- 1Sid and Reva Dewberry Department of Civil, Environmental, and Infrastructure Engineering, George Mason University, Fairfax, VA, United States
- 2School of Marine and Atmospheric Sciences, Stony Brook University, Stony Brook, NY, United States
As anthropogenic climate signals have intensified, precipitation patterns have changed over the contiguous United States (CONUS) and may continue to change in the future. Comparing historical climate model simulations to ground-based observations can help us quantify uncertainties in climate models when simulating precipitation and its changes. This work evaluates precipitation simulated by the Community Earth System Model Version 2 large ensemble (CESM2-LE) against observations from the National Oceanic and Atmospheric Administration Climate Prediction Center Unified CONUS (CPC) during 1948–2022. Next, past precipitation patterns from CPC are compared to future projections (2023–2100) of CESM2-LE for a medium-to-high emission scenario (Shared Socioeconomic Pathways, SSP3-7.0) from a 70-member ensemble. A pixel-by-pixel bias correction is then conducted to remove systemic errors between the model and observations. Results indicate that precipitation variability is drastically reduced in the ensemble mean and suggest caution when using it to draw conclusions regarding precipitation changes. CESM2-LE is shown to underestimate (overestimate) ground observations over CONUS in summer (winter) during 1948–2022. Climate model simulations struggle particularly to capture high-magnitude precipitation (i.e., annual averages larger than 10 mm/day), especially in the Northwestern US. Historical precipitation data show slightly upward patterns in annual, spring, fall, and winter averages, patterns that are projected to continue in the future. Future annual precipitation will increase with respect to historical observations by as much as 11% and 15% in the Northeast and Southeast US (which are already wet regions), respectively, whereas the arid Northern Great Plains region will experience a 15% decrease. Overall results indicate drier summers and wetter winters in the future with respect to the past. Furthermore, the 75th and 95th percentiles of seasonal precipitation will become more extreme during winter by as much as 100% but will decrease during summer by as much as 80%. This study places a strong emphasis on understanding reliable future climate projections, which can be useful when designing community-driven adaptation and mitigation plans for climate change.
1 Introduction
Precipitation is the major driving force of the hydrologic cycle (Kidd and Huffman, 2011; Gajbhiye et al., 2015; Praveen et al., 2020) and changes in its patterns can impact the hydrological, meteorological, agricultural, environmental, and water resource management sectors (Ebert et al., 2007; Kanniah et al., 2011; Duan et al., 2016; Mallakpour et al., 2020; Sharif et al., 2020). Therefore, a better understanding of the variations in precipitation patterns is critical (Duan and Bastiaanssen, 2013; Chatterjee et al., 2016; He et al., 2022; Dollan I. J. et al., 2022). Global rising temperatures have the potential to increase climate variability (Held and Soden, 2006; Trenberth, 2011; Wasko et al., 2015; Rodgers et al., 2021). Such changes alter the hydrologic cycle and impacts precipitation variability (Trenberth et al., 2003; Meehl et al., 2009; Chen et al., 2013; Zhang et al., 2019; Sharif et al., 2022; Rahat et al., 2022; Saki et al., 2023), which can result in an intensification of extreme precipitation events (Easterling et al., 2000; Zhai et al., 2005; Hallegatte et al., 2013; Nguyen et al., 2018; Willner et al., 2018; Yang et al., 2020; Mathbout et al., 2021; Slater et al., 2021). Carbon emissions from agriculture, industry, transportation, and households drive climate change and trigger extreme weather events (Abbas et al., 2022a; Abbas et al., 2022b; Huang et al., 2024; Fan et al., 2024). According to the Intergovernmental Panel on Climate Change (IPCC) Sixth Assessment Report (AR6), climate extremes like heavy precipitation, flooding, cyclones, sea level rise, droughts, and heat waves have already intensified (IPCC 2022). This is evident over mid-latitudinal regions, including the Contiguous United States, CONUS (Tebaldi et al., 2006; Kharin et al., 2013; Fischer and Knutti, 2016; Prein et al., 2017). Moreover, studies such as Kunkel et al. (2013), Easterling et al. (2017), and Prein and Holland (2018) indicate that extreme precipitation events are becoming more frequent and intense, particularly in the Northeast and Midwest contiguous US. However, the extent to which these trends are captured by climate models remains uncertain.
Past studies suggested that temperatures across CONUS might increase (Karmalkar and Bradley, 2017), which could intensify precipitation events (Kunkel, 2003; Monier and Gao, 2015; Neri et al., 2019) and worsen drought conditions (Strzepek et al., 2010). Mallakpour et al. (2022) showed statistically significant changes in annual precipitation maxima based on six daily gridded precipitation data over CONUS from 1983 to 2017. Furthermore, CONUS is characterized by different regional climates with high annual and seasonal variation, resulting in complex climate change patterns (Martin-vide 2004; Roy´e and Martin-Vide, 2017). For example, since the 1950s, eastern CONUS has experienced higher precipitation increase than the western side (Huang et al., 2021; Li et al., 2022). Gensini and Harold (2018) further observed that convective precipitation has been increasing, particularly in the Central U.S., with great implications for flooding and severe weather. Other studies have linked CONUS precipitation variability to large-scale atmospheric patterns, showing interannual variations that affect drought and flood risks (Seager et al., 2015; Swain et al., 2018). Nevertheless, a comprehensive analysis of the spatial and temporal variability of long-term precipitation changes across different CONUS regions is still lacking. This study aims to investigate historical and future precipitation patterns over CONUS using both observed data and climate projections.
An efficient strategy for developing effective climate change adaptation and mitigation strategies is to predict future climates using high-resolution climate models (Forestieri et al., 2018; Hosseinzadehtalaei et al., 2020; Ukkola et al., 2020; Alaminie et al., 2021). Global Climate Models (GCMs) are the primary source of information for assessing future climate changes (Navarro-Racines et al., 2015; Randall et al., 2019; Agel and Barlow, 2020; Akinsanola et al., 2020; Oruc, 2022). However, GCM simulations are affected by large uncertainties due to future emission scenarios, model resolution, internal climate variability, mathematical formulation, initial assumptions, and calibration processes (Gutowski et al., 2003; Latif, 2010; Ramirez-villegas et al., 2013; Su et al., 2013; Navarro-Racines et al., 2015; Xu et al., 2021). It is generally assumed that newer models with higher resolution and complexity outperform previous-generation models and provide more reliable projections (O’ Neill et al., 2016). The CMIP6 ensemble now includes the latest state-of-the-art climate model experiments. In 1995, the World Climate Research Program’s (WCRP) Working Group on Coupled Modelling (WGCM) launched the Coupled Model Intercomparison Project (CMIP), which used coupled simulations of the atmosphere, ocean, land surface, and sea ice (Meehl et al., 1997; Edwards, 2011). It has since evolved over five phases with scientific development and the progressively improved comprehension of the mechanisms underlying climate change (Meehl et al., 2000; 2007; Taylor et al., 2012), contributing to cutting-edge research activity based on an ensemble of models (Lalande et al., 2021) as well as to various IPCC assessment reports (IPCC, 2007; IPCC 2013). CMIP6 differs from its predecessors in links of forcing scenarios, carbon emissions, high spatial resolutions, different start year of the future scenarios and newly proposed SSPs, which describe different socioeconomic reference assumptions (Moss et al., 2010; Vuuren et al., 2014; Gidden et al., 2019; Almazroui et al., 2020). CMIP6 also has updated development of the intercomparison model, focusing on biases, enhanced parameters of the cloud microphysical process, and climate model feedback (Kawai et al., 2019).
Large ensemble (LE) simulations conducted with the Community Atmosphere Model version 6 (CAM6), namely, CESM2-LE, offer a novel tool for studying potential changes in climate and ecosystem statistics caused by human activities over various time periods. The simulations consist of a 100-member LE suite for the 1850–2100 period with SSP3-7.0 forcings. SSP3-7.0 is a medium-to-high reference scenario resulting from no additional climate policy under the SSP3 socioeconomic development narrative; it is characterized by high non-CO2 emissions, including high aerosols emissions. The large ensemble size aids in overcoming difficulties in the estimation of higher order statistical moments (Milinski et al., 2020). The size of the ensemble combined with a 1° spatial resolution is unprecedented, as it enables robust forced changes in internal variability (Simpson et al., 2020; Fasullo, 2020). Earlier versions of LE GCMs also exist (Zelle et al., 2005; Drijfhout et al., 2008; Branstator and Selten, 2009) and were used to investigate the influence of global warming on precipitation variability (Raisanen, 2002; Wetherald, 2009; Pendergrass et al., 2017). Dollan IshratJahan et al. (2022) studied seasonal totals and long-term trends from 2015–2100 for SSP3-7.0 across CONUS. However, a comprehensive comparison between historical precipitation patterns and future projections in a medium-to-high emission scenario with a large ensemble remains a gap in the literature. This study aims to address such gap.
Evaluating the performance of climate model simulations is important to assess uncertainties in climate models and better understand long-term trends, climatology, and possible climate change impacts on hydrological variables (Rupp et al., 2015; Ahmadalipour et al., 2017; Raghavan et al., 2018). Moreover, evaluating individual climate models aids in gaining confidence in future model projections (Flato et al., 2013; Moise et al., 2015). The performance of climate models can only be evaluated over historical time series and is commonly assessed against observational data (Jiang et al., 2015). Jiang et al. (2012) reported that while models could replicate the spatial distribution of ground-based precipitation and trends of extreme distributions, differences between the two estimates still existed. While previous studies such as Fu et al. (2020), Gusain et al. (2020), and Zamani et al. (2020) have assessed CMIP6 historical simulations against ground observations and compared them with CMIP5, these analyses do not extensively examine the variability in seasonal distribution and regional precipitation patterns using large ensemble simulations, which are crucial for understanding long-term climate impacts. The multi-model ensemble method, which involves averaging simulations from multiple models, has been employed in previous studies to assess the model performance (Thackeray et al., 2018; Akinsanola et al., 2021). Following an evaluation of historical data, Chen et al. (2020) examined future precipitation projections and observed how climate indicators have changed in recent decades in comparison to historical records. However, these studies have not focused on CONUS specifically.
While previous studies have examined historical precipitation changes and future climate projections using different climate models, a comprehensive comparison of observed precipitation data with CMIP6-based large ensemble simulations in a medium-to-high emission scenario across CONUS remains limited. This work evaluates historical precipitation data (1948–2022) from the 70 ensemble members of CESM2-LE simulated precipitation against ground-based observations from the National Oceanic and Atmospheric Administration’s (NOAA) Climate Prediction Center Unified CONUS (CPC) dataset. This is performed for three randomly selected ensemble members and for the average of all members (i.e., ensemble mean). As a second objective, this work compares past precipitation patterns (1948–2022) from observations to future projections (2023–2100) of CESM2-LE for a medium-to-high emission scenario (SSP3-7.0). This work aims to answer the following research questions: i) how does a LE climate model compares to ground observations when detecting changes in past precipitation? ii) how have precipitation patterns changed in the past century across CONUS? and iii) how will they change in the later decades of 21st century? By addressing these questions, this study provides insights into the reliability of large ensemble simulations for projecting future precipitation patterns and contributes to the growing knowledge of climate-induced precipitation variability over CONUS.
2 Methodology
2.1 Study area
The study investigates precipitation patterns across CONUS, which is divided into 7 NCA regions to ease the discussion (Figure 1). The NCA reports assess climate change impact, risk, and responses over CONUS (Melillo et al., 2014; Reidmiller et al., 2018).
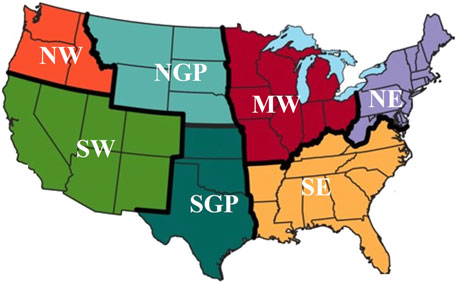
Figure 1. National Climate Assessment regions of CONUS (Source: https://www.c2es.org/content/national-climate-assessment/).
NE is characterized by a humid continental climate with warm-summers (Beck et al., 2023). The average annual precipitation variation in the region is approximately 500 mm and is often hit by extreme hydroclimatic events such as floods, droughts, hurricanes, heat waves, and coastal floods caused by rising sea levels and storm surges (Horton et al., 2012; Horton et al., 2014; Dupigny-Giroux et al., 2018). Climate in SE is generally humid and sub-tropical, influenced by seasons, latitude, topography, and the proximity to the Atlantic Ocean and the Gulf of Mexico. There are large areas of low-lying inland terrain and coastal plains in the region that are highly vulnerable to sea level rise, extreme heat events, decreased water availability, heavy precipitation, and hurricanes (Carter et al., 2014; Carter et al., 2018). The MW region experiences humid continental climate with warm summers and its diverse landscape is dominated by agricultural land use. The region has a moderate amount of precipitation throughout the year, but winter and spring precipitation are important to flood risk (Angel et al., 2018). Temperature, heavy precipitation events, droughts, and floods are increasing in MW (Pryor et al., 2014). The predominantly arid NGP region features a highly variable climate with a strong east-to-west gradient of decreasing precipitation. Most of the precipitation in the area falls during the spring. The region is highly susceptible to a range of climate change effects, particularly those arising from hydrological changes, including seasonality and timing of precipitation events, and extreme flooding and droughts (Conant et al., 2018). SGP exhibits a range of climates, spanning from arid, high-elevation borders adjacent to the mountains in the western region to humid areas in the eastern region. The average annual precipitation varies between 255 mm and 1500 mm, from the western to the southeastern corner (Kloesel et al., 2018). Both Great Plains regions are characterized by frequent floods, droughts, severe storms, tornadoes, hurricanes, and winter storms. High regional diversity in the NGP and SGP climate is a result of changes in elevation (Shafer et al., 2014). The NW region exhibits an intricate and diverse topography and several climate zones ranging from dry-summer-sub-Arctic to dry-summer-Mediterranean with varying patterns of precipitation (Mote et al., 2014). Extreme weather occurrences like heat waves, droughts, and flooding, with associated landslide risk, together with climate change, have had a detrimental impact on the NW’s regional ecosystem (May et al., 2018). SW, characterized by arid landscapes and desert-like conditions, is considered the driest region in CONUS, with hot summers and mild winters. The region is confronted with climate change challenges such as severe droughts, intermittent large-scale flooding, and high-water demand (Garfin et al., 2014; Gonzalez et al., 2018).
