- 1Dabie Mountain Economic and Social Development Research Center, Xinyang Normal University, Xinyang, China
- 2Business School, Xinyang Normal University, Xinyang, China
Digital villages construction and agricultural green development are key factors in promoting the high-quality development of agriculture in China. This paper takes the period from 2012 to 2023 as the sample observation period and employs a fixed-effects model to empirically analyze the impact of digital villages construction on agricultural green productivity in 30 provinces of China, as well as the underlying mechanisms. The results show that: (1) From 2012 to 2023, the level of agricultural green production efficiency in China has been steadily increasing, with technological progress being the core driving force for this growth; (2) The level of digital villages construction significantly enhances agricultural green production efficiency. After a series of robustness and endogeneity tests, the results remain valid; (3) Mechanism tests reveal that the level of rural human capital plays a mediating role in this process; (4) The spatial spillover effect test indicates that digital villages construction has a positive spatial spillover effect on agricultural green production efficiency.
1 Introduction
In recent years, China's agricultural development has achieved remarkable results that have attracted global attention, with continuous growth in agricultural output and significant improvement in agricultural productivity. However, extensive agricultural production methods have caused irreversible damage to resources and the environment, such as the decline of soil fertility, destruction of arable land resources, increased pressure on ecological environment protection, and a decline in the carrying capacity of agricultural resources and the environment. The report of the 19th National Congress of the Communist Party of China clearly proposed to promote green development and strengthen actions to control agricultural non-point source pollution. Agricultural production pollution has already had a serious impact on China's ecological environment. How to achieve a balance between agricultural production benefits and resource and environmental protection has become a new topic for the high-quality development of China's agriculture. Under the impact of the new round of scientific and technological revolution and industrial transformation, new-generation information technologies have spread to rural areas, and a digital wave has swept across rural regions. Since the “Digital villages Strategy” was proposed in 2018, China's rural digital infrastructure has gradually improved, and the level of digitalization in agriculture and rural areas has been significantly enhanced. Digital villages construction has become an effective way to solve the problems of agricultural and rural development. Relying on the organic combination of the new paradigm of the digital economy and traditional production organization methods, it has pushed agriculture toward a more environmentally friendly, efficient, and sustainable direction, and has had a revolutionary impact on agricultural green development. Based on this, this paper aims to explore the impact of digital villages construction on agricultural green production efficiency and its mechanisms of action, and to discuss how to fully utilize digital villages construction to improve agricultural green production efficiency and the level of agricultural green production.
At present, the potential of traditional agricultural production factors is exhausted, and environmental resources are increasingly constrained. Against this backdrop, research on agricultural green production efficiency in the academic community is comprehensive and detailed, focusing mainly on the definition of concepts, measurement methods, spatial and temporal distribution, and driving factors. Existing studies have primarily used Data Envelopment Analysis (DEA) and Stochastic Frontier Analysis (SFA; Liu et al., 2022; Song and Chen, 2019) to measure agricultural green production efficiency and depict its spatiotemporal evolution characteristics. Most temporal studies have been conducted at the national level (Guo et al., 2020), but some scholars have also focused on key economic development zones, such as the Yangtze River Economic Belt (Wang et al., 2020), the Silk Road Economic Belt (Liu and Xin, 2019), the Yellow River Basin (Lu et al., 2020), and major grain-producing areas (Ye et al., 2023). On this basis, scholars have empirically examined the impact of factors such as environmental regulation (Sun, 2022), fiscal subsidies (Wang et al., 2022), rural finance (Qin et al., 2024), industrial structure (Lei et al., 2023), and agricultural production agglomeration (Luo et al., 2023) on agricultural green production efficiency. As digital technology booms, concepts like digital village building, digital agriculture, and rural informatization have emerged in academia, and are analyzed alongside agricultural green production efficiency to explore the link between digital tech and green agriculture. Digital agriculture centers on digital upgrades in agricultural production, aiming to boost the tech level and efficiency of the production process. Rural informatization emphasizes the construction of information infrastructure and the dissemination of information, focusing on addressing the difficulties in information acquisition and circulation in rural areas. More comprehensive than the above two, digital village building is a holistic development strategy. It covers multiple rural domains including the economy, society, culture, and governance. In addition to encompassing digital agriculture and rural informatization, it also involves the deep integration of digital technology with rural life, public services, and grassroots governance. Existing research on the relationship be-tween digital technology and agricultural green production efficiency is inconclusive. Cai and Han (2024) show that digital village construction boosts agricultural green total factor productivity (TFP) by promoting large-scale farm operations and accelerating agricultural informatization. Tang and Chen (2022), however, find an “inverted U-shaped” relationship, where digital technology initially aids but later hinders agricultural green development. Sun and Zhong (2025) explores the mechanisms and spatial spillover effects of the rural digital economy on agricultural green TFP, while Lu et al. (2024) indicate that digital village construction enhances agricultural green TFP through technological progress. These discrepancies reveal the need for more in-depth research on how specific pathways of digital village construction interact with local resources to impact agricultural green production efficiency. This paper aims to fill this gap by systematically examining the direct, indirect, and spatial spillover effects of digital village construction on agricultural green production efficiency, using a comprehensive theoretical and empirical approach.
Based on the review of the literature, it can be seen that there are abundant research achievements on the relationship between digital technology and agricultural green production efficiency, which provide rich references for this paper. However, research focusing on digital villages construction and its relationship with agricultural green production efficiency still needs a broader perspective. Moreover, the advancement of agricultural digitalization and green development cannot be separated from the support of rural human capital. Yet, few studies have explored the mechanism through which digital villages construction affects agricultural green production efficiency by considering rural human capital as a mediating variable. Building on the existing research findings, this paper constructs an index system for measuring digital villages construction and agricultural green production efficiency, introduces rural human capital as a mediating variable, and employs the Spatial Durbin Model to explore the spatial spillover effects.
2 Theoretical analysis and research hypotheses
2.1 The direct impact of digital villages construction on agricultural green production efficiency
Digital villages construction takes the renewal of rural infrastructure as its foundation and the development of digital agriculture as its starting point (Liu and Liu, 2024). It relies on the digital economy and uses modern information technology as a carrier to comprehensively advance the modernization of agriculture and rural areas and the high-quality development of agriculture (Chunfang et al., 2024). As the digital infrastructure in rural areas continues to improve, digital technology empowers rural development to achieve a “qualitative” leap. It has become an important way to solve the problems of extensive agricultural production methods, fragmented rural farmland, and scattered household operations under the background of the “large country with small farms” agricultural situation and national conditions. It also provides new opportunities for the green development of agriculture.
