- 1Lincoln Institute for Agri-Food Technology, University of Lincoln, Lincoln, United Kingdom
- 2School of Computer Science, University of Lincoln, Lincoln, United Kingdom
- 3Interdisciplinary Centre for Data and AI, School of Natural and Computing Sciences, University of Aberdeen, Aberdeen, United Kingdom
- 4Lincoln International Business School, University of Lincoln, Lincoln, United Kingdom
- 5Faculty of Science, Technology, Engineering and Mathematics, Open University, Milton Keynes, United Kingdom
- 6School of Computing Sciences, University of East Anglia, Norwich, United Kingdom
- 7School of Chemistry, University of Southampton, Southampton, United Kingdom
- 8Business School, University of Exeter, Exeter, United Kingdom
The food system is undergoing a digital transformation that connects local and global supply chains to address economic, environmental, and societal drivers. Digitalisation enables firms to meet sustainable development goals (SDGs), address climate change and the wider negative externalities of food production such as biodiversity loss, and diffuse pollution. Digitalising at the business and supply chain level through public–private mechanisms for data exchange affords the opportunity for greater collaboration, visualising, and measuring activities and their socio-environmental impact, demonstrating compliance with regulatory and market requirements and providing opportunity to capture current practice and future opportunities for process and product improvement. Herein we consider digitalisation as a tool to drive innovation and transition to a decarbonised food system. We consider that deep decarbonisation of the food system can only occur when trusted emissions data are exchanged across supply chains. This requires fusion of standardised emissions measurements within a supply chain data sharing framework. This framework, likely operating as a corporate entity, would provide the foci for measurement standards, data exchange, trusted, and certified data and as a multi-stakeholder body, including regulators, that would build trust and collaboration across supply chains. This approach provides a methodology for accurate and trusted emissions data to inform consumer choice and industrial response of individual firms within a supply chain.
Introduction
In order to limit the Earth’s mean surface temperature increase to 2°C above pre-industrial levels, there is a need for global economies to achieve close to net zero greenhouse gas emissions (GHGEs) by 2050 (Bataille et al., 2016). To reach this goal, effective decarbonisation strategies need to be implemented. These need to measure and influence the demand and supply aspects of a process, organisation or wider complex system and drive sociotechnical transition at multiple levels (Geels et al., 2017; Rogelj et al., 2018). Decarbonisation in simple terms is the elimination or reduction of GHGEs associated with a given process or activity such as a food supply chain. Decarbonisation can be achieved by either reducing the intensity of GHGEs per unit of output (insetting) or alternatively, or in combination, offsetting GHGEs associated with the unit of output by equivalent GHGEs capture through another activity (Rogelj et al., 2018). When considering decarbonisation pathways, they can be described as deep, i.e., delivering a significant reduction from a single intervention, or alternatively, shallow where a significant but low reduction is associated with a specific intervention. A decarbonisation pathway can be implemented that encompasses both deep and shallow decarbonisation interventions, (e.g., adopting innovation strategies and/or behaviour change) that is rapid or slow in terms of impact. These factors combined will influence the timescales for transition to a low carbon economy, and then net zero (Rogelj et al., 2018).
The food system has been described as “encompassing food production, processing, and packaging, alternative forms of food production, food distribution, marketing and value chains, data and analytics, addressing waste, energy and water consumption and food access and affordability” [National Academies of Sciences, Engineering, and Medicine (NASEM), 2020]. Rapid and deep decarbonisation in sociotechnical food systems requires the integration of policy and regulations, market normative standards and procedures, organisational strategies, optimum infrastructures, changes to behavioural practices and innovation and technologies (Geels et al., 2017). Indeed, Grubb et al. (2014) propose that direct regulation combined with public investment and policy nudges, such as targeted carbon pricing, are crucial to enable the investment required for deep decarbonisation pathways. The resistance to decarbonisation, or carbon lock-in is “the tendency for certain carbon-intensive technological systems to persist over time, ‘locking out’ lower-carbon alternatives, owing [due] to a combination of linked technical, economic, and institutional factors.” (Erickson et al., 2015, p. 1). Carbon lock-in can be driven by strategic and financial investment in existing modes of operation preventing innovation and agility, especially if there is limited infrastructure and resources required to transition such as information asymmetry, limited technologies, inputs prone to lock-in, or weak core competencies and skills (Erickson et al., 2015; Geels et al., 2017). Circular reinforcing of past decisions (Erickson et al., 2015) is heightened when there is a lack of relevant, trustworthy data. We position that digitalisation is an essential pre-requisite for implementing effective decarbonisation strategies and pathways in the food system. This commentary paper considers the benefits, challenges and vulnerabilities of digitalising food supply chains to enable effective decarbonisation strategies.
Why digitalise?
Multiple sectors across global economies are undergoing digital transformations that include changes in data flow across decentralised, multi-tier complex systems (Brewer et al., 2021). In food systems, data represents intended inputs (such as finance, resources, water, energy, and labour), the bi-directional flows of resources, and information within and between organisations, and the intended (food products) and unintended outputs such as GHGEs, waste, point source or diffuse pollution (Brewer et al., 2021). Digital technologies can empower actors to design and drive system transformation by enabling data-driven decision-making, e.g., enabling the decarbonisation strategy within a process, organisation or wider food system (Poore and Nemecek, 2018), but supply chain complexity, power dynamics and friction can negatively impact data sharing and exchange. Immutable data, such as that enabled by distributed ledger technology, enhances trust, provides novel routes to certify processes, improves fidelity and can reduce fraud.