2.2 Datasets
The National Center for Atmospheric Research (NCAR) developed CESM2-LE, the most recent version of a coupled climate/Earth system model with 100-km grid spacing and a 100-member ensemble, in accordance with the CMIP6 protocols (Danabasoglu et al., 2020). CMIP6 is based on GCMs, which include high resolution ocean, atmosphere, land, sea-ice, land-ice, river, and wave models, as well as SSP scenarios (Babaousmail et al., 2021). SSPs integrate five distinct socioeconomic developments: sustainable development (SSP1), middle-of-the-road development (SSP2), regional rivalry (SSP3), inequality (SSP4), and fossil fuel-driven development (SSP5) ranging from aggressive climate action (SSP1-2.6) to the absence of climate change policy (SSP5-8.5) along with radiative forcing levels (Riahi et al., 2017; Alaminie et al., 2021; Supharatid et al., 2022). SSP3-7.0 with radiative forcing 7.0 W/m2 falls between the moderate SSP4-6.0 and worst-case SSP5-8.5 scenarios. The CMIP6 projects have a simulation span from 1850 to 2100, comprising historical experiments (1850–2014) and future projections (2015–2100), with input from natural forcing and anthropogenic influence as well as scenarios for future climate change (Eyring et al., 2016). In this study, the simulated precipitation dataset from 70 ensemble members of the CESM2-LE of the CMIP6 for a medium-to-high emission scenario (SSP3-7.0) at 100-km resolution is used from 1948 to 2100. The reduced number of ensemble members (70 instead of 100) is based on data availability at the time of the study. However, the authors believe their results would not be largely impacted by the addition of 30 ensemble members.
The CPC dataset is available on a 0.25° regular grid (corresponding to approximately 28 km) over CONUS and is based on station measurements from the U.S. unified rain gauge data (Higgins et al., 2000). Station records are extensively quality controlled through comparison with available sources such as historical records, nearby independent in situ measurements, satellite estimates, and numerical model forecasts (Hou et al., 2014). The optimal interpolation algorithm is used to interpolate quality-controlled station data from NOAA/National Climatic Data Center (NCDC) River Forecast Centers (RFCs) to create CPC daily precipitation analyzed fields that account for orographic effects (Xie et al., 2007; Cui et al., 2017). According to past studies, the bias in the CPC dataset is less than 0.5% compared to the average amount of precipitation observed by gauges over CONUS (Chen et al., 2008). This study evaluates historical climate model simulations against ground-based CPC daily observations across CONUS from 1948 to 2022. CPC has been chosen for this study because of its long record and its consistent, high-quality gridded precipitation product. In the second part of this study, the historical dataset CPC is used for comparison with future projections simulated by CESM2-LE.
2.3 Methodological approach
This study presents an assessment of CESM2-LE Climate Model precipitation simulations against CPC gauge data during 1948–2022 across CONUS. Specifically, the average of the 70 CESM2-LE ensemble members and three randomly selected members are compared to CPC ground-based observations. A seasonal analysis is performed, and seasons are defined as follows: spring includes March, April, May; summer includes June, July, August; fall includes September, October, November; and winter includes the months of December, January, and February. CPC observations at their native spatial resolution of 28 km are aggregated to a regular 100-km grid to match the spatial resolution of CESM2-LE using the nearest neighbor interpolation method. As models and ground observations are inherently biased (as proven in Section 3.1), a pixel-by-pixel bias correction is performed on future projections for the results presented in Section 3.2. The bias correction method used in this study follows a multiplicative bias ratio approach, which has been widely applied in climate model bias correction (e.g., Teutschbein and January 2012; Hempel et al., 2013). The bias ratio is computed as the ratio of the mean CESM2-LE model simulations to the mean CPC observations over the historical period (1948–2022). The CESM2-LE model projections are then bias-corrected by dividing the model data by this ratio. This approach ensures that the long-term mean climatology of the model aligns with observations while preserving interannual variability, making it suitable for hydrological applications. Historical annual and seasonal mean of CPC observations from 1948 to 2022 are also compared to future precipitation projections of CESM2-LE from 2023 to 2100. The selection of the historical period (1948–2022) and future projections (2023–2100) is based on data availability of both observations and future climate projections at the time of the study. The length discrepancy between the historical and future timeframes is not expected to impact our analysis of long-term climate trends. The two datasets are also compared based on the 75th and 95th probability distribution percentiles.
3 Results and discussions
3.1 Evaluation of historical climate model simulations
Monthly mean precipitation from three randomly selected CESM2-LE ensemble members and from the 70-member ensemble mean is compared to CPC precipitation. The box plots in Figure 2 present monthly precipitation averaged across CONUS during 1948–2022. As expected, larger magnitudes of precipitation are observed in winters compared to the summer months (especially July and August). Precipitation variability (i.e., the interquartile range) during the summer months is narrower compared to other seasons. Although the three single ensemble members struggle to capture high precipitation magnitudes in June, precipitation variability as observed by CPC is well captured by the ensemble members. However, the ensemble mean shows a largely reduced variability in precipitation across all months due to the averaging of all 70 ensemble members. The ensemble mean exhibits reduced precipitation variability compared to individual ensemble members, as averaging inherently smooths internal climate fluctuations. While single ensemble members capture short-term variability more effectively, the ensemble mean provides a more stable representation of long-term climate signals, which is crucial for identifying trends in future precipitation patterns (Frankcombe et al., 2018).
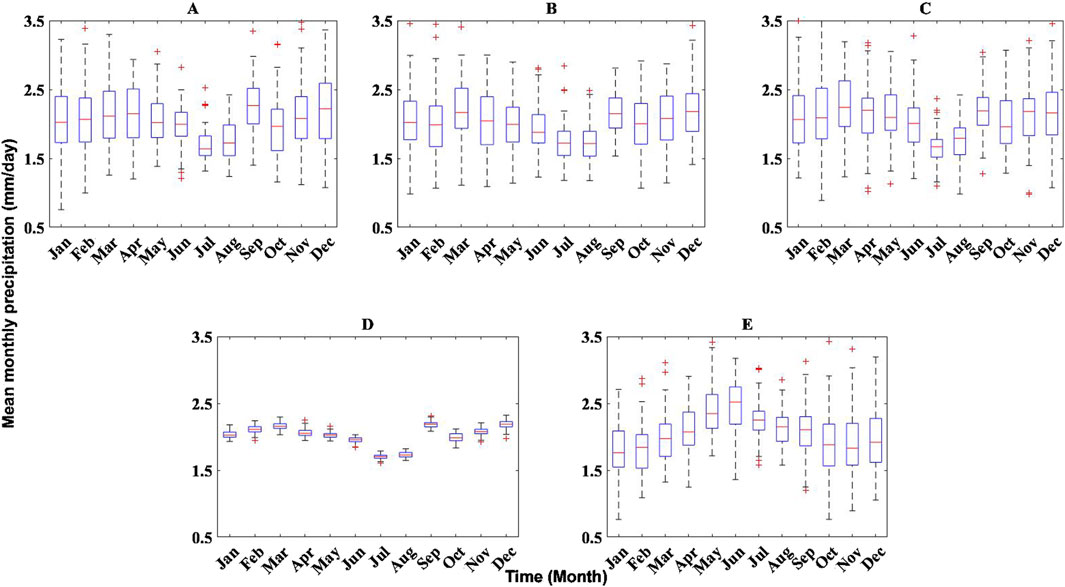
Figure 2. Box plots of monthly mean precipitation during 1948–2022 averaged over CONUS for (A–C) three randomly selected CESM2-LE ensemble members; (D) the mean of 70 ensemble members; and (E) CPC gauge observations. The central red mark indicates the median, and the bottom/top edges indicate the 25th/75th percentiles, the whiskers show the most extreme points not considered outliers, whereas the red crosses represent outliers.
Time series of seasonal and annual precipitation averages in Figure 3 corroborate what has been observed in the box plots, i.e., the ensemble mean does not fully capture precipitation variability as detected by the ground data and the single ensemble members. During the summer season, the ensemble mean remains consistently below 2 mm/day over the study period. The CESM2-LE single ensemble members show good agreement with CPC in spring and fall, but they present a strong negative bias in the summer (i.e., the model consistently underestimate the ground observations) and a strong positive bias in the winter (i.e., the model consistently overestimate the reference). Despite progressive improvements in the recent past, CMIP climate models are still affected by uncertainties due to errors in boundary conditions, inaccurate model parameterization as well as systematic summer warm biases in air temperature especially in the U.S. Central Great Plains (CGP) regions (Knutti and January 2012; Mueller and Seneviratne, 2014; Wang et al., 2014; Merrifield and Xie, 2016). As air temperature plays a central role in the interconnected feedback loops between various components of the climate system, warm biases can influence CMIP model simulations of different climate variables (Cheruy et al., 2014). When analyzing annual precipitation estimated by CESM2-LE, the three model ensemble members present a better agreement with CPC than the ensemble mean (Panel E in Figure 3). This is particularly evident when assessing precipitation variability throughout the study period, which is clearly dampened by the ensemble mean. This dampening effect occurs because averaging multiple ensemble members smooths out internal variability, reducing the influence of short-term fluctuations and stochastic climate processes (Deser et al., 2012; Kay et al., 2015).
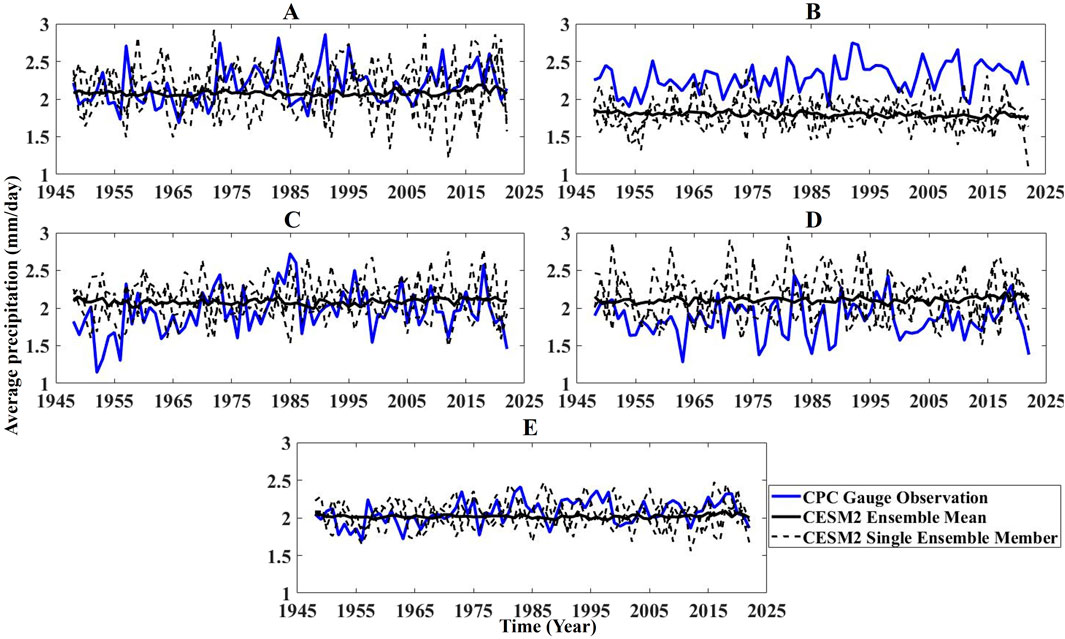
Figure 3. Time series of (A) spring; (B) summer; (C) fall; (D) winter; and (E) annual precipitation averages over CONUS in mm/day from 1948 to 2022 for CPC gauge observation (blue solid line), CESM2-LE mean of 70 ensemble members (black solid line), and three CESM2-LE ensemble members (black dashed line).
In terms of spatial analysis, both CESM2-LE single ensemble members and the ensemble mean display similar patterns (Figure 4). However, CESM2-LE struggles to capture the high intensity daily precipitation in the NW (as high as 10 mm/day) measured by CPC. Furthermore, large annual precipitation is concentrated in the SE region (as high as 5 mm/day) in CPC, whereas CESM2-LE model shows high amount of daily precipitation (as high as 4 mm/day) in the NE and certain areas of MW. CESM2-LE tends to overestimate precipitation in NE (by as much as ∼47%) as shown in relative difference maps in Figure 4. CESM2-LE exhibits a dry bias in the western US, particularly in the NW (as high as ∼89%), southern SGP, and SE (up to ∼63%). On the other hand, the model presents a wet bias in certain NGP, SW (as high as ∼100%), and MW regions (with values up to ∼62%).