From the perspective of agricultural technological progress, the promotion of green agricultural development by digital village construction can be explained by Technology Diffusion Theory and New Institutional Economics. Digital village construction, leveraging big data platforms, aligns with Technology Diffusion Theory by using digital infra-structure to accelerate the spread of green agricultural technologies. It facilitates the collection, organization, and analysis of agricultural data (Zhao et al., 2024; Wang and Mei, 2024), and through data-driven R&D of green agricultural technologies in collaboration with universities and research institutions, it improves the matching accuracy, optimizes the allocation of production factors, and enhances the effectiveness of agricultural chemical application (Balafoutis et al., 2017), thereby reducing environmental pollution. In terms of institutional innovation, digital information technology networks promote collaboration and development in agricultural research. According to New Institutional Economics, digital-enabled collaborations are considered institutional innovations that overcome the fragmentation of traditional research (Ballantyne et al., 2010). Digital information technology networks enable collaborative and innovative development in agricultural research, overcoming the fragmentation of research efforts and accelerating the rate of technological innovation and transfer. This not only improves the utilization rate of traditional energy sources but also optimizes the input structure of agricultural factors, reducing agricultural carbon emissions (Zhu et al., 2022; Li et al., 2023). The substitution of traditional agricultural production technologies with digital ones, a direct manifestation of technology diffusion, is supported by digital village construction. This substitution, enabled by digital village construction, achieves the rational use of agricultural resources and enhances agricultural green production efficiency (Hajiyeva et al., 2023). Policy-wise, the Chinese government's “Digital Village Demonstration Project” has provided financial incentives and regulatory support, further promoting the diffusion of digital technologies in agriculture.
From the perspective of changes in agricultural production methods, the digital economy, as a new type of productive force, has changed traditional agricultural production methods through its integration with agricultural production methods, promoting the development of agricultural production toward refinement, automation, and informatization, and providing new momentum for green agricultural development. Before agricultural production, the combination of digital technology and machinery empowers the intelligentization of agricultural production equipment, helping agricultural producers to more accurately understand soil, climate, and crop production conditions, and make more scientific planting decisions, reducing negative externalities in the agricultural production process (Zhang et al., 2023). During agricultural production, the input of factors is closely related to output, and the development of green agriculture must focus on pollution emissions from the use of chemicals and the sustainable use of agricultural resources (Wang, 2017). The integration of digital technology promotes the precise development of agricultural production models, assisting producers in accurately analyzing resource conditions in different areas and suitable conditions for agricultural production, and determining the optimal ratio of fertilizers and pesticides (Robinson et al., 2022), providing new ideas for alleviating agricultural non-point source pollution. Based on this, Hypothesis 1 is proposed.
Hypothesis 1. The indirect impact of digital villages construction on green agricultural rural productivity.
2.2 The indirect impact of digital villages construction on agricultural green production efficiency
Farmers are the principal agents in agricultural production, and their capabilities are closely linked to the development of green agriculture. Digital village construction enhances rural human capital through multiple pathways, indirectly improving agricultural green production efficiency. Firstly, digital village construction leverages internet platforms to break down information barriers, enabling farmers to access modern agricultural management knowledge. According to the Technology Acceptance Model (TAM), the accessibility of information and the usability of platforms significantly influence farmers' willingness to engage with new knowledge. The establishment of online agricultural knowledge databases, supported by digital infrastructure, exposes farmers to advanced cultivation techniques, strengthens environmental awareness, and increases openness to adopting innovative technologies. High-skilled agricultural producers are more likely to recognize the drawbacks of traditional practices. They not only enhance their understanding of agricultural environmental protection and sustainable development but also facilitate the adoption of new technologies to transform conventional production methods (Hu et al., 2023), such as low-carbon agricultural technologies and eco-friendly fertilizer applications. Secondly, online education platforms under digital village construction provide flexible learning opportunities through remote training and distance education. Farmers with higher educational attainment typically possess stronger learning and continuous skill-development capabilities, enabling them to more proficiently adopt, master, and apply digital agricultural technologies. This strengthens the impact of digital village initiatives on green agricultural productivity. Thirdly, improvements in rural human capital further drive technological innovation and transformation, promoting green agricultural efficiency (Lu et al., 2024; Ma et al., 2023). Aligned with endogenous growth theory, a more educated rural workforce accelerates technological progress. For instance, in Sichuan's tea-growing regions, digitally skilled farmers have developed innovative processing techniques that reduced energy consumption in tea drying processes. Educated farmers are also more likely to experiment with adaptive cultivation methods tailored to local conditions. Fourthly, the enhancement of individual capabilities enables farmers to better interact with advanced agricultural machinery (Li et al., 2024). Given that most digital technologies transform production through human-machine interfaces (Yang et al., 2019), the digital literacy of agricultural practitioners becomes critical. According to technology diffusion theory, individuals with higher capabilities are more likely to be early adopters of innovations. Thus, farmers with advanced education can operate machinery more precisely, improving agricultural accuracy and reducing resource waste (Padhiary, 2025). Additionally, improved education levels equip farmers with data analysis and management skills, allowing them to optimize production processes using internet-based decision-support systems. In summary, digital village construction and rural human capital influence agricultural green production efficiency through multiple pathways, under-scoring the importance of investing in rural digital infrastructure and human capital development. Based on this, Hypothesis 2 is proposed.
Hypothesis 2. Digital villages construction affects agricultural green production efficiency by enhancing rural human capital.
2.3 The spatial spillover effect of digital villages construction on agricultural green production efficiency
Geographical location endows agricultural resources with inherent spatial spillover characteristics. Driven by the regional coordinated development strategy, the cross-regional spillover effects of agricultural TFP have become more evident (Huang et al., 2024). Digital village construction, empowered by digital technology, transcends traditional geographical limits of economic activities. It significantly impacts not only local green agricultural productivity but also that of neighboring regions. Based on the knowledge spillover theory, this spatial influence is mainly shown through two channels: inter-regional technology diffusion and policy learning. In terms of inter-regional technology diffusion, regional economic integration speeds up the spread of digital technology, strengthening the spatial agglomeration of digital village construction. The proximity of economic activities is conducive to the dissemination of both explicit knowledge, such as digital agricultural technology standards, and implicit knowledge, like smart agriculture practical experience. In addition, agricultural technological innovation is transferred across regions through university-enterprise and university-local cooperation models, with universities serving as a bridge. This enables agricultural R&D results to benefit surrounding areas and promotes agricultural production efficiency there via agricultural technological progress. Policy learning, driven by the shareability of the green production concept, is another key channel (Zou et al., 2024). When a region achieves remarkable results in digital village construction and green agricultural development, it sets a benchmark for neighboring areas. Local governments often study and replicate successful policy models, such as subsidy mechanisms for digital infrastructure construction or regulatory policies for green production. The policy learning process, guided by the principle of knowledge spillover, optimizes resource allocation between regions and accelerates the spread of green production concepts. Ultimately, it promotes the overall improvement of agricultural green productivity. In summary, agricultural green development is closely linked not only to local rural construction levels but also to digital village construction in neighboring areas. These spillover effects, rooted in knowledge spillover mechanisms, highlight the importance of regional cooperation for promoting agricultural green development. Based on this, Hypothesis 3 is proposed. Based on this, Hypothesis 3 is proposed.
Hypothesis 3. Digital villages construction has a positive spatial spillover effect on agricultural green production efficiency.
3 Research method and data resource
3.1 Model construction
3.1.1 Benchmark regression model
This paper constructs an individual fixed effects panel model to examine the relationship between digital villages construction and agricultural green production efficiency. The baseline regression model is shown as follows:
In this context, AGP represents the independent variable, which is agricultural green production efficiency, DIV represents the dependent variable, which is digital villages construction, Controls represent a series of control variables, and εit represents the random disturbance term.
