- Key Laboratory of Obstetrics and Gynecologic and Pediatric Diseases and Birth Defects of Ministry of Education, West China Second University Hospital, Sichuan University, Chengdu, China
Background: Cardiometabolic diseases are prevalent among ageing populations and have a close association with frailty. However, the cumulative impact multiple cardiometabolic diseases have on frailty remains underexplored.
Methods: This study used data from four international cohorts – HRS, CHARLS, ELSA and SHARE – to examine the correlation between frailty and cardiometabolic diseases (CMD). The frailty index was used for assessing frailty and statistical analyses were performed as a means of analysing the correlation between the number of cardiometabolic conditions and frailty severity. Linear regression models were employed to evaluate the associations between CMD and frailty severity.
Results: The study found that as the number of cardiometabolic diseases increased, the frailty index rose significantly [one disease, β = 7.80 (95% CI: 7.70 to 7.90) p < 0.05; two diseases, β = 17.92 (95% CI: 17.76 to 18.08) p < 0.05; three diseases, β = 28.79 (95% CI: 28.41 to 29.17) p < 0.05]. Stroke was found to have the most pronounced impact on frailty (β = 12.34 [95%CI 12.20 to 12.48] p < 0.05) and the coexistence of multiple conditions served to amplify the symptoms of frailty.
Conclusion: This study highlights the compounded impact multiple cardiometabolic diseases have on frailty and also emphasizes the necessity for early intervention.
Introduction
Cardiometabolic diseases (CMD), including stroke, diabetes and heart disease, have become increasingly prevalent among the ageing population (1). As people age, their risk of developing these chronic conditions increases significantly as a result of a combination of genetic, lifestyle and environmental factors (2–4). Diabetes, which is characterized by impaired glucose regulation, is particularly common in older adults and is often compounded by obesity and physical inactivity (5). CMDs, which involve heart failure, hypertension and coronary artery diseases are prevalent in ageing populations and contribute to increased morbidity and mortality (6). Similarly, stroke incidence increases with age and is driven by factors including hypertension, atherosclerosis and atrial fibrillation (7). These diseases are highly prevalent and also frequently coexist, which compounds the health burden of older adults, increasing the risk of disability and frailty and lowering their quality of life (8, 9).
In older people, frailty is a prevalent and debilitating condition that is marked by an increased susceptibility to unfavourable health problems and reduced physiological reserves (10). The key factors that contribute to frailty include ageing, chronic diseases, physical inactivity, malnutrition and psychological stress (11). These factors serve to create a vicious cycle, with frailty negatively impacting multiple health domains, including functional impairment, reduced mobility and a loss of independence (12, 13). As frailty progresses, life quality is significantly diminished by impaired mental health, physical capacities and social interaction (14, 15). In addition, frailty has a close association with higher risks of hospitalization, disability and mortality (16, 17).
Cardiometabolic diseases such as stroke, diabetes and heart disease make a significant contribution to this decline in physical function by impairing critical physiological processes (18, 19). These conditions lead to vascular damage, insulin resistance, muscle wasting and neurodegeneration, which all undermine physical capacity and increase frailty risk (20–22). Frailty becomes more pronounced as the number of cardiometabolic diseases increases, with a progressive decline in physical strength and overall resilience (23).
Cardiometabolic diseases are highly prevalent among older populations and have been shown to be strongly associated with frailty (24–26). However, existing research has predominantly focused on the impact of single cardiometabolic conditions on frailty, with limited exploration of the cumulative effects of comorbid cardiometabolic diseases (27). Moreover, most studies to date are based on data from a single region, lacking cross-national validation. This study leverages four international longitudinal cohorts (HRS, CHARLS, ELSA, and SHARE) to systematically examine how multiple cardiometabolic diseases interact to influence frailty, while also investigating the moderating effects of gender and age in this relationship.
Although previous studies have examined the associations between CMD or cardiometabolic multimorbidity (CMM) and outcomes such as depression, cognitive decline, and disability (28–30), these studies primarily focus on individual health outcomes rather than the broader construct of frailty. The uniqueness of our study lies in its comprehensive approach to assessing how the cumulative impact of CMD exacerbates frailty. By constructing a Frailty Index, we evaluate an individual’s health holistically, considering physiological, psychological, and functional domains. This approach moves beyond the limitations of studying isolated diseases or impairments and provides a more integrated understanding of health outcomes. Our research fills a significant gap in the current literature and offers critical insights that can inform the development of public health policies and targeted intervention strategies.
Methods
Study design and population
This integrated multicohort analysis used data from four international longitudinal cohorts targeting older and middle-aged people: the China Health and Retirement Longitudinal Study (CHARLS), the Health and Retirement Study (HRS), the Survey of Health, Ageing and Retirement in Europe (SHARE) and the English Longitudinal Study of Ageing (ELSA). The study used data from approximately overlapping time frames: HRS covers waves 10 to 15 (2010–2020), CHARLS includes waves 1 to 4 (2011–2018), ELSA encompasses waves 7 to 9 (2014–2018) and SHARE spans waves 4 to 7 (2011–2017) (Supplementary Table 2). Participants needed to be 50 years of age or older to be included and exclusions were applied to individuals with missing data relating to cardiometabolic diseases or frailty index. The study received ethical approval from the relevant committees for each study and participants were recruited after providing written informed consent.
Exposure assessment
In the context of cardiometabolic diseases, the study focused on diabetes, heart disease and stroke as these conditions all have the potential to exacerbate frailty. The presence of these diseases was determined by face-to-face interviews between researchers and participants and supplemented by self-reported medical histories that were obtained from structured questionnaires. The cardiometabolic disease status of participants was classified on the basis of the total number of conditions they had (i.e., diabetes, heart disease or stroke). Participants were then categorized into three groups: those without any cardiometabolic disease, those with a single cardiometabolic condition and those who exhibited cardiometabolic multimorbidity (with two or more coexisting conditions).
Outcome assessment
The frailty index, which quantifies the cumulative burden of age-related health deficits, was used to assess frailty (31). In accordance with previous studies, items that could be consistently applied across all four cohorts were selected (32, 33). After data screening, 30 items from the CHARLS, HRS, ELSA and SHARE surveys were included to construct the frailty index. These items encompassed self-reported health status, a range of chronic disorders, depression, functional limitations and cognitive impairment (Supplementary Table 3). Most of the items were dichotomised based on established cut-off values, with a score of 0 denoting the lack of a deficit and a score of 1 denoting its existence. Self-reported measures of general vision, hearing, health status and cognition were scored on a scale from 0 to 1, with higher scores indicating more serious deficits. The frailty index was computed by summing the deficits present in each individual, dividing by 30 and then multiplying by 100 for this study. Therefore, the frailty index was theoretically a continuous variable with a range from 0 to 100. Participants who missed any of the 30 items in the four databases were excluded from the process of frailty index calculation.
