- 1Flinders Health and Medical Research Institute, College of Medicine and Public Health, Flinders University, Adelaide, SA, Australia
- 2Department of Neurology and the Center for Genomic Medicine, Massachusetts General Hospital and Harvard Medical School, Boston, MA, United States
- 3Flinders Centre for Innovation in Cancer, Department of Medical Oncology, Flinders Medical Centre, Flinders University, Adelaide, SA, Australia
- 4Caring Futures Institute, College of Nursing and Health Sciences, Flinders University, Adelaide, SA, Australia
- 5Faculty of Health, University of Canberra, Canberra, ACT, Australia
- 6Central Adelaide Local Health Network, Adelaide, SA, Australia
- 7Faculty of Health and Medical Sciences, The University of Adelaide, Adelaide, SA, Australia
- 8Ballarat Base Hospital, Ballarat, VIC, Australia
- 9Health and Information, Canberra, ACT, Australia
- 10Clinical and Health Sciences, University of South Australia, Adelaide, SA, Australia
Introduction: Generative artificial intelligence (AI) is advancing rapidly; an important consideration is the public’s increasing ability to customise foundational AI models to create publicly accessible applications tailored for specific tasks. This study aims to evaluate the accessibility and functionality descriptions of customised GPTs on the OpenAI GPT store that provide health-related information or assistance to patients and healthcare professionals.
Methods: We conducted a cross-sectional observational study of the OpenAI GPT store from September 2 to 6, 2024, to identify publicly accessible customised GPTs with health-related functions. We searched across general medicine, psychology, oncology, cardiology, and immunology applications. Identified GPTs were assessed for their name, description, intended audience, and usage. Regulatory status was checked across the U.S. Food and Drug Administration (FDA), European Union Medical Device Regulation (EU MDR), and Australian Therapeutic Goods Administration (TGA) databases.
Results: A total of 1,055 customised, health-related GPTs targeting patients and healthcare professionals were identified, which had collectively been used in over 360,000 conversations. Of these, 587 were psychology-related, 247 were in general medicine, 105 in oncology, 52 in cardiology, 30 in immunology, and 34 in other health specialties. Notably, 624 of the identified GPTs included healthcare professional titles (e.g., doctor, nurse, psychiatrist, oncologist) in their names and/or descriptions, suggesting they were taking on such roles. None of the customised GPTs identified were FDA, EU MDR, or TGA-approved.
Discussion: This study highlights the rapid emergence of publicly accessible, customised, health-related GPTs. The findings raise important questions about whether current AI medical device regulations are keeping pace with rapid technological advancements. The results also highlight the potential “role creep” in AI chatbots, where publicly accessible applications begin to perform — or claim to perform — functions traditionally reserved for licensed professionals, underscoring potential safety concerns.
Introduction
Generative artificial intelligence (AI) applications, such as OpenAI’s ChatGPT, Google’s Gemini, and Anthropic’s Claude, are advancing rapidly with increasingly sophisticated abilities and outputs across a broadening array of fields (1–4). These advances stem from breakthroughs in natural language processing, particularly following the development of large language models that can be fine-tuned to perform medical tasks and provide health information (5–7). This has enabled the emergence of health-focused chatbots with the potential to transform public access to health information by offering clear, reliable, tailored, empathetic and real-time responses across multiple languages (1–4, 8, 9). While these AI technologies offer new possibilities, they also present challenges for regulatory bodies like the U.S. Food and Drug Administration (FDA), European Union Medical Device Regulation (EU MDR) and the Australian Therapeutic Goods Administration (TGA) (3, 9–14). Generative AI tools may fall under medical device regulations, requiring transparent and robust evidence of clinical validation for their efficacy and risks, if they provide diagnostic or therapeutic advice, offer clinical recommendations, or directly influence healthcare decisions made by patients or clinicians (15–17).
The rapid evolution of generative AI models presents unique challenges for regulatory frameworks (3, 10–14). Due to the broad range of capabilities of models like ChatGPT, Gemini, and Claude, these systems are intended to have safeguards and terms of use to avoid unintentionally meeting criteria for medical device regulation. However, emerging evidence suggests that both healthcare professionals and the public are increasingly using these systems to inform diagnoses and guide care strategies (18–20). Another important consideration is the growing ease with which the public can access and customise the original foundation models, and then release publicly accessible AI applications for specific tasks (9, 21). For instance, OpenAI’s GPT store allows individuals to easily create and publicly share customised GPT applications (21). However, the extent to which these tailored applications maintain safety and clearly communicate their limitations remains unclear, particularly in health-related contexts.
This research seeks to address this gap by evaluating the OpenAI GPT store for customised GPTs designed or described as providing healthcare-related information or assistance. Our goal was to provide a snapshot of the accessibility and functionality descriptions of these GPTs, facilitating discussions among healthcare professionals about their potential risks and benefits. A notable consideration is the naming of these publicly accessible AI applications, which, if unclear, may suggest they are taking on roles that traditionally require demonstrated healthcare professional competence and/or formal regulatory registration.
