- 1College of Computer and Control Engineering, Northeast Forestry University, Harbin, China
- 2Department of Information Technology, Faculty of Computing and Information Technology, King Abdulaziz University, Jeddah, Saudi Arabia
Introduction: Remote sensing super-resolution (RS-SR) plays a crucial role in the analysis of remote sensing images, aiming to improve the spatial resolution of images with lower resolutions. Recent advancements in RS-SR research have been largely driven by the integration of deep learning techniques, especially through the application of Generative Adversarial Networks (GANs), which have shown significant effectiveness in advancing this field. While GAN has achieved notable advancements in this field, its tendency toward pattern collapse often introduces artifacts and distorts textures in the reconstructed images.
Methods: This study introduces a novel RS-SR model, termed the Diffusion Enhanced Generative Adversarial Network (DEGAN), designed to improve the quality of RS-SR images through the incorporation of a diffusion model. At the heart of DEGAN lies an innovative GAN architecture that fuses the adversarial mechanisms of both the generator and discriminator with an integrated diffusion module. This additional component utilizes the noise reduction capabilities of the diffusion process to refine the intermediate stages of image generation, ultimately improving the clarity of the final output and enhancing the performance of remote sensing super-resolution.
Results: In the test dataset, the peak signal-to-noise ratio (PSNR) increased by 0.345 dB at 2× scaling and 0.671 dB at 4× scaling, while the structural similarity index (SSIM) was improved by 0.0087 and 0.0166, respectively, compared to the current state-of-the-art (SOTA) approach.
Discussion: These results indicate that DEGAN significantly improves the super-resolution reconstruction performance of remote sensing images. The introduction of the diffusion module and attention mechanism effectively reduces noise and enhances image clarity, addressing common issues of texture distortion and artifacts in remote sensing image super-resolution reconstruction.
1 Introduction
Recently, remote sensing imagery has found increasing use in applications including environmental monitoring, object detection, and scene categorization. However, inherent physical and technological constraints during the data acquisition process often result in image resolutions that fall short of the stringent accuracy requirements. Consequently, various RS-SR techniques have been proposed to generate high-resolution images from low-resolution inputs, providing an effective approach to improve spatial resolution without the need for new sensor hardware or optimizing data transmission.
At present, image super-resolution techniques are commonly divided into three main categories: interpolation-based methods, traditional machine learning approaches, and deep learning strategies. Interpolation-based SR methods enlarge an image using a straightforward mathematical formula, offering computational efficiency. However, they struggle to recover fine details and texture, often resulting in lower-quality images. Traditional machine learning-based SR techniques typically adopt strategies such as dictionary learning, sparse representation, and neighborhood embedding. By capturing the relationship between low- and high-resolution data, these techniques generate high-resolution images. Although they can restore fine details to some extent, their performance is often constrained by the quantity and quality of the training data, which leads to reduced generalizability and lower adaptability in complex real-world applications. Deep learning-based SR techniques have gained widespread adoption in recent years, and by constructing deep models such as convolutional neural networks (CNN), generative adversarial networks (GAN), Transformers, etc., more complex and abstract image features can be learned from massive data, thus realizing a more ideal super-resolution effect.
In recent years, super-resolution techniques based on deep learning have been widely used. By constructing deep models such as Convolutional Neural Networks (CNN), Generative Adversarial Networks (GAN), Transformer, etc., more complex and abstract image features can be learned from massive data, thus realizing more desirable super-resolution effects. In the field of computer vision, end-to-end trained super-resolution techniques excel in detail recovery and perceptual quality. The first end-to-end CNN framework was introduced to facilitate the conversion from low-resolution (LR) to high-resolution (HR) images using lightweight networks (Dong et al., 2014); Lim et al.'s EDSR achieved then-optimal performance by streamlining residuals and scaling up the model (Lim et al., 2017), while MDSR achieves single-model multiscale reconstruction. Dong et al.’s SMSR achieved lightweight multiscale modeling using feature reuse (Dong et al., 2020), and Wang et al. revolutionized contextual feature extraction (Wang et al., 2021). Liebel and Körner verified the advantages of CNN on Sentinel-2 multispectral data (Liebel and Körner, 2016); Zhang et al. introduced a scene-adaptive strategy to efficiently extract multilevel features (Zhang et al., 2020). The EDCNN was proposed for super-resolution reconstruction of remote sensing images (Keshk and Yin, 2021). Lei et al. designed LGCNet to capture the interaction of local and global features (Lei et al., 2017), and their follow-up work enhances the cross-scale structure with a mixed-scale self-similarity module (Lei and Shi, 2021). Haut et al. (2019) and Huan et al. (2021) enhance contextual understanding using deep cataloging models and multiscale pyramidal residual networks combined with null convolution, respectively; the multiscale attention networks proposed by Zhang et al. (2020) and FeNet with RDBPN (Wang et al., 2022a; Pan et al., 2019) further strike a balance between efficiency and large-scale reconstruction balance between them. However, convolutional neural networks often face the problems of overfitting and local feature emphasis during reconstruction, limiting the global context capturing ability and thus affecting the generalization effect in complex scenes.