Data collection
The following information was collected for this study: (i) demographic information: this consisted of marital status, sex, age and educational attainment. Three groups were created based on educational attainment: lower secondary education or below, upper secondary and higher than upper secondary. Marital status was divided into married and other marital statuses (such as separated, single, widowed and divorced). (ii) Lifestyle information: information was collected related to drinking and smoking patterns. Physical activity was defined as engaging in moderate or vigorous exercise at least once per week. (iii) Anthropometric measurements: body mass index (BMI). (iv) Medical history: information was gathered relating to the presence of hypertension, lung disease and cancer. For further details, please see Supplementary Table 4. In the data preprocessing of this study, we employed a complete case analysis method, including only samples with complete data for analysis. Variables or samples with a high proportion of missing values were subject to rigorous screening and exclusion to ensure data integrity.
Statistical analysis
For continuous variables with a normal distribution, the data was presented as mean ± standard deviation (SD) and group differences were assessed through the use of ANOVA. Categorical variables were expressed as numerical values (percentages) and intergroup differences were analysed using Pearson’s chi-square test.
In the assessment of cardiometabolic diseases, we not only considered the classification based on the number of cardiometabolic diseases but also further analyzed the impact of different cardiometabolic diseases combinations on the frailty index. Specifically, we categorized the subjects into the following groups based on whether they had diabetes, heart disease, or stroke: (1) diabetes only, (2) heart disease only, (3) stroke only, (4) both diabetes and heart disease, (5) both diabetes and stroke, (6) both heart disease and stroke, and (7) all three diseases. Linear regression models were used to assess the relationship between cardiometabolic diseases and frailty index. Multiple models were constructed, each adjusting for a different set of covariates to provide a more detailed understanding of their impact on the observed association. Model 1 included no adjustments, Model 2 adjusted for age and gender, and Model 3 adjusted for age, gender, marital status, education, obesity, hypertension, cancer, lung disease, physical activity, and current smoking and drinking status.
Subgroup analyses were conducted to assess whether the association between CMD and the frailty index varies in strength across different populations. Participants were divided into different subgroups based on their gender, age (less than 65 years vs. more than 65 years), marital status, drinking and smoking habits and physical activity. MSTATA software1 and R software (version 4.3.1) were used for all of the statistical analyses. A two-sided p-value of below 0.05 was considered to be statistically significant.
Results
The flowchart for the investigation population screening procedure in HRS, CHARLS, ELSA and SHARE can be seen in Supplementary Figures 1–4. There was a total of 403,609 participants and their mean age was 66.7 (SD 10.3) years. 224,867 (55.7%) of the participants in the final analytic cohort were female and 178,740 (44.3%) were male. Figure 1 shows the distribution of cardiometabolic diseases in HRS, CHARLS, ELSA and SHARE. 257,525 (63.8%) of the participants were free from any cardiometabolic diseases, while 146,084 (36.2%) exhibited at least one form of cardiometabolic disease. The frailty index for participants without cardiometabolic diseases was 14.2 (SD 11.2). In contrast, those with a single cardiometabolic disease had a higher frailty index of 25.1 (SD 14.4), while those with two or more cardiometabolic diseases exhibited an even further increase, with a frailty index of 37.5 (SD 17.6) (Table 1). The baseline table classified by diabetes, heart disease and stroke is shown in Supplementary Table 5. The distribution of the frailty index across the various databases can be seen in Supplementary Figure 5.
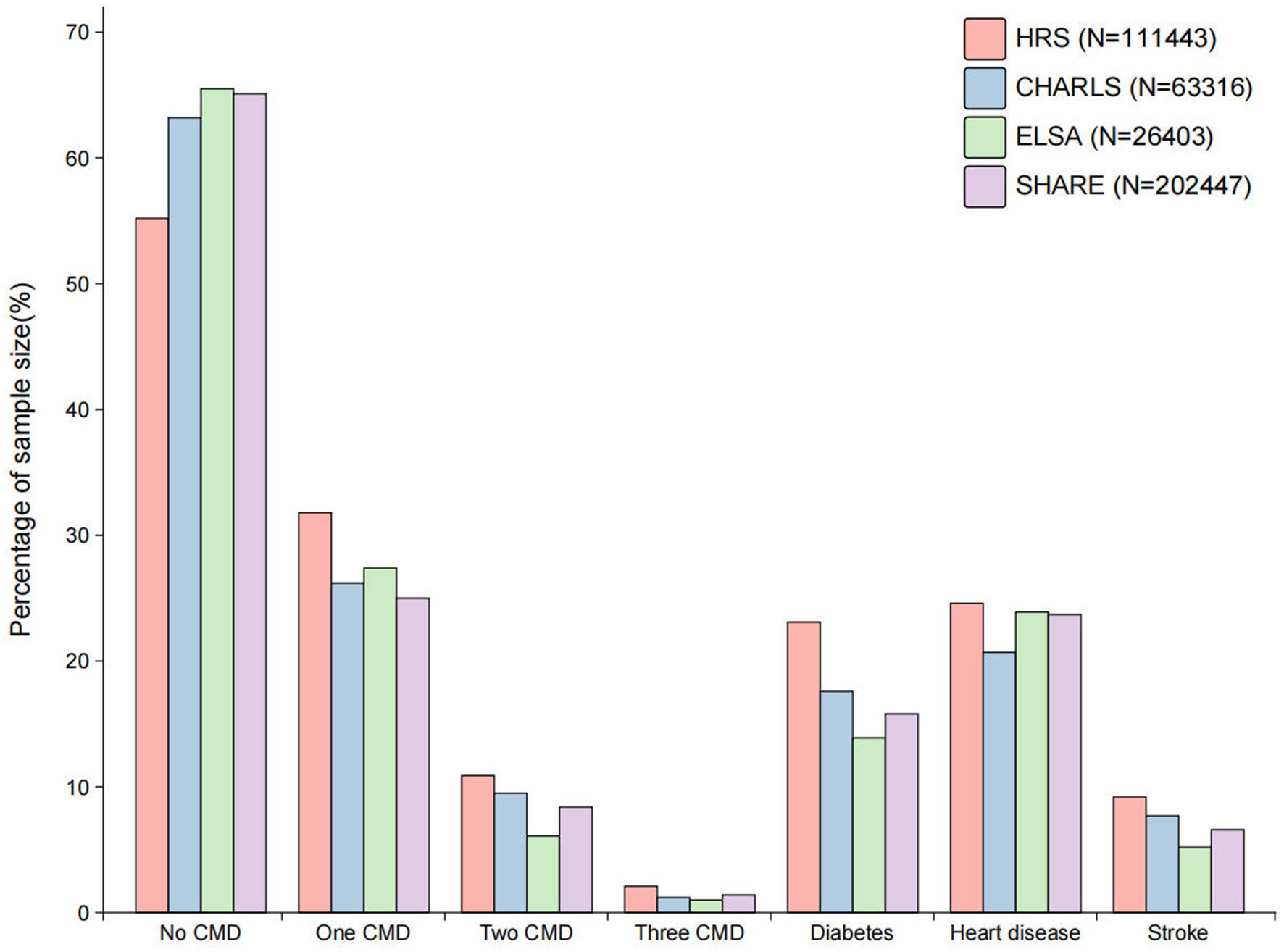
Figure 1. The distribution of cardiometabolic diseases across the various databases. CMD, cardiometabolic diseases; HRS, Health and Retirement Study; CHARLS, China Health and Retirement Longitudinal Study; ELSA, English Longitudinal Study of Ageing; SHARE, Survey of Health, Ageing and Retirement in Europe.
