- 1Independent Consultant, Kennett Square, PA, United States
- 2Innovatune, Padova, Italy
- 3Sanofi Preclinical Safety, Frankfurt, Germany
- 4Magnolia Toxicology Consulting, Traverse City, MI, United States
- 5Instem, Cambridge, United Kingdom
- 6Instem, Columbus, OH, United States
- 7Independent Consultant, Chapel Hill, NC, United States
- 8Medline Industries, Northfield, IL, United States
- 9Nestlé Research, Lausanne, Switzerland
- 10Chemotargets SL, Parc Científic de Barcelona, Barcelona, Spain
- 11ToxStrategies LLC, Asheville, NC, United States
- 12ForthTox, Linlithgow, United Kingdom
- 13RTice Consulting, Hillsborough, NC, United States
In silico toxicology protocols are meant to support computationally-based assessments using principles that ensure that results can be generated, recorded, communicated, archived, and then evaluated in a uniform, consistent, and reproducible manner. We investigated the availability of in silico models to predict the carcinogenic potential of pregabalin using the ten key characteristics of carcinogens as a framework for organizing mechanistic studies. Pregabalin is a single-species carcinogen producing only one type of tumor, hemangiosarcomas in mice via a nongenotoxic mechanism. The overall goal of this exercise is to test the ability of in silico models to predict nongenotoxic carcinogenicity with pregabalin as a case study. The established mode of action (MOA) of pregabalin is triggered by tissue hypoxia, leading to oxidative stress (KC5), chronic inflammation (KC6), and increased cell proliferation (KC10) of endothelial cells. Of these KCs, in silico models are available only for selected endpoints in KC5, limiting the usefulness of computational tools in prediction of pregabalin carcinogenicity. KC1 (electrophilicity), KC2 (genotoxicity), and KC8 (receptor-mediated effects), for which predictive in silico models exist, do not play a role in this mode of action. Confidence in the overall assessments is considered to be medium to high for KCs 1, 2, 5, 6, 7 (immune system effects), 8, and 10 (cell proliferation), largely due to the high-quality experimental data. In order to move away from dependence on animal data, development of reliable in silico models for prediction of oxidative stress, chronic inflammation, immunosuppression, and cell proliferation will be critical for the ability to predict nongenotoxic compound carcinogenicity.
1 Introduction
Cancer is a multifaceted, multimodal disease. Whereas advances in cancer treatment over the last five decades have been remarkable (Arnold, et al., 2019; Kratzer, et al., 2022), many causes of cancer in the human population are still largely unknown. Given that there are tens of thousands of chemicals in commerce that have not had adequate carcinogenicity testing, there is a need for a swift and reliable assessment of the carcinogenic potential of chemicals. Over 50 years after coming into common use, the 2-year rodent carcinogenicity bioassay is still considered by many regulatory authorities, legislative bodies, industrial entities and other authoritative groups to be the gold standard for assessment of carcinogenicity. This bioassay has many flaws, including low sensitivity, dose levels that are often irrelevant to human exposure, expense, and high animal usage (Cohen, 2017; Goodman, 2018; Madia, et al., 2019). The environmental and agrochemical sectors generally require 2-year rat and mouse studies for carcinogenicity assessment of new chemical entities, with varying levels of acceptance of mechanistic data to modify risk assessment across regulatory bodies. The 6-month transgenic mouse assay has largely supplanted the 2-year mouse study in pharmaceutical development, and the International Council for Harmonisation of Technical Requirements for Pharmaceuticals for Human Use (ICH) S1B(R1) guideline allows for a weight of evidence (WoE) assessment to determine the need for a 2-year rat study. However, replacement of the bioassay with alternative methods including in vitro or computational tools has not been well accepted as a definitive tool for risk assessment and regulatory purposes.
There are many theories about the origins of cancer and multiple attempts have been made to categorize chemicals into classes of carcinogens for the purposes of hazard or risk assessment (Doll and Peto, 1982; Wolf et al., 2019). Classification systems such as those from the International Agency for Research on Cancer (IARC) and the US National Toxicology Program (NTP) are generally hazard classification systems, with little account for exposure to assess risk. Other classification systems focus mode of action (MOA) (Boobis et al., 2006; Cohen et al., 2019) and also account for exposure where key characteristics are altered to give an estimate of risk. Smith et al. (2016, Smith et al., 2020) describe the ten key characteristics (KC) of carcinogens as an approach to evaluate mechanistic evidence in cancer hazard identification. Many of the KCs described by Smith et al. are also part of the assessment system proposed by Cohen et al. (2019) but are described in more detail and with proposed experimental methods of assessment in the Smith papers. The KCs are a method that can be used to organize data relevant to the MOA of a carcinogen, and to provide a systematic evaluation of cancer hazards.
Tice, et al. (2021) extended these concepts and described the current status and future needs for in silico carcinogenicity assessment based on the attributes of the KCs of carcinogens (Smith, et al., 2016; Smith, et al., 2020). In this context, in silico (computational) approaches refer to different methodologies that aim at predicting adverse effects from the structure of molecules. These approaches are based on structure activity relationships (SARs) between structural information and biological activity; SARs may be either qualitative or quantitative in nature and are commonly referred to as (Q)SARs. Tice, et al. (2021) make clear that additional in silico models are needed to describe many of the KCs for carcinogens in order to expedite the analysis of the potential carcinogenicity of the many thousands of chemicals in commerce (Tice, et al., 2021). Moreover, the ultimate goal is the integration of such in silico approaches in a hazard assessment framework of carcinogens in a transparent, consistent, and defendable manner.
This view follows the in silico toxicology (IST) protocol initiative for the development of standardized approaches for the prediction of toxicity from a chemical structure (Myatt, et al., 2018; Myatt, et al., 2022). Similar to the published test guidelines for in vivo or in vitro test methods, the IST protocols are meant to support in silico assessments using principles that ensure that results can be generated, recorded, communicated, archived, and then evaluated in a uniform, consistent, and reproducible manner. The protocols define the effects and/or mechanisms to be predicted by the in silico methods as part of the assessment of interest. They describe how the data are combined to assess one or more endpoints including creation of an overall confidence score based on a weight of evidence. To further illustrate the needs for in silico model development in support of carcinogenicity assessment as well as to gain knowledge for the development of a corresponding IST protocol, an international consortium has undertaken a series of case studies of chemicals and drugs with varying carcinogenicity bioassay outcomes. This consortium workgroup builds upon the efforts that were undertaken to evaluate the extent to which in silico models exist for each of the 10 KCs (Tice, et al., 2021).
Here, we report on pregabalin, a compound that is carcinogenic in mice via a nongenotoxic mechanism (Pegg, et al., 2012). The overall goal of this exercise is to test the ability of in silico models to predict nongenotoxic carcinogenicity with pregabalin as a case study. Available experimental data and computational model predictions are organized in terms of the KC framework, and gaps in the availability of models are discussed. The 10 KCs conceptual framework (Smith, et al., 2016; Smith, et al., 2020) offers a construct that supports the expert review of available evidence, with a focus on the ability of in silico tools to predict the outcome of carcinogenicity studies. Smith, et al. (2020) also present a summary of in vitro assays and in vivo biomarkers that can be used to investigate certain aspects of modes of action (MOAs) and the KCs.
Insights from Adverse Outcome Pathways are also integrated in such a process. Nongenotoxic rodent carcinogenicity is often considered to be non-relevant to human health (Silva Lima and van der Laan, 2000), but significant experimental research is needed to substantiate that claim. The use of in vitro and in silico tools to support this process could increase the speed of research and decrease animal use.
The following sections describe, for each of the ten KCs of carcinogens, a summary of the available data for pregabalin, computational modeling of the KC, and modeling and data gaps. Additional details of these data can be found in the references cited. Since pregabalin was not carcinogenic in rats at up to 14 (males) and 24 (females) times the maximum recommended human dose, data described in the KC focus on data from studies in mice or in vitro. Confidence in these data and computational approaches is assigned based on criteria described by Johnson et al. (Johnson, et al., 2022).
2 Materials and methods
2.1 Chemical information
Pregabalin (CAS Number 148553-50-8; Figure 1) is a small molecule ligand of the auxiliary α2δ subunit site of certain voltage-dependent calcium channels (VDCCs), and acts as an inhibitor of α2δ subunit-containing VDCCs. It is used to treat seizures, neuropathic pain, and generalized anxiety disorders (Mico and Prieto, 2012; Alles, et al., 2020) and is globally available. The molecular weight of pregabalin is 159 g/mol and the log P is 1.3. It is freely soluble (high solubility) in water.
2.2 Metabolism data
Pregabalin is rapidly absorbed orally, with a bioavailability of approximately 80%. There is no significant metabolism in humans or other species, with the exception of the dog. The major in vivo metabolite is N-methyl pregabalin, accounting for <3% of drug-related material in most species, and approximately 45% of drug-related material in the dog. The principal route of excretion is in the urine. Pregabalin is not a CYP inhibitor in vitro at concentrations up to 1 mM (EMA, 2005).
2.3 Carcinogenicity data
During development, pregabalin was tested for potential carcinogenicity in 2-year bioassays in mice and rats (Pegg, et al., 2012). Pregabalin did not induce tumors in rats but did in mice. The induced tumors in mice were hemangiosarcomas, primarily in the spleen, liver, and bone marrow. Additional studies were performed to elucidate the mechanism of hemangiosarcoma formation and potential human relevance (Criswell, et al., 2012a; Criswell, et al., 2012b; Criswell, et al., 2012c). These studies showed that pregabalin induces hemangiosarcomas through increased hypoxia and endothelial cell (EC) proliferation in a species-specific manner. In addition to these studies on mice, rats were included in many studies to validate that the effects observed and the mode of action were specific to the mouse.
