- 1Fraunhofer Institute for Toxicology and Experimental Medicine, Chemical Safety and Toxicology, Hannover, Germany
- 2GeneXplain GmbH, Wolfenbüttel, Germany
- 3Medizinische Hochschule Hannover, Hannover, Germany
- 4Leiden University Medical Center, Leiden, Netherlands
This case study explores the applicability of transcriptome data to characterize a common mechanism of action within groups of short-chain aliphatic α-, β-, and γ-diketones. Human reference in vivo data indicate that the α-diketone diacetyl induces bronchiolitis obliterans in workers involved in the preparation of microwave popcorn. The other three α-diketones induced inflammatory responses in preclinical in vivo animal studies, whereas beta and gamma diketones in addition caused neuronal effects. We investigated early transcriptional responses in primary human bronchiolar (PBEC) cell cultures after 24 h and 72 h of air-liquid exposure. Differentially expressed genes (DEGs) were assessed based on transcriptome data generated with the EUToxRisk gene panel of Temp-O-Seq®. For each individual substance, genes were identified displaying a consistent differential expression across dose and exposure duration. The log fold change values of the DEG profiles indicate that α- and β-diketones are more active compared to γ-diketones. α-diketones in particular showed a highly concordant expression pattern, which may serve as a first indication of the shared mode of action. In order to gain a better mechanistic understanding, the resultant DEGs were submitted to a pathway analysis using ConsensusPathDB. The four α-diketones showed very similar results with regard to the number of activated and shared pathways. Overall, the number of signaling pathways decreased from α-to β-to γ-diketones. Additionally, we reconstructed networks of genes that interact with one another and are associated with different adverse outcomes such as fibrosis, inflammation or apoptosis using the TRANSPATH-database. Transcription factor enrichment and upstream analyses with the geneXplain platform revealed highly interacting gene products (called master regulators, MRs) per case study compound. The mapping of the resultant MRs on the reconstructed networks, visualized similar gene regulation with regard to fibrosis, inflammation and apoptosis. This analysis showed that transcriptome data can strengthen the similarity assessment of compounds, which is of particular importance, e.g., in read-across approaches. It is one important step towards grouping of compounds based on biological profiles.
1 Introduction
In Europe, human risk assessment is undergoing a paradigm shift towards the integration of mechanistic data from primarily human in vitro and in silico models, referred to as new approach methodologies (NAMs). The use of mechanistic evidence, has the potential to significantly improve risk assessment and reduce uncertainties and, thus, seems promising in many respects. A better understanding of the biological mechanisms causing adverse toxicological effects will help to identify new ways of prevention and thus risk management. NAMs such as high-content or high-throughput data will also enable faster and more efficient assessment, providing data that is directly relevant to humans.
In line with animal welfare and the 3R principle, several European agencies, such as EFSA (EFSA, 2021), EMA (European Medicines Agency, 2021), have expressed their intention to reduce, refine and replace animal testing as far as possible within in the next decade by integration of NAMs. In other regulatory areas, e.g., for cosmetics, animal testing is banned (Pistollato et al., 2021) or, as under REACH, is only considered appropriate when all other options have been explored (European Commission. Regulation, 2019).
A challenge for the implementation of NAMs into regulatory decision-making lies in the development of concepts and overarching frameworks for the interpretation and integration of NAM data (Knight et al., 2021).
Case studies on the integration of mechanistic data, e.g., in the context of Adverse Outcome Pathways (AOPs) or Integrated Assessment and Evaluation (IATA), help to develop new assessment concepts, learn about their advantages and limitations, address uncertainties and thus advance their implementation in risk assessment.
In line with this idea, read-across is an established approach that is well suited to evaluate the new methods and gain more confidence in the use of NAMs as they offer the possibility to compare the traditional approach based on in vivo animal data with evidence from NAM data (Escher et al., 2019).
Read-across is a well-known approach in chemical risk assessment to estimate the toxicity of a target compound for which substance-specific experimental data are not available. It is of particular importance for high tier endpoints like long-term toxicity caused by low level exposure to substances occurring at workplace or in household products (Ball et al., 2016). The read-across assessment framework (ECHA, 2017) provides guidance on read-across approaches using traditional preclinical animal studies, while similar guidance for the integration of new approach methods such as “omic” data is missing to date.
The read-across approach assumes that substances with similar toxicokinetic and toxicodynamic properties will cause similar toxicological effects in an exposed organism or follow a consistent trend (ECHA, 2017). The most challenging step in the read-across assessment is usually to justify the similarity of the source to the target compound(s), as often only the structural and physicochemical properties of the target compound are known. Many read-across assessments are therefore often not accepted by authorities, as they fail to provide sufficient evidence on share toxicodynamic and kinetic properties of the grouped compounds (Ball et al., 2016).
The starting point of most read-across assessments is, therefore, an assessment of shared structural and physicochemical properties leading to the identification of an initial list source compounds. Subsequently, the available in vivo endpoint data are used to conclude on shared toxicodynamic properties within the grouped compounds. As in vivo studies are usually descriptive and do not provide insights into the mechanisms leading to the observed toxic effects, a shared toxicological effect pattern can be justified but usually not a shared mode of action. The assessment is complicated by the fact that often study results from different laboratories need to be compared. These studies differ with regard of study design (e.g., species tested, study size, dose selection and dose intervals), which leads to some variability in the observed apical findings (Ball et al., 2016; Judson et al., 2016).
A more precise assessment of shared toxicodynamic properties within the grouped compounds, based on, e.g., the description of their molecular mechanisms, will significantly reduce the uncertainty in read-across assessments. Few case studies have been published in which NAMs, like in vitro and in silico models, are used to substantiate the assessment of shared mode of actions in read-across approaches (Pawar et al., 2019; Grimm et al., 2019; Escher et al., 2019).
Although transcriptome data provide information on the perturbation of gene activities (Escher et al., 2019), it is still a challenge to infer the associated cellular processes and mechanisms that may finally result in the development of diseases or adverse outcomes (Sebastian-Leon et al., 2014). These data are, therefore, to date seldomly used in regulatory decision making (Karahalil, 2016). In addition, several analytical and technical challenges have been reported, including a large variety of alternative analysis strategies and the complexity of the data. Also, conceptual frameworks for the integration of omic data into regulatory hazard assessment are lacking, as there is no consensus to date on the approach to assess pathways and adverse effects, derive benchmark doses for gene perturbations and pathway analyses, and quantify uncertainties. Case studies with relevant regulatory problem formulations can serve as a tool to gain more confidence into new approaches such as omic supported hazard assessments and by this help to close these conceptual data gaps.
The read-across case study presented here investigates the use of transcriptome data for the evaluation of (dis)similar mechanism of action between grouped compounds. For this purpose, a group four α-diketones is analysed, of which three compounds are suspected to induce pulmonary fibrosis (bronchiolitis obliterans) in rodents (Brass and Palmer, 2017). In addition to these structurally very similar α-diketones, one structurally related β- and one γ-diketone are included into the assessment to identify potential (dis)similarities between these volatile diketone compounds. One compound with an entirely different mode of action, tunicamycin, is tested to be able to identify to some extent normal adaptive cellular responses. Two ketones, butanone, and acetone, are tested as negative compounds because both compounds did not induce any adverse effect in preclinical rodent studies with repeated exposure (Chen and Hee, 1995).
2 Materials and methods
2.1 Chemicals
Chemicals were purchased at the highest purity available. α-diketones: 2,3-Butandione (diacetyl, purity 99.5%, Lot No. SHBG3507V, Sigma B85307-100 ML), 2,3-Pentanedione (purity 97.1%, Lot No. MKBB7504V, Sigma 241962-25G), 2,3-Hexanedione (purity 96.0%, Lot No. MKBV4849V, Sigma W255807-250G-K), and 3,4-Hexanedione (purity 96.7%, Lot No. STBF5886V, Sigma 306932-25G), one β-diketone: acetylacetone (2,4-Pentanedione, purity 99.8%, Lot No. STBF8568V, Sigma 10916-1 KG), one γ-diketone: acetonylacetone (2.5-Hexanedione, purity 99.7%, Lot No. WXBC2583V, Sigma 165131-25G), butanone (purity 99.9%, Lot No. MKBV9520V, Sigma W217012-100G-K) and acetone (purity 99.9%, Lot No. MKBR5795V, Sigma W332615-100G), tunicamycin (purity ≥98.0%, Lot No. 037M4047V, Sigma T7765-1 MG).