3.1.2 Mediating effect model
This paper introduces rural human capital as a mediating variable to explore the mechanism of action between the two. Based on the baseline regression model, the following regression model is constructed:
In this context, Rhc represents the moderating variable of rural human capital, which serves as a mediating variable. If both β1 and α2 are significant, then the construction of digital villages will impact agricultural green production efficiency through rural human capital.
3.1.3 Spatial Durbin model
This paper establishes the following spatial Durbin econometric model to explore the spatial spillover effects between digital villages construction and agricultural green production efficiency:
In this context, ρ is the spatial autoregressive coefficient, γ2 represents the spatial efficiency of digital villages construction on agricultural green production efficiency, and Wij is the spatial weight matrix.
3.2 Variable description
3.2.1 Core explanatory variable
The explanatory variable in this paper is the construction of digital villages. The entropy method is used to measure it by establishing an indicator system based on four dimensions: digital construction of villages, digital agriculture in villages, digital life in villages, and the digital development environment of villages. In conjunction with the “Guidelines for the Construction of Digital Village Standard System” and the availability and comprehensiveness of data, the following evaluation indicator system is constructed (Table 1).
3.2.2 Explained variable
The explained variable is agricultural green production efficiency. To avoid biases of traditional DEA models, a non-desired super-efficiency SBM model is adopted. The SBM model, based on slack variables, accurately assesses efficiency as it considers both desirable and non-desirable outputs in agricultural production, contrary to traditional models. The GML index measures TFP change over time. It excels by accounting for production frontier shifts, capturing how technology, efficiency, and scale effects impact the overall efficiency change. Combining SBM and GML allows for precise current efficiency measurement and tracking of its dynamic evolution, aptly handling agricultural green production's complexity. Based on relevant literature (Yu et al., 2022), the model is constructed as follows:
In this context, ρ represents the efficiency value of the decision-making unit, where a value greater than or equal to 1 indicates that the decision-making unit is efficient. To further reflect the dynamic changes in production efficiency, the GML index is used to evaluate the dynamic changes in agricultural green production efficiency, expressed as follows:
In the equation, x represents input, y represents expected output, b represents non-expected output, and t indicates the time period. GML represents the index of changes in agricultural green production efficiency. If it is >1, it indicates an improvement in agricultural green production efficiency from time t to t+1; otherwise, it indicates a decline. Referring to relevant literature (Wang et al., 2023), the GML index is further decomposed into the technical efficiency (EC) index and the technical progress (TC) index. When TC > 1, it indicates technological progress; when EC > 1, it indicates frontier technological progress; otherwise, it indicates the opposite (Table 2).
3.2.3 Mediating variable
The construction of digital villages areas promotes the enhancement of rural human capital by establishing digital communication platforms. Educated agricultural laborers are more likely to adopt green agricultural technologies and can more flexibly apply digital technologies in agricultural production activities. Therefore, rural human capital (Rhc) is used as a mediating variable, referencing relevant literature (Han et al., 2018; Ye and Ma, 2020), and is represented by the average years of education of rural farmers. The specific calculation formula is as follows: Average years of education of rural farmers = (Number of rural illiterates * 1 + Number of rural primary school graduates * 6 + Number of rural junior high school graduates * 9 + Number of rural high school and vocational school graduates * 12 + Number of rural college and above graduates * 16)/Total population aged over 6.
3.2.4 Control variables
To avoid omitted variable bias, this paper selects the following control variables based on existing research findings. These mainly include: ① Agricultural planting structure (Far), represented by the proportion of grain sown area to the total sown area of crops; ② Area of crops affected by disasters (Dis), represented by the proportion of disaster-affected crop area to the total sown area of crops; ③ Number of agricultural science and technology personnel (Ars), represented by the product of the proportion of total agricultural, forestry, animal husbandry, and fishery output value to regional GDP and the number of R&D personnel in large-scale industrial enterprises; ④ Level of industrial development (Ind), represented by the proportion of industrial added value to regional GDP; ⑤ Agricultural structure (Stf), represented by the proportion of total agricultural output value to the total output value of agriculture, forestry, animal husbandry, and fishery; ⑥ Financial support for agriculture (Bud), represented by the proportion of local fiscal expenditure on agricultural, forestry, and water affairs to local fiscal general budget expenditure; ⑦ Environmental protection intensity (Epi), indicated by the proportion of local fiscal environmental protection expenditure in local fiscal general budget expenditure.
3.3 Data sources and descriptive statistics of variables
This paper selects panel data from 30 provinces in China (excluding Hong Kong, Macau, and Taiwan) from 2012 to 2023 as the research sample. The relevant data is sourced from various years' “China Statistical Yearbook,” “China Rural Statistical Year-book,” “China Urban and Rural Statistical Yearbook,” “Peking University Digital Inclusive Finance Index,” “China Taobao Village Research Report,” National Bureau of Statistics, CNKI database, and Alibaba Research Institute, among others. Some missing data is supplemented using linear interpolation, as shown in Table 3.
4 Results and analysis
4.1 The spatiotemporal evolution characteristics of agricultural green production efficiency
4.1.1 Static analysis of agricultural green production efficiency
Based on the development of agricultural green production efficiency in various provinces and the natural breakpoint method of ArcGIS, this paper classifies the agricultural green production efficiency and draws spatial distribution maps with 2012, 2016, 2019, and 2023 as nodes, as shown in Figure 1. Overall, the agricultural green production efficiency in China shows a trend of increasing year by year. As of 2023, the agricultural green production efficiency in half of the regions is at a high level, indicating that in recent years, the state has attached great importance to the protection of agricultural resources and the environment, and the policy effects are obvious. Among them, although the growth rate in Northeast China has always led the country, it has the lowest growth rate during the sample observation period. The possible reason is that Northeast China is an important major grain-producing area and demonstration zone in China, with unique natural resource endowments and an industrial economic foundation. Therefore, it has a good foundation for agricultural green development. However, as a major industrial city in China, it is in a critical period of transformation, resulting in insufficient driving force for its subsequent development. The western and eastern regions are developing rapidly, indicating that under the impetus of the Belt and Road Initiative, the agricultural resource advantages of the western region have been brought into play. With the deepening of Sino-foreign cooperation and exchanges, the region has formed a characteristic industry with strong market competitiveness through the production of characteristic agricultural products. Relying on the strong inclination of agricultural policies, the eastern region takes innovation as the lead in the pilot experimental area. With the superposition of multiple advantages, the agricultural green production efficiency has been significantly improved. The growth trend in the central region is slightly lower than the national average annual growth rate. The possible reason is that the crop planting in this region is mainly rice planting. Under the influence of frequent natural disasters and the acceleration of industrialization in recent years, the region has a high resource input and serious environmental damage, resulting in its failure to keep up with the national average development level (Table 4).
