- Wadsworth Center, New York State Department of Health, Albany, NY, United States
Mycobacterium tuberculosis complex (MTBC) infections are treated with combinations of antibiotics; however, these regimens are not as efficacious against multidrug and extensively drug resistant MTBC. Phenotypic (growth-based) drug susceptibility testing on slow growing bacteria like MTBC requires many weeks to months to complete, whereas sequencing-based approaches can predict drug resistance (DR) with reduced turnaround time. We sought to develop a multiplexed, targeted next generation sequencing (tNGS) assay that can predict DR and can be performed directly on clinical respiratory specimens. A multiplex PCR was designed to amplify a group of thirteen full-length genes and promoter regions with mutations known to be involved in resistance to first- and second-line MTBC drugs. Long-read amplicon libraries were sequenced with Oxford Nanopore Technologies platforms and high-confidence resistance mutations were identified in real-time using an in-house developed bioinformatics pipeline. Sensitivity, specificity, reproducibility, and accuracy of the tNGS assay was assessed as part of a clinical validation study. In total, tNGS was performed on 72 primary specimens and 55 MTBC-positive cultures and results were compared to clinical whole genome sequencing (WGS) performed on paired patient cultures. Complete or partial susceptibility profiles were generated from 82% of smear positive primary specimens and the resistance mutations identified by tNGS were 100% concordant with WGS. In addition to performing tNGS on primary clinical samples, this assay can be used to sequence MTBC cultures mixed with other mycobacterial species that would not yield WGS results. The assay can be effectively implemented in a clinical/diagnostic laboratory with a two to three day turnaround time and, even if batched weekly, tNGS results are available on average 15 days earlier than culture-derived WGS results. This study demonstrates that tNGS can reliably predict MTBC drug resistance directly from clinical specimens or cultures and provide critical information in a timely manner for the appropriate treatment of patients with DR tuberculosis.
1. Introduction
Tuberculosis (TB) continues to be a major contributor to global infectious disease deaths, with an estimated 10.6 million cases and 1.6 million deaths worldwide in 2021 (1). TB patients are treated with combination drug regimens; however, the emergence of increasingly drug-resistant forms of Mycobacterium tuberculosis complex (MTBC) in recent decades necessitates the use of alternative therapies (2). Currently, strategies for therapy are still mostly decided based on culture-based phenotypic drug susceptibility testing (DST); however, MTBC DST requires weeks or months to complete due to the slow growth rate of this organism (3–7). During this time, patients can be prescribed ineffective drug regimens, leading to treatment failure or the promotion of drug resistance (DR) (8). The potential for these negative patient outcomes underscores the need for quicker methods to detect DR TB (DR-TB).
Molecular and sequencing-based assays offer a faster alternative for profiling DR in slow-growing organisms like M. tuberculosis. Commercially available molecular methods include Xpert MTB/RIF (Cepheid, Sunnyvale, CA) (9) and the GenoType MTBDRplus line probe assay (Hain Lifescience Nehren, Germany) (10), which detect mutations within specific “hot spot” regions to predict DR. These rapid diagnostics, endorsed by World Health Organization (WHO), have contributed to improved global detection of DR, particularly for the first-line drug rifampin (11–14). These assays, however, may miss mutations outside of the targeted “hot spot” regions and incur false negative results (15, 16), or, in rare circumstances, silent or neutral mutations may incur false positive results for DR (17, 18).
Sequencing-based methods provide greater resolution of these loci. Assays developed for the detection of DR include pyrosequencing (19–22) and Sanger sequencing (23) of individual targets; however, these methods are typically limited to single-plex reactions analyzing limited sections of DR determining loci. NGS assays offer more comprehensive DR profiles by identifying novel and high confidence DR-associated mutations throughout the genome (24, 25). Whole genome sequencing (WGS) assays, such as the one implemented by Wadsworth Center, identify high-confidence mutations that allow accurate prediction of phenotypic DR (26). These assays provide comprehensive DR profiling and the bioinformatic analysis can be routinely updated to include new loci and mutations in accordance with national and global WHO databases (27). WGS results can be generated before phenotypic DST is available (7, 26, 28, 29); however, most clinically validated WGS assays are performed on MTBC-positive cultures that can require several weeks of incubation (30).
Targeted NGS (tNGS) assays can further reduce the time required for comprehensive DR profiling by amplifying numerous loci directly from clinical specimens. Several tNGS assays for DR profiling have been described in the literature, including laboratory-developed assays (31–35) and the commercially available Genoscreen Deeplex (36, 37) and Ion AmpliSeq (38). These assays vary in a number of ways including the selection and size of targets, how multiplexed the PCR reactions are, and the sequencing platforms employed, which include Illumina (31, 35, 36), Ion Torrent (32, 34, 38), and Oxford Nanopore Technologies (33, 39, 40).
In this paper, we describe the design, validation, and implementation of a tNGS assay for direct DR profiling on MTBC-positive clinical specimens at the Wadsworth Center. This assay includes a simplified set up with two multiplexed PCR amplification reactions that target thirteen full-length loci implicated in DR to first- and second-line MTBC antimicrobials. The assay was optimized for sequencing on an Oxford Nanopore Technologies platform, enabling real-time analysis, a two-to-three-day turnaround time with typically <2 h of sequencing time, and a cost of less than $80 per sample. In addition to performing tNGS on primary specimens, this assay was found to be accurate and generated susceptibility profiles comparable to those currently obtained with our existing WGS assay, which can only be performed on cultured isolates. These results demonstrate that tNGS-based assays can provide a reliable and cost-effective tool for early detection of DR-TB and should be considered for implementation in public health and clinical laboratories with MTBC testing needs and resources.
2. Materials and methods
2.1. Sample preparation
Samples submitted for mycobacterial testing were handled in a BSL-3 laboratory. Sterile tissue specimens (e.g., lung tissue, lymph node tissue) were homogenized in sterile saline. Respiratory specimens (e.g., sputum, bronchial washes, and bronchoalveolar lavages) underwent digestion and decontamination to optimize mycobacteria recovery. This procedure uses a 3.5% sodium hydroxide solution to dissolve mucus, lyse organic material, and inactivate other bacteria. Following incubation, the solution was neutralized, bacteria were concentrated by centrifugation, and the pellet was resuspended in a buffer. Processed samples were used to inoculate liquid cultures (MGIT 960, BACTEC) and underwent differential staining and smear microscopy. Aliquots for molecular testing were heat inactivated (80°C for 1 h) before handling in a BSL-2 laboratory.
2.2. Direct smear microscopy for acid-fast bacilli
Processed primary specimens were stained using the Ziehl-Neelsen Carbol Fuchsin method according to manufacturer instructions (Remel Inc., San Diego, CA) and examined under a microscope for the presence of Acid-Fast Bacilli (AFB). Samples positive for AFB were further categorized based on the number of AFB observed, with numerous defined as >9 AFB per high power field (HPF-1000X) (++++), moderate as 1–9 AFB per HPF (+++), few as 1–9 AFB per 10 HPF (++), and rare as 1–9 AFB per 300 HPF (+). Smear negative samples are defined as those with no AFB observed.
2.3. Real-time PCR for MTBC detection
DNA was extracted via mechanical lysis with FastPrep24 (MP Biomedicals, Solon, Ohio) and tested for M. tuberculosis complex (MTBC) DNA using previously described real-time PCR assay (41). This multiplexed assay includes a single-copy (ext-RD9) and multi-copy (IS6110) target for MTBC detection and a target for Mycobacterium avium complex detection (ITS). All specimens included in this study were positive for MTBC DNA via a real-time PCR.
2.4. Whole genome sequencing
Samples included in this study were analyzed using a NYS-validated WGS assay as previously described (26). Briefly, a manual DNA extraction utilizing InstaGene reagent (Bio-Rad Laboratories, Hercules, CA), mechanical lysis, and centrifugation was performed on heat-killed isolates identified as MTBC-positive. Concentration of DNA was assessed using Qubit DNA fluorometry (Thermo Fisher Scientific, Waltham, MA) and samples were prepared for Illumina sequencing on a MiSeq or NextSeq instrument (Illumina, San Diego, CA). Results were analyzed using a clinically validated in-house developed bioinformatics pipeline that identifies high-confidence and unknown/novel mutations (26).
2.5. DNA extraction and controls for tNGS
For tNGS, an automated lysis and purification-based DNA extraction method (EZ1 Virus DSP Kit, Qiagen) was used to minimize DNA shearing. On this platform, 100 μL of specimen was extracted and eluted in 60 μL. Each run included a positive and negative control, consisting of 100 μL of Mycobacterium bovis BCG MGIT positive culture and 100 μL of sterile molecular grade water, respectively. Both extraction controls were processed in parallel with clinicals specimens and serve as reagent and sequencing controls for the entire tNGS assay.