2.2 Exposure of primary human epithelial cells (PBECs) via ALI application
PBECs were isolated from tumor-free resected lung tissue from 4 donors by enzymatic digestion, cells were expanded in keratinocyte serum-free medium (KSFM, Gibco) and stored until usage as previously described in Van Wetering et al. (2000). PBECs were cultured as described in Boei et al. (2017). Briefly, cells were seeded after the first passage on coated transwell tissue culture inserts (Corning Costar, 0.4 µm pore size, 1.12 cm2 surface) to grow under submerged conditions for 6 days at 37°C, 5% CO2 using a 1:1 mixture of DMEM (Life Technologies, Bleiswijk, the Netherlands) and ‘bronchial epithelial growth medium’ (Lonza, Verviers, Belgium) (B/D medium) with supplementation of BEGM BulletKit singlequots (0.4% [w/v] bovine pituitary extract, 1 mM hydrocortisone, 0.5 μg/ml human hEGF, 0.5 μg/ml epinephrine, 10 μg/ml transferrin and 5 μg/ml insulin, T3 (Lonza) and additional 1 mM HEPES (Lonza), 1 μg/ml BSA (Sigma-Aldrich), 100 U/ml penicillin and 100 μg/ml streptomycin (Lonza) and 15 ng/ml retinoic acid (Sigma-Aldrich). Subsequently, the medium on the cell surface (apical side) was removed to culture the PBECs at the air-liquid interface (ALI), which was maintained up to 28 days at 37°C, 5% CO2 with exchange of the ‘bronchial epithelial growth medium’ every 2-3 days followed by apical washing with 100 µL PBS to remove accumulated mucus. Mucociliary epithelial cells differentiation occurs during the maintenance under ALI conditions.
PBECs were exposed to the selected test chemicals under ALI conditions using the P.R.I.T.® ExpoCube® device for 1 h once (acute exposure) or repeatedly on 3 consecutive days (repeated exposure). Supplementary Figure S1 provides an illustration of the exposure setup. Exposures were conducted using a volume flow of 350 ml/min as primary flow for transporting the gas through the exposure device (inline) to the FT-IR monitor. From this primary flow sampling for cell exposure was done using exposure flows of 3 ml/min for each culture. For a detailed description of the P.R.I.T.® ExpoCube® workflow see Ritter and Knebel (2014). Briefly, PBECs cultivated on inserts of a 12-well plate (Corning Costar) included three exposure test lines simultaneously: Exposure to (1) the test chemical, (2) to clean air (negative control) or (3) no exposure (non-exposure control). The test atmosphere was generated by conducting clean air over the surface of the chemical inside a gas washing bottle at 25°C. The resulting atmosphere was diluted with clean air to achieve the desired concentrations for each chemical respectively (see Table 1). The analysis of chemicals during exposure was performed by online measurement using a FT-IR spectroscopy (GASMET, Ansyco, Germany). PBECs were exposed to each chemical in 4-5 concentrations according to pre-experiments. The background of the dose selection is described in detail in supplemental material (SM1). Cellular viability was measured by LDH-leakage and barrier function by measuring the transepithelial electrical resistance (TEER) 24 h after the final exposure (Results in Supplementary Figure S2). At the same timepoint, lysis of PBEC-ALI models for TempO-Seq analysis at BioSpyder was performed. Deviating from this, the non-volatile compound tunicamycin was added to the medium for 24 h, directly followed by lysis. The highest concentration without cytotoxic effect was selected for further omics testing. 500 μL lysis buffer (BioSpyder) was added to the apical side of the PBEC-ALI models, incubated at RT for 10-15 min and transferred to a 96-well plate, this procedure was carried out based on (Yeakley et al., 2017). Samples were stored at −80°C and subsequently shipped on dry ice to BioSpyder technologies (Bioclavis, United Kingdom) for sequencing. All data is from 4 donors as replicates, three of the donors are male and one donor is female.
2.3 Identification of differentially expressed genes
Transcriptome analyses were carried out with the human TempO-Seq S1500+ assay, which comprises 3,565 genes (Mav et al., 2018). These 3,565 genes were selected by the EUTOXTISK consortium because they are particularly frequently differentially expressed in toxicological experiments, and care was taken to ensure that the selected gene panel covers well-annotated pathways (Mav et al., 2018).
The RNA sequences per probe were mapped against the human ensemble transcriptome (hg38 aka GRCh38v100) to obtain ensemble gene IDs.
Counts were normalized by counts per million. Within quality control, samples with low read counts corresponding to a library size <500 k and genes with an overall variance of 1 were discarded. The controls were grouped by time point and compound. A batch correction was carried out according to gender. The differentially gene expression analysis was done with DESeq2 in R (version 1.32.0) (Love et al., 2014), considering an adjusted p-value of <0.05 (Benjamini-Hochberg method) and an absolute log2FoldChange of 1 or higher.
2.4 Pathway analysis
A pathway analysis was carried out taking into account the six databases from ConsensusPathDB (Version 35, Barel and Herwig, 2018). 2,603 signaling pathways were considered, these signaling pathways included at least four genes of the TempO-Seq S1500+ gene panel.
The preselection of the most responsive genes interferes with pathway enrichment analysis, that is normally performed, as it uses statistical significance tests such as the Fisher exact test to distinguish a random finding from a specific activation (García-Campos et al., 2015).
In this study, we therefore used an approach recently described by (Barel and Herwig, 2018). Differentially expressed genes (g) were weighted for the analysis of pathways by calculating a gene score (S) for each condition (c). A condition is the combination of time point, compound, and concentration. Gene scores take into account the significance (adjusted p-value) and the observed absolute log2fold change of the differentially expressed genes.
Thereafter, a pathway score (M) for each pathway (l) was calculated for each condition (c) using the gene scores. For this purpose, the scores of all DEGs are summed and divided by the number of genes (m) included in the pathway and measured in the S1500+-panel.
In order to be able to compare the results obtained, a normalization over all pathways for a condition was carried out (RPR).
Pathways with a RPR greater than two were considered as activated.
2.5 Upstream analysis
Upstream Analysis is an integrated promoter–pathway analysis.
Upstream analyses were performed on gene sets of 300 differentially expressed genes per time point and substance and group using the geneXplain platform (Stegmaier et al., 2017).
In addition, a group specific DEG profile was developed for the α-diketones. To determine the most representative genes being affected by α-diketone exposure, DEGs commonly observed following exposure to all α-diketones (in at least one concentration group tested) were selected. For the substance profiles, the top 300 DEGs were selected based on the average absolute highest log2 fold change.
To obtain information about potentially involved transcription factors, promoters of the differentially regulated genes are retrieved and analyzed for potential transcription factor (TF) binding sites in a first step. From these binding sites, a set of postulated TFs is identified that potentially have regulated the found DEGs.
The search for enriched transcription factor binding sites (TFBSs) from promoters of DEGs per substance, time point and concentration and for the groups and substance profiles was performed using the geneXplain platform (Kolpakov et al., 2011) and the corresponding ‘Identify enriched motifs in promoters (TRANSFAC®)’ workflow (Koschmann et al., 2015). Gene promoters with a range from −1000bp to +100bp from transcriptional start sites were screened for potential TFBSs based on known motifs in the TRANSFAC® database, which is a collection of positional weight matrices (PWMs) of binding sites and corresponding transcription factors. A total number of 394 matrices were used whose associated transcription factors are known to be expressed in lung tissue (TRANSFAC® database version 2021.1. The algorithm estimates an enrichment analysis for binding sites that occur more frequently compared to a background set, which was a random set of 900 genes and corresponding promoters that are expressed in human lung tissue (source: HumanPSD database). The result is a list of the binding and the associated set of human transcription factors that are likely responsible for the differential regulation of the observed set of DEGs.
Starting from this, a further step of upstream analysis was performed to search for master regulators (MR) that are known to activate the identified potential TFs. Master regulators are viewed as those regulation units showing a potential key functionality of several underlying biological and toxicological processes. A molecular network analysis tool called “regulator search” was used to identify MRs (Kel et al., 2006). The principle of the regulator search can be briefly summarized as follows: the TRANSPATH database (version 2021.1) comprises a large directed protein network, where the nodes are proteins and the edges are reactions in different pathways. The postulated TFs are represented as nodes in this network.
As mentioned above the analysis started from the identified TFs, the algorithm searches for up to 10 upstream nodes and postulates MRs, which represent highly interconnected proteins (by a score >0.2) and specific proteins for the DEG input set (by a z score >1) (Kel et al., 2006).
2.6 Reconstructed signaling protein networks
The reconstructed networks were developed to visualize a connection to the Mechanism of Action and the MRs. The reconstructed networks connect genes that could be assigned to a specific mode of action and the MRs were then mapped onto this network in order to obtain comparability between the MRs of the different group/substance profiles.
Protein lists were obtained by multiple queries from different databases such as HumanPSD™ KEGG, Wikipathways (Wingender et al., 2007), which contain disease and signaling network information observed in humans for three adverse outcomes, namely, “pulmonary fibrosis,” “inflammation” and “apoptosis.” With the obtained three protein lists a clustering algorithm within the geneXplain platform was used to reconstruct signaling protein networks based on evidence of protein-protein interactions from the TRANSPATH® database (Krull et al., 2006). In the TRANSPATH® database protein-protein interactions occurring in humans manual curated from peer-reviewed publications and assigned evidence levels and reliability scores for protein reactions obtained from experiments for proteins existing in vivo are represented. Inside the procedure for a reconstruction of protein networks we allowed a maximum of three predicted protein reactions apart from the input protein list. Cytoscape (version 3.8.2) was used for the visualization of all reconstructed protein networks (Kohl et al., 2011).