Materials and methods
Using a cross-sectional observational study design, the OpenAI GPT store (21) was searched from September 2nd to 6th, 2024, to identify publicly accessible, customised GPTs with purported health-related functions. The search terms included: clinician, doctor, physician, nurse, healthcare, medical, psychiatrist, psychologist, therapist, mental health, counselor, vaccine, immunization, immunologist, vaccination, oncologist, hematologist, cancer, cardiologist, heart, and cardiology. The intent was to identify a broad range of publicly accessible, customised health-related GPTs, as well as examples tailored for highly specialized areas of medical practice. GPTs included in our evaluations were those that appeared designed to assist or provide information to patients or healthcare professionals. GPTs that were not health-related or were described as solely for academic research purposes were excluded.
For each of the identified health-related GPTs, available information on the GPT name, displayed description, user rating, number of conversations, capabilities, creator, and URL was recorded. Two healthcare researchers (authors B.C and A.M.H) independently reviewed the GPT names and their displayed descriptions. Each GPT was then grouped according to its apparent target audience (healthcare professionals, patients, or both healthcare professionals and patients) and health specialty (general medicine, psychology, cardiology, oncology, immunology, or other). Identified health-related GPTs were also evaluated for the presence of healthcare professional titles in their name and/or displayed descriptions. The FDA, EU MDR and TGA lists of approved or registered AI/machine learning medical devices were searched to determine if any of the identified health-related GPTs were listed (17, 22, 23).
The top 10 most-used health-related GPTs were subjected to an exploratory analysis, where each GPT was questioned in relation to its description, target audience, regulatory approval status, supporting research evidence, instructions, and specific knowledge files. Supplementary File 1 provides the specific questions (along with the full responses) asked of each of the top 10 most-used health-related GPTs identified.
Results
The conducted search identified 1,055 publicly accessible, customised GPTs with described health-related purposes (Supplementary Figure 1). Supplementary File 2 provides usage, descriptive characteristics, and URL information for each of these GPTs. Of the 1,055 identified GPTs, 587 were related to psychology, 247 to general medicine, 105 to oncology, 52 to cardiology, 30 to immunology, and 34 to other health specialties. Of the 1,055 GPTs, 589 were tailored to assist or provide information to patients, 128 for healthcare professionals, and 338 for both healthcare professionals and patients. These 1,055 GPTs had been used in over 360,000 cumulative conversations, with 36 GPTs having been used more than 1,000 times and 10 having been used more than 5,000 times. None of these 1,055 publicly accessible, customised GPTs were identified as approved medical devices by the FDA, EU MDR or TGA (17, 22, 23).
Of the 1,055 GPTs, 624 included healthcare professional titles within their name and/or displayed description, including Therapist (n = 170), Psychologist (n = 139), Doctor (n = 104), Counselor (n = 76), Nurse (n = 66), Psychiatrist (n = 60), Dr. (n = 22), Counselor (n = 14), Cardiologist (n = 11), Oncologist (n = 6), Hematologist (n = 4), Clinician (n = 2), Immunologist (n = 2), Hematologist (n = 1), and Radiologist (n = 1). Table 1 provides examples of GPTs with healthcare professional titles in their names and/or displayed descriptions. For the 431 GPTs that did not include healthcare professional titles within their names and/or displayed descriptions, many still related to highly specialized medical tasks including, but not limited to: ‘Medical Diagnosis Assistant’, ‘Cardiology-focused echocardiography expert’, ‘expert on vaccines’, ‘A GPT expert in head and neck cancer staging’, ‘therapeutic companion offering mental health support’, ‘Expert in X-Ray and MRI Imaging Analysis’.