2.6. Primer design and PCR
Thirteen primer sets were designed to amplify full-length genes (rpoB, katG, mabA, inhA, embB, gyrA, gyrB, ethA, rrs, rpsL, and pncA) and/or promoter regions (oxyR-ahpC, mabA-inhA, embC-A, pncA, and eis) implicated in DR to first- and second-line MTBC antimicrobials, including rifampin, isoniazid, ethambutol, pyrazinamide, fluoroquinolones, ethionamide, streptomycin, and kanamycin/amikacin (Supplementary Table S1). Possible primer pairs were generated using Primer3 (42) and checked for in silico interactions with ThermoFisher’s Multiple Primer Analyzer Tool (ThermoFisher Scientific, Waltham, MA) (43). Primer sets were multiplexed into two PCR reactions referred to as “Pool A” and “Pool B” and primer concentrations were optimized to obtain balanced amplification of each target (Supplementary Table S2). Each 40 μL PCR reaction contained Long Amp Hot Start Taq Mastermix (New England Biolabs, Ipswich, MA), DMSO (5% final concentration), and 5 μL of template. PCR was run for 40 cycles (with a 3 min and 30 s extension time) according to manufacturer instructions. Amplicons were visualized via gel electrophoresis alongside a 1 kilobase ladder.
2.7. Library preparation for nanopore sequencing
PCR reactions for each sample were combined and prepared for sequencing using ligation-based reagents from Oxford Nanopore Technologies (ONT; Oxford, United Kingdom) and adapted protocols (44). An overview of library preparation steps is illustrated in Figure 1A. Briefly, amplicons were purified using AMPure XP (Beckman Coulter, Brea, CA) and quantified using a Qubit™ Flex Fluorometer (ThermoFisher Scientific, Waltham, MA). Samples were normalized for concentration prior to a two-step “spike-in” method for DNA end repair and barcode ligation (44). Barcoded products were purified using AMPure XP, followed by adapter ligation and a final AMPure XP clean-up. Final eluate concentrations were measured, samples were pooled in equal ratios, and the final library was diluted to a concentration of 35 ng/μL. A 12 μL volume of the library was loaded onto an R9 flow cell according to manufacturer instructions. The run was sequenced on either a MinION Mk1C or a GridION platform with high-accuracy base calling until approximately 50 k reads per sample were obtained. Flow cells were washed according to manufacturer instructions and reused only if the flow cell retained sufficient active pores (>450) and only with uniquely barcoded samples to limit potential cross-contamination.
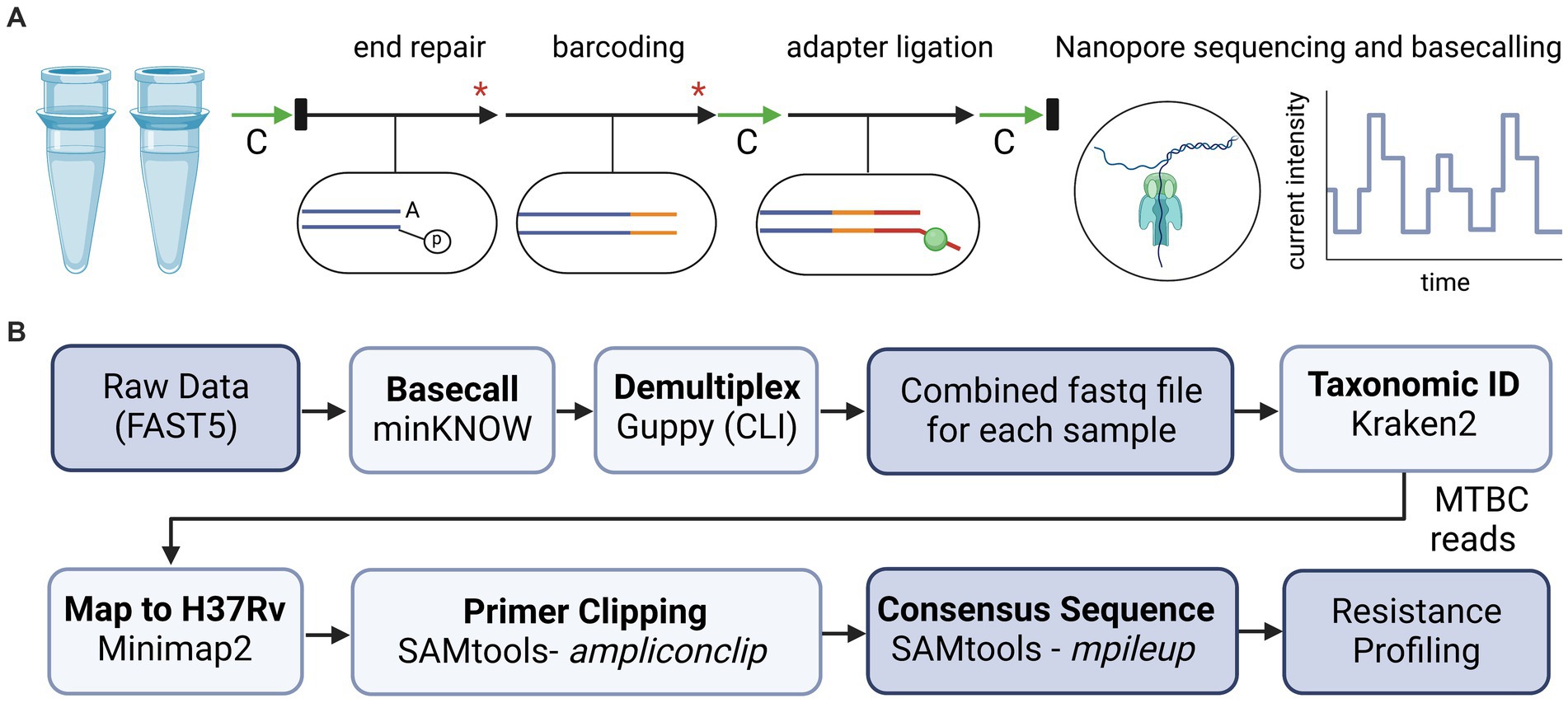
Figure 1. Overview of library preparation steps and bioinformatic analyses for tNGS nanopore sequencing. (A) For library preparation, two multiplex PCR reactions for each sample were combined and processed with AMPure bead-based clean-up steps (green arrows, “C”), enzymatic reactions (black arrows), dsDNA quantification via Qubit and normalization (black rectangles), and heat inactivation steps (red asterisk). (B) Bioinformatic tools used to analyze sequencing data and identify high confidence resistance mutations in MTBC. Diagrams created with BioRender.com.
2.8. Bioinformatic analysis
Oxford Nanopore Technologies sequencing data is analyzed in real-time using a custom bioinformatics pipeline (Figure 1B), akin to the NYS-validated WGS pipeline described in Shea et al. (26). The pipeline reads in each of the raw fastq files as they are generated using the MinKNOW interface on the instrument. Fastq files are demultiplexed using Guppy on a separate server via command line interface (CLI) with default parameters. Reads are combined into a final fastq file for each analyzed sample. The pipeline then assesses the taxonomic content of each file using Kraken2 (version 2.1.2) and the k2_standard_08gb_20220607 database (45). All non-Mycobacterium genus reads are filtered out for the rest of the downstream analyses. Reads are mapped to Mycobacterium tuberculosis H37Rv reference genome with minimap2 (version 2.24-r1122) (46) and amplicon primers sequences are hard-clipped from both ends using SAMtools (v 1.15.1) with ampliconclip (47). Finally, a high-quality consensus sequence is generated for each sample using SAMtools mpileup (47) with minimum mapping quality and base quality of Phred 30 and 12, respectively, and minimum depth of 10× and 60% allele agreement. Indels require 40× minimum depth and 55% allele agreement. In cases where indels are directly adjacent or inside homopolymeric regions of three or more identical bases, percent allele agreement is raised to 75%. If a position (variant or invariant) does not reach these requirements, it is assigned as ‘N’ on the consensus sequence. The pipeline identifies 86 high-confidence resistance mutations across the 51 positions listed in Supplementary Table S1, and notes novel/unknown mutations. The different cutoffs for single nucleotide polymorphisms (SNPs) and insertions/deletions (INDELs) were empirically determined by assessing the different allele frequencies (AF) over several runs and determining the best AF cutoff to avoid calling any false positive SNP or INDEL variants.
