- 1School of Geography and Planning, Sun Yat-sen University, Guangzhou, China
- 2Department of Scientific Research and Discipline Development, The First Affiliated Hospital of Sun Yat-sen University, Guangzhou, China
- 3State Key Laboratory of Earth Surface Processes and Resource Ecology, Beijing Normal University, Beijing, China
- 4Faculty of Geo-Information Science and Earth Observation (ITC), University of Twente, Enschede, Netherlands
- 5School of Civil and Environment Engineering, Georgia Institute of Technology, Atlanta, GA, United States
- 6National Tibetan Plateau Data Center, Beijing, China
Background: Epidemiological studies have widely proven the impact of ozone (O3) on respiratory mortality, while only a few studies compared the association between different O3 indicators and health.
Methods: This study explores the relationship between daily respiratory hospitalization and multiple ozone indicators in Guangzhou, China, from 2014 to 2018. It uses a time-stratified case–crossover design. Sensitivities of different age and gender groups were analyzed for the whole year, the warm and the cold periods. We compared the results from the single-day lag model and the moving average lag model.
Results: The results showed that the maximum daily 8 h average ozone concentration (MDA8 O3) had a significant effect on the daily respiratory hospitalization. This effect was stronger than for the maximum daily 1 h average ozone concentration (MDA1 O3). The results further showed that O3 was positively associated with daily respiratory hospitalization in the warm season, while there was a significantly negative association in the cold season. Specifically, in the warm season, O3 has the most significant effect at lag 4 day, with the odds ratio (OR) equal to 1.0096 [95% confidence intervals (CI): 1.0032, 1.0161]. Moreover, at the lag 5 day, the effect of O3 on the 15–60 age group was less than that on people older than 60 years, with the OR value of 1.0135 (95% CI: 1.0041, 1.0231) for the 60+ age group; women were more sensitive than men to O3 exposure, with an OR value equal to 1.0094 (95% CI: 0.9992, 1.0196) for the female group.
Conclusion: These results show that different O3 indicators measure different impacts on respiratory hospitalization admission. Their comparative analysis provided a more comprehensive insight into exploring associations between O3 exposure and respiratory health.
Introduction
Ozone (O3) is a secondary pollutant, forming through chemical reactions from precursors mainly including volatile organic compounds (VOCs) and nitrogen oxides (NOx). In recent years, O3 pollution has received increasing scientific attention due to the large number of environmental problems caused by rapid urbanization and industrial activities worldwide. Ozone (O3) exposure would trigger bronchial inflammation and respiratory tract oxidative stress, which further causes many serious health problems (1, 2) such as respiratory and lung-related diseases are very common (3–6).
Darrow et al. and Wise explored the relationship between O3 and respiratory health and provided their exposure-response coefficients but mainly in developed countries (7, 8). Developing countries, such as China and India, however, face severe O3 pollution and have a high population density (9, 10). Epidemiological studies about O3 concentration are still lacking, and therefore, there exists a lack of local exposure-response coefficients, resulting in large uncertainty in environmental health assessment. In addition, the relationship between O3 concentration and human health may vary across cities or regions because of differences in the nature and level of O3 pollution (10–12), and directly adopting the relationships established in developed countries to Chinese cities may result in large biases. It is, therefore, necessary to use local O3 concentration and health data to obtain local exposure-response coefficients.
With the improvement of the quality of China's air pollution monitoring data and increasing O3 concentrations (13), increasing attention can now be paid to the impact of O3 concentration on human health. Many epidemiological publications have confirmed that short-term O3 exposure is related to human health in China (4, 14, 15). However, few studies investigated how well the different O3 indicators (MDA8 O3 and MDA1 O3) measure the effects on human health. In fact, different O3 indicators have varying associations with human health (5, 16, 17). For example, Li et al. (16) used different O3 indicators to explore the impact of short-term O3 exposure on all-cause mortality in Guangzhou. Their results showed that MDA8 O3 was closely related to all-cause mortality, which was the key to study the impact of environmental O3 exposure on health (16). Yang et al. (17) examined the effect of three O3 indicators (MDA8 O3, MDA1 O3, and 24 h average O3) on daily mortality in Suzhou. They found that MDA8 O3 and MDA1 O3 were strongly associated with increased mortality than the 24 h average O3 (17). A cohort study by Abbey and Burchette investigated the impact of different O3 indicators on respiratory disease, and they found that MDA8 O3 provided the strongest impact on human health (18). As O3 concentration shows large diurnal and seasonal variation related to the variability in the release of O3 precursors, O3 indicators may be affected by spatial factors, such as region, urbanization, and population density, and temporal factors, such as season and weather. Therefore, in studies relating O3 to health, it is important to know how the O3 concentration is obtained. If the differences among O3 indicators are not considered appropriately, then this may lead to misleading health risk conclusions (16).
Previous studies examined the effects of short-term O3 exposure on daily all-cause, cardiovascular, and respiratory mortality (19–21), while a few studies addressed the problem that various O3 indicators were used to examine the relationship between different O3 indicators exposure and respiratory hospitalization.
To address this research issue, this study aimed (1) to conduct a time-stratified case–crossover model to explore the short-term effect of two O3 indicators (MDA8 O3 and MDA1 O3) on daily respiratory hospitalization in a single city; (2) to investigate whether the two O3 indicators show different relationships with daily respiratory hospitalization; and (3) to examine the associations between the two O3 indicators and daily respiratory hospitalization for different age, gender, and season groups. Then, the results of the single-day lag model and the moving average lag model were compared. As the study area, we selected the city of Guangzhou, China, where an excellent dataset was available.
Materials and methods
Data collection
Guangzhou, located in Southern China, is a metropolis with a high population density and high O3 concentration. It belongs to the typical subtropical humid monsoon climate, and its annual average temperature is 22°C and relative humidity is 68%. Due to the rapid economic development and increased energy consumption in the past few decades, Guangzhou has suffered from severe air pollution. Moreover, Guangzhou is a typical Chinese megacity, representing a city with urgent public health problems caused by air pollution. Therefore, Guangzhou is a unique city to evaluate the health effects of O3 concentration. We collected data on daily respiratory hospitalization from 1 January 2014 to 31 August 2018 from the First Affiliated Hospital of Sun Yat-sen University, which is located in Yuexiu District, central district of Guangzhou (Figure 1). This hospital is surrounded by universities, a large community of residents. Therefore, the daily respiratory hospitalization in this hospital can reflect the changes in the respiratory health status of residents in Guangzhou. The information on respiratory data contains the date of hospitalization, gender, age, diagnosis from the 10th International Classification of Diseases (ICD-10), and residential address. In this study, respiratory diseases (ICD-10: J00-J99) include upper and lower respiratory tract diseases. Since 89.4% of people in this dataset were above the age of 15, therefore, in this study, hospitalization visits were divided into two groups: 15–60 years (15 ≤ age < 60 years old) and 60+ years (≥60 years old). The screening was performed according to the patient's residential address, and the inpatients in this study were all local residents of Guangzhou.
