- 1Department of Critical Care Medicine, Gansu Provincial Hospital, Lanzhou, China
- 2The First Clinical Medical College, Gansu University of Chinese Medicine, Lanzhou, China
- 3College of Natural Resources and Environment, Northwest A&F University, Yangling, China
- 4Department of Respiratory Medicine, Gansu Provincial Hospital, Lanzhou, China
- 5Department of Medicine Solna, Karolinska Institutet and Center for Molecular Medicine, Karolinska University Hospital, Stockholm, Sweden
- 6Department of Respiratory Medicine, University of Helsinki, Helsinki, Finland
Introduction: Post-Acute Sequelae of SARS-CoV-2 Infection (PASC) presents a multifaceted interplay of demographic, clinical, environmental, and socioeconomic factors. Quantification at the individual level of these factors remains underexplored. Our study aims to address this knowledge gap by analyzing the long-term health implications of PASC, utilizing a comprehensive integration of spatiotemporal, clinical, environmental, and socioeconomic data.
Methods and analysis: The study will enroll over 4,000 confirmed COVID-19 patients from Gansu Provincial Hospital, treated from December 2022 to May 2023, as the baseline. These patients are spread across 14 cities in Gansu Province, with geographic coordinates ranging from 92°13′E to 108°46′E and 32°31’N to 42°57’N. Follow-ups will be conducted via structured telephone interviews at 24, 36, and 48 months post-discharge, from 2024 to 2027, to assess PASC and long-term health outcomes. Participants will be categorized into three age groups: children and teenagers (birth to 18 years), adults (18–65 years), and the older adult (over 65 years). Environmental and socioeconomic data corresponding to each case are also integrated. The primary objective is to assess the persistence and long-term health outcomes of PASC symptoms. Secondary objectives focus on evaluating the acute infection phase, its progression, and the efficacy of medical management strategies in influencing PASC trajectories. Mixed-effects models will be utilized to evaluate the impact of various factors on PASC, while spatiotemporal analyses will explore the correlations between environmental and socioeconomic conditions and the diagnosis and recovery trajectories of PASC.
Ethics and dissemination: The Gansu Provincial Hospital’s research ethics committee has approved this study protocol. Participation will be voluntary, with informed consent obtained from all participants. Study results will be published in peer-reviewed journals.
Clinical trial registration: ChiCTR2400091805.
Introduction
Post-Acute Sequelae of SARS-CoV-2 Infection (PASC), commonly referred to as Long COVID, encompasses a broad spectrum of persistent symptoms and medical complications that continue well beyond the initial COVID-19 infection phase (1–6). Symptoms varies widely, ranging from mild, non-specific issues like fatigue and headaches to severe, debilitating conditions including cardiopulmonary abnormalities and significant neurological deficits (7, 8). These manifestations significantly impair quality of life and pose ongoing challenges to global healthcare systems (9).
The risk factors contributing to PASC are not well understood. A complex array of demographic, clinical, environmental, and socioeconomic elements, may influence PASC (10, 11). There is substantial evidence suggesting that the interplay between biological and environmental components, including air quality, temperature, humidity, and pollution levels, significantly impacts respiratory illnesses, including COVID-19 (12–16). These conditions exacerbate respiratory symptoms and increase the risk of severe complications (14, 15). Socioeconomic disparities also play a crucial role, influencing individuals’ vulnerability and resilience to COVID-19 and its prolonged effects (17). Factors such as income inequality, access to healthcare, housing quality, and employment status profoundly affect an individual’s risk of virus exposure and their ability to receive timely and adequate medical care (18). Education level, social support networks, and community resources are pivotal in determining an individual’s capacity to manage and recover from the infection (19).
Despite established correlations between these variables and outcomes in respiratory and viral diseases, the specific impacts of environmental and socioeconomic conditions on the severity and manifestation of PASC remain insufficiently explored (20–22). Furthermore, the interactions between individual characteristics and environmental risks introduce complex pathways through which PASC develops and progresses (23). For example, residents of socioeconomically disadvantaged areas may encounter increased exposure to environmental hazards and have restricted access to healthcare services, thereby intensifying their risk of developing severe PASC symptoms. Nevertheless, there is a significant gap in comprehensively quantifying these risk factors at the level of individual patients, especially in identifying the interactions between personal characteristics and environmental risks. This deficiency underscores the necessity for an integrated approach that accounts for both individual and environmental risks in the personalized management of PASC patients. Developing a better understanding of these interactions is essential for formulating holistic strategies to prevent and mitigate the long-term health effects of COVID-19 across diverse populations. Such strategies will necessitate enhancing the precision and efficacy of interventions tailored to meet the unique needs of individual patients.
Given the complexities inherent in PASC, our research adopts a comprehensive and methodical approach to elucidate how environmental and socioeconomic variables influence the progression and recovery patterns of PASC across diverse demographic segments. This longitudinal study encompasses over 4,000 hospitalized COVID-19 patients from 14 cities across Gansu Province (425,800 square kilometers) (24), leveraging its unique geographic and climatic diversity (Figure 1). Positioned at the intersection of China’s Qinghai-Tibet, Loess, and Inner Mongolia plateaus, Gansu spans a wide range of landscapes, from mountainous terrains to vast plateaus, with elevations ranging from approximately 600 to over 5,600 meters and mean annual temperatures varying between −8 and 14°C (24–26). These environmental conditions are coupled with significant disparities in air pollution levels, as median annual concentrations of fine particulate matter (PM2.5) range from approximately 12–41 μg/m3, while sulfur dioxide (SO2) concentrations vary from 7 to 40 μg/m3 (27, 28). Such fluctuations in air quality, combined with varying levels of urbanization and green space coverage—quantified by the normalized difference vegetation index (NDVI), which ranges from nearly 0 to 1 (29), are likely to influence respiratory health and long-term COVID-19 outcomes. Economic and healthcare disparities further compound these environmental differences, as per capita gross domestic product (GDP) across the region varies nearly tenfold, from approximately 20,000 to over 150,000 Chinese yuan (30). Access to healthcare resources also differs significantly, with some cities having nearly twice the number of hospital beds per thousand people compared to others (30). This broad spectrum of environmental, economic, and healthcare conditions provides an ideal framework for investigating how these factors collectively shape the long-term impacts of COVID-19, particularly in relation to PASC risk and recovery trajectories. By systematically integrating these variables, our study aims to develop a more comprehensive understanding of PASC and inform targeted interventions that address both individual and population-level health disparities.