Participants without cardiometabolic diseases were used as the reference group in this study. Among those with a single cardiometabolic condition, a modest yet statistically significant increase in frailty index was observed [unadjusted β = 7.80 (95% CI: 7.70 to 7.90)]. In addition, a more rapid increase in the frailty index was observed with the increasing number of cardiometabolic conditions. More specifically, the frailty index increased significantly in individuals with two cardiometabolic diseases [unadjusted β = 17.92 (95% CI: 17.76 to 18.08)] and more markedly in those with three cardiometabolic diseases [unadjusted β = 28.79 (95% CI: 28.41 to 29.17)]. Model 2 made adjustments for both gender and age, while Model 1 was an unadjusted model. Several covariates, including obesity, cancer, lifestyle factors and educational attainment, were taken into consideration by Model 3 (Table 2). These patterns remained significant even after adjustments for covariates were made.
Analysis of the relationships between specific combinations of individual cardiometabolic diseases and frailty indicated that stroke had the most significant effect on the frailty index of all the single cardiometabolic conditions (Figure 2). Among combinations of two coexisting cardiometabolic diseases, the pair of diabetes and stroke was found to be most strongly associated with a more rapid increase in the frailty index. Furthermore, in comparison to the presence of one or two cardiometabolic diseases, the coexistence of three cardiometabolic diseases was found to have a significant association with a more substantial increase in the frailty index (Supplementary Tables 6–9).
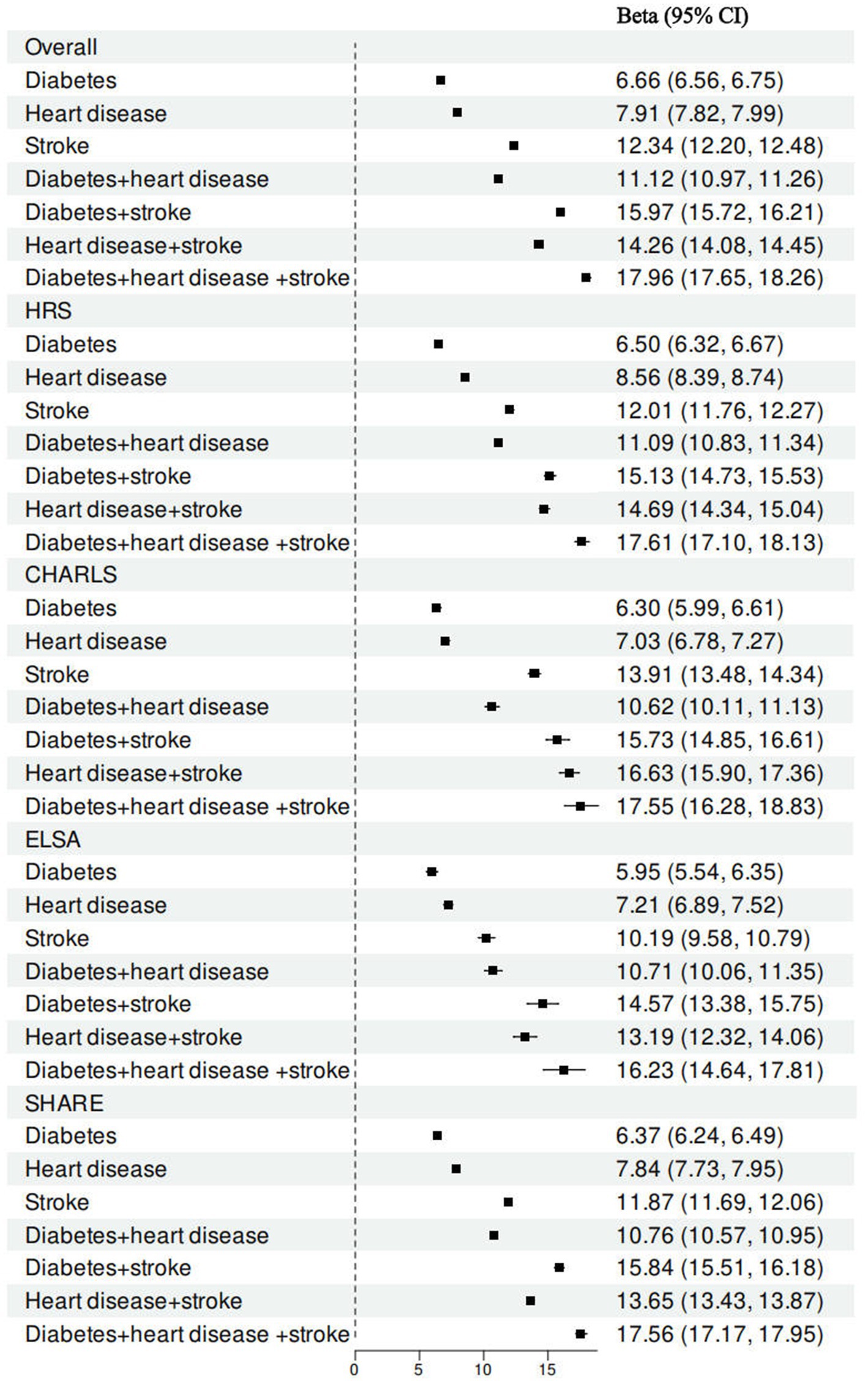
Figure 2. Associations between cardiometabolic disease status and frailty index by specific combination of individual cardiometabolic diseases. All models were adjusted for age gender, marital status, educational attainment, obesity, hypertension, cancer, lung disease, current drinking, current smoking, and physical activity.