Supply chain complexity, power and friction in data sharing
Addressing the challenges currently facing organisations can require a supply chain management perspective, where each individual supply chain, due to the particularities of decarbonisation approaches, will embrace net zero in a unique way (Fankhauser et al., 2022). For Environmental and Social Governance (ESG) purposes companies within a supply chain are encouraged to report their GHGE aggregated (scope 1, 2 & 3) emissions (GHG Protocol, 2011). This approach is being adopted across the food industry globally, but implementation has been sporadic, Schulman et al. (2021) reported in a survey of 153 firms that disclosure was “incomplete and inconsistent.” Figure 1 illustrates the complexity for tracking GHGE emissions across a complex supply or value chain. Food supply chains are ephemeral. For decarbonisation strategies to be effective, accurate GHGE data needs to be captured and allowed to flow into (scope 1 and 2 emissions) the supply chain and longitudinally along the chain for estimation of scope 3 emissions. Orchestrating the flow, standards and certification of these data at a system level will require exceptional levels of integration and collaboration underpinned by effective governance, potentially supported by regulation.
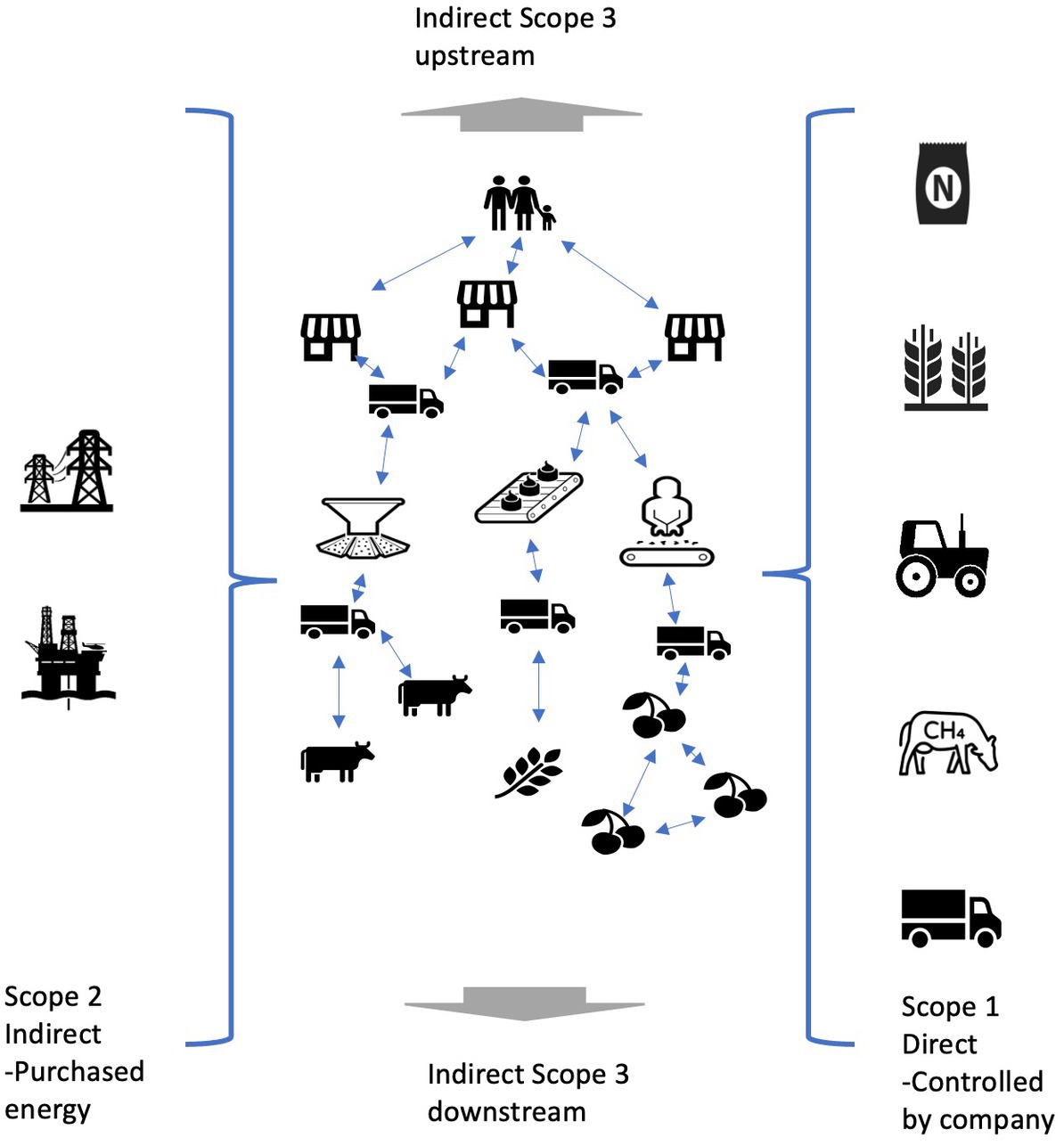
Figure 1. Archetypes of data and GHGE flow through food supply chains. The illustration is based on the GHG Protocol Value Chain (scope 3) articulation (GHG Protocol, 2011) that provides a standardised methodology to calculate GHG emissions for an individual firm. Here we show how data from an individual firm interacts with others in a food supply chain. The illustration shows the flow of goods from farm to consumers. It shows that food supply chains are ephemeral networks, consumers can buy from multiple retailers who in turn can select products from any number of connected suppliers. Data that measures the GHGE cost of that food (CO2e/kg) needs to flow between each trading firm in the chain (one step up, one step back). GHGE emission data (CO2e/kg) for any specific firm, but ultimately product, comprises the direct GHGE cost incurred and controlled by the firm [scope 1], at farm level this could aggregate GHGE cost of fertiliser inputs, methane etc. By convention scope 1 emission are added to the indirect costs of GHGE emission from fossil fuel inputs into the firm [scope 2], such as purchased energy. The additional scope 3 emissions for any firm in a supply chain are the sum of the indirect upstream and downstream GHGE emission costs of the firms’ product (s) as they move through the supply chain from farm to fork. If all GHGE costs are accounted, the scope 3 emissions for the whole supply chain will approximate to the sum of the scope 1 and 2 emissions of all the firms in a supply chain. GHGE emissions for any firm will be dynamic, dependent on order cycles, routes to market, seasonality etc. If a collective data sharing agreement can apply for the sharing of all scope 1 and 2 data between firms up and down a supply chain, then each individual firm can calculate their scope 3 costs.