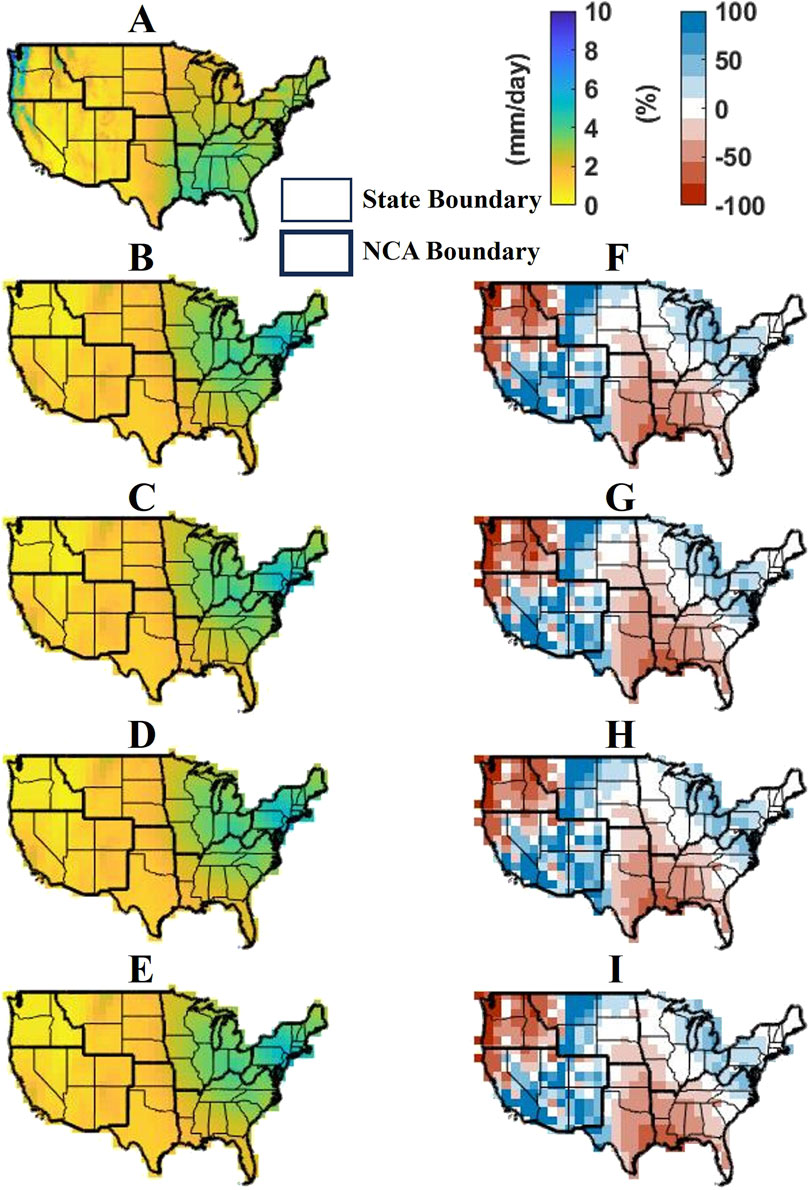
Figure 4. Mean annual precipitation during 1948–2022 for (A) CPC; (B–D) three CESM2-LE ensemble members; (E) CESM2-LE ensemble mean; The relative difference (%) between CPC and (F–H) each ensemble member and (I) the ensemble mean is presented in the second column panels. Red (blue) color refers to an underestimation (overestimation) of the model with respect to the reference.
The missed precipitation patterns might be attributed to inaccurate simulations of mesoscale convective systems due to problematic convective parameterizations in models (Dai et al., 1999; Liang et al., 2007; Lin et al., 2017; Al-Yaari et al., 2019; Srivastava et al., 2020). Climate model simulations may not be able to account for small-scale convective processes to initiate short duration precipitation events (Maraun et al., 2010; Jones and Randall, 2011; Kendon et al., 2017; Barbero et al., 2019; Moustakis et al., 2021; Coelho et al., 2022; Emmanouil et al., 2022). Though improvements might be made by using convection permitting models, it can be computationally expensive and only available for limited regions and short time periods (Cannon and Innocenti, 2019; Ban et al., 2020).
3.2 Historical versus future precipitation patterns
As the three randomly selected ensemble members show very similar spatial patterns in our previous results, a single member will be used in this section. Furthermore, CPC observations are chosen here to study historical patterns and compare them to future projections. Given the biases observed in the model in the previous section, a pixel-by-pixel bias correction is performed on the model future projections prior to the analyses presented in this section. The annual cycles (1948–2022) of percentiles of CPC average precipitation are compared to the corresponding percentiles obtained from a CESM2-LE randomly selected ensemble member and the mean of the 70 ensemble members during 2023–2100 (Figure 5). The variability around the mean is higher in the future model projections than the historical CPC data when one single ensemble member is chosen, showing a very similar range of precipitation values. However, such variability is drastically reduced when considering the ensemble mean, with values very close to 2 mm/day across the year. Although historical data show a peak between June and July, future projections indicate a decay in summer precipitation with values lower than spring and fall precipitation. This will be critical for water resources management, e.g., irrigation of crops during the summer.
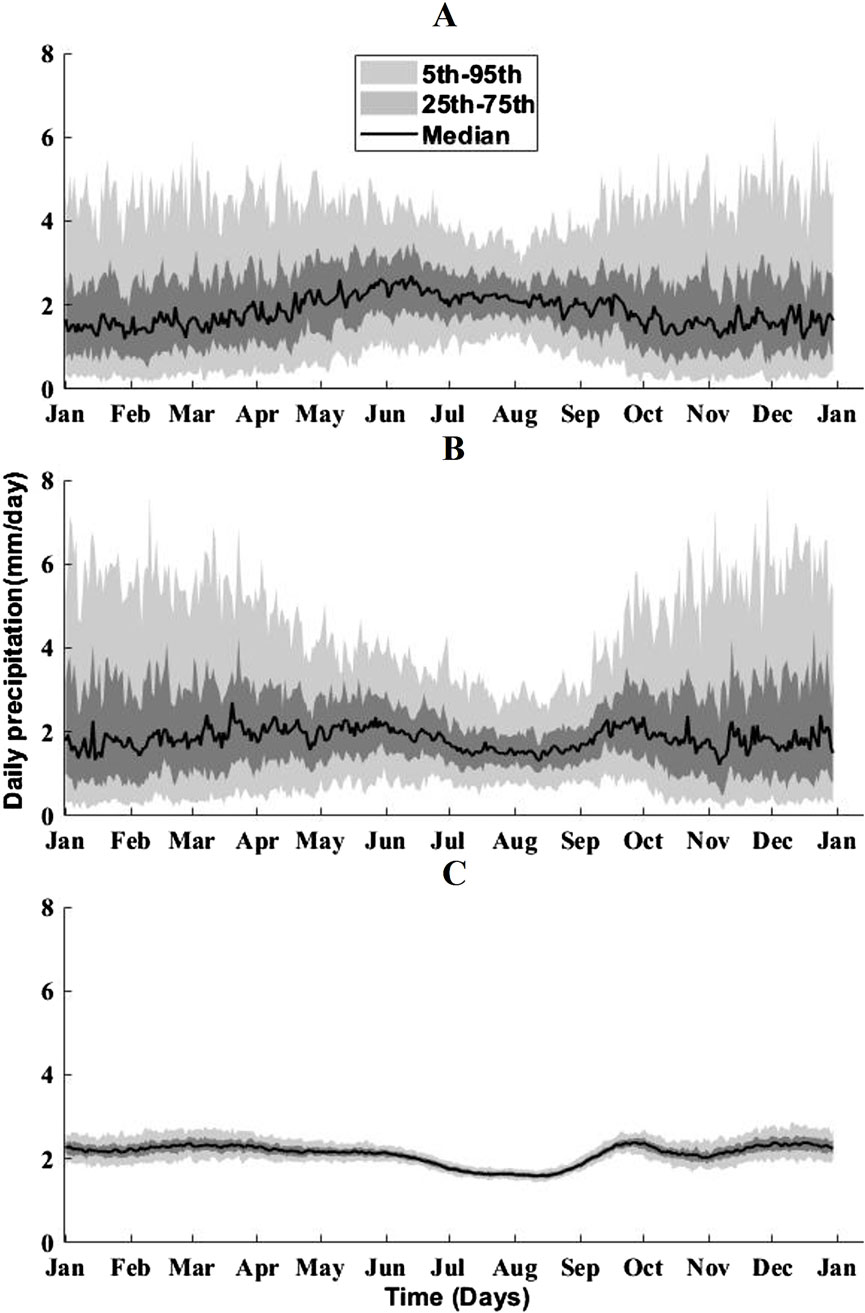
Figure 5. Time series of daily precipitation averaged across CONUS for (A) CPC observations (1948–2022); (B) a bias-corrected CESM2-LE single ensemble member (2023–2100); and (C) the bias-corrected ensemble mean (2023–2100). The 5th, 25th, 50th (median), 75th, and 95th percentiles of the precipitation distribution are shown.
Historical and future time series of seasonal and annual precipitation averages are presented in Figure 6. For historical precipitation, CPC and bias-corrected model simulations are compared to future model projections. Future summer precipitation presents a slightly decreasing pattern with respect to historical CESM2, whereas it shows a large decrease (∼50%) compared to the past observation of CPC, which can be attributed to the bias between the two datasets during this season that the overall bias correction was not able to fix. Nevertheless, this (even if slight) decreasing pattern is concerning and can have serious consequences especially for crops that require water in the root zone during the summer. On the other hand, an upward pattern is observed in winter future projections when compared to historical data. It would be interesting to investigate whether this trend is mainly due to an increase of rainfall or snowfall, which can have very different effects on the local and regional hydrology. Spring, fall, and annual precipitation present a slight increase in the future compared to the historical records as well. The variability is reduced in the ensemble mean with respect to single ensemble member in the future, which aligns with our findings in the previous chapter. The intrinsic stochastic nature of individual ensemble members is clearly overlooked when averaging all the ensemble members, resulting in such reduced variability.
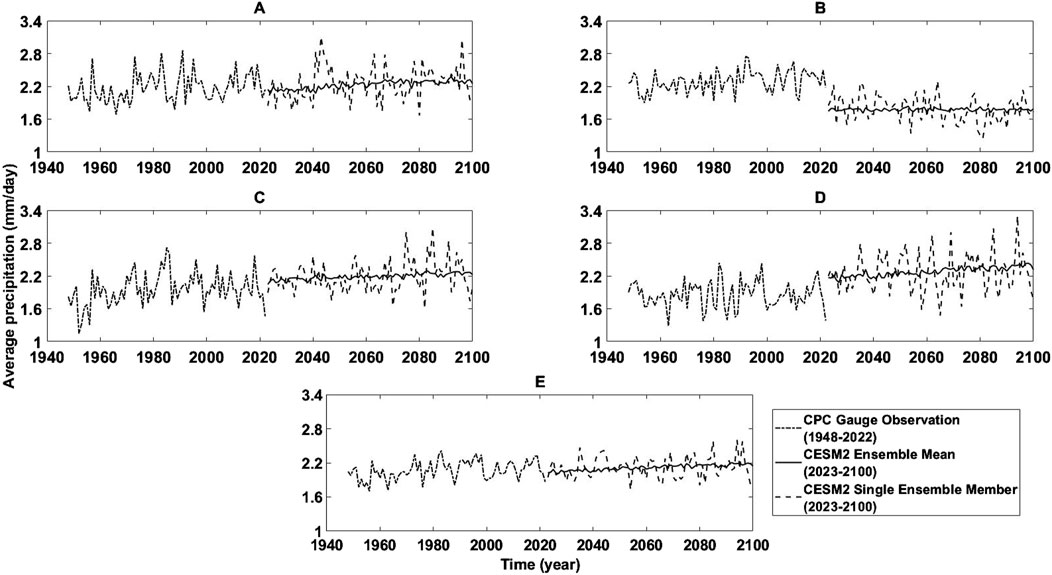
Figure 6. Time series of (A) spring; (B) summer; (C) fall; (D) winter; and (E) annual precipitation averages across CONUS from 1948 to 2100.
The Mann-Kendall (Mann, 1945; Kendall, 1948) test was applied to the time series in Figure 6 to assess the statistical significance of projected precipitation changes. M-K statistically assesses if there is a monotonic (upward or downward) trend in a time series, but the trend may or may not be linear. The M-K test is commonly employed to measure the significance of trends in hydrometeorological time series (Silva et al., 2015; Cooley and Chang, 2021). While historical trends were not found significant, the ensemble mean of future projections showed statistically significant increasing trends at a ∼90–95% confidence level for spring, fall, winter, and annual precipitation (Table 1). Future trends from a single ensemble member were found not significant. This highlights the ensemble mean’s effectiveness in capturing long-term precipitation patterns that individual ensemble members would not be able to.
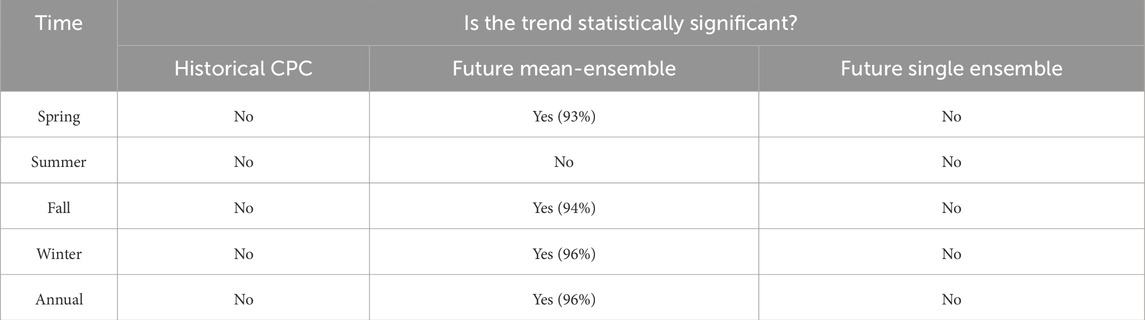
Table 1. Results of a M-K statistical significance test of time-series showed in Figure 6. For statistically significant trends, the relative confidence level (in %) is shown in parenthesis.
Maps of average past and future precipitation are displayed in Figure 7. Past precipitation shows large magnitudes in NW (higher than ∼2,500 mm) and northern SW (as much as ∼2,400 mm). The SE region also receives large amount of precipitation (up to ∼2,000 mm) along with NE (by as much as ∼1,600 mm) and MW (up to ∼1,300 mm). On one hand, SE, a historically wet region, shows an increase in the future with annual averages ∼150 mm higher than in the past along with NE (also a historically wet region), which is projected to increase, with annual averages ∼90 mm higher than in the past in some areas. On the other hand, the NGP, already an arid region, will get even drier in the future. The relative difference maps suggest that future precipitation could increase by as much as ∼11% in the MW and NE, which are already susceptible to intense precipitation events, and up to ∼15% in the already wet SE region. Certain areas in the eastern NGP show up to ∼15% increase in future precipitation, which could favor the arid landscapes of these regions, but it may also worsen the already existing large-scale floods. Western NGP precipitation will decrease up to ∼15%, which might exacerbate its arid conditions. Increased precipitation in already-wet regions and decreased precipitation in already-dry regions are observed globally and appear to persist into the future (Oki and Kanae, 2006; Feng and Zhang, 2015; Wu, 2015; Li et al., 2019). The SW region precipitation will increase by up to ∼10% in the future, which might be beneficial for the region’s arid conditions. Certain regions of NW show some precipitation decrease up to ∼11% in the future. Maps of future precipitation estimated by the single member and by the ensemble mean show similar patterns. However, the difference maps in Figure 7 highlight stronger decreases in precipitation in a few pixels in NW and SW, when one single member is used in place of the ensemble mean. Similarly, increases of future precipitation (compared to past precipitation) in the SE region are more evident in the single member analysis.