A search for potency (ChEMBL, 2022) values for pregabalin and structurally similar compounds was performed in ChEMBL (release 31). The Comparative Toxicogenomics Database (CTDB, 2022) was searched for data on pregabalin potentially related to mechanisms of carcinogenicity. The ClarityPV platform (CLARITY, 2023) was searched for neoplasm side effects associated with pregabalin use.
In silico predictions for pregabalin carcinogenicity and carcinogenicity potency were obtained from commercially and freely available in silico platforms (Leadscope Model Applier (v. 3.1.0-40), Derek Nexus 6.1.0, VEGA v. 1.3.10, Toxtree v.3.1.0, LAZAR v. 1.4.2).
2.4 Key characteristics
The available experimental data and in silico predictions for pregabalin were reviewed by organizing such information within the KC of carcinogens framework. Data were assessed in terms of the reliability score (RS) and relevance as discussed by Myatt et al. (Myatt, et al., 2018) and Johnson et al. (Johnson, et al., 2022), that, at the experimental level, may consider different factors such as compliance with guidelines, concordance with other studies, and/or deviations from test protocols (see Table 1). At the in silico prediction level, reliability refers to the extent that an in silico result is predictive of an experimental result. On the other hand, the expert conclusions that integrate and combine evidence from various experimental or in silico results (each of this can be associated with a specific RS) can be scored according to the confidence categories (high, medium, low, or no confidence), that have been specifically developed for a given toxicological assessment by Johnson et al. (Johnson, et al., 2022). Table 1 summarizes the scoring system adopted in the current work for assessing the reliability of available experimental data and in silico predictions (reliability scores) and for assessing the confidence of the conclusions related to the key characteristics (confidence categories).
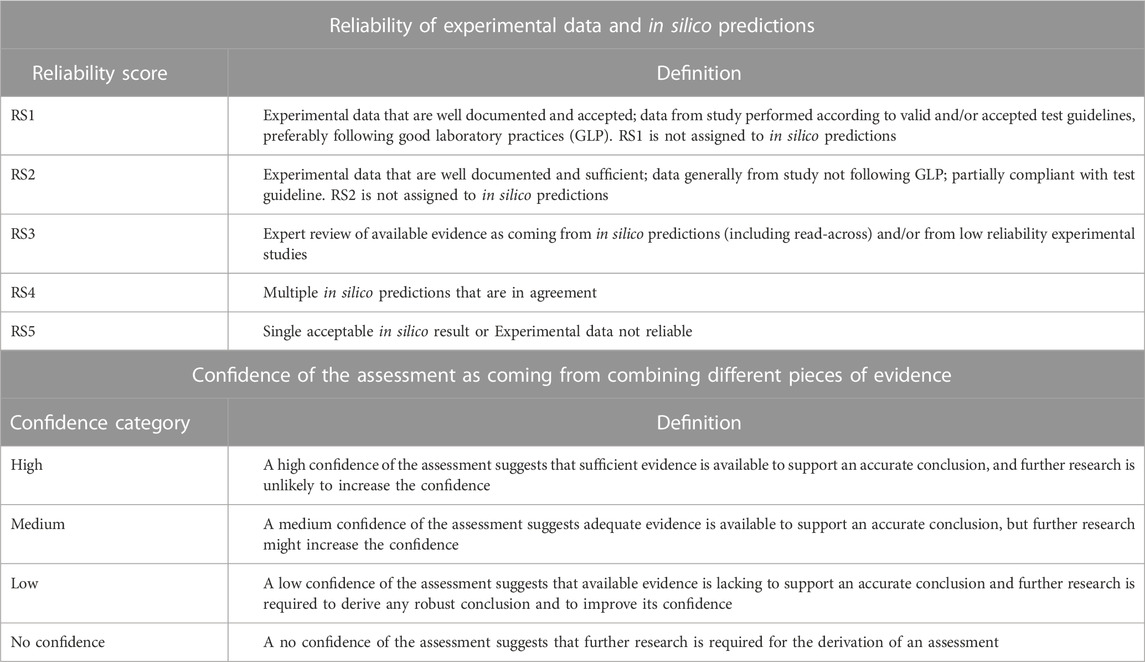
TABLE 1. Reliability scores (RSs) and confidence categories used to respectively assess data and conclusions on KCs in the present work. The RS is applied for assessing experimental data and in silico predictions (Myatt, et al., 2018; Johnson, et al., 2022); the RS framework integrates the Klimish scoring system for experimental data (Klimisch, et al., 1997). The confidence categories have been developed to grade the confidence of the assessment of a toxicological endpoint (Johnson, et al., 2022) and they can be applied here to grade the expert conclusions related to the key characteristics of carcinogens.
3 Results
3.1 Key characteristics
3.1.1 KC1: Is electrophilic or can be metabolically activated
3.1.1.1 Experimental data
Pregabalin is not electrophilic based on its absence of activity in bacterial mutagenicity assays that incorporate metabolic activation, which are considered to be an acceptable surrogate for the electrophilicity endpoint (Ashby and Tennant, 1988). Furthermore, the lack of significant metabolism in all species tested except for the dog supports a lack of concern about a possible electrophilic metabolite, as none could be generated.
3.1.1.2 In silico approaches
Only one metabolite of pregabalin, N-methylpregabalin, has been experimentally isolated and it is a minor component, comprising only a few percent of the dose in all species except the dog (Pharmapendium, 2022). In silico predictions for bacterial mutagenicity of N-methyl pregabalin suggested the absence of electrophilicity character. Details of the in silico predictions are reported in the Supplementary Material.
3.1.1.3 Reliability and confidence
The experimental data are assigned a reliability score of RS1. The in silico prediction for N-methyl pregabalin is assigned a reliability score of RS3. Given the reliability of available evidence and its corresponding relevance for the evaluation of electrophilicity, a medium confidence can be assigned to the conclusion that pregabalin is not electrophilic (or cannot be metabolized to active intermediates).
3.1.1.4 Data gaps
Standard regulatory metabolism studies were performed with pregabalin and are complete for animal species. Additional human metabolism data would increase the confidence in extrapolation of the animal data to humans and better clarify the human relevance. Specific in vitro experiments for electrophilicity endpoints would be useful.
3.1.2 KC2: Is genotoxic
3.1.2.1 Experimental data
Several genotoxicity studies using standard Organisation for Economic Co-operation and Development (OECD) test guideline assays were submitted to the U.S, Food and Drug Administration (FDA) during the registration of pregabalin (Pegg, et al., 2012). In bacterial reverse mutation assays, pregabalin was reported negative in Salmonella typhimurium strains TA-98, TA-100, TA-1535, and TA-1537 at maximal concentrations of 5,000 µg/plate, with and without metabolic activation, and in Escherichia coli WP2uvrA at maximal concentrations of 4,980 µg/plate. Additional genotoxicity studies have been conducted on pregabalin and are shown in the Supplementary Table S1 and support a lack of genotoxic activity.
Other experimental data can be evaluated within KC2. Based on an analysis of Tox21 high throughput screening data, at concentration up to 92 μM, pregabalin was negative for induction of ELG1 or p53 and was negative for differential cytotoxicity in the chicken cell DT40 assay using several DNA repair knockout isogenic strains (ICE, 2022). At the concentrations tested in these assays pregabalin was not cytotoxic, indicating that, according to OECD criteria, these tests would not be considered adequate in terms of the maximum concentration tested.
3.1.2.2 In silico approaches
A number of in silico models provide predictions that relate to genotoxicity including predictions for mutagenicity in bacteria and mouse lymphoma cells as well for the induction of both in vitro and in vivo chromosomal aberrations and micronuclei (Tice, et al., 2021). The in silico predictions leading to the genotoxicity assessment are shown in Figure 2 (the details of the corresponding predictions are reported in Supplementary Table S2). The genetic toxicology in silico protocol formulated by Hasselgren and co-workers (Hasselgren, et al., 2019) is used to integrate all of the available information related to genotoxicity. More specifically, the in silico predictions are combined with available experimental data from standardized tests to generate an overall endpoint call; in parallel, the corresponding reliability scores of the assessments are used to derive the overall endpoint confidence (Myatt, et al., 2018; Johnson, et al., 2022). Given the lack of significant metabolism for pregabalin in all species tested but the dog, and the knowledge that models and alerts for bacterial mutation based upon parent compounds using the Ames test (with and without metabolic activation) infers assessment of mutation by the metabolites, an analysis of the genotoxicity of possible metabolites was not conducted.