It is increasingly accepted that in the transition towards net zero, supply chains will need new approaches to deal with the complexity of decarbonisation related data which flows between supply chain agents and tiers. Transparency, traceability, and security for net zero supply chains will require the development and adoption of new digital tools and potentially, new business models around these, for example, immutable distributed ledger technology that secures trust in data could enable certification whilst reducing fraud/greenwashing and information asymmetry between actors (Saberi et al., 2019). Engagement with consumers when designing and managing greener supply chains will be key to understanding the viability of net zero supply chains’ initiatives by society, enabling enhanced information sharing and the solving of green issues (Sarkis et al., 2011). However, to gather emissions information from suppliers as well as specific internal and external operations, focal companies (such as retailers, or large brands) or industry standards bodies may need to champion the adoption of digitally-enabled decarbonisation and net zero initiatives in their supply chains.
If champions are not forthcoming, then regulation may be required to standardise data collection and sharing. Friction might occur in supply chains where partners have casual transactional relationships (commodity trading where there are few incentives to share), those where there is no clear supply chain leader or representative body, supply chains with many smaller companies without the capacity to collaborate, measure and manage data, highly complex multi-step inter-industry chains (e.g., food production to logistics) or where there is significant mistrust of other supply chain partners. However, data sharing dead lock, due to a failure of collective governance will inhibit society’s drive to net zero; “a tragedy of the data commons.” Ostrom (1990) showed that society can evolve processes to share resources and avoid common’s tragedies, but this requires an evolution of trust over time, along with accountability guided by clear rules and incentives for all actors.
Standards, scale, and incentives
Decarbonisation strategies can be implemented at individual product, organisational, supply chain and system level. Regulatory and market incentives, singularly or in combination, can drive more environmentally and/or ecologically responsible management and inform decarbonisation (Hildingsson et al., 2018, p. 924). These incentives are embedded in normative standards, including agreed measurement standards for emissions, actioned within business contracts where compliance is often a pre-requisite to supply. For example, as an incentive to decarbonise, as well as addressing other socio-environmental aspects more widely, the United Kingdom food retailer Tesco plc now requires all their 14,000 produce suppliers to be LeafMarque certified (Tesco plc, 2021).
Another market-based incentive to decarbonise can be consumer focused carbon labelling at product level (Gadema and Oglethorpe, 2011; Tan et al., 2014), but to date labelling has engendered limited engagement from consumers (Carrero et al., 2021). However, the boundaries of a given food supply chain for a product or service can be difficult to define, which may cause uncertainty in expression of the carbon accounting results; thus, decreasing the credibility of the labelling scheme (Wu et al., 2014). As Rondoni and Grasso (2021) state, consumer engagement with carbon labelling is mediated by knowledge and this is influenced by access to information, which is informed by supply chain data. Providing carbon labelling that is accurate, trustworthy, and meaningful is dependent upon application of consistent measurement standards by individual actors fused with food system digitalisation to underpin data exchange and transparency, enabled by effective data collection, curation and communication processes. However, Hildingsson et al. (2018) argue that whilst corporate incentives can drive industrial decarbonisation, market dynamics alone, are far from sufficient to initiate the transition required. Instead, strong incentives generated by regulatory interventions and long-term policy support are likely required.
Stimulate the private sector and market to decarbonise
Stimulating the market to decarbonise can be achieved by regulation, legislation, or standards. This top down, prescriptive approach can quickly become a race to the bottom where companies do the bare minimum to comply or prevaricate and lobby to minimise any organisational impact (Manning, 2020). The alternative is for companies themselves to engage and take action because of changing market conditions and/or cost pressures.