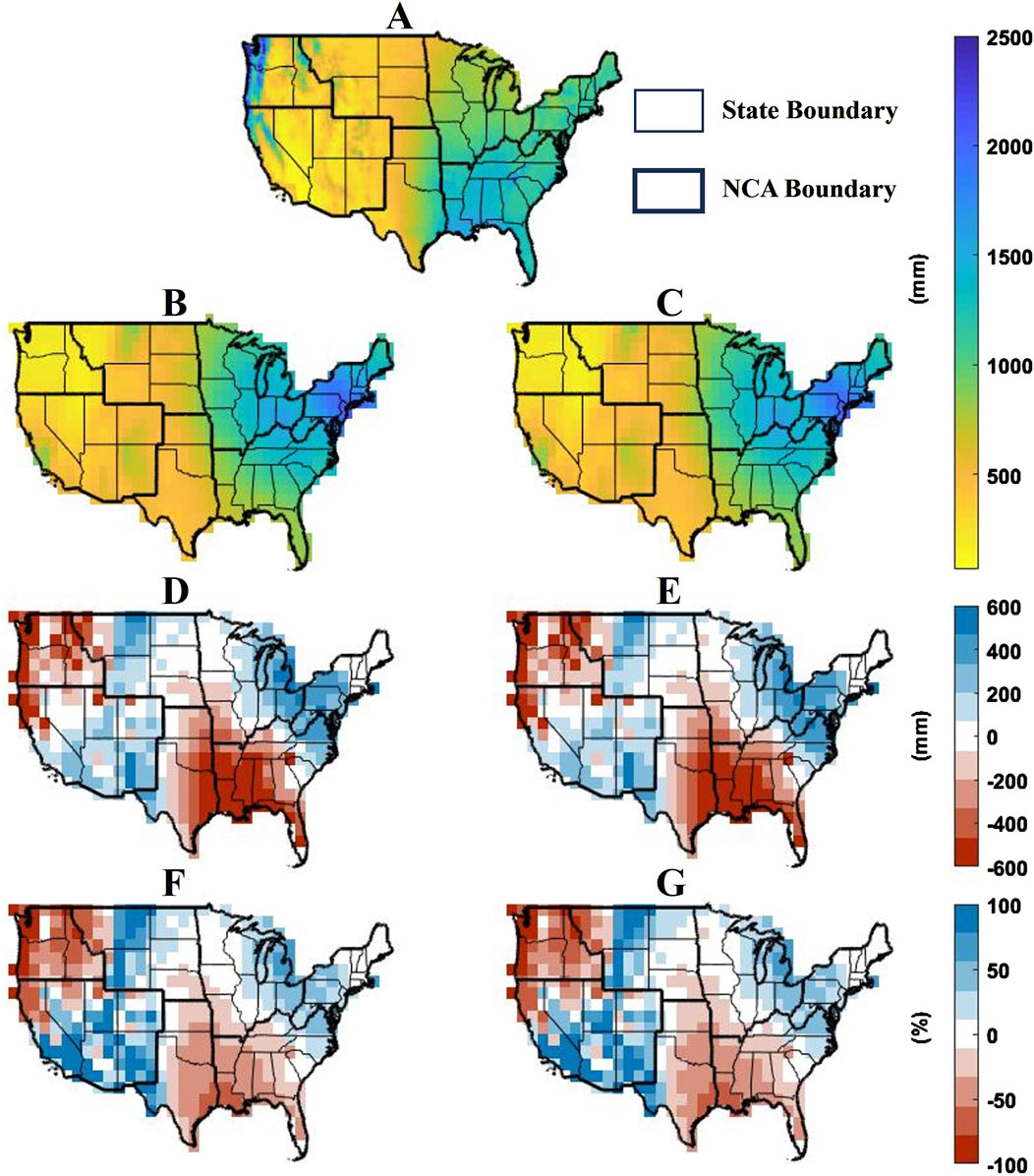
Figure 7. Annual average precipitation (mm) for (A) CPC historical observations; (B) future simulations from a single CESM2-LE ensemble member; (C) the ensemble mean of future CESM2-LE simulations. Panels in the third row show the difference in mm between (D) future projection from the single ensemble member; (E) future projection from the ensemble mean and historic CPC. The corresponding relative differences (%) are shown in panels (F) and (G).
Figure 8 presents past (CPC) and future (CESM2) total seasonal precipitation. Historically, the western region of NW and northern SW received the largest amount of precipitation (>∼1,000 mm) during the winter months, with relatively high magnitudes in spring and fall (up to ∼800 mm and ∼1,000 mm respectively), and low magnitudes (below ∼260 mm) in the summer. In the eastern CONUS, SE receives precipitation during all seasons (as much as ∼500 mm), with southern SE presenting higher magnitudes (as high as ∼700 mm) during the summer. MW, NE, and eastern SGP show precipitation as high as ∼400 mm during spring and summer. NGP records the lowest precipitation amounts among all regions, especially during fall (by as much as ∼300 mm).
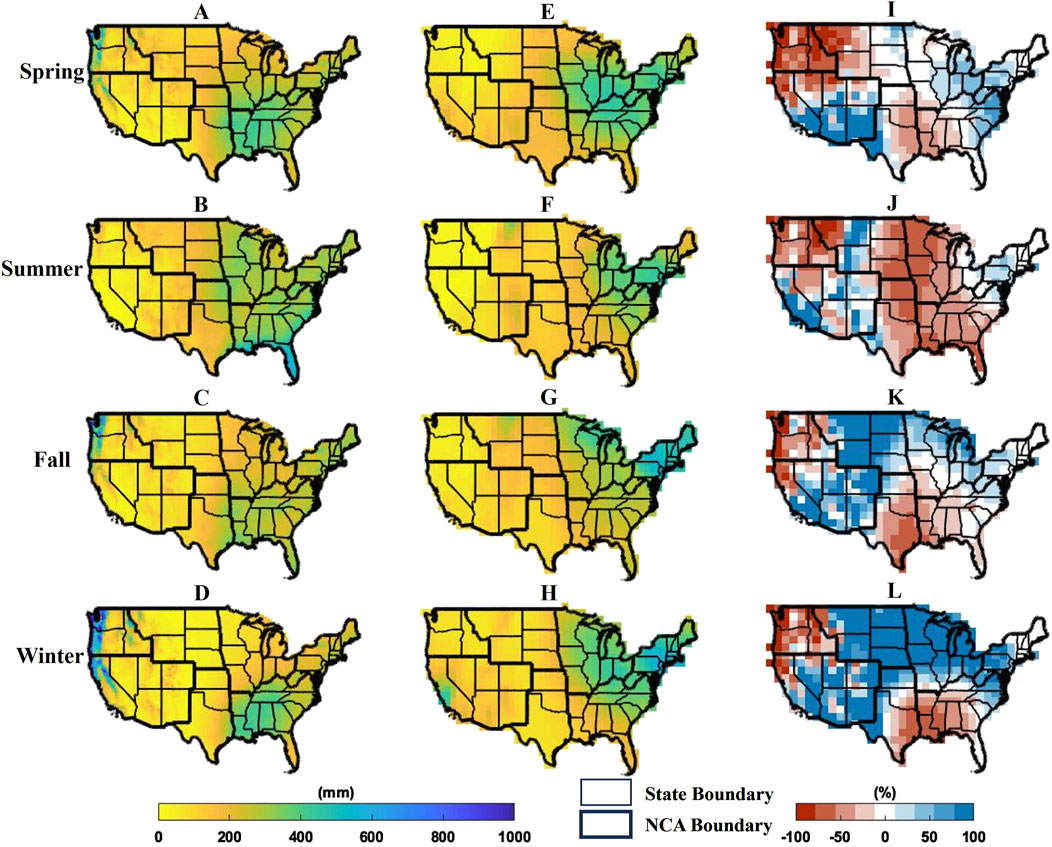
Figure 8. Total precipitation averages (mm) across different seasons (rows) for (A–D) historical CPC observations (1948–2022) and (E–H) future projections (2023–2100) from one CESM2-LE randomly selected ensemble member. Relative difference (%) between future projections and historic CPC are presented in (I–L).
Precipitation patterns in the western NW and SW areas will not change much in the future, except for summer and spring increases (up to ∼100%) in some sub-regions (e.g., southern SW). The precipitation amount in the southern SE will decrease during summer (∼50%) and increase in other seasons (∼100%). MW, NGP, and northern SGP precipitation will increase during winter (by as much as ∼100%) and decrease during summer (by as much as ∼75%, ∼80%, and 60% respectively). NGP precipitation will also increase in fall (∼100%) and decrease in spring (∼80%), which might negatively affect the predominantly arid NGP region, where the majority of precipitation occur during the spring season. Winter precipitation in the already wet NE region will increase (∼80%). Overall results indicate drier summers and wetter winters in the future with respect to the past. Only future projections from a single ensemble member of CESM2-LE model are shown here, as seasonal spatial patterns of the ensemble mean and the single member are quite similar.
The 75th percentiles of total seasonal precipitation are displayed in Figure 9. In the CPC observations, NW exhibits the largest values, especially in winter (>∼1,800 mm). Other regions generally show less than ∼600 mm for the 75th percentile in all four seasons, except for a few pixels in Florida during summer (as high as ∼800 mm) and northern SW (up to ∼1,500 mm) during winter, fall, and spring. Future precipitation projections present the largest magnitudes in NW and northern SW (as high as ∼700 mm) during winter, while southern SW shows the lowest magnitudes (∼10 mm) during summer. Relative difference maps between future and historical summer precipitation indicate a decrease in the 75th percentile in MW (up to ∼70%), NGP (∼80%), and eastern SW (∼75%). However, western SW shows an increase in 75th percentiles (∼95%) during summer and southern SW shows increased percentiles (∼75%) during spring. In future winters, 75th percentiles are projected to increase (up to ∼100%) in MW, northern SGP, and NGP. NGP also shows an increase (∼100%) in fall and a decrease (∼80%) in spring. Certain areas in NGP and SW show lower values in spring (as low as ∼80%), whereas SW and SGP show decreases in fall (up to ∼55%). Summer 75% percentiles are not projected to change much in SE. NE region shows an increase (∼70%) in the 75th percentile during winter. Overall, 75th percentiles of precipitation show increases during winter and decreases during summer in most of the NCA regions.
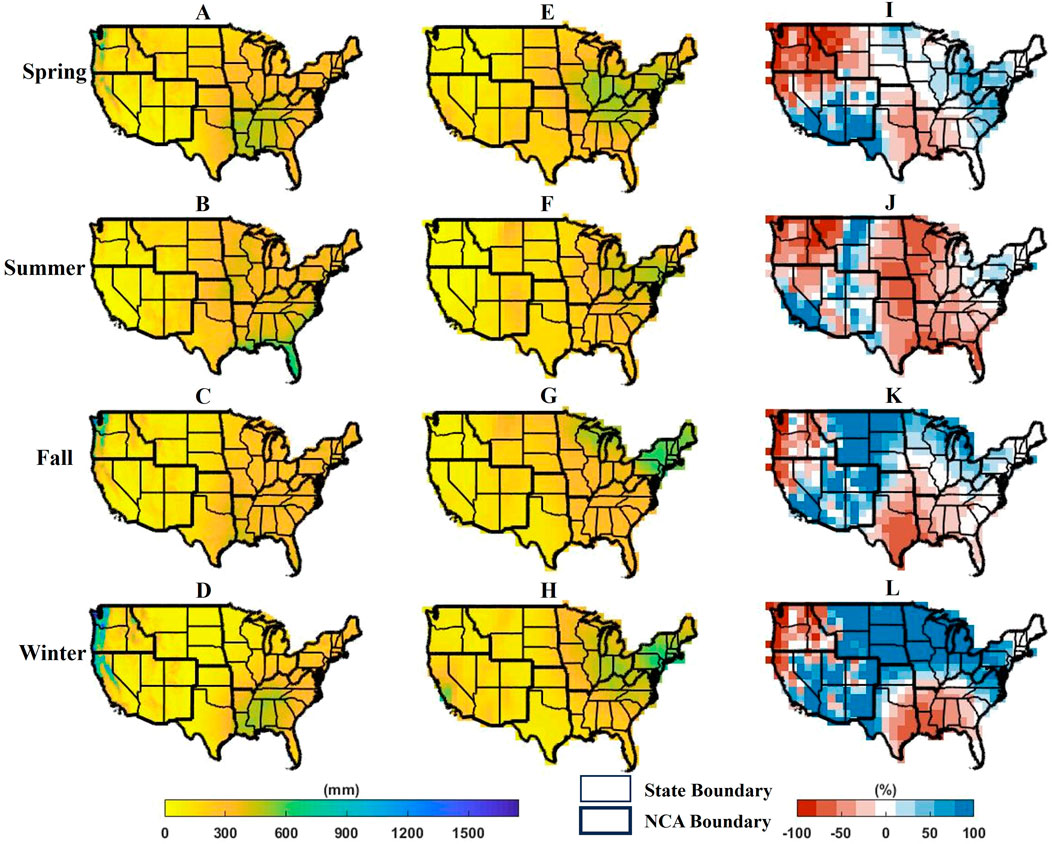
Figure 9. 75th percentile of total seasonal precipitation averaged over the years (mm) across different seasons (rows) for (A–D) historical CPC observations (1948–2022) and (E–H) future projections (2023–2100) from one CESM2-LE randomly selected ensemble member. Relative difference (%) between future projections and historic CPC are presented in (I–L).
The high end of the precipitation probability distributions is analyzed by looking at maps of the 95th percentiles for each season (Figure 10). NW shows the largest amount of historical extreme precipitation among all NCA regions during winter with magnitudes greater than ∼2,000 mm, with future projections showing even higher percentiles (up to ∼100% higher). Several other regions will also experience similar increases in extreme winter precipitation, i.e., NE, NGP, MW, and northern SGP. Summers will generally experience lower 95th percentiles with respect to the past in many areas across CONUS including SW, NGP, MW, and northern SGP.