3 Results
3.1 Substance characterization
The read-across case study investigates nine compounds, which are grouped into five groups based on their structural as well as toxicological properties (Figure 1, grouping indicated by coloured boxes). One group contains four α-diketones, whose structure differs in the length of the hydrocarbon side chains (purple box). The α-diketone Diacetyl is known to cause bronchiolitis obliterans in employees who made butter-flavored microwave popcorn. Its mechanism of action has been well studied in vivo (Zaccone et al., 2013). It is also known from preclinical in vivo studies in rats that the two other α-diketone 2,3-pentanedione and 2,3-hexanedione have the same mode of action (Zaccone et al., 2013). The observed progression of toxicological findings of both compounds proceeds from injuries to the epithelial tissue via immune processes to bronchial epithelial scarring (Gwinn et al., 2017). The toxicological properties of 3,4-hexanedione are largely unknown. 3,4-Hexanedione was tested in a subchronic study in rats wherein no effect was observed up to a single oral dose of 17 mg/kg bw/day (Posternak et al., 1969). An inhalation study is not available.
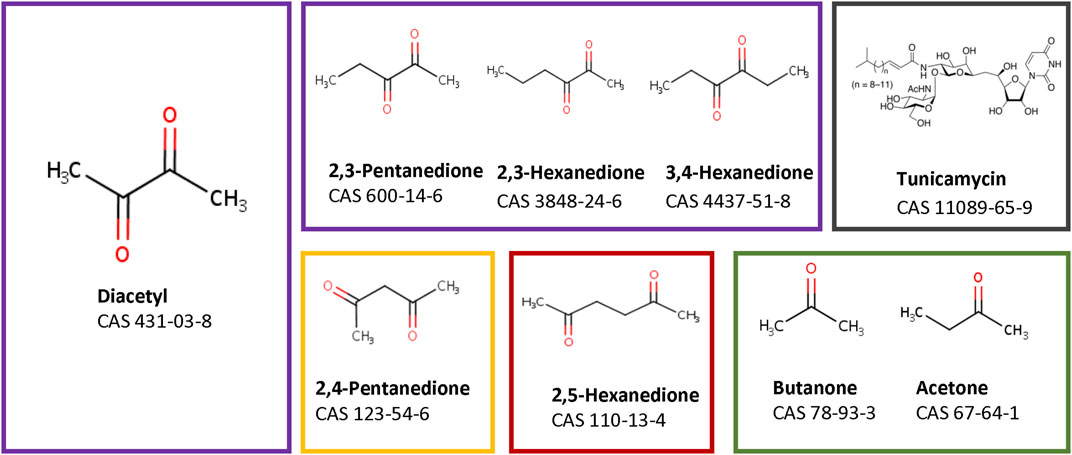
FIGURE 1. Chemical structures of the tested substances. Purple edges the α-diketones, orange the β-diketones, red edges the γ-diketones, the negative substances are edged in green, and tunicamycin as a substance with a different MoA has a black edge.
Two structurally slightly different diketone compounds, 2,4-pentanedione (β-diketone, yellow box) and 2,5-hexanedione (γ-diketone, red box) were also included into the assessment. 2,4-Pentanedione causes inflammation in the upper airways of rats (DODD et al., 1986) whereas 2,5-hexanedione induce peripheral neuropathy after subcutaneous injection (Spencer and Schaumburg, 1975; Ichihara et al., 2019).
In addition, two ketones, acetone and butanone, were selected as negative substances (green box), as both substances did not provoke any adverse effect after repeated inhalation exposure in preclinical studies (Bruckner, 1981; Cavender et al., 1983).
Also the structurally very different compound tunicamycin was chosen because of its well-known mode of action, which is not related to pulmonary inflammation and fibrosis. Tunicamycin blocks protein folding of glycoproteins by inhibiting N-glycosylation (Wang et al., 2015), resulting in induction of the unfolded protein response. This is under the control of three sensors, namely, PERK, IRE1a and ATF6, each activating different signaling cascades (Yang et al., 2020).
3.2 Concentration dependency and impact of exposure duration on transcriptome data and pathways analysis
The impact of exposure time on the resulting transcriptome data was evaluated using the α-diketone Diacetyl as an example. In parallel the concentration dependent effects of diacetyl on the transcriptome were analyzed. Overall, Diacetyl shows an increasing number of DEGs with increasing concentration levels (Figure 2A). Acute exposure led to 10 downregulated DEGs at the lowest concentration. At the highest concentration 257 up- and 271 downregulated DEGs could be shown whereas repeated application over 3 days resulted overall in more DEGs, with 551 up- and 668 downregulated genes at the highest concentration (Figure 2A).
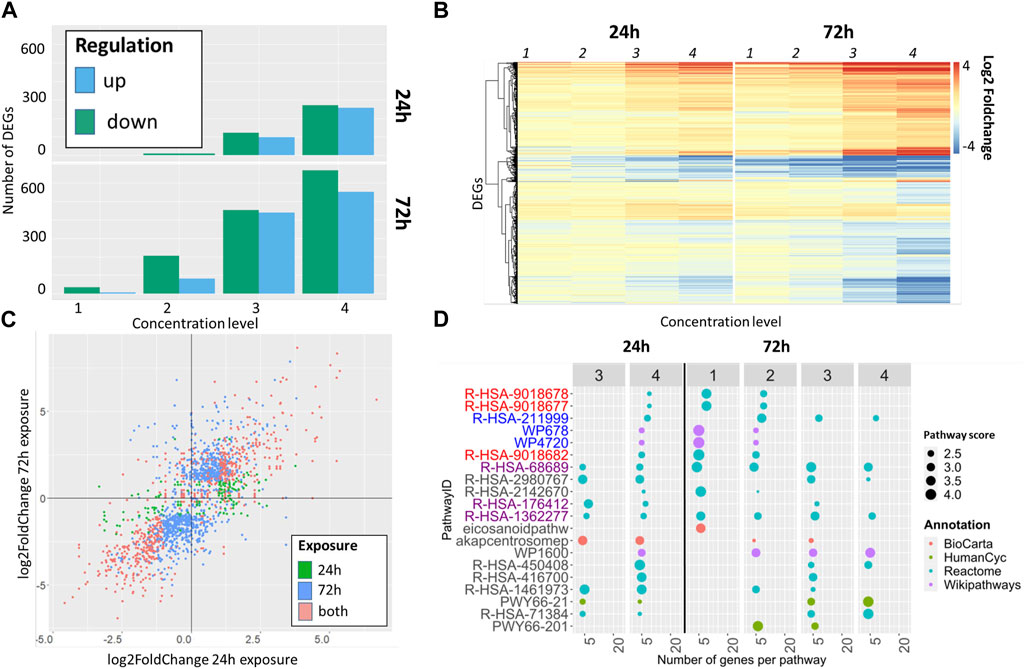
FIGURE 2. Comparison of DEG and pathway enrichment analyses for diacetyl after single (24 h) and repeated exposure (72 h): (A) Number of DEGs for diacetyl classified by upregulated and downregulated genes. (B) heatmap of DEGS showing similarities of log2foldchanges between single and repeated exposure. (C) Correlation of log2fold changes of DEGs at the highest tested concentration (concentration level 4), the colour schema indicates differentially expressed genes obtained after single (green), repeated (blue) or in both approaches (red). (D) Comparison of 10 pathways per time point with highest pathways scores. Pathways are colored according to their known mode of action: red—inflammation, blue - drug metabolism, purple - fibrosis, black—other.
The heat map shows that the DEGs have a common expression pattern, i.e., they are up- or downregulated together, both within the single and repeated exposure, as well as considering the two exposure times. The absolute log2FoldChange of the observed DEGs revealed a concentration dependent increase (Figure 2B).
DEGs obtained from acute and repeated exposure show a high concordance with regard to their up- and downregulation (Figure 2C, colored in red). The same trend is observed for the majority of differentially expressed genes after repeated exposure (colored in blue) and for the few DEGs from acute exposure (Figure 2C, colored in green).
The pathway enrichment analysis resulted in a total of 296 pathways for diacetyl. 173 pathways were obtained after acute exposure, 212 after repeated exposure, with 89 shared pathways for both time points. The ten top regulated pathways, defined by the highest pathways score, were compared in more detail per time point. There are no overlaps between the pathways for these top 10 pathways per time point, but all pathways that were already active in the case of a single exposure also appear in the case of repeated exposure, and only two pathways are not active in the case of the single exposure. Despite this high qualitative concordance, repeated exposure tends to induce more pathways at overall lower concentrations compared to acute exposure conditions.
Pathways are colored according to their MoA (Figure 3D; Table 1). All three pathways associated with fibrosis are observed after single and repeated exposure (Figure 2D, purple).