The incentive to reduce scope 1 and 2 GHGEs for all companies is clear. All organisations are constrained by established principles on return on investment which in turn are subject to estimates of energy costs (both capital and recurring) over estimated time periods and subject to uncertainty over consumer sentiment. Governmental regulation and the opportunities to out compete rivals based on lower GHGEs continues to stimulate the move to lower emissions. The real challenge relates to scope 3 GHGEs which include all purchased goods and services, waste disposal, transportation and ‘costs of use’ by customers. Aggregated and accurate measurement of the scope 3 GHGEs of any individual product requires trusted emission data to flow through complex supply chains.
We anticipate that the ability to quantify GHGEs at product rather than whole firm level will drive new product development and supply chain configurations that ultimately lead to decarbonisation. However, the design of the product, and the collective processes by which it is made, is often opaque leading to challenging investment decisions. In practice it is not just capital costs, organisations have core rigidities that create inertia, inhibiting their ability to transform, so they require a level of ambidexterity (O’Reilly III and Tushman, 2013) to manage both their existing products and processes and at the same time innovate with new products and processes. These transition costs can be expensive and difficult to estimate, and can add cost in the short term, before resource efficiency benefits are realised. These short-term costs and risks need to be balanced against the long-term impacts of not transitioning, making the justification for change challenging.
What remains unclear when planning decarbonisation pathways and strategies is how consumers will evaluate the tradeoff between lower GHGEs and any increases in costs. The quantification and qualification of this ‘tradeoff’ in different markets and segments is a live research challenge requiring well specified longitudinal empirical work. Sovacool et al. (2021) promote optimisation as a crucial element in decarbonising food socio-technical systems. If technology, poor data availability and weak infrastructure are barriers to decarbonising, then the food system may have to commence with decarbonisation strategies that are shallow rather than deep and then increase ‘the gradient’ as technologies emerge and infrastructure improves (Hildingsson et al., 2018).
Shared infrastructure
Food supply chains encompass and interact with many environments, technologies, domains, and sectors, but the focus herein will be on digital aspects and information sharing. Some supply chain aspects and activities are inherently digital, or can readily be digitised, but to provide the benefits of digitalisation (e.g., more effective analysis and optimising of specific processes), all data producers and data consumers need to be identified. In data rich environments, data collection, collation, curation, analysis and reporting (e.g., assisted by AI/ML) are all key and, once in the digital realm, the IT and digital communications network backbone(s) are crucial shared resources. Suitable sharing, especially in a strategically sensitive environment, requires resilience structures to be in place especially the management of access and safety. Resilience can be achieved through ensuring redundancy within digital infrastructure (e.g., in Cloud storage or computing, and the Internet in its many shapes) and by making sure that all key nodes, communication links and critical data are properly safeguarded and protected from adverse events, either malicious or unintended.
To avoid data being lost, tampered with, or viewed by unauthorised parties, strict access control needs to be enforced for all actors, and technologies. Options such as blockchain, should be deployed in addition to broader cyber-security measures to guarantee the integrity of data along the whole supply chain (Brewer et al., 2021). While such protected infrastructures already exist, they tend to be restricted to the entities that built them for their own supply chains, leading to developmental duplication, weak interoperability, and poor transparency for supply chain actors. In an increasingly global and interdependent world, where decarbonisation and delivering net zero is a mutual goal, secrecy and ensuring competitive advantage at all costs may be replaced as prime market drivers, broadening the opportunity for greater information collaboration, potentially within a single shared infrastructure, and sharing of more of the physical components of supply chains, benefiting all actors and wider Society.
Interoperability
In order to support sharing of GHGEs data in the food supply chain, it is essential to assure interoperability between multiple heterogeneous data sources, the various systems in the supply chain, and the data users in the system. Interoperability is a well-known and studied problem, but not in the context of decarbonisation strategies. Interoperability is defined in the literature as the ability to exchange services and data among large-scale distributed systems, based on agreements between requesters and providers (Heiler, 1995). These agreements may range from message passing protocols, common application programming interfaces (API), domain models, names, or even ontologies to define concepts and relationships in a certain domain. One example is the FoodOn harmonised food ontology developed to improve food traceability, quality control and data integration (Dooley et al., 2018). However, despite its technical development, interoperability in food supply chains is still a challenging problem as data are captured at different levels of granularity, stored in different formats, are available in distinct, sometimes discrete systems, and may not be completely available (Brewer et al., 2021; Manning et al., 2022). Thus, higher levels of interoperability need to be available to allow the food supply chain to be digitised and efficient in serving consumers’ needs (Fernandez, 2021), including supporting their role in the transition to net zero.
A recent study by GS1 and the Global Dialogue on Seafood Traceability (GS1, 2019) highlights the requirement for (i) a standard-based framework with data models and APIs to share data across platforms, (ii) the need to use several technologies to interoperate and not only rely on blockchain technology, and (iii) the urgency for the food community to make use of electronic data exchange over manual sharing of supply chain data. Despite the need for and importance of interoperability in food supply chains, there are still several barriers that need to be overcame (Hardt et al., 2017). Examples of some of these barriers include, but are not limited to: (i) the need for more collaboration across the industry sector, (ii) the lack of resources and funding for some actors in the supply chain (e.g., small farms and producers, start-up-companies), (iii) the incompatibility among systems (e.g., old and legacy systems), (iv) poor agreement on standards alignment, (v) the lack of motivation and incentives among the industry, and (vi) the threats for small companies regarding the power dynamics associated with big companies with respect to data sharing. These barriers need to be tackled through industry collaboration together with enabling behavioural change by both food producers and consumers so that decarbonisation of the sector can be achieved.