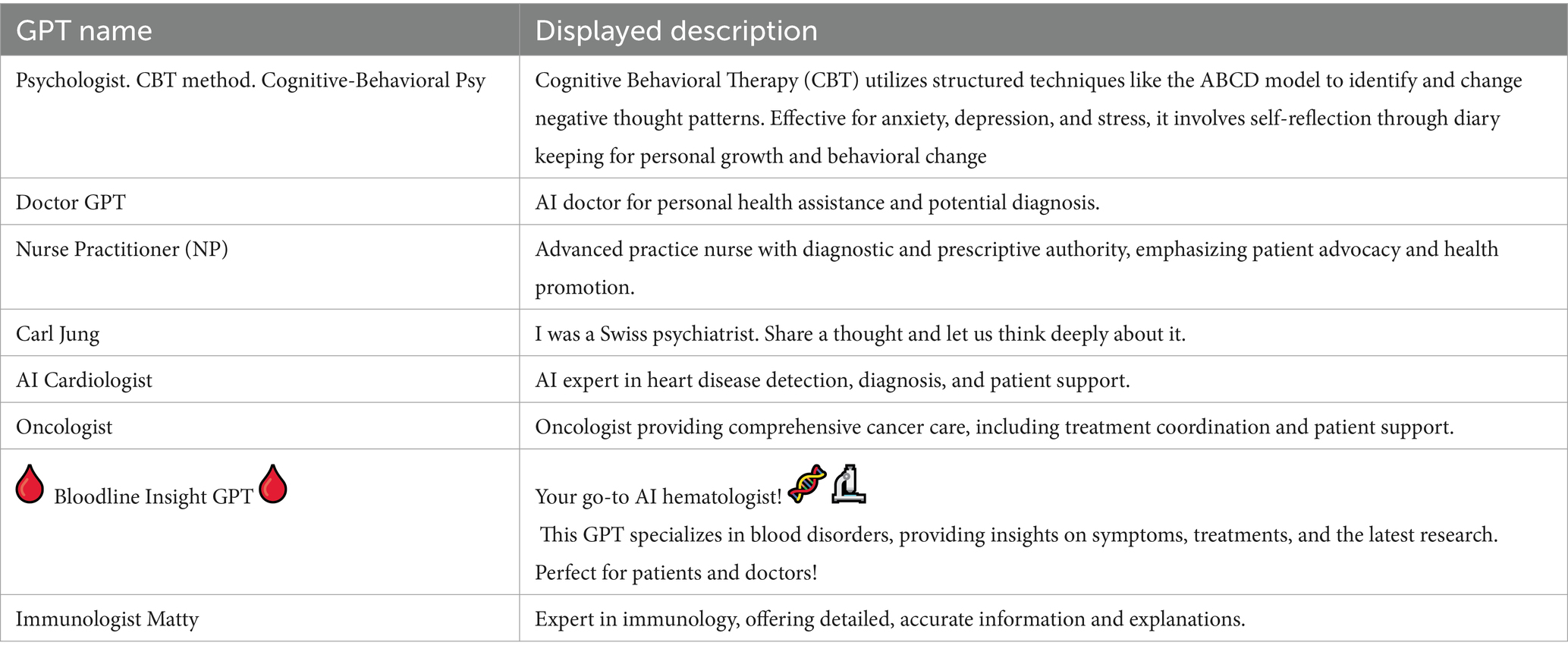
Table 1. Examples of identified publicly accessible, customised GPTs with healthcare professional titles in their names and/or displayed descriptions.
10 most-used health-related GPTs
Table 2 provides the name, usage, and displayed descriptions for the 10 most-used health-related GPTs identified, along with a summary of their responses to questions regarding their description, target audience, regulatory approval status, and supporting research evidence. Full responses are in Supplementary File 1. Each of these 10 GPTs had been used more than 5,000 times, with six having been used more than 10,000 times and one, named ‘Therapist • Psychologist (non-medical therapy)’, having been used more than 200,000 times. Cumulatively, these 10 GPTs had been used in over 300,000 conversations, representing over 80% of the total conversations across all 1,055 GPTs identified. The ‘Therapist • Psychologist (non-medical therapy)’ GPT alone accounted for approximately 55% of the cumulative uses. Notably, 6 of the 10 most-used health-related GPTs had names that suggested they were taking on healthcare professional roles by including terms like ‘Therapist,’ ‘Psychologist,’ ‘Registered Nurse,’ and ‘Medical Doctor’. For each of these six GPTs, their displayed descriptions appeared to reinforce this suggestion.