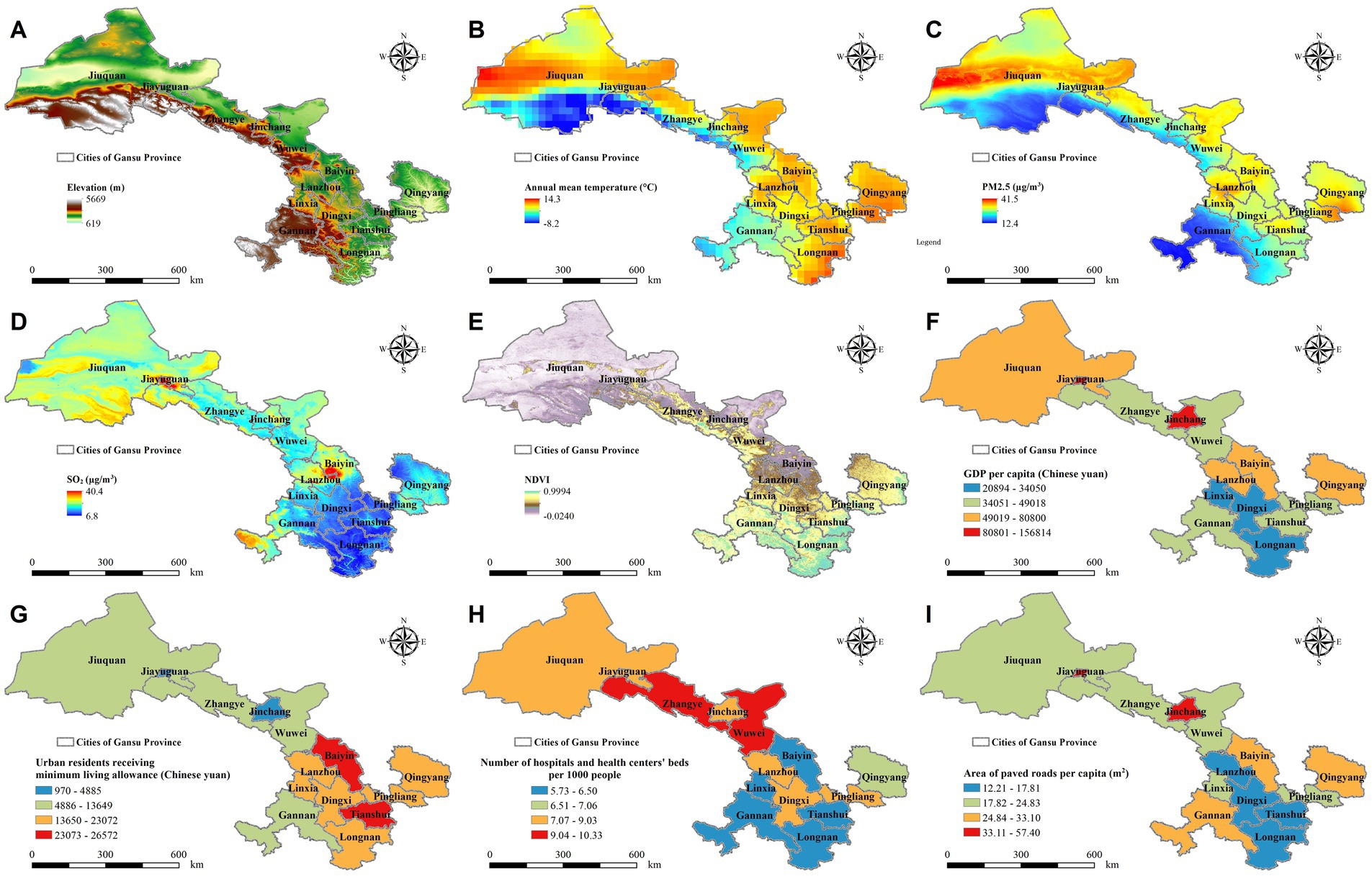
Figure 1. Geographic distribution of environmental and socioeconomic factors across 14 cities in Gansu Province, 2022. This figure illustrates key environmental and socioeconomic indicators across the study region, including: (A) Elevation, (B) Annual Mean Temperature, (C) PM2.5 Concentration, (D) Sulfur Dioxide (SO₂) Levels, (E) Normalized Difference Vegetation Index (NDVI), (F) Gross Domestic Product (GDP) per Capita, (G) Median Income of Urban Residents Receiving Minimum Living Allowance, (H) Number of Hospital and Health Center Beds per 1,000 People, and (I) Paved Road Area per Capita.
The diverse environmental and socioeconomic conditions across the study region provide a unique framework for investigating how these factors interact with age-related biological differences to shape the progression and recovery of PASC. This study employs a longitudinal design to systematically track COVID-19 patients over time, allowing for an in-depth analysis of how environmental exposures, economic disparities, and individual characteristics influence PASC risk and long-term outcomes. By integrating spatiotemporal data with patient health records, we aim to capture the dynamic nature of disease progression across different demographic groups. Furthermore, examining the interplay between environmental pollutants, healthcare accessibility, and socioeconomic determinants will offer a more comprehensive understanding of the broader public health implications of PASC. Through this multidisciplinary approach, our study seeks to inform the development of targeted interventions and personalized treatment strategies, ultimately contributing to improved patient care and more effective public health policies.
Methods and analysis
Study population
This prospective cohort study, will enroll over 4000 individuals with RT-PCR-confirmedSARS-CoV-2 at baseline, hospitalized at Gansu Provincial Hospital in China from December 2022 to May 2023 (Gansu PASC dataset). Aligning with methodologies from other PASC research, we will gather comprehensive health data during the patients’ initial hospitalization due to COVID-19 (31). The data collection will include extensive hospital records from their acute COVID-19 admissions, capturing demographic information, clinical data, comorbidities, pre-existing symptoms, and other relevant health details.
The patients were from all 14 cities in Gansu Province (92°13′E-108°46′E, 32°31’N-42°57’N), with various environmental and socioeconomic backgrounds. The municipal geographic coordinates of the 14 cities are detailed as follows: Lanzhou (103°51′E, 36°3’N); Jiayuguan (98°23′E, 39°80’N); Jinchang (102°19′E, 38°52’N); Baiyin (104°14′E, 36°55’N); Tianshui (105°72′E, 34°58’N); Wuwei (102°64′E, 37°93’N); Zhangye (100°45′E, 38°92’N); Pingliang (106°66′E, 35°54’N); Jiuquan (98°49′E, 39°73’N); Qingyang (107°64′E, 35°71’N); Dingxi (104°59′E, 35°61’N); Longnan (104°96′E, 33°37’N); Linxia Hui Autonomous Prefecture (103°21′E, 35°60’N) and Gannan Zang Autonomous Prefecture (102°91′E, 34°98’N). Baseline data will be collected using hospital records, and follow-up assessments will be performed at 24-, 36-, and 48-months post-discharge via structured telephone interviews (Table 1). Exclusion criteria are limited to individuals without available contact details, those who deceased during hospitalization or follow-up, those who declined to participate, and those unreachable for follow-up assessments.
Outcomes
Primary outcome
The primary objective of this study is to assess the persistence and long-term health outcomes of PASC symptoms. The diagnosis of PASC will be formally established during the first follow-up visit to ensure accurate identification and differentiation from pre-existing conditions, while long-term health outcomes will be evaluated at the second and third follow-up visits (Table 1). Our diagnostic protocol follows the criteria outlined in the WHO definition (32), and utilizes structured telephone interviews for initial PASC assessments (33, 34). PASC symptoms typically emerge at least 3 months after the initial SARS-CoV-2 infection and persist for a minimum of 2 months. To ensure an accurate diagnosis, symptoms must not have been present in the 180 days before infection (35). The initial follow-up visit, conducted 12 months post-diagnosis, confirms the presence and persistence of PASC symptoms, which are systematically evaluated across multiple organ systems, including respiratory, digestive, cardiovascular, musculoskeletal, and neurological functions, as well as general symptoms such as fatigue and fever. Symptom severity is quantitatively assessed using a four-point Likert scale to provide a structured and standardized evaluation of PASC’s impact on patient well-being (36, 37). To further validate symptom emergence and progression, our follow-up protocol differentiates post-COVID-19 manifestations from pre-existing conditions. Aligned with established research protocols, our multidisciplinary (MDR) team conducts follow-ups to collect comprehensive data on new diagnoses and medical consultations, allowing for the identification of secondary conditions potentially associated with PASC (38).