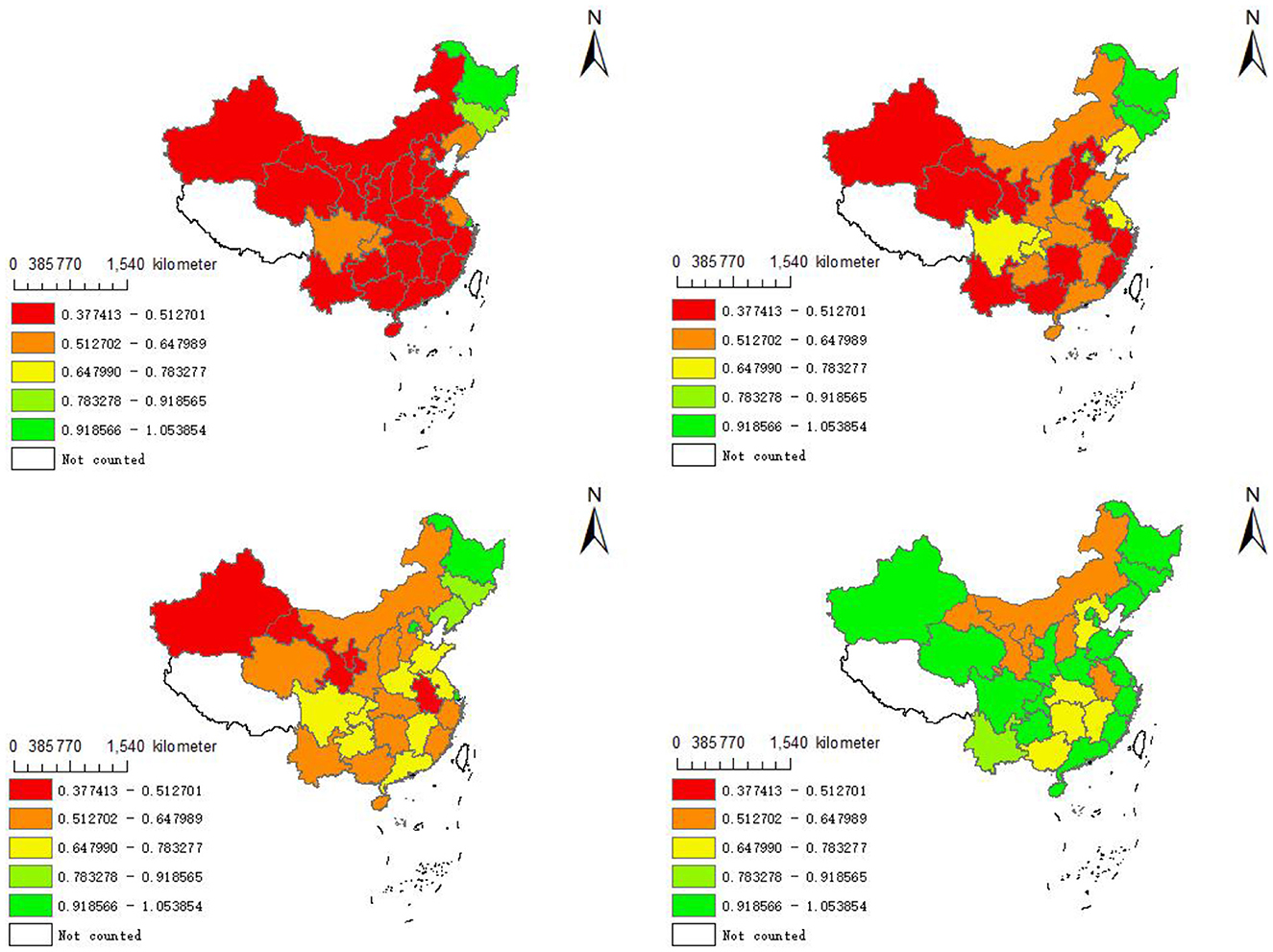
Figure 1. Visual representation of agricultural green production efficiency in various provinces and cities of China for specific years.
4.1.2 Dynamic analysis of agricultural green production efficiency
To highlight the trend of changes in agricultural green production efficiency over time, this paper further conducts a dynamic analysis of the efficiency values using the GML index. The calculation results are shown in Table 5. Overall, the annual changes in the GML index of agricultural green production efficiency fluctuate significantly, with an average annual growth rate of 5.8%. During the sample observation period, the GML index is >1, reaching a maximum value of 1.0976 in 2020–2021. This reflects that the concept of green development has taken root in people's minds and relevant measures have been highly effective, such as the promotion of organic agriculture, the adoption of green planting techniques, and strengthened supervision of agricultural chemical applications. The average annual growth rate of the EC index (technical efficiency) is 0.92%, fluctuating around 1.00 during the observation period. This indicates that the agricultural technical efficiency in China is not very stable and there is still much room for improvement. The TC index (technology progress) grew at an average annual rate of 5.26%, indicating an overall upward trend in agricultural technology during this paper period. After a gradual decline from 2012 to 2018, the TC index rebounded to a peak of 1.0828 in 2018–2019, likely due to increased agricultural R&D investment and digital technology adoption in major producing regions. However, its growth slowed markedly in 2019–2020, with a slight year-on-year decrease. This was not just because of the pandemic, but also due to region-specific disruptions and policy adjustments. For example, provinces highly dependent on migrant agricultural technicians faced significant slowdowns as lockdowns restricted labor mobility, hindering on-site technical guidance and innovation diffusion. In contrast, regions with well-developed digital extension systems maintained relatively stable technological progress by adopting online training and remote monitoring. It is easy to see that during the sample observation period, the improvement of China's agricultural green production efficiency mainly benefited from technological progress, reflecting that China's significant investment in promoting the research and development of agricultural green production technologies, as well as measures to enhance the transformation and application of agricultural scientific research achievements, have been effective.
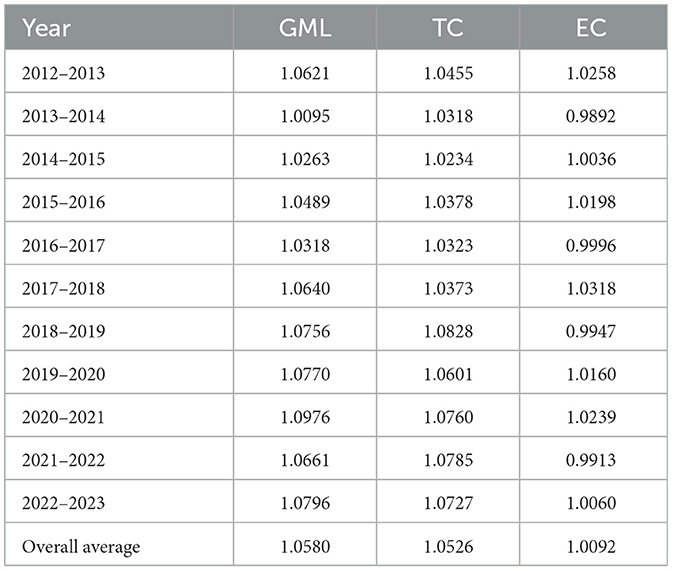
Table 5. The GML index of green agricultural production efficiency in China from 2012 to 2023 and its decomposition.