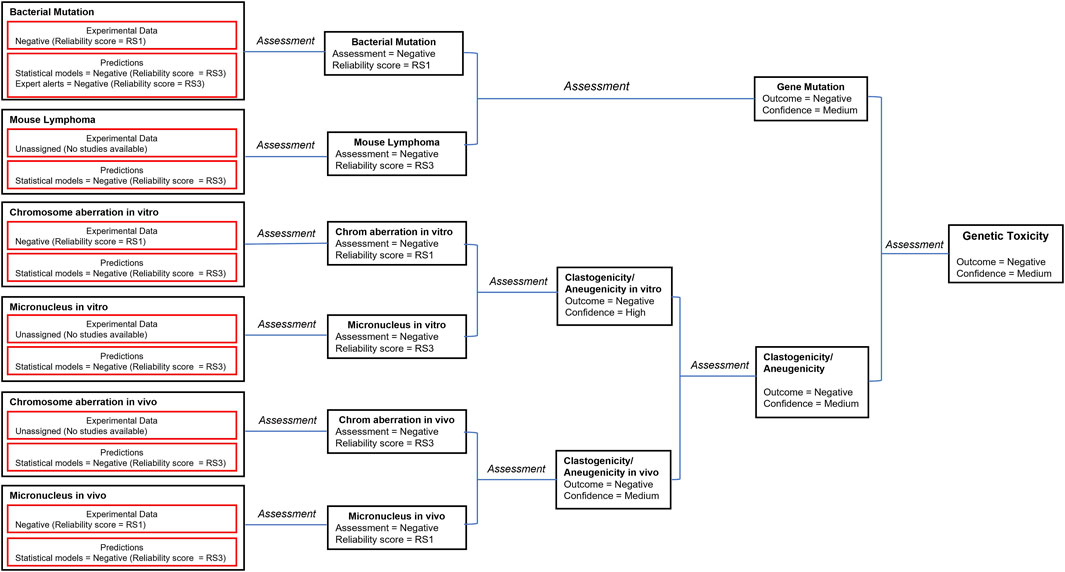
FIGURE 2. The in silico assessments most relevant to genotoxicity are combined with available experimental data according to the genetic toxicology in silico protocol (Hasselgren, et al., 2019). Selected models and corresponding reliability scores are shown in the figure. The details of available predictions are reported in the Supplementary Material. The reliability score (RS) of each prediction is documented in the Supplementary Material. This is used to derive the overall endpoint confidence (Myatt, et al., 2018; Johnson, et al., 2022) based on the published rules (Hasselgren, et al., 2019).
3.1.2.3 Reliability and confidence
The consensus outcome from the integration of the in silico genotoxicity models interrogated with the structure of pregabalin was that the compound was negative for genotoxicity (see Figure 2). Based on consideration of both the experimental results and the in silico predictions, the overall confidence is high to medium that pregabalin is not genotoxic, and pregabalin is not classified as a genotoxic compound.
3.1.2.4 Data gaps
Although some of the non-regulatory tests were conducted at lower concentrations, given the totality of the data no significant data gaps exist for genotoxicity endpoints.
3.1.3 KC3: Alters DNA repair or causes genomic instability
3.1.3.1 Experimental data
Pregabalin was inactive in the Tox21 DT40 assays that can reflect DNA repair capabilities (ICE, 2022) (Supplementary Table S3). However, this assay does not directly assess DNA repair, so no adequate data are available.
3.1.3.2 In silico approaches
There are no in silico methods available for evaluating this endpoint.
3.1.3.3 Reliability and confidence in the data
A confidence score cannot be assigned. Based on available evidence, a robust conclusion on whether pregabalin alters DNA repair or causes genomic instability cannot be derived.
3.1.3.4 Data gaps
Directly relevant studies in mammalian systems are needed to evaluate this KC.
3.1.4 KC4: Induces epigenetic alterations
3.1.4.1 Experimental data
No direct evidence was identified to link pregabalin to an epigenetic mode of action (LYRICA, 2018). Pregabalin functions as an anti-anxiety drug; however, the mode of action is not fully elucidated (LYRICA, 2018). Epigenetic drugs can be used to treat anxiety disorders (Peedicayil, 2020). So, it is possible that pregabalin treats anxiety by epigenetic therapy, but this relationship has yet to be proven. In addition, a number of reviews highlight the role of epigenetic mechanisms in the pathophysiology and treatment of chronic pain (Descalzi, et al., 2015; Geranton and Tochiki, 2015; Liang, et al., 2015). Therefore, although pregabalin is not currently linked to epigenetic mechanisms, there could be a yet undiscovered epigenetic mechanism in line with the therapeutic properties seen in pregabalin. This topic of epigenetic regulation of neurological activity is receiving increased research and regulatory attention (Banik, et al., 2017; EMA, 2018).
However, in contrast to this, Notartomaso et al. (Notartomaso, et al., 2017) studied different painkillers and suggested that pregabalin was not functioning via an epigenetic mechanism. In the study, mice were injected with pregabalin (30 mg/kg) over a series of different experiments. In this study (Notartomaso, et al., 2017) it was specifically chosen to use pregabalin as an active comparator; pregabalin’s known interaction with the α2δ subunit of voltage-sensitive Ca2+ channels was used to compare analgesia alongside drugs that enhance acetylation of histones or transcription factors.
There is very little experimental data directly linking pregabalin with any epigenetic interaction or modulation.
3.1.4.2 In silico approaches
There are no in silico methods for predicting the ability of pregabalin to induce epigenetic alterations.
3.1.4.3 Reliability and confidence
A confidence score cannot be assigned. Based on available evidence, a robust conclusion on whether pregabalin induces epigenetic alterations cannot be derived.
3.1.4.4 Data gaps
Expert literature review found no conclusive link documenting pregabalin with an epigenetic mechanism. As there are no models to address epigenetic modulation directly, this is a gap in our understanding, and, therefore, should be reflected in the confidence of the overall assessment.
3.1.5 KC5: Induces oxidative stress
3.1.5.1 Experimental data
A key event in the mechanism of action for pregabalin carcinogenicity is tissue hypoxia resulting from a sustained alkalosis (Criswell, et al., 2012a; Criswell, et al., 2012b). In mice, chronic tissue hypoxia leads to inflammation (discussed in detail in the next section) characterized by erythrophagocytosis, iron accumulation in macrophages and Kupffer cells, and activated macrophages that release reactive oxygen species (ROS). The inflammation then causes increases in tissue vascular endothelial growth factor (VEGF), platelet-derived growth factor (PDGF), and basic fibroblast growth factor (bFGF) which drive endothelial cell proliferation. Iron deposits in tissues can also lead to increases in ROS. These events do not occur in rats treated with pregabalin (Criswell, et al., 2012a).
Pregabalin was inactive in three p53 assays potentially related to oxidative stress listed in the National Toxicology Program’s Integrated Chemical Environment database (ICE, 2022) (Supplementary Table S3).
3.1.5.2 In silico approaches
In silico methods are available for some mechanisms that induce oxidative stress (reviewed in (Tice, et al., 2021)). While none were used for pregabalin, models for ROS generation and ARE/Nrf-2 activation could be useful to generate additional understanding of mechanisms involved in carcinogenesis.
3.1.5.3 Reliability and confidence
The experimental data are assigned an overall reliability score of RS2. Given this reliability and consideration of their relevance for the evaluation of oxidative stress, a high confidence can be assigned to the conclusion that pregabalin causes oxidative stress in vivo.
3.1.5.4 Data gaps
While the data are considered reliable and relevant, the amount of data on oxidative stress is not large. Additional in vitro and in silico studies could enhance the understanding of this MOA.
3.1.6 KC6: Induces chronic inflammation
3.1.6.1 Experimental data
Female B6C3F1/CrlBR mice received 1,000 mg/kg bw of pregabalin in the diet for up to 12 months or 5,000 mg/kg bw for up to 29 days (Criswell, et al., 2012c). Dysregulation of angiogenesis and resultant cell death due to chronic hypoxia induced a chronic inflammatory state as evidenced by an increase in activated platelets and an increase in Kupffer cells in the liver and iron-laden macrophages in the bone marrow and spleen in mice, but not in rats. According to the authors (data not shown (Criswell, et al., 2012a),), pregabalin treatment resulted in a dose- and time-dependent increase in activated macrophages in the bone marrow, spleen, and liver in mice–all tissues where hemangiosarcomas were observed. No increases were observed in rats. The authors also reported an increase in the absolute number of white blood cells in treated mice, but the relative distribution of cell types was similar in control and treated animals (data not shown (Criswell, et al., 2012c). Macrophage activation has been shown in other studies to result in cytokine release and subsequent generation of ROS (Corthals, et al., 2006) and platelet activation releases platelet-derived growth factor which is a known chemotactic agent for fibroblasts, vascular smooth muscle cells, and monocytes and can stimulate eosinophils to form superoxide anions (Mannaioni, et al., 1997). In a companion study, addition of vitamin E, an antioxidant, to the mouse diet significantly decreased EC proliferation in mice treated with pregabalin, but not in untreated mice, suggesting that pregabalin treatment was activating EC growth pathways in the mouse, most likely through ROS and inflammation pathways (Criswell, et al., 2012b).
3.1.6.2 In silico approaches
While some in silico models exist for various parts of inflammatory cascades, these mechanisms are complex and the understanding of factors that promote and sustain the effect are not fully known.
3.1.6.3 Reliability and confidence
The experimental data are overall assigned a reliability score of RS2. Given this reliability and consideration of their corresponding relevance for the evaluation of chronic inflammation, a high to medium confidence can be assigned to the conclusion that pregabalin induces chronic inflammation.
3.1.6.4 Data gaps
Although inflammation is evident with the increase in activated macrophages in liver, spleen, and bone marrow and activated platelets in the peripheral circulation, specific chemical markers of inflammation (i.e., evidence of cytokine/chemokines or myeloperoxidase in the area) were not reported. Furthermore, the presence of ROS was not experimentally verified by direct measurements, although addition of an antioxidant to the diet (vitamin E) provided indirect evidence that these reactive species are required for tumor formation.