Federated and trustless solutions
Effective decarbonisation strategies require, simple additive, emission data exchange across food supply chains so individual actors or organisations can start to determine their own and collective GHGEs. Next generation analytics tools, such as AI, could provide hitherto unimagined insight to inform decisions that enable decarbonisation across supply chains, including the benefits of enacting circularity. Privacy concerns, commercial sensitivity, and issues around intellectual property are significant barriers to digital collaboration in food supply chains, hindering even the additive exchange of data, as well as next generation analytics that can drive productivity and accelerated deep decarbonisation (Durrant et al., 2021). However, with the advent of data-related privacy-preserving technologies, there are now a novel opportunity to alleviate these issues and support sector level digitalisation. Approaches, such as federated learning (McMahan et al., 2017), differential privacy (Dwork and Roth, 2014), and fully homomorphic encryption (Gentry, 2009) could transform the agri-food sector by enabling the development of AI models to support decision making, while maintaining data privacy and trust. In the federated learning context, a centralised model can be trained collaboratively via many clients while keeping underpinning client data decentralised and confidential, e.g., grower data never leaving the premises yet can be used to train an AI model to support decarbonisation. However, this process, without effective cyber protection, is still susceptible to malicious attacks on the models developed. For example, via intercepting communications, which could reveal the contribution and content of individual data exchange and as such disclose information about individual clients that participated in the model training. Cyber-crime is a key concern especially unauthorised access to electronic communication and databases, networks, programmes and data with the intent of compromising confidentiality, integrity and availability of information (Bendovschi, 2015). Cybersecurity countermeasures must be in place to protect systems, storage and individual appliances (Manning, 2019; Soon et al., 2019).
One way to overcome this is through differential privacy approaches whereby uncertainty is introduced into the released models through addition of noise, masking the effect of individual user contribution to the model development, as demonstrated by Durrant et al. (2022) in a soybean yield forecasting context. Another option is full homomorphic encryption, whereby data are encrypted throughout computation without the need to share any unencrypted data with others. This newly encrypted data can be used to train machine learning models and infer outcomes or support decarbonisation strategies. Onoufriou et al. (2021) proposed such a system for milk yield prediction, demonstrating the feasibility of this approach to transform data sharing principles and enable trust in the agri-food sector, facilitating a faster transition to net zero.
Data governance and ethics
Establishing trusted multi-lateral data exchange of standardised GHGE measurements between parties, across a food system, is an essential prerequisite to data exchange in complex systems and ultimately, decarbonisation. Any governance system needs to address both business to business (B2B) and business to consumer (B2C) interactions (Brewer et al., 2021). Data trusts have been proposed as a form of participatory governance (Milne et al., 2021; Sadowski et al., 2021) defined by trust law and where data are securely shared for the greater good, under the care of a stewardship function (trustee) that has fiduciary responsibility to act in the interest of all parties (Brewer et al., 2021). Trust framework models, that enable emissions’ data sharing across food systems require four logical components: a governance structure that defines, rules, roles and the levels and processes of governance; a security and permissioning layer where participant access and network connectivity is controlled by an agreed process; a knowledge mapping component which establishes interoperability at interfaces between distinct system elements and an operational component where the business processes are enacted (Brewer et al., 2021). The governance structure could provide a forum to define both measurement standards as well as data exchange. The operational component is the location where the monitoring of compliance with regulatory and industry normative standards takes place. There have also been calls to develop a common language for technology adoption across food supply chains so that as AI and associated technologies are adopted the ethical aspects and impacts of technology design, implementation and use can be suitably considered (Manning et al., 2022).
Role of regulation
In the United Kingdom public health in relation to food is overseen by the Food Standards Agency (FSA). The use of data plays an important part in the role of the food regulator, not only in terms of measuring and monitoring oversight, but also in measuring the level of trust that the Agency has acquired in the eyes of the public (Krebs, 2004). Addressing the complexity of decarbonising the food system will necessitate integrating regulators within carbon governance mechanisms such as trust frameworks. Trust frameworks for carbon data exchange could include both multiple government regulators (environment agencies, EU Emission Trading System, financial services etc) and non-government bodies (certification, standards, and registration bodies).
Regulation, particularly in the context of new technologies, requires a reasonable precautionary attitude that addresses multiple factors, moral hazards and socio-political dimensions of risk (Wolff, 2018). Ensuring the independence of regulators, whilst addressing the challenge of integrating the regulatory process across multiple contributory sectors, can be facilitated using intermediaries working within clear governance boundaries. The role of intermediaries in the regulatory process has been theoretically captured in the RIT Framework which describes the roles of Regulators (R), Intermediaries (I), and Targets (T) (Abbott et al., 2017). Whilst there may be multiple actors adopting these roles, the RIT Framework allows for an approach that enables “complex, hybrid governance” and serves to reduce the risk of regulatory capture by, for example, the use of accreditation bodies and third-party certifiers (Abbott et al., 2017). This hybridisation of regulatory governance has already been seen as transcending national state boundaries through coordinated of public and private regulatory activities (Verbruggen, 2017; Kowalska and Manning, 2022). Addressing decarbonisation challenges will require more diverse intermediaries to be integrated into a RIT Framework that is polycentric and interdependent with robust regulatory stewardship. The trust framework approach can further support this through the permissioned sharing of critical data between independent organisations representing various roles and agencies (Brewer et al., 2021).