3. Results
3.1. Validation of tNGS for clinical use
To validate the tNGS assay for clinical use, we assessed sensitivity, reproducibility, specificity, and accuracy. To assess sensitivity of tNGS on respiratory specimens, a culture of the M. tuberculosis reference strain H37Rv (ATCC 25618) was serially diluted and spiked into processed negative sputa to determine the limit of detection (LOD). Average Ct-values for MTBC detection ranged from 24.2 to undetected and the concentration of M. tuberculosis in each PCR reaction ranged from 108 CFU to 0.00108 CFU (21,600 CFU to 0.216 CFU per mL). tNGS was performed on three replicate dilution series and sequenced to a total of approximately 80 k reads per sample. Quality control (QC) metrics were met for all targets (and corresponding drug classes) down to a lower detection limit of 0.108 CFU per reaction (Supplementary Table S3).
To measure reproducibility, three replicates of three smear positive specimens were processed in parallel (intra-assay) or on separate days (inter-assay). Results were concordant within and between runs, as shown in Supplementary Table S4. Specificity was tested against a panel of five organisms – including two mycobacteria (Mycobacterium fortuitum and Mycobacterium abscessus) and three other organisms common in sputa (Klebsiella pneumoniae, Streptococcus pneumoniae, and Haemophilus influenzae). No cross-reactivity was detected in this panel of organisms (Supplementary Table S5).
3.2. tNGS detection of drug resistance directly on respiratory specimens
To measure assay accuracy, tNGS was performed on a panel of 72 extracted primary specimens that were selected for their diverse mutations and drug resistance profiles. All specimens included in the panel were confirmed positive for MTBC DNA via real-time PCR. The panel consisted of 35 retrospective blinded samples and 37 prospective samples received over a period of 8 months (May 2022 to January 2023). The panel included predominantly sputa (n = 58, 81%) along with other respiratory specimens (i.e., bronchoalveolar lavages and bronchial washes) and rarer specimen types (i.e., lymph nodes and lung tissue). Specimens covered a range of MTBC concentrations (assessed by AFB smear and real-time PCR); most specimens included were AFB positive (n = 65, 90%), but five AFB negative samples and two untested samples were also included in the study (Supplementary Table S6).
The two multiplex PCR reactions were performed on the panel of specimens and amplification was confirmed by gel electrophoresis. Amplicons that could be visualized with ethidium bromide gel staining following PCR were present in 78% of the samples tested. High confidence resistance and unknown/novel mutations and DR profiles identified by tNGS were compared to those obtained from the NYS-validated WGS assay on isolates from the matched specimens. Profiles were defined according to CDC definitions: multidrug resistant (MDR; INH and RIF resistant), pre-extensively drug resistant (pre-XDR; INH, RIF, FQ), extensively drug resistant (XDR; INH, RIF, FQ, KAN/AMI). Resistance to other MTBC antimicrobials not meeting the criteria above is defined here as other mono- or poly-resistant (R). The results for each specimen are shown in Supplementary Table S6 and an aggregate summary is provided in Table 1. Of the MTBC-positive samples sequenced, tNGS correctly identified 44 pan-susceptible, 5 mono/poly-resistant, and 5 MDR, and 1 pre-XDR, and 1 XDR strain, all determined to have a DR profile identical to the WGS DR profile obtained from the culture isolate from the same case. At the mutation level, two tNGS reports identified additional unknown mutations in primary specimens that were not identified by WGS performed on cultured isolates. This raises the potential for tNGS to detect subpopulations in the primary clinical specimens. Overall, these results demonstrate that tNGS can accurately detect susceptible and DR forms of MTBC directly from primary specimens.
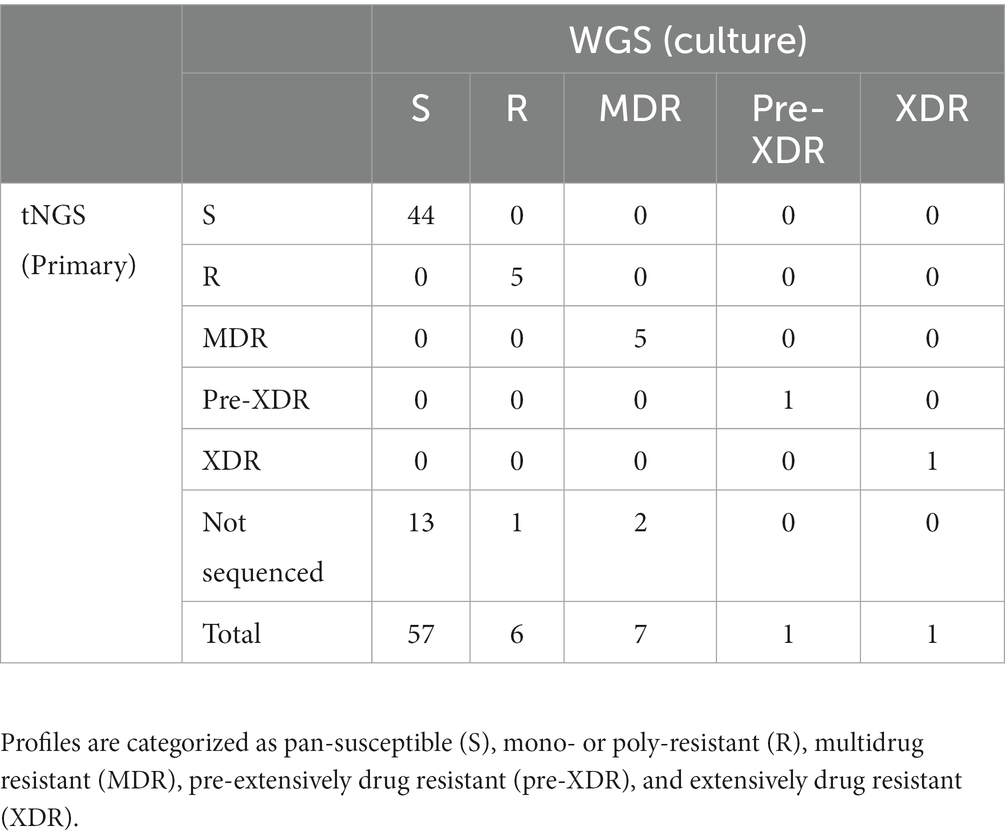
Table 1. Comparison of DR profiles identified by tNGS performed on primary specimens to WGS performed on matched MTBC-positive cultures.
3.3. Primary specimen tNGS data quality
To evaluate data quality, samples were categorized based on the number of targets that met quality control thresholds defined by the analysis pipeline, either as complete susceptibility profiles (all 13 targets pass QC), partial susceptibility profiles (≥10 targets pass QC), or no profile (not sequenced). In the panel of 72 primary specimens, tNGS produced 68% complete profiles, 10% partial profiles, and the remaining 22% were not sequenced due to PCR failure. These results indicate that targeted sequencing data can be obtained direct from primary specimens, although there is some variability in data quality.
To determine the factors that influence tNGS target failure and establish quality criteria for testing, the bacterial load in samples was estimated using AFB smear microscopy and MTBC real-time PCR Ct-values. Complete or partial profiles were obtained for 83% of the smear positive specimens tested (n = 65) (Figure 2A). Within the subset of smear positive samples, these percentages correlated with AFB smear results: 100% of samples with numerous AFB produced complete profiles, whereas complete or partial profiles were obtained for 93% of AFB moderate, 86% of AFB few, and 55% of AFB rare samples (Figure 2B). Of the five smear negative samples tested, only one specimen yielded a susceptibility profile; however, this sample had a low Ct-value uncharacteristic of a smear negative result (Supplementary Table S6). A smear could not be performed on several specimens due to specimen viscosity or insufficient volume for testing. These results indicate that target amplification is dependent on the quantity of AFB cells in the specimen and suggest that AFB smear-positive specimens are the most likely to yield complete susceptibility profiles.