Secondary outcomes
The secondary outcomes of this study focus on assessments of the acute infection phase, its progression, the effectiveness of medical management strategies in shaping the trajectory of PASC (38). These assessments are conducted during the follow-up visits, designed to reassess the persistence or resolution of symptoms and evaluate changes in health status since the acute phase of the infection.
The evaluation includes documenting persisting symptoms and identifying any new clinical conditions diagnosed since the initial COVID-19 infection, as well as reviewing medical management strategies such as the reasons for doctor visits and treatments received during the follow-up period. Analysis objectives includes the persistence and progression of symptoms, with a specific focus on respiratory difficulties, overall quality of life, and mental health issues, including anxiety and depression (39). For pediatric populations, the assessment is tailored to specifically monitor the recovery of symptoms relevant to children and adolescents (40), addressing the unique challenges faced by these age groups in the aftermath of COVID-19.
Variables and data collection
Baseline variables
Individual baseline variables will be collected from the Gansu PASC dataset. We gather comprehensive health data during patients’ initial hospitalization for COVID-19. This baseline information serves as a reference to distinguish between pre-existing conditions and symptoms that may be attributed to PASC (41). These variables include demographic factors such as age, gender, ethnicity, marital status, educational background, employment status, and body mass index (BMI). Comorbidities recorded include hypertension, diabetes, respiratory diseases, liver diseases, and other relevant conditions. The Charlson Comorbidity Index (CCI) was adapted using a modified formula to assess morbidity and mortality risks. Conditions were scored as follows: one point for congestive heart failure, chronic pulmonary disease, diabetes, and other conditions; two points for chronic kidney disease and malignant neoplasms; three points for moderate to severe liver disease; and six points for Acquired Immunodeficiency Syndrome (AIDS) (42). Based on the CCI, comorbidities were categorized as mild (0–1), moderate (2–6), or severe (>6). Baseline laboratory assessments included complete blood counts, electrolyte panels, renal and liver function tests, inflammatory markers, and coagulation profiles. Initial treatment protocols involved various medications and supportive care measures, such as oxygen therapy, mechanical ventilation, and immunoglobulin treatments, with emergency interventions including tracheal intubation and cardiopulmonary resuscitation as required.
Follow-up assessments
We will conduct telephone follow-ups to diagnose PASC, employing methodologies consistent with those used in major global studies (43, 44). This approach is designed to enhance patient engagement and follow-up compliance, ensuring the inclusion of a broad demographic across diverse geographic locations. Follow-up visits will take place at three time points between 2024 and 2027: Visit 1 (24 months post-discharge) for PASC diagnosis, Visit 2 (36 months post-discharge) primarily for long-term outcome assessment, and Visit 3 (48 months post-discharge) for the final long-term outcome evaluation. During these follow-ups, we will assess the persistence of symptoms and track overall health status, alongside collecting updated information on demographics, environmental exposures, residential status post-infection, lifestyle changes, vaccination history, and hospitalization duration. The monitoring process will also include tracking reinfection cases, post-discharge mortality, the emergence of new symptoms, and changes in health status compared to the initial COVID-19 onset. Consistent with methodologies applied in similar studies (45, 46), our research incorporates patient consultations and provides recommendations for further medical evaluation based on symptoms reported during telephone interviews. However, direct medical interventions are not conducted as part of this study.
Environmental data
Environmental data will be collected to assess short-term exposures, including monthly average meteorological conditions and air pollutant concentrations, matched to each patient based on their location and hospital admission period (47). Meteorological data will be obtained from the ECMWF Reanalysis v5 (ERA5) dataset, which provides monthly averaged measurements of temperature, precipitation, relative humidity, and wind speed at a spatial resolution of 0.25° × 0.25° (approximately 25 km at the equator) (48). Air pollution data will be sourced from the China High-Resolution and High-Quality Near-Surface Air Pollutants (CHAP) Dataset, which provides daily concentrations of PM2.5, PM10, ozone (O₃), nitrogen dioxide (NO₂), sulfur dioxide (SO₂), and carbon monoxide (CO) at a spatial resolution of 1 km (49–52). Green space coverage will be assessed using the NDVI, derived from NASA Moderate Resolution Imaging Spectroradiometer (MODIS) Vegetation Indices. NDVI data will be provided annually at a spatial resolution of 1 km (53).
Socioeconomic data
Socioeconomic data including city-level per capita, income levels, employment status, job types, education level, and living conditions will be collected during follow-up visits and sourced from the Gansu Development Yearbook, published by the Gansu Province Bureau of Statistic.
Assessment tools
To ensure a comprehensive and nuanced evaluation across different age groups, the study employs a range of validated assessment instruments. For more detailed descriptions of these tools, please refer to Supplementary Material 1.
For adults
(1) The 5-level EQ-5D version (EQ-5D-5L) (54) is used to evaluate health-related quality of life; (2) The Modified Medical Research Council (mMRC) Dyspnea Scale (55) is employed to assess respiratory symptoms; (3) The Generalized Anxiety Disorder-7 (GAD-7) (56) and the Patient Health Questionnaire-9 (PHQ-9) (57) are used for mental health screening; (4) The Fatigue Severity Scale (FSS) (58) is utilized to measure fatigue levels; and (5) The Insomnia Severity Index (ISI) (59, 60) is applied to assess sleep disturbances.
For children and adolescents
(1) The EuroQol Five-Dimension Youth Questionnaire (EQ-5D-Y) (61) assesses health-related quality of life; (2) The Strengths and Difficulties Questionnaire (SDQ) (62) evaluates behavioral and emotional health; and (3) The Patient-Reported Outcomes Measurement Information System (PROMIS) (63) is used to assess physical, emotional, and social health indicators.
Statistical analysis
A variety of statistical methodologies will be employed to analyze the primary and secondary outcomes in this study. The data analysis begins with descriptive and inferential statistical methods to identify patterns and establish correlations. Continuous data will be presented as means with standard deviations (SD), while categorical variables will be reported as percentages. For comparisons across groups, analysis of variance (ANOVA) will be used for continuous variables, and chi-square tests for categorical variables, both with Bonferroni corrections applied in post-hoc analyses. A significance level of p < 0.05 will be consistently applied, with adjustments made for multiple comparisons to enhance the reliability of results. Logistic regression models will be employed to evaluate the impact of multiple factors on the severity of PASC. We will utilize a multivariable logistic regression model to analyze the relationship between various independent variables, including demographic factors, clinical characteristics, and environmental exposures, and the binary outcome of PASC presence or absence. The model will integrate variables selected based on clinical relevance and statistical significance derived from preliminary univariate analyses, ensuring that only factors significantly associated with the outcome are included in the final analysis. Variables for inclusion will be chosen based on their potential impact on PASC, informed by both clinical judgment and empirical evidence from initial analyses. We plan to investigate interaction terms, particularly between key demographic and clinical variables, to explore complex interactions that may affect PASC risk. Model fit will be assessed using the Hosmer-Lemeshow test for goodness-of-fit and Receiver Operating Characteristic curve analysis to evaluate predictive accuracy. We will also employ validation techniques such as split-sample validation or cross-validation to confirm the model’s robustness. Sensitivity analyses will be conducted to examine the stability of our results under various assumptions regarding data missingness and potential measurement errors, ensuring that our findings are reliable and applicable across different scenarios. Additionally, advanced analytical techniques will complement these methods, utilizing R (version 4.3.0) with the ‘gpboost’ and ‘stats’ packages and Python (version 3.10) with ‘sklearn’ and ‘gpboost.’