In addition to the overall analysis, the association between the prevalence of cardiometabolic diseases and frailty across the four datasets was examined. A dose–response correlation between the number of cardiometabolic diseases and the frailty index was found in the HRS, with β values increasing progressively as the number of comorbidities increased [single cardiometabolic disease: β = 3.72 (95% CI: 3.55 to 3.89), two cardiometabolic diseases: β = 10.15 (95% CI: 9.91 to 10.38), three cardiometabolic diseases: β = 17.61 (95% CI: 17.10 to 18.13)]. The CHARLS, ELSA and SHARE datasets all exhibited a similar trend (Figure 3).
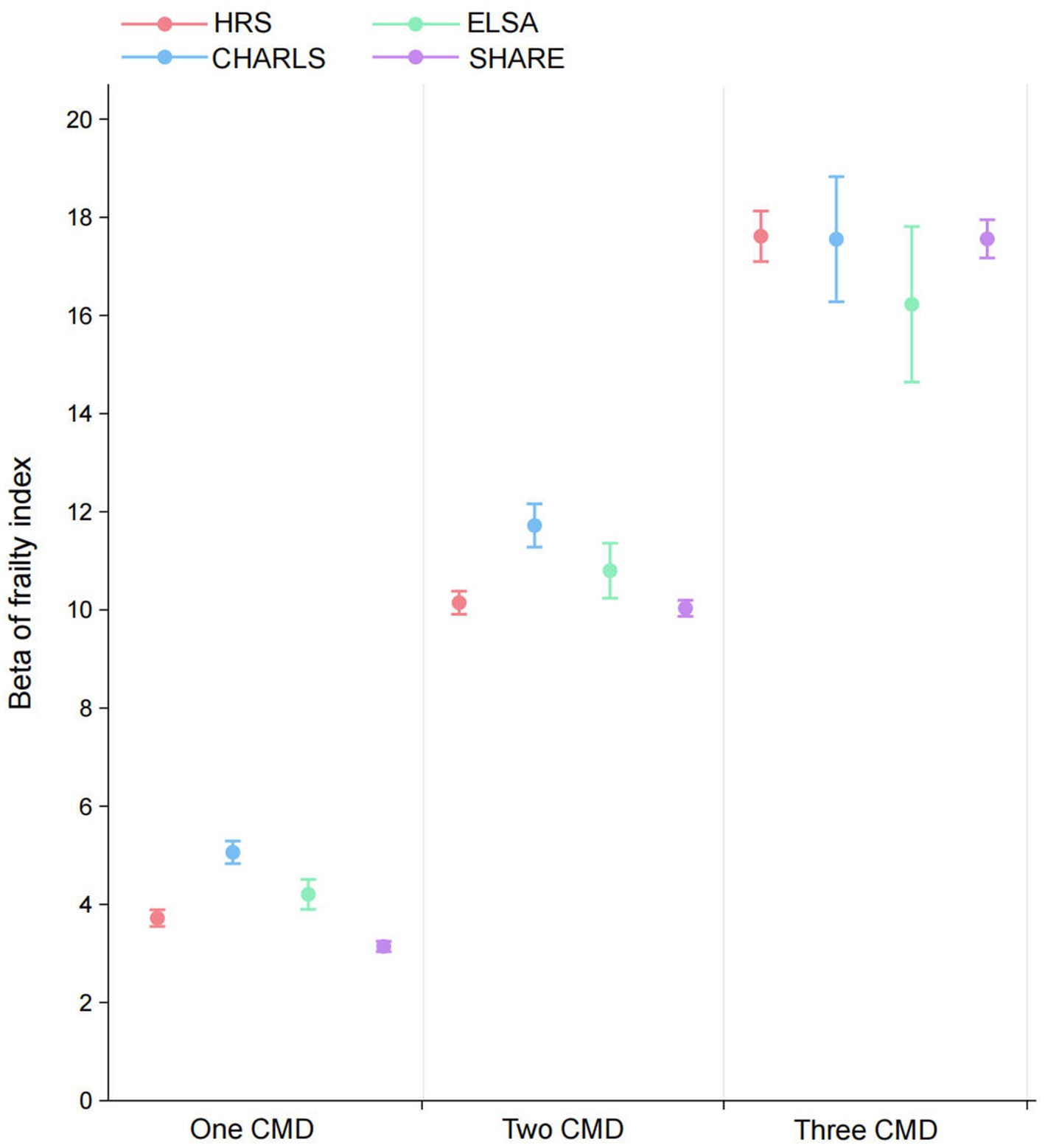
Figure 3. Associations between cardiometabolic multimorbidity status and frailty index in HRS, CHARLS, ELSA, and SHARE. All models were adjusted for age gender, marital status, educational attainment, obesity, hypertension, cancer, lung disease, current drinking, current smoking, and physical activity. CMD, cardiometabolic diseases; HRS, Health and Retirement Study; CHARLS, China Health and Retirement Longitudinal Study; ELSA, English Longitudinal Study of Ageing; SHARE, Survey of Health, Ageing and Retirement in Europe.
In the subgroup analysis, we employed interaction tests (P for interaction) to assess the differences in the strength of the association between CMD and the frailty index across different subgroups. The results showed that the interaction effects of age (P for interaction <0.01) and gender (P for interaction <0.01) were statistically significant, while the interaction effects of current drinking (P for interaction = 0.912), current smoking (P for interaction = 0.993), and physical activity (P for interaction = 0.134) were not significant. Specifically, in the older population (age ≥ 65), the association between CMD and the frailty index was stronger (β = 6.74, 95% CI: 6.62–6.86, p < 0.01), whereas in the younger population (age < 65), the association was weaker (β = 5.00, 95% CI: 4.87–5.14, p < 0.01). Similarly, in the female subgroup, the association was more pronounced (β = 7.37, 95% CI: 7.24–7.51, p < 0.01), while in the male subgroup, the association was weaker (β = 5.76, 95% CI: 5.63–5.88, p < 0.01). These results suggest that gender and age may be important moderating factors in the relationship between CMD and the frailty index, while drinking, smoking, and physical activity do not significantly impact this relationship (Supplementary Figure 6).
Discussion
This study has summarized the prevalence of cardiometabolic diseases across four international cohorts (HRS, CHARLS, ELSA and SHARE) and explored the relationship between cardiometabolic multimorbidity and frailty. The findings revealed a significant increase in frailty index as the number of cardiometabolic conditions increased, with greater multimorbidity having a significant correlation with more severe frailty. More specifically, those with a single cardiometabolic disease already had a higher frailty index, while those with two or more conditions had a more pronounced increase in frailty. Further analysis served to demonstrate a distinct dose–response correlation between the frequency of cardiometabolic diseases and the frailty index, particularly in individuals with stroke, where the increase in frailty was particularly marked. The coexistence of diabetes and stroke was found to have the most significant impact on frailty, potentially due to the compounded effect these conditions have on physical decline and overall health burden. These findings serve to highlight the substantial impact cardiometabolic multimorbidity has on frailty in older populations, which shows that there is a need for clinical interventions to address multiple comorbidities in older adult patients.