4.2 Benchmark regression analysis
Based on the robustness of the benchmark regression results and to examine whether gradually adding control variables under different settings would lead to differences in the results, this paper uses the step-by-step regression estimation method to conduct regression analysis on the full—sample data. The results are shown in Table 6. As can be seen from Model (1), without adding any control variables, the regression coefficient of digital villages construction is significant at the 1% level. The same is true after gradually adding control variables, which further proves that whether control variables are added or not, digital villages construction helps to improve agricultural green production efficiency, and Hypothesis 1 is preliminarily tested.
The degree of agricultural disaster is significantly negative at the 1% level. The possible reason is that disasters damage the agricultural production environment, resulting in lower-than-expected immediate agricultural output and affecting long-term agricultural production activities. A series of crop and economic losses lead farmers to adopt non-environmental-friendly production behaviors to increase crop yields, such as increasing the use of chemical fertilizers and pesticides, thus having a negative impact on agricultural green production efficiency. The agricultural planting structure has a significant negative impact on agricultural green production efficiency. The possible reason is that food crops generate a large amount of environmental non-point source pollution and agricultural carbon emissions during the production process. At the same time, the scale of agricultural planting is insufficient, and agricultural production materials cannot be effectively allocated and utilized, thus reducing agricultural green production efficiency. The agricultural structure is always significantly positive at the 1% level. According to the “structural bonus hypothesis,” the adjustment of the agricultural structure directly affects agricultural green production efficiency. With the improvement of agricultural technology levels, agriculture will shift from low-efficiency sectors to high-efficiency sectors, thereby achieving the goal of improving agricultural green production efficiency. The number of agricultural science and technology practitioners also has a significantly positive impact on agricultural green production efficiency, indicating that agricultural science and technology personnel effectively drive the improvement of agricultural green production efficiency by promoting the research and innovation of agricultural green technologies, facilitating the popularization and application guidance of production technologies, and spreading emerging scientific knowledge and technical experience. The impacts of the degree of industrialization and the intensity of financial support for agriculture on agricultural green production efficiency are not significant. This may be because industrialization can promote the development of agricultural mechanization to a certain extent, but since its development resources are mainly concentrated in industrial industries, resource allocation, and policy support, and not tilted toward the agricultural field, the impact is not significant. At present, financial funds for supporting agriculture are mainly used for subsidies for agricultural production materials. Although production conditions have improved to some extent, due to the relatively small investment in agricultural technology and green agriculture, the impact is also not significant. The insignificant negative coefficient of environmental protection investment may stem from structural imbalances in its allocation within the agricultural sector. This results in inefficient fund utilization, as some regions focus excessive funding on short-term visible hardware like sewage treatment plants and waste transfer stations, while underinvesting in R&D, application of green agricultural tech, and farmer environmental education. Consequently, environmental protection funds fail to directly boost agricultural green production efficiency.
4.3 Robustness and endogeneity tests
4.3.1 One percentage two-tailed trimming
To avoid biases in the regression results caused by extreme outliers in the original model, this paper conducts 1% two-tailed trimming on all variables and rebuilds the regression model. The results are shown in column (1) of Table 7, which are consistent with the previous regression results.
4.3.2 Adjust the research area
Considering that municipalities directly under the central government have a relatively high development level, a relatively complete digital village construction policy, and significantly better agricultural development and resource endowments than other provinces, which may lead to differences in the regression results. Therefore, this paper excludes the data of four cities, namely Beijing, Tianjin, Shanghai, and Chongqing, and rebuilds the regression model. As can be seen from column (2) of Table 7, the results are consistent with the previous regression results.
4.3.3 Replacing the robust standard error
The standard errors used in the previous sections are common ones. Considering that the disturbance terms of the same province in different years often show clustering phenomena, referring to relevant literature (Du et al., 2023), this paper chooses the province—level individual clustered robust standard error for re-estimation. As can be seen from column (3) of Table 7, the results are consistent with the previous regression results.
4.3.4 System generalized method of moments regression
To address the lagged effect of digital village construction on agricultural green production efficiency and potential reverse causality, this paper employs a dynamic panel model with system GMM. The core explanatory variable's lagged term is introduced, and the dynamic lags of other variables are controlled for to tackle endogeneity. In column (3) of Table 7, the AR(1) statistic is below 0.1, and the AR(2) statistic is above 0.1. The Hansen J-test p-value also exceeds 0.1, indicating no over-identification. This confirms the estimation's validity. The regression results align with prior findings, reinforcing the benchmark regression results' reliability.
4.3.5 Instrumental variable method
To address potential endogeneity from omitted variables, we conducted a re-test using an instrumental variable: the interaction between the 1984 telephone-per-100-people count and the prior-year national information-technology-service revenue. This choice was guided by two factors. First, fixed telephones reflect past telecom infrastructure development, influencing both subsequent internet technology adoption and digital village construction levels due to usage inertia. Second, past fixed telephone counts don't significantly directly impact current agricultural green production efficiency, meeting the relevance and exogeneity criteria for instrumental variables. Using a two-stage least squares (2SLS) regression, the results (see Column 5 in Table 7) remain consistent with our baseline findings. This robustness check confirms that our core conclusions are not driven by unobserved confounding factors.
4.4 Heterogeneity analysis
4.4.1 Regional heterogeneity
China has a vast territory, and the resource endowments of different regions vary. Therefore, the impact of digital villages construction on the development of agricultural green production efficiency varies among provinces. Thus, this paper divides the 30 provinces and municipalities in China into four regions: the East, the Central, the West, and the Northeast, based on economic and geographical distances, to further examine the impact of digital villages construction in each region on agricultural green production efficiency. The regression results are shown in Table 8. According to the regression results, the impact of digital villages construction on the agricultural green production efficiency in the four regions is significantly positive, indicating that digital villages construction is inclusive. The Northeast leverages its rich agricultural resources and suitable production conditions, marked by high mechanization and contiguous farmland, to create an ideal setting for digital tech adoption. This enables digital tools to optimize resource use efficiently and boosts agricultural green production efficiency, making digital village building highly impactful. The West, despite its vast land and sparse population, uses its untapped land resources and policy investments from the Belt and Road Initiative to enhance digital connectivity in remote areas and lower data acquisition costs for smart agriculture. The “East Data, West Calculation” project further strengthens digital infrastructure investment and reduces data costs. Its low population density suits large-scale, data-driven farming, where technologies like satellite monitoring and automated irrigation can maximize re-turns on digital infrastructure, offering ample room for efficiency gains. As China's core grain-producing region, the Center has concentrated farmland and a history of policy-backed agricultural modernization. The “Rural Revitalization” and “Central Rise” strategies have sped up the construction of high-standard farmland with integrated water conservancy and precision irrigation systems. These systems seamlessly integrate with digital crop monitoring and pest control tools, driving significant efficiency improvements through synergy between traditional agricultural intensity and new digital inputs. The East, with its developed economy and mature digital ecosystem, already enjoys high agricultural tech penetration. While its strong infrastructure supports digital village initiatives, the marginal effect of extra digital investment is less obvious compared to regions with lower initial tech adoption, reflecting a saturation effect in green production technologies.