Generalized linear mixed-effects models (GLMM) for risk factor estimation
To estimate the influence of risk factors on PASC, Generalized Linear Mixed-Effects Models (GLMM) will be applied. These models will allow for a quantitative assessment of how various environmental and individual-level risk factors contribute to PASC, while controlling for potential confounders. A random intercept for each city will be included to account for non-measurable inter-city variability. Interaction terms between individual and environmental factors will be constructed, with only those demonstrating statistical significance and theoretical or empirical relevance retained for further analysis.
GPBoost models and SHapley additive exPlanations (SHAP) for factor quantification
The GPBoost model, which integrates tree-boosting techniques with Gaussian processes and mixed-effects models, will be used to quantify the impact of various factors on PASC. This model is well-suited for handling complex nonlinearities, discontinuities, and higher-order interactions. Gaussian processes provide the flexibility to model random effects and correlations commonly observed in clinical data. Recursive Feature Elimination (RFE) will be employed to streamline the model by iteratively removing the least significant features, as identified through the GLMM, ensuring focus on the most influential variables. Hyperparameter tuning will be performed using five-fold cross-validation to optimize model settings and improve robustness. The SHAP algorithm will be used to quantify each feature’s contribution to the outcome, providing an interpretable measure of feature importance through mean absolute SHAP values.
Causal inference models for risk factors and recovery trajectories
Causal inference models will be used to investigate the complex relationships between risk factors and the development of PASC, as well as the recovery trajectories of affected individuals. These models will aim to identify and quantify causal pathways, offering insights into how various factors influence both the onset and progression of PASC. Variables will be selected based on prior assessments, and longitudinal data will be collected to track changes over time. Directed Acyclic Graphs (DAGs) will be employed to visualize potential causal pathways and identify confounding factors that require control during analysis. Structural equation modeling and propensity score matching will be used to estimate the strength and direction of causal relationships. Sensitivity analyses will ensure the robustness and reliability of the findings.
Patient and public involvement
Patients and public will not involve in the design, recruitment and conduct of the study.
Ethics and dissemination
This study adheres to the ethical standards outlined in the Declaration of Helsinki and complies with national and local regulations concerning ethical research practices and patient data protection. The Gansu Provincial Hospital research ethics committee has approved this study protocol (ID: 2024–542), and it is registered as a clinical trial (ChiCTR2400091805). Ethical monitoring and data dissemination will be maintained throughout the study.
Informed consent will be obtained from all participants. For pediatric and geriatric populations, specific considerations include obtaining parental consent for minors and ensuring appropriate consent procedures for seniors, particularly those with cognitive impairments. The study commits to maintaining the confidentiality of all medical information, disclosing it only when required by law and with appropriate consent from involved parties. All clinical research data will be retained for a minimum of 5 years following the completion of the study. Study results will be published in peer-reviewed journals.
Discussion
This study will employ a longitudinal design to investigate how environmental, socioeconomic, and clinical factors shape PASC outcomes in a demographically diverse population. Comprehensive health data collected during the initial COVID-19 hospitalization help distinguish pre-existing conditions from symptoms emerging as part of PASC. By integrating these clinical records with environmental and socioeconomic variables through mixed-effects models and spatiotemporal analyses, the research provides a comprehensive understanding of how both internal factors (e.g., individual health status, comorbidities) and external factors (e.g., air quality, socioeconomic conditions) influence long-term health outcomes following COVID-19. To ensure a representative sample, the cohort is stratified by age, sex, and initial symptom severity, allowing for a detailed and subgroup-specific analysis of PASC manifestations. This multidimensional approach is essential for uncovering the complex interactions between patient-level susceptibility and broader external influences, ultimately informing targeted strategies to improve the management and mitigation of long-term COVID-19 effects.
Existing literature has widely documented the potential influences of underlying comorbidities, demographic factors, and environmental conditions on PASC outcomes (64). Pre-existing conditions such as cardiovascular disease, diabetes, and chronic respiratory disorders have been shown to exacerbate the severity of PASC symptoms, leading to prolonged recovery and an increased risk of complications (65). Individuals with chronic illnesses are more likely to experience severe acute COVID-19, which may predispose them to long-term sequelae due to sustained systemic inflammation and immune dysregulation (66). For instance, patients with diabetes and obesity have demonstrated heightened susceptibility to persistent inflammatory states post-COVID-19, contributing to ongoing fatigue, dyspnea, and cardiovascular dysfunction (67, 68). By examining how pre-existing health conditions interact with PASC, this study aims to elucidate the mechanisms through which chronic illnesses impact recovery trajectories and to identify potential intervention strategies.
Beyond comorbidities, demographic factors such as gender, education level, and marital status may also influence PASC trajectories (69). Gender differences in immune response and health-seeking behaviors have been linked to variations in symptom persistence and severity, with women more frequently reporting prolonged PASC symptoms, including fatigue, neurological issues, and autonomic dysfunction (70). Additionally, education level and marital status serve as proxies for social support and healthcare access, both of which are critical for managing chronic conditions and facilitating recovery (71). Limited health literacy, often associated with lower education levels, may impact adherence to post-COVID care recommendations, while marital status may influence mental health resilience and access to medical resources (72–74). By incorporating these demographic variables into our analysis, we aim to identify groups with greater susceptibility or resilience to PASC and assess how these interactions affect recovery trajectories.
Environmental pressures, such as air quality, urbanization, and access to green spaces, are increasingly recognized as key determinants of post-COVID-19 health outcomes (15, 20, 75). Air pollution has been linked to increased COVID-19 severity and mortality, and higher exposure to PM2.5 and NO2 has been associated with a higher prevalence of long-term respiratory and cardiovascular symptoms in PASC patients (75). Conversely, access to green spaces has been shown to provide protective benefits, improving mental and respiratory health and facilitating recovery (76). Given the environmental diversity of our study sites, this research will assess how these factors contribute to symptom persistence and recovery, leveraging existing knowledge on environmental determinants of health outcomes to refine analytical approaches and enhance the understanding of PASC progression.
A key strength of this study is its extended follow-up period of up to 48 months post-discharge, allowing for a comprehensive assessment of long-term PASC progression and persistence across different age groups. This prolonged observation provides valuable insights into age-specific recovery patterns, refining predictive models for long-term health outcomes and facilitating the development of targeted interventions. Additionally, the use of Geographic Information Systems (GIS) enhances the study by generating disease distribution maps, which help identify environmental and geographic factors associated with PASC. These insights are not only essential for detecting high-risk areas but also for guiding individualized patient care strategies.