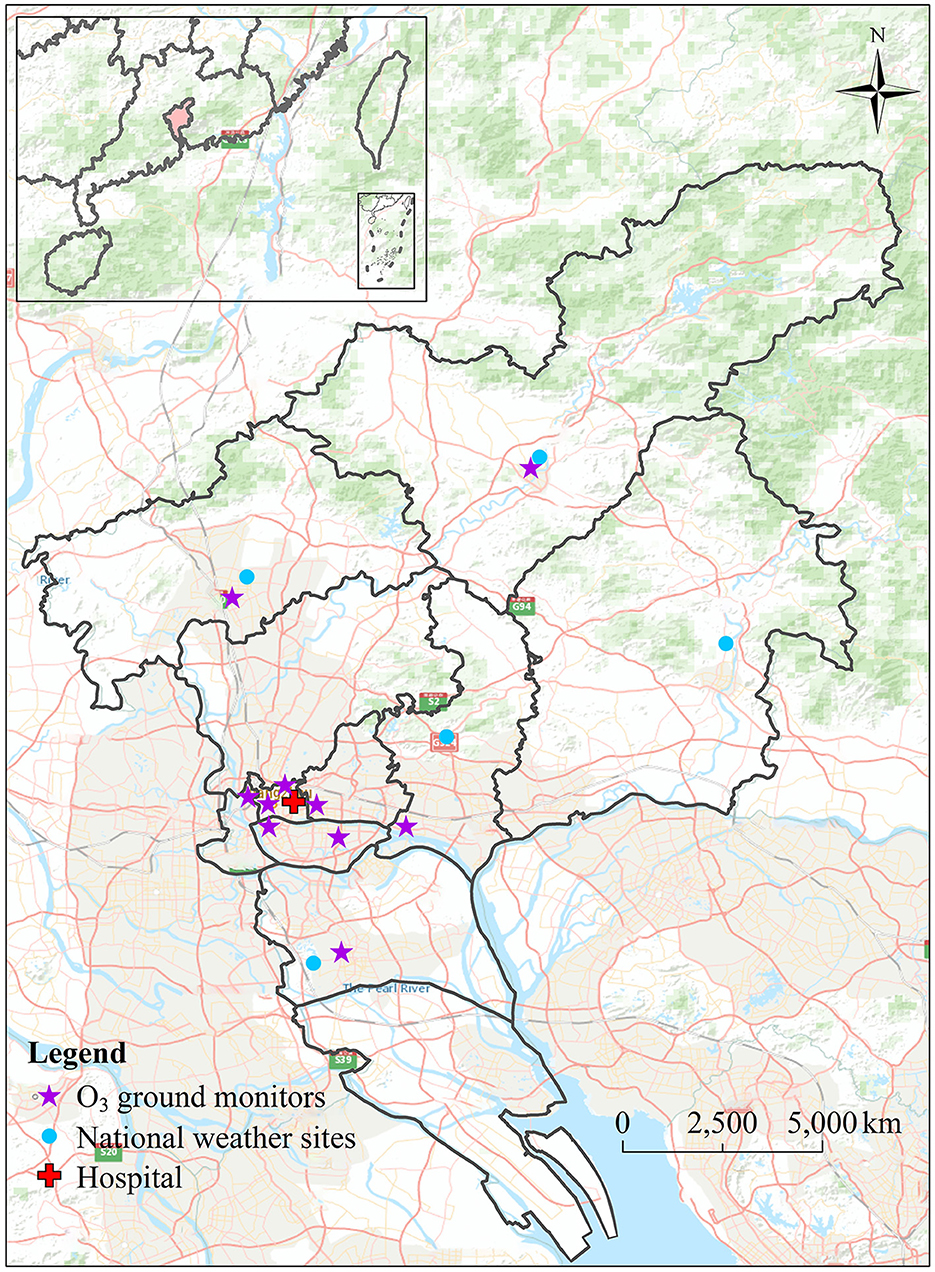
Figure 1. The spatial distribution of air quality monitoring stations, weather sites, and targeted hospital in Guangzhou.
Data on air pollutants include the two O3 indicators (MDA8 O3 and MDA1 O3), NO2, and PM2.5 concentration in Guangzhou from 1 January 2014 to 31 August 2018, which were collected from the air quality monitoring sites in Guangzhou. The data are published by the China National Environmental Monitoring Centre (http://quotsoft.net/air/). Because the hospitalized patients come from different districts of Guangzhou, the average value of 10 pollutant monitor sites was used. Data on PM2.5 and NO2 were used to test the sensitivity of the relationship between different O3 indicators and respiratory hospitalization in the multi-pollutant model.
Meteorological factors were obtained from the China Meteorological Data Sharing Service System (http://data.cma.gov.cn/). Daily average temperature and relative humidity were included to adjust for meteorological effects on respiratory hospitalization. All data are divided into two periods: warm and cold periods, based on the average temperature of each month, that is, months with an average temperature above 20°C are classified as warm period (May to October) and those below 20°C as cold period (November to April).
Statistical analysis
We applied a time-stratified case–crossover (TSCC) design in this study. The case–crossover design combines the advantages of case–control and cross-sectional studies and can be seen as an extension of the traditional case–control design (22). It uses the case itself as the control to avoid the bias caused by the selection of the control group and some uncontrollable factors between cases (such as age, intelligence, and job). Compared with traditional case–control studies, case–crossover designs have advantages in controlling for time-invariant confounders of individual characteristics, since each individual is under his or her own control (23). The TSCC design has been widely used to examine the impact of air pollution or extreme weather conditions on health outcomes such as morbidity and mortality (24–26).
We used a TSCC design to examine the relationship between two different O3 indicators and daily respiratory hospitalization. Of these, all cases served as their own controls. Odds ratios (ORs) and their 95% confidence intervals (CIs) between short-term O3 exposure and daily respiratory hospitalization were calculated using a conditional logistic regression model that was conducted with a Cox proportional hazards regression model. If the p-values were < 0.05, the results were considered statistically significant. The formula was as follows:
where log (h (t, X)) is the risk function of exposure to the independent variable X (X includes pollutants and meteorological factors) on day t (t is the date of hospital admission), log(h0(t)) is the baseline risk function, Ct is the daily O3 concentrations, AT is the average temperature, and RH is the relative humidity, with coefficients β1, β2, and β3. The results are presented as changes in percentage and their 95% CI of hospital admissions by a rise of per interquartile range (IQR) in O3 concentration. We choose the same day of the week 1 month before the patient's admission as a control. For instance, if patient visits on a Tuesday in June 2016, all Tuesdays a month ago are control days. According to this design, each case has 3–4 control days (27).
To capture the delayed (or “lag”) effects of O3 on respiratory hospitalization, we investigated the delayed associations of O3 exposure on hospitalization visits in Guangzhou. We both used a single-day lag model (lag0 to lag5), moving the average lag model to explore their cumulative effects (lag01 to lag05). Notably, the O3 concentration of lag0 refers to the concentration of O3 on the current day, and lag1 refers to the O3 concentration of the previous day. The O3 concentration of lag01 was calculated by the 2-day (the current day and the previous day) average, and similarly, the O3 concentration of lag05 was calculated by the average O3 concentration on the current day and the 5 days ago.
We performed a series of subgroup analyses stratified by age (15–60 years old and 60+ years old) and gender (male and female) to identify potentially susceptible subgroups. These age stratifications refer to the division from the previously published studies (4, 28). Moreover, a seasonal analysis of O3-related effects was also performed by dividing the entire study period into warm (May–October) and cold (November–April) periods. In these subgroup analyses, the effects of different O3 indicators on daily respiratory hospitalization in Guangzhou were examined separately.
Sensitivity analysis
To test the stability of these results, we performed the following sensitivity analyses: (1) Multi-pollutants analyses were performed by including the other two serious pollutants, NO2 and PM2.5; (2) change the lag days of meteorological factors (temperature and relative humidity) from 0 to 3, which were used to check whether the results are sensitive to changes in meteorological factors. All the aforementioned analyses were performed using the “survival” package in R software (version 3.6.3).
Results
Descriptive analysis
Table 1 describes the basic characteristics of daily respiratory hospitalization data, two different O3 indicators, two other pollutants (PM2.5 and NO2), and meteorological factors (average temperature and relative humidity). The cumulative number of respiratory hospitalization visits was equal to 5,229 cases from 1 January 2014 to 31 August 2018, and the average number of hospitalization visits per day was 6. Approximately 62.6% of patients were male and 37.4% were female. The number of hospitalization visits for respiratory diseases in the age group of 15–60 (44.1%) was slightly lower than that in the 60+ age group (45.2%) (Table 1).