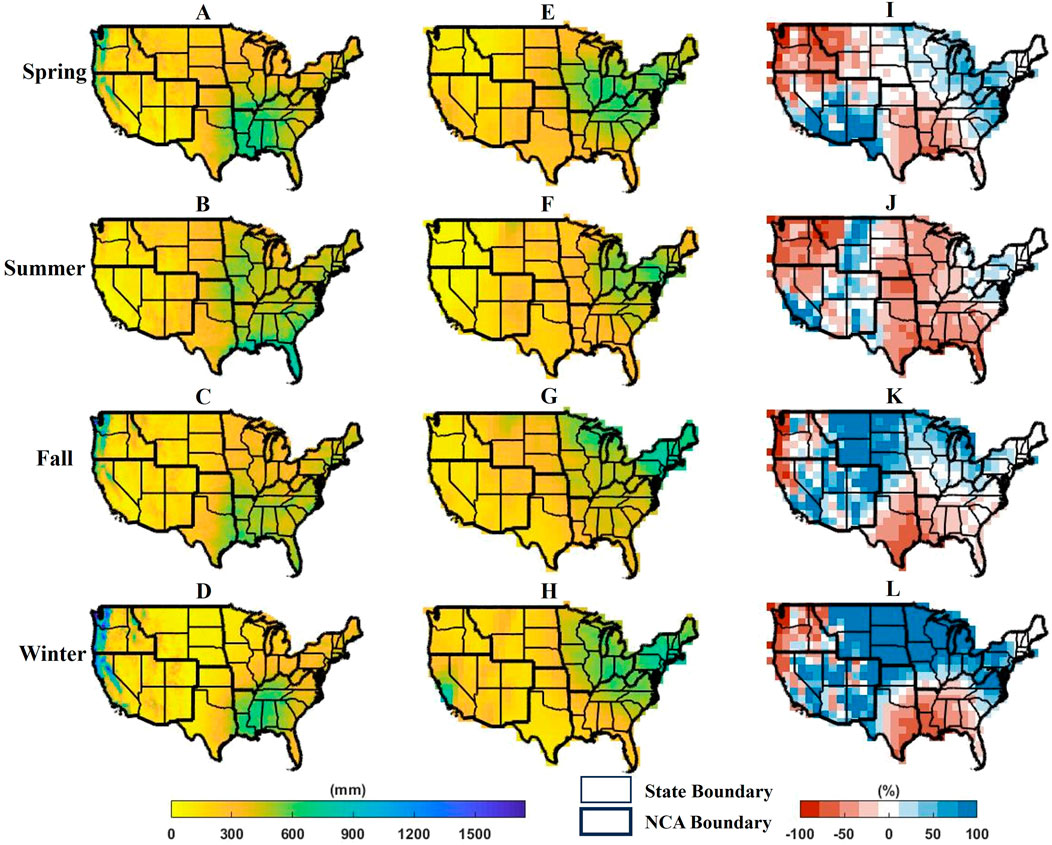
Figure 10. 95th percentile of total seasonal precipitation averaged over the years (mm) across different seasons (rows) for (A–D) historical CPC observations (1948–2022) and (E–H) future projections (2023–2100) from one CESM2-LE randomly selected ensemble member. Relative difference (%) between future projections and historic CPC are presented in (I–L).
Although SE presents high 95th percentiles in the historical time series, especially during summer (up to ∼1,000 mm), changes are minimal in the future. The SW extreme precipitation will decrease in all seasons, especially during the summer (up to ∼75%). NGP shows the lowest extreme precipitation across CONUS, especially during fall (∼70–500 mm), but future projections indicate an increase by ∼100–1,100 mm. Spatial patterns of the 75th and 95th percentiles are similar for all NCA regions, except for NW which shows increases up to ∼100% in 95th percentile precipitation (but lower in the 75th percentile) and some cases in which the 75th percentile shows larger future changes. For instance, spring precipitation in SGP presents changes by up to ∼100% in the 75th percentile, but ∼60% in the 95th.
Overall findings indicate that, in comparison to the past, extreme precipitation magnitudes will decrease in the summer and increase in the winter in most of the NCA regions across CONUS. If extremes become more extreme in the future, it will worsen existing climatic conditions for some NCA regions. Besides the NE, SE, and NGP regions, MW and SW also face critical climate challenges. Specifically, MW is already facing flood risk due to heavy precipitation during spring and winter–results from this study indicate that extreme precipitation will not change much in the spring but will get more extreme in winter threatening the existing conditions. Similarly, SW, characterized by arid conditions, may see further reductions in summer precipitation, intensifying drought risks and water scarcity concerns. These findings highlight the complex regional disparities in future precipitation trends. The projected increase in winter precipitation, particularly in flood-prone regions like MW and NE, may exacerbate flooding risks, threatening infrastructure, agriculture, and water resource management. Conversely, declining summer precipitation in regions like NGP and MW could intensify drought conditions, affecting crop yields and increasing water scarcity. These findings underscore the need for adaptive strategies in flood mitigation and water resource management to address changing hydroclimatic risks and minimize socio-economic impacts.
Although this study highlights broad seasonal trends, the complexity of inter-seasonal variability should not be overlooked. Transitional seasons (spring-to-summer and fall-to-winter) remain an important factor influencing agriculture, water availability, and ecosystem resilience. Fluctuations within seasons—such as shifts in the timing of precipitation onset, intensity variations, and extreme precipitation events—can significantly impact growing seasons, reservoir management, and biodiversity. Recent studies on South Asian precipitation extremes and variability suggest that large-scale climate drivers greatly influence these transitional periods, contributing to hydroclimatic uncertainties (Abbas et al., 2022b; Abbas et al., 2023; Wijeratne et al., 2023). Recognizing these variations is essential for developing more adaptive strategies in climate impact assessments.
4 Conclusion
This study assessed the performance of the CESM2-LE large ensemble climate model against ground observations and examined past and future precipitation patterns. Results indicate that precipitation variability is reduced when the ensemble mean is adopted in place of individual ensemble members. Nevertheless, the spatial patterns of precipitation for a single random ensemble member and for the average of the 70 ensemble members are very similar.
Considering CPC as reference, CESM2-LE was shown to underestimate summer precipitation and overestimate winter precipitation across CONUS. CESM2-LE struggles to capture the high daily precipitation in the NW (as high as 10 mm/day) measured by CPC. The missed precipitation patterns might be attributed to the problematic convective parameterizations in models. Bias correction was performed in the comparison of historical and future precipitation for model projections to remove any systematic error between model simulations and ground observations.
Historical precipitation observations show slightly increasing patterns in annual, spring, fall, and winter averages, which are projected to continue in the future. Wet regions like NE and SE will become wetter, on average, in the future by ∼11% and ∼15%, respectively, whereas already dry regions (like some NGP areas) will get drier by as much as ∼15%. Seasonal spatial patterns show drier summers and wetter winters in the future with respect to the past. A ∼100% increase is observed in most NCA regions (e.g., MW, NGP, and northern SGP) during winter, but precipitation will decrease during summer by as much as ∼80%. Extreme (75th and 95th percentiles) precipitation will become more extreme during winter by as much as ∼100% and less extreme during summer in the future by as much as ∼80%. Spring and fall extreme precipitation will also increase in the future in most of the NCA regions, except for certain areas in NGP, SW, and SGP.
The findings place strong emphasis on reliable climate model projections to better understand long term-precipitation patterns, climatology, and possible climate change impacts. A fundamental step to develop climate change adaptation and mitigation strategies is to project future climates using high-resolution models and compare future precipitation patterns to past observations. Findings from this work point to future wetter winters and dryer summers, which may raise concerns in agriculture and water resources management. Investigating projected changes also offers information on regional vulnerability and encourages the development of region-specific policy implementation across CONUS. Actionable strategies, such as climate-resilient agriculture, sustainable infrastructure development, urban planning measures including improved stormwater management, and drought mitigation activities, can support community-driven adaptation plans. This study focuses on leveraging the large ensemble size of CESM2-LE to ensure consistent model physics and resolution, avoiding inter-model discrepancies. However, a single-model approach limits intercomparison, and results may not capture the full range of uncertainty that arises from structural differences in other models. While CESM2-LE offers valuable insights, its limitations in capturing high-magnitude precipitation highlight the need for inter-model comparisons. In addition, a single emission scenario is employed in this study and, although SSP3-7.0 aids in detecting strong climate signals for worst-case risk assessments, relying on a single emission scenario approach might limit policy-relevant insights across different future pathways. Future studies are encouraged to expand our analysis to multiple future scenarios. Further work should explore multi-model ensemble approaches and multiple emission scenarios, which may better characterize the uncertainty of future projections and provide more robust signals of changing climatology. Other regions in the world should also be investigated, given the global nature of climate models.
Data availability statement
Publicly available datasets were analyzed in this study. This data can be found here: https://www2.cesm.ucar.edu/CESM2-LENS2/.
Author contributions
RS: Formal Analysis, Investigation, Methodology, Validation, Writing – original draft, Writing – review and editing. VM: Conceptualization, Project administration, Resources, Supervision, Visualization, Writing – review and editing. ID: Conceptualization, Writing – review and editing.
Funding
The author(s) declare that no financial support was received for the research and/or publication of this article.
Acknowledgments
The authors acknowledge the CESM2 Large Ensemble Community Project for providing data. The authors also would like to thank Gustavo de A. Coelho, Assistant Professor of Water Resources at Furman University who originally downloaded and cropped the climate model data used in the analysis, while doing his PhD in George Mason university.
Conflict of interest
The authors declare that the research was conducted in the absence of any commercial or financial relationships that could be construed as a potential conflict of interest.
The author(s) declared that they were an editorial board member of Frontiers, at the time of submission. This had no impact on the peer review process and the final decision.
Generative AI statement
The author(s) declare that no Generative AI was used in the creation of this manuscript.
Publisher’s note
All claims expressed in this article are solely those of the authors and do not necessarily represent those of their affiliated organizations, or those of the publisher, the editors and the reviewers. Any product that may be evaluated in this article, or claim that may be made by its manufacturer, is not guaranteed or endorsed by the publisher.