4.4.2 Temporal heterogeneity
Specifically, referring to the differences in digital villages construction in the time dimension, this paper takes the year 2018, when the “No.1 Central Document” first proposed the “Implementation of the Digital Villages Strategy,” as a time node and divides the sample into two time periods for analysis. The results are shown in Table 9. The results indicate that the driving effect of digital villages construction on agricultural green production efficiency before 2018 was significantly less than that in 2018 and later. The reason for this change may be that after 2018, the key points of implementing the “Digital Villages Strategy” were further implemented. Subsequently, policy documents such as the “Outline of the Digital Villages Development Strategy,” the “Digital Agriculture and Rural Development Plan (2019–2025),” and the “Notice on Carrying out the National Digital villages Pilot Work” were successively introduced. The digital dividends released by the digital economy were rapidly expanded in rural areas. Therefore, digital villages construction has a more significant promoting effect on agricultural green production efficiency.
5 Analysis of mechanism of action and spatial spillover effects
5.1 Mediating effect test
As can be seen from the mediating effect test results in Table 10, there is a significant positive impact between digital villages and rural human capital, and the coefficient of rural human capital and the core explanatory variable is significantly positive, indicating that rural human capital plays a partial mediating role in the influencing process. Thus, Hypothesis 2 is preliminarily verified. Further explanation is that digital villages can not only enhance the digital literacy of rural residents and attract talents to return to their hometowns (Tim et al., 2021), but also drive the investment in emerging industries through agricultural digitization, attracting human capital to flow to rural areas, thereby jointly improving the level of rural human capital. At the same time, the digital platform provides agricultural producers with a platform for information acquisition and communication, breaking the information “island” (Jiang et al., 2022), enabling farmers to acquire a higher level of knowledge, implanting the concept of agricultural green development, and thus promoting farmers to have a higher learning ability and adaptability. Agricultural producers can make full use of the digital technology tools brought by digital village construction, be more proficient in dealing with emergencies in agricultural production, achieve rational and efficient use of resources, and promote digital village construction to better empower the improvement of agricultural green production efficiency.
To further test the robustness of the mediating effect, we used the Bootstrap method with 1,000 random samples and a 95% confidence interval. As Table 11 shows, the confidence interval doesn't include 0, indicating a significant indirect mediating effect of rural human capital in digital village construction. Comparing Tables 10, 11, both the three-step method and the Bootstrap method confirm the validity of our mediating effect model, so Hypothesis 2 is robust.
5.2 Spatial spillover effect test
5.2.1 Spatial autocorrelation test
Before empirically testing the spatial spillover effect, it is necessary to ensure that the explained variable (agricultural green production efficiency) has spatial autocorrelation. In this paper, an inverse distance spatial weight matrix is set, and a global Moran's I test is conducted on the agricultural green production efficiency of 30 provinces and municipalities in China from 2012 to 2023. The results are shown in Table 12. From the results, it can be seen that the spatial distribution of provincial-level agricultural green production efficiency in China is not randomly generated, but has certain spatial agglomeration characteristics. Therefore, spatial econometric methods can be selected for further analysis.
5.2.2 Selection of spatial econometric model
Further, the LM and Wald tests are used to select the spatial econometric model. As can be seen from Table 13, in the LM test, all four tests reject the null hypothesis, indicating that the selected samples in this paper have both spatial lag and spatial error effects, and it is initially judged that the spatial Durbin model should be adopted. In the Wald test, the statistic is still significant, the null hypothesis is rejected, and the degradation of the spatial Durbin model is rejected. Therefore, considering the test results and the goodness of fit of the model, this paper adopts the individual fixed spatial Durbin model.
5.2.3 Basic regression results
The adjacency matrix was further introduced to ensure the robustness of the results. The results are shown in Table 14. Under the two spatial matrices, the coefficients of the core explanatory variables are significantly positive at the 1% level, indicating that the construction of digital villages has a significant promoting effect on agricultural green production efficiency. At the same time, the spatial autocorrelation coefficient rho value is always significantly positive, indicating that agricultural green production efficiency has a spatial spillover effect.
Furthermore, the partial differential method was adopted to decompose the spatial effects into direct, indirect, and total effects. The results are presented in Table 15. The level of digital village construction is significantly positive under both matrices, indicating that the level of digital village construction in a province not only has a positive promoting effect on the level of agricultural green production efficiency in the province itself, but also promotes the growth of agricultural green production efficiency in other regions. Hypothesis 3 is verified. In terms of the direct effect, digital village building boosts local agricultural green production efficiency by driving agricultural progress, optimizing resource allocation, and cutting environmental impact. For instance, in pilot villages in Shandong, smart irrigation systems reduced water use by 25%. Regarding the indirect effect, digital village building has a significantly positive impact on agricultural green production, indicating that local digital village building can enhance the agricultural green production efficiency in other regions through “knowledge spillover” and “technology diffusion.” For example, Zhejiang's “Digital Agriculture Alliance” platform shared AI-based pest detection and precision fertilization technologies with neighboring Jiangsu and Anhui provinces. Micro-survey data from 2023 shows that in areas within 100 km of Zhejiang's digital villages, such as Suqian in Jiangsu and Wuhu in Anhui, farmers' adoption rate of these technologies was 32% higher than in non-neighboring areas, significantly reducing local chemical fertilizer use and agricultural carbon emissions., the spatial spillover effects come from both explicit knowledge diffusion and implicit experience sharing via cross-regional agricultural technician exchanges. This forms a “demonstration-learning-improvement” cycle, effectively translating local digital village achievements into an increase in regional green productivity.
In terms of the direct effect, digital village construction has a significant positive impact on agricultural green production efficiency. This means that digital village construction can promote the rationalization of the allocation of agricultural production factors by driving agricultural technological progress and transforming agricultural production methods, improve agricultural production efficiency, reduce agricultural carbon emissions, and decrease environmental pollution, thereby boosting the local agricultural green production efficiency. In terms of the indirect effect, digital village construction has a significantly positive impact on agricultural green production, indicating that local digital village construction can drive the improvement of agricultural green production efficiency in other regions through knowledge spillover and the “role-model effect.”
6 Conclusions and discussion
This paper empirically examines the impact of digital village construction on agricultural green production efficiency in 30 provinces of China from 2012 to 2023, as well as the mechanism of rural human capital in the relationship between them, and draws the following conclusions: First, at the national level, digital village construction can effectively improve agricultural green production efficiency, and the result still holds after a series of robustness tests. Regionally, digital village construction has a greater promoting effect in the Northeast region, which is richer in agricultural resource endowments. Second, during the sample observation period, the agricultural green production efficiency in 30 provinces of China shows an upward trend, and its main driving force is technological progress. Third, rural human capital plays an indirect mediating role in the influencing process. Fourth, compared with the intra-provincial area, the spatial spillover effect of the digital village construction level in adjacent cities outside the province on agricultural green production efficiency is more significant.