Beyond mapping geographic influences, the integration of environmental and socioeconomic data with clinical outcomes strengthens the understanding of how external factors, such as air pollution, economic disparities, and urbanization, affect the severity and trajectory of PASC. Identifying these factors has direct implications for patient care, enabling healthcare providers to incorporate external influences into personalized treatment plans. Time-series analyses further enhance this approach by tracking temporal trends and evaluating the effects of environmental changes on health outcomes, providing a dynamic perspective on how fluctuating conditions contribute to disease progression. Meanwhile, causal inference models help establish robust causal relationships between environmental, socioeconomic, and clinical variables, ensuring a more rigorous evaluation of COVID-19’s long-term effects on PASC. By combining these analytical techniques, the study offers a multidimensional framework for understanding how environmental and socioeconomic conditions interact with individual health factors, ultimately informing more precise treatment and intervention strategies. This research also carries significant implications for patient care, particularly in identifying specific risk factors that exacerbate PASC in vulnerable populations. By considering both internal and external determinants of health, this study supports a shift toward precision medicine, ensuring that interventions address not only biological vulnerabilities but also environmental and socioeconomic disparities that influence recovery.
Despite the strengths of our study, we acknowledge several limitations and have devised strategies to address them effectively. Firstly, our study is based in Gansu Province, China, involving data from 14 cities, each with unique environmental and socioeconomic characteristics. While this provides a rich dataset within a specific geographic region, it limits broader geographic representation. To address this, we have included multiple sites chosen for their environmental diversity, particularly focusing on urban settings across different regions. This strategic selection helps control environmental factors that could influence health outcomes related to PASC. Secondly, our current study setup does not include direct comparisons with other cities or countries, which restricts our ability to generalize findings across different global environments. We plan to expand our analysis to include such comparisons as comparable data becomes available, allowing for a more comprehensive evaluation of environmental and socioeconomic impacts on PASC rates and health outcomes. Thirdly, the non-random nature of our patient recruitment might introduce selection bias, and the reliance on telephone questionnaires could lead to recall bias, particularly as participants may not accurately remember past symptoms. To mitigate these issues, we use advanced statistical methods to control for potential confounders and perform sensitivity analyses to assess the impact of data attrition. Fourthly, an oversight committee is actively involved in monitoring and evaluating the study’s processes to address any unforeseen issues that arise, thereby ensuring the integrity and validity of our research. Plans for further validation through clinical assessments are in place to enhance the reliability of our findings, particularly to address challenges associated with self-reported data. By implementing these strategies, we aim to refine our understanding of PASC and its diverse impacts across different populations, ensuring that our findings are robust and reflective of the true effects of environmental and socioeconomic factors on health outcomes post-COVID-19.
In conclusion, this research represents a critical advancement in understanding PASC by mapping recovery trajectories and identifying both internal and external factors influencing patient outcomes. The insights gained from this study have the potential to transform precision medicine approaches, allowing for more targeted and effective interventions that address the long-term consequences of COVID-19. Furthermore, these findings will contribute to public health strategies aimed at mitigating the impact of PASC, while also supporting the development of tailored patient care approaches based on individual risk factors.
Platform and data storage
Data from telephone interviews will be meticulously recorded and stored in a password-protected Microsoft Excel file. All study data will be securely housed on a dedicated server at Specific-Disease Database of Gansu Provincial Hospital, with access strictly limited to authorized members of the research team. Rigorous data management protocols will be enforced to ensure the utmost privacy and security of patient information, adhering to the highest standard of data protection.
Ethics statement
The studies involving humans were approved by Gansu Provincial Hospital Research Ethics Committee Board. The studies were conducted in accordance with the local legislation and institutional requirements. Written informed consent for participation in this study was provided by the participants’ legal guardians/next of kin.
Author contributions
DZ: Conceptualization, Data curation, Formal analysis, Funding acquisition, Investigation, Methodology, Resources, Supervision, Writing – original draft, Writing – review & editing. MT: Data curation, Formal analysis, Investigation, Methodology, Project administration, Software, Validation, Writing – original draft, Visualization, Writing – review & editing. XD: Formal analysis, Investigation, Methodology, Software, Writing – original draft. CZ: Conceptualization, Data curation, Formal analysis, Investigation, Resources, Visualization, Writing – review & editing. YY: Conceptualization, Formal analysis, Funding acquisition, Methodology, Project administration, Resources, Supervision, Writing – review & editing. XW: Data curation, Formal analysis, Investigation, Methodology, Project administration, Software, Supervision, Visualization, Writing – review & editing. JG: Conceptualization, Data curation, Formal analysis, Investigation, Methodology, Software, Supervision, Validation, Visualization, Writing – original draft, Writing – review & editing. LG: Conceptualization, Data curation, Formal analysis, Funding acquisition, Investigation, Project administration, Resources, Writing – review & editing.
Funding
The author(s) declare financial support was received for the research, authorship, and/or publication of this article. This research was supported by the Major Science and Technology Special Programme of Gansu Province, China (Funding Reference Number 22ZD1FA001); the Science and Technology Programme Project of Gansu Province, China (Funding Reference Number 21JR1RA029); Intramural Research Project of Gansu Provincial Hospital (Funding Reference Number 21GSSYB-42). Jing Gao was supported by Karolinska Institutet Research Foundation Grants, Sweden (Grant no. 2022-02329), the Research Foundation of the Pulmonary Diseases (Grant at 2024), Finland, and the Scientists Fund of the Gansu Provincial Hospital of China (Grant no. 2024KYQDJ-A-2; no. 24GSSYA-9). Xiaojun Wang was supported by the Research Project from Health Commission of Gansu Province of China (Grant no. GSWSQN2024-07), and the Scientists Fund of the Gansu Provincial Hospital of China (Grant no. 24GSSYE-3). The funder has no role in the data collection, data analysis, preparation of manuscript and decision to submission.
Conflict of interest
The authors declare that the research was conducted in the absence of any commercial or financial relationships that could be construed as a potential conflict of interest.
Generative AI statement
The authors declare that no Gen AI was used in the creation of this manuscript.
Publisher’s note
All claims expressed in this article are solely those of the authors and do not necessarily represent those of their affiliated organizations, or those of the publisher, the editors and the reviewers. Any product that may be evaluated in this article, or claim that may be made by its manufacturer, is not guaranteed or endorsed by the publisher.