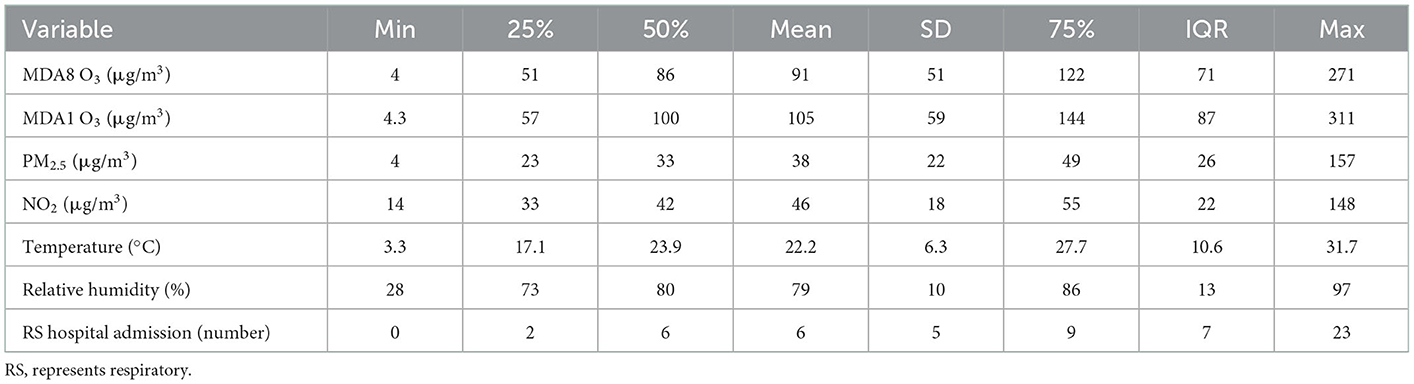
Table 1. Descriptive statistics of different O3 indicators, meteorological factors and hospitalization for respiratory diseases in Guangzhou.
Detailed information on air pollutants and meteorological factors from 2014 to 2018 in Guangzhou is listed in Table 1. From 2014 to 2018, the daily concentration of MDA8 O3 and MDA1 O3 ranged from 4.0 to 271.0 μg m−3 and 4.3 to 311.1 μg m−3, respectively. The annual average value of PM2.5 was 38.2 μg m−3, which was 9.14% higher than the Grade II Annual Standard (35 μg m−3) of the Chinese Ambient Air Quality Standards (CAAQS) and 2.8 times higher than the annual average value (10 μg m−3) reported by the World Health Organization. The daily concentration of NO2 ranges from 14 to 148 μg m−3, with an annual average of 46.1 μg m−3. From 2014 to 2018, there are 907 days that NO2 exceeded the Grade II Annual Standard CAAQS (40 μg m−3). Notably, PM2.5 and NO2 concentrations were higher in the cold season than that in the warm season, but O3, on the contrary, was higher in the warm season than that in the cold season. In addition, the average daily temperature was included as a confounder in the model, ranging from 3.3 to 31.7°C (the annual average is 22.2°C). Daily relative humidity ranges from 28 to 97% (the annual average is 78.7%). Specifically, the statistical characteristics of four pollutants, MDA8 O3, MDA1 O3, PM2.5, and NO2, and two meteorological factors, average temperature and relative humidity, are shown in Table 1.
Comparison of all different age and gender groups
Figure 2 shows the relationships between two O3 indicators and respiratory hospitalization visits in a single-pollutant model. At lag 5 day, MDA8 O3 has a significant and negative impact on the risk of respiratory hospitalization visits, with the OR value of 0.9945 (95% CI: 0.9897, 0.9993). As for MDA1 O3, there is no significant impact for all people.
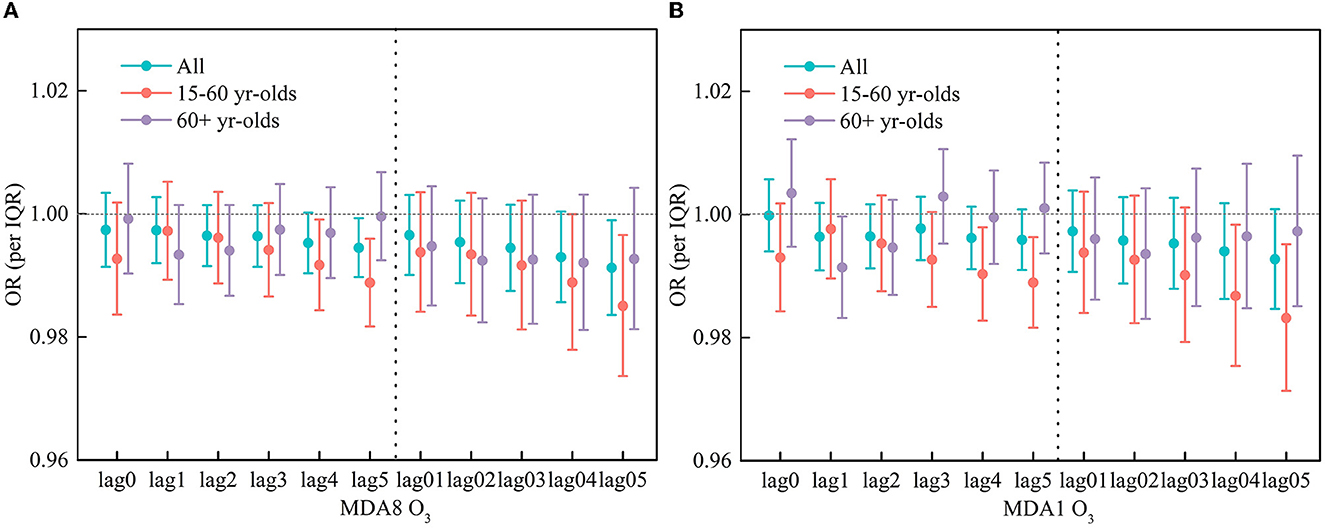
Figure 2. OR of respiratory hospitalization visits in different age group for per IQR increase in MDA8 O3 (A) and MDA1 O3 (B).
The respiratory risk in different age groups was further examined by applying the same model to identify whether there are differences in different stratifications. As shown in Figure 2A, MDA8 O3 was inversely associated with respiratory hospitalization visits in Guangzhou. At the lag of 4 and 5 days, MDA8 O3 had a significant impact on respiratory hospitalization in the 15–60 age group, and the OR value was 0.9917 (95% CI: 0.9843, 0.9991) and 0.9888 (95% CI: 0.9817, 0.9960), respectively. As for lag 04 and lag 05, there also have significant OR values, and they are 0.9889 (95% CI: 0.9779, 0.9999) and 0.9850 (95% CI: 0.9736, 0.9966), respectively. Meanwhile, no significant correlation was observed for the effect of MDA8 O3 on the 60+ age group.
Figure 2B shows the association between MDA1 O3 and respiratory hospitalization visits in different age groups in a single-pollutant model. At the lag4 and lag5 days, there was a significant effect of MDA1 O3 on the 15–60 years old, with values of 0.9903 (95% CI: 0.9828, 0.9979) and 0.9889 (95% CI: 0.9816, 0.9963). As for the 60+ age group, at the lag 1 day, the OR value is 0.9914 (95% CI: 0.9832, 0.9997).
Figure 3 shows OR estimates for subgroups stratified by gender. No obvious differences were identified. Specifically, in almost all gender subgroups, there was no significant association between MDA8O3/MDA1O3 and respiratory hospitalization visits except for MDA8 O3 in the OR at the lag4 day for the female group, and its significant OR value is 0.9905 (95% CI: 0.9826, 0.9986).
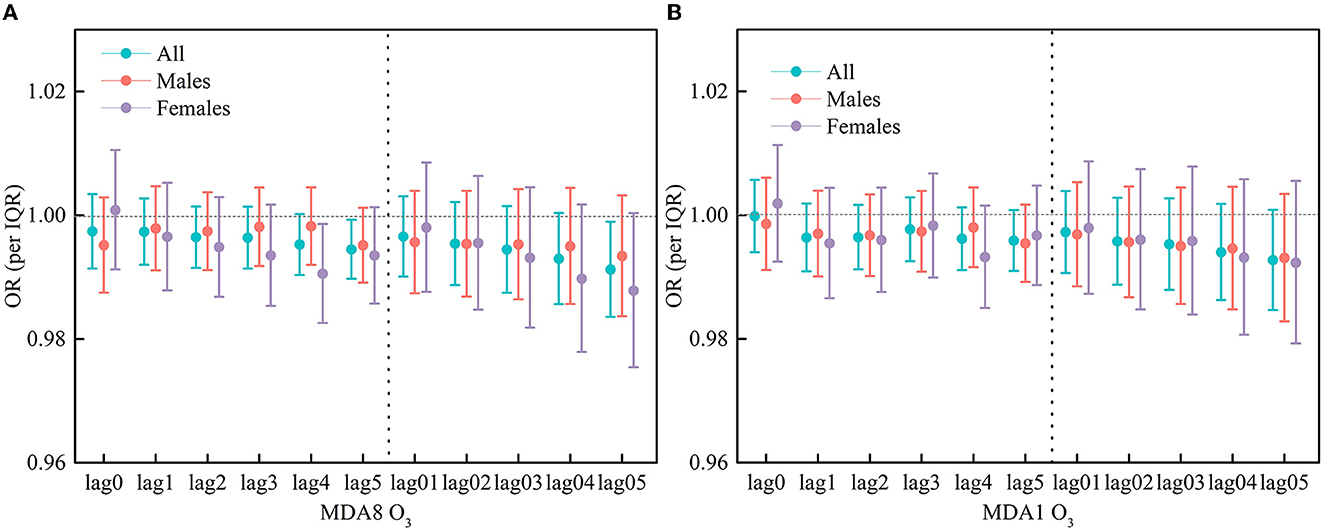
Figure 3. OR of respiratory hospitalization visits in different gender group for per IQR increase in MDA8 O3 (A) and MDA1 O3 (B).