Based on the above analysis conclusions, this paper puts forward the following countermeasures and suggestions:
On the one hand, enhance the systematic and coordinated regional approach to digital village building. Boost the digital village strategy by strengthening local capacity and spurring regional collaboration. On one hand, solidify rural digital foundations by improving infrastructure, expanding internet access, and creating digital platforms. This ensures agricultural entities can obtain digital resources. Accelerate agricultural IoT deployment and use big data and cloud computing to raise green agricultural productivity. On the other hand, given the significant spatial spillover effects from out-of-province adjacent areas found in the analysis, set up cross-regional digital governance cooperation platforms. These should share digital resources like agricultural big data and technical standards, coordinate regional digital development plans, and spread green technologies across regions. At the same time, implement different regional strategies. The Northeast should develop “digital + agricultural modernization” models, build innovation centers, promote smart farming tech, and enhance the digital management of cold-chain logistics and traceability systems. The central and western regions need to close the digital gap through infrastructure investment and human resource development, use policy incentives to undertake eastern tech spillover, and strengthen cross-regional agricultural e-commerce cooperation to expand markets for local specialty products. The eastern coastal areas, as “digital innovation hubs,” should export advanced governance and green tech to the central, western, and Northeast regions. They should also establish cross-regional industrial alliances to promote the flow of capital, technology, and talent, and explore benefit—sharing mechanisms in green tech innovation.
On the other hand, promote human capital growth and regionally appropriate development. Combine strengthening basic education with targeted skills training to upgrade rural human capital. On one hand, improve the modern agricultural knowledge training system, build digital communication platforms to boost workers' digital literacy, integrate digital tech into rural basic education, and conduct skills training that suits local industries. For example, e-commerce training for areas rich in agricultural products and smart farming tech training for large-scale farming areas. On the other hand, considering the spatial spillover effects of digital development, innovate cross-regional talent collaboration mechanisms. For instance, joint training programs between the east and west can promote two-way practical guidance and skill enhancement. Meanwhile, develop differential human capital plans for regions with different characteristics. In resource—transition regions, focus on “digital + industrial transition” training to help farmers acquire skills in agricultural product processing and e-commerce operations, thus promoting the integration of primary, secondary, and tertiary industries. In ecologically fragile regions, combine digital literacy with ecological protection technologies to cultivate professional farmers with both environmental awareness and digital skills. In specialty agriculture regions, rely on local resources to develop “digital marketing + cultural innovation” training projects. Use social media and e-commerce platforms to promote regional brands and increase product value—added.
Data availability statement
The original contributions presented in the study are included in the article/Supplementary material, further inquiries can be directed to the corresponding author.
Author contributions
XL: Investigation, Writing – review & editing. YC: Data curation, Writing – original draft, Investigation.
Funding
The author(s) declare that financial support was received for the research and/or publication of this article. This research was funded by Soft Science Project of Henan Province in 2025 (252400411208), Results of the Henan Province Philosophy and Social Science Planning Project (2024CJJ205), Henan Province Graduate Education Reform and Quality Improvement Project (YJS2022JD30), and Nanhu Scholars Program for Young Scholars of XYNU.
Conflict of interest
The authors declare that the research was conducted in the absence of any commercial or financial relationships that could be construed as a potential conflict of interest.
Generative AI statement
The author(s) declare that no Gen AI was used in the creation of this manuscript.
Publisher's note
All claims expressed in this article are solely those of the authors and do not necessarily represent those of their affiliated organizations, or those of the publisher, the editors and the reviewers. Any product that may be evaluated in this article, or claim that may be made by its manufacturer, is not guaranteed or endorsed by the publisher.
Supplementary material
The Supplementary Material for this article can be found online at: https://www.frontiersin.org/articles/10.3389/fsufs.2025.1597762/full#supplementary-material
References
Balafoutis, A., Beck, B., Fountas, S., Vangeyte, J., Van der Wal, T., Soto, I., et al. (2017). Precision agriculture technologies positively contributing to GHG emissions mitigation, farm productivity and economics. Sustainability 9:1339. doi: 10.3390/su9081339
Ballantyne, P., Maru, A., and Porcari, E. M. (2010). Information and communication technologies—opportunities to mobilize agricultural science for development. Crop Sci. 50, S-63-S-69. doi: 10.2135/cropsci2009.09.0527
Cai, Q., and Han, X. (2024). Impacts and mechanisms of digital village construction on agricultural green total factor productivity. Front. Sustain. Food Syst. 8:1431294. doi: 10.3389/fsufs.2024.1431294
Chunfang, Y., Xing, J., Changming, C., Shiou, L., Obuobi, B., and Yifeng, Z. (2024). Digital economy empowers sustainable agriculture: Implications for farmers' adoption of ecological agricultural technologies. Ecological Indicators 159:111723. doi: 10.1016/j.ecolind.2024.111723
Du, J. G., Li, B., and Yang, H. (2023). The impact of agricultural human capital on the green total factor productivity of agriculture under population aging. China Popul. Resour. Environ. 33, 215–228. doi: 10.12062/cpre20230501
Guo, B., He, D., Zhao, X., Zhang, Z., and Dong, Y. (2020). Analysis on the spatiotemporal patterns and driving mechanisms of China's agricultural pro-duction efficiency from 2000 to 2015. Phys. Chem. Earth 120:102909. doi: 10.1016/j.pce.2020.102909
Hajiyeva, A., Mammadova, U., Tanriverdiyeva, G., and Kovalenko, O. (2023). Technological innovations in agriculture: impact on production efficiency. 27, 172–182. doi: 10.48077/scihor1.2024.172
Han, H., Zhong, Z., Guo, Y., Xi, F., and Liu, S. (2018). Coupling and decoupling effects of agricultural carbon emissions in China and their driving factors. Environ. Sci. Pollut. Res. 25, 25280–25293. doi: 10.1007/s11356-018-2589-7
Hu, Y., Yu, H., and Yang, X. (2023). Can rural human capital improve agricultural ecological efficiency? Empirical evidence from China. Sustainability 15:12317. doi: 10.3390/su151612317
Huang, Y., Chen, Z., and Yin, S. (2024). Spatial spillover effects of the digital economy on the green total factor productivity of China's manufacturing industry. Environ. Res. Commun. 6:65006. doi: 10.1088/2515-7620/ad53aa
Jiang, S., Zhou, J., and Qiu, S. (2022). Digital agriculture and urbanization: mechanism and empirical research. Technol. Forecast. Soc. Change 180:121724. doi: 10.1016/j.techfore.2022.121724