3.1.7 KC7: Is immunosuppressive
3.1.7.1 Experimental data
Data on whether pregabalin exerts direct immunomodulatory effects in mammalian systems is mixed, but the weight of evidence suggests it likely has no direct immunosuppressive effects. Minimal to mild effects on the lymphoid system were observed only at very high doses (≥15-fold the human exposure) in rats and at high doses in monkeys. In Health Authority reviews of the data submitted for registration (US)/marketing (EU), dermatopathy on the tail skin of rats and monkeys was noted in nearly all studies; however, skin lesions in other areas were not reported and the effect was not recapitulated in clinical trials. Follow-up studies (LYRICA, 2018) (Pfizer Report 745-03326) (Pfizer Report 250-01888) evaluating the time course of dermatopathy development and its relationship to a wide variety of immune-related endpoints did not support an immune-related cause.
Pregabalin has been investigated in several animal models of disease (Jang, et al., 2012; Hundehege, et al., 2017) in both prophylactic and therapeutic treatment. No effect on the immune responses was observed. Similarly, Silva et al. (Silva, et al., 2014) showed no significant change in the levels of IL-6, IL-10, IL-27 and TGFb in lymph nodes of mice with experimental autoimmune encephalomyelitis that were treated with pregabalin. And while data from a clinical study (Mercan, et al., 2021) seemed to suggest an association between pregabalin treatment and increased immunologic markers in peripheral blood of individuals with neuropathic pain, when confounders such as comorbidities were removed the data did not show any differences.
Of interest, Gao et al. (Gao, et al., 2020) identified the adaptor protein DOK3 as a key regulator microglial cell activation in a model of neuropathic pain. Here, pregabalin was shown to reduce expression of DOK3 mRNA and the induction of inflammatory biomarkers produced by upregulated DOK3, suggesting a role (direct or indirect) on inflammatory responses. In the GLP toxicology studies in mice (Pegg, et al., 2012), there was an increase in the number of macrophages present in bone marrow, spleen, and liver (5-fold greater than controls after 1 year). In a lipopolysaccharide sepsis model in aged rats, Asci et al. (Asci, et al., 2021) showed that pregabalin can inhibit LPS-induced lesions as shown by changes in several immune system markers. The LPS-induced response, however, likely causes the damage through inflammatory processes so no conclusion can be reached from these data regarding direct immunosuppressive effects of pregabalin. Together, these data suggest a possible role for pregabalin in the inflammatory response which is discussed above, though the data are conflicting (e.g., pro-inflammatory in (Pegg, et al., 2012) and anti-inflammatory (Gao, et al., 2020; Asci, et al., 2021)).
3.1.7.2 In silico approaches
There are no in silico methods for predicting the ability of pregabalin to induce immunomodulatory changes.
3.1.7.3 Reliability and confidence
The data for pregabalin, which are derived from summaries of the original GLP toxicology studies (Pegg, et al., 2012) are assigned a reliability score of RS1. The remainder of the experimental data are assigned a reliability score of RS3. Given these reliability scores and consideration of their corresponding relevance for the evaluation of immunosuppression, a medium confidence can be assigned to the conclusion that pregabalin does not affect immune system function.
3.1.7.4 Data gaps
No other studies were found in the publicly available literature where pregabalin was specifically evaluated for immunosuppressive activity in normal animals (e.g., T-cell-dependent antibody response, assessment of cell-mediated or innate immunity, or evaluation in host resistance models).
3.1.8 KC8: Modulates receptor-mediated effects
3.1.8.1 Experimental data
Criswell et al. (Criswell, et al., 2012b) described the effects of pregabalin treatment in mice on VEGF, PDGF, bFGF, and thrombopoietin (TPO), as well as VEGFR2. There was no increase in serum VEGF or TPO in the study, while serum PDGF increased by 4-fold after 12 months of treatment, and by 47% after 24 months. No increase was observed after 18 months of treatment. Bone marrow and splenic macrophages and erythroid precursor cells were positive for bFGF staining after 6 months of treatment and were strongly positive after 12 months. VEGF levels were increased in spleen at the highest dose level tested after 6 months of treatment, and VEGF was increased in spleen and sternal bone marrow at 1,000 mg/kg after 12 months. No increased VEGF staining was observed in liver. VEGFR2 levels were increased in EC in the liver of female mice at 1,000 mg/kg after 12 months, but not at lower dose levels.
In vitro, the only activity in a panel of 182 assays in the ICE database was for estrogen receptor agonist activity, with an AC50 of 11.7 µM (ICE, 2022) (Supplementary Table S3). Pregabalin was considered inactive in the other receptor-mediated assays conducted.
3.1.8.2 In silico approaches
The authors of the original studies performed during the development of pregabalin (Criswell, et al., 2012a; Criswell, et al., 2012b; Criswell, et al., 2012c; Pegg, et al., 2012) did not conduct any computational study on their endpoints of interest. Though not necessarily relevant to hemangiosarcoma formation, QSAR models were applied to evaluate androgenic activity, estrogenic (ER) activity, and thyroid peroxidase activity of pregabalin with the Leadscope model applier (Instem, Inc.) and ADMET Predictor (SimulationsPlus) platforms. Such predictions can provide insights on the potential interactions with receptors relevant to other mechanisms of carcinogenicity. Predictions were negative for different endpoints including androgen receptor (AR) binding, aromatase inhibition, thyroperoxidase (TPO) inhibition, thyroid hormone receptor binding and transactivation. Details of the predictions are reported in the Supplementary Table S4.
3.1.8.3 Reliability and confidence
The experimental data are assigned a reliability score of RS2 and standard relevance. The in silico results are assigned a RS5. Overall, there is medium confidence that pregabalin does not affect receptor-mediated pathways known to be associated with carcinogenicity.
3.1.8.4 Data gaps
Several computational models have been published for VEGF interactions with VEGFR2 and subsequent proliferation of cells (Mac Gabhann and Popel, 2005; Kleinstreuer, et al., 2013; Clegg and Mac Gabhann, 2015) and use of these models may have strengthened the associations postulated for the mechanism proposed in (Criswell, et al., 2012a; Criswell, et al., 2012c). Similarly, models exist for PDGF activity, and recently a model was reported for PDGF-VEGF interactions with VEGFR2 (Mamer, et al., 2017). However, all of these models are computational biology models and do not predict growth factor activity based on the chemical structure of the binding ligand.
3.1.9 KC9: Causes immortalization
3.1.9.1 Experimental data
No data are available on immortalization of cells exposed to pregabalin, other than the presence of hemangiosarcomas in mice treated with the compound, which implies immortalization of cells.
3.1.9.2 In silico approaches
While some in silico methods for predicting immortalization in the SHE cell assay are available (Tice, et al., 2021), none were used for pregabalin.
3.1.9.3 Reliability and confidence
Based on a lack of evidence, a robust conclusion on whether pregabalin causes immortalization cannot be derived.
3.1.9.4 Data gaps
Immortalization of cells is typically considered to be an in vitro property, relating to the lack of senescence after long-term passaging of cells in culture. Cells taken from malignant tumors usually are immortal when cultured, and non-malignant cells can become immortal in culture when manipulated with certain viruses, proteins, etc., or arise from spontaneous mutations. The utility of in silico modeling for immortalization is unknown, as not all immortal cells will progress to malignant tumors.
3.1.10 KC10: Alters cell proliferation, cell death, or nutrient supply
3.1.10.1 Experimental data
Criswell et al. (Criswell, et al., 2012a) described several experiments where EC proliferation was measured in mouse liver, bone marrow, or spleen, and rat liver. Pregabalin increased hepatic endothelial and Kupffer cell proliferation in mice after 12 months of treatment at 200 and 1,000 mg/kg bw, while there was no effect at 50 mg/kg. In another mouse experiment, 5,000 mg/kg bw pregabalin increased the number of proliferating ECs in the liver after 2 and 4 weeks of treatment, and in the bone marrow after 12 weeks. Only minor increases in absolute numbers of proliferating ECs were found in the spleen. Vitamin E supplementation in the diet abolished the EC proliferation in the liver observed after 2 weeks. No increased proliferation of EC was seen in rat liver after up to 18 months of treatment at the maximum tolerated dose. Increases in release of tissue growth factors (VEGF, bFGF, PDGF) could also play a role in proliferation of EC.
No data were found for cell death or nutrient supply.
3.1.10.2 In silico approaches
No computational studies were performed on the EC data. As described in Tice and Bassan et al. (Tice, et al., 2021), global in silico methods for cell death, cell proliferation, and alteration of nutrient supply are not available.
3.1.10.3 Reliability and confidence
The experimental data are assigned a reliability score of RS2 with standard relevance. Confidence is high to medium that pregabalin affects cell proliferation.
3.1.10.4 Data gaps
The understanding of the mechanistic drivers for proliferation of EC are incomplete, and in silico models for the processes involved in KC10 are not available.
3.2 Other data related to carcinogenicity
A ChEMBL search retrieved no evidence of activity at targets related to known mechanisms of carcinogenicity. For pregabalin itself, no pChEMBL values were found other than for its recognized target: voltage-dependent calcium channels, α2δ subunit. A search for compounds with ≥50% structural similarity to pregabalin retrieved only two compounds (50% and 53% similar to pregabalin), both with only very low potencies (>30 µM) at four targets: CYP1A2, putative fructose-1,6-bisphosphate aldolase, carbonic anhydrase II, and solute carrier family 22 member 20.
Data for pregabalin in the Comparative Toxicogenomics Database (CTDB, 2022) shows gene interactions with angiotensin-related receptors and a few other genes that have no direct relationship to carcinogenesis.