Cardiometabolic diseases, which include diabetes, heart disease and stroke, share several overlapping pathophysiological processes that make a collective contribution to the exacerbation of frailty (34). One of the primary mechanisms is chronic inflammation, which is a common feature with all of these conditions (35, 36). In diabetes, insulin resistance promotes a pro-inflammatory state (37), while heart disease is associated with systemic inflammation that is driven by endothelial dysfunction and atherosclerosis (38, 39). Stroke leads to neuroinflammation as a result of cerebral ischemia and neuronal injury, particularly in its chronic phase (40, 41). The systemic elevation of inflammatory markers, such as tumour necrosis factor-α (TNF-α) and C-reactive protein (CRP) (42), is important in the pathogenesis of frailty as it contributes to vascular damage (43), muscle atrophy (44) and diminished functional capacity (45), thereby accelerating frailty progression.
Oxidative stress is another shared pathological process that plays a significant role in the cellular damage that is observed in these diseases (46). In diabetes, hyperglycaemia leads to an excess of ROS, which causes damage to cellular structures, including endothelial cells, thereby exacerbating cardiovascular complications (47). Similarly, in heart disease, persistent heart failure contributes to impaired tissue oxygenation, which further amplifies oxidative stress (48). After a stroke, cerebral ischemia induces mitochondrial dysfunction, increasing ROS production and damaging both neuronal and muscle tissues (49, 50). The cumulative oxidative damage in skeletal muscles results in muscle wasting and weakness, which are key components of frailty (51, 52).
Autonomic dysfunction is a critical common pathway in cardiometabolic diseases, which affects the neuro-muscular coordination that is necessary for maintaining physical strength and stability (53). Diabetic neuropathy and the disruption of central nervous system control following a stroke impair motor coordination, which leads to reduced physical performance and mobility (54, 55). These mechanisms act synergistically and this results in more severe frailty in those with multiple cardiometabolic comorbidities.
In comparison to previous studies, the results of this study are largely consistent and demonstrate broader applicability. Gao et al. identified a significant association between cardiometabolic multimorbidity, frailty and healthcare utilization and highlighted that the presence of multiple cardiometabolic diseases increases the incidence of frailty and healthcare expenditure (23). In this study, the findings from datasets from the United States, England and Europe similarly indicate a clear dose–response relationship between cardiometabolic multimorbidity and frailty. More specifically, as the number of cardiometabolic conditions increases, the severity of frailty and the corresponding healthcare demands both exhibit a substantial increase.
There is a notable alignment when comparing the findings of Tang et al. with the results from the subgroup analysis of this study, particularly regarding gender differences. Tang et al. found women to have a higher frailty index than men, particularly in the presence of cardiometabolic diseases, such as stroke (56). The subgroup analysis in this study found that the association between CMD and the frailty index was significantly stronger in females than in males. Several factors may have contributed to these differences, such as hormonal variations, particularly the decline in oestrogen post-menopause, which accelerates muscle loss and increases frailty risk in women (57). In addition, it is typical for women to experience a higher burden of chronic illnesses, including CVD and osteoporosis, which further exacerbates frailty (58).
Furthermore, our study was compared with the study by Luo et al. (59), which explored the relationship between multimorbidity and frailty transitions in USA, finding that multimorbidity significantly increased the risk of frailty deterioration, with distinct patterns affecting frailty transitions. In contrast, our research utilized data from four international cohorts and focused on cardiometabolic diseases, revealing that stroke had the most pronounced impact on frailty. This study not only corroborates previous findings but also provides novel insights, emphasizing the cumulative impact of multiple cardiometabolic conditions on frailty, thus offering a new perspective for clinical intervention strategies.
Our findings suggest that CMD significantly increases the risk of frailty, particularly among older adults and women. Therefore, public health policies should place greater emphasis on high-risk populations, such as through regular health screenings and personalized health management, to identify and intervene in potential frailty risks at an early stage (15, 60). From a disease prevention perspective, promoting healthy lifestyles should be incorporated into national and regional public health programs. For example, optimizing nutritional guidance, encouraging regular physical activity, and managing chronic disease risk factors can effectively reduce the incidence of frailty.
From a healthcare policy standpoint, our study provides a foundation for optimizing chronic disease management. As CMD patients often require long-term, multidisciplinary medical support, healthcare systems should strengthen the capacity of primary healthcare institutions in managing chronic diseases, such as by offering integrated health management services, reducing repeated hospitalizations, and minimizing medical resource waste. These measures will not only improve patient health outcomes but also reduce the burden on the healthcare system and enhance resource utilization efficiency.
The strengths of this study include the use of multinational data, its large sample size and the use of harmonized standards across four international cohorts, which served to significantly enhance the generalisability and representativeness of the findings. By including diverse populations from different geographical regions, the study provided a robust analysis of cardiometabolic multimorbidity and frailty, which allowed for more accurate comparisons in a variety of healthcare systems and cultural contexts.
However, the study also has a number of limitations that must be noted. Firstly, some health conditions and lifestyle factors were based on self-reported data, which may present biases such as social desirability bias and recall bias, thereby potentially compromising the accuracy of the information that was supplied. Although our study employed complete case analysis to ensure the robustness of the data, we recognize that methods such as multiple imputation could further enhance data utilization and reduce potential selection bias. Secondly, although this study utilized multiple international cohorts (HRS, CHARLS, ELSA, SHARE), offering a broad sample representation, we acknowledge that there are methodological differences in the data collection processes across these cohorts. For instance, variations in disease diagnosis, health indicator measurements, and survey design may introduce certain biases during data integration. While we employed linear regression models to examine the relationship between cardiometabolic diseases and frailty, the statistical analysis may still be influenced by unmeasured variables, such as dietary habits and socioeconomic factors, which could potentially confound the results. Future research could consider employing more rigorous standardization methods or statistical strategies like propensity score matching to reduce inter-cohort biases and enhance the robustness of the findings. In addition, cross-sectional data was used in this investigation, which served to restrict the capacity to draw clear causal inferences between cardiometabolic multimorbidity and frailty. Furthermore, although this study has employed multilevel regression models and subgroup analyses to ensure the accuracy and robustness of the analyses, we acknowledge that further statistical techniques, such as more advanced Bayesian methods or machine learning approaches, could offer a more comprehensive assessment of the relationship between cardiometabolic diseases and the frailty index. However, due to constraints in time and resources, we have not yet conducted more extensive statistical expansions. In future research, we intend to incorporate additional statistical methodologies to further enhance the depth and precision of the study.