GAN, an important technique in deep learning, has been successfully introduced to super-resolution tasks. By combining perceptual loss with adversarial training, the generated images become more realistic in subjective vision. The proposal of SRGAN combines adversarial training with perceptual loss for the first time, which not only significantly improves the detailed performance and overall visual effect of the generated images, but also provides a new way of thinking for super-resolution reconstruction (Yang et al., 2017). Hu et al. (2024) proposed a super-resolution reconstruction model combining a multi-scale attention mechanism and dense residual connectivity for improving detail recovery and overall reconstruction quality of remotely sensed images. Wang et al. (2025) improved the visual effect and evaluation index of remote sensing images by improving the SRGAN algorithm. The resolution of real images from the Worldview satellite and Sentinel-2 was improved by the improved ESRGAN algorithm (Salgueiro Romero et al., 2020). The reconstruction quality and resolution of Sentinel-2 images were significantly improved (Jain and Vatsavai, 2024). However, although GAN-based super-resolution reconstruction methods perform well in many scenes, there are still some problems. In some cases, the generator is prone to pattern collapse, i.e., the generated images exhibit too homogeneous patterns and lack the necessary diversity. This phenomenon leads to limitations in detail recovery in the reconstructed super-resolution images, which cannot adequately restore the subtle features in the original images. In addition, since the model may be affected by data distribution bias during the training process, the generated high-resolution images may suffer from missing details or uneven reconstruction in some regions.
Recently, super-resolution models that leverage visual Transformers have been developed to boost the model’s performance in complex scenes by incorporating a global self-attention mechanism. Swin Transformer overcomes the computational bottleneck of the traditional Transformer by utilizing local window attention (Liu et al., 2021), and DAT realizes alternate channel-spatial attention through alternate cross-layer feature aggregation (Chen et al., 2023), providing a new paradigm for SR tasks. Based on this, SwinIR establishes a benchmark model for image recovery (Liang et al., 2021). In remote sensing, Lei et al. were the first to integrate Transformer into super-resolution, enabling multi-scale feature fusion through multilevel enhancement (Lei et al., 2021). SWCGAN combines the advantages of Swin Transformer and convolution (Tu et al., 2022) and strengthens deep layer feature extraction through residual dense blocks (Shang et al., 2023). Sharifi and Safari proposed a Transformer-based deep learning model for super-resolution reconstruction of Sentinel-2 images through multi-head attention and spatial-channel attention mechanisms (Sharifi and Safari, 2025). Rossi et al. proposed an enhanced super-resolution model that effectively improves the super-resolution performance of remote sensing single images (Rossi et al., 2025). Furthermore, a GAN model utilizing Transformer architecture was introduced, which effectively learns global context to recover intricate global features (Esser et al., 2021). Although Transformer is good at capturing long-distance pixel relationships, it may pay too much attention to global information and ignore local details, resulting in blurred or unnatural reconstruction results.
In the field of remote sensing, traditional super-resolution methods, such as interpolation and dictionary learning-based methods, can provide smoother images, but it is difficult to recover the high-frequency details of the image in complex backgrounds and is computationally efficient. In contrast, GAN, as one of the deep learning methods, can effectively improve the quality of image detail recovery through adversarial training.The advantage of the GAN model is that it can generate more realistic high-resolution images, but pattern collapse may occur during training, resulting in a lack of diversity in the generated images.