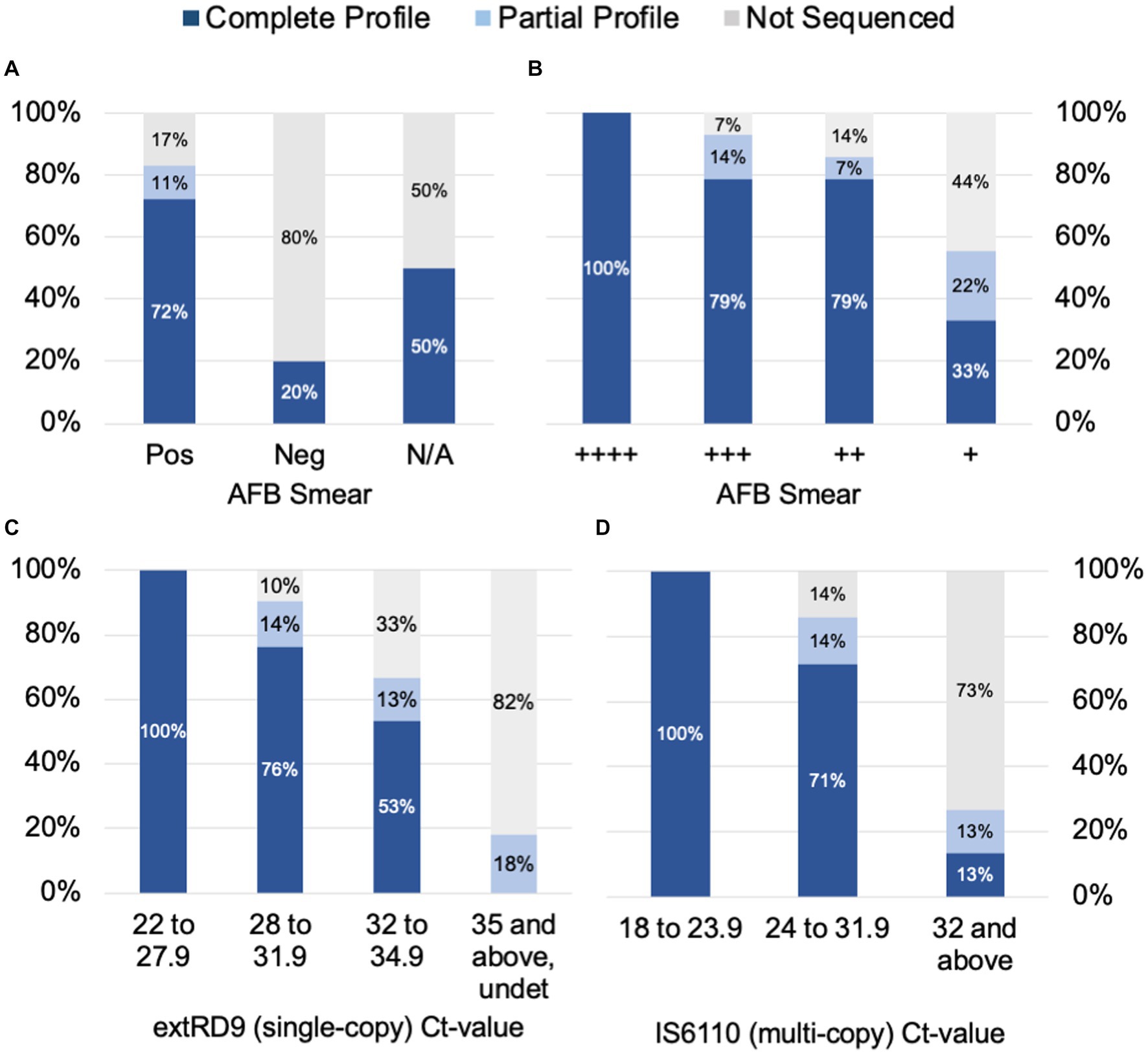
Figure 2. tNGS data completeness is arranged by AFB smear (A) and (B) tNGS data completeness is arranged by AFB smear and real-time PCR values for (C) a single-copy target RD9 and (D) multi-copy target IS6110 for MTBC. Profiles are defined as complete (all 13 targets pass QC), partial (≥10 targets pass QC), or not sequenced.
Samples were also stratified by Ct-values derived from MTBC real-time PCR testing. Ct-values for the single-copy MTBC target (ext-RD9) ranged from 22.1 to 37.4 (or undetected) (Supplementary Table S6). Lower Ct-values yielded more complete tNGS sequencing results (Figure 2C); for values of 34.9 and below, 89% of samples yielded either complete or partial susceptibility profiles. In contrast, samples with Ct-values ≥35 were more prone to PCR failure (82%) and only two samples above this threshold produced a partial profile. Examination of Ct-values for IS6110, which is a multi-copy target and considered a more sensitive marker for MTBC detection, showed similar trends but with different ranges (Figure 2D). IS6110 Ct-values ranged from 18.4 to 38.0 in the primary specimens tested (Supplementary Table S6). Samples with Ct-values ≤31.9 yielded either complete or partial susceptibility profiles (91%), whereas Ct-values ≥32 more were more prone to PCR failure (73%). These results indicate the quality of tNGS data is dependent on the amount of MTBC DNA present in the specimen and further suggests that quantification via real-time PCR may be used as a reliable metric for assessing sample quality for tNGS.
3.4. tNGS improves turnaround times
The ability of tNGS to generate comprehensive susceptibility profiles directly from a patient specimen has the potential to reduce turnaround times. A subset of 16 primary specimens with matched WGS results were used to calculate turnaround times; samples included in the analysis had tNGS performed as part of the routine testing algorithm (i.e., initiated within 1 week of MTBC detection) and yielded a positive MGIT culture suitable for WGS (Figure 3A). The average number of days required for MTBC detection via real-time PCR, tNGS (from extraction to result), MTBC isolation, and WGS (from extraction to result) are shown in Figure 3B.
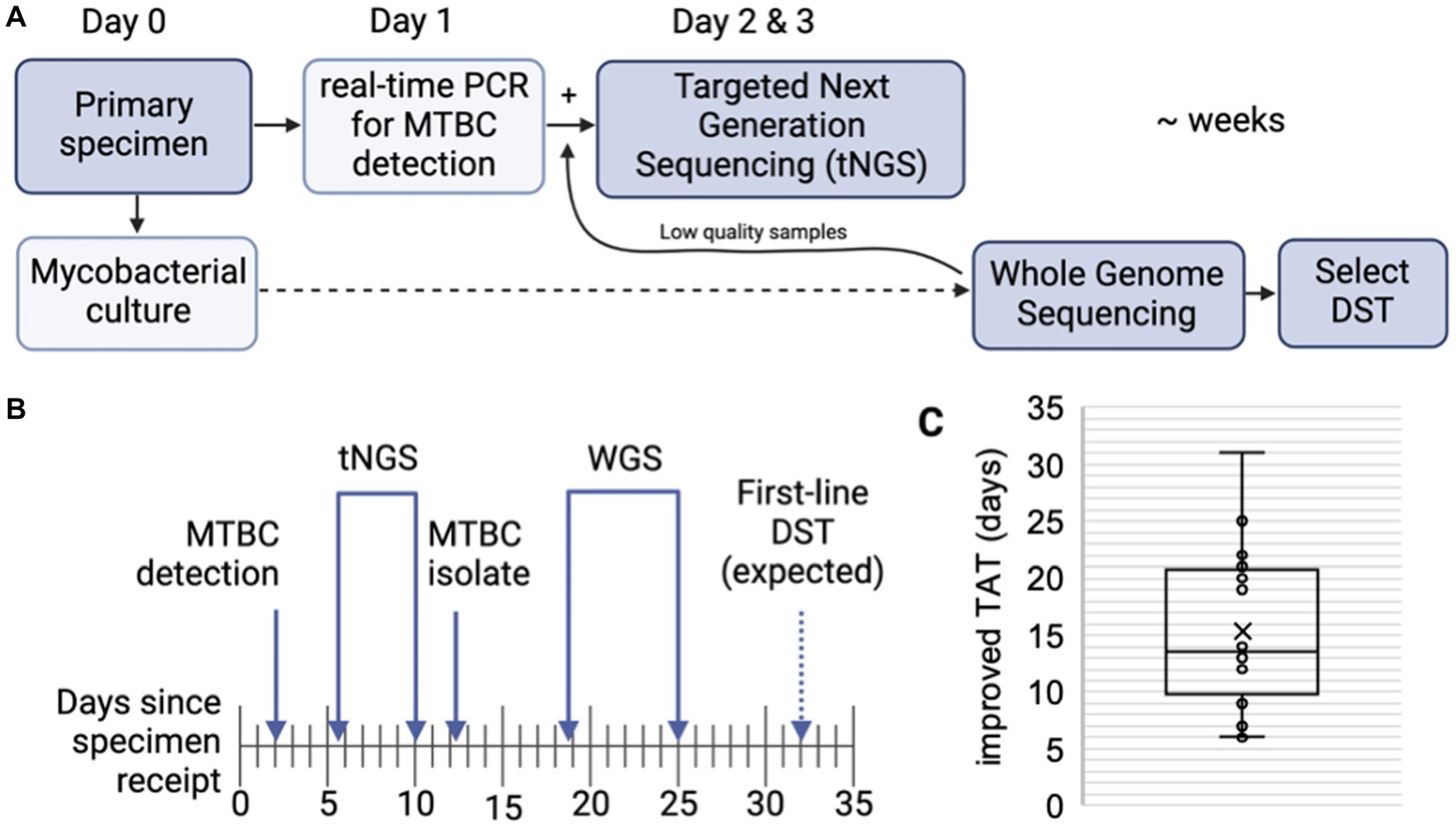
Figure 3. Turnaround times for MTBC molecular testing and sequencing. (A) MTBC testing algorithm at the Wadsworth Center. Processed specimens are used for mycobacterial culture. Heat killed aliquots are tested for MTBC DNA via real-time PCR and positive specimens are then referred to tNGS. When positive cultures are available, WGS is performed. Phenotypic drug-susceptibility testing (DST) is performed only if unknown/novel mutations or multidrug resistant strains are detected. (B) Timeline showing the average number of days required for MTBC DNA detection via real-time PCR, tNGS (from extraction to result), MTBC isolation, and WGS (from extraction to result) (n = 16). Note that tNGS and WGS assays are batched weekly and average turnaround times include non-business days (i.e., weekends). Estimated time for first-line DST results are indicated with a dashed arrow. (C) Turnaround time (TAT) improvements (in days) of direct tNGS compared to culture-derived WGS.