A safety signal analysis of spontaneous reporting systems was performed with the ClarityPV platform (https://claritypv.com/) (CLARITY, 2023). The analysis was based on a total number of 397,205 unique spontaneous reports deposited between 1 January 2005 and 31 August 2023 (average reporting rate of 1,773 reports/month or 21,278 reports/year) in FAERS (250,457), VigiBase (130,039), JADER (13,973) and VAERS (2,736). A list of 22 side effects disproportionally reported (PRR05 > 2.0) for pregabalin was identified (Supplementary Table S5), of which pituitary tumour benign shows also levels of suspiciousness and instantiation that warn some cautionary vigilance. Pregabalin was first approved for marketing in the US in 2004 and in the EU in 2005. Of note, some patients treated with pregabalin may also have been treated with dopamine-active agents, which are associated with pituitary tumors.
3.3 Carcinogenicity models and their predictions
The in silico models available for predicting in vivo carcinogenicity are based on resources that collect carcinogenicity results from the corresponding animal studies (Benigni, et al., 2008; Golbamaki and Benfenati, 2016; Bossa, et al., 2018; Bower, et al., 2020). The specific carcinogenicity predictions for pregabalin are reported in Table 2, where detailed results are included for both statistical- and expert-based systems (i.e., structural alerts). No alerts for both the genotoxic and the non-genotoxic carcinogenicity mechanisms are reported and, similarly, the statistical-based predictions falling in the applicability domain of the corresponding models (as in the case of the in vivo rodent carcinogenicity models for female rat, male rat, female mouse and male mouse (Instem, 2022)) are negative. There are also models providing a quantitative prediction (TD50); however, these models generally have a limited predicting capacity. Two of these software programs, ADMET Predictor and LAZAR, predict carcinogenicity potency expressed as TD50 (the oral daily dose administered over the course of lifetime required to produce tumors in 50 percent of animals); these values were compared with those reported by Pegg, et al. (2012). Both ADMET predictor and Lazar are statistically-based models that use Carcinogenicity Potency Database (CPDB, 2022) data to model endpoints of interest. In the case of Lazar prediction for rat carcinogenicity, the confidence in the prediction was considered low by the program. Comparison of the predictions by ADMET predictor (predicted TD50 rat = 92.2 mg/kg/day and predicted TD50 mouse = 376.8 mg/kg/day) to the bioassay results shows the in silico predictions to be far away from the actual TD50 of >5,000 mg/kg in the mouse. The reason for ADMET prediction of pregabalin being more potent for rat carcinogenicity than mouse is not clear. Overall, the in silico outcome for carcinogenicity for pregabalin in vivo does not highlight any element of concern. However, reliability scores of the predictions (Myatt, et al., 2018; Johnson, et al., 2022) as evaluated by means of analysis and expert review of the results are not high (mostly RS5) and this lowers the confidence of the negative overall assessment for in vivo carcinogenicity. The models did not predict the experimental outcome in the mouse, indicating that while they were correct in predicting a low likelihood of carcinogenicity with pregabalin across species, consideration of mechanisms of carcinogenicity is not a strength of these models.
3.4 Species differences/human relevance
Pregabalin treatment for up to 2 years caused hemangiosarcoma formation in mice, but not in rats (Pegg, et al., 2012). The mode of action of pregabalin-induced hemangiosarcomas is formulated in (Criswell, et al., 2012a; Criswell, et al., 2012b; Pegg, et al., 2012). The data and conclusions in these publications are consistent with the mode of action for hemangiosarcoma formation described in (Cohen, 2017). Criswell et al. (Criswell, et al., 2012c) further describe the relevance of mouse hemangiosarcomas to humans, including some data from studies with human blood or cells, and in vivo from human subjects. Regarding human relevance of results from rodent studies, it is recognized that tumors observed in animal studies that result from genotoxic mechanisms are generally considered to be relevant to humans even when occurring in tissues with no direct human equivalent (ECHA, 2017). On the other hand, non-genotoxic compounds causing tumors in animals may act through modes of action that are not human relevant (ECHA, 2017; Goodman, 2018). The available data point to a lack of relevance of the pregabalin-induced mouse hemangiosarcomas to humans.
4 Discussion
Lifetime rodent carcinogenicity studies are extremely resource intensive, requiring the use of over 500 rodents, costing over $1 million and taking approximately 3 years of time to complete. As it is not possible or desirable to test all chemicals and drugs under these conditions, the development and use of in vitro and in silico tools to predict carcinogenicity is imperative. Tice and Bassan et al. (Tice, et al., 2021) described the state-of-the-art for the use of in silico tools to predict the outcome of in vitro and in vivo assays (other than the traditional rodent carcinogenicity assay) relevant to carcinogenicity hazard assessment. Using pregabalin as a case study, we reviewed the in vivo, in vitro, and in silico data organized within the framework of the 10 KCs of carcinogens. We show where the experimental and in silico models give results that are useful in predicting carcinogenicity, and where there are gaps in the data and models that need to be addressed to more reliably predict nongenotoxic compound carcinogenicity.
Pregabalin is a single species, nongenotoxic rodent carcinogen. The MOA for pregabalin carcinogenicity has been proposed by (Criswell, et al., 2012c) and has been accepted by regulators globally. This MOA is consistent with the MOA of other agents that cause hemangiosarcomas in rodents (Cohen, 2017). Figure 1 in (Tice, et al., 2021) showed the relationship between the KCs of carcinogens and the stages of carcinogenesis. Here, the figure has been revised to highlight the roles of the KCs involved in the carcinogenic process for pregabalin (Figure 3).
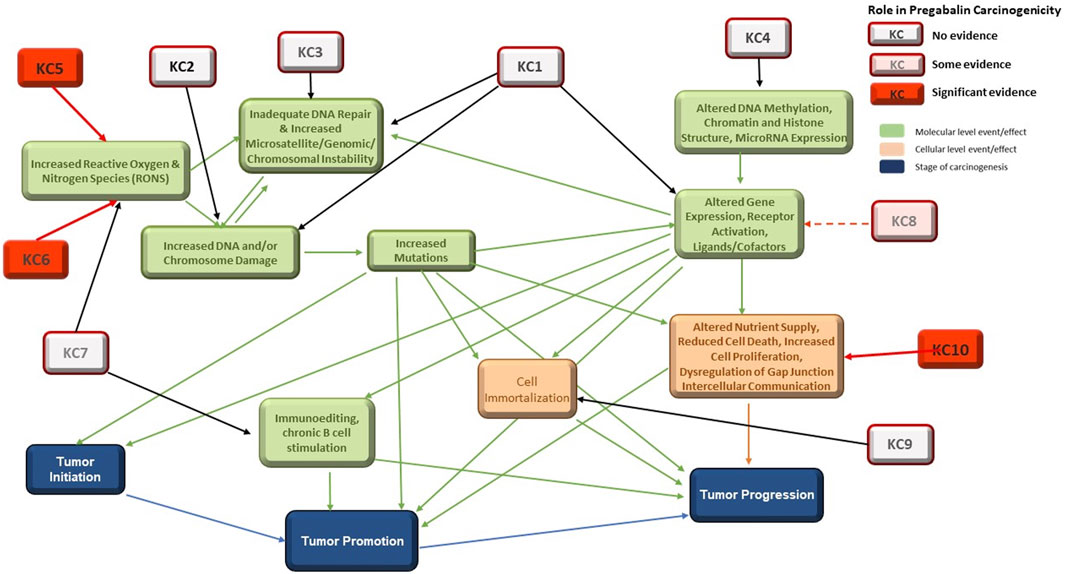
FIGURE 3. Interactions among the 10 KCs and with the Three Stages of Carcinogenesis. KC1 = is electrophilic, KC2 = is genotoxic, KC3 = alters DNA repair or causes genomic instability, KC4 = induces epigenetic alterations, KC5 = induces oxidative stress, KC6 = induces chronic inflammation, KC7 = is immunosuppressive, KC8 = modulates receptor-mediated effects, KC9 = causes immortalization, KC10 = alters cell proliferation, cell death, or nutrient supply. Specific evidence for involvement in pregabalin carcinogenicity is highlighted in red.
Outcomes for KC1 (is electrophilic or can be metabolically activates), KC2 (is genotoxic), and KC8 (modulates receptor-mediated effects) can, at least in part, be reliably predicted with in vitro systems and in silico models (Table 3). These are discussed in detail in (Tice, et al., 2021). While models to predict electrophilicity may be overly sensitive, bacterial mutagenicity models that incorporate metabolic activation are an acceptable and more accurate surrogate for the electrophilicity endpoint. Prediction of KC2 (genotoxicity) is the most well-developed area of in vitro and in silico tools for carcinogenicity assessment and in vivo tests are rarely needed. Prediction of KC8 outcomes (modulates receptor-mediated effects) was applied in this work for some nuclear receptor activities, with the most effort centered on ER and AR which have been linked to certain mechanisms of carcinogenicity. Interactions with some additional receptors, CYPs and AhR can be modeled (Vedani, et al., 2012). While these activities are not part of the pregabalin carcinogenicity MOA, they could play a role in the carcinogenicity of endocrine-disrupting chemicals (Heusinkveld, et al., 2020). Some receptor-mediated mechanisms of carcinogenicity, such as PPARɑ and AhR driven tumors, could lack relevance to humans, which highlights the need for species-specific understanding of mechanisms and models.