Supplementary material
The Supplementary material for this article can be found online at: https://www.frontiersin.org/articles/10.3389/fpubh.2025.1533315/full#supplementary-material
References
1. Peng, P, Wang, Y, Li, Z, Zhou, Y, Wang, J, Qu, M, et al. A network analysis of the long-term quality of life and mental distress of COVID-19 survivors 1 year after hospital discharge. Front Public Health. (2023) 11:1223429. doi: 10.3389/fpubh.2023.1223429
2. Huang, C, Huang, L, Wang, Y, Li, X, Ren, L, Gu, X, et al. 6-month consequences of COVID-19 in patients discharged from hospital: a cohort study. Lancet. (2023) 401:e21–33. doi: 10.1016/s0140-6736(23)00810-3
3. Xie, Y, Xu, E, Bowe, B, and Al-Aly, Z. Long-term cardiovascular outcomes of COVID-19. Nat Med. (2022) 28:583–90. doi: 10.1038/s41591-022-01689-3
4. Seeßle, J, Waterboer, T, Hippchen, T, Simon, J, Kirchner, M, Lim, A, et al. Persistent symptoms in adult patients 1 year after coronavirus disease 2019 (COVID-19): a prospective cohort study. Clin Infect Dis. (2022) 74:1191–8. doi: 10.1093/cid/ciab611
5. Wu, X, Liu, X, Zhou, Y, Yu, H, Li, R, Zhan, Q, et al. 3-month, 6-month, 9-month, and 12-month respiratory outcomes in patients following COVID-19-related hospitalisation: a prospective study. Lancet Respir Med. (2021) 9:747–54. doi: 10.1016/s2213-2600(21)00174-0
6. Taquet, M, Dercon, Q, Luciano, S, Geddes, JR, Husain, M, and Harrison, PJ. Incidence, co-occurrence, and evolution of long-COVID features: a 6-month retrospective cohort study of 273,618 survivors of COVID-19. PLoS Med. (2021) 18:e1003773. doi: 10.1371/journal.pmed.1003773
7. Del Rio, C, Collins, LF, and Malani, P. Long-term health consequences of COVID-19. JAMA. (2020) 324:1723–4. doi: 10.1001/jama.2020.19719
8. Nalbandian, A, Sehgal, K, Gupta, A, Madhavan, MV, McGroder, C, Stevens, JS, et al. Post-acute COVID-19 syndrome. Nat Med. (2021) 27:601–15. doi: 10.1038/s41591-021-01283-z
9. Piontkovskaya, K, Luo, Y, Lindberg, P, Gao, J, Runold, M, Kolosenko, I, et al. CORACLE (COVID-19 liteRAture CompiLEr): a platform for efficient tracking and extraction of SARS-CoV-2 and COVID-19 literature, with examples from post-COVID with respiratory involvement. Comput Struct Biotechnol J. (2024) 23:2661–8. doi: 10.1016/j.csbj.2024.06.018
10. Al-Aly, Z, Xie, Y, and Bowe, B. High-dimensional characterization of post-acute sequelae of COVID-19. Nature. (2021) 594:259–64. doi: 10.1038/s41586-021-03553-9
11. Lopez-Leon, S, Wegman-Ostrosky, T, Perelman, C, Sepulveda, R, Rebolledo, P, Cuapio, A, et al. More than 50 long-term effects of COVID-19: a systematic review and Meta-analysis. Res Sq. (2021). doi: 10.21203/rs.3.rs-266574/v1
12. Huang, C, Huang, L, Wang, Y, Li, X, Ren, L, Gu, X, et al. 6-month consequences of COVID-19 in patients discharged from hospital: a cohort study. Lancet. (2021) 397:220–32. doi: 10.1016/s0140-6736(20)32656-8
13. Dabisch, P, Schuit, M, Herzog, A, Beck, K, Wood, S, Krause, M, et al. The influence of temperature, humidity, and simulated sunlight on the infectivity of SARS-CoV-2 in aerosols. Aerosol Sci Technol. (2021) 55:142–53. doi: 10.1080/02786826.2020.1829536
14. Covert, HH, Abdoel Wahid, F, Wenzel, SE, and Lichtveld, MY. Climate change impacts on respiratory health: exposure, vulnerability, and risk. Physiol Rev. (2023) 103:2507–22. doi: 10.1152/physrev.00043.2022
15. Zhang, Y, Hu, H, Fokaidis, V, CL, V, Xu, J, Zang, C, et al. Identifying environmental risk factors for post-acute sequelae of SARS-CoV-2 infection: an EHR-based cohort study from the recover program. Environ Adv. (2023) 11:100352. doi: 10.1016/j.envadv.2023.100352
16. Moriyama, M, Hugentobler, WJ, and Iwasaki, A. Seasonality of respiratory viral infections. Annu Rev Virol. (2020) 7:83–101. doi: 10.1146/annurev-virology-012420-022445
17. Mena, GE, Martinez, PP, Mahmud, AS, Marquet, PA, Buckee, CO, and Santillana, M. Socioeconomic status determines COVID-19 incidence and related mortality in Santiago, Chile. Science. (2021) 372:eabg5298. doi: 10.1126/science.abg5298
18. Mateu, L, Tebe, C, Loste, C, Santos, JR, Lladós, G, López, C, et al. Determinants of the onset and prognosis of the post-COVID-19 condition: a 2-year prospective observational cohort study. Lancet Reg Health Eur. (2023) 33:100724. doi: 10.1016/j.lanepe.2023.100724
19. Shabnam, S, Razieh, C, Dambha-Miller, H, Yates, T, Gillies, C, Chudasama, YV, et al. Socioeconomic inequalities of long COVID: a retrospective population-based cohort study in the United Kingdom. J R Soc Med. (2023) 116:263–73. doi: 10.1177/01410768231168377
20. Ferreira, JC, Moreira, TCL, de Araújo, AL, Imamura, M, Damiano, RF, Garcia, ML, et al. Clinical, sociodemographic and environmental factors impact post-COVID-19 syndrome. J Glob Health. (2022) 12:05029. doi: 10.7189/jogh.12.05029
21. De Angelis, E, Renzetti, S, Volta, M, Donato, F, Calza, S, Placidi, D, et al. COVID-19 incidence and mortality in Lombardy, Italy: an ecological study on the role of air pollution, meteorological factors, demographic and socioeconomic variables. Environ Res. (2021) 195:110777. doi: 10.1016/j.envres.2021.110777
22. Ssentongo, P, Ssentongo, AE, Heilbrunn, ES, Ba, DM, and Chinchilli, VM. Association of cardiovascular disease and 10 other pre-existing comorbidities with COVID-19 mortality: a systematic review and meta-analysis. PLoS One. (2020) 15:e0238215. doi: 10.1371/journal.pone.0238215
23. Patel, JA, Nielsen, FBH, Badiani, AA, Assi, S, Unadkat, VA, Patel, B, et al. Poverty, inequality and COVID-19: the forgotten vulnerable. Public Health. (2020) 183:110–1. doi: 10.1016/j.puhe.2020.05.006
24. Gansu Inspection 2012: Department of Geography and Resource Management (GRM), The Chinese University of Hong Kong. Available online at: https://www.grm.cuhk.edu.hk/en/field-trip/gansu-2012/.
25. Global Multi-resolution Terrain Elevation Data (GMTED2010): The U.S. Geological Survey. Available online at: https://www.usgs.gov/coastal-changes-and-impacts/gmted2010.
26. ERA5 monthly averaged data on single levels from 1940 to present: Copernicus Climate Change Service. Available online at: https://cds.climate.copernicus.eu/datasets/reanalysis-era5-single-levels-monthly-means?tab=overview.
27. China 1km high-resolution, high-quality, year-by-year PM2.5 dataset (2000-2023): National Earth System Science Data Center. Available online at: https://www.geodata.cn/main/face_science_detail?id=57642&guid=241344160541332.
28. China 1km high-resolution high-quality year-by-year near-surface SO2 dataset (2019-2023): National Earth System Science Data Center. Available online at: https://www.geodata.cn/main/face_science_detail?id=63024&guid=210612525134625.
29. China annual NDVI, EVI 1KM dataset: Resource and Environmental Science Data Registry and Publishing System. Available online at: https://www.resdc.cn/DOI/DOI.aspx?DOIID=49.
30. Gansu Development Yearbook: Gansu Province Bureau Of Statistic. Available online at: https://tjj.gansu.gov.cn/tjj/c117468/info_disp.shtml.