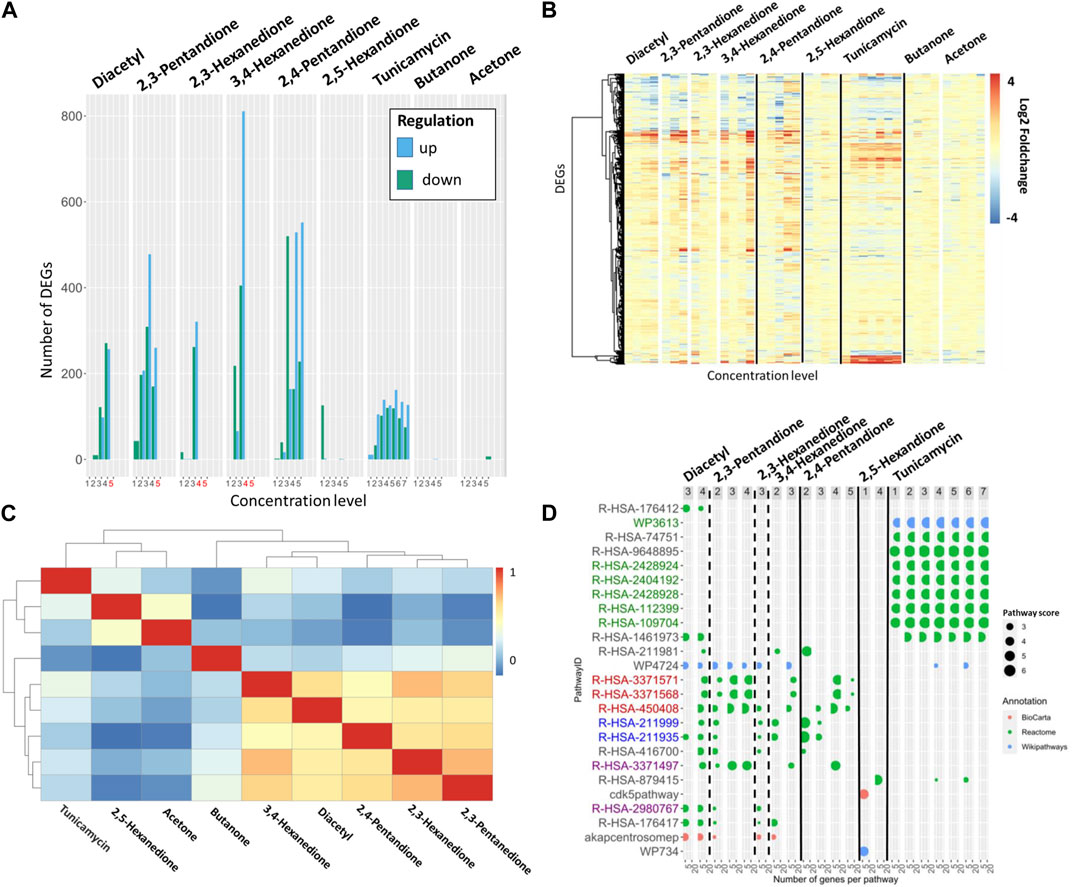
FIGURE 3. (A) Number of DEGs per substance and concentration. Dose groups with a cytotoxicity above 20% are indicated in red. All compounds were tested using 5 dose levels, tunicamycin was tested with 7 dose levels. (B) Log2FoldChange of all genes that are DEG at least once per substance in increasing concentration. The genes are arranged with hierarchical clustering. (C) Correlation matrix. The correlation scores ordered with hierarchical clustering were determined using the Spearman coefficient of the log2FoldChange-values. (D) 30 pathways are shown, which are derived from the ten pathways with highest pathway scores for diacetyl, α- and β-diketones and tunicamycin. Pathways listed in the table are colored according to their mode of action, red for inflammation, blue for drug metabolism, green for protein folding and purple for fibrosis.
These results indicate that under the exposure conditions chosen in this study, the exposure time has an impact on the transcriptome response. Repeated exposure led to more pronounced responses regarding the number and the fold change of differentially expressed genes and the thereof resulting number of enriched pathways. Further a trend towards responses at lower dosing was observed.
Both single and repeated exposure lead however to inflammation- and fibrosis-related signalling pathways relevant to the presumed mode of action of diacetyl.
All other compounds in this study were tested under acute exposure conditions for the inter- and intra-group comparisons in order to keep the experimental approach as simple as possible, but as complex as necessary.
3.3 Inter- and intra-group comparison
As observed for diacetyl, the other diketone compounds in this case study also show dose-dependent responses at the transcriptome level, indicated by an increase in the number of differentially expressed genes and the absolute increase in log2FoldChange (Figures 3A, B).
The highest concentration doses tested for diacetyl and the two highest doses tested for 2,3-hexanedione and 3,4-hexanedione resulted in greater than twenty percent cell death. Therefore, these cytotoxic concentrations were excluded from subsequent transcriptome analyzes as it is expected that they predominantly exhibit DEGs and signaling pathways associated with apoptosis and necrosis. This phenomenon is known as a cytotoxic burst (Judson et al., 2016) and it is assumed that it does not provide relevant information about the compound-specific mechanism of action of the investigated compounds.
Within the α-diketones, a high correlation of up- or downregulated DEGs is observed, as indicated by a Spearman’s coefficient above 0.6 (Figure 3C). The β-diketone 2,4-pentanedione shows a very similar DEG profile compared to the α-diketones such as, e.g., visualized in the heat map of DEGs (Figure 3B) or the overall high Spearman coefficient of >0.5 compare to the α-diketones (Figure 3C); while the γ-diketone 2,5-hexanedione remains inactive up to the highest dose tested in vitro. In line with the absence of toxicity findings in vivo studies, the two negative control compounds, butanone and acetone, are inactive and do not induce DEGs.
Tunicamycin induces a total of 417 differentially expressed genes in a dose dependent manner. With increasing concentrations an increasing number of DEGs and also increasing absolute log2FoldChanges per DEG are observed. The DEGs expression pattern of tunicamycin differs remarkably from the one observed for the α- and β-diketones (Figure 3C; Spearman correlation score <0.4). 144 DEGs of tunicamycin are not differentially expressed in the α- and β-diketones, whereas 137 DEGS are differentially expressed in both analyses but with opposite log2FoldChange (Figures 3A–C). 230 DEGs, almost half of the DEGs of tunicamycin, are in common with the DEGs seen for α- and β-diketones and show similar up and downregulation.
The total number of pathways for the active diketones includes 861 different pathways and for tunicamycin 293 pathways. Again, the pathways with highest pathway scores are compared between α-, β-diketones, diacetyl and tunicamycin to better understand similarities and differences in biological processes. A literature review was performed to assign a mode of action to the best pathways (Table 2 showing these 10 top regulated pathways).
Most pathways associate with α-diketones occur in at least one concentration level in each substance, including pathways that have been linked to inflammation, fibrosis, and drug metabolism (Table 2). The fibrosis pathways occur within all α-diketone substances, except for 3,4-hexanedione. A possible explanation for the absence of fibrotic pathways in the case of 3,4-hexanedione is that only one subcytotoxic concentration contributed to the analysis.
The β-diketone 2,4-pentanedione shares 119 out of 688 (union) pathways with the α-diketones, but derives overall a lower number of active pathways (308 pathways, Figure 2D; Table 2). Also in this case, the fibrosis pathways were not identified within the top regulated pathways.
Of the top ten pathways for tunicamycin, seven could be associated with protein folding, consistent with the known mechanism of action. There is only one pathway that overlaps with the diketone (R-HAS-1461973) and it is related to immune responses (Bevins, 2006).
3.4 Upstream analysis
An upstream analysis for individual compounds and the group of α-diketones investigated the regulation of the observed DEGs via transcription factors (TFs) and master regulators (MRs) (Table 3).
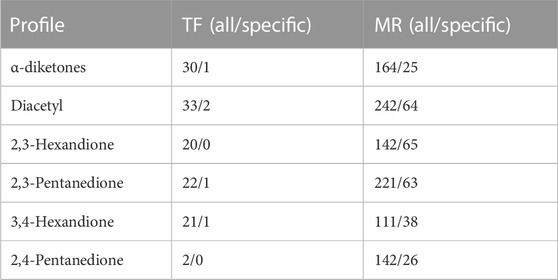
TABLE 3. Number of transcription factors (TFs) and master regulators (MRs) for different gene profiles; all-total number of identified TFs/MRs; specific - number remaining when TFs/MRs after exclusion of TFs/MRs detected for tunicamycin, acetone and butanone.