In our analysis, the effect of O3 on respiratory hospitalization was statistically significant for some lag days. It can be seen that there is a different lagged effect of O3 on daily respiratory hospitalization in Guangzhou. In the different lag days, the effect of O3 on the respiratory hospitalization visits was different. Taking MDA8 O3 as an example for the 15–60 age group, the single-day lag effect at the lag4 has the greatest impact, that is, when the O3 concentration delay at 4 days, it has the greatest impact on the number of respiratory hospitalization visits. No significant effect, however, was observed in the 60+ age group. For women, the single-day lag effect was greatest at lag4; for men, no significant effect was observed. Moreover, compared with the single-day lag effect, the OR value of the moving average lag model is not significant, which indicates that for the whole year, the cumulative effect of O3 on respiratory hospitalization visits is also not significant.
The influence of seasonal effects
O3 concentration has obvious seasonal variation. Due to the strong sunlight in summer and the strong photochemical reaction at high temperature, the phenomenon of high O3 concentration in summer and low O3 concentration in winter is formed. Therefore, we analyzed the effect of O3 during the warm period (May–October) and the cold period (November–April). Notable differences were identified during different periods. Specifically, in the warm period, there are positive and significant associations between MDA8 O3/MDA1 O3 and respiratory hospitalization visits; conversely, there is a negative association in the cold period.
In the warm season, as for MDA8 O3 and all people, at the lag of 3–5 days, there have significant and positive associations, with the OR values being 1.0094 (95% CI: 1.0029, 1.0160), 1.0096 (95% CI: 1.0032, 1.0161), and 1.0084 (95% CI: 1.0021, 1.0146), respectively. As for the moving average lag model, there has a greater association at the lag 04 and lag05 days, and their OR values were 1.0130 (95% CI: 1.0030, 1.0231) and 1.01573 (95% CI: 1.0052, 1.0263), respectively (Figure 4). As for MDA1 O3 and all people, at the lag of 3–5 days, there is also a positive association but not statistically significant (Figure 5).
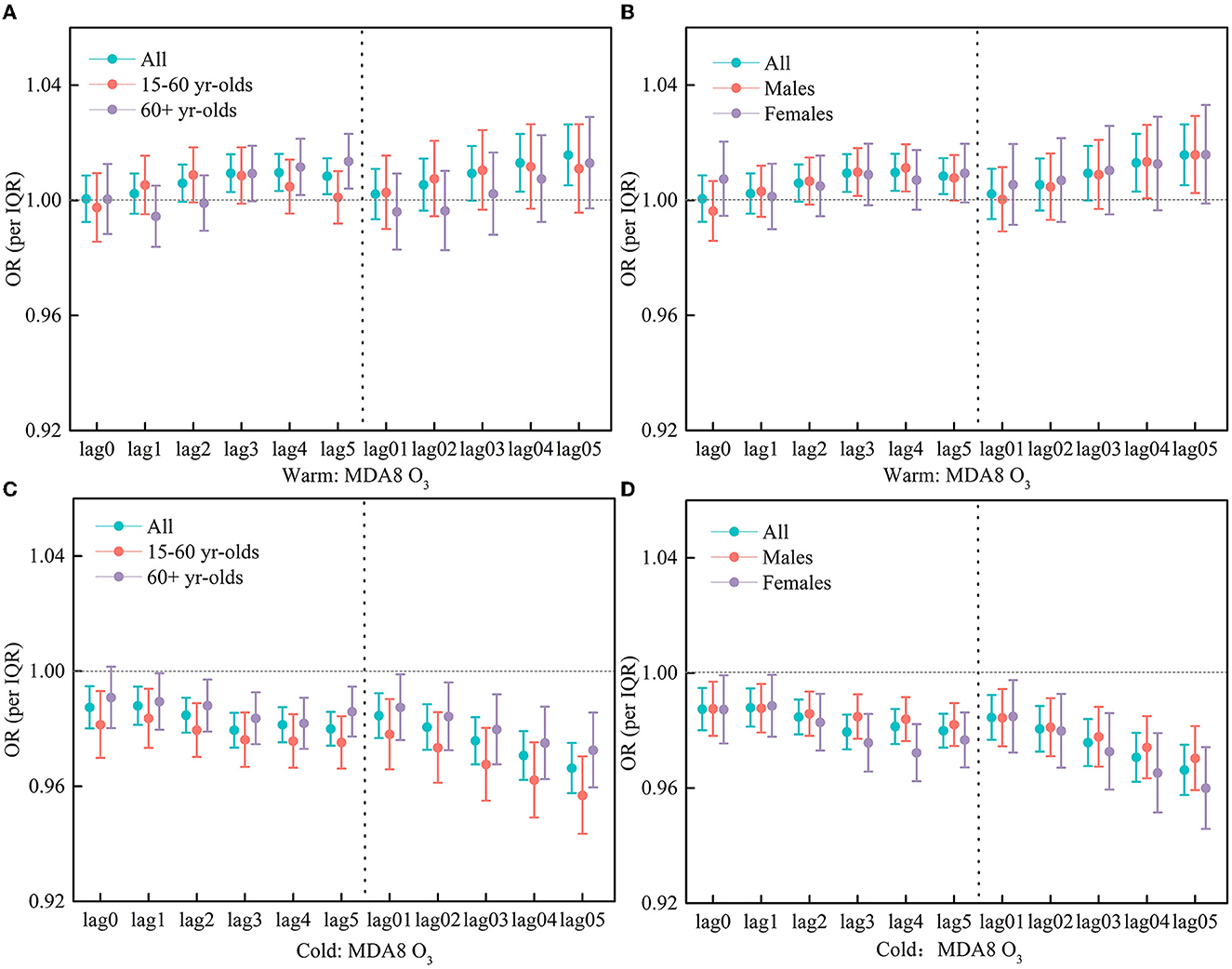
Figure 4. (A–D) OR of respiratory hospitalization visits for an increase of per IQR in MDA8 O3 during the warm periods (May to October) and the cold periods (November to April).