Both tNGS and WGS samples were batched and run weekly. On average, tNGS results were available 10 days from sample receipt (or 7 business days if excluding weekends) (Figure 3B). This represents a 15 day reduction in turnaround time for tNGS versus WGS, with the improvement among samples ranging from 6 to 31 days (Figure 3C). Notably, one specimen included in this study was identified as XDR using tNGS and these results were available within 5 days from sample receipt, whereas culture-based WGS results were not available for an additional 3 weeks. These differences in turnaround time can largely be attributed to the incubation period required to obtain an AFB-positive culture and subsequent characterization (average 12.2 days); however, we also found that processing times – from sample extraction to final result – were shorter for nanopore-based tNGS (4.3 days or 2.3 business days) compared to Illumina-based WGS (6.3 days) in our current workflows (Figure 3B). These results demonstrate nanopore-based tNGS can offer comprehensive DR detection before MTBC isolates are available for WGS or culture-based DST.
3.5. tNGS on MTBC-positive cultures
tNGS may also provide additional utility for identifying high-confidence and unknown/novel mutations within MTBC-positive cultures. tNGS was performed on a panel of 55 MTBC-positive cultures, 21 of which were dual-positive for M. avium complex. Complete profiles were obtained for 100% isolates, whereas dual-positive cultures yielded either complete (86%) or partial profiles (14%) (Supplementary Table S7). Profiles were in 100% concordance with WGS (Table 2); however, two dual-positive samples did not have WGS available for comparison due to failure to obtain pure MTBC culture. These results demonstrate that tNGS can build comprehensive DR profiles from cultured material, even for mixed cultures that may not meet quality criteria for WGS analysis.
3.6. tNGS SNP-based lineage prediction
In addition to DR profiling, tNGS data can also be used to identify the seven main phylogenetic MTBC lineages to provide supporting data during epidemiological investigations. In silico lineage predictions tools often utilize single-nucleotide polymorphisms (SNPs) to classify each lineage, but these SNP catalogs may vary (48). A SNP-based algorithm for lineage prediction was designed using the targets available in the tNGS assay (Figure 4). This algorithm initially relies on gyrB mutations that distinguish M. tuberculosis (gyrB 403 GCG mutation) from other Mycobacterium species (gyrB 403 TCG). For M. tuberculosis strains, the algorithm identifies markers for lineage 1 (gyrB 291 ATC), lineage 3 (oxyR-ahpC, −88 A), and lineage 4 (katG 463 CGG). MTBC strains not falling into these categories are classified as likely lineage 2; “likely” reflects the limitation that lineage 2 cannot be distinguished from the rarer lineage 7 with this set of loci. Other members of the MTBC are identified as lineage 5 (ethA 124 GAC), lineage 6 or 9 (inhA 78 GCG), M. bovis or bovis BCG (pncA 57 GAC), M. orygis (gyrB 290 GCA), or M. caprae (gyrB 356 GCG). Strains not fitting these criteria are not assigned with a lineage determination.
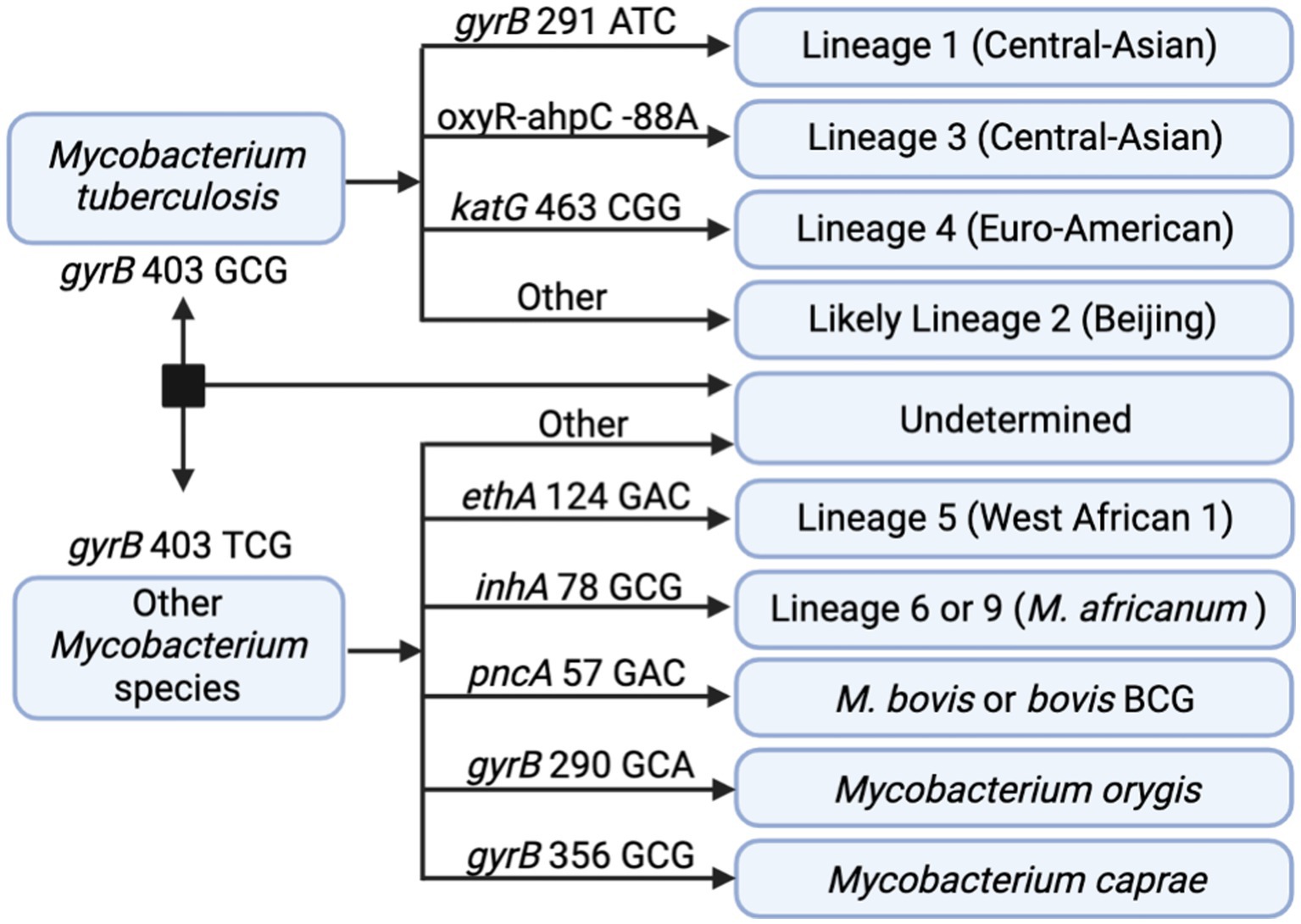
Figure 4. In silico SNP-based lineage classifications for MTBC. The SNP-based ID algorithm looks for unique SNPs in gyrB, oxyR-ahpC, katG, ethA, inhA, and pncA in the order shown below. Diagram created with BioRender.com.
These SNP-based lineage predictions were performed on all samples included in this study (primary specimens and cultures) where both tNGS and WGS results were available (n = 109). This panel included lineages 1–4 and included one M. bovis BCG strain. Comparison of lineages derived in silico from tNGS and WGS are shown in Table 3. 98.2% of lineages were correctly identified by tNGS, 0.9% were undetermined due to target failure, and one lineage 4 strain (0.9%) was identified as “likely lineage 2” due to a heterogeneous SNP at katG 463. These results show that SNP-based lineage predictions are possible and highly accurate using a small number of loci.
3.7. Fiscal analysis of tNGS
The cost associated with nanopore-based tNGS is detailed in Table 4. The fixed cost per sample includes reagents for extraction ($12.17), PCR ($5.90) and library preparation ($37.21). The cost of gel electrophoresis is not included as this is considered an optional step. Some tNGS costs per sample are dependent on batch size; for example, each tNGS sequencing run requires $25.90 of reagents for flow cell priming, loading, and washing/storing regardless of the number of samples run. Other costs depend on flow cell reusage; we determined costs based on an average of 8 samples per run and up to three flow cell uses. Based on these estimates, the total estimated cost is $78.31 per sample. This analysis also does not include plastic consumables, technician time, instrumentation, or facility overhead as these factors may be facility specific and add to the overall price of the test. The cost per sample is similar to the cost of high-throughput WGS sequencing currently performed at the Wadsworth Center (49). These analyses indicate that tNGS assays can be cost-effective for implementation in diagnostic/clinical laboratories.