Significant binding site enrichment led to comparable numbers of postulated TFs, ranging from 33 TFs for diacetyl to 20 TFs for 2,3-hexanedione (Table 3, TF (all)); whereas the number of MRs differ a bit more ranging from 242 master regulators for diacetyl to 111 for 3,4-Hexandione (Table 3; MR (all)). In order to obtain more specific TFs and MRs per group/compound, the TFs and MRs also obtained from the negative control compounds acetone and butanone were not taken into account. Also, TFs and MRs shared with tunicamycin, which has a different mode of action, were not considered. For the α-diketones, the number of specific TFs and MRs decreased to 5 and 33, respectively. Diacetyl showed most specific TFs (N = 2) and MRs (N = 64) (Table 3). Overall, a total of three different specific transcription factors were identified (Table 3).
All three specific postulated TFs are known to be expressed in the human lung according to protein atlas version 21.0 (Table 4). All specific transcription factors were found in profiles for the alpha-diketones. Two are associated with pulmonary fibrosis (NKX2-1 and GATA6), another is involved in various cellular stress signaling pathways (ATF5).

TABLE 4. The specific transcription factors with expression in the lung, the profiles in which they occurred and the mode of action to which they could be assigned.
Over all profiles 145 specific master regulators were found. Due to this high number, it was analyzed whether regulators for the well-known mode of action can be found within the α-diketones profile by considering master regulators that appeared in at least four gene profiles and appeared in the α group profile or diacetyl compound profile (Table 5). Of these six master regulators, three master regulators are found to be associated with pulmonary fibrosis (ERBB2, EGFR and FGFR2) and one with inflammation (FYN). All three master regulators associated with lung fibrosis are also included in the β-diketone compound profile.
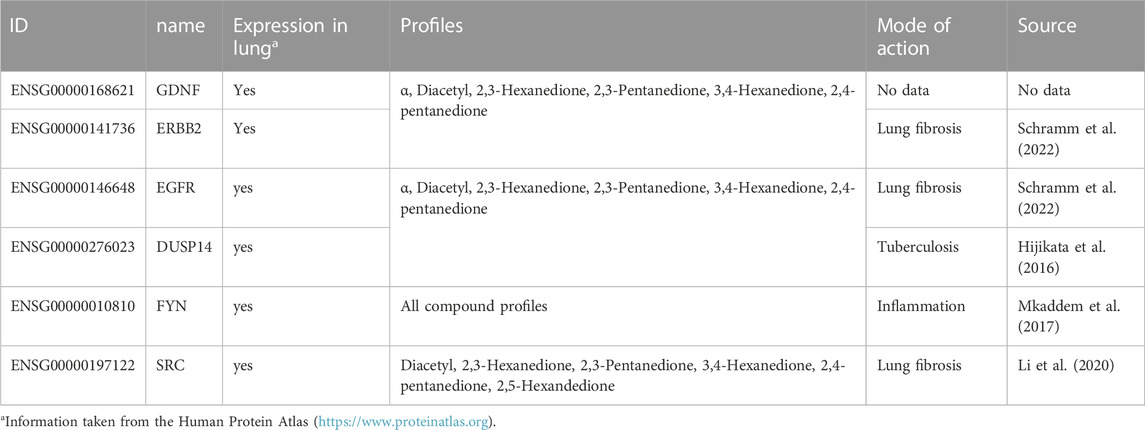
TABLE 5. Master regulators present in at least four gene profiles and present in the diacetyl compound profile or the α group profile.
All diketone profiles examined show that the most common specific MRs support a common mechanism of action, and this is the well-known MoA.
3.5 Reconstructed networks
Another challenge in the read across assessment is the visualization of similarities with regard to the observed mode of actions.
In order to address this question, reconstructed protein networks were developed for three different biological processes, namely, lung fibrosis, inflammation, and apoptosis. Each node in the network represents a protein which plays a role in the three MoAs. These annotations are based on manually curated data from human stored in the Transpath database.
213 nodes are obtained specifically for pulmonary fibrosis, 429 for inflammation and 39 for apoptosis. Other nodes belong to more than one MoA. Seven nodes are present in all three biological processes, whereas 42 nodes intersect between lung fibrosis and apoptosis and seven nodes overlap between inflammation and lung fibrosis (Figure 4).
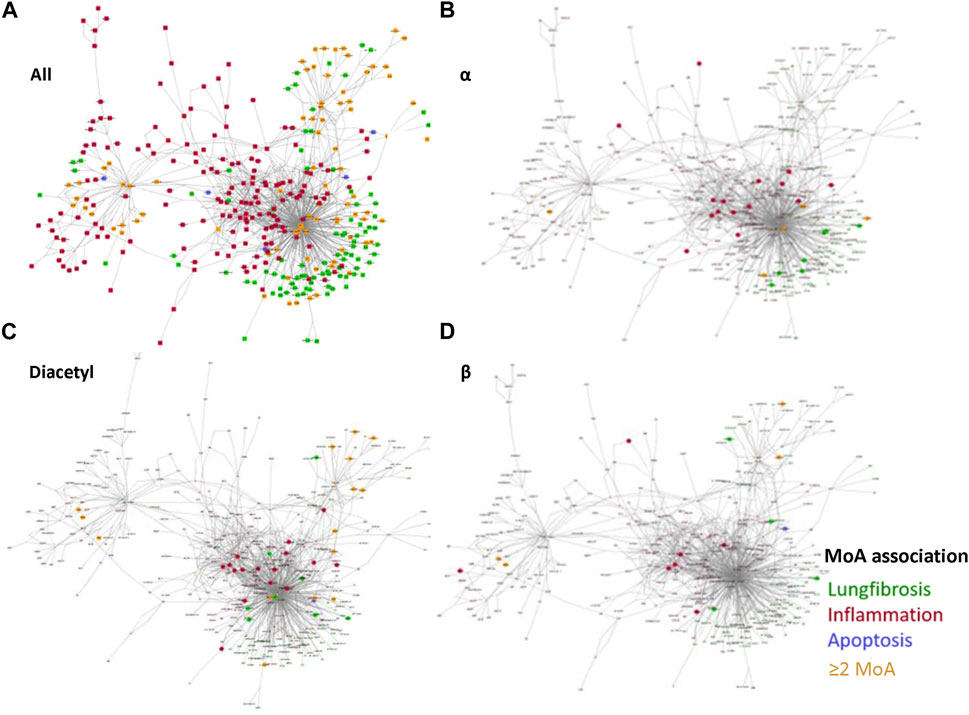
FIGURE 4. Reconstructed networks to visualize the obtained MRs in this read-across case study. (A) All MRs related to pulmonary fibrosis (green); inflammation (red) and apoptosis (blue). Nodes belonging to more than one of the three MoAs are colored in yellow. The same color code is applied to visualize the MoAs of three different profiles: (B) MRs identified in the α-diketone group, (C) MRs identified for the α-diketone diacetyl, and (D) MRs identified for the β-diketone 2,4-pentanedione.
To visualize common MoAs per group and compound profile, the master regulators were mapped to the nodes of the network.
The master regulators of the alpha group profile map primarily to the nodes for inflammatory processes, but also to multiple nodes associated with pulmonary fibrosis (Figure 4B).
Diacetyl is shown as one example representing the outcomes of the four different alpha diketone profiles. Diacetyl show a large number of nodes associated to inflammation and also some associated to pulmonary fibrosis (Figure 4C). Similar results were obtained for the three other alpha compound profiles (data not shown). Compared to the alpha-diketone profiles, the beta-diketone profile shows a smaller number of nodes, which are primarily associated inflammatory processes (Figure 4D).
4 Discussion
Even though RAx is a well-known and often used approach in regulatory chemical risk assessment to fill a data gap and to avoid animal testing (ECHA, 2020), it is often not accepted by regulatory agencies. One reason for this is a lack of evidence for common toxicodynamic properties within the grouped compounds, as in vivo studies reveal apical findings whose underlying mechanisms are often unknown (Ball et al., 2016).
There is therefore a need to integrate mechanistic data into read-across assessments, to provide evidence that the grouped compounds will cause similar toxic effects in the human organism or a consistent trend. An open question is how mechanistic data from omics approaches such as transcriptome data, can support the assessment of a similar mode of action.
Similarity assessment is highly dependent on the problem formulation. Similarity for genotoxic compounds will need other evidence compared to compounds which inhibit specific enzymes, like organophosphates inhibiting cholesterinesterase. The main question is therefore, to what extent are compounds similar in relation to which endpoint?
Similarity scores can be calculated in many different ways. This is a well-known phenomenon, e.g., from chemical similarity assessments, where binary data on the presence or absence of unique and common molecular features are used to calculate scores with simple algorithms such as Tanimoto or Dice (Willett et al., 1998). A similar simplistic approach could be used for transcriptome data comparing the absence of presence of unique and shared DEGs, pathways or regulated proteins/master regulators. Similarity scores alone do, however, not evaluate the underlying mechanisms and can therefore be probably considered as starting point of the assessment assuming that similar gene profiles indicate a regulation of similar biological processes. Low similarity on the gene level, does however, not directly indicate a dissimilar mode of action, as a pathway enrichment or upstream analysis might reveal that same biological processes regulated. Transcriptome data need thus other analyses strategies compared to similarity assessment of physico-chemical parameters.