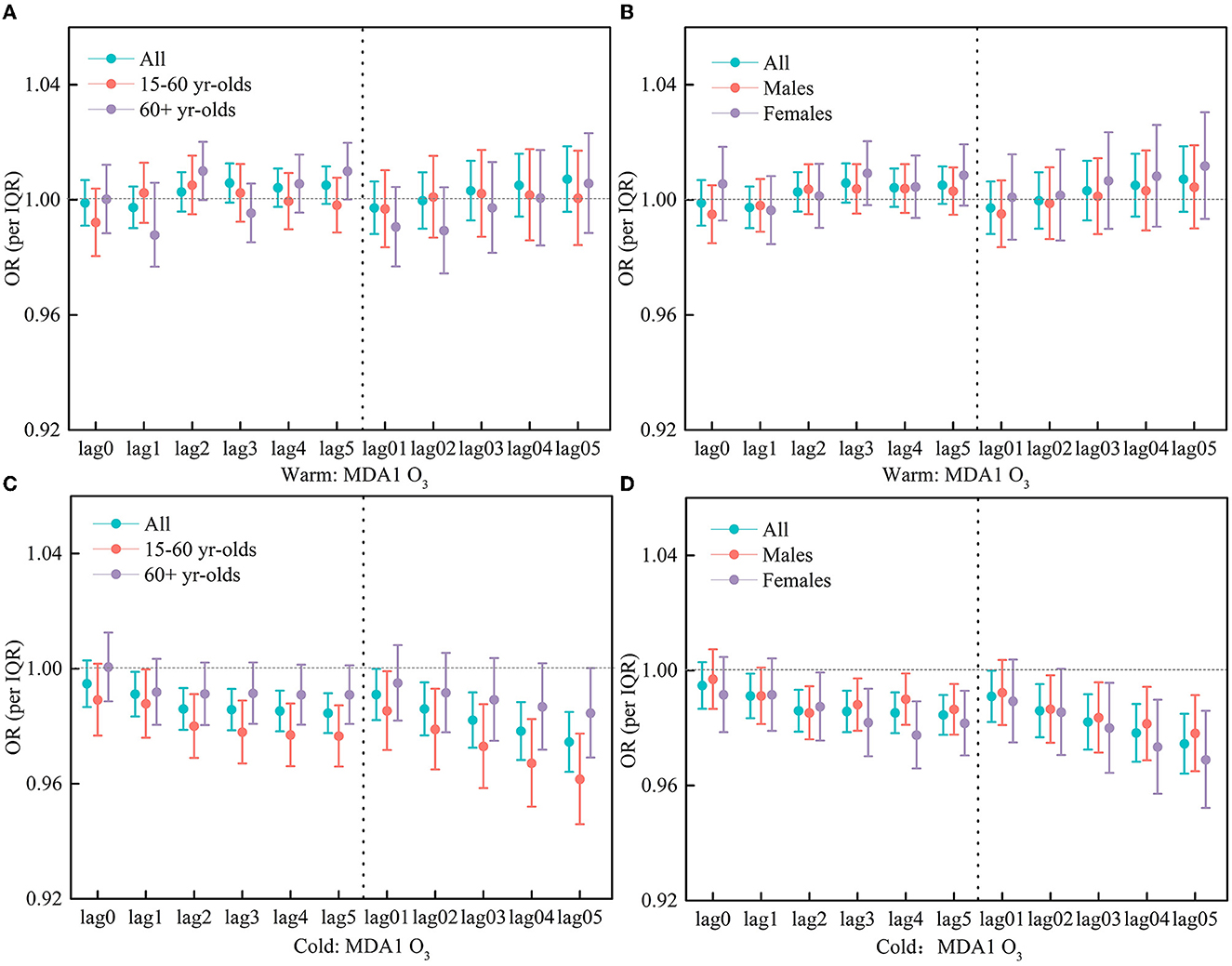
Figure 5. (A–D) OR of respiratory hospitalization visits for an increase of per IQR in MDA1 O3 during the warm periods (May to October) and the cold periods (November to April).
While in the cold season, MDA8 O3/MDA1 O3 was significantly and negatively correlated with respiratory hospitalization visits, both peaked at the lag05 day, with the OR being 0.9663 (95% CI: 0.9576, 0.9751) and 0.9745 (95% CI: 0.9641, 0.9849), respectively (Figures 4, 5).
The associations between short-term O3 exposure and respiratory hospitalization in different age groups were further examined in the warm period. At the lag 4 and 5 days, MDA8 O3 had a significant and positive impact on the respiratory hospitalization for the 60+ age group, with the OR being 1.0116 (95% CI: 1.0018, 1.0214) and 1.0135 (95% CI: 1.0041, 1.0231), respectively. For MDA1 O3, at the lag5 day, there is also a significant association, with the OR being 1.0099 (95% CI: 1.0001, 1.0198). For MDA8 O3/MDA1 O3, there is no significant association for the 15–60 age group of people (Figures 4, 5).
In different gender groups, the associations between O3 exposure and respiratory hospitalization were also examined. At the lag3, lag4, lag04, and lag05 days, MDA8 O3 had a significant and positive impact on respiratory hospitalization for the male group, with the OR values being 1.0097 (95%CI: 1.0015, 1.0181), 1.0112 (95%CI: 1.0030, 1.0194), 1.0133 (95%CI: 1.0006, 1.0262), and 1.0158 (95% CI: 1.0024, 1.0293), respectively. There is also a positive association for the female group but not statistically significant. Meanwhile, no significant association was observed for the effect of MDA1 O3 on different gender groups (Figures 4, 5).
Sensitivity analysis
Sensitivity analyses demonstrated the robustness of our main findings. In addition to the single-pollutant model for O3, we also tested a multi-pollutant model, including PM2.5 and NO2. After adding PM2.5 and NO2 as risk factors, the OR value of the single-pollutant model for O3 did not change much. The OR values changed to −0.26, 0.20, −0.26, and 0.18%, respectively. The OR values of MDA1O3 changed to −0.01, 0.32, 0.01, and 0.26%, respectively.
In addition, after adjusting for different lagged days of meteorological factors, the results of the single-pollutant model for MDA8 O3/MDA1 O3 did not change much, and their values for MDA8 O3 were −0.319, −0.065, 0.045, and 0.147%, respectively. The OR value of MDA1O3 changed values were −0.016, 0.147, 0.223, and 0.308%, respectively. These results were not materially affected, suggesting that these results of this study are relatively stable.
Discussion
Ozone had a negative impact on human health (29). To our knowledge, this is one of the few studies in China reporting the effects of two different O3 indicators on daily respiratory hospitalization visits. In this study, a time-stratified case–crossover design was used to evaluate the short-term effects of MDA8 O3/MDA1 O3 on daily respiratory hospitalization. The results showed that in the warm period, MDA8 O3 indicator appeared to be strongly associated with respiratory hospitalization visits risk than the MDA1 O3 indicator. The health effects of O3 on respiratory hospitalization visits are stronger in the 60+ age group than that in the 15–60 age group, and the female group is slightly more sensitive than the male group. Moreover, the association of both MDA8 O3 and MDA1 O3 and daily respiratory hospitalization visits was significant and positive in the warm period and negative in the cold period. Our findings help to understand the short-term health impact associated with different O3 indicators in Guangzhou, China, and present differences in the effects of O3 on different age and gender groups.
A comparative analysis of the two O3 indicators provided a comprehensive perspective to explore the relationships between O3 and respiratory health. From the year 2000 onward, various studies on respiratory mortality as measured globally by different indicators are reviewed in Table 2. These provide a comparative analysis, and we note large differences in the results. In the time-series analysis presented in this study, we observed different estimates for the MDA8 O3 and MDA1 O3 indicators. These were significant and negative and were associated with daily respiratory hospitalization visits at the lag 5 day, while MDA8 O3 had stronger associations than MDA1 O3. These results were similar to those obtained in previous studies. For instance, using a Poisson generalized linear model, Darrow et al. examined the association between daily respiratory emergency department visits and various O3 indicators (7). Their study showed that MDA8 O3 and MDA1 O3 were positively related to daily respiratory emergency department visits and that MDA8 O3 had a stronger association than MDA1 O3. Meanwhile, their OR values for MDA8 O3 and MDA1 O3 were greater than that in this study (Table 2). Moreover, Sun et al. conducted an epidemiological study on 34 counties in China exploring the associations between short-term exposure to different O3 indicators and respiratory mortality using three types of O3 indicators (MDA8 O3, MDA1 O3, and daily average) from 2013 to 2015 (32). Their results showed that the association between MDA8 O3 and respiratory mortality was stronger than that between MDA1 O3 and respiratory mortality, which is consistent with the results of this study. Notably, their OR value for MDA8 O3 was similar to that in our study, while the OR value was larger than that in our study. The potential reason for differences among the OR values of other studies and our study is that each city/region has different O3 levels and characteristics, as well as different population exposure patterns. Therefore, short-term exposure to different levels of O3 would have different health effects. Meanwhile, a possible explanation for MDA8 O3 had a greater impact is that the MDA8 O3 may be the most relevant indicator for individual exposure levels, as many people pour into the city during the day and move out at night. Moreover, high O3 exposure in the city during the daytime and 8-h maximum period may have higher health impacts than commute time and nighttime (16). Therefore, it has been suggested that health effects are related to short-term exposure to slightly higher O3 concentrations, such as MDA8 O3, rather than peak concentrations, such as MDA1 O3 (16).