References
Abbas, A., Bhatti, A. S., Ullah, S., Ullah, W., Waseem, M., Zhao, C., et al. (2023). Projection of precipitation extremes over South asia from CMIP6 GCMs. J. Arid Land 15 (3), 274–296. doi:10.1007/s40333-023-0050-3
Abbas, A., Ullah, S., Ullah, W., Waseem, M., Dou, X., Zhao, C., et al. (2022a). Evaluation and projection of precipitation in Pakistan using the coupled model intercomparison project phase 6 model simulations. Int. J. Climatol. 42 (13), 6665–6684. doi:10.1002/joc.7602
Abbas, A., Zhao, C., Waseem, M., khan, K. A., and Ahmad, R. (2022b). Analysis of energy input–output of farms and assessment of greenhouse gas emissions: a case study of cotton growers. Front. Environ. Sci. 9 (February), 1–11. doi:10.3389/fenvs.2021.826838
Agel, L., and Barlow, M. (2020). How well do CMIP6 historical runs match observed Northeast U . S. Precipitation and extreme precipitation – related circulation. J. Clim. 33, 9835–9848. doi:10.1175/JCLI-D-19-1025.1
Ahmadalipour, A., Rana, A., Moradkhani, H., and Sharma, A. (2017). Multi-criteria evaluation of CMIP5 GCMs for climate change impact analysis. Springer, 71–87. doi:10.1007/s00704-015-1695-4
Akinsanola, A. A., Kooperman, G. J., Reed, K. A., Pendergrass, A. G., and Hannah, W. M. (2020). Projected changes in seasonal precipitation extremes over the United States in CMIP6 simulations. Environ. Res. Lett. 15, 104078. doi:10.1088/1748-9326/abb397
Akinsanola, A. A., Ongoma, V., and Kooperman, G. J. (2021). Evaluation of CMIP6 models in simulating the statistics of extreme precipitation over eastern africa. Atmos. Res. 254, 105509. doi:10.1016/j.atmosres.2021.105509
Alaminie, A. A., Tilahun, S. A., Legesse, S. A., Zimale, F. A., Tarkegn, G. B., and Jury, M. R. (2021). Evaluation of past and future climate trends under CMIP6 scenarios for the UBNB (abay), Ethiopia. Water 13, 2110. doi:10.3390/w13152110
Almazroui, M., Saeed, F., Saeed, S., Islam, M. N., Ismail, M., Klutse, N. A. B., et al. (2020). Projected change in temperature and precipitation over africa from CMIP6. Earth Syst. Environ. 4 (3), 455–475. doi:10.1007/s41748-020-00161-x
Al-Yaari, A., Ducharne, A., Cheruy, F., Crow, W. T., and Wigneron, J. (2019). Satellite-based soil moisture provides missing link between summertime precipitation and surface temperature biases in CMIP5 simulations over conterminous United States. Sci. Rep. 9, 1657–1712. doi:10.1038/s41598-018-38309-5
Angel, J., Swanston, C., Boustead, B. M., Conlon, K. C., Hall, K. R., Jorns, J. L., et al. (2018). in Ch 21: midwest. Impacts, risks, and adaptation in the United States: fourth national climate assessment, volume II. Editors D. R. Reidmiller, C. W. Avery, D. R. Easterling, K. E. Kunkel, K. L. M. Lewis, T. K. Maycocket al. (Washington, DC, USA: U.S. Global Change Research Program), 872–940. doi:10.7930/NCA4.2018.CH21
Babaousmail, H., Hou, R., Ayugi, B., Ojara, M., Ngoma, H., Karim, R., et al. (2021). Evaluation of the performance of CMIP6 models in reproducing rainfall patterns over north africa. Atmosphere 12, 475–525. doi:10.3390/atmos12040475
Ban, N., Rajczak, J., Schmidli, J., and Schär, C. (2020). Analysis of alpine precipitation extremes using generalized extreme value theory in convection - resolving climate simulations. Springer, 61–75. doi:10.1007/s00382-018-4339-4
Barbero, R., Fowler, H. J., Blenkinsop, S., Westra, S., Vincent, M., Elizabeth, L., et al. (2019). A synthesis of hourly and daily precipitation extremes in different climatic regions. Weather Clim. Extrem. 26, 100219. doi:10.1016/j.wace.2019.100219
Beck, H. E., McVicar, T. R., Vergopolan, N., Berg, A., Lutsko, N. J., Dufour, A., et al. (2023). High-resolution (1 Km) köppen-geiger maps for 1901–2099 based on constrained CMIP6 projections. Sci. Data 10 (1), 1–17. doi:10.1038/s41597-023-02549-6
Branstator, G., and Selten, F. (2009). Modes of variability and climate change. J. Clim. 22, 2639–2658. doi:10.1175/2008JCLI2517.1
Cannon, A. J., and Innocenti, S. (2019). Projected intensification of sub-daily and daily rainfall extremes in convection-permitting climate model simulations over north America: implications for future intensity – duration – frequency curves. Nat. Hazards Earth Syst. Sci. 19, 421–440. doi:10.5194/nhess-19-421-2019
Carter, L., Terando, A., Dow, K., Hiers, K., Kunkel, K. E., Lascurain, A., et al. (2018). “Ch 19: Southeast,”. Impacts, risks, and adaptation in the United States: fourth national climate assessment. Editors D. R. Reidmiller, C. W. Avery, D. R. Easterling, K. E. Kunkel, K. L. M. Lewis, T. K. Maycocket al. Washington, DC, USA: U.S. Global Change Research Program, II, 743–808. doi:10.7930/NCA4.2018.CH19
Carter, L. M., Jones, J. W., Berry, L., Burkett, V., Murley, J. F., Obeysekera, J., et al. (2014). “Chapter 17: Southeast and the Caribbean,”. In Climate Change Impacts in the United States: The Third National Climate Assessment. Editors J. M. Melillo, T. T. C. Richmond, and G. W. Yohe (U.S. Global Change Research Program), 396–417. doi:10.7930/J0NP22CB
Chatterjee, S., Khan, A., Akbari, H., and Wang, Y. (2016). Monotonic trends in spatio-temporal distribution and concentration of monsoon precipitation (1901–2002), West Bengal, India. Atmos. Res. 182, 54–75. doi:10.1016/j.atmosres.2016.07.010
Chen, H., Sun, J., Lin, W., and Xu, H. (2020). Comparison of CMIP6 and CMIP5 models in simulating climate extremes. Sci. Bull. 65 (17), 1415–1418. doi:10.1016/j.scib.2020.05.015
Chen, M., Shi, W., Xie, P., Silva, V. B. S., Kousky, V. E., Wayne Higgins, R., et al. (2008). Assessing objective techniques for gauge-based analyses of global daily precipitation. J. Geophys. Res. 113. doi:10.1029/2007JD009132
Chen, S., Gourley, J. J., Hong, Y., Kirstetter, P. E., Zhang, J., Howard, K., et al. (2013). Evaluation and uncertainty estimation of NOAA/NSSL next-generation national mosaic quantitative precipitation estimation product (Q2) over the continental United States. J. Hydrometeorol. 14 (4), 1308–1322. doi:10.1175/JHM-D-12-0150.1
Cheruy, F., Dufresne, J. L., Hourdin, F., and Ducharne, A. (2014). Role of clouds and land-atmosphere coupling in midlatitude continental summer warm biases and climate change amplification in CMIP5 simulations. Geophys. Res. Lett. 41, 6493–6500. doi:10.1002/2014GL061145
Coelho, G.De A., Ferreira, C. M., Johnston, J., Kinter, J. L., Dollan, I. J., and Maggioni, V. (2022). Potential impacts of future extreme precipitation changes on flood engineering design across the contiguous United States. Water Resour. Res. 58. doi:10.1029/2021WR031432
Conant, R. T., Kluck, D., Anderson, M., Badger, A., Boustead, B. M., Derner, J., et al. (2018). in Ch 22: northern great plains. Impacts, risks, and adaptation in the United States: fourth national climate assessment, volume II. Editors D. R. Reidmiller, C. W. Avery, D. R. Easterling, K. E. Kunkel, K. L. M. Lewis, T. K. Maycocket al. (Washington, DC, USA: U.S. Global Change Research Program), 941–986. doi:10.7930/NCA4.2018.CH22
Cooley, A. K., and Chang, H. (2021). Detecting change in precipitation indices using observed (1977-2016) and modeled future climate data in portland, Oregon, USA. J. Water Clim. Change 12, 1135–1153. doi:10.2166/wcc.2020.043
Cui, W., Dong, X., Xi, B., and Kennedy, A. (2017). Evaluation of reanalyzed precipitation variability and trends using the gridded gauge-based analysis over the CONUS. J. Hydrometeorol. 18, 2227–2248. doi:10.1175/JHM-D-17-0029.1
Dai, A., Giorg, F., and Trenbert, K. E. (1999). Observed and Model-Simulated Diurnal Cycles of Precipitation over the Contiguous United States. Journal of Geophysical Research: Atmospheres 104, 6377–6402. doi:10.1029/98JD02720
Danabasoglu, G., Lamarque, J.-F., Bacmeister, J., Bailey, D. A., DuVivier, A. K., Edwards, J., et al. (2020). The community Earth system model version 2 (CESM2). J. Adv. Model. Earth Syst. 2, 1–35. doi:10.1029/2019MS001916
Deser, C., Adam, P., Vincent, B., and Teng, H. (2012). Uncertainty in climate change projections: the role of internal variability. Clim. Dyn. 38 (3–4), 527–546. doi:10.1007/s00382-010-0977-x
Dollan, I. J., Maggioni, V., and Johnston, J. (2022a). Investigating temporal and spatial precipitation patterns in the southern mid-atlantic United States. Front. Clim. 3. doi:10.3389/fclim.2021.799055
Dollan, I. J., Maggioni, V., Johnston, J., Coelho, G.De A., and Kinter, J. L. (2022b). Seasonal variability of future extreme precipitation and associated trends across the Contiguous U.S. Front. Clim. 4. doi:10.3389/fclim.2022.954892
Drijfhout, S., Hazeleger, W., Selten, F., and Haarsma, R. (2008). Future changes in internal variability of the atlantic meridional overturning circulation. Clim. Dyn. 30, 407–419. doi:10.1007/s00382-007-0297-y
Duan, Z., and Bastiaanssen, W. G. M. (2013). First results from version 7 TRMM 3B43 precipitation product in combination with a new downscaling – calibration procedure. Remote Sens. Environ. 131, 1–13. doi:10.1016/j.rse.2012.12.002
Duan, Z., Liu, J., Tuo, Y., Chiogna, G., and Disse, M. (2016). Evaluation of eight high spatial resolution gridded precipitation products in adige basin (Italy) at multiple temporal and spatial scales. Sci. Total Environ. 573, 1536–1553. doi:10.1016/j.scitotenv.2016.08.213
Dupigny-Giroux, L.-A. L., Mecray, E. L., Lemcke-stampone, M. D., Hodgkins, G. A., Lentz, E. E., Mills, K. E., et al. (2018). “Ch 18: Northeast,”. Impacts, risks, and adaptation in the United States: fourth national climate assessment. Editors D. R. Reidmiller, C. W. Avery, D. R. Easterling, K. E. Kunkel, K. L. M. Lewis, T. K. Maycocket al. (Washington, DC, USA: U.S. Global Change Research Program), 669–742. doi:10.7930/NCA4.2018.CH18
Easterling, D. R., Evans, J. L., Groisman, P.Ya., Karl, T. R., Kunkel, K. E., and Ambenje, P. (2000). Observed variability and trends in extreme climate events: a brief review. Bull. Am. Meteorological Soc. 0477 (March). doi:10.1175/1520-0477(2000)081<0417
Easterling, D. R., Kunkel, K. E., Arnold, J. R., Knutson, T., LeGrande, A. N., Leung, L. R., et al. (2017). Ch 7: precipitation change in the United States. Fourth Natl. Clim. Assess. I. doi:10.7930/J0H993CC
Ebert, E., Janowiak, J. E., and Kidd, C. (2007). Comparison of near-real-time precipitation estimates from satellite observations and numerical models. Am. Meteorological Soc. 88, 47–64. doi:10.1175/BAMS-88-1-47
Emmanouil, S., Langousis, A., Nikolopoulos, E. I., and Anagnostou, E. N. (2022). The spatiotemporal evolution of rainfall extremes in a changing climate: a CONUS-wide assessment based on multifractal scaling arguments. Earth’s Future 10, 1–16. doi:10.1029/2021EF002539
Eyring, V., Bony, S., Meehl, G. A., Senior, C., Stevens, B., Stou, R. J., et al. (2016). “Overview of the coupled model intercomparison project phase 6 (CMIP6) experimental design and organisation,” 10539–10583. doi:10.5194/gmdd-8-10539-2015
Fan, L., Dong, H., Xiao, C., Feng, Z., and Yan, J. (2024). Energy consumption, structural transformation and related carbon dioxide emissions of rural households on the Tibetan plateau. Energy 308 (August), 132789. doi:10.1016/j.energy.2024.132789
Fasullo, J. T. (2020). Evaluating simulated climate patterns from the CMIP archives using satellite and reanalysis datasets using the climate model assessment tool (CMATv1). Geosci. Model Dev. 13, 3627–3642. doi:10.5194/gmd-13-3627-2020
Feng, H., and Zhang, M. (2015). Global land moisture trends: drier in dry and wetter in wet over land. Sci. Rep. 5, 18018. doi:10.1038/srep18018
Fischer, E. M., and Knutti, R. (2016). Observed heavy precipitation increase confirms theory and early models. Nature Climate Change 6, 986–991. doi:10.1038/nclimate3110
Flato, G., Marotzke, J., Abiodun, B., Braconnot, P., Chou, S., Collins, W., et al. (2013). “Evaluation of climate models,” in Climate change 2013: the physical science basis, contribution of working Group I to the fifth assessment report of the intergovernmental panel on climate change. Cambridge, United Kingdom and New York, USA: Cambridge University Press.
Forestieri, A., Arnone, E., Blenkinsop, S., Candela, A., Fowler, H., and Noto, L. V. (2018). The impact of climate change on extreme precipitation in sicily, Italy. Hydrol. Process. 32, 332–348. doi:10.1002/hyp.11421
Frankcombe, L. M., England, M. H., Kajtar, J. B., Mann, M. E., and Steinman, B. A. (2018). On the choice of ensemble mean for estimating the forced signal in the presence of internal variability. J. Clim. 31 (14), 5681–5693. doi:10.1175/JCLI-D-17-0662.1
Fu, Y., Lin, Z., and Guo, D. (2020). Improvement of the simulation of the summer east asian westerly jet from CMIP5 to CMIP6. Atmos. Ocean. Sci. Lett. 00 (00), 550–558. doi:10.1080/16742834.2020.1746175
Gajbhiye, S., Meshram, C., Singh, S. K., Srivastava, P. K., and Islam, T. (2015). Precipitation trend analysis of sindh river basin, India, from 102-year record. Atmos. Sci. Lett. 17, 71–77. doi:10.1002/asl.602
Garfin, G., Franco, G., Blanco, H., Comrie, A., Gonzalez, P., Piechota, T., et al. (2014). “Chapter 20: Southwest”,. In Climate Change Impacts in the United States: The Third National Climate Assessment. Editors J. M. Melillo, T. T. C. Richmond, and G. W. Yohe (U.S. Global Change Research Program), 462–486. doi:10.7930/J08G8HMN
Gensini, V. A., and Harold, E. B. (2018). Spatial trends in United States tornado frequency. Npj Clim. Atmos. Sci. 1 (1), 38. doi:10.1038/s41612-018-0048-2
Gidden, M. J., Riahi, K., Smith, S. J., Fujimori, S., Luderer, G., Kriegler, E., et al. (2019). Global emissions Pathways under different socioeconomic scenarios for use in CMIP6: a dataset of harmonized emissions trajectories through the end of the century. Geosci. Model Dev. 12, 1443–1475. doi:10.5194/gmd-12-1443-2019
Gonzalez, P., Garfin, G. M., Breshears, D. D., Brooks, K. M., Brown, H. E., Elias, E. H., et al. (2018). “Ch 25: southwest,” in Impacts, risks, and adaptation in the United States: fourth national climate assessment, volume II. Editors D. R. Reidmiller, C. W. Avery, D. R. Easterling, K. E. Kunkel, K. L. M. Lewis, T. K. Maycocket al. (Washington, DC, USA: U.S. Global Change Research Program), 1101–1184. doi:10.7930/NCA4.2018.CH25
Gusain, A., Ghosh, S., and Karmakar, S. (2020). Added value of CMIP6 over CMIP5 models in simulating Indian summer monsoon rainfall. Atmos. Res. 232 (June 2019), 104680. doi:10.1016/j.atmosres.2019.104680
Gutowski, W. J., Decker, S. G., Donavon, R. A., Pan, Z., Arritt, R. W., and Takle, E. S. (2003). Temporal – spatial scales of observed and simulated precipitation in central U . S. Climate. Am. Meteorological Soc. 16, 3841–3847. doi:10.1175/1520-0442(2003)016<3841:TSOOAS>2.0.CO;2
Hallegatte, S., Green, C., Nicholls, R. J., and Corfee-morlot, J. (2013). Future flood losses in major coastal cities. Nat. Clim. Change 3 (9), 802–806. doi:10.1038/nclimate1979
He, J., Feng, P., Wang, B., Zhuang, W., Zhang, Y., Liu, D. L., et al. (2022). Centennial annual rainfall pattern changes show an increasing trend with higher variation over northern Australia. Am. Meteorological Soc. 23, 1333–1349. doi:10.1175/JHM-D-21-0116.1
Held, I. M., and Soden, B. J. (2006). Robust responses of the hydrological cycle to global warming. Am. Meteorological Soc. 19, 5686–5699. doi:10.1175/JCLI3990.1
Hempel, S., Frieler, K., Warszawski, L., Schewe, J., and Piontek, F. (2013). A trend-preserving bias correction – the ISI-mip approach. Earth Syst. Dyn. 4 (2), 219–236. doi:10.5194/esd-4-219-2013
Higgins, R. W., Shi, W., Yarosh, E., and Joyce, R. (2000). Improved United States precipitation quality control system and analysis. NCEP/Climate Predict. Cent. Atlas. No. 7. U.S. Department of Commerce, National Oceanic and Atmospheric Administration, National Weather Service.
Horton, R., Solecki, W., and Rosenzweig, C. (2012). “Climate change in the Northeast: a sourcebook. Draft technical input report prepared for the U.S. National climate assessment.”