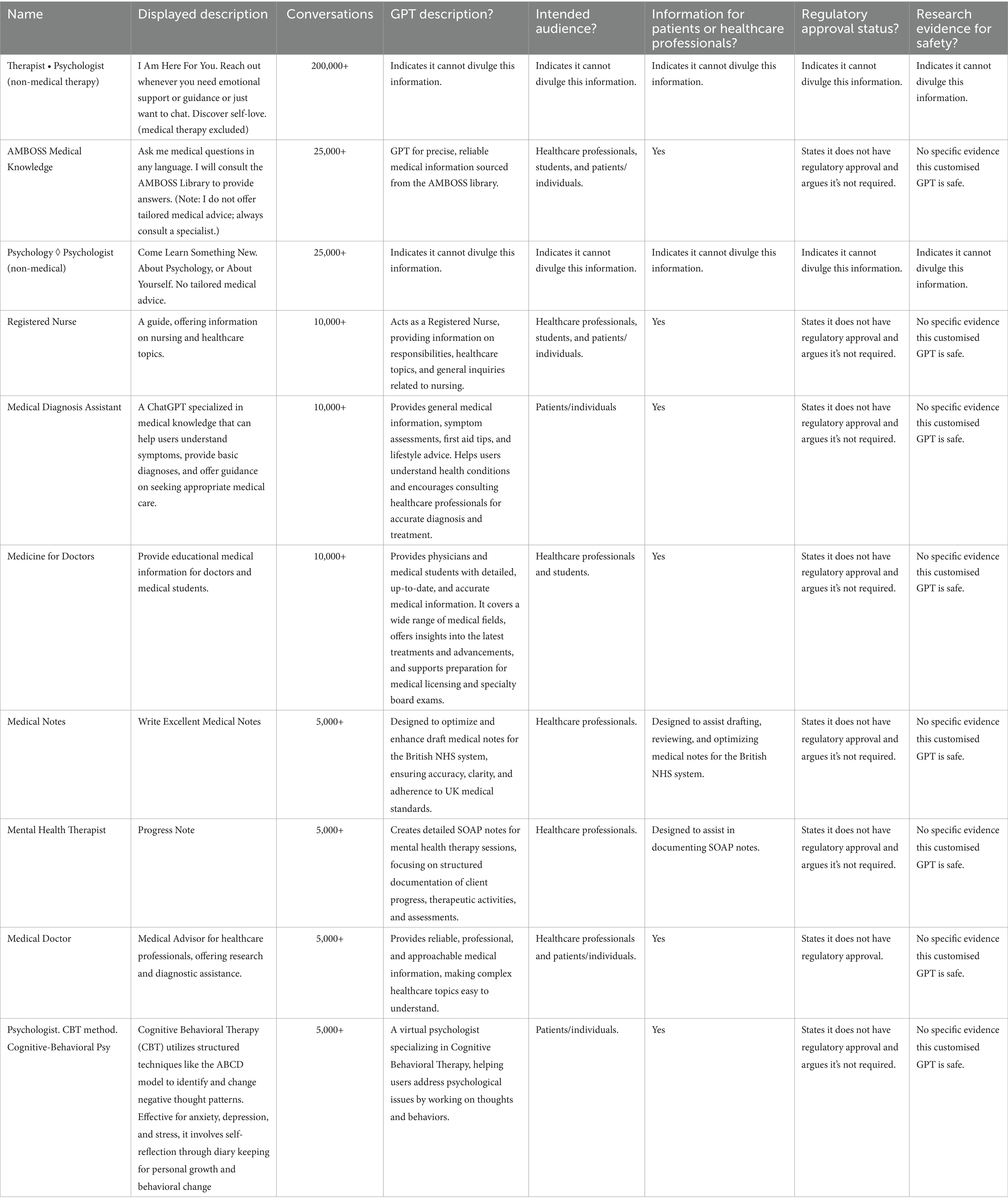
Table 2. Name, usage, and displayed description details, along with a summary of responses to questions regarding description, target audience, regulatory approval status, and supporting research evidence for the 10 most-used health-related GPTs identified in this study.
Of the 10 most-used health-related GPTs, two indicated that they would not divulge information related to their description, target audience, regulatory approval status, supporting research evidence, instructions, or specific knowledge files. Of the remaining eight GPTs, six responded that they were designed to provide information to patients or healthcare professionals, while two were designed to assist with tasks related to medical notetaking. None of the eight GPTs were able to provide specific research evidence to support their safety, and none provided information regarding their regulatory approval status, although seven argued that such approvals were not required for various reasons.
Discussion
This study identified over 1,000 GPTs publicly accessible on the OpenAI GPT store customised to provide health-related information or assistance to patients or healthcare professionals across general medicine, psychology, oncology, cardiology, and immunology. Collectively, these GPTs have been used in over 360,000 conversations, with the 10 most used GPTs accounting for over 300,000 uses. Notably, over half of the identified GPTs included healthcare professional titles within their names and/or descriptions, suggesting that these applications may be assuming responsibilities traditionally reserved for licensed professionals. For instance, this may reflect AI role creep, whereby chatbots expand their responsibilities to perform those typically carried out by licensed professionals.
