035. doi: 10.1038/sdata.2015.35
72. Sardelis MR, Turrell MJ, O'Guinn ML, Andre RG, Roberts DR. Vector competence of three North American strains of Aedes albopictus for West Nile virus. J Am Mosq Control Assoc. (2002) 18:284–9.
73. Gratz NG. Critical review of the vector status of Aedes albopictus. Med Vet Entomol. (2004) 18:215–27. doi: 10.1111/j.0269-283X.2004.00513.x
74. Rowe RD, Odoi A, Paulsen D, Moncayo AC, Fryxell RTT. Spatial-temporal clusters of host-seeking Aedes albopictus, Aedes japonicus, and Aedes triseriatus collections in a La Crosse virus endemic county (Knox County, Tennessee, USA). PLoS ONE. (2020) 15:e0237322. doi: 10.1371/journal.pone.0237322
75. O'Donnell K, Bixby M, Morin K, Bradley D, Vaughan J. Potential of a Northern Population of Aedes vexans (Diptera: Culicidae) to Transmit Zika Virus. J Med Entomol. (2017) 54:1354–9. doi: 10.1093/jme/tjx087
76. Reiter ME, Lapointe DA. Landscape factors influencing the spatial distribution and abundance of mosquito vector Culex quinquefasciatus (Diptera: Culicidae) in a mixed residential–agricultural community in Hawai‘I. J Med Entomol. (2007) 44:8. doi: 10.1603/0022-2585(2007)44<861:LFITSD>2.0.CO;2
77. Poh KC, Medeiros MCI, Hamer GL. Landscape and demographic determinants of Culex infection with West Nile virus during the 2012 epidemic in Dallas County, TX. Spat Spatio-Temporal Epidemiol. (2020) 33:100336. doi: 10.1016/j.sste.2020.100336
78. Kothera L, Zimmerman EM, Richards CM, Savage HM. Microsatellite characterization of subspecies and their hybrids in Culex pipiens complex (Diptera: Culicidae) mosquitoes along a north-south transect in the central United States. J Med Entomol. (2009) 46:236–48. doi: 10.1603/033.046.0208
79. Shaikevich EV PCR-RFLP. of the COI gene reliably differentiates Cx. pipiens, Cx. pipiens f molestus and Cx. torrentium of the pipiens complex. Eur Mosq Bull. (2007) 23:25–30.
80. Harrington LC, Poulson RL. Considerations for accurate identification of adult Culex restuans (Diptera: Culicidae) in field studies. J Med Entomol. (2008) 45:1–8. doi: 10.1603/0022-2585(2008)45<1:CFAIOA>2.0.CO;2
81. Pfeiler E, Flores-López CA, Mada-Vélez JG, Escalante-Verdugo J, Markow TA. Genetic Diversity and Population Genetics of Mosquitoes (Diptera: Culicidae: Culex spp.) from the sonoran desert of North America. Sci World J. (2013) 2013:e724609. doi: 10.1155/2013/724609
82. Werblow A, Klimpel S, Bolius S, Dorresteijn AWC, Sauer J, Melaun C. Population structure and distribution patterns of the sibling mosquito species Culex pipiens and Culex torrentium (Diptera: Culicidae) reveal different evolutionary paths. PLoS ONE. (2014) 9:e102158. doi: 10.1371/journal.pone.0102158
83. Lampman R, Slamecka M, Krasavin N, Kunkel K, Novak R. Culex population dynamics and west nile virus transmission in east-central Illinois. J Am Mosq Control Assoc. (2006) 22:390–400. doi: 10.2987/8756-971X(2006)22<390:CPDAWN>2.0.CO;2
84. Shaikevich EV, Vinogradova EB, Bouattour A, Gouveia de Almeida AP. Genetic diversity of Culex pipiens mosquitoes in distinct populations from Europe: contribution of Cx. quinquefasciatus in Mediterranean populations. Parasit Vectors. (2016) 9:47. doi: 10.1186/s13071-016-1333-8
Keywords: Aedes albopictus, biodiversity, Culicidae, land use, mosquito surveillance, species assemblage
Citation: Trivellone V, Cao Y, Blackshear M, Kim C-H and Stone C (2022) Landscape Composition Affects Elements of Metacommunity Structure for Culicidae Across South-Eastern Illinois. Front. Public Health 10:872812. doi: 10.3389/fpubh.2022.872812
Received: 10 February 2022; Accepted: 23 March 2022;
Published: 03 May 2022.
Edited by:
Francisco Collantes, University of Murcia, SpainReviewed by:
Gabriel Laporta, Faculdade de Medicina do ABC, BrazilShannon L. LaDeau, Cary Institute of Ecosystem Studies, United States
Ahmed Tabbabi, Jichi Medical University, Japan
Copyright © 2022 Trivellone, Cao, Blackshear, Kim and Stone. This is an open-access article distributed under the terms of the Creative Commons Attribution License (CC BY). The use, distribution or reproduction in other forums is permitted, provided the original author(s) and the copyright owner(s) are credited and that the original publication in this journal is cited, in accordance with accepted academic practice. No use, distribution or reproduction is permitted which does not comply with these terms.
*Correspondence: Valeria Trivellone, valeria3@illinois.edu; Christopher Stone, cstone@illinois.edu