31. Zahouani, I, Desmeules, F, Perreault, K, Campeau-Lecours, A, Best, K, Beaulieu-Bonneau, S, et al. Physical and cognitive impairments in people suffering from long COVID: protocol for a longitudinal population-based cohort study. BMJ Open. (2023) 13:e064054. doi: 10.1136/bmjopen-2022-064054
32. Soriano, JB, Murthy, S, Marshall, JC, Relan, P, and Diaz, JV. A clinical case definition of post-COVID-19 condition by a Delphi consensus. Lancet Infect Dis. (2022) 22:e102–7. doi: 10.1016/s1473-3099(21)00703-9
33. Haddad, A, Janda, A, Renk, H, Stich, M, Frieh, P, Kaier, K, et al. Long COVID symptoms in exposed and infected children, adolescents and their parents one year after SARS-CoV-2 infection: a prospective observational cohort study. EBioMedicine. (2022) 84:104245. doi: 10.1016/j.ebiom.2022.104245
34. Fernández-de-Las-Peñas, C, Palacios-Ceña, D, Gómez-Mayordomo, V, Rodríuez-Jiménez, J, Palacios-Ceña, M, Velasco-Arribas, M, et al. Long-term post-COVID symptoms and associated risk factors in previously hospitalized patients: a multicenter study. J Infect. (2021) 83:237–79. doi: 10.1016/j.jinf.2021.04.036
35. Català, M, Mercadé-Besora, N, Kolde, R, Trinh, NTH, Roel, E, Burn, E, et al. The effectiveness of COVID-19 vaccines to prevent long COVID symptoms: staggered cohort study of data from the UK, Spain, and Estonia. Lancet Respir Med. (2024) 12:225–36. doi: 10.1016/s2213-2600(23)00414-9
36. Tyumeneva, Y, Sudorgina, Y, Kislyonkova, A, and Lebedeva, M. Ordering motivation and Likert scale ratings: when a numeric scale is not necessarily better. Front Psychol. (2022) 13:942593. doi: 10.3389/fpsyg.2022.942593
37. Burton, C, Dawes, H, Goodwill, S, Thelwell, M, and Dalton, C. Within and between-day variation and associations of symptoms in long Covid: intensive longitudinal study. PLoS One. (2023) 18:e0280343. doi: 10.1371/journal.pone.0280343
38. Horwitz, LI, Thaweethai, T, Brosnahan, SB, Cicek, MS, Fitzgerald, ML, Goldman, JD, et al. Researching COVID to enhance recovery (RECOVER) adult study protocol: rationale, objectives, and design. PLoS One. (2023) 18:e0286297. doi: 10.1371/journal.pone.0286297
39. Rudenstine, S, Schulder, T, Bhatt, KJ, McNeal, K, Ettman, CK, and Galea, S. Long-COVID and comorbid depression and anxiety two years into the COVID-19 pandemic. Psychiatry Res. (2022) 317:114924. doi: 10.1016/j.psychres.2022.114924
40. Zimmermann, P, Pittet, LF, and Curtis, N. Long covid in children and adolescents. BMJ. (2022) 376:o143. doi: 10.1136/bmj.o143
41. Gentilotti, E, Górska, A, Tami, A, Gusinow, R, Mirandola, M, Rodríguez Baño, J, et al. Clinical phenotypes and quality of life to define post-COVID-19 syndrome: a cluster analysis of the multinational, prospective ORCHESTRA cohort. EClinicalMedicine. (2023) 62:102107. doi: 10.1016/j.eclinm.2023.102107
42. Charlson Comorbidity Index (CCI). MDCalc. Available online at: https://www.mdcalc.com/calc/3917/charlson-comorbidity-index-cci.
43. Borch, L, Holm, M, Knudsen, M, Ellermann-Eriksen, S, and Hagstroem, S. Long COVID symptoms and duration in SARS-CoV-2 positive children - a nationwide cohort study. Eur J Pediatr. (2022) 181:1597–607. doi: 10.1007/s00431-021-04345-z
44. Dinis Teixeira, JP, Santos, M, Soares, P, Azevedo, L, Barbosa, P, Boas, AV, et al. LOCUS (LOng Covid-understanding symptoms, events and use of services in Portugal): a three-component study protocol. PLoS One. (2023) 18:e0285051:e0285051. doi: 10.1371/journal.pone.0285051
45. Busatto, GF, de Araújo, AL, Duarte, A, Levin, AS, Guedes, BF, Kallas, EG, et al. Post-acute sequelae of SARS-CoV-2 infection (PASC): a protocol for a multidisciplinary prospective observational evaluation of a cohort of patients surviving hospitalisation in Sao Paulo, Brazil. BMJ Open. (2021) 11:e051706. doi: 10.1136/bmjopen-2021-051706
46. Pires, L, Reis, C, Mesquita Facão, AR, Moniri, A, Marreiros, A, Drummond, M, et al. Fatigue and mental illness symptoms in long COVID: protocol for a prospective cohort multicenter observational study. JMIR Res Protoc. (2024) 13:e51820. doi: 10.2196/51820
47. Orellano, P, Reynoso, J, Quaranta, N, Bardach, A, and Ciapponi, A. Short-term exposure to particulate matter (PM(10) and PM(2.5)), nitrogen dioxide (NO(2)), and ozone (O(3)) and all-cause and cause-specific mortality: systematic review and meta-analysis. Environ Int. (2020) 142:105876. doi: 10.1016/j.envint.2020.105876
48. Copernicus Climate Data Store. Copernicus climate change service. Available online at: https://cds.climate.copernicus.eu/cdsapp#!/dataset/reanalysis-era5-single-levels-monthly-means?tab=form.
49. Wei, J, Li, Z, Lyapustin, A, Sun, L, Peng, Y, Xue, W, et al. Reconstructing 1-km-resolution high-quality PM2.5 data records from 2000 to 2018 in China: spatiotemporal variations and policy implications. Remote Sens Environ. (2020) 252:112136. doi: 10.1016/j.rse.2020.112136
50. Wei, J, Li, Z, Xue, W, Sun, L, Fan, T, Liu, L, et al. The ChinaHighPM10 dataset: generation, validation, and spatiotemporal variations from 2015 to 2019 across China. Environ Int. 146:106290. doi: 10.1016/j.envint.2020.106290
51. Wei, J, Li, Z, Li, K, Dickerson, RR, Pinker, RT, Wang, J, et al. Full-coverage mapping and spatiotemporal variations of ground-level ozone (O3) pollution from 2013 to 2020 across China. Remote Sens Environ. (2022) 270:112775. doi: 10.1016/j.rse.2021.112775
52. Wei, J, Li, Z, Wang, J, Li, C, Gupta, P, and Cribb, M. Ground-level gaseous pollutants (NO2, SO2, and CO) in China: daily seamless mapping and spatiotemporal variations. Atmos Chem Phys. (2023) 23:1511–32. doi: 10.5194/acp-23-1511-2023