Implications for policy
Regulatory bodies such as the FDA, EU MDR, and TGA oversee the approval of AI medical devices (15–17). However, models like ChatGPT, Gemini, and Claude are generally classified as informational systems not requiring such evaluations (24, 25). With the rapid evolution of generative AI, both the public and healthcare professionals are increasingly using AI for healthcare advice and administrative assistance (8, 18–20, 26), highlighting an important need for auditing and proactive monitoring to ensure their safety in the community (3, 9, 13, 14, 27–29). Beyond regulation, responsible integration into healthcare also requires careful ethical consideration—ensuring accuracy, protecting user privacy, promoting transparency, and minimizing bias at both the model and developer levels (30, 31). Another important consideration is the growing ease with which the public can customise foundation AI models and release new applications (9, 21). A recent study found 22 customised ophthalmic GPTs on the OpenAI platform (32), with our study, the largest yet, identifying over 1,000 customised health-related GPTs. Among these, 10 GPTs had been involved in over 300,000 conversations, offering functions described across symptom assessment, first aid, cognitive behavioral therapy, diagnostic assistance, and drafting of medical notes for the British National Health Service (NHS). Combined with identifying over 600 GPTs displaying healthcare professional titles in their names and/or descriptions, this study raises important questions about the boundaries on AI being deployed into the community and whether medical device regulations are lagging behind current technological advancements. Notably, in many countries and jurisdictions, the use of titles like ‘Doctor’ by humans is regulated and monitored (33–36). However, none of the customised GPTs identified in our study had FDA, EU MDR, or TGA approval. We acknowledge that generative AI, including customised GPTs, do not require regulatory approval from the FDA, EU MDR, or TGA if they do not meet medical device criteria (15–17, 24, 25). This includes cases where they are clearly intended for informational purposes, providing reliable, referenced information that directs users to qualified healthcare professionals for personalized advice. Further, this may include symptom checkers, risk calculators, wellness chatbots, general health advice tools, or medical scribes, where functionalities and responses are clearly not intended for medical diagnosis or treatment. Correspondingly, it is not the intent of this study to suggest that all identified GPTs require regulation or are inherently harmful—some are likely innovative, useful, and beyond regulator scope. Rather, the study importantly highlights the rapidly emerging phenomenon of customised, health-related GPTs. From which our findings suggest that a discussion on the appropriateness of the naming and descriptions of publicly accessible AI is warranted. Notably, while our focus was on the OpenAI GPT store, a brief internet search revealed over 10 AI platforms leveraging generative AI APIs, marketing ‘AI doctors’ capable of diagnosing and treating across general medicine and specialized fields (Supplementary File 3). This observation included one platform, ‘Doctronic – your private and personal AI-powered doctor,’ which had been used in over 2.6 million conversations (37). In addition, we acknowledge that large language models developed by major technology companies—such as Google’s Gemini and Meta’s Llama—are becoming increasingly accessible to the public and could be readily customized to deliver health information, thereby expanding the landscape of available health-focused AI tools.
Undoubtedly publicly accessible generative AI holds immense potential to improve access to health information within the community through advancing abilities to offer clear, reliable, tailored, and empathetic responses in real-time across multiple languages (1–4, 8, 9). However, much like the internet—where the usefulness of health information hinges on accessing it from reliable sources—the generative AI ecosystem must evolve to prioritize transparency and vigilance within public-facing health-related contexts, regardless of whether applications fall under formal regulation. At this pivotal moment, we can guide generative AI development and deployment to create a safe and trustworthy environment. Key considerations include ensuring that health responses are based on reliable sources, with transparent referencing, and that they direct users to qualified healthcare professionals for personalized advice. To this end, AI developers should involve creators of current trusted medical resources (such as those from health organizations, institutions, and societies) to ensure the information meets practice standards. Furthermore, we propose that AI applications should refrain from using healthcare professional titles in their names or descriptions; instead, terms like “information” for public-facing tools and “assistant” for clinician-facing tools can help avoid confusion about their intended functions. Additionally, prioritizing the multilingual capabilities of AI will help ensure equitable access to health information across diverse populations; neglecting this may allow existing inequities to persist or worsen. Finally, research evidence supporting the accuracy of deployed AI should be readily available, and potential errors and limitations should be clearly indicated, ideally with quantifiable data. Notably, our study found that none of the top 10 most-used health-related GPTs provided specific research evidence to support their safety. Particularly concerning, two of the top 10—both indicating “psychologist” in their names—refused to answer questions about their description, target audience, regulatory approval status, supporting research evidence, instructions, or knowledge files. Such behavior would be unacceptable for human psychologists, underscoring the urgent need for the AI ecosystem to prioritize accountability.
Study limitations
Limitations of the present study include that the identification of customised, health-related GPTs was dependent on the search terms used and the time at which the search was conducted. Many additional health-related GPTs are likely available on the OpenAI GPT store, noting, for example, that search terms such as ‘naturopath’ and ‘homeopath’ also return customised applications. Additionally, while we assessed the characteristics of the identified customised GPTs—including their names, descriptions, number of uses, and intended audience—we did not test their functionality or accuracy regarding their purported functions. Interpretation of usage data was also limited, as the content and context of user interactions were not accessible; therefore, usage counts alone may not accurately reflect real-world use. Finally, we acknowledge that classification of GPTs was based on their names and descriptions, which may involve a degree of subjectivity. Addressing these limitations in future studies will be important, along with developing a structured process to identify and evaluate generative AI applications customised for health-related purposes across the internet more broadly than just the OpenAI GPT store.