Currently, diffusion models have demonstrated significant advantages in image translation and hyper-segmentation tasks. Diffusion model is a generative model that restores an image from a noisy state to a high-quality state through a step-by-step denoising and reconstruction process. In remote sensing super-resolution tasks, the diffusion model helps to remove noise and artifacts from the image generation process, thus improving the detail and clarity of super-resolution images. DDPM, proposed by Ho et al., constructs a complete denoising diffusion framework (Ho et al., 2020), and its first application in SISR is realized by SR3 (Saharia et al., 2022). ControlNet achieves stabilization control through zero convolution (Zhang et al., 2023), and Stable Diffusion’sinnovations in the latent space offer a new way for high score generation provides new ideas (Rombach et al., 2022). Yang et al. and Li and Ren reviewed the application of diffusion modeling in image restoration and real scene repair (Yang et al., 2023; Li and Ren, 2023). Despite the advantages of DDPM in modeling complex distributions and mitigating GAN training instability, ab initio training is costly and may corrupt pre-training to generate a priori. To this end, Wang et al. employed a time-sensitive encoder to achieve effective restoration while maintaining the pre-trained model (Wang et al., 2024). The DiffBIR framework of Lin et al. divides the blind image restoration into degradation removal and information regeneration phases (Lin et al., 2024), while the PASD network proposed by Yang et al. achieves excellent performance in a variety of image enhancement tasks through pixel-aware cross-attention and degradation removal modules (Yang et al., 2024). By integrating the diffusion model into the GAN architecture, image reconstruction performance is significantly improved, even in challenging conditions, positioning it as a key area of focus in remote sensing image super-segmentation research.
Recently, DiffGAN (Wang et al., 2022b) and SRDiff (Li et al., 2022) combine the advantages of diffusion models and Generative Adversarial Networks (GANs), which perform well under complex image degradation.DiffGAN proposes to incorporate adversarial training into the traditional diffusion process, and SRDiff generates low-resolution (LR) images by stepwise back-diffusing the high resolution (HR) images. Although these methods have made breakthroughs in image reconstruction, they still face problems such as insufficient recovery of high-frequency details, excessive image smoothing, and noise effects. In this study, a new remote sensing super-resolution (RS-SR) model called diffusion-enhanced generative adversarial network (DEGAN) is proposed. Unlike the above two frameworks, this paper focuses on applying the diffusion module to the generator part of the GAN, which is introduced in the intermediate step of the process of generating images for processing the extracted features. The DEGAN framework effectively captures high-frequency detail in images by incorporating the diffusion model into the GAN generator, thereby improving the super-resolution performance of remote sensing images and enhancing efficiency in reconstruction tasks. Additionally, it maintains high image quality even in the presence of complex environmental factors, such as atmospheric perturbation, tele-imaging, and spectral noise. Through comparative experiments, the model proposed in this study outperforms current super-resolution methods across all evaluation metrics, particularly in handling remote sensing images with intricate textures and structures. This results in notable enhancements in both the visual appearance and numerical performance. In addition, this study investigates the model’s applicability across various remote sensing scenarios, aiming to offer a novel technical solution in the field of RS-SR. The main findings of this research are outlined as follows:
1. We present a new super-resolution network, DEGAN, specifically designed for remote sensing tasks, which incorporates the diffusion model into the GAN framework. By leveraging the noise addition and stage denoising capabilities of the diffusion model, DEGAN refines the intermediate outputs from the generator, further improving the generated outputs and enhancing the overall accuracy of the final reconstruction. Comprehensive experiments show that DEGAN exhibits significant advantages in recovering the detailed information of remote sensing images.
2. To improve the detail generation capability of DEGAN, we introduce Diffusion, a diffusion module based on the U-Net architecture, which extracts feature information through the encoder, obtains deeper feature representations, and gradually recovers the resolution and details of the image through the decoder. In addition, the incorporation of skip connections to directly transfer feature data between the encoder and the decoder significantly enhances the detail recovery, enabling the Diffusion model to extend its detail generation capabilities for DEGAN.
3. In the DEGAN model, we utilize a variety of techniques to further enhance the quality of image reconstruction. By incorporating an attention mechanism, the model can focus on crucial regions of the image, thereby enhancing the detail quality of the reconstructed image. Additionally, sub-pixel convolution efficiently maps the input image’s channel information to the spatial dimensions of the output, effectively avoiding the blurring issues associated with traditional transposed convolutions. By incorporating residual learning, the model’s image reconstruction performance is significantly improved, ensuring that the produced high-resolution images maintain enhanced quality and better detail retention.