Limitations
There are clear limitations and barriers to data sharing and exchange that will need to be overcome. Our model envisaged that multiple architypes of data (e.g., GHGE, food safety) might be governed by a solus data framework, yet this pre-supposes that the competing interests for the exchange of multi-purpose data are the similar. This may not be the case, some supply chain actors might permission access to specific data sets and not others. Permissioning could be accommodated in a single or indeed separate and distinct governance systems.
Developing data sharing communities requires bodies who come together for mutual purpose; however, additional behavioural research is required to understand the symmetry and asymmetry for data sharing across a supply chain. It is highly likely that elements within a supply chain may feel threatened by data exchange, not least firms trading with dominant actors such as multiple retailers. Studies to inform multi-perspective attitudes to data sharing are required to understand and resolve these primary barriers, or to understand how to stage implementation to enable partners to evolve trust.
The founding of any data sharing process will require significant leadership that can convene a community for defined purposes and to develop the technical, legal and governance structures. It is not yet clear where this leadership will emerge, our hypothesis was that this may emerge from dominant supply chain actors (large retailers, branded good producers). These actors have well established supply chains and are well placed to convene and agree standards. However, there is a risk that different dominant firms might each found different data sharing and measurement systems, without co-ordination a number of different supply chain standards and systems will emerge, decreasing interoperability and increasing cost as well as confusion.
Whilst data sharing agreements and governance systems have been developed to increase trust between partners, there will still need to be robust auditing standards of data fidelity and accuracy. The potential for fraud is still significant, not least if carbon is traded as a financial product but also to falsely influence other supply chain actors including consumers.
Whilst this paper was developed with a United Kingdom centric perspective, the food system is truly global, as is the need to secure the SDG’s. It is essential that standards for data sharing to drive decarbonization are global.
Conclusion
The food system needs to decarbonise. Here we posit that digitalisation will be a key enabler of positive change, where the flow of standardised emissions data across complex supply chains provides accurate, trustworthy measurements of GHGEs and decarbonisation costs. This underpins decision support, responsive action and socially responsible business development, especially the delivery of the SDGs. In particular, more frictionless data flow enables supply chains to aggregate scope 3 emission costs with assessment that enable deep cross-sector rather than shallow and isolated, sometimes piecemeal, decarbonisation strategies to be adopted. Comprehensive digitalisation of the food system poses complex socio-economic-technical challenge that requires enhanced collaboration between industry, supply-chain actors, consumers, regulators, and governments. Here we consider that enhanced, multi-actor governance systems such as trust frameworks can facilitate trusted exchange of standardised emissions data and could play a pivotal role among other market and policy incentives in developing decarbonisation pathways and the delivery of net zero. The ethical and technical aspects of transparency and trust that arise will need to be addressed by novel, responsible, and targeted technologies that protect personal and organisational privacy whilst allowing data to be shared that enables informed and evidence-based decision making. The development of these technologies and associated trust frameworks in the context of decarbonisation pathways for food systems should be explored further.
Data availability statement
The original contributions presented in the study are included in the article/supplementary material, further inquiries can be directed to the corresponding author.
Author contributions
SP prepared the draft supported by LM and SB, all other authors contributed to the research and reviewed the manuscript. All authors contributed to the article and approved the submitted version.
Funding
This paper presents work funded by the Food Standards Agency (Project Ref FS301083) and the EPSRC Internet of Food Things DE Network (EP/R045127/1).
Conflict of interest
The authors declare that the research was conducted in the absence of any commercial or financial relationships that could be construed as a potential conflict of interest.
Publisher’s note
All claims expressed in this article are solely those of the authors and do not necessarily represent those of their affiliated organizations, or those of the publisher, the editors and the reviewers. Any product that may be evaluated in this article, or claim that may be made by its manufacturer, is not guaranteed or endorsed by the publisher.