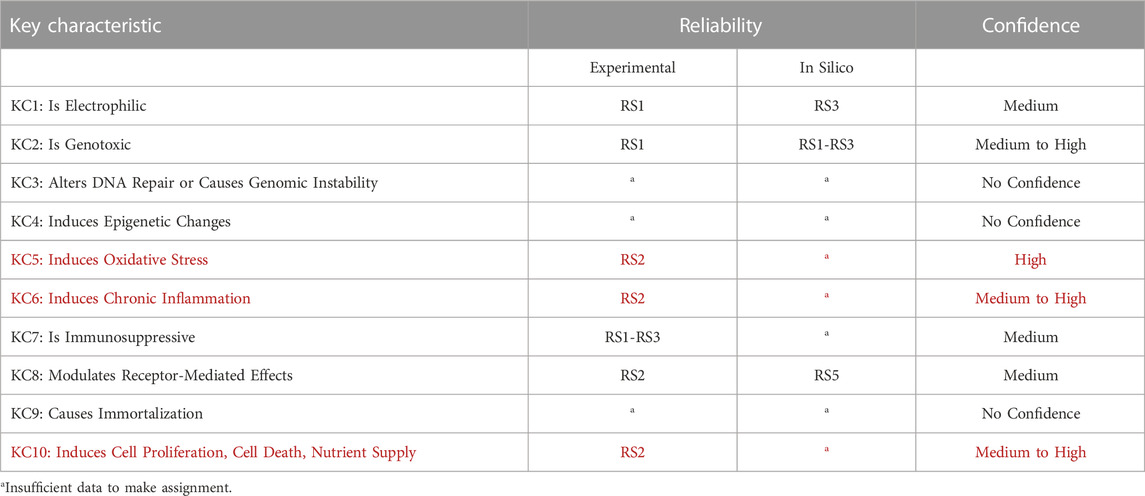
TABLE 3. Summary of data reliability and confidence. Reliability scores and confidence levels are assigned according to (Myatt, et al., 2018; Johnson, et al., 2022). Confidence considers the reliability, relevance, and coverage of information available. KCs listed in red are involved in the mode of action of pregabalin carcinogenicity in mice.
A variety of in vitro systems are available for prediction of KC5 (induce oxidative stress), but in silico model development has lagged. As described in (Tice, et al., 2021) in silico models are available for several hard chemistry endpoints related to oxidative stress, such as peroxide and quinone formation. Of note, a quantum model for Nrf-2/ARE activation has been reported in the literature to identify the structures predicted to activate the Nrf2-antioxidant response element pathways (Williamson, et al., 2012). As oxidative stress plays a role in the MOA for pregabalin carcinogenicity, a readily available model for the prediction of these types of effects would be desirable.
In contrast, no significant in silico models exist for prediction of KC3 (alerts DNA repair or causes genomic instability), 4 (induces epigenetic alterations), 6 (induces chronic inflammation), 7 (is immunosuppressive), 9 (causes immortalization), and 10 (alters cell proliferation, cell death, or nutrient supply). In silico models exist for only a small number of the endocrine and hormonal endpoints that can be associated with carcinogenicity. In vivo and in vitro assays are available for some aspects of these KCs, but prediction from the chemical structure of the molecule (i.e., drug, xenobiotics) is not possible at this time. This is a major obstacle for prediction of nongenotoxic carcinogenicity, as the MOA is often dependent on KC6 (inflammation) and/or KC10 (cell proliferation). DNA repair (KC3) and epigenetic factors (KC4 (Shin, 2020)) could play significant roles in carcinogenicity of agents that are not positive in traditional genotoxicity assays (KC2). While many immunosuppressive drugs carry a warning for increased cancer risk (Cangemi, et al., 2019), the extent of immunosuppression associated with this risk in humans is unknown. Additional in vitro assays and in silico models to predict these effects would be extremely useful.
Data from other studies such as Tox21-type screening, toxicogenomics, target activity profiles such as ChEMBL, and human data, when available, can add value to carcinogenicity assessments, but in silico models are not available for any of these. Cook et al. (Cook, et al., 2018) investigated three additional, structurally diverse compounds that caused hemangiosarcomas in mice (fenretinide, troglitazone, and elmiron), further testing the MOA proposed in (Cohen, et al., 2009; Criswell, et al., 2012c). These studies included some of the same analyses used in the pregabalin studies (bone marrow, hematology, and hypoxia parameters) as well as transcriptomics. The results indicated that the three additional compounds initiated the same MOA as pregabalin, with the potency of biological effects following the potency of hemangiosarcoma formation by these compounds. Additionally, the studies showed that transcriptomics were consistent with the MOA and potency of the compounds but was not more sensitive than hydroxyprobe for detection of hypoxia. Given the structural diversity of compounds that cause hemangiosarcomas in mice, the availability of in silico models to predict some of the key elements of this MOA could save significant amounts of effort, time, and animals. The use of in silico models to predict hazard for the different KC could be very useful in determining what targeted biological assays to perform to confirm an effect.
While known human carcinogens are almost exclusively genotoxic compounds, nongenotoxic carcinogenicity has been shown in experimental systems and in most cases the relevance to humans is not known with any degree of confidence (Silva Lima and van der Laan, 2000; Cohen, 2017). This is the case for environmental chemicals, food source chemicals, and drugs, highlighting the need for reliable methods for predicting the key events in an MOA, and KCs of carcinogens can be a useful method as an initial step to organize and process the data. As an example of where reliable in silico methods for prediction of nongenotoxic carcinogens would be of use, the ICH has revised the S1B guideline to allow for drug developers to develop a weight of evidence (WoE) argument to assess whether a rat carcinogenicity study would add value over existing data for determination of human carcinogenicity. The proposed WoE assessment (ICH, 2022) combines certain factors such as target biology, genotoxicity, secondary pharmacology, immunomodulation, hormonal perturbation, and repeated-dose histopathology into an integrated human risk assessment. The KCs framework may be one approach to support the identification and interpretation of relevant evidence and assays for each factor supporting how such evidence might be combined, and relevant in silico predictions would provide additional insights into the ICH S1B weight of evidence approach. This can be particularly useful when a specific MOA is not postulated.
5 Conclusion
The overall goal of this exercise was to evaluate the ability of in silico models to predict nongenotoxic carcinogenicity with pregabalin as a case study while being guided by the KC framework in the organization and combination of the collected information. Pregabalin is a single-species carcinogen producing only one type of tumor, hemangiosarcomas. The established MOA is triggered by tissue hypoxia, leading to oxidative stress (KC5), chronic inflammation (KC6), and increased cell proliferation (KC10) of EC (Criswell, et al., 2012a). Of these KCs, in silico models are available only for selected endpoints in KC5, limiting the usefulness of computational tools in prediction of pregabalin carcinogenicity. KC1, KC2, and KC8, for which predictive in silico models exist, do not play a role in this MOA. Additionally, as the pregabalin MOA is considered not relevant to humans, experimental assays and in silico models used to predict endpoints for the KC involved must either account for species differences or produce results that can be interpreted in the context of species-specific biology.
We investigated the availability of in silico models to predict the ten KCs of carcinogens for a nongenotoxic compound, pregabalin. In silico approaches are available for some of the mechanisms associated with the KCs but are particularly lacking for the KCs involved in the MOA specific for pregabalin carcinogenicity. Development of reliable in silico models for prediction of oxidative stress, chronic inflammation, immunosuppression, and cell proliferation will be critical for the ability to predict nongenotoxic compound carcinogenicity.
Data availability statement
The original contributions presented in the study are included in the article/Supplementary Material, further inquiries can be directed to the corresponding author.
Author contributions
DK: Conceptualization, Investigation, Writing–original draft, review and editing. AB: Conceptualization, Investigation, Writing–review and editing. AA: Investigation, Writing–review and editing. LB: Investigation, Writing–review and editing. JC: Investigation, Writing–review and editing. KC: Conceptualization, Investigation, Writing–review and editing. FH: Investigation, Writing–review and editing. GJ: Investigation, Writing–review and editing. AL: Investigation, Writing–review and editing. SM: Investigation, Writing–review and editing. JM: Investigation, Writing–review and editing. AM-B: Investigation, Writing–review and editing. DW: Investigation, Writing–review and editing. RT: Conceptualization, Supervision, Investigation, Writing–review and editing. All authors contributed to the article and approved the submitted version.
Acknowledgments
The authors wish to thank Glenn Myatt (Instem) for constructive suggestions during the development of this work.
Conflict of interest
DK, LB, GJ and RT are independent contractors. AB is employed by Innovatune. AA is employed by Sanofi. JC, KC and FH are employed by Instem. AL is employed by Medline Industries. SM is employed by Nestle Research. JM is employed by Chemotargets SL. Amy Mihalchik-Burhans is employed by ToxStrategies, LLC. David Wooley is employed by ForthTox.
The authors declare that the research was conducted in the absence of any commercial or financial relationships that could be construed as a potential conflict of interest.
Publisher’s note
All claims expressed in this article are solely those of the authors and do not necessarily represent those of their affiliated organizations, or those of the publisher, the editors and the reviewers. Any product that may be evaluated in this article, or claim that may be made by its manufacturer, is not guaranteed or endorsed by the publisher.
Supplementary material
The Supplementary Material for this article can be found online at: https://www.frontiersin.org/articles/10.3389/ftox.2023.1234498/full#supplementary-material
Abbreviations
bFGF, basic fibroblast growth factor; EC, Endothelial cell; GLP, Good laboratory practices; ICH, International Council for Harmonisation of Technical Requirements for Pharmaceuticals for Human Use; IST, In silico toxicology protocol; KC, Key characteristic; MOA, Mode of action; OECD, Organisation for Economic Cooperation and Development; PDGF, Platelet-derived growth factor (Q)SAR, Quantitative structure-activity relationship; ROS, Reactive oxygen species; RS, Reliability score; TD50, Toxic dose 50; TPO, Thrombopoietin; VDCC, voltage-dependent calcium channel; VEGF, Vascular endothelial growth factor.