4. Extensive and in-depth experiments are carried out using three publicly available remote sensing datasets: UC Merced, AID, and NEG-Scene. The experimental results demonstrate that, compared to current SOTA methods, the DEGAN model delivers significantly superior performance in SR tasks, further validating its cutting-edge design and effectiveness in enhancing remote sensing image quality.
This paper is organized as follows: Section 2 reviews the relevant literature on super-resolution; Section 3 provides an in-depth explanation of the DEGAN network architecture introduced in this work; Section 4 presents the experimental setup, which includes both objective and subjective evaluations, along with ablation studies; and Section 5 wraps up with a summary of the contributions.
2 Materials and methods
This section begins with the overall architecture presented. Then the components of our proposed model are described in sequence, starting with the generator, followed by the discriminator, and finally the loss function.
2.1 Network infrastructure
Numerous studies have shown that training frameworks relying only on traditional super-resolution (SR) models often lead to loss of image detail information as well as poor adaptation in complex scenes. Particularly in remote sensing image super-resolution tasks, current methods often face difficulties in effectively recovering high-frequency details, and their performance is further constrained when dealing with complex textures and fine edge features. To overcome these challenges, we propose a novel framework for enhancing remote sensing image super-resolution, termed the Diffusion Enhanced Generative Adversarial Network (DEGAN), which aims to improve reconstruction quality significantly.
As shown in Figure 1, DEGAN combines a traditional super-resolution training framework and a diffusion model network. Contrary to conventional GAN-based methods, DEGAN integrates a diffusion model to produce superior images while diligently restoring high-frequency details. This approach effectively overcomes the detail loss typically associated with pattern collapse in standard GAN frameworks. Specifically, the introduction of the diffusion model effectively improves the performance of images in complex scenes, improving its capacity to recover fine details, textures, and edges.
In the DEGAN generator, we incorporate a diffusion module based on the U-Net architecture, aimed at overcoming the limitations in detail recovery commonly observed in traditional GANs. Initially, the module builds a hierarchy of multiscale features through a sequence of convolutional downsampling steps. During this process, the encoder gradually extracts features, progressing from low-level to intermediate and then high-level representations, with the high-level features (reduced to one-quarter of the original resolution) processed at the bottleneck layer. To better leverage the key role of the diffusion step in the generation process, we treat the noise level as the diffusion step t and map it to a high-dimensional vector using a fully connected layer. This vector is then passed through a nonlinear activation function, aligned with the bottleneck features, and incorporated into the bottleneck features as residuals, enabling the network to dynamically adjust the denoising intensity based on varying noise levels. This design explicitly uses the number of diffusion steps t as a control variable, which helps to fine-grain the effect of recovering image details during the generation process. Unlike previous work that relied only on the convolutional residual block, we introduce a Transformer encoder at the bottleneck layer, which realizes the self-attentive modeling of global features by spreading the feature maps into a sequence format, effectively capturing the long-distance dependencies within the image, and further enhancing the detail recovery capability. Subsequently, the decoder employs inverse convolutional upsampling combined with jump connections from the corresponding levels of the encoder to fuse low-level features via 1 × 1 convolution to mitigate information loss and suppress excessive smoothing. The entire diffusion module enables the generated image to effectively suppress noise while avoiding detail loss in reconstructing high-frequency information by gradually removing noise and artifacts while preserving and enhancing the original image details.
To further enhance the restoration of intricate image details and overall reconstruction quality, DEGAN incorporates a Convolutional Block Attention Module (CBAM) within the generator, which merges channel and spatial attention mechanisms. Our design inserts the CBAM module before up-sampling: specifically, after extracting high-dimensional features through the Conv layer, the CBAM module is used to adaptively adjust these features before passing them to the sub-pixel up-sampling module for up-sampling. This design enables fine-tuning of the features before up-sampling to ensure that the key details are preserved and enhancing the precision of the following reconstruction stages. The CBAM module adaptively adjusts the significance of each channel using the channel attention mechanism, while also incorporating the spatial attention mechanism to prioritize important local information in the image, effectively reducing unwanted noise interference. The features processed by the CBAM module can be more accurately transferred to the subsequent up-sampling and refinement stages, enabling the network to generate more detailed and accurate super-resolution images when processing remote sensing images with complex texture and edge information.