References
Abbott, K. W., Levi-Faur, D., and Snidal, D. (2017). Enriching the RIT framework. Ann. Am. Acad. Politic. Soc. Sci. 670, 280–288. doi: 10.1177/0002716217694593
Bataille, C., Waisman, H., Colombier, M., Segafredo, L., and Williams, J. (2016). The deep decarbonization pathways project (DDPP): insights and emerging issues. Clim. Pol. 16, S1–S6. doi: 10.1080/14693062.2016.1179620
Bendovschi, A. (2015). Cyber-attacks-trends, patterns and security countermeasures. Proc. Econ. Finance 28, 24–31. doi: 10.1016/S2212-5671(15)01077-1
Brewer, S., Pearson, S., Maull, R., Godsiff, P., Frey, J. G., Zisman, A., et al. (2021). A trust framework for digital food systems. Nat. Food 2, 543–545. doi: 10.1038/s43016-021-00346-1
Carrero, I., Valor, C., Díaz, E., and Labajo, V. (2021). Designed to be noticed: a reconceptualization of carbon food labels as warning labels. Sustainability 13:1581. doi: 10.3390/su13031581
Dooley, D. M., Griffiths, E. J., Gosal, G. S., Buttigieg, P. L., Hoehndorf, R., Lange, M. C., et al. (2018). Food on: a harmonized food ontology to increase global food traceability, quality control and data integration. NPJ Sci. Food 2, 1–10. doi: 10.1038/s41538-018-0032-6
Durrant, A., Markovic, M., Matthews, D., May, D., Enright, J., and Leontidis, G. (2022). The role of cross-silo federated learning in facilitating data sharing in the agri-food sector. Comput. Electron. Agric. 193:106648. doi: 10.1016/j.compag.2021.106648
Durrant, A., Markovic, M., Matthews, D., May, D., Leontidis, G., and Enright, J. (2021). How might technology rise to the challenge of data sharing in agri-food? Glob. Food Sec. 28:100493. doi: 10.1016/j.gfs.2021.100493
Dwork, C., and Roth, A. (2014). The algorithmic foundations of differential privacy. Found. Trends Theor. Comput. Sci. 9, 211–407. doi: 10.1561/0400000042
Erickson, P., Kartha, S., Lazarus, M., and Tempest, K. (2015). Assessing carbon lock-in. Environ. Res. Lett. 10:084023. doi: 10.1088/1748-9326/10/8/084023
Fankhauser, S., Smith, S. M., Allen, M., Axelsson, K., Hale, T., Hepburn, C., et al. (2022). The meaning of net zero and how to get it right. Nat. Clim. Chang. 12, 15–21. doi: 10.1038/s41558-021-01245-w
Fernandez, A. (2021). A deeper dive into interoperability: exploring the flow of data in the seafood supply chain. Food Safety Mag. Available at: https://www.food-safety.com/articles/7237-a-deeper-dive-into-interoperability-exploring-the-flow-of-data-in-the-seafood-supply-chain
Gadema, Z., and Oglethorpe, D. (2011). The use and usefulness of carbon labelling food: a policy perspective from a survey of UK supermarket shoppers. Food Policy 36, 815–822. doi: 10.1016/j.foodpol.2011.08.001
Geels, F. W., Sovacool, B. K., Schwanen, T., and Sorrell, S. (2017). Sociotechnical transitions for deep decarbonization. Science 357, 1242–1244. doi: 10.1126/science.aao3760
Gentry, C. (2009). Fully homomorphic encryption using ideal lattices. In Proceedings of the forty-first annual ACM symposium on theory of computing, Bethesda, MD, USA, 31 May–2 June, 2009; pp. 169–178.
GHG Protocol,. (2011). Corporate Value Chain (Scope 3) Accounting and Reporting Standard. World Resources Institute and World Business Council for Sustainable Development, Washington, DC.
Grubb, M., Hourcade, J. C., and Neuhoff, K. (2014). Planetary Economics: Energy, Climate Change and the Three Domains of Sustainable Development. Routledge Earthscan, Abingdon, UK and New York, NY, USA, p. 520.
GS1 (2019). Foundation for Fish, Seafood and Aquaculture Traceability Guideline. Available at: https://www.gs1.org/sites/default/files/docs/traceability/GS1_Foundation_for_Fish_Seafood_Aquaculture_Traceability_Guideline.pdf (Accessed July 4, 2022).
Hardt, M. J., Flett, K., and Howell, C. J. (2017). Current barriers to large-scale interoperability of traceability technology in the seafood sector. J. Food Sci. 82, A3–A12. doi: 10.1111/1750-3841.13796
Heiler, S. (1995). Semantic interoperability. ACM Comput. Surv. 27, 271–273. doi: 10.1145/210376.210392
Hildingsson, R., Kronsell, A., and Khan, J. (2018). The green state and industrial decarbonisation. Environ. Politic. 28, 909–928. doi: 10.1080/09644016.2018.1488484. doi: 10.1016/j.jclepro.2012.09.035
Kowalska, A., and Manning, L. (2022). Food safety governance and guardianship: the role of the private sector in addressing the EU ethylene oxide incident. Foods 11:204. doi: 10.3390/foods11020204
Krebs, J. (2004). Establishing a single, independent food standards agency: the United Kingdom’ s experience. Food Drug Law J. 59, 387–397.
Manning, L. (2019). Food defence: refining the taxonomy of food defence threats. Trends Food Sci. Technol. 85, 107–115. doi: 10.1016/j.tifs.2019.01.008
Manning, L. (2020). Moving from a compliance-based to an integrity based organizational climate in the food industry. Compr. Rev. Food Sci. Food Saf. 19, 995–1017. doi: 10.1111/1541-4337.12548
Manning, L., Brewer, S., Craigon, P. J., Frey, J., Gutierrez, A., Jacobs, N., et al. (2022). Artificial intelligence and ethics within the food sector: developing a common language for technology adoption across the supply chain. Trends Food Sci. Technol. 125, 33–42. doi: 10.1016/j.tifs.2022.04.025
McMahan, B., Moore, E., Ramage, D., Hampson, S., and y Arcas, B. A. (2017). “Communication-efficient learning of deep networks from decentralized data” in Artificial Intelligence and Statistics (mlr press), 1273–1282.