Conclusion
This study demonstrates a significant dose–response relationship between cardiometabolic multimorbidity and frailty, with stroke having the most pronounced impact. Older adults and women, in particular, are more susceptible to the exacerbation of frailty due to the influence of multiple cardiometabolic conditions. These findings underscore the importance of implementing early intervention strategies targeting the aging population to mitigate the risk of frailty.
Data availability statement
Publicly available datasets were analyzed in this study. This data can be found here: CHARLS (https://charls.pku.edu.cn/en), ELSA (https://www.elsa-project.ac.uk/), SHARE (https://share-eric.eu/), and HRS (https://hrs.isr.umich.edu/). The original contributions presented in the study are included in the article/Supplementary material. Further inquiries can be directed to the corresponding author.
Ethics statement
Ethical review and approval was not required for the study on human participants in accordance with the local legislation and institutional requirements. Written informed consent from the [patients/ participants OR patients/participants legal guardian/next of kin] was not required to participate in this study in accordance with the national legislation and the institutional requirements.
Author contributions
KZ: Data curation, Methodology, Software, Writing – original draft. AW: Data curation, Software, Writing – original draft. KY: Formal analysis, Methodology, Project administration, Software, Supervision, Writing – review & editing.
Funding
The author(s) declare that no financial support was received for the research and/or publication of this article.
Acknowledgments
We thank the all participants, related workers in data creating and cleaning, depositors and funders of HRS, CHARLS, ELSA, and SHARE.
Conflict of interest
The authors declare that the research was conducted in the absence of any commercial or financial relationships that could be construed as a potential conflict of interest.
Generative AI statement
The authors declare that no Gen AI was used in the creation of this manuscript.
Publisher’s note
All claims expressed in this article are solely those of the authors and do not necessarily represent those of their affiliated organizations, or those of the publisher, the editors and the reviewers. Any product that may be evaluated in this article, or claim that may be made by its manufacturer, is not guaranteed or endorsed by the publisher.
Supplementary material
The Supplementary material for this article can be found online at: https://www.frontiersin.org/articles/10.3389/fpubh.2025.1565682/full#supplementary-material
Footnotes
References
1. Ferreira, SRG, Chiavegatto Filho, ADP, Lebrão, ML, Duarte, YADO, and Laurenti, R. Cardiometabolic diseases. Rev Bras Epidemiol. (2019) 21:e180008. doi: 10.1590/1980-549720180008.supl.2
2. Yang, K, Hou, R, Zhao, J, Wang, X, Wei, J, Pan, X, et al. Lifestyle effects on aging and CVD: a spotlight on the nutrient-sensing network. Ageing Res Rev. (2023) 92:102121. doi: 10.1016/j.arr.2023.102121
3. Galkin, F, Kovalchuk, O, Koldasbayeva, D, Zhavoronkov, A, and Bischof, E. Stress, diet, exercise: common environmental factors and their impact on epigenetic age. Ageing Res Rev. (2023) 88:101956. doi: 10.1016/j.arr.2023.101956
4. Smith, JA, Kho, M, Zhao, W, Yu, M, Mitchell, C, and Faul, JD. Genetic effects and gene-by-education interactions on episodic memory performance and decline in an aging population. Soc Sci Med. (2021) 271:112039. doi: 10.1016/j.socscimed.2018.11.019
5. Bellary, S, Kyrou, I, Brown, JE, and Bailey, CJ. Type 2 diabetes mellitus in older adults: clinical considerations and management. Nat Rev Endocrinol. (2021) 17:534–48. doi: 10.1038/s41574-021-00512-2
6. Ciumărnean, L, Milaciu, MV, Negrean, V, Orășan, OH, Vesa, SC, Sălăgean, O, et al. Cardiovascular risk factors and physical activity for the prevention of cardiovascular diseases in the elderly. Int J Environ Res Public Health. (2021) 19:207. doi: 10.3390/ijerph19010207
7. Spence, JD, Azarpazhooh, MR, Larsson, SC, Bogiatzi, C, and Hankey, GJ. Stroke prevention in older adults: recent advances. Stroke. (2020) 51:3770–7. doi: 10.1161/STROKEAHA.120.031707
8. Bhattarai, U, Bashyal, B, Shrestha, A, Koirala, B, and Sharma, SK. Frailty and chronic diseases: a bi-directional relationship. Aging Med (Milton). (2024) 7:510–5. doi: 10.1002/agm2.12349
9. Ida, S, Kaneko, R, Imataka, K, and Murata, K. Relationship between frailty and mortality, hospitalization, and cardiovascular diseases in diabetes: a systematic review and meta-analysis. Cardiovasc Diabetol. (2019) 18:81. doi: 10.1186/s12933-019-0885-2
10. Kim, DH, and Rockwood, K. Frailty in older adults. N Engl J Med. (2024) 391:538–48. doi: 10.1056/NEJMra2301292
11. Wang, X, Hu, J, and Wu, D. Risk factors for frailty in older adults. Medicine (Baltimore). (2022) 101:e30169. doi: 10.1097/MD.0000000000030169
12. Maheshwari, V, and Basu, S. Sarcopenic obesity burden, determinants, and association with risk of frailty, falls, and functional impairment in older adults with diabetes: a propensity score matching analysis. Cureus. (2023) 15:e49601. doi: 10.7759/cureus.49601
13. Gielen, E, Dupont, J, Dejaeger, M, and Laurent, MR. Sarcopenia, osteoporosis and frailty. Metabolism. (2023) 145:155638. doi: 10.1016/j.metabol.2023.155638
14. Iwasaki, M, Shirobe, M, Motokawa, K, Tanaka, T, Ikebe, K, Ueda, T, et al. Prevalence of Oral frailty and its association with dietary variety, social engagement, and physical frailty: results from the Oral frailty 5-item checklist. Geriatr Gerontol Int. (2024) 24:371–7. doi: 10.1111/ggi.14846