This case study provides further insights into the assessment of mode of actions and similarity within grouped compounds by using transcriptome data. We believe that case studies such as this are necessary to develop analysis strategies and acceptance criteria, in particular the level of evidence considered sufficient to establish similarity.
To date, it is not known, which cellular assay is most appropriate to investigate mechanistic data for read-across assessments. The recently published EUTOXRISK read-across strategy recommends that the in vivo data and potentially available AOPs shall guide and inform the NAM testing strategy (Escher et al., 2019). For this case study on compounds that might induce lung fibrosis, primary human lung epithelial cells were chosen, as they are relatively close to the human in vivo situation. A comparison to other test systems was not carried out, but it is likely that other models like 3D cell, ex vivo or co-culture models could also give valuable information. A systematic comparison of test systems differing with regard to complexity could help to distinguish between problems in which a relatively simple cell test already provides sufficient evidence and those in which more complex test systems are required. This was however, not in the scope of the current case study.
In our case study, the group of four α-diketones show a highly similar expression pattern of DEGs. A large number of shared genes were upregulated or downregulated after both single and for diacetyl also after repeated exposure and across different concentrations. This DEG pattern clearly differed from those of tunicamycin, as shown by Spearman correlation, in agreement with its different mode of action. These data confirm the recent described hypothesis that gene expression data, even from short term in vitro assays, can serve as first indication of similar biological properties (Escher et al., 2019; Vrijenhoek et al., 2022). However, despite their completely different MoA, tunicamycin and the a-diketones has about 230 genes in common. It can be hypothesized that these DEGs represent to a large extent adaptive cellular responses to any kind of chemical stressor. This hypothesis has to be further investigated by testing compounds with different MoAs and could help to distinct unspecific from MoA-specific cellular responses.
The β-diketone caused a very similar gene expression pattern, thus indicating that this compound has a very similar mode of action as compared to the α-diketones. This finding is in good agreement with the available data from preclinical studies, in which the β-diketone also induced pulmonary fibrosis.
The γ-diketone and the two negative compounds were inactive up to the highest in vitro tested concentration. An analysis of biological similarity is therefore not possible based on transcriptome data. Two hypotheses can be made to explain the observed inactivity, the compounds might either be less potent compared to the other diketones or has another mode of action. The latter seems to be most probable for the γ-diketone, which induces neurotoxic effects after oral exposure in rodent (Dorry et al., 2020) studies (Ichihara et al., 2019). The transcriptome data did however not help to elucidate the difference. A statement on a similar or dissimilar mechanism of action cannot be made based on inactivity, a finding that was also recently reported by Vrijenhoek et al. (2022).
Transcriptome data can also be used to investigate potency difference between the grouped compounds by analyzing benchmark concentrations per DEG or signaling pathway (Phillips et al., 2019). In this case study a quantitative assessment of potency differences was not possible, mainly because of the non-optimal dose selection. Some of the tested concentrations already induced cytotoxicity and the number of remaining available concentrations were insufficient for benchmark modelling. This aspect needs to be further addressed in future investigations.
A pathway and an upstream analysis were carried out to investigate the biological processes associated with the observed DEGs. The pathway analyses, just like the DEG analysis, revealed common most active pathways between the alpha- and beta-diketones and a distinct demarcation from the pathways to tunicamycin. The S1500+ panel measured in this read-across case study contains a relatively small number of genes, which are selected because of their particularly responsiveness. A classic signaling pathway enrichment analysis was therefore not possible due to the relatively high number of DEGs compared to the number of genes measured. One way to deal with this problem is to extrapolate the gene set to the entire transcriptome (Mav et al., 2020) or set thresholds to set a minimum number of DEGs per pathway (Ramaiahgari et al., 2019). The latter approach was followed in this case study and was also successfully applied in the NTP and ToxCast project (Judson et al., 2016). In this work we calculated a pathway score based on the values for the differentially expressed genes. The advantage of the method we have chosen is that with the log2FoldChange and the p-value a qualitative evaluation was included, since the pathways with a particularly high average log2FoldChange and a small average p-value receive a higher pathway score and thus a higher value. As part of the upstream analysis and the subsequent visualization with the reconstructed networks, many common postulated TFs and MRs were found for the α− and β−diketones as well as tunicamycin compound. The identified TFs and MRs relate to many basic cell functions, including cell proliferation and stress responses, in addition to specific responses leading to the mechanism of action. To distinguish potentially non-specific adaptive cellular responses from more specific effect responses, a filtering step was applied in which TFs and MRs observed under multiple conditions and test compounds were excluded. Using this approach, a common mode of action within the α- and β-diketones was identified and distinctions to the tunicamycin compound could also be made.
Further examples, exploring, e.g., groups of compounds with more diverse mode of actions are needed to generalize this approach and to enhance the understanding about the specific and potentially adaptive TFs and MRs.
Also in the upstream analysis, the relatively small gene set of the S1500+ panel was a disadvantage, since potential postulated TFs and MRs that indicate the mode of action might have been missed.
Six AOPs on pulmonary fibrosis are currently described in the AOP-Wiki. These pathways all have in common that binding to different types of receptors lead to pulmonary fibrosis via inflammation and collagen accumulation (Society for the Advancement of AOPs, [2022]). Proteins associated with inflammation were identified in the reconstructed networks after a single exposure to the α- and β-diketones and are consistent with the AOPs. The reconstructed networks appear to be a useful tool to visualize mechanistic similarities and to infer an adverse outcome such as fibrosis based on early processes in vitro cell cultures. All active alpha- and beta-diketone substances show master regulators that can be assigned to pulmonary fibrosis and inflammation, which corresponds to their known mechanism of action. It is however noted that many nodes are inactive in the reconstructed networks. One possible explanation is that the short-term in vitro assays used in this case study do not show the full development process of fibrosis, like, e.g., collagen formation (Lynch, 2009). In addition, many different signaling pathways are known to lead to inflammation and fibrosis, such as EGFR signaling pathway, TORC2 pathway and TGFβ pathway (Chang et al., 2014; Schramm et al., 2022)) which do not all have to be relevant for diketones. One limitation of the reconstructed networks is, that they currently do not include information about the relationship between the obtained nodes such as inhibition or activation. Further work has to be done to include these interactions, to allow a more detailed mechanistic interpretation and to better discriminate between the biological processes.
5 Conclusion
Read-across assessments are always based on a read-across hypothesis, which is derived from the available in vivo data of data rich source compounds. For the purpose of this case study, we can assume that only diacetyl is a data rich source compound, and its toxicity data after repeated exposure shall be used to predict the structurally similar α,β, and γ-diketones. The read-across hypothesis is thus, that all structurally similar compounds will cause pulmonary fibrosis.
Overall, there is high concordance between the in vivo and in vitro results for the source compound diacetyl. This finding is necessary to gain confidence that the in vitro approach is able to address the read-across hypothesis.
This case study shows that transcriptome data can be used to indicate a common mechanism of action for compounds that are active at the level of gene expression. Inactive compounds cannot be evaluated. We have shown that there are similarities within the alpha and beta diketones at the level of gene expression and also in the biological interpretation. It was helpful to include tunicamycin, a compound with different mode of action, as it allows to discriminate better the differences in the obtained gene and pathway profiles.
The investigation of transcriptome data and their inference of an adverse outcome can thus substantiate the assessment of similar toxicodynamic properties with regard to a read-across hypothesis. We therefore believe that this approach can significantly reduce the uncertainty within read-across assessments and by this increase its acceptability. It has however, to be investigated further which level of similarity would be considered “acceptable” e.g., by regulatory bodies.
Data availability statement
The datasets presented in this study can be found in online repositories. The names of the repository/repositories and accession number(s) can be found below: https://www.ebi.ac.uk/biostudies/eu-toxrisk/studies?facet.eutoxrisk.project_part=cs8, S-TOXR1829, S-TOXR1814, S-TOXR1824, S-TOXR1825, S-TOXR1826, S-TOXR1827.
Author contributions
AB and SE contributed to conception and design of the study. JB, HV, TH, JK, BK, and DR performed the cell experiments. CD, MW, WZ, JK, DL, and SE contribute the development of the analysis methods. CD performed the analyses. CD and SE wrote the first draft of the manuscript. All authors wrote sections of the manuscript. All authors contributed to manuscript revision, read, and approved the submitted version.
Funding
This work has received funding from the European Union’s Horizon 2020 research and innovation program under grant agreements No. 681002 (EU-ToxRisk), No. 964537 (RISK-HUNT3R) and by the Ministry of Science and Culture of the State Lower Saxony (Germany) through the project FibrOmics.
Conflict of interest
JK was employed by GeneXplain GmbH.
The remaining authors declare that the research was conducted in the absence of any commercial or financial relationships that could be construed as a potential conflict of interest.
Publisher’s note
All claims expressed in this article are solely those of the authors and do not necessarily represent those of their affiliated organizations, or those of the publisher, the editors and the reviewers. Any product that may be evaluated in this article, or claim that may be made by its manufacturer, is not guaranteed or endorsed by the publisher.