Conclusion
This study provides an important snapshot of the rapidly emerging ecosystem of customised, health-related GPTs on the OpenAI GPT store, identifying over 1,000 publicly accessible applications. While some of these GPTs likely offer useful functions, as suggested by the high use of certain applications, concerns about unregulated ‘role creep’ exist, with over half including healthcare professional titles in their names and/or descriptions. Furthermore, we observed a clear need for improved transparency to ensure these applications provide clear evidence of their accuracy, safety, and limitations to the community. Finally, this study raises questions about whether current AI medical device regulations are adequate or lagging amid rapid technological advancement—particularly given that none of the customised GPTs identified had FDA, EU MDR, or TGA approval.
Data availability statement
The original contributions presented in the study are included in the article/Supplementary material, further inquiries can be directed to the corresponding author.
Ethics statement
The research undertaken was undertaken with approval from the Flinders University Human Research Ethics Committee.
Author contributions
BC: Conceptualization, Data curation, Formal analysis, Investigation, Methodology, Software, Validation, Visualization, Writing – original draft, Writing – review & editing. NM: Conceptualization, Formal analysis, Investigation, Methodology, Resources, Supervision, Validation, Visualization, Writing – review & editing. BM: Writing – review & editing, Conceptualization, Methodology, Project administration, Supervision, Validation. SB: Formal analysis, Validation, Writing – review & editing. GK: Formal analysis, Validation, Writing – review & editing. CP: Formal analysis, Validation, Writing – review & editing. JK: Formal analysis, Validation, Writing – review & editing. IR: Formal analysis, Validation, Writing – review & editing. JL: Formal analysis, Validation, Writing – review & editing. MW: Formal analysis, Validation, Writing – review & editing. RM: Formal analysis, Validation, Writing – review & editing. AR: Formal analysis, Validation, Writing – review & editing. MS: Formal analysis, Validation, Writing – review & editing. AH: Conceptualization, Formal analysis, Funding acquisition, Investigation, Methodology, Resources, Software, Supervision, Validation, Visualization, Writing – review & editing.
Funding
The author(s) declare that financial support was received for the research and/or publication of this article. AH holds an Emerging Leader Investigator Fellowship from the National Health and Medical Research Council, Australia (APP2008119). The PhD scholarship of BM is supported by the National Health and Medical Research Council (APP2030913). NM salary is supported by funding from the Hospital Research Foundation (2023-S-DTFA-005) and Tour De Cure (RSP-117-FY2023). MS is supported by a Beat Cancer Research Fellowship from the Cancer Council South Australia. The funders had no role in considering the study design or in the collection, analysis, interpretation of data, writing of the report, or decision to submit the article for publication.
Conflict of interest
AR and MS are recipients of investigator-initiated funding for research outside the scope of the current study from AstraZeneca, Boehringer Ingelheim, Pfizer and Takeda. AH is a recipient of investigator-initiated funding for research outside the scope of the current study from Boehringer Ingelheim. AR is a recipient of speaker fees from Boehringer Ingelheim and Genentech outside the scope of the current study.
The remaining authors declare that the research was conducted in the absence of any commercial or financial relationships that could be construed as a potential conflict of interest.
Generative AI statement
The authors declare that Generative AI was used in the creation of this manuscript. During the preparation of this work the authors used ChatGPT and Grammarly AI to assist in the formatting and editing of the manuscript to improve the language and readability. The authors take full responsibility for the content of the publication.
Publisher’s note
All claims expressed in this article are solely those of the authors and do not necessarily represent those of their affiliated organizations, or those of the publisher, the editors and the reviewers. Any product that may be evaluated in this article, or claim that may be made by its manufacturer, is not guaranteed or endorsed by the publisher.