15. Payne, ME, Porter Starr, KN, Orenduff, M, Mulder, HS, McDonald, S, Spira, AP, et al. Quality of life and mental health in older adults with obesity and frailty: associations with a weight loss intervention. J Nutr Health Aging. (2018) 22:1259–65. doi: 10.1007/s12603-018-1127-0
16. Fried, LP, Tangen, CM, Walston, J, Newman, AB, Hirsch, C, Gottdiener, J, et al. Frailty in older adults: evidence for a phenotype. J Gerontol A Biol Sci Med Sci. (2001) 56:M146–57. doi: 10.1093/gerona/56.3.M146
17. Yoon, SJ, and Kim, KI. Frailty and disability in diabetes. Ann Geriatr Med Res. (2019) 23:165–9. doi: 10.4235/agmr.19.0036
18. Molsted, S, Jensen, TM, Larsen, JS, Olesen, LB, Eriksen, SBM, Rehling, T, et al. Changes of physical function and quality of life in patients with type 2 diabetes after exercise training in a municipality or a hospital setting. J Diabetes Res. (2022) 2022:5751891. doi: 10.1155/2022/5751891
19. Yudkovicz, JJ, Minster, RL, Barinas-Mitchell, E, Christensen, K, Feitosa, M, Barker, MS, et al. Pleiotropic effects between cardiovascular disease risk factors and measures of cognitive and physical function in long-lived adults. Sci Rep. (2021) 11:17980. doi: 10.1038/s41598-021-97298-0
20. El Assar, M, Laosa, O, and Mañas, LR. Diabetes and frailty. Curr Opin Clin Nutr Metab Care. (2019) 22:52–7. doi: 10.1097/MCO.0000000000000535
21. Stewart, R. Cardiovascular disease and frailty: what are the mechanistic links? Clin Chem. (2019) 65:80–6. doi: 10.1373/clinchem.2018.287318
22. Palix, C, Felisatti, F, Gonneaud, J, Kuhn, E, Mézenge, F, Landeau, B, et al. Relationships between diabetes-related vascular risk factors and neurodegeneration biomarkers in healthy aging and Alzheimer's disease. Neurobiol Aging. (2022) 118:25–33. doi: 10.1016/j.neurobiolaging.2022.06.004
23. Gao, K, Li, BL, Yang, L, Zhou, D, Ding, KX, Yan, J, et al. Cardiometabolic diseases, frailty, and healthcare utilization and expenditure in community-dwelling Chinese older adults. Sci Rep. (2021) 11:7776. doi: 10.1038/s41598-021-87444-z
24. Wang, Y, Li, R, Yuan, L, Yang, X, Lv, J, Ye, Z, et al. Association between diabetes complicated with comorbidities and frailty in older adults: a cross-sectional study. J Clin Nurs. (2023) 32:894–900. doi: 10.1111/jocn.16442
25. Jia, Y, Li, D, Yu, J, Liu, Y, Li, F, Li, W, et al. Subclinical cardiovascular disease and frailty risk: the atherosclerosis risk in communities study. BMC Geriatr. (2022) 22:321. doi: 10.1186/s12877-022-02974-z
26. Huang, YN, Yan, FH, Wang, XY, Chen, XL, Chong, HY, Su, WL, et al. Prevalence and risk factors of frailty in stroke patients: a Meta-analysis and systematic review. J Nutr Health Aging. (2023) 27:96–102. doi: 10.1007/s12603-023-1879-z
27. Zazzara, MB, Vetrano, DL, Carfì, A, and Onder, G. Frailty and chronic disease. Panminerva Med. (2019) 61:486–92. doi: 10.23736/S0031-0808.19.03731-5
28. Huang, ZT, Luo, Y, Han, L, Wang, K, Yao, SS, Su, HX, et al. Patterns of cardiometabolic multimorbidity and the risk of depressive symptoms in a longitudinal cohort of middle-aged and older Chinese. J Affect Disord. (2022) 301:1–7. doi: 10.1016/j.jad.2022.01.030
29. Jin, Y, Liang, J, Hong, C, Liang, R, and Luo, Y. Cardiometabolic multimorbidity, lifestyle behaviours, and cognitive function: a multicohort study. Lancet Healthy Longev. (2023) 4:e265–73. doi: 10.1016/S2666-7568(23)00054-5
30. Fishbook, BN, Brinton, CD, Siever, J, Klassen, TD, and Sakakibara, BM. Cardiometabolic multimorbidity and activity limitation: a cross-sectional study of adults using the Canadian longitudinal study on aging data. Fam Pract. (2022) 39:455–63. doi: 10.1093/fampra/cmab129
31. Searle, SD, Mitnitski, A, Gahbauer, EA, Gill, TM, and Rockwood, K. A standard procedure for creating a frailty index. BMC Geriatr. (2008) 8:24. doi: 10.1186/1471-2318-8-24
32. Sun, J, Kong, X, Li, H, Chen, J, Yao, Q, Li, H, et al. Does social participation decrease the risk of frailty? Impacts of diversity in frequency and types of social participation on frailty in middle-aged and older populations. BMC Geriatr. (2022) 22:553. doi: 10.1186/s12877-022-03219-9
33. He, D, Qiu, Y, Yan, M, Zhou, T, Cheng, Z, Li, J, et al. Associations of metabolic heterogeneity of obesity with frailty progression: results from two prospective cohorts. J Cachexia Sarcopenia Muscle. (2023) 14:632–41. doi: 10.1002/jcsm.13169
34. Tazzeo, C, Rizzuto, D, Calderón-Larrañaga, A, Roso-Llorach, A, Marengoni, A, Welmer, AK, et al. Multimorbidity patterns and risk of frailty in older community-dwelling adults: a population-based cohort study. Age Ageing. (2021) 50:2183–91. doi: 10.1093/ageing/afab138
35. Ferrucci, L, and Fabbri, E. Inflammageing: chronic inflammation in ageing, cardiovascular disease, and frailty. Nat Rev Cardiol. (2018) 15:505–22. doi: 10.1038/s41569-018-0064-2
36. Tamura, Y, Omura, T, Toyoshima, K, and Araki, A. Nutrition Management in Older Adults with diabetes: a review on the importance of shifting prevention strategies from metabolic syndrome to frailty. Nutrients. (2020) 12:3367. doi: 10.3390/nu12113367
37. Chait, A, and den Hartigh, LJ. Adipose tissue distribution, inflammation and its metabolic consequences, including diabetes and cardiovascular disease. Front Cardiovasc Med. (2020) 7:22. doi: 10.3389/fcvm.2020.00022
38. De Leonardis, F, Colalillo, G, Finazzi Agrò, E, Miano, R, Fuschi, A, and Asimakopoulos, AD. Endothelial dysfunction, erectile deficit and cardiovascular disease: an overview of the pathogenetic links. Biomedicines. (2022) 10:1848. doi: 10.3390/biomedicines10081848
39. Henein, MY, Vancheri, S, Longo, G, and Vancheri, F. The role of inflammation in cardiovascular disease. Int J Mol Sci. (2022) 23:12906. doi: 10.3390/ijms232112906