Supplementary material
The Supplementary Material for this article can be found online at: https://www.frontiersin.org/articles/10.3389/ftox.2023.1155645/full#supplementary-material
Abbreviations
DEGs, differentially expressed genes; ECHA, European Chemical Agency; EFSA, European Food Safety Authority; GLP, good laboratory practice; hOED, human oral equivalent dose; MoA, mode of action; NAM, new approach methodology; RAx, read across.
References
Ball, N., Cronin, M. T. D., Shen, J., Blackburn, K., Booth, E. D., Bouhifd, M., et al. (2016). Toward good read-across practice (GRAP) guidance. ALTEX 33 (2), 149–166. doi:10.14573/altex.1601251
Barel, G., and Herwig, R. (2018). Network and pathway analysis of toxicogenomics data. Front. Genet. 9, 484. doi:10.3389/fgene.2018.00484
Bevins, C. L. (2006). Paneth cell defensins: Key effector molecules of innate immunity. Biochem. Soc. Trans. 34 (2), 263–266. doi:10.1042/BST20060263
Boei, J. J. W. A., Vermeulen, S., and Klein, B. (2017). Xenobiotic metabolism in differentiated human bronchial epithelial cells. Arch Toxicol 91, 2093–2105. doi:10.1007/s00204-016-1868-7
Borie, R., Funalot, B., Epaud, R., Delestrain, C., Cazes, A., Gounant, V., et al. (2021). NKX2.1 (TTF1) germline mutation associated with pulmonary fibrosis and lung cancer. ERJ open Res. 7 (4), 00356. doi:10.1183/23120541.00356-2021
Brass, D. M., and Palmer, S. M. (2017). Models of toxicity of diacetyl and alternative diones. Toxicology 388, 15–20. doi:10.1016/j.tox.2017.02.011
Bruckner, J., and Peterson, R. G. (1981). Evaluation of toluene and acetone inhalant abuse. II. Model development and toxicology. Toxicol. Appl. Pharmacol. 61 (3), 302–312. doi:10.1016/0041-008X(81)90351-3
Cavender, F., Casey, H., Salem, H., Swenberg, J., and Gralla, E. (1983). A 90-day vapor inhalation toxicity study of methyl ethyl ketone. Fundam. Appl. Toxicol. 3 (4), 264–270. doi:10.1016/S0272-0590(83)80138-9
Chang, W., Wei, K., Ho, L., Berry, G. J., Jacobs, S., Chang, C. H., et al. (2014). A critical role for the mTORC2 pathway in lung fibrosis. PLOS ONE 9 (8), e106155. doi:10.1371/journal.pone.0106155
Chen, H. F., and Hee, S. S. (1995). Ketone EC50 values in the Microtox test. Ecotoxicology and environmental safety 30 (2), 120–123. doi:10.1006/eesa.1995.1014
Dodd, D. E., Garman, R. H., Pritts, I. M., Troup, C. M., Snellings, W. M., and Ballantyne, B. (1986). 2,4-Pentanedione: 9-Day and 14-week vapor inhalation studies in fischer-344 rats. Toxicol. Sci. 7 (2), 329–339. doi:10.1016/0272-0590(86)90163-6
Dorry, J., Ansbro, B. O., Ornitz, D. M., Mutlu, G. M., and Guzy, R. D. (2020). FGFR2 is required for AEC2 homeostasis and survival after bleomycin-induced lung injury. Am. J. Respir. Cell Mol. Biol. 62 (5), 608–621. doi:10.1165/rcmb.2019-0079OC
EFSA (2021). EFSA Strategy 2027: Science, safe food, sustainability. Publications Office of the European Union, Luxembourg.
Escher, E., Kamp, H., Bennekou, H., Bitsch, A., Fisher, C., Graepel, R., et al. (2019). Towards grouping concepts based on new approach methodologies in chemical hazard assessment: The read-across approach of the EU-ToxRisk project. Archives Toxicol. 93 (12), 3643–3667. doi:10.1007/s00204-019-02591-7
European Commission. Regulation (2019). No 1907/2006 of the European Parliament and of the Council of 18 December 2006 concerning the registration, evaluation, authorisation and restriction of chemicals (REACH), establishing a European Chemicals Agency, amending Directive 1999/45/EC and repealing Council Regulation (EEC) No 793/93 and Commission Regulation (EC) No 1488/94 as well as Council Directive 76/769/EEC and Commission Directives 91/155/ EEC, 93/67/EEC, 93/105/EC and 2000/21/EC. Off J Euro Union 2006 L396, 1–849.
European Medicines Agency (2021). EMA implements new measures to minimise animal testing during medicines development. Available at: https://www.ema.europa.eu/en/news/ema-implements-new-measures-minimise-animal-testing-during-medicines-development, zuletzt aktualisiert am 29.09.2021.
García-Campos, M. A., Espinal-Enríquez, J., and Hernández-Lemus, E. (2015). Pathway analysis: State of the art. Front. physiology 6, 383. doi:10.3389/fphys.2015.00383
Gonzalez, F. J. (2005). Role of cytochromes P450 in chemical toxicity and oxidative stress: Studies with CYP2E1. Mutat. Res. 569, 101–110. doi:10.1016/j.mrfmmm.2004.04.021
Grimm, F. A., House, J. S., Wilson, M. R., Sirenko, O., Iwata, Y., and Wright, F. A. (2019). Multi-dimensional in vitro bioactivity profiling for grouping of glycol ethers. Regulatory toxicology and pharmacology : RTP 101, 91–102. doi:10.1016/j.yrtph.2018.11.011
Guengerich, F. P. (2006). Cytochrome P450s and other enzymes in drug metabolism and toxicity. AAPS J. 8 (1), E101–E111. doi:10.1208/aapsj080112
Gwinn, W. M., Flake, G. P., Bousquet, R. W., Taylor, G. J., and Morgan, D. L. (2017). Airway injury in an in vitro human epithelium-fibroblast model of diacetyl vapor exposure: Diacetyl-induced basal/suprabasal spongiosis. Inhal. Toxicol. 29 (7), 310–321. doi:10.1080/08958378.2017.1369604
Hijikata, M., Matsushita, I., Le Hang, N. T., Thuong, P. H., Tam, D. B., Maeda, , et al. (2016). Influence of the polymorphism of the DUSP14 gene on the expression of immune-related genes and development of pulmonary tuberculosis. Genes Immun. 17 (4), 207–212. doi:10.1038/gene.2016.11
Ichihara, G., Amarnath, V., Valentine, H. L., Takeshita, T., Morimoto, K., Sobue, T., et al. (2019). Pyrrole adducts in globin and plasma of workers exposed to hexane. Int. archives Occup. Environ. health 92 (6), 873–881. doi:10.1007/s00420-019-01430-7
Judson, R., Houck, K., Martin, M., Richard, A. M., Knudsen, T. B., Shah, I., et al. (2016). Analysis of the effects of cell stress and cytotoxicity on in vitro assay activity across a diverse chemical and assay space. Toxicol. Sci. 153 (2), 409. doi:10.1093/toxsci/kfw148
Karahalil, B. (2016). Overview of systems biology and omics technologies. Current medicinal chemistry 23 (37), 4221–4230. doi:10.1016/j.yrtph.2018.11.011
Knight, D. J., Deluyker, H., Chaudhry, Q., Vidal, J-M., and de Boer, A. (2021). A call for action on the development and implementation of new methodologies for safety assessment of chemical-based products in the EU - a short communication. Regul. Toxicol. Pharmacol. RTP 119, 104837. doi:10.1016/j.yrtph.2020.104837
Kohl, M., Wiese, S., and Warscheid, B. (2011). Cytoscape: Software for visualization and analysis of biological networks. Methods Mol. Biol. 696, 291–303. doi:10.1007/978-1-60761-987-1_18
Kolpakov, F., Poroikov, V., Selivanova, G., and Kel, A. (2011). GeneXplain—identification of causal biomarkers and drug targets in personalized cancer pathways. Journal of biomolecular techniques: JBT 22, 16.
Korthagen, N. M., van Moorsel, C. H. M., Barlo, N. P., Kazemier, K. M., Ruven, H. J. T., and Grutters, J. C. (2012). Association between variations in cell cycle genes and idiopathic pulmonary fibrosis. PloS one 7 (1), e30442. doi:10.1371/journal.pone.0030442
Koschmann, J. (2014). "Upstream Analysis": An Integrated Promoter-Pathway Analysis Approach to Causal Interpretation of Microarray Data. Microarrays (Basel) 4, 270-86.