53. Vegetation Indices: NASA. Available online at: https://ladsweb.modaps.eosdis.nasa.gov/search/.
54. Herdman, M, Gudex, C, Lloyd, A, Janssen, M, Kind, P, Parkin, D, et al. Development and preliminary testing of the new five-level version of EQ-5D (EQ-5D-5L). Qual Life Res. (2011) 20:1727–36. doi: 10.1007/s11136-011-9903-x
55. Mahler, DA, and Wells, CK. Evaluation of clinical methods for rating dyspnea. Chest. (1988) 93:580–6. doi: 10.1378/chest.93.3.580
56. Johnson, SU, Ulvenes, PG, Øktedalen, T, and Hoffart, A. Psychometric properties of the general anxiety disorder 7-item (GAD-7) scale in a heterogeneous psychiatric sample. Front Psychol. (2019) 10:1713. doi: 10.3389/fpsyg.2019.01713
57. Kroenke, K, Spitzer, RL, and Williams, JB. The PHQ-9: validity of a brief depression severity measure. J Gen Intern Med. (2001) 16:606–13. doi: 10.1046/j.1525-1497.2001.016009606.x
58. Krupp, LB, LaRocca, NG, Muir-Nash, J, and Steinberg, AD. The fatigue severity scale. Application to patients with multiple sclerosis and systemic lupus erythematosus. Arch Neurol. (1989) 46:1121–3. doi: 10.1001/archneur.1989.00520460115022
59. Morin, CM, Belleville, G, Bélanger, L, and Ivers, H. The insomnia severity index: psychometric indicators to detect insomnia cases and evaluate treatment response. Sleep. (2011) 34:601–8. doi: 10.1093/sleep/34.5.601
60. Bastien, CH, Vallières, A, and Morin, CM. Validation of the insomnia severity index as an outcome measure for insomnia research. Sleep Med. (2001) 2:297–307. doi: 10.1016/s1389-9457(00)00065-4
61. Wille, N, Badia, X, Bonsel, G, Burström, K, Cavrini, G, Devlin, N, et al. Development of the EQ-5D-Y: a child-friendly version of the EQ-5D. Qual Life Res. (2010) 19:875–86. doi: 10.1007/s11136-010-9648-y
62. Goodman, R. The strengths and difficulties questionnaire: a research note. J Child Psychol Psychiatry. (1997) 38:581–6. doi: 10.1111/j.1469-7610.1997.tb01545.x
63. Lai, JS, Stucky, BD, Thissen, D, Varni, JW, DeWitt, EM, Irwin, DE, et al. Development and psychometric properties of the PROMIS(®) pediatric fatigue item banks. Qual Life Res. (2013) 22:2417–27. doi: 10.1007/s11136-013-0357-1
64. Mincuzzi, A, Carone, S, Galluzzo, C, Tanzarella, M, Lagravinese, GM, Bruni, A, et al. Gender differences, environmental pressures, tumor characteristics, and death rate in a lung cancer cohort: a seven-years Bayesian survival analysis using cancer registry data from a contaminated area in Italy. Front Public Health. (2023) 11:1278416. doi: 10.3389/fpubh.2023.1278416
65. Subramanian, A, Nirantharakumar, K, Hughes, S, Myles, P, Williams, T, Gokhale, KM, et al. Symptoms and risk factors for long COVID in non-hospitalized adults. Nat Med. (2022) 28:1706–14. doi: 10.1038/s41591-022-01909-w
66. Wong, MC, Huang, J, Wong, YY, Wong, GL, Yip, TC, Chan, RN, et al. Epidemiology, symptomatology, and risk factors for long COVID symptoms: population-based, multicenter study. JMIR Public Health Surveill. (2023) 9:e42315. doi: 10.2196/42315
67. Harding, JL, Oviedo, SA, Ali, MK, Ofotokun, I, Gander, JC, Patel, SA, et al. The bidirectional association between diabetes and long-COVID-19 - a systematic review. Diabetes Res Clin Pract. (2023) 195:110202. doi: 10.1016/j.diabres.2022.110202
68. Oguz, SH, and Yildiz, BO. Endocrine disorders and COVID-19. Annu Rev Med. (2023) 74:75–88. doi: 10.1146/annurev-med-043021-033509
69. Giannico, OV, Ambrosino, I, Patano, F, Germinario, C, Quarto, M, and Moretti, AM. Educational level, marital status and sex as social gender discharge determinants in chronic obstructive pulmonary disease exacerbations: a time-to-event analysis. Monaldi Arch Chest Dis. (2019) 89. doi: 10.4081/monaldi.2019.1017
70. Bai, F, Tomasoni, D, Falcinella, C, Barbanotti, D, Castoldi, R, Mulè, G, et al. Female gender is associated with long COVID syndrome: a prospective cohort study. Clin Microbiol Infect. (2022) 28:611.e9-.e16. doi: 10.1016/j.cmi.2021.11.002
71. Müller, SA, Isaaka, L, Mumm, R, Scheidt-Nave, C, Heldt, K, Schuster, A, et al. Prevalence and risk factors for long COVID and post-COVID-19 condition in Africa: a systematic review. Lancet Glob Health. (2023) 11:e1713–24. doi: 10.1016/s2214-109x(23)00384-4
72. Aldhawyan, AF, BuSaad, MA, Almaghlouth, NE, Alnasser, AH, Alnasser, JA, Almansour, AH, et al. Understanding long COVID: prevalence, characteristics, and risk factors in the Eastern Province of Saudi Arabia. Front Med. (2024) 11:1459583. doi: 10.3389/fmed.2024.1459583
73. Kudoh, R, Komiya, K, Shinohara, A, Kageyama, T, Hiramatsu, K, and Kadota, JI. Marital status and post-COVID-19 conditions. Respir Investig. (2023) 61:181–5. doi: 10.1016/j.resinv.2023.01.001
74. Obeidat, M, Abu Zahra, A, and Alsattari, F. Prevalence and characteristics of long COVID-19 in Jordan: a cross sectional survey. PLoS One. (2024) 19:e0295969. doi: 10.1371/journal.pone.0295969
75. Yu, Z, Ekström, S, Bellander, T, Ljungman, P, Pershagen, G, Eneroth, K, et al. Ambient air pollution exposure linked to long COVID among young adults: a nested survey in a population-based cohort in Sweden. Lancet Reg Health Eur. (2023) 28:100608. doi: 10.1016/j.lanepe.2023.100608
Keywords: Post-Acute Sequelae of SARS-CoV-2 Infection (PASC), environmental factors, socioeconomic factors, COVID-19, age, long-term health outcomes
Citation: Zhang D, Tong M, Dong X, Zhang C, Yuan Y, Wang X, Gao J and Guo L (2025) Long-term outcomes of post-acute sequelae of SARS-CoV-2 infection: a cohort study protocol. Front. Public Health. 13:1533315. doi: 10.3389/fpubh.2025.1533315
Edited by:
Khalid Mehmood, Jiangsu University, ChinaReviewed by:
Orazio Valerio Giannico, Local Health Authority of Taranto, ItalyKarla Maria Tamez-Torres, Instituto Nacional de Ciencias Médicas y Nutrición Salvador Zubirán, Mexico
Copyright © 2025 Zhang, Tong, Dong, Zhang, Yuan, Wang, Gao and Guo. This is an open-access article distributed under the terms of the Creative Commons Attribution License (CC BY). The use, distribution or reproduction in other forums is permitted, provided the original author(s) and the copyright owner(s) are credited and that the original publication in this journal is cited, in accordance with accepted academic practice. No use, distribution or reproduction is permitted which does not comply with these terms.
*Correspondence: Longfei Guo, Z3VvbG9uZ2ZlaTA5MjBAMTI2LmNvbQ==; Jing Gao, amluZy5nYW9Aa2kuc2U=; Xiaojun Wang, d2FuZ3hpYW9qdW5fd29ya0BvdXRsb29rLmNvbQ==
†These authors have contributed equally to this work