40. Candelario-Jalil, E, Dijkhuizen, RM, and Magnus, T. Neuroinflammation, stroke, blood-brain barrier dysfunction, and imaging modalities. Stroke. (2022) 53:1473–86. doi: 10.1161/STROKEAHA.122.036946
41. Yang, C, Hawkins, KE, Doré, S, and Candelario-Jalil, E. Neuroinflammatory mechanisms of blood-brain barrier damage in ischemic stroke. Am J Physiol Cell Physiol. (2019) 316:C135–c153. doi: 10.1152/ajpcell.00136.2018
42. Gonçalves, RSDSA, Maciel, ÁCC, Rolland, Y, Vellas, B, and de Souto Barreto, P. Frailty biomarkers under the perspective of geroscience: a narrative review. Ageing Res Rev. (2022) 81:101737. doi: 10.1016/j.arr.2022.101737
43. Avila-Funes, JA, Meillon, C, González-Colaço Harmand, M, Tzourio, C, Dartigues, JF, and Amieva, H. Association between frailty and carotid central structure changes: the Three-City study. J Am Geriatr Soc. (2014) 62:1906–11. doi: 10.1111/jgs.13062
44. Picca, A, Coelho-Junior, HJ, Calvani, R, Marzetti, E, and Vetrano, DL. Biomarkers shared by frailty and sarcopenia in older adults: a systematic review and meta-analysis. Ageing Res Rev. (2022) 73:101530. doi: 10.1016/j.arr.2021.101530
45. Subías-Perié, J, Navarrete-Villanueva, D, Fernández-García, ÁI, Moradell, A, Gesteiro, E, Pérez-Gómez, J, et al. Prevalence of metabolic syndrome and association with physical activity and frailty status in Spanish older adults with decreased functional capacity: a cross-sectional study. Nutrients. (2022) 14:2302. doi: 10.3390/nu14112302
46. Barteková, M, Adameová, A, Görbe, A, Ferenczyová, K, Pecháňová, O, Lazou, A, et al. Natural and synthetic antioxidants targeting cardiac oxidative stress and redox signaling in cardiometabolic diseases. Free Radic Biol Med. (2021) 169:446–77. doi: 10.1016/j.freeradbiomed.2021.03.045
47. Iacobini, C, Vitale, M, Haxhi, J, Pesce, C, Pugliese, G, and Menini, S. Mutual regulation between redox and hypoxia-inducible factors in cardiovascular and renal complications of diabetes. Antioxidants (Basel). (2022) 11:2183. doi: 10.3390/antiox11112183
48. Ndrepepa, G. Myeloperoxidase - a bridge linking inflammation and oxidative stress with cardiovascular disease. Clin Chim Acta. (2019) 493:36–51. doi: 10.1016/j.cca.2019.02.022
49. Sarkar, S, Chakraborty, D, Bhowmik, A, and Ghosh, MK. Cerebral ischemic stroke: cellular fate and therapeutic opportunities. Front Biosci (Landmark Ed). (2019) 24:435–50. doi: 10.2741/4727
50. Chen, H, He, Y, Chen, S, Qi, S, and Shen, J. Therapeutic targets of oxidative/nitrosative stress and neuroinflammation in ischemic stroke: applications for natural product efficacy with omics and systemic biology. Pharmacol Res. (2020) 158:104877. doi: 10.1016/j.phrs.2020.104877
51. Latham, CM, Balawender, PJ, Thomas, NT, Keeble, AR, Brightwell, CR, Ismaeel, A, et al. Overexpression of manganese superoxide dismutase mitigates ACL injury-induced muscle atrophy, weakness and oxidative damage. Free Radic Biol Med. (2024) 212:191–8. doi: 10.1016/j.freeradbiomed.2023.12.037
52. Bielecka‐Dabrowa, A, Ebner, N, Dos Santos, MR, Ishida, J, Hasenfuß, G, and Von Haehling, S. Cachexia, muscle wasting, and frailty in cardiovascular disease. Eur J Heart Fail. (2020) 22:2314–26. doi: 10.1002/ejhf.2011
53. Iturriaga, R. Carotid body ablation: a new target to address central autonomic dysfunction. Curr Hypertens Rep. (2018) 20:53. doi: 10.1007/s11906-018-0849-z
54. Sinacore, DR, Jones, MA, and Kline, PW. Major adverse foot events and functional mobility deficits associated with diabetic neuropathy and nephropathy. Metab Target Organ Damage. (2024) 4:15. doi: 10.20517/mtod.2024.02
55. Moncion, K, Rodrigues, L, Wiley, E, Noguchi, KS, Negm, A, Richardson, J, et al. Aerobic exercise interventions for promoting cardiovascular health and mobility after stroke: a systematic review with Bayesian network meta-analysis. Br J Sports Med. (2024) 58:392–400. doi: 10.1136/bjsports-2023-107956
56. Tang, Z, Wang, C, Song, X, Shi, J, Mitnitski, A, Fang, X, et al. Co-occurrence of cardiometabolic diseases and frailty in older Chinese adults in the Beijing longitudinal study of ageing. Age Ageing. (2013) 42:346–51. doi: 10.1093/ageing/aft004
57. Ko, SH, and Jung, Y. Energy metabolism changes and dysregulated lipid metabolism in postmenopausal women. Nutrients. (2021) 13:4556. doi: 10.3390/nu13124556
58. Xu, W, Zhao, X, Zeng, M, Wu, S, He, Y, and Zhou, M. Exercise for frailty research frontiers: a bibliometric analysis and systematic review. Front Med (Lausanne). (2024) 11:1341336. doi: 10.3389/fmed.2024.1341336
59. Luo, Y, Chen, Y, Wang, K, de Fries, CM, Huang, Z, Xu, H, et al. Associations between multimorbidity and frailty transitions among older Americans. J Cachexia Sarcopenia Muscle. (2023) 14:1075–82. doi: 10.1002/jcsm.13197
Keywords: multicohort cardiometabolic diseases, frailty index, diabetes, heart disease, stroke, middle-aged and older people
Citation: Zhou K, Wang A and Yi K (2025) Cardiometabolic multimorbidity and frailty in middle-aged and older adults: a cross-nationally harmonized study. Front. Public Health. 13:1565682. doi: 10.3389/fpubh.2025.1565682
Edited by:
Jaideep Menon, Amrita Vishwa Vidyapeetham University, IndiaReviewed by:
Karolina Sobczyk, Medical University of Silesia, PolandYan Luo, City University of Hong Kong, Hong Kong SAR, China
Copyright © 2025 Zhou, Wang and Yi. This is an open-access article distributed under the terms of the Creative Commons Attribution License (CC BY). The use, distribution or reproduction in other forums is permitted, provided the original author(s) and the copyright owner(s) are credited and that the original publication in this journal is cited, in accordance with accepted academic practice. No use, distribution or reproduction is permitted which does not comply with these terms.
*Correspondence: Ke Yi, eWlrZUBzY3UuZWR1LmNu