Milne, R., Sorbie, A., and Dixon-Woods, M. (2021). What can data trusts for health research learn from participatory governance in biobanks? J. Med. Ethics 48, medethics-2020-107020–medethics-2020-107328. doi: 10.1136/medethics-2020-107020
National Academies of Sciences, Engineering, and Medicine (NASEM) (2020). Chapter 2. Taking a broad look at the food system. Innovations in the food system: exploring the future of food: Proceedings of a Workshop. Washington, DC: The National Academies Press
O’Reilly, C. A. III, and Tushman, M. L. (2013). Organizational ambidexterity: past, present, and future. Acad. Manag. Perspect. 27, 324–338. doi: 10.5465/amp.2013.0025
Onoufriou, G., Mayfield, P., and Leontidis, G. (2021). Fully homomorphically encrypted deep learning as a service. Mach. Learn. Knowl. Extract. 3, 819–834. doi: 10.3390/make3040041
Ostrom, E., (1990). Governing the Commons: The Evolution of Institutions for Collective Action. Cambridge University Press, Cambridge.
Poore, J., and Nemecek, T. (2018). Reducing food’s environmental impacts through producers and consumers. Science 360, 987–992. doi: 10.1126/science.aaq0216
Rogelj, J., Shindell, D., Jiang, K., Fifita, S., Forster, P., Ginzburg, V., et al., (2018). Mitigation pathways compatible with 1.5 C in the context of sustainable development. In global warming of 1.5 C. Intergovernmental panel on climate change. 93–174, Available at: https://www.ipcc.ch/site/assets/uploads/sites/2/2019/05/SR15_Chapter2_Low_Res.pdf
Rondoni, A., and Grasso, S. (2021). Consumers behaviour towards carbon footprint labels on food: a review of the literature and discussion of industry implications. J. Clean. Prod. 301:127031. doi: 10.1016/j.jclepro.2021.127031
Saberi, S., Kouhizadeh, M., Sarkis, J., and Shen, L. (2019). Blockchain technology and its relationships to sustainable supply chain management. Int. J. Prod. Res. 57, 2117–2135. doi: 10.1080/00207543.2018.1533261
Sadowski, J., Viljoen, S., and Whittaker, M. (2021). Everyone should decide how their digital data are used—not just tech companies. Nature 595, 169–171. doi: 10.1038/d41586-021-01812-3
Sarkis, J., Zhu, Q., and Lai, K. H. (2011). An organizational theoretic review of green supply chain management literature. Int. J. Prod. Econ. 130, 1–15. doi: 10.1016/j.ijpe.2010.11.010
Schulman, D. J., Bateman, A. H., and Greene, S. (2021). Supply chains (scope 3) toward sustainable food systems: an analysis of food & beverage processing corporate greenhouse gas emissions disclosure. Cleaner Prod. Lett. 1:100002. doi: 10.1016/j.clpl.2021.100002
Soon, J. M., Manning, L., and Smith, R. (2019). Advancing understanding of pinch-points and crime prevention in the food supply chain. Crime Preven. Commun. Saf. 21, 42–60. doi: 10.1057/s41300-019-00059-5
Sovacool, B. K., Bazilian, M., Griffiths, S., Kim, J., Foley, A., and Rooney, D. (2021). Decarbonizing the food and beverages industry: a critical and systematic review of developments, sociotechnical systems and policy options. Renew. Sust. Energ. Rev. 143:110856. doi: 10.1016/j.rser.2021.110856
Tan, M. Q. B., Tan, R. B. H., and Khoo, H. H. (2014). Prospects of carbon labelling–a life cycle point of view. J. Clean. Prod. 72, 76–88. doi: 10.1016/j.jclepro.2012.09.035
Tesco plc, (2021). Tesco strengthens environmental standards for its growers with adoption of global LEAF Marque Standard. September 30, 2021. Available at: https://www.tescoplc.com/news/2021/tesco-strengthens-environmental-standards-for-its-growers-with-adoption-of-global-leaf-marque-standard/ (Accessed April 14, 2022).
Verbruggen, P. (2017) Hybridization of food governance: an analytical framework, Hybridization of Food Governance, (Intersentia), 1–28.
Wolff, Jonathan (Blavatnik School of Government, U. of O). (2018). Risk and the Regulation of New Technologies. p. 23. Available at: https://jonathanwolff.files.wordpress.com/2018/09/wolff-risk-and-the-regulation-of-new-technologies
Keywords: digital systems, trust framework, data exchange, governance, net zero
Citation: Pearson S, Brewer S, Manning L, Bidaut L, Onoufriou G, Durrant A, Leontidis G, Jabbour C, Zisman A, Parr G, Frey J and Maull R (2023) Decarbonising our food systems: contextualising digitalisation for net zero. Front. Sustain. Food Syst. 7:1094299. doi: 10.3389/fsufs.2023.1094299
Edited by:
Miguel Angel Taboada, University of Buenos Aires, ArgentinaReviewed by:
Sonal Choudhary, University of York, United KingdomCopyright © 2023 Pearson, Brewer, Manning, Bidaut, Onoufriou, Durrant, Leontidis, Jabbour, Zisman, Parr, Frey and Maull. This is an open-access article distributed under the terms of the Creative Commons Attribution License (CC BY). The use, distribution or reproduction in other forums is permitted, provided the original author(s) and the copyright owner(s) are credited and that the original publication in this journal is cited, in accordance with accepted academic practice. No use, distribution or reproduction is permitted which does not comply with these terms.
*Correspondence: Simon Pearson, U1BlYXJzb25AbGluY29sbi5hYy51aw==