Lei, S., Yang, X., and Qin, J. (2023). Does agricultural factor misallocation hinder agricultural green production efficiency? Evidence from China. Sci. Total Environ. 891:164466. doi: 10.1016/j.scitotenv.2023.164466
Li, F., Hou, J., Yu, H., Ren, Q., and Yang, Y. (2024). Harnessing the digital economy for sustainable agricultural carbon productivity: a path to green innovation in China. J. Knowl. Econ. 2024, 1–27. doi: 10.1007/s13132-024-02158-7
Li, J., Huang, X., Yang, T., Su, M., and Guo, L. (2023). Reducing the carbon emission from agricultural production in China: do land transfer and urbanization matter? Environ. Sci. Pollut. Res. 30, 68339–68355. doi: 10.1007/s11356-023-27262-0
Liu, M., and Liu, H. (2024). The influence and mechanism of digital village construction on the urban–rural income gap under the goal of common prosperity. Agriculture 14:775. doi: 10.3390/agriculture14050775
Liu, S., Lei, P., Li, X., and Li, Y. (2022). A nonseparable undesirable output modified three-stage data envelopment analysis application for evaluation of agricultural green total factor productivity in China. Sci. Total Environ. 838:155947. doi: 10.1016/j.scitotenv.2022.155947
Liu, Z., and Xin, L. (2019). Dynamic analysis of spatial convergence of green total factor productivity in China's primary provinces along its Belt and Road Initiative. Chin. J. Popul. Resour. Environ. 17, 101–112. doi: 10.1080/10042857.2019.1611342
Lu, S., Zhuang, J., Sun, Z., and Huang, M. (2024). How can rural digitalization improve agricultural green total factor productivity: empirical evidence from counties in China. Heliyon 10:e35296. doi: 10.1016/j.heliyon.2024.e35296
Lu, X., Qu, Y., Sun, P., Yu, W., and Peng, W. (2020). Green transition of cultivated land use in the Yellow River Basin: a perspective of green utilization efficiency evaluation. Land 9:475. doi: 10.3390/land9120475
Luo, J., Huang, M., Hu, M., and Bai, Y. (2023). How does agricultural production agglomeration affect green total factor productivity? Empirical evidence from China. Environ. Sci. Pollut. Res. 30, 67865–67879. doi: 10.1007/s11356-023-27106-x
Ma, G., Li, M., Luo, Y., and Jiang, T. (2023). Agri-ecological policy, human capital and agricultural green technology progress. Agriculture 13:941. doi: 10.3390/agriculture13050941
Padhiary, M. (2025). “The convergence of deep learning, IoT, sensors, and farm Machinery in agriculture,” in Designing Sustainable Internet of Things Solutions for Smart Industries, 109–142. doi: 10.4018/979-8-3693-5498-8.ch005
Qin, L., Zhang, Y., Wang, Y., Pan, X., and Xu, Z. (2024). Research on the impact of digital green finance on agricultural green total factor productivity: evidence from China. Agriculture 14:1151. doi: 10.3390/agriculture14071151
Robinson, S. V. J., Nguyen, L. H., and Galpern, P. (2022). Livin'on the edge: precision yield data shows evidence of ecosystem services from field boundaries. Agric. Ecosyst. Environ. 333:107956. doi: 10.1016/j.agee.2022.107956
Song, J., and Chen, X. (2019). Eco-efficiency of grain production in China based on water footprints: a stochastic frontier approach. J. Clean. Prod. 236:117685. doi: 10.1016/j.jclepro.2019.117685
Sun, H. Y., and Zhong, Q. (2025). The impact of rural digital economy on agricultural green total factor productivity: a dual examination based on spatial spillover and threshold effect models. Ecol. Econ. 41, 131–138.
Sun, Y. (2022). Environmental regulation, agricultural green technology innovation, and agricultural green total factor productivity. Front. Environ. Sci. 10:955954. doi: 10.3389/fenvs.2022.955954
Tang, Y., and Chen, M. (2022). The impact of agricultural digitization on the high-quality development of agriculture: an empirical test based on provincial panel data. Land 11:2152. doi: 10.3390/land11122152
Tim, Y., Cui, L., and Sheng, Z. (2021). Digital resilience: how rural communities leapfrogged into sustainable development. Inf. Syst. J. 31, 323–345. doi: 10.1111/isj.12312
Wang, J. (2017). Sustainable agricultural development in China: an assessment of problems, policies and perspectives (Doctoral Thesis). doi: 10.26481/dis.20171018jw
Wang, L., Qi, Z., Pang, Q., Xiang, Y., and Sun, Y. (2020). Analysis on the agricultural green production efficiency and driving factors of urban agglomerations in the middle reaches of the Yangtze River. Sustainability 13:97. doi: 10.3390/su13010097
Wang, S., Zhu, J., Wang, L., and Zhong, S. (2022). The inhibitory effect of agricultural fiscal expenditure on agricultural green total factor productivity. Sci. Rep. 12:20933. doi: 10.1038/s41598-022-24225-2
Wang, W., and Mei, T. (2024). Research on the effect of digital economy development on the carbon emission intensity of agriculture. Sustainability 16:1457. doi: 10.3390/su16041457
Wang, Z., Zhang, X., Lu, H., Kang, X., and Liu, B. (2023). The effect of industrial agglomeration on agricultural green production efficiency: evidence from China. Sustainability 15:12215. doi: 10.3390/su151612215
Yang, Y., Fan, Y., and Sun, R. A. (2019). Human-computer interaction system for agricultural tools museum based on virtual reality technology. Adv. Multimedia 2019:2659313. doi: 10.1155/2019/2659313
Ye, C. S., and Ma, Y. T. (2020). How do human capital and its compatibility with technological progress affect the agricultural planting structure? Rural Econ. China 34–55.
Ye, D., Zhen, S., Wang, W., and Liu, Y. (2023). Spatial double dividend from China's main grain-producing areas policy: total factor productivity and the net carbon effect. Hum. Soc. Sci. Commun. 10, 1–22. doi: 10.1057/s41599-023-01962-x
Yu, Z., Lin, Q., and Huang, C. (2022). Re-measurement of agriculture green total factor productivity in China from a carbon sink perspective. Agriculture 12:2025. doi: 10.3390/agriculture12122025
Zhang, Y., Ji, M., and Zheng, X. (2023). Digital economy, agricultural technology innovation, and agricultural green total factor productivity. Sage Open 13:21582440231194388. doi: 10.1177/21582440231194388
Zhao, L., Chen, H., Ding, X., and Chen, Y. (2024). Does digital village construction empower the green allocation of agricultural water resources? Systems 12:214. doi: 10.3390/systems12060214
Zhu, Y., Zhang, Y., and Piao, H. (2022). Does agricultural mechanization improve the green total factor productivity of China's planting industry? Energies 15:940. doi: 10.3390/en15030940
Keywords: digital villages construction, agricultural green production efficiency, rural human capital, spatial spillover effect, mediating effect
Citation: Lei XK and Chen YX (2025) Impact of digital villages construction on agricultural green production efficiency: evidence from China. Front. Sustain. Food Syst. 9:1597762. doi: 10.3389/fsufs.2025.1597762
Received: 24 March 2025; Accepted: 21 April 2025;
Published: 15 May 2025.
Edited by:
Qihua Cai, Zhengzhou University, ChinaReviewed by:
Ailiang Xie, Linyi University, ChinaXieqihua Liu, Macao Polytechnic University, China
Qundi Feng, Southwest University of Political Science & Law, China
Copyright © 2025 Lei and Chen. This is an open-access article distributed under the terms of the Creative Commons Attribution License (CC BY). The use, distribution or reproduction in other forums is permitted, provided the original author(s) and the copyright owner(s) are credited and that the original publication in this journal is cited, in accordance with accepted academic practice. No use, distribution or reproduction is permitted which does not comply with these terms.
*Correspondence: Xian Kai Lei, bGVpeGlhbmthaUB4eW51LmVkdS5jbg==