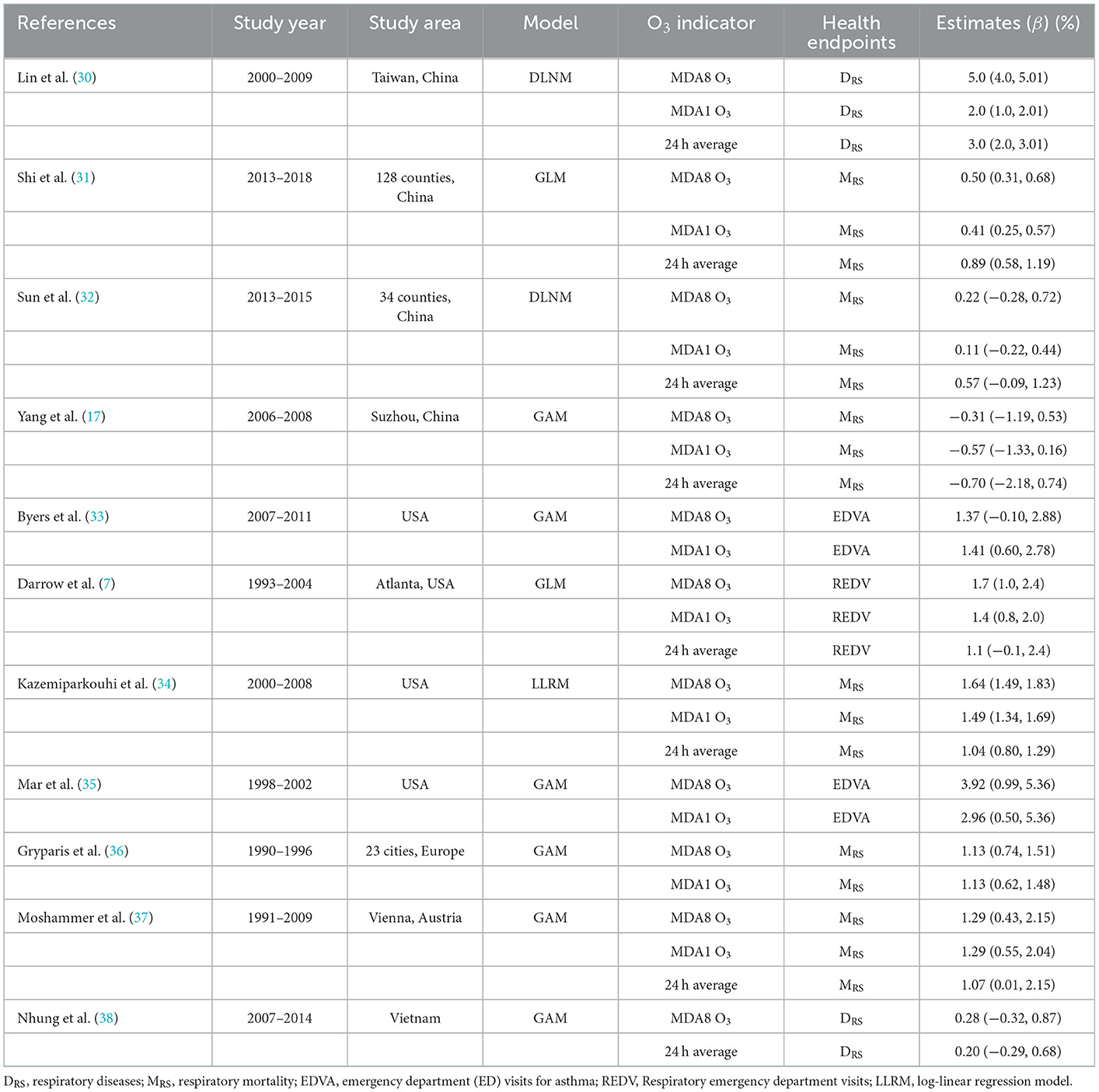
Table 2. Various studies on respiratory mortality attributed to different O3 indicators after the year 2000 worldwide.
Strong sunlight in summer and high photochemical production at high temperature result in high O3 concentration in summer and low O3 concentration in winter (13, 39). Therefore, we analyzed the effect of the two O3 indicators on the daily respiratory hospitalization visits in Guangzhou, China, during the warm period (May–October) and the cold period (November–April). The positive associations in the warm period and the negative ones in the cold period were identified, which were consistent with previous studies. In both periods, the sensitivity to O3 concentration was also studied in men and women aged 15–60 and older than 60 years, respectively. We found that during the warm period, MDA8 O3 was significantly positively correlated with the daily respiratory hospitalization visits at lag3–lag5, with a higher risk occurring in the 60+ age group. The relationship between MDA1 O3 and daily respiratory hospitalization visits was almost insignificant. During the cold period, the two O3 indicators were significantly and negatively associated with respiratory hospitalization visits, with a higher risk in the 60+ age group than that in the 15–60 age group. These results are consistent with studies from other time-series studies (40).
For example, Wang et al. showed that O3 was positively related to respiratory outpatient visits in the warm period but negatively associated with that in the cold period (4), while Malig et al. showed that O3 exposure was significantly and positively related to respiratory emergency department visits, and there has a slightly larger association in the warm period (41). The possible reason is that people tend to go outdoors/open windows in the warm period, so people are easily exposed to such a high O3 environment at that case, which will have larger effects on human health. Li et al. showed that the association between O3 and daily mortality in Guangzhou seems to be more prominent in the cold period than in the warm period (16), while Yang et al. also demonstrated that O3 had a significant effect on human health as in the cold period, and the relationship between O3 and daily mortality seemed to be more evident than that in the warm period (17). Other studies showed that there is no significant relationship between O3 and human health during the warm period (42, 43). This difference with the results in this study is possibly caused by different interactions of O3 exposure and season at different locations. Other factors such as exposure patterns and levels of local residents, air conditioners usage (44), and ventilation rates between indoor and outdoor may influence the season to modify the relationship between daily O3 concentration and respiratory hospitalization visits.
Lag effects of O3 on the daily respiratory hospitalization visits may exist in Guangzhou, China. Compared with the single-day lagged model, O3 showed similar impacts in the results of the moving average lag model for different age and gender subgroups. Especially in the warm period, the OR of the single-day lag model is slightly lower than that of the moving average lag model, indicating that O3 also had a cumulative effect on respiratory hospitalization visits, which were similar to the previous studies (4, 45). The reason for the lag effects may be that O3 produces an acute inflammatory response in the lungs. Studies have demonstrated that this inflammatory response is caused by repeated exposure over several days (46, 47). Notably, inflammation may play a key role in the increased O3-related mortality and morbidity (48).
There are some limitations to this study. First, data on the ambient O3 were averaged using fixed monitoring sites rather than individual measurements, which can result in underestimating the health effects of O3. Second, our data on respiratory hospitalization visits came from a large comprehensive and famous hospital, rather than from all hospitals in Guangzhou, which may lead to the underestimation of O3 effects on respiratory diseases, reflected in relatively small OR values. Therefore, caution should be paid when generalizing the results to other regions. Third, although we adjusted the confounders such as meteorological conditions (average temperature and relative humidity), there is still the possibility that some unmeasured confounders could have influenced the results. Finally, we do not have any information about patients' smoking behavior, which is also responsible for respiratory problems.
Conclusion
This study analyzed the relationship between daily respiratory hospitalization visits and two common O3 indicators in Guangzhou, China. It showed that the two O3 indicators were significantly and positively related to respiratory hospitalization visits in the warm period, and negatively in the cold period. In the warm period, women were more sensitive to O3 than men, and the 60+ age group was more sensitive than the 15–60 age group. Both the single-day lag model and the moving average lag model showed a significant effect on respiratory hospitalization visits. In the multi-pollutant model, adding one or all pollutants as a risk factor, the results for two different indicators are similar, indicating that the results are stable. These findings provide a comprehensive insight into the impact of different O3 indicators on human health in densely populated cities. They may serve as well as a reference for local governments to formulate air pollution measures to optimize emergency medical resources.