DEGAN utilizes a deep convolutional neural network architecture, which generates a scalar value representing the authenticity of an image through several layers of convolution, activation, pooling, and fully connected operations. It is worth noting that to cope with the slight blurring effect that may be introduced by the diffusion model during the generation process, the discriminator is designed with a feature extraction module in both the shallow and deep layers, where the shallow layer can capture details and edge information, while the deep layer focuses on global texture and structural features. This multi-scale design allows the discriminator to effectively detect local blurring introduced by diffusion enhancement while maintaining the overall structural coherence of the image. The discriminator’s intricate architecture strengthens its ability to analyze complex images, effectively differentiating synthetic images from real ones and thereby elevating the quality and authenticity of the generated outputs.
Unlike traditional super-resolution models, DEGAN greatly enhances the recovery of image details by introducing a diffusion module, especially in the processing of complex textures and edges in remote sensing images, which demonstrates excellent performance. By integrating the diffusion module into the generator’s U-Net structure and utilizing skip connections with an attention mechanism, DEGAN is better equipped to recover fine-grained details and enhance the resolution of remote sensing images. This method not only improves both visual quality and quantitative performance but also maintains exceptional image fidelity, even in the presence of challenges like atmospheric interference, tele-imaging distortions, and spectral noise.
2.2 Generator design
In this study, we design a generator that integrates diffusion modules, as shown in Figure 1. The upper part of the figure illustrates the generator component. The low-resolution (LR) image is generated through bicubic interpolation, with input dimensions of N × 3×w × h, where N represents the batch size, 3 corresponds to the RGB image channels, and w and h denote the image’s width and height. In contrast, the super-resolved image has dimensions of N × 3×w·s × h·s, with s being the magnification factor. The primary goal of the generator is to model the transformation from low-resolution (LR) images to high-resolution (HR) super-resolved images.
Initially, the input image undergoes processing through several convolutional layers that progressively expand the feature map’s channel count from 3 to 128. The detailed convolution process is mathematically defined by the equation shown below (Equations 1–4):
After the initial convolutional layers, the model employs a series of residual blocks, each consisting of convolutional layers and skip connections that facilitate direct gradient flow through the network during backpropagation. The key to the residual blocks is to learn the “residuals” between the inputs and outputs through the jump connections, rather than predicting the outputs directly. This technique effectively mitigates the vanishing gradient issue commonly encountered in deep networks. The formula for the residual module is as follows (Equations 5–7):
Following the processing through multiple residual blocks, the features are further refined using the CBAM module, where channel weights are calculated by performing global average pooling. This process helps to emphasize the crucial channel information in the image, thereby improving the reconstruction quality, as shown in the following formula (see Equation 8):
Super-resolution reconstruction demands meticulous feature selection across various channels. The channel attention mechanism plays a crucial role in emphasizing vital information, minimizing the impact of less significant channels, and ultimately boosting the model’s overall performance.
Next, the model performs super-resolution upscaling using sub-pixel convolution, where each block increases the feature map resolution, effectively doubling the image resolution to the target dimensions. The sub-pixel convolution operation is described as follows (see Equation 9):
After applying sub-pixel convolution, the model utilizes a diffusion module. This module leverages a U-Net architecture to refine and enhance the images, aiming to substantially improve the final super-resolved output’s overall quality. The operation of the diffusion module can be represented as follows (see Equation 10):
Finally, the model further processes the image through multiple convolutional layers (conv44, conv4, conv55, conv6)and generates the final high-resolution output image. The specific operation formula is given below (see Equations 11–14):
Finally, the output of high-resolution image is normalized by the Tanh activation function (see Equation 15):
The network structure in this paper aims to improve the reconstruction performance of super-resolution images through the collaboration of multiple modules. In this paper, we integrate the residual block, CBAM module, and diffusion module of the generator. The inclusion of jump connections in the residual block mitigates the gradient vanishing problem and ensures that the gradient can pass through the deep network smoothly, thus capturing the image details efficiently.The CBAM module combines the channel and spatial attention mechanisms to focus on the key feature regions of the image, thus improving the recovery of image details and structures. The diffusion module, based on the U-Net architecture, progressively denoises the image while preserving high-frequency details, thereby significantly improving the quality and visual realism of the image. By combining these three modules, the generator is able to effectively recover details from low-resolution images, enhance the global structure and texture details of the image, and ensure that the final high-resolution image generated is of higher quality.