Krull, M., Pistor, S., Voss, N., Kel, A., Reuter, I., Kronenberg, D., et al. (2006). Transpath: An information resource for storing and visualizing signaling pathways and their pathological aberrations. Nucleic acids Res. 34, D546–D551. doi:10.1093/nar/gkj107
Leppäranta, O., Pulkkinen, V., Koli, K., Vähätalo, R., Salmenkivi, K., Kinnula, V. L., et al. (2010). Transcription factor GATA-6 is expressed in quiescent myofibroblasts in idiopathic pulmonary fibrosis. Am. J. Respir. Cell Mol. Biol. 42 (5), 626–632. doi:10.1165/rcmb.2009-0021OC
Li, H., Zhao, C., Tian, Y., Lu, J., Zhang, G., Liang, , et al. (2020). Src family kinases and pulmonary fibrosis: A review. Biomed. Pharmacother. = Biomedecine Pharmacother. 127, 110183. doi:10.1016/j.biopha.2020.110183
Love, M. I., Huber, W., and Anders, S. (2014). Moderated estimation of fold change and dispersion for RNA-seq data with DESeq2. Genome biology 15 (12), 550. doi:10.1186/s13059-014-0550-8
Lynch, D. A. (2009). Lung disease related to collagen vascular disease. J. Thorac. Imaging 24 (4), 299–309. doi:10.1097/RTI.0b013e3181c1acec
Mav, D., Phadke, D. P., Balik-Meisner, M. R., Merrick, B. A., Auerbach, S., Niemeijer, M., et al. (2020). Utility of extrapolating human S1500+ genes to the whole transcriptome: Tunicamycin case study. Bioinforma. Biol. insights 14, 1177932220952742. doi:10.1177/1177932220952742
Mav, D., Shah, R. R., Howard, B. E., Auerbach, S., Bushel, P. R., Collins, J. B., et al. (2018). A hybrid gene selection approach to create the S1500+ targeted gene sets for use in high-throughput transcriptomics. PloS one 13 (2), e0191105. doi:10.1371/journal.pone.0191105
Mkaddem, B., Murua, A., Flament, H., Titeca-Beauport, D., Bounaix, C., Danelli, L., et al. (2017). Lyn and Fyn function as molecular switches that control immunoreceptors to direct homeostasis or inflammation. Nat. Commun. 8 (1), 246. doi:10.1038/s41467-017-00294-0
Pawar, G., Madden, J. C., Ebbrell, D., Firman, J. W., and Cronin, M. T. D. (2019). In Silico Toxicology Data Resources to Support Read-Across and (Q)SAR. Frontiers in pharmacology 10, 561. doi:10.3389/fphar.2019.00561
Phillips, J. R., Svoboda, D. L., Tandon, A., Patel, S., Sedykh, A., Mav, D., et al. (2019). BMDExpress 2: Enhanced transcriptomic dose-response analysis workflow. Bioinformatics 35 (10), 1780–1782. doi:10.1093/bioinformatics/bty878
Pistollato, F., Madia, F., Corvi, R., Munn, S., Grignard, E., Paini, A., et al. (2021). Current EU regulatory requirements for the assessment of chemicals and cosmetic products: Challenges and opportunities for introducing new approach methodologies. Archives Toxicol. 95 (6), 1867–1897. doi:10.1007/s00204-021-03034-y
Posternak, J. M., Linder, A., and Vodoz, C. A. (1969). Toxicological tests on flavouring matters. Food Cosmet. Toxicol 7, 405-407.
Quintana, F. J., and Cohen, I. R. (2005). Heat shock proteins as endogenous adjuvants in sterile and septic inflammation. J. Immunol. 175 (5), 2777–2782. doi:10.4049/jimmunol.175.5.2777
Ramaiahgari, C., Auerbach, S., Saddler, T. O., Rice, J. R., Dunlap, P. E., Sipes, N. S., et al. (2019). The power of resolution: Contextualized understanding of biological responses to liver injury chemicals using high-throughput transcriptomics and benchmark concentration modeling. Toxicol. Sci. 169 (2), 553–566. doi:10.1093/toxsci/kfz065
Ritter, D., and Knebel, J. (2014). Investigations of the Biological Effects of Airborne and Inhalable Substances by Cell-Based In Vitro Methods: Fundamental Improvements to the ALI Concept. Advances in Toxicology 2014, 11. doi:10.1155/2014/185201
Schramm, F., Schaefer, L., and Wygrecka, M. (2022). EGFR signaling in lung fibrosis. Cells 11 (6), 986. doi:10.3390/cells11060986
Sebastian-Leon, P., Vidal, E., Minguez, P., Conesa, A., Tarazona, S., Amadoz, A., et al. (2014). Understanding disease mechanisms with models of signaling pathway activities. BMC Syst. Biol. 8, 121. doi:10.1186/s12918-014-0121-3
Spencer, P. S., and Schaumburg, H. H. (1975). Experimental neuropathy produced by 2,5-hexanedione--a major metabolite of the neurotoxic industrial solvent methyl n-butyl ketone. J. neurology, Neurosurg. psychiatry 38 (8), 771–775. doi:10.1136/jnnp.38.8.771
Stegmaier, P., Kel, A., and Wingender, E. (2017). geneXplainR: An R interface for the geneXplain platform. JOSS 2 (18), 412. doi:10.21105/joss.00412
van Wetering, S., van der Linden, A. C., van Sterkenburg, M. A., Rabe, K. F., Schalkwijk, J., and Hiemstra, P. S. (2000). Regulation of secretory leukocyte proteinase inhibitor (SLPI) production by human bronchial epithelial cells: increase of cell-associated SLPI by neutrophil elastase. J Investig Med 48(5), 359–366.
Vrijenhoek, N. G., Wehr, M. M., Kunnen, S. J., Wijaya, L. S., Callegaro, G., and Moné, M. J. (2022). Application of high-throughput transcriptomics for mechanism-based.
Wang, H. (2015). Tunicamycin-induced unfolded protein response in the developing mouse brain. Toxicology and applied pharmacology 283.3 (2015), 157-167.
Weijer, R., Broekgaarden, M., van Golen, R. F., Bulle, E., Nieuwenhuis, E., Jongejan, A., et al. (2015). Low-power photodynamic therapy induces survival signaling in perihilar cholangiocarcinoma cells. BMC cancer 15, 1014. doi:10.1186/s12885-015-1994-2
Willett, P., Barnard, J. M., and Downs, G. M. (1998). Chemical similarity searching. J. Chem. Inf. Comput. Sci. 38 (6), 983–996. doi:10.1021/ci9800211
Wingender, E., Crass, T., Hogan, J. D., Kel, A. E., Kel-Margoulis, O. V., and Potapov, A. P. (2007). Integrative content-driven concepts for bioinformatics "beyond the cell. J. Biosci. 32 (1), 169–180. doi:10.1007/s12038-007-0015-2
Yang, H., Niemeijer, M., van de Water, B., and Beltman, J. B. (2020). ATF6 is a critical determinant of CHOP dynamics during the unfolded protein response. iScience 23 (2), 100860. doi:10.1016/j.isci.2020.100860
Yeakley, J. M., Shepard, P. J., Goyena, D. E., VanSteenhouse, H. C., McComb, J. D., and Seligmann, B. E. (2017). A trichostatin A expression signature identified by TempO-Seq targeted whole transcriptome profiling. PLOS ONE 12 (5), e0178302. doi:10.1371/journal.pone.0178302
Zaccone, E. J., Thompson, J. A., Ponnoth, D. S., Cumpston, A. M., Goldsmith, W. T., Jackson, M. C., et al. (2013). Popcorn flavoring effects on reactivity of rat airways in vivo and in vitro. J. Toxicol. Environ. health Part A 76 (11), 669–689. doi:10.1080/15287394.2013.796302
Keywords: read across, new approach methodology, transcriptomics, TempO-seq, PBEC, protein network analysis
Citation: Drake C, Wehr MM, Zobl W, Koschmann J, De Lucca D, Kühne BA, Hansen T, Knebel J, Ritter D, Boei J, Vrieling H, Bitsch A and Escher SE (2023) Substantiate a read-across hypothesis by using transcriptome data—A case study on volatile diketones. Front. Toxicol. 5:1155645. doi: 10.3389/ftox.2023.1155645
Received: 31 January 2023; Accepted: 17 April 2023;
Published: 03 May 2023.
Edited by:
Logan J. Everett, United States Environmental Protection Agency, United StatesReviewed by:
Kevin Gerrish, National Institute of Environmental Health Sciences (NIH), United StatesJessica Murray, Center for Public Health and Environmental Assessment, United States
Copyright © 2023 Drake, Wehr, Zobl, Koschmann, De Lucca, Kühne, Hansen, Knebel, Ritter, Boei, Vrieling, Bitsch and Escher. This is an open-access article distributed under the terms of the Creative Commons Attribution License (CC BY). The use, distribution or reproduction in other forums is permitted, provided the original author(s) and the copyright owner(s) are credited and that the original publication in this journal is cited, in accordance with accepted academic practice. No use, distribution or reproduction is permitted which does not comply with these terms.
*Correspondence: Christina Drake, Y2hyaXN0aW5hLmRyYWtlQGl0ZW0uZnJhdW5ob2Zlci5kZQ==