Data availability statement
The original contributions presented in the study are included in the article/supplementary material, further inquiries can be directed to the corresponding authors.
Author contributions
XZ and KM: conceptualization and methodology. GL, ZW, FY, and XZ: data curation, resources, and formal analysis. XZ: writing the original draft preparation. AS, CC, KM, and FO: supervision and writing, reviewing, and revising the manuscript. All authors contributed to the article and approved the submitted version.
Funding
This study was supported by the National Natural Science Foundation of China (42071178 and 41671139); the National Key Research and Development Plan of China (2019YFA0606901); and the Visiting Fellowship from China Scholarship Council (No. 202106040072).
Acknowledgments
The authors gratefully acknowledge the Faculty of Geo-Information Science and Earth Observation (ITC), the University of Twente for hosting XZ to carry out this study.
Conflict of interest
The authors declare that the research was conducted in the absence of any commercial or financial relationships that could be construed as a potential conflict of interest.
Publisher's note
All claims expressed in this article are solely those of the authors and do not necessarily represent those of their affiliated organizations, or those of the publisher, the editors and the reviewers. Any product that may be evaluated in this article, or claim that may be made by its manufacturer, is not guaranteed or endorsed by the publisher.
References
1. Huang J, Pan XC, Guo XB, Li GX. Health impact of China's Air Pollution Prevention and Control Action Plan: an analysis of national air quality monitoring and mortality data. Lancet Planet Health. (2018) 2:E313–23. doi: 10.1016/S2542-5196(18)30141-4
2. Maji KJ, Namdeo A. Continuous increases of surface ozone and associated premature mortality growth in China during 2015-2019. Environ Pollut. (2021) 269:116183. doi: 10.1016/j.envpol.2020.116183
3. Lim SS, Vos T, Flaxman AD, Danaei G, Shibuya K, Adair-Rohani H, et al. A comparative risk assessment of burden of disease and injury attributable to 67 risk factors and risk factor clusters in 21 regions, 1990-2010: a systematic analysis for the Global Burden of Disease Study 2010. Lancet. (2012) 380:2224–60. doi: 10.1016/S0140-6736(12)61766-8
4. Wang YY, Zu YQ, Huang L, Zhang HL, Wang CH, Hu JL. Associations between daily outpatient visits for respiratory diseases and ambient fine particulate matter and ozone levels in Shanghai, China. Environ Pollut. (2018) 240:754–63. doi: 10.1016/j.envpol.2018.05.029
5. Long L, Zhu LT, Huang Q. Correlation between lung cancer markers and air pollutants in western China population. Environ Sci Pollut Res. (2022) 29:1–9. doi: 10.1007/s11356-022-20354-3
6. You Y, Wang D, Liu J, Chen Y, Ma X, Li W. Physical exercise in the context of air pollution: An emerging research topic. Front Physiol. (2022) 13:784705. doi: 10.3389/fphys.2022.784705
7. Darrow LA, Klein M, Sarnat JA, Mulholland JA, Strickland MJ, Sarnat SE, et al. The use of alternative pollutant metrics in time-series studies of ambient air pollution and respiratory emergency department visits. J Expo Sci Environ Epidemiol. (2011) 21:10–9. doi: 10.1038/jes.2009.49
8. Wise J. Better air quality reduces respiratory symptoms among children in southern California. Br Med J. (2016) 353:i2083. doi: 10.1136/bmj.i2083
9. Guo H, Kota SH, Sahu SK, Hu JL, Ying Q, Gao AF, et al. Source apportionment of PM25 in North India using source-oriented air quality models. Environ Pollut. (2017) 231:426–36. doi: 10.1016/j.envpol.2017.08.016
10. Zhang XX, Cheng CX, Zhao H. A health impact and economic loss assessment of O3 and PM2.5 exposure in China from 2015 to 2020. GeoHealth. (2022) 6:e2021GH000531 doi: 10.1029/2021GH000531
11. Maji KJ, Ye WF, Arora M, Nagendra SMS. Ozone pollution in Chinese cities: assessment of seasonal variation, health effects and economic burden. Environ Pollut. (2019) 247:792–801. doi: 10.1016/j.envpol.2019.01.049
12. Shang Y, Sun ZW, Cao JJ, Wang XM, Zhong LJ, Bi XH, et al. Systematic review of Chinese studies of short-term exposure to air pollution and daily mortality. Environ Int. (2013) 54:100–11. doi: 10.1016/j.envint.2013.01.010
13. Zhang XX, Yan B, Du CJ, Cheng CX, Zhao H. Quantifying the interactive effects of meteorological, socioeconomic, and pollutant factors on summertime ozone pollution in China during the implementation of two important policies. Atmos Pollut Res. (2021) 12:101248. doi: 10.1016/j.apr.2021.101248
14. Liao YX, Sun J, Qian ZM, Mei SJ, Li Y, Lu Y, et al. Modification by seasonal influenza and season on the association between ambient air pollution and child respiratory diseases in Shenzhen, China. Atmos Environ. (2020) 234:117621. doi: 10.1016/j.atmosenv.2020.117621
15. Zhang JY, Chen Q, Wang QQ, Ding Z, Sun H, Xu Y. The acute health effects of ozone and PM25 on daily cardiovascular disease mortality: a multi-center time series study in China. Ecotox Environ Safe. (2019) 174:218–23. doi: 10.1016/j.ecoenv.2019.02.085
16. Li TT, Yan ML, Ma WJ, Ban J, Liu T, Lin HL, et al. Short-term effects of multiple ozone metrics on daily mortality in a megacity of China. Environ Sci Pollut Res. (2015) 22:8738–46. doi: 10.1007/s11356-014-4055-5
17. Yang CX, Yang HB, Guo S, Wang ZS, Xu XH, Duan XL, et al. Alternative ozone metrics and daily mortality in Suzhou: The China Air Pollution and Health Effects Study (CAPES). Sci Total Environ. (2012) 426:83–9. doi: 10.1016/j.scitotenv.2012.03.036
18. Abbey DE, Burchette RJ. Relative power of alternative ambient air pollution metrics for detecting chronic health effects in epidemiological studies. Environmetrics. (1996) 7:453–70. doi: 10.1002/(SICI)1099-095X(199609)7:5<453::AID-ENV220>3.0.CO;2-X
19. Chen L, Wang XJ, Qian ZM, Sun LW, Qin LJ, Wang CJ, et al. Ambient gaseous pollutants and emergency ambulance calls for all-cause and cause-specific diseases in China: a multicity time-series study. Environ Sci Pollut Res. (2022) 29:28527–37. doi: 10.1007/s11356-021-18337-x
20. Xu H, Wang XY, Tian YH, Tian J, Zeng YP, Guo YL, et al. Short-term exposure to gaseous air pollutants and daily hospitalizations for acute upper and lower respiratory infections among children from 25 cities in China. Environ Res. (2022) 212:113493. doi: 10.1016/j.envres.2022.113493
21. Yang HH, Yan CX, Li M, Zhao L, Long Z, Fan YL, et al. Short term effects of air pollutants on hospital admissions for respiratory diseases among children: a multi-city time-series study in China. Int J Hyg Environ Health. (2021) 231:113638. doi: 10.1016/j.ijheh.2020.113638
22. Maclure M. The case-crossover design - A method for studying transient effects on the risk of acute events. Am J Epidemiol. (1991) 133:144–53. doi: 10.1093/oxfordjournals.aje.a115853
23. Lumley T, Levy D. Bias in the case-crossover design: implications for studies of air pollution. Environmetrics. (2000) 11:689–704. doi: 10.1002/1099-095X(200011/12)11:6<689::AID-ENV439>3.0.CO;2-N
24. Bhaskaran K, Hajat S, Armstrong B, Haines A, Herrett E, Wilkinson P, et al. The effects of hourly differences in air pollution on the risk of myocardial infarction: case crossover analysis of the MINAP database. Br Med J. (2011) 343:d5531. doi: 10.1136/bmj.d5531