References
Alles, S., Cain, S., and Snutch, T. (2020). Pregabalin as a pain therapeutic: beyond calcium channels. Front. Cell. Neurosci. 14, 83. doi:10.3389/fncel.2020.00083
Arnold, M., Rutherford, M., Bardot, A., Ferlay, J. e. a., Andersson, T. M. L., Myklebust, T. Å., et al. (2019). Progress in cancer survival, mortality, and incidence in seven high-income countries 1995-2014 (ICBP SURVMARK-2): a population-based study. Lancet Oncol. 20, 1493–1505. doi:10.1016/S1470-2045(19)30456-5
Asci, H., Ozmen, O., Erzurumlu, Y., Savas, H. B., Temel, E. N., Icten, P., et al. (2021). Ameliorative effects of pregabalin on LPS induced endothelial and cardiac toxicity. Biotech. Histochem. 96 (5), 364–375. doi:10.1080/10520295.2020.1810315
Ashby, J., and Tennant, R. (1988). Chemical structure, Salmonella mutagenicity and extent of carcinogenicity as indicators of genotoxic carcinogenesis among 222 chemicals tested in rodents by the U.S. NCI/NTP. Mutat. Research/Genetic Toxicol. 204 (1), 17–115. doi:10.1016/0165-1218(88)90114-0
Banik, A., Kandilya, D., Ramya, S., Stünkel, W., Chong, Y. S., and Dheen, S. T. (2017). Maternal factors that induce epigenetic changes contribute to neurological disorders in offspring. Genes. 8 (6), 150. doi:10.3390/genes8060150
Benigni, R., Bossa, C., Richard, A., and Yang, C. (2008). A novel approach: chemical relational databases, and the role of the ISSCAN database on assessing chemical carcinogenicity. Ann. Ist. Super. Sanita 44, 48–56.
Boobis, A., Cohen, S. M., Dellarco, V., McGregor, D., Meek, M. E. B., Vickers, C., et al. (2006). IPCS framework for analyzing the relevance of a cancer mode of action for humans. Crit. Rev. Toxicol. 36 (10), 781–792. doi:10.1080/10408440600977677
Bossa, C., Benigni, R., Tcheremenskaia, O., and Battistelli, C. (2018). “(Q)SAR methods for predicting genotoxicity and carcinogenicity: scientific rationale and regulatory frameworks,” in Computational toxicology, methods in molecular biology. Editor O. Nicolotti (New York: Springer), 447–473.
Bower, D., Cross, K., and Myatt, G. (2020). “Organisation of toxicological data in databases,” in Big data in predictive toxicology, issues in toxicology. Editor D. a. R. A. Neagu (Cambridge: Royal Society of Chemistry), 108–165.
Cangemi, M., Montico, B., Faè, D. A., Steffan, A., and Dolcetti, R. (2019). Dissecting the multiplicity of immune effects of immunosuppressive drugs to better predict the risk of de novo malignancies in solid organ transplant patients. Front. Oncol. 9, 160. doi:10.3389/fonc.2019.00160
ChEMBL. 2022 Available at: https://www.ebi.ac.uk/chembl/g/#search_results/compounds/query=pregabalin (Accessed August 22, 2022).
CLARITY (2023). ClarityPV. Available at: https://claritypv.com/ (Accessed September 29, 2023).
Clegg, L., and Mac Gabhann, F. (2015). Site-specific phosphorylation of VEGFR2 is mediated by receptor trafficking: insights from a computational model. PLoS Comput. Biol. 11 (6), e1004158. doi:10.1371/journal.pcbi.1004158
Cohen, S. (2017). The relevance of experimental carcinogenicity studies to human safety. Curr. Opin. Toxicol. 3, 6–11. doi:10.1016/j.cotox.2017.04.002
Cohen, S., Boobis, A. R., Dellarco, V. L., Doe, J. E., Fenner-Crisp, P. A., Moretto, A., et al. (2019). Chemical carcinogenicity revisited 3: risk assessment of carcinogenic potential based on the current state of knowledge of carcinogenesis in humans. Regul. Toxicol. Pharmacol. 103, 100–105. doi:10.1016/j.yrtph.2019.01.017
Cohen, S., Storer, R. D., Criswell, K. A., Doerrer, N. G., Dellarco, V. L., Pegg, D. G., et al. (2009). Hemangiosarcoma in rodents: mode-of-action evaluation and human relevance. Toxicol. Sci. 111, 4–18. doi:10.1093/toxsci/kfp131
Cook, J., Obert, L. A., Koza-Taylor, P., Coskran, T. M., Opsahl, A. C., Ziemek, D., et al. (2018). From the cover: fenretinide, troglitazone, and elmiron add to weight of evidence support for hemangiosarcoma mode-of-action from studies in mice. Toxicol. Sci. 161, 58–75. doi:10.1093/toxsci/kfx195
Corthals, S., Kamendulis, L., and Klaunig, J. (2006). Mechanisms of 2-butoxyethanol-induced hemangiosarcomas. Toxicol. Sci. 92, 378–386. doi:10.1093/toxsci/kfl007
CPDB (2022). Carcinogenicity potency database. Available at: https://www.nlm.nih.gov/databases/download/cpdb.html (Accessed August 23, 2022).
Criswell, K., Cook, J. C., Morse, D., Lawton, M., Somps, C., Obert, L., et al. (2012b). Pregabalin induces hepatic hypoxia and increases endothelial cell proliferation in mice, a process inhibited by dietary vitamin E supplementation. Toxicol. Sci. 128, 42–56. doi:10.1093/toxsci/kfs148
Criswell, K., Cook, J. C., Wojcinski, Z., Pegg, D., Herman, J., Wesche, D., et al. (2012c). Mode of action associated with development of hemangiosarcoma in mice given pregabalin and assessment of human relevance. Toxicol. Sci. 128, 57–71. doi:10.1093/toxsci/kfs149
Criswell, K., Wojcinski, Z., Pegg, D., Albassam, M., Duddy, S., Olsen, E., et al. (2012a). Key components of the mode of action for hemangiosarcoma induction in pregabalin-treated mice: evidence of increased bicarbonate, dysregulated erythropoiesis, macrophage activation, and increased angiogenic growth factors in mice but not in rats. Toxicol. Sci. 128, 22–41. doi:10.1093/toxsci/kfs147
CTDB (2022). CTDB Available at: http://ctdbase.org/basicQuery.go?bqCat=reference&bq=pregabalin (Accessed August 22, 2022).
Descalzi, G., Ikegami, D., Ushijima, T., Nestler, E. J., Zachariou, V., and Narita, M. (2015). Epigenetic mechanisms of chronic pain. Trends Neurosci. 38 (4), 237–246. doi:10.1016/j.tins.2015.02.001
Doe, J., Boobis, A. R., Dellarco, V., Fenner-Crisp, P. A., Moretto, A., Pastoor, T. P., et al. (2019). Chemical carcinogenicity revisited 2: current knowledge of carcinogenesis shows that categorization as a carcinogen or non-carcinogen is not scientifically credible. Regul. Toxicol. Pharmacol. 103, 124–129. doi:10.1016/j.yrtph.2019.01.024
Doll, R., and Peto, R. (1982). The causes of cancer: quantitative estimates of avoidable risks of cancer in the United States today. JNCI 66 (6), 1192–1308. doi:10.1093/jnci/66.6.1192
ECHA (2017). Guidance on information requirements and chemical safety assessment Chapter R.7a: endpoint specific guidance, s.l. USA: Publications Office of the EU.
EMA (2005). Lyrica - EPAR - scientific discussion. Available at: https://www.ema.europa.eu/documents/scientific-discussion/lyrica-epar-scientific-discussion_en.pdf.
EMA (2018). Valproate and related substances. Available at: https://www.ema.europa.eu/en/medicines/human/referrals/valproate-related-substances-0 (Accessed August 22, 2022).
Gao, W., Qu, Y. J., Huai, J., Wei, H., Zhang, Y., and Yue, S. W. (2020). DOK3 is involved in microglial cell activation in neuropathic pain by interacting with GPR84. Aging 13 (1), 389–410. doi:10.18632/aging.202144
Geranton, S., and Tochiki, K. (2015). Regulation of gene expression and pain states by epigenetic mechanisms. Prog. Mol. Biol. Transl. Sci. 131, 147–183. doi:10.1016/bs.pmbts.2014.11.012
Golbamaki, A., and Benfenati, E. (2016). “In silico methods for carcinogenicity assessment,” in In silico methods for predicting drug toxicity, Methods in Molecular Biology. Editor E. Benfenati (New York(NY): Springer), 107–119.
Goodman, J. (2018). Goodbye to the bioassay. Toxicol. Res. (Camb) 7, 558–564. doi:10.1039/c8tx00004b
Hasselgren, C., Ahlberg, E., Akahori, Y., Amberg, A., Anger, L. T., Atienzar, F., et al. (2019). Genetic toxicology in silico protocol. Regul. Toxicol. Pharmacol. 107, 104403. doi:10.1016/j.yrtph.2019.104403
Heusinkveld, H., Braakhuis, H., Gommans, R., Botham, P., Corvaro, M., van der Laan, J. W., et al. (2020). Towards a mechanism-based approach for the prediction of nongenotoxic carcinogenic potential of agrochemicals. Crit. Rev. Toxicol. 50, 725–739. doi:10.1080/10408444.2020.1841732
Hundehege, P., Epping, L., and Meuth, S. (2017). Calcium homeostasis in multiple sclerosis. Neurol. Int. Open 1, E127–E135. doi:10.1055/s-0043-109031
ICH (2022). S1B(R1) guideline. Available at: https://database.ich.org/sites/default/files/S1B-R1_FinalGuideline_2022_0719.pdf (Accessed August 17, 2022).