Horton, R., Yohe, G., Easterling, W., Kates, R., Ruth, M., Sussman, E., et al. (2014). “Chapter 16: Northeast,”In Climate Change Impacts in the United States: The Third National Climate Assessment. Editors J. M. Melillo, T. T. C. Richmond, and G. W. Yohe (U.S. Global Change Research Program), 371–395. doi:10.7930/J0SF2T3P
Hosseinzadehtalaei, P., Tabari, H., and Willems, P. (2020). Climate change impact on short-duration extreme precipitation and intensity – duration – frequency curves over europe. J. Hydrology 590 (June), 125249. doi:10.1016/j.jhydrol.2020.125249
Hou, D., Charles, M., Luo, Y., Toth, Z., Zhu, Y., Roman, K., et al. (2014). Climatology-calibrated precipitation analysis at fine scales: statistical adjustment of stage IV toward CPC gauge-based analysis. Am. Meteorological Soc. 15 (6), 2542–2557. doi:10.1175/JHM-D-11-0140.1
Huang, H., Patricola, C. M., Winter, J. M., Osterberg, E. C., and Mankin, J. S. (2021). Rise in Northeast US extreme precipitation caused by atlantic variability and climate change. Weather Clim. Extrem. 33, 100351. doi:10.1016/j.wace.2021.100351
Huang, Y., Elahi, E., You, J., Sheng, Y., Li, J., and Meng, A. (2024). Land use policy implications of demographic shifts: analyzing the impact of aging rural populations on agricultural carbon emissions in China. Land Use Policy 147, 107340. doi:10.1016/j.landusepol.2024.107340
IPCC (2007). in Climate change 2007: the physical science basis. Contribution of working Group I to the fourth assessment report of the intergovernmental panel on climate change. Editors S. Solomon, D. Qin, M. Manning, M. Marquis, K. Averyt, M. M. B. Tignoret al. (Cambridge: Cambridge University Press).
IPCC (2013). in Climate change 2013: the physical science basis. Working Group I contribution to the fifth assessment report of the intergovernmental panel on climate change. Editors T. F. Stocker, D. Quin, G. Plattner, M. M. B. Tignor, S. K. Allen, J. Boschunget al. (Cambridge: Cambridge University Press).
IPCC (2022). in Climate change 2022: impacts, adaptation and vulnerability working Group II contribution to the Sixth assessment report of the intergovernmental panel on climate change. Editors H.-O. Pörtner, D. C. Roberts, M. Tignor, E. S. Poloczanska, K. Mintenbeck, A. Alegríaet al. (Cambridge, UK and New York, NY, USA: Cambridge University Press. Cambridge University Press), 3056. doi:10.1017/9781009325844
Jiang, Z., Li, W., Xu, J., and Li, L. (2015). Extreme precipitation indices over China in CMIP5 models. Part I: model evaluation. Am. Meteorological Soc. 28, 8603–8619. doi:10.1175/JCLI-D-15-0099.1
Jiang, Z., Song, J., Li, L., Chen, W., Wang, Z., and Wang, J. (2012). Extreme climate events in China: IPCC-AR4 model evaluation and projection. Clim. Change 110 (July), 385–401. doi:10.1007/s10584-011-0090-0
Jones, T. R., and Randall, D. A. (2011). Quantifying the limits of convective parameterizations. J. Geophys. Res. 116 (August 2010), D08210–D08219. doi:10.1029/2010JD014913
Kanniah, K. D., Beringer, J., and Hutley, L. B. (2011). Environmental controls on the spatial variability of savanna productivity in the Northern Territory, Australia. Agric. For. Meteorology 151 (11), 1429–1439. doi:10.1016/j.agrformet.2011.06.009
Karmalkar, A. V., and Bradley, R. S. (2017). Consequences of global warming of 1. 5 ˚ C and 2 ˚ C for regional temperature and precipitation changes in the contiguous United States. PLoS ONE 12, 01686977–e168717. doi:10.1371/journal.pone.0168697
Kawai, H., Yukimoto, S., Koshiro, T., Oshima, N., Tanaka, T., Yoshimura, H., et al. (2019). “Significant improvement of cloud representation in the global climate model MRI-ESM2.” Geosci. Model Dev. 2: 2875–2897. doi:10.5194/gmd-12-2875-2019
Kay, J. E., Deser, C., Phillips, A., Mai, A., Hannay, C., Strand, G., et al. (2015). The community Earth system model (cesm) large ensemble project: a community resource for studying climate change in the presence of internal climate variability. Bull. Am. Meteorological Soc. 96 (8), 1333–1349. doi:10.1175/BAMS-D-13-00255.1
Kendon, E. J., Ban, N., Roberts, N. M., Fowler, H. J., Roberts, M. J., Chan, S. C., et al. (2017). Do convection-permitting regional climate models improve projections of future precipitation change? Am. Meteorological Soc. 98, 79–93. doi:10.1175/BAMS-D-15-0004.1
Kharin, V. V., Zwiers, F. W., Zhang, X., and Wehner, M. (2013). Changes in temperature and precipitation extremes in the CMIP5 ensemble. Springer, 345–357. doi:10.1007/s10584-013-0705-8
Kidd, C., and Huffman, G. (2011). Review global precipitation measurement. Meteorological 353, 334–353. doi:10.1002/met.284
Kloesel, K., Bartush, B., Banner, J., Brown, D., Lemery, J., Lin, X., et al. (2018). in Ch 23: southern great plains. Impacts, risks, and adaptation in the United States: fourth national climate assessment, volume II. Editors D. R. Reidmiller, C. W. Avery, D. R. Easterling, K. E. Kunkel, K. L. M. Lewis, T. K. Maycocket al. (Washington, DC, USA: U.S. Global Change Research Program), 987–1035. doi:10.7930/NCA4.2018.CH23
Knutti, R., and Jan, S. (2012). Robustness and uncertainties in the new CMIP5 climate model projections. Nat. Clim. Change, 1–6. doi:10.1038/nclimate1716
Kunkel, K. E. (2003). North American trends in extreme precipitation. Nat. Hazards 29, 291–305. doi:10.1023/a:1023694115864
Kunkel, K. E., Karl, T. R., Brooks, H., Kossin, J., Lawrimore, J. H., Arndt, D., et al. (2013). Monitoring and understanding trends in extreme storms: state of knowledge. Bull. Am. Meteorological Soc. 94 (4), 499–514. doi:10.1175/BAMS-D-11-00262.1
Lalande, M., Ménégoz, M., Krinner, G., Naegeli, K., and Wunderle, S. (2021). Climate change in the high mountain asia in CMIP6. Earth Syst. Dyn. 12, 1061–1098. doi:10.5194/esd-12-1061-2021
Latif, M. (2010). Uncertainty in climate change projections. J. Geochem. Explor. 110, 1–7. doi:10.1016/j.gexplo.2010.09.011
Li, C., Zwiers, F., Zhang, X., Chen, G., Lu, J., Li, G., et al. (2019). Larger increases in more extreme local precipitation events as climate warms. Geophys. Res. Lett. 46, 6885–6891. doi:10.1029/2019GL082908
Li, M., Sun, Q., Lovino, M. A., Ali, S., Islam, M., Li, T., et al. (2022). Non-uniform changes in different daily precipitation events in the contiguous United States. Weather Clim. Extrem. 35, 100417. doi:10.1016/j.wace.2022.100417
Liang, X.-Z., Xu, M., Kunkel, K. E., Grell, G. A., and Kain, J. S. (2007). Regional climate model simulation of U . S.– Mexico summer precipitation using the optimal ensemble of two cumulus parameterizations. Am. Meteorological Soc. 20, 5201–5207. doi:10.1175/JCLI4306.1
Lin, Y., Dong, W., Zhang, M., Xie, Y., Xue, W., Huang, J., et al. (2017). Causes of model dry and warm bias over central U.S. And impact on climate projections. Nat. Commun. 8, 881–888. doi:10.1038/s41467-017-01040-2
Mallakpour, I., Sadegh, M., and Aghakouchak, A. (2020). Changes in the exposure of California’s levee- protected critical infrastructure to flooding hazard in a warming climate. Environ. Res. Lett. 15, 064032. doi:10.1088/1748-9326/ab80ed
Mallakpour, I., Sadeghi, M., Mosaffa, H., Akbari, A., Sadegh, M., Nguyen, P., et al. (2022). Discrepancies in changes in precipitation characteristics over the contiguous United States based on six daily gridded precipitation datasets. Weather Clim. Extrem. 36, 100433. doi:10.1016/j.wace.2022.100433
Mann, H. B. (1945). Nonparametric tests against trend. Econometrica 13 (3), 245–259. doi:10.2307/1907187
Maraun, D., Wetterhall, F., Ireson, A. M., Chandler, R. E., Kendon, E. J., Widmann, M., et al. (2010). Precipitation downscaling under climate change: recent developments to bridge the gap between dynamical models and the end user. Rev. Geophys. 2009, RG3003–34. doi:10.1029/2009RG000314
Martin-vide, J. (2004). Spatial distribution of a daily precipitation concentration index in peninsular Spain. Int. J. Climatol. 971 (May), 959–971. doi:10.1002/joc.1030
Mathbout, S., Lopez-bustins, J. A., Royé, D., and Martin-vide, J. (2021). Mediterranean-scale drought: regional datasets for exceptional meteorological drought events during 1975-2019. Atmosphere 12, 941. doi:10.3390/atmos12080941
May, C., Luce, C., Casola, J., Chang, M., Cuhaciyan, J., Dalton, M., et al. (2018). “Ch 24: northwest,”. Impacts, risks, and adaptation in the United States: fourth national climate assessment. Editors D. R. Reidmiller, C. W. Avery, D. R. Easterling, K. E. Kunkel, K. L. M. Lewis, and T. K. Maycock, (Washington, DC, USA: U.S. Global Change Research Program), II, 1036–1100. doi:10.7930/NCA4.2018.CH24
Meehl, G. A., Boer, G. J., Covey, C., Latif, M., and Ronald, S. (2000). The coupled model intercomparison project (CMIP). Bull. Am. Meteorological Soc. 0477 (January). doi:10.1175/1520-0477(2000)081<0313
Meehl, G. A., Boer, G. J., Covey, C., Latif, M., and Stouffer, R. J. (1997). Intercomparison makes for a better climate model. Eos Trans. Am. Geophys. Union 78 (41), 445–451. doi:10.1029/97EO00276
Meehl, G. A., Covey, C., Delworth, T., Latif, M., Mcavaney, B., Mitchell, J. F. B., et al. (2007). The WCRP CMIP3 multimodel dataset - a new era in climate change research. Bull. Am. Meteorological Soc. 88 (September), 1383–1394. doi:10.1175/BAMS-88-9-1383
Meehl, G. A., Tebaldi, C., Walton, G., Easterling, D., and Mcdaniel, L. (2009). Relative increase of record high maximum temperatures compared to record low minimum temperatures in the U . S. Geophys. Res. Lett. 36 (December), 1–5. doi:10.1029/2009GL040736
Melillo, J., Richmond, T. C., and Yohe, G. (2014). “Climate change impacts in the United States: the third national climate assessment.” doi:10.7930/J0Z31WJ2
Merrifield, A. L., and Xie, S.-P. (2016). Summer U . S. Surface air temperature variability: controlling factors and AMIP simulation biases. J. Clim. 29, 5123–5139. doi:10.1175/JCLI-D-15-0705.1
Milinski, S., Maher, N., and Olonscheck, D. (2020). How large does a large ensemble need to Be. Earth Syst. Dyn. 11, 885–901. doi:10.5194/esd-11-885-2020
Moise, A., Wilson, L., Grose, M., Whetton, P., Watterson, I., Bhend, J., et al. (2015). Evaluation of CMIP3 and CMIP5 models over the Australian region to inform confidence in projections. Aust. Meteorological Oceanogr. J. 5 (October), 19–53. doi:10.22499/2.6501.004
Monier, E., and Gao, X. (2015). Climate change impacts on extreme events in the United States: an uncertainty analysis. Clim. Change 131, 67–81. doi:10.1007/s10584-013-1048-1
Moss, R. H., Edmonds, J. A., Hibbard, K. A., Manning, M. R., Rose, S. K., Vuuren, D. P. V., et al. (2010). The next generation of scenarios for climate change research and assessment. Nature 463 (7282), 747–756. doi:10.1038/nature08823
Mote, P. W., Snover, A. K., Capalbo, S., Eigenbrode, S. D., Glick, P., Littell, J., et al. (2014). “Chapter 21: Northwest,”. In Climate Change Impacts in the United States: The Third National Climate Assessment. Editors J. M. Melillo, T. T. C. Richmond, and G. W. Yohe (U.S. Global Change Research Program), 487–513. doi:10.7930/J04Q7RWX
Moustakis, Y., Papalexiou, S. M., Onof, C. J., and Paschalis, A. (2021). Seasonality, intensity, and duration of rainfall extremes change in a warmer climate. Earth’s Future 9, 1–15. doi:10.1029/2020EF001824
Mueller, B., and Seneviratne, S. I. (2014). Systematic land climate and evapotranspiration biases in CMIP5 simulations. Geophys. Res. Lett. 41 (December 2013), 128–134. doi:10.1002/2013GL058055
Navarro-Racines, C. E., Tarapues-Montenegro, J. E., and Ramírez-Villegas, J. A. (2015). “BIAS-CORRECTION IN THE CCAFS-CLIMATE PORTAL: A DESCRIPTION OF MEHOTODOLOGIES,” in Decision and policy analysis (DAPA) research area. International center for tropical agriculture (CIAT). Colombia: Cali.