4. Discussion
4.1. tNGS is sensitive, scalable, and reliable for rapid prediction of drug resistance
tNGS represents a sensitive, reliable, and cost-effective method for detecting DR-TB direct from primary specimens in a clinical or diagnostic laboratory setting. This assay accurately identified diverse DR profiles – including MDR and XDR strains – with easier set-up than single-target assays and faster turnaround times than testing performed on cultured MTBC isolates, including WGS and phenotypic DST. This laboratory-developed tNGS assay represents an improvement to our current testing algorithm by offering comprehensive DR profiling shortly after TB diagnosis. Our study revealed a 15 day improvement in turnaround time compared to culture-based WGS, but additional experience will continue to inform tNGS implementation and improve the time to result.
4.2. tNGS assays require careful selection of targets and high-confidence mutations
tNGS assays require careful selection of targets and high confidence resistance mutations (50). Our assay targets full-length loci associated with resistance to first- and second-line MTBC antimicrobials and is consistent with other targeted assays (31–38), with some variation in number and size of loci included. In contrast to molecular beacon and line probe assays which focus just on hot spot regions, the assay described in this study examines full-length genes and promoter regions of many targets to allow for detection of rare and atypical resistance mutations. Although most smear positive specimens yielded complete susceptibility profiles, we found that longer targets (i.e., embB, rpoB) were more prone to low-coverage or amplification failure, resulting in partial susceptibility profiles. This observation suggests that sensitivity may be improved by splitting larger loci into multiple overlapping amplicons. One additional limitation of tNGS assays is that amplification may fail if strains carry mutations or deletions in primer binding regions, but these undetermined results will be further understood upon reflexing to WGS or culture-based DST in diagnostic testing algorithms (Figure 3A).
The accuracy of sequencing-based predictions for TB DR compared to phenotypic DST have been previously established for WGS (26); however, both tNGS and WGS assays require regular updates to keep pace with the emergence of new DR mutations. In the current Wadsworth Center testing algorithm, isolates with novel mutations undergo DST in order to characterize the potential impact of these mutations. A minimum of three isolates with paired phenotypic DST results or strong supporting literature are required to move novel mutations – initially reported as “unknowns” – to either a neutral or high confidence DR mutation list (26). Laboratories with smaller testing catalogs may refer to the WHO database (51) or other supporting literature to supplement their high confidence DR mutation list. tNGS assays may be updated with additional targets or multiplex pools to keep pace with emerging need, such as genotypic DST predictions for drugs included in the BPaLM/BPaL (bedaquiline, pretomanid, linezolid, moxifloxacin) regimens for treating MDR and XDR infections (52).
4.3. Considerations for implementing Oxford Nanopore sequencing
Special considerations and workflow adaptations are required for using Oxford Nanopore Technologies sequencing platforms. Raw data files from nanopore sequencing devices are basecalled into fastq files that are available for analysis. Newer versions of these basecalling algorithms continually provide better sequencing accuracy and, depending on the algorithm and flow cell version used, these accuracies can approach and even surpass Illumina-based platforms (53). These improvements demand greater processing requirements and thus can create lag times between sequencing and fastq file generation, thus, the use of graphics processing unit (GPU) or Cloud computing resources are highly recommended for basecalling and data post-processing (54, 55). Both the MinION Mk1C and GridION platforms from Oxford Nanopore Technologies were used in this study. While both platforms were able to take advantage of their GPUs for basecalling, we found that the compute power of GridION was able to perform high accuracy basecalling in real-time, enhancing turnaround times compared to the MinION Mk1C. The GridION, however, occupies a larger footprint in the laboratory and is less portable than the Mk1C for applications in resource-limited settings.
Future applications of this technology include detection of heterozygous positions, but this is currently limited by the accuracy of the sequencing data. Newer nanopore chemistries paired with more advanced basecalling algorithms show improved accuracy and potential for heterozygous detection (56). However, these updates to chemistry also necessitate frequent validation and verification. Thus, adoption of commercial products with longevity are critical for clinical implementation and use.
Consistent with other studies (40), we found that nanopore-based tNGS was cost-effective and comparable to current high-throughput WGS assays. Nevertheless, nanopore costs can vary widely depending on batch sizes and flow cell usage. To minimize cost, this validation study successfully obtained accurate tNGS data with re-used flow cells; however, we suggest using unique barcodes for each run to limit potential cross-contamination in clinical testing. Laboratories with lower testing volumes may consider combing multiple targeted assays onto one nanopore flow cell.
In conclusion, this study demonstrates the utility of a clinical tNGS assay as an early detection method for drug resistance direct from MTBC-positive specimens. This particular tNGS assay showed more than a two-week improvement in turnaround time compared to culture and WGS workflows at a similar cost. This method also offers additional utility for cultures that are low quality for WGS analysis due to mixed organisms or low MTBC DNA concentration. Early detection methods are an essential part of TB testing algorithms to ensure that patients are expeditiously placed on appropriate drug treatment regimens.
Data availability statement
Illumina and MinION sequencing datasets are available in BioProject PRJNA766641 (https://www.ncbi.nlm.nih.gov/bioproject/?term=PRJNA766641) under the accession numbers listed in Supplementary Table S8.
Author contributions
SM, CS, PL, and KM contributed to conception and design of the study. SM and CS optimized the targeted sequencing methods. SM, CS, KP, and JS collected data. JS identified mutations for in silico lineage prediction. PL developed bioinformatic pipelines and wrote sections of the manuscript. SM, PL, TH, MD, VE, M-CR, and KM provided feedback for implementation in the public health laboratory. SM wrote the first draft of the manuscript. All authors contributed to the article and approved the submitted version.
Funding
This research project was supported by a grant from the National Institute of Allergy and Infectious Diseases (5R21AI13985602) and partially supported by NU50CK000516 (Epidemiology and Laboratory Capacity for Prevention and Control of Emerging Infectious Diseases in New York State).
Acknowledgments
We acknowledge the Wadsworth Center Applied Genomic Technologies Core Facility for next generation DNA sequencing on Illumina platforms. We also thank Wolfgang Haas, Donna Kohlerschmidt, Susan Wolfe, Amy Chiefari, Alexandra Fiero, Kara Tomlin, Emily McGrath, James Long, and Justine Edwards for their technical contributions. Figures were created with BioRender.com and licensed under the following agreements: MC25HMBAEH, QT258GKZIS, and NC258GL23E.
Conflict of interest
The authors declare that the research was conducted in the absence of any commercial or financial relationships that could be construed as a potential conflict of interest.
Publisher’s note
All claims expressed in this article are solely those of the authors and do not necessarily represent those of their affiliated organizations, or those of the publisher, the editors and the reviewers. Any product that may be evaluated in this article, or claim that may be made by its manufacturer, is not guaranteed or endorsed by the publisher.
Supplementary material
The Supplementary material for this article can be found online at: https://www.frontiersin.org/articles/10.3389/fpubh.2023.1206056/full#supplementary-material
References
1. World Health Organization. Annual report of tuberculosis. Annual Global TB Report of WHO (2022) 8:1–68. Available at: https://www.who.int/teams/global-tuberculosis-programme/tb-reports/global-tuberculosis-report-2022 (Accessed March 13, 2023).