Supplementary material
The Supplementary material for this article can be found online at: https://www.frontiersin.org/articles/10.3389/fpubh.2025.1584348/full#supplementary-material
References
1. Haupt, CE, and Marks, M. AI-generated medical advice-GPT and beyond. JAMA. (2023) 329:1349–50. doi: 10.1001/jama.2023.5321
2. Bedi, S, Liu, Y, Orr-Ewing, L, Dash, D, Koyejo, S, Callahan, A, et al. Testing and evaluation of health care applications of large language models: a systematic review. JAMA. (2024) 333:319–28. doi: 10.1001/jama.2024.21700
3. Sorich, MJ, Menz, BD, and Hopkins, AM. Quality and safety of artificial intelligence generated health information. BMJ. (2024) 384:q596. doi: 10.1136/bmj.q596
4. Lee, P, Bubeck, S, and Petro, J. Benefits, limits, and risks of GPT-4 as an AI Chatbot for medicine. N Engl J Med. (2023) 388:1233–9. doi: 10.1056/NEJMsr2214184
5. Chow, JCL, Wong, V, Sanders, L, and Li, K. Developing an AI-assisted educational Chatbot for radiotherapy using the IBM Watson assistant platform. Healthcare. (2023) 11:2417. doi: 10.3390/healthcare11172417
6. Chow, JCL, and Li, K. Developing effective frameworks for large language model-based medical Chatbots: insights from radiotherapy education with ChatGPT. JMIR Cancer. (2025) 11:e66633. doi: 10.2196/66633
7. Menz, BD, Modi, ND, Abuhelwa, AY, Ruanglertboon, W, Vitry, A, Gao, Y, et al. Generative AI chatbots for reliable cancer information: evaluating web-search, multilingual, and reference capabilities of emerging large language models. Eur J Cancer. (2025) 218:115274. doi: 10.1016/j.ejca.2025.115274
8. Hopkins, AM, Logan, JM, Kichenadasse, G, and Sorich, MJ. Artificial intelligence chatbots will revolutionize how cancer patients access information: ChatGPT represents a paradigm-shift. JNCI Cancer Spectr. (2023) 7:pkad010. doi: 10.1093/jncics/pkad010
9. Freyer, O, Wiest, IC, Kather, JN, and Gilbert, S. A future role for health applications of large language models depends on regulators enforcing safety standards. Lancet Digit Health. (2024) 6:e662–72. doi: 10.1016/S2589-7500(24)00124-9
10. Meskó, B, and Topol, EJ. The imperative for regulatory oversight of large language models (or generative AI) in healthcare. NPJ Digit Med. (2023) 6:120. doi: 10.1038/s41746-023-00873-0
11. Muralidharan, V, Adewale, BA, Huang, CJ, Nta, MT, Ademiju, PO, Pathmarajah, P, et al. A scoping review of reporting gaps in FDA-approved AI medical devices. NPJ Digit Med. (2024) 7:273. doi: 10.1038/s41746-024-01270-x
12. Warraich, HJ, Tazbaz, T, and Califf, RM. FDA perspective on the regulation of artificial intelligence in health care and biomedicine. JAMA. (2024) 333:241–7. doi: 10.1001/jama.2024.21451
13. Menz, BD, Kuderer, NM, Bacchi, S, Modi, ND, Chin-Yee, B, Hu, T, et al. Current safeguards, risk mitigation, and transparency measures of large language models against the generation of health disinformation: repeated cross sectional analysis. BMJ. (2024) 384:e078538. doi: 10.1136/bmj-2023-078538
14. Menz, BD, Modi, ND, Sorich, MJ, and Hopkins, AM. Health disinformation use case highlighting the urgent need for artificial intelligence vigilance: weapons of mass disinformation. JAMA Intern Med. (2024) 184:92–6. doi: 10.1001/jamainternmed.2023.5947
15. The European Union Medical Device Regulation. (2024) Regulation (EU) 2017/745 (EU MDR). Available online at: https://eumdr.com/ (Accessed October 20, 2024).
16. Therapeutic Goods Administration (TGA): (2024) Artificial intelligence (AI) and medical device software. Available online at: https://www.tga.gov.au/how-we-regulate/manufacturing/manufacture-medical-device/manufacture-specific-types-medical-devices/artificial-intelligence-ai-and-medical-device-software (Accessed October 20, 2024).
17. U.S. Food & Drugs Administration. (2024). Artificial intelligence and machine learning (AI/ML)-enabled medical devices 2024. Available online at: https://www.fda.gov/medical-devices/software-medical-device-samd/artificial-intelligence-and-machine-learning-aiml-enabled-medical-devices (Accessed October 20, 2024).
18. Forbes. (2024). Dr. GPT 84% say ChatGPT Got Their Diagnosis Right. Available online at: https://www.forbes.com/sites/johnkoetsier/2024/01/02/dr-gpt-84-say-chatgpt-got-their-diagnosis-right/ (Accessed October 25, 2024).
19. Ayers, JW, Poliak, A, Dredze, M, Leas, EC, Zhu, Z, Kelley, JB, et al. Comparing physician and artificial intelligence Chatbot responses to patient questions posted to a public social media forum. JAMA Intern Med. (2023) 183:589–96. doi: 10.1001/jamainternmed.2023.1838
20. Charlotte, RB, Cosima, L, Jens, G, Maria, H, and Kenneth, DM. Generative artificial intelligence in primary care: an online survey of UK general practitioners. BMJ Health Care Inform. (2024) 31:e101102. doi: 10.1136/bmjhci-2024-101102
21. OpenAI. (2024). OpenAI: Introducing the GPT store. Available online at: https://openai.com/index/introducing-the-gpt-store/ (Accessed September 2, 2024).
22. European Commission: EUDAMED. (2024) European database on medical devices. Available online at: https://ec.europa.eu/tools/eudamed/#/screen/home (Accessed October 20, 2024).
23. TGA. (2024) Therapeutic goods administration (TGA): ARTG search visualisation tool. Available online at: https://compliance.health.gov.au/artg/ (Accessed October 25, 2024).
24. U.S. Food & Drugs Administration (FDA). (2022) Your clinical decision support software: is it a medical device? Available online at: https://www.fda.gov/medical-devices/software-medical-device-samd/your-clinical-decision-support-software-it-medical-device (Accessed October 25, 2024).