Neri, A., Villarini, G., Slater, L. J., and Napolitano, F. (2019). On the statistical attribution of the frequency of flood events across the U.S. Midwest. Adv. Water Resour. 127 (March), 225–236. doi:10.1016/j.advwatres.2019.03.019
Nguyen, P., Thorstensen, A., Sorooshian, S., Hsu, K., Aghakouchak, A., Ashouri, H., et al. (2018). Global precipitation trends across spatial scales using satellite observations. Am. Meteorological Soc. 99 (April), 689–697. doi:10.1175/BAMS-D-17-0065.1
Oki, T., and Kanae, S. (2006). Global hydrological cycles and world water resources. Science 313 (August), 1068–1072. doi:10.1126/science.1128845
O’ Neill, B. C., Tebaldi, C., Van Vuuren, D., Eyring, V., Fridelingstein, P., George, H., et al. (2016). The scenario model intercomparison project (ScenarioMIP) for CMIP6. Geosci. Model Dev. (April), 1–35. doi:10.5194/gmd-2016-84
Oruc, S. (2022). Performance of bias corrected monthly CMIP6 climate projections with different reference period data in Turkey. Hydrology 70, 777–789. doi:10.1007/s11600-022-00731-9
Pendergrass, A. G., Knutti, R., Lehner, F., Deser, C., and Sanderson, B. M. (2017). Precipitation variability increases in a warmer climate. Sci. Rep. 7, 17966–18010. doi:10.1038/s41598-017-17966-y
Praveen, B., Talukdar, S., Mahato, S., and Mondal, J. (2020). “Analyzing trend and forecasting of rainfall changes in India using non- parametrical and machine learning approaches,” 1–21. doi:10.1038/s41598-020-67228-7
Prein, Andreas F., and Holland, G. J. (2018). Global estimates of damaging hail hazard. Weather Clim. Extrem. 22 10–23. doi:10.1016/j.wace.2018.10.004
Prein, Andreas F., Rasmussen, R. M., Ikeda, K., Liu, C., Clark, M. P., and Holland, G. J. (2017). The future intensification of hourly precipitation extremes. Nature 7, 48–52. doi:10.1038/NCLIMATE3168
Pryor, S. C., Scavia, D., Downer, C., Gaden, M., Iverson, L., Nordstrom, R., et al. (2014). “Chapter 18: Midwest”. In Climate Change Impacts in the United States: The Third National Climate Assessment. Editors J. M. Melillo, T. T. C. Richmond, and G. W. Yohe (U.S. Global Change Research Program), 418–440. doi:10.7930/J0J1012N
Raghavan, S. V., Liu, J., Nguyen, N. S., Vu, M. T., and Liong, S. (2018). Assessment of CMIP5 historical simulations of rainfall over Southeast asia. Springer, 989–1002. doi:10.1007/s00704-017-2111-z
Rahat, S. H., Steinschneider, S., Kucharski, J., Arnold, W., Olzewski, J., Walker, W., et al. (2022). Characterizing hydrologic vulnerability under nonstationary climate and antecedent conditions using a process-informed stochastic weather generator. J. Water Resour. Plan. Manag. 148 (April). doi:10.1061/(ASCE)WR.1943-5452.0001557
Raisanen, J. (2002). CO 2 -induced changes in interannual temperature and precipitation variability in 19 CMIP2 experiments. J. Clim. 15, 2395–2411. doi:10.1175/1520-0442(2002)015<2395:ciciit>2.0.co;2
Ramirez-villegas, J., Challinor, A. J., Thornton, P. K., and Jarvis, A. (2013). Implications of regional improvement in global climate models for agricultural impact research. Environ. Res. Lett. 8, 024018. doi:10.1088/1748-9326/8/2/024018
Randall, A. A., Bitz, C. M., Danabasoglu, G., Scott Denning, A., Gent, P. R., Gettelman, A., et al. (2019). 100 Years of Earth system model development. Meteorol. Monogr. 59, 12.1–12.66. doi:10.1175/AMSMONOGRAPHS-D-18-0018.1
Reidmiller, D. R., Avery, C. W., Easterling, D. R., Kunkel, K. E., Lewis, K. L. M., Maycock, T. K., et al. (2018). “Impacts, risks, and adaptation in the United States: fourth national climate assessment,”, II. Washington, DC, USA: U.S. Global Change Research Program, 186. doi:10.7930/NCA4.2018.RiB
Riahi, K., Van Vuuren, D. P., Kriegler, E., Edmonds, J., Neill, B. C. O., Fujimori, S., et al. (2017). The shared socioeconomic Pathways and their energy, land use, and greenhouse gas emissions implications: an overview. Glob. Environ. Change 42, 153–168. doi:10.1016/j.gloenvcha.2016.05.009
Rodgers, K. B., Lee, S., Rosenbloom, N., Timmermann, A., Danabasoglu, G., Deser, C., et al. (2021). Ubiquity of human-induced changes in climate variability. Earth Syst. Dyn. doi:10.5194/esd-2021-50
Roy´e, D., and Martin-Vide, J. (2017). Concentration of daily precipitation in the contiguous United States. Atmos. Res. doi:10.1016/j.atmosres.2017.06.011
Rupp, D. E., Abatzoglou, J. T., Hegewisch, K. C., and Mote, P. W. (2015). Evaluation of CMIP5 20 Th century climate simulations for the pacific northwest USA. J. Geophys. Res. Atmos. 118 (April 2013), 884–907. doi:10.1002/jgrd.50843
Saki, S. A., Sofia, G., and Anagnostou, E. N. (2023). Characterizing CONUS-wide spatio-temporal changes in daily precipitation, flow, and variability of extremes. J. Hydrology 626 (PB), 130336. doi:10.1016/j.jhydrol.2023.130336
Seager, R., Hoerling, M., Schubert, S., Wang, H., Lyon, B., Kumar, A., et al. (2015). Causes of the 2011-14 California drought. J. Clim. 28 (18), 6997–7024. doi:10.1175/JCLI-D-14-00860.1
Shafer, M., Ojima, D., Antle, J. M., Kluck, D., McPherson, R. A., Petersen, S., et al. (2014). “Chapter 19: Great Plains,”. In Climate Change Impacts in the United States: The Third National Climate Assessment. Editors J. M. Melillo, T. T. C. Terese, and G. W. Yohe (U.S. Global Change Research Program), 441–461. doi:10.7930/J0D798BC
Sharif, R. B., Habib, E. H., and ElSaadani, M. (2020). Evaluation of radar-rainfall products over coastal Louisiana. Remote Sens. 12, 1477. doi:10.3390/rs12091477
Sharif, R. B., Houser, P., Aquila, V., and Maggioni, V. (2022). Investigating rainfall patterns in the hubei province, China and northern Italy during the covid-19 lockdowns. Front. Clim. 3 (January), 1–10. doi:10.3389/fclim.2021.799054
Silva, R. M. da, Santos, C. A. G., Moreira, M., Corte-Real, J. ˜o, Silva, V. C. L., and Medeiros, I. C. (2015). Rainfall and river flow trends using Mann – Kendall and sen ’ s slope estimator statistical tests in the cobres river basin. Nat. Hazards 77, 1205–1221. doi:10.1007/s11069-015-1644-7
Simpson, I. R., Bacmeister, J., Neale, R. B., Hannay, C., Gettelman, A., Garcia, R. R., et al. (2020). An evaluation of the large-scale atmospheric circulation and its variability in CESM2 and other CMIP models. J. Geophys. Res. Atmos. 125. doi:10.1029/2020JD032835
Slater, L., Villarini, G., Archfield, S., Faulkner, D., Lamb, R., Khouakhi, A., et al. (2021). Global changes in 20-year 50-year and 100-year river floods. Geophys. Res. Lett. 48. doi:10.1029/2020GL091824
Srivastava, A., Grotjahn, R., and Ullrich, P. A. (2020). Evaluation of historical CMIP6 model simulations of extreme precipitation over contiguous US regions. Weather Clim. Extrem. 29, 100268. doi:10.1016/j.wace.2020.100268
Strzepek, K., Yohe, G., Neumann, J., and Boehlert, B. (2010). Characterizing changes in drought risk for the United States from climate change. Environ. Res. Lett. 5, 044012. doi:10.1088/1748-9326/5/4/044012
Su, F., Duan, X., Chen, D., Zhenchun, H., and Cuo, L. (2013). Evaluation of the global climate models in the CMIP5 over the Tibetan plateau. J. Clim. 26, 3187–3208. doi:10.1175/JCLI-D-12-00321.1
Supharatid, S., Aribarg, T., and Nafung, J. (2022). Bias - corrected CMIP6 climate model projection over Southeast asia. Theor. Appl. Climatol. 147, 669–690. doi:10.1007/s00704-021-03844-1
Swain, D. L., Baird, L., Neelin, J. D., and Hall, A. (2018). Increasing precipitation volatility in twenty-first-century California. Nat. Clim. Change 8 (5), 427–433. doi:10.1038/s41558-018-0140-y
Taylor, K. E., Stouffer, R. J., and Meehl, G. A. (2012). An overview of CMIP5 and the experiment design. Bull. Am. Meteorological Soc. 93, 485–498. doi:10.1175/BAMS-D-11-00094.1
Tebaldi, C., Hayhoe, K., Arblaster, J. M., and Meehl, G. A. (2006). Going to the extremes. Springer, 185–211. doi:10.1007/s10584-006-9051-4
Teutschbein, C., and Jan, S. (2012). Bias correction of regional climate model simulations for hydrological climate-change impact studies: review and evaluation of different methods. J. Hydrology 456–457, 12–29. doi:10.1016/j.jhydrol.2012.05.052
Thackeray, C. W., DeAngelis, A. M., Hall, A., Swain, D. L., and Qu, X. (2018). On the connection between global hydrologic sensitivity and regional wet extremes. Geophys. Res. Lett. 45, 343–351. doi:10.1029/2018GL079698
Trenberth, K. E. (2011). Changes in precipitation with climate change. Cliimate Res. 47, 123–138. doi:10.3354/cr00953
Trenberth, K. E., Dai, A., Rasmussen, R. M., and Parsons, D. B. (2003). The changing character of precipitation. Am. Meteorological Soc. 84, 1205–1218. doi:10.1175/BAMS-84-9-1205
Ukkola, A. M., De Kauwe, M. G., Roderick, M. L., Abramowitz, G., and Pitman, A. J. (2020). Robust future changes in meteorological drought in CMIP6 projections despite uncertainty in precipitation. Geophys. Res. Lett. 47, 1–9. doi:10.1029/2020GL087820
Vuuren, D. P. V., Kriegler, E., O’ Neill, B. C., Ebi, K. L., Riahi, K., Carter, T. R., et al. (2014). A new scenario framework for climate change research: scenario matrix architecture. Clim. Change 122, 373–386. doi:10.1007/s10584-013-0906-1
Wang, C., Zhang, L., Lee, S.-ki, Wu, L., and Mechoso, C. R. (2014). A global perspective on CMIP5 climate model biases. Nat. Clim. Change 4, 201–205. doi:10.1038/nclimate2118
Wasko, C., Sharma, A., and Johnson, F. (2015). Does storm duration modulate the extreme precipitation-temperature scaling relationship? Geophys. Res. Lett. 42, 8783–8790. doi:10.1002/2015GL066274
Wetherald, R. T. (2009). Changes of variability in response to increasing greenhouse gases. Part II: hydrology. J. Clim. 2002, 6089–6103. doi:10.1175/2009JCLI2834.1
Wijeratne, V. P. I. S., Li, G., Mehmood, M. S., and Abbas, A. (2023). Assessing the impact of long-term ENSO, SST, and IOD dynamics on extreme hydrological events (EHEs) in the kelani river basin (KRB), Sri Lanka. Atmosphere 14 (1), 79. doi:10.3390/atmos14010079
Willner, S. N., Otto, C., and Levermann, A. (2018). Global economic response to river floods. Nat. Clim. Change 8 (July), 594–598. doi:10.1038/s41558-018-0173-2
Wu, S.-ye (2015). Changing characteristics of precipitation for the contiguous United States. Clim. Change 132, 677–692. doi:10.1007/s10584-015-1453-8
Xie, P., Chen, M., Yang, S., Yatagai, A., Hayasaka, T., Fukushima, Y., et al. (2007). A gauge-based analysis of daily precipitation over east asia. Am. Meteorological Soc. 8, 607–626. doi:10.1175/JHM583.1
Xu, Z., Han, Y., Tam, C.-Y., Yang, Z.-L., and Fu, C. (2021). Bias-corrected CMIP6 global dataset for dynamical downscaling of the historical and future climate (1979 – 2100). Nature 8, 293–311. doi:10.1038/s41597-021-01079-3
Yang, T., Li, Q., Chen, X., Maeyer, P. D., Yan, X., Liu, Y., et al. (2020). Spatiotemporal variability of the precipitation concentration and diversity in central asia. Atmos. Res. 241, 104954. doi:10.1016/j.atmosres.2020.104954
Zamani, Y., Monfared, S. A. H., Moghaddam, M. A., and Hamidianpour, M. (2020). A comparison of CMIP6 and CMIP5 projections for precipitation to observational data: the case of northeastern Iran. Theor. Appl. Climatol. 142, 1613–1623. doi:10.1007/s00704-020-03406-x
Zelle, H., Jan Van Oldenborgh, G., Burgers, G., and Dijkstra, H. (2005). El niño and greenhouse warming: results from ensemble simulations with the NCAR CCSM. J. Clim. 18, 4669–4683. doi:10.1175/jcli3574.1
Zhai, P., Zhang, X., Wan, H., and Pan, X. (2005). Trends in total precipitation and frequency of daily precipitation extremes over China. J. Clim. 18, 1096–1108. doi:10.1175/JCLI-3318.1
Keywords: precipitation patterns, trends, climate model projections, CESM2, CPC observations
Citation: Sharif RB, Maggioni V and Dollan IJ (2025) Changes in historical and future precipitation patterns across the contiguous United States. Front. Earth Sci. 13:1542536. doi: 10.3389/feart.2025.1542536
Received: 10 December 2024; Accepted: 04 April 2025;
Published: 16 April 2025.
Edited by:
Marco Mancini, Polytechnic of Milan, ItalyReviewed by:
Rosmeri Porfirio da Rocha, University of São Paulo, BrazilAdnan Abbas, Nanjing University of Information Science and Technology, China
Copyright © 2025 Sharif, Maggioni and Dollan. This is an open-access article distributed under the terms of the Creative Commons Attribution License (CC BY). The use, distribution or reproduction in other forums is permitted, provided the original author(s) and the copyright owner(s) are credited and that the original publication in this journal is cited, in accordance with accepted academic practice. No use, distribution or reproduction is permitted which does not comply with these terms.
*Correspondence: Ridwana Binte Sharif, cnNoYXJpZkBnbXUuZWR1