25. Liu LJ, Song FJ, Fang JY, Wei J, Ho HC, Song YM, et al. Intraday effects of ambient PM1 on emergency department visits in Guangzhou, China: a case-crossover study. Sci Total Environ. (2021) 750:142347. doi: 10.1016/j.scitotenv.2020.142347
26. Yorifuji T, Suzuki E, Kashima S. Cardiovascular emergency hospital visits and hourly changes in air pollution. Stroke. (2014) 45:1264–8. doi: 10.1161/STROKEAHA.114.005227
27. Ding L, Zhu D, Peng D, Zhao Y. Air pollution and asthma attacks in children: a case–crossover analysis in the city of Chongqing, China. Environ Pollut. (2017) 220:348–53. doi: 10.1016/j.envpol.2016.09.070
28. Jiao AQ, Xiang QQ, Ding Z, Cao JG, Ho HC, Chen DY, et al. Short-term impacts of ambient fine particulate matter on emergency department visits: comparative analysis of three exposure metrics. Chemosphere. (2020) 241:125012. doi: 10.1016/j.chemosphere.2019.125012
29. Hsu HT, Wu CD, Chung MC, Shen TC, Lai TJ, Chen CY, et al. The effects of traffic-related air pollutants on chronic obstructive pulmonary disease in the community-based general population. Respir Res. (2021) 22:1–12. doi: 10.1186/s12931-021-01812-x
30. Lin YK, Chang SC, Lin C, Chen YC, Wang YC. Comparing ozone metrics on associations with outpatient visits for respiratory diseases in Taipei Metropolitan area. Environ Pollut. (2013) 177:177–84. doi: 10.1016/j.envpol.2012.12.010
31. Shi W, Sun Q, Du P, Tang S, Chen C, Sun Z, et al. Modification effects of temperature on the ozone-mortality relationship: a nationwide multicounty study in China. Environ Sci Technol. (2020) 54:2859–68. doi: 10.1021/acs.est.9b05978
32. Sun Q, Wang W, Chen C, Ban J, Xu D, Zhu P, et al. Acute effect of multiple ozone metrics on mortality by season in 34 Chinese counties in 2013-2015. J Intern Med. (2018) 283:481–8. doi: 10.1111/joim.12724
33. Byers N, Ritchey M, Vaidyanathan A, Brandt AJ, Yip F. Short-term effects of ambient air pollutanta on asthma-related emergency department visits in Indianapolis, Indiana, 2007-2011. J Asthma. (2015) 53:245–52. doi: 10.3109/02770903.2015.1091006
34. Kazemiparkouhi F, Eum KD, Wang BY, Manjourides J, Suh HH. Long-term ozone exposures and cause-specific mortality in a US Medicare cohort. J Expo Sci Environ Epidemiol. (2020) 30:650–8. doi: 10.1038/s41370-019-0135-4
35. Mar TF, Koenig JQ. Relationship between visits to emergency departments for asthma and ozone exposure in greater Seattle, Washington. Ann Allergy Asthma Immunol. (2009) 103:474–9. doi: 10.1016/S1081-1206(10)60263-3
36. Gryparis A, Forsberg B, Katsouyanni K, Analitis A, Touloumi G, Schwartz J, et al. Acute effects of ozone on mortality from the “air pollution and health: a European approach” project. Am J Respir Crit Care Med. (2004) 170:1080–7. doi: 10.1164/rccm.200403-333OC
37. Moshammer H, Hutter HP, Kundi M. Which metric of ambient ozone to predict daily mortality? Atmos Environ. (2013) 65:171–6. doi: 10.1016/j.atmosenv.2012.10.032
38. Nhung NTT, Schindler C, Dien TM, Probst-Hensch N, Perez L, Kunzli N. Acute effects of ambient air pollution on lower respiratory infections in Hanoi children: An eight-year time series study. Environ Int. (2018) 110:139–48. doi: 10.1016/j.envint.2017.10.024
39. Zhao H, Wang L, Zhang Z, Qi Q, Zhang HJ. Quantifying ecological and health risks of ground-level O3 across China during the implementation of the “Three-year Action Plan for Cleaner Air”. Sci Total Environ. (2022) 817:153011. doi: 10.1016/j.scitotenv.2022.153011
40. Álvaro-Meca A, Sepúlveda-Crespo D, Resino R, Ryan P, Martínez I, Resino S. Neighborhood environmental factors linked to hospitalizations of older people for viral lower respiratory tract infections in Spain: a case-crossover study. Environ Health. (2022) 21:1–10. doi: 10.1186/s12940-022-00928-x
41. Malig BJ, Pearson DL, Chang YB, Broadwin R, Basu R, Green RS, et al. A time-stratified case-crossover study of ambient ozone exposure and emergency department visits for specific respiratory diagnoses in California (2005-2008). Environ Health Perspect. (2016) 124:745–53. doi: 10.1289/ehp.1409495
42. Chang CC, Tsai SS, Ho SC, Yang CY. Air pollution and hospital admissions for cardiovascular disease in Taipei, Taiwan. Environ Res. (2005) 98:114–9. doi: 10.1016/j.envres.2004.07.005
43. Phung D, Hien TT, Linh HN, Luong LMT, Morawska L, Chu C, et al. Air pollution and risk of respiratory and cardiovascular hospitalizations in the most populous city in Vietnam. Sci total Environ. (2016) 557:322–30. doi: 10.1016/j.scitotenv.2016.03.070
44. Bell ML, Dominici F. Effect modification by community characteristics on the short-term effects of ozone exposure and mortality in 98 US communities. Am J Epidemiol. (2008) 167:986–97. doi: 10.1093/aje/kwm396
45. Wong CM, Vichit-Vadakan N, Kan HD, Qian ZM, Teams PP. Public Health and Air Pollution in Asia (PAPA): A multicity study of short-term effects of air pollution on mortality. Environ Health Perspect. (2008) 116:1195–202. doi: 10.1289/ehp.11257
46. Folinsbee LJ, Horstman DH, Kehrl HR, Harder S, Abdulsalaam S, Ives PJ. Respiratory responses to repeated prolonged exposure to 012-ppm Ozone. Am J Respir Crit Care Med. (1994) 149:98–105. doi: 10.1164/ajrccm.149.1.8111607
47. Frank R, Liu MC, Spannhake EW, Mlynarek S, Macri K, Weinmann GG. Repetitive ozone exposure of young adults - Evidence of persistent small airway dysfunction. Am J Respir Crit Care Med. (2001) 164:1253–60. doi: 10.1164/ajrccm.164.7.2010043
48. Brook RD, Franklin B, Cascio W, Hong YL, Howard G, Lipsett M, et al. Air pollution and cardiovascular disease - A statement for healthcare professionals from the expert panel on population and prevention science of the American Heart Association. Circulation. (2004) 109:2655–71. doi: 10.1161/01.CIR.0000128587.30041.C8
Keywords: O3 indicators, respiratory health, hospitalization visits, time-stratified case, air pollution
Citation: Lin G, Wang Z, Zhang X, Stein A, Maji KJ, Cheng C, Osei F and Yang FF (2023) Comparison of the association between different ozone indicators and daily respiratory hospitalization in Guangzhou, China. Front. Public Health 11:1060714. doi: 10.3389/fpubh.2023.1060714
Received: 03 October 2022; Accepted: 03 January 2023;
Published: 30 January 2023.
Edited by:
Chao Song, Sichuan University, ChinaReviewed by:
Yuquan Chen, Chinese Academy of Medical Sciences and Peking Union Medical College, ChinaYuexia Sun, Tianjin University, China
Copyright © 2023 Lin, Wang, Zhang, Stein, Maji, Cheng, Osei and Yang. This is an open-access article distributed under the terms of the Creative Commons Attribution License (CC BY). The use, distribution or reproduction in other forums is permitted, provided the original author(s) and the copyright owner(s) are credited and that the original publication in this journal is cited, in accordance with accepted academic practice. No use, distribution or reproduction is permitted which does not comply with these terms.
*Correspondence: Zhuoqing Wang, d3podW9xQG1haWwuc3lzdS5lZHUuY24=; Xiangxue Zhang,
enh4QGxyZWlzLmFjLmNu
†These authors have contributed equally to this work