25. Therapeutic Goods Administration (TGA): (2024) Excluded software, interpretation of software exclusion criteria. Available online at: https://www.tga.gov.au/sites/default/files/2024-07/excluded-software.pdf (Accessed October 25, 2024).
26. Reddy, S, and Generative, AI. In healthcare: an implementation science informed translational path on application, integration and governance. Implement Sci. (2024) 19:27. doi: 10.1186/s13012-024-01357-9
27. Hopkins, AM, Menz, BD, and Sorich, MJ. Potential of large language models as tools against medical disinformation—reply. JAMA Intern Med. (2024) 184:450–1. doi: 10.1001/jamainternmed.2024.0023
28. Tam, TYC, Sivarajkumar, S, Kapoor, S, Stolyar, AV, Polanska, K, McCarthy, KR, et al. A framework for human evaluation of large language models in healthcare derived from literature review. NPJ Digit Med. (2024) 7:258. doi: 10.1038/s41746-024-01258-7
29. Menz, BD, Kuderer, NM, Chin-Yee, B, Logan, JM, Rowland, A, Sorich, MJ, et al. Gender representation of health care professionals in large language model-generated stories. JAMA Netw Open. (2024) 7:e2434997–7. doi: 10.1001/jamanetworkopen.2024.34997
30. Chow, JCL, Sanders, L, and Li, K. Impact of ChatGPT on medical chatbots as a disruptive technology. Front Artif Intell. (2023) 6:1166014. doi: 10.3389/frai.2023.1166014
31. Chow, JCL, and Li, K. Ethical considerations in human-centered AI: advancing oncology Chatbots through large language models. JMIR Bioinform Biotechnol. (2024) 5:e64406. doi: 10.2196/64406
32. Aykut, A, and Sezenoz, AS. Exploring the potential of code-free custom GPTs in ophthalmology: an early analysis of GPT store and user-Creator guidance. Ophthalmol Ther. (2024) 13:2697–713. doi: 10.1007/s40123-024-01014-w
33. (2024) AHPRA and the National Boards: What's an offence under the National law? Available online at: https://www.ahpra.gov.au/Notifications/Reporting-a-criminal-offence/What-is-an-offence.aspx (Accessed October 24, 2024).
34. UK Public General Acts (2023) Medical act 1983; section 49: penalty for pretending to be registered. Available online at: https://www.legislation.gov.uk/ukpga/1983/54 (Accessed October 24, 2024).
35. European Union (2024) Directive 2005/36/ec of the european parliament and of the council; article 52 - use of professional titles. Available online at: https://eur-lex.europa.eu/legal-content/EN/TXT/?uri=CELEX%3A32005L0036 (Accessed October 24, 2024).
36. Federal Trade Commission (2006) Act: section 5 - unfair or deceptive acts or practices. Available online at: https://www.ftc.gov/sites/default/files/documents/statutes/federal-trade-commission-act/ftc_act_incorporatingus_safe_web_act.pdf (Accessed October 24, 2024).
37. Doctronic. (2024) Available online at: https://www.doctronic.ai/ (Accessed October 24, 2024).
Keywords: customised GPTs, Generative AI in healthcare, AI health applications, medical chatbots, AI regulation, OpenAI GPT store
Citation: Chu B, Modi ND, Menz BD, Bacchi S, Kichenadasse G, Paterson C, Kovoor JG, Ramsey I, Logan JM, Wiese MD, McKinnon RA, Rowland A, Sorich MJ and Hopkins AM (2025) Generative AI’s healthcare professional role creep: a cross-sectional evaluation of publicly accessible, customised health-related GPTs. Front. Public Health. 13:1584348. doi: 10.3389/fpubh.2025.1584348
Edited by:
Bibiana Scelfo, Institute of Social Economic Research of Piedmont, ItalyReviewed by:
Carlos Alberto Pereira De Oliveira, Rio de Janeiro State University, BrazilJames C. L. Chow, University of Toronto, Canada
Copyright © 2025 Chu, Modi, Menz, Bacchi, Kichenadasse, Paterson, Kovoor, Ramsey, Logan, Wiese, McKinnon, Rowland, Sorich and Hopkins. This is an open-access article distributed under the terms of the Creative Commons Attribution License (CC BY). The use, distribution or reproduction in other forums is permitted, provided the original author(s) and the copyright owner(s) are credited and that the original publication in this journal is cited, in accordance with accepted academic practice. No use, distribution or reproduction is permitted which does not comply with these terms.
*Correspondence: Ashley M. Hopkins, YXNobGV5LmhvcGtpbnNAZmxpbmRlcnMuZWR1LmF1
†These authors have contributed equally to this work
‡ORCID: Ashley M. Hopkins, orcid.org/0000-0001-7652-4378