2. Torres, NMC, Julieth, J, Rodríguez, JJQ, Andrade, PSP, Arriagaid, MB, and Martins, NE. Factors predictive of the success of tuberculosis treatment: a systematic review with meta-analysis. PLoS One. (2019) 14:e0226507. doi: 10.1371/journal.pone.0226507
3. Lam, E, Nateniyom, S, Whitehead, S, Anuwatnonthakate, A, Monkongdee, P, Kanphukiew, A, et al. Use of drug-susceptibility testing for Management of Drug-Resistant Tuberculosis, Thailand, 2004–2008. Emerg Infect Dis. (2014) 20:408–16. doi: 10.3201/EID2003.130951
4. Xu, C, Li, R, Shewade, HD, Jeyashree, K, Ruan, Y, Zhang, C, et al. Attrition and delays before treatment initiation among patients with MDR-TB in China (2006–13): magnitude and risk factors. PLoS One. (2019) 14:e0214943. doi: 10.1371/JOURNAL.PONE.0214943
5. Doulla, BE, Squire, SB, MacPherson, E, Ngadaya, ES, Mutayoba, BK, and Langley, I. Routine surveillance for the identification of drug resistant tuberculosis in Tanzania: a cross-sectional study of stakeholders’ perceptions. PLoS One. (2019) 14:e0212421. doi: 10.1371/JOURNAL.PONE.0212421
6. Zhu, J, Bao, Z, Xie, Y, Werngren, J, Hu, Y, Davies Forsman, L, et al. Additional drug resistance for Mycobacterium tuberculosis during turnaround time for drug-susceptibility testing in China: a multicenter observational cohort study. Int J Infect Dis. (2021) 108:81–8. doi: 10.1016/J.IJID.2021.04.027
7. van Beek, J, Haanperä, M, Smit, PW, Mentula, S, and Soini, H. Evaluation of whole genome sequencing and software tools for drug susceptibility testing of Mycobacterium tuberculosis. Clin Microbiol Infect. (2019) 25:82–6. doi: 10.1016/J.CMI.2018.03.041
8. Van Der Werf, MJ, Langendam, MW, Huitric, E, and Manissero, D. Multidrug resistance after inappropriate tuberculosis treatment: a meta-analysis. Eur Respir J. (2012) 39:1511–9. doi: 10.1183/09031936.00125711
9. Blakemore, R, Story, E, Helb, D, Kop, JA, Banada, P, Owens, MR, et al. Evaluation of the analytical performance of the Xpert MTB/RIF assay. J Clin Microbiol. (2010) 48:2495–501. doi: 10.1128/JCM.00128-10
10. Ling, DI, Zwerling, AA, and Pai, M. GenoType MTBDR assays for the diagnosis of multidrug-resistant tuberculosis: a meta-analysis. Eur Respir J. (2008) 32:1165–74. doi: 10.1183/09031936.00061808
11. Lawn, SD, and Nicol, MP. Xpert® MTB/RIF assay: development, evaluation and implementation of a new rapid molecular diagnostic for tuberculosis and rifampicin resistance. Future Microbiol. (2011) 6:1067–82. doi: 10.2217/FMB.11.84
12. Theron, G, Zijenah, L, Chanda, D, Clowes, P, Rachow, A, Lesosky, M, et al. Feasibility, accuracy, and clinical effect of point-of-care Xpert MTB/RIF testing for tuberculosis in primary-care settings in Africa: a multicentre, randomised, controlled trial. Lancet. (2014) 383:424–35. doi: 10.1016/S0140-6736(13)62073-5
13. Chakravorty, S, Simmons, AM, Rowneki, M, Parmar, H, Cao, Y, Ryan, J, et al. The new Xpert MTB/RIF ultra: improving detection of Mycobacterium tuberculosis and resistance to rifampin in an assay suitable for point-of-care testing. MBio. (2017) 8:e00812. doi: 10.1128/MBIO.00812-17
14. The use of molecular line probe assays for the detection of resistance to second-line anti-tuberculosis drugs: policy guidance. Available at: https://apps.who.int/iris/handle/10665/246131 (Accessed March 13, 2023).
15. Akalu, GT, Tessema, B, and Petros, B. High proportion of RR-TB and mutations conferring RR outside of the RRDR of the rpoB gene detected in GeneXpert MTB/RIF assay positive pulmonary tuberculosis cases, in Addis Ababa Ethiopia. PLoS One. (2022) 17:e0277145. doi: 10.1371/JOURNAL.PONE.0277145
16. Mvelase, NR, Cele, LP, Singh, R, Naidoo, Y, Giandhari, J, Wilkinson, E, et al. Consequences of rpoB mutations missed by the GenoType MTBDRplus assay in a programmatic setting in South Africa. Afr J Lab Med. (2023) 12:1975. doi: 10.4102/AJLM.V12I1.1975
17. Fitzgibbon, MM, Roycroft, E, Sheehan, G, Mc Laughlin, AM, Quintyne, KI, Brabazon, E, et al. False detection of rifampicin resistance using Xpert® MTB/RIF ultra assay due to an A451V mutation in Mycobacterium tuberculosis. JAC Antimicrob Resist. (2021) 3:dlab101. doi: 10.1093/JACAMR/DLAB101
18. Ajileye, A, Alvarez, N, Merker, M, Walker, TM, Akter, S, Brown, K, et al. Some synonymous and nonsynonymous gyrA mutations in Mycobacterium tuberculosis lead to systematic false-positive fluoroquinolone resistance results with the Hain GenoType MTBDRsl assays. Antimicrob Agents Chemother. (2017) 61:e02169. doi: 10.1128/AAC.02169-16
19. Getachew, E, Adebeta, T, Gebrie, D, Charlie, L, Said, B, Assefa, DG, et al. Pyrosequencing for diagnosis of multidrug and extensively drug-resistant tuberculosis: a systemic review and meta-analysis. J Clin Tuberc Other Mycobact Dis. (2021) 24:100254. doi: 10.1016/J.JCTUBE.2021.100254
20. Halse, TA, Edwards, J, Cunningham, PL, Wolfgang, WJ, Dumas, NB, Escuyer, VE, et al. Combined real-time PCR and rpoB gene pyrosequencing for rapid identification of Mycobacterium tuberculosis and determination of rifampin resistance directly in clinical specimens. J Clin Microbiol. (2010) 48:1182–8. doi: 10.1128/JCM.02149-09
21. Zheng, R, Zhu, C, Guo, Q, Qin, L, Wang, J, Lu, J, et al. Pyrosequencing for rapid detection of tuberculosis resistance in clinical isolates and sputum samples from re-treatment pulmonary tuberculosis patients. BMC Infect Dis. (2014) 14:1–8. doi: 10.1186/1471-2334-14-200/TABLES/5
22. Zhao, JR, Bai, YJ, Zhang, QH, Wang, Y, Luo, M, and Yan, XJ. Pyrosequencing-based approach for rapid detection of rifampin-resistant Mycobacterium tuberculosis. Diagn Microbiol Infect Dis. (2005) 51:135–7. doi: 10.1016/J.DIAGMICROBIO.2004.10.001
23. Mesfin, EA, Merker, M, Beyene, D, Tesfaye, A, Shuaib, YA, Addise, D, et al. Prediction of drug resistance by sanger sequencing of Mycobacterium tuberculosis complex strains isolated from multidrug resistant tuberculosis suspect patients in Ethiopia. PLoS One. (2022) 17:e0271508. doi: 10.1371/JOURNAL.PONE.0271508
24. Witney, AA, Cosgrove, CA, Arnold, A, Hinds, J, Stoker, NG, and Butcher, PD. Clinical use of whole genome sequencing for Mycobacterium tuberculosis. BMC Med. (2016) 14:46. doi: 10.1186/S12916-016-0598-2
25. Cox, H, Goig, GA, Salaam-Dreyer, Z, Dippenaar, A, Reuter, A, Mohr-Holland, E, et al. Whole-genome sequencing has the potential to improve treatment for rifampicin-resistant tuberculosis in high-burden settings: a retrospective cohort study. J Clin Microbiol. (2022) 60:e0236221. doi: 10.1128/jcm.02362-21
26. Shea, J, Halse, TA, Lapierre, P, Shudt, M, Kohlerschmidt, D, Van Roey, P, et al. Comprehensive whole-genome sequencing and reporting of drug resistance profiles on clinical cases of Mycobacterium tuberculosis in New York state. J Clin Microbiol. (2017) 55:1871–82. doi: 10.1128/JCM.00298-17
27. Catalogue of mutations in Mycobacterium tuberculosis complex and their association with drug resistance. Available at: https://www.who.int/publications/i/item/9789240028173 (Accessed March 13, 2023).