Instem 2022 Computational toxicology. Available at: www.instem.com/solutions.insilico/computational-toxicology.php [Accessed 12 October 2022].
Jang, Y., Song, H.-K., Yeom, M., and Jeong, D. (2012). The immunomodulatory effect of pregabalin on spleen cells in neuropathic mice. Anesth. Analgesia 115, 830–836. doi:10.1213/ANE.0b013e31825d2ad1
Johnson, C., Anger, L. T., Benigni, R., Bower, D., Bringezu, F., Crofton, K. M., et al. (2022). Evaluating confidence in toxicity assessments based on experimental data and in silico predictions. Comput. Toxicol. 21, 100204. doi:10.1016/j.comtox.2021.100204
Kleinstreuer, N., Dix, D., Rountree, M., Baker, N., Sipes, N., Reif, D., et al. (2013). A computational model predicting disruption of blood vessel development. PLoS Comput. Biol. 9 (4), e1002996. doi:10.1371/journal.pcbi.1002996
Klimisch, H.-J., Andreae, M., and Tillmann, U. (1997). A systematic approach for evaluating the quality of experimental toxicological and ecotoxicological data. Regul. Toxicol. Pharmacol. 25, 1–5. doi:10.1006/rtph.1996.1076
Kratzer, T. B., Siegel, R. L., Miller, K. D., Sung, H., Islami, F., and Jemal, A. (2022). Progress against cancer mortality 50 Years after passage of the national cancer act. JAMA Oncol. 8 (1), 156–159. doi:10.1001/jamaoncol.2021.5668
Liang, L., Lutz, B., Bekker, A., and Tao, Y. (2015). Epigenetic regulation of chronic pain. Epigenomics 7 (2), 235–245. doi:10.2217/epi.14.75
LYRICA (2018). Lyrica - drug approval package. Available at: https://www.accessdata.fda.gov/drugsatfda_docs/nda/2004/021446_LyricaTOC.cfm (Accessed August 17, 2022).
LYRICA 2018. LYRICA label data. Available at: https://www.accessdata.fda.gov/drugsatfda_docs/label/2018/021446s035, [Accessed 17 August 2022].
Mac Gabhann, F., and Popel, A. (2005). Differential binding of VEGF isoforms to VEGF receptor 2 in the presence of neuropilin-1: a computational model. Am. J. Physiol. - Heart Circulatory Physiology 288 (6), H2851–H2860. doi:10.1152/ajpheart.01218.2004
Madia, F., Worth, A., Whelan, M., and Corvi, R. (2019). Carcinogenicity assessment: addressing the challenges of cancer and chemicals in the environment. Environ. Int. 128, 417–429. doi:10.1016/j.envint.2019.04.067
Mamer, S., Chen, S., Weddell, J. C., Palasz, A., Wittenkeller, A., Kumar, M., et al. (2017). Discovery of high-affinity PDGF-VEGFR interactions: redefining RTK dynamics. Sci. Rep. 7, 16439. doi:10.1038/s41598-017-16610-z
Mannaioni, P., DiBello, M., and Masini, E. (1997). Platelets and inflammation: role of platelet-derived growth factor, adhesion molecules and histamine. Inflamm. Res. 46, 4–18. doi:10.1007/PL00000158
Mercan, A., Uzun, S. T., Keles, S., Hacibeyoglu, G., Yilmaz, R., and Reisli, R. (2021). Immunological mechanism of postherpetic neuralgia and effect of pregabalin treatment on the mechanism: a prospective single-arm observational study. Korean J. Pain 34 (4), 463–470. doi:10.3344/kjp.2021.34.4.463
Mico, J., and Prieto, R. (2012). Elucidating the mechanism of action of pregabalin: α(2)δ as a therapeutic target in anxiety. CNS Drugs 26, 637–648. doi:10.2165/11634510-000000000-00000
Myatt, G., Ahlberg, E., Akahori, Y., Allen, D., Amberg, A., Anger, L. T., et al. (2018). In silico toxicology protocols. Regul. Toxicol. Pharmacol. 96, 1–17. doi:10.1016/j.yrtph.2018.04.014
Myatt, G., Bassan, A., Bower, D., Crofton, K. M., Cross, K. P., Graham, J. C., et al. (2022). Increasing the acceptance of in silico toxicology through development of protocols and position papers. Comput. Toxicol. 21, 100209. doi:10.1016/j.comtox.2021.100209
Notartomaso, S., Mascio, G., Bernabucci, M., Zappulla, C., Scarselli, P., Cannella, M., et al. (2017). Analgesia induced by the epigenetic drug L-acetylcarnitine, outlasts the end of treatment in mouse models of chronic inflammatory and neuropathic pain. Mol. Pain 13, 1744806917697009. doi:10.1177/1744806917697009
Peedicayil, J. (2020). The potential role of epigenetic drugs in the treatment of anxiety disorders. Neuropsychiatr. Dis. Treat. 16, 597–606. doi:10.2147/NDT.S242040
Pegg, D., Bleavins, M., Herman, J., Wojcinski, Z., Graziano, M., Henck, J., et al. (2012). Hemangiosarcoma in mice administered pregabalin: analysis of genotoxicity, tumor incidence, and tumor genetics. Toxicol. Sci. 128, 9–21. doi:10.1093/toxsci/kfs146
Shin, T.-J. L. K.-H. C. J.-Y., Lee, K. H., and Cho, J. Y. (2020). Epigenetic mechanismsof LncRNAs binding to protein in carcinogenesis. Cancers 12, 2925. doi:10.3390/cancers12102925
Siegel, R., Miller, K., Fuchs, H., and Jemal, A. (2022). Cancer statistics 2022. CA Cancer J. Clin. 72, 7–33. doi:10.3322/caac.21708
Silva, G., Pradella, F., Moraes, A., Farias, A., dos Santos, L. M. B., and de Oliveira, A. L. R. (2014). Impact of pregabalin treatment on synaptic plasticity and glial reactivity during the course of experimental autoimmune encephalomyelitis. Brain Behav. 4 (6), 925–935. doi:10.1002/brb3.276
Silva Lima, B., and van der Laan, J. (2000). Mechanisms of nongenotoxic carcinogenesis and assessment of the human hazard. Regul. Toxicol. Pharmacol. 32 (2), 135–143. doi:10.1006/rtph.2000.1427
Smith, M., Guyton, K. Z., Gibbons, C. F., Fritz, J. M., Portier, C. J., Rusyn, I., et al. (2016). Key characteristics of carcinogens as a basis for organizing data on mechanisms of carcinogenesis. Environ. Health Perspect. 124, 713–721. doi:10.1289/ehp.1509912
Smith, M., Guyton, K. Z., Kleinstreuer, N., Borrel, A., Cardenas, A., Chiu, W. A., et al. (2020). The key characteristics of carcinogens: relationship to the hallmarks of cancer, relevant biomarkers, and assays to measure them. Cancer Epidemio., Biomarkers Prev. 29, 1887–1903. doi:10.1158/1055-9965.EPI-19-1346
Tice, R., Bassan, A., Amberg, A., Anger, L. T., Beal, M. A., Bellion, P., et al. (2021). In silico approaches in carcinogenicity hazard assessment: current status and future needs. Comput. Toxicol. 20, 100191. doi:10.1016/j.comtox.2021.100191
Vedani, A., Dobler, M., and Smiesko, M. (2012). VirtualToxLab - a platform for estimating the toxic potential of drugs, chemicals and natural products. Toxicol. Appl. Pharmacol. 261 (2), 142–153. doi:10.1016/j.taap.2012.03.018
Williamson, T., Amirahmadi, S., Joshi, G., Kaludov, N. K., Martinov, M. N., Johnson, D. A., et al. (2012). Discovery of potent, novel Nrf2 inducers via quantum modeling, virtual screening and in vitro experimental validation. Chem. Biol. Drug Des. 80 (6), 810–820. doi:10.1111/cbdd.12040
Keywords: In silico toxicology protocol, mode of action, pregabalin, non-genotoxic carcinogen, oxidative stress, chronic inflammation, cell proliferation
Citation: Keller DA, Bassan A, Amberg A, Burns Naas LA, Chambers J, Cross K, Hall F, Jahnke GD, Luniwal A, Manganelli S, Mestres J, Mihalchik-Burhans AL, Woolley D and Tice RR (2023) In silico approaches in carcinogenicity hazard assessment: case study of pregabalin, a nongenotoxic mouse carcinogen. Front. Toxicol. 5:1234498. doi: 10.3389/ftox.2023.1234498
Received: 04 June 2023; Accepted: 30 October 2023;
Published: 13 November 2023.
Edited by:
Jan Willem Van Der Laan, Medicines Evaluation Board, NetherlandsReviewed by:
John Vahle, Lilly Research Laboratories, United StatesDouglas Wolf, Syngenta, United States
Copyright © 2023 Keller, Bassan, Amberg, Burns Naas, Chambers, Cross, Hall, Jahnke, Luniwal, Manganelli, Mestres, Mihalchik-Burhans, Woolley and Tice. This is an open-access article distributed under the terms of the Creative Commons Attribution License (CC BY). The use, distribution or reproduction in other forums is permitted, provided the original author(s) and the copyright owner(s) are credited and that the original publication in this journal is cited, in accordance with accepted academic practice. No use, distribution or reproduction is permitted which does not comply with these terms.
*Correspondence: Douglas A. Keller, MjFzdGNlbnR1cnl0b3hAZ21haWwuY29t