28. Park, M, Lalvani, A, Satta, G, and Kon, OM. Evaluating the clinical impact of routine whole genome sequencing in tuberculosis treatment decisions and the issue of isoniazid mono-resistance. BMC Infect Dis. (2022) 22:349. doi: 10.1186/S12879-022-07329-Y
29. Olaru, ID, Patel, H, Kranzer, K, and Perera, N. Turnaround time of whole genome sequencing for mycobacterial identification and drug susceptibility testing in routine practice. Clin Microbiol Infect. (2018) 24:659.e5–7. doi: 10.1016/J.CMI.2017.10.001
30. Tortoli, E, Cichero, P, Piersimoni, C, Simonetti, MT, Gesu, G, and Nista, D. Use of BACTEC MGIT 960 for recovery of mycobacteria from clinical specimens: multicenter study. J Clin Microbiol. (1999) 37:3578–82. doi: 10.1128/JCM.37.11.3578-3582.1999
31. Leung, KSS, Tam, KKG, Ng, TTL, Lao, HY, Shek, RCM, Ma, OCK, et al. Clinical utility of target amplicon sequencing test for rapid diagnosis of drug-resistant Mycobacterium tuberculosis from respiratory specimens. Front Microbiol. (2022) 13:974428. doi: 10.3389/FMICB.2022.974428
32. Wu, S-H, Xiao, Y-X, Hsiao, H-C, and Jou, R. Development and assessment of a novel whole-gene-based targeted next-generation sequencing assay for detecting the susceptibility of Mycobacterium tuberculosis to 14 drugs. Microbiol Spectr. (2022) 10:e0260522. doi: 10.1128/spectrum.02605-22
33. Gliddon, HD, Frampton, D, Munsamy, V, Heaney, J, Pataillot-Meakin, T, Nastouli, E, et al. A rapid drug resistance genotyping workflow for Mycobacterium tuberculosis, using targeted isothermal amplification and nanopore sequencing. Microbiol Spectr. (2021) 9:e0061021. doi: 10.1128/SPECTRUM.00610-21
34. Colman, RE, Anderson, J, Lemmer, D, Lehmkuhl, E, Georghiou, SB, Heaton, H, et al. Rapid drug susceptibility testing of drug-resistant Mycobacterium tuberculosis isolates directly from clinical samples by use of amplicon sequencing: a proof-of-concept study. J Clin Microbiol. (2016) 54:2058–67. doi: 10.1128/JCM.00535-16
35. Barbosa-Amezcua, M, Cuevas-Córdoba, B, Fresno, C, Haase-Hernández, JI, Carrillo-Sánchez, K, Mata-Rocha, M, et al. Rapid identification of drug resistance and phylogeny in M. tuberculosis, directly from sputum samples. Microbiol Spectr. (2022) 10:e0125222. doi: 10.1128/SPECTRUM.01252-22
36. Jouet, A, Gaudin, C, Badalato, N, Allix-Béguec, C, Duthoy, S, Ferré, A, et al. Deep amplicon sequencing for culture-free prediction of susceptibility or resistance to 13 anti-tuberculous drugs. Eur Respir J. (2021) 57:2002338. doi: 10.1183/13993003.02338-2020
37. Bonnet, I, Enouf, V, Morel, F, Ok, V, Jaffré, J, Jarlier, V, et al. A comprehensive evaluation of GeneLEAD VIII DNA platform combined to Deeplex Myc-TB® assay to detect in 8 days drug resistance to 13 Antituberculous drugs and transmission of Mycobacterium tuberculosis complex directly from clinical samples. Front Cell Infect Microbiol. (2021) 11:707244. doi: 10.3389/FCIMB.2021.707244/FULL
38. Park, J, Jang, W, Kim, M, Kim, Y, Shin, SY, Park, K, et al. Molecular drug resistance profiles of Mycobacterium tuberculosis from sputum specimens using ion semiconductor sequencing. J Microbiol Methods. (2018) 145:1–6. doi: 10.1016/J.MIMET.2017.12.003
39. Cabibbe, AM, Spitaleri, A, Battaglia, S, Colman, RE, Suresh, A, Uplekar, S, et al. Application of targeted next-generation sequencing assay on a portable sequencing platform for culture-free detection of drug-resistant tuberculosis from clinical samples. J Clin Microbiol. (2020) 58:e00632. doi: 10.1128/JCM.00632-20
40. Tafess, K, Ng, TTL, Lao, HY, Leung, KSS, Tam, KKG, Rajwani, R, et al. Targeted-sequencing workflows for comprehensive drug resistance profiling of Mycobacterium tuberculosis cultures using two commercial sequencing platforms: comparison of analytical and diagnostic performance, turnaround time, and cost. Clin Chem. (2020) 66:809–20. doi: 10.1093/CLINCHEM/HVAA092
41. Tran, AC, Halse, TA, Escuyer, VE, and Musser, KA. Detection of Mycobacterium avium complex DNA directly in clinical respiratory specimens: opportunities for improved turn-around time and cost savings. Diagn Microbiol Infect Dis. (2014) 79:43–8. doi: 10.1016/J.DIAGMICROBIO.2014.01.019
42. Untergasser, A, Cutcutache, I, Koressaar, T, Ye, J, Faircloth, BC, Remm, M, et al. Primer3—new capabilities and interfaces. Nucleic Acids Res. (2012) 40:e115. doi: 10.1093/NAR/GKS596
43. Breslauer, KJ, Frank, R, Blocker, H, and Marky, LA. Predicting DNA duplex stability from the base sequence. Proc Natl Acad Sci U S A. (1986) 83:3746–50. doi: 10.1073/PNAS.83.11.3746
44. One-pot native barcoding of amplicons v2. Available at: https://www.protocols.io/view/one-pot-native-barcoding-of-amplicons-v2-bp2l6n3rkgqe/v1?step=21 (Accessed March 29, 2023).
45. Wood, DE, Lu, J, and Langmead, B. Improved metagenomic analysis with kraken 2. Genome Biol. (2019) 20:1–13. doi: 10.1186/S13059-019-1891-0/FIGURES/2
46. Li, H. Minimap2: pairwise alignment for nucleotide sequences. Bioinformatics. (2018) 34:3094–100. doi: 10.1093/BIOINFORMATICS/BTY191
47. Danecek, P, Bonfield, JK, Liddle, J, Marshall, J, Ohan, V, Pollard, MO, et al. Twelve years of SAMtools and BCFtools. Gigascience. (2021) 10:1–4. doi: 10.1093/GIGASCIENCE/GIAB008
48. Schleusener, V, Köser, CU, Beckert, P, Niemann, S, and Feuerriegel, S. Mycobacterium tuberculosis resistance prediction and lineage classification from genome sequencing: comparison of automated analysis tools. Sci Rep. (2017) 7:46327. doi: 10.1038/SREP46327
49. Rowlinson, MC, and Musser, KA. Current methods and role of next-generation sequencing in the diagnosis of antimicrobial resistance in tuberculosis. Clin Microbiol Newsl. (2022) 44:1–12. doi: 10.1016/J.CLINMICNEWS.2021.12.001
50. Cohen, KA, Manson, AL, Desjardins, CA, Abeel, T, and Earl, AM. Deciphering drug resistance in Mycobacterium tuberculosis using whole-genome sequencing: progress, promise, and challenges. Genome Medicine 2019 11:1. (2019) 11:1–18. doi: 10.1186/S13073-019-0660-8
51. WHO Announces updated definitions of extensively drug-resistant tuberculosis. Available at: https://www.who.int/news/item/27-01-2021-who-announces-updated-definitions-of-extensively-drug-resistant-tuberculosis (Accessed March 22, 2023).
52. Conradie, F, Diacon, AH, Ngubane, N, Howell, P, Everitt, D, Crook, AM, et al. Treatment of highly drug-resistant pulmonary tuberculosis. N Engl J Med. (2020) 382:893–902. doi: 10.1056/NEJMOA1901814/SUPPL_FILE/NEJMOA1901814_DATA-SHARING.PDF
53. Sanderson, ND, Kapel, N, Rodger, G, Webster, H, Lipworth, S, Street, TL, et al. Comparison of R9.4.1/Kit10 and R10/Kit12 Oxford Nanopore flowcells and chemistries in bacterial genome reconstruction. Microb Genom. (2023) 9:mgen000910. doi: 10.1099/mgen.0.000910
54. Lin, B, Hui, J, and Mao, H. Nanopore technology and its applications in gene sequencing. Biosensors. (2021) 11:214. doi: 10.3390/bios11070214
55. Gorzynski, JE, Goenka, SD, Shafin, K, Jensen, TD, Fisk, DG, Grove, ME, et al. Ultrarapid nanopore genome sequencing in a critical care setting. N Engl J Med. (2022) 386:700–2. doi: 10.1056/NEJMc2112090
Keywords: mycobacterium, tuberculosis, drug susceptibility, resistance, targeted sequencing, nanopore
Citation: Murphy SG, Smith C, Lapierre P, Shea J, Patel K, Halse TA, Dickinson M, Escuyer V, Rowlinson MC and Musser KA (2023) Direct detection of drug-resistant Mycobacterium tuberculosis using targeted next generation sequencing. Front. Public Health. 11:1206056. doi: 10.3389/fpubh.2023.1206056
Edited by:
Peera Hemarajata, Association of Public Health Laboratories, United StatesReviewed by:
Jennifer K. Spinler, Baylor College of Medicine, United StatesHannah Gray, University of California, Los Angeles, United States
Copyright © 2023 Murphy, Smith, Lapierre, Shea, Patel, Halse, Dickinson, Escuyer, Rowlinson and Musser. This is an open-access article distributed under the terms of the Creative Commons Attribution License (CC BY). The use, distribution or reproduction in other forums is permitted, provided the original author(s) and the copyright owner(s) are credited and that the original publication in this journal is cited, in accordance with accepted academic practice. No use, distribution or reproduction is permitted which does not comply with these terms.
*Correspondence: Kimberlee A. Musser, a2ltYmVybGVlLm11c3NlckBoZWFsdGgubnkuZ292