2.2.1 Diffusion module
The module mainly features an encoder-decoder architecture with skip connections, as illustrated in Figure 2. The encoder section uses a convolutional layer to progressively extract higher-order features from the image, while downsampling the spatial resolution (with the convolutional layer’s stride set to 2) to create a multi-scale feature representation. The feature map
On this basis, the diffusion time step
In the diffusion module, we gradually reduce the intensity of the noise by diffusion time step (t). The time step (t) controls the noise intensity in the image generation process. During the diffusion process, the noise intensity is gradually reduced as the time step (t) progresses to remove noise and artifacts from the image and to recover the image details. The time step (t) is embedded through a fully connected network and then added to the feature map element by element, injecting the noise information into the network as a conditional input, thus ensuring effective denoising of the image.
Subsequently, the decoder employs transposed convolution operations to progressively restore the image’s spatial resolution. After the first layer of transpose convolution for upsampling
The overall network structure improves the accuracy and detail of image reconstruction by merging shallow and deep features to achieve both efficient information compression and full utilization of detailed information in the reconstruction stage.
2.2.2 Attention mechanism CBAM
In this paper, the design of the CBAM incorporates several important hyperparameter configurations aimed at improving the visual quality of images. The channel attention module computes attention through a shared multilayer perceptron, as well as using two convolutional layers with a convolutional kernel size of 1. A ReLU activation function is inserted between them to enhance nonlinear representation. To reduce the computational complexity, a reduction ratio of 16 is set to efficiently extract important channel information.
The spatial attention module to enhance the information related to key spatial locations in the image uses a 2D convolutional layer of size 7 to generate the spatial attention weights by calculating the average and maximum values of each channel and finally connecting them.
Channel attention focuses on the importance of different channels, while spatial attention emphasizes the key spatial locations in the image. Through the effective combination of channel attention and spatial attention, CBAM ensures the balance of both in feature enhancement. Utilizing this combination, CBAM can adaptively adjust the feature response to effectively improve the detail recovery and high-frequency parts, which significantly improves the overall image reconstruction quality.
In the CBAM module, the network’s ability to emphasize important features is enhanced by sequentially applying both channel and spatial attention mechanisms. This process adaptively refines the feature responses across both the channel and spatial axes, as shown in Figure 3.
Initially, the input feature map is
The result
2.3 Discriminator design
The discriminator section employs a convolutional neural network (CNN) to construct a deep model comprising several convolutional layers, activation functions, pooling operations, and fully connected layers, which ultimately produces a scalar value indicating the image’s authenticity.
First, the discriminator passes through multiple convolutional blocks, each comprising a convolutional layer, batch normalization, and a LeakyReLU activation function (see Equation 16). The operation of each block can be described as follows:
where
where
where α is a small constant used to solve the “dead neuron” problem of ReLU.
In the first convolutional block, the output channels are set to n_channels, and this value is doubled after every other convolutional layer in the subsequent blocks. The convolution kernel size of each convolution block is kernel_size, and the convolution step stride is 1 or 2, when the step is 1, the image size remains unchanged, and when the step is 2, the image size is halved. The overall convolution process can be expressed as follows (see Equation 19):
After multiple convolutional layers, the image’s spatial resolution is gradually reduced and the number of channels is gradually increased to capture more abstract features.
After multiple convolutional blocks, the output feature maps undergo changes in their spatial dimensions, and the result is then processed with an adaptive average pooling operation. Adaptive pooling resizes the output’s spatial dimensions to 6 × 6, ensuring that the feature map size remains constant, regardless of the input image size. The pooling operation can be expressed as (see Equation 20):
In this case, the output after adaptive pooling is a fixed-size feature map adaptive_pool_output with the size of N × C×6 × 6 and C is the number of channels. The fundamental concept of adaptive pooling is to reduce the input image to a fixed output size through average pooling.
Next, the pooled feature map is flattened and passed through a fully connected layer, which maps all values in the feature map to a fixed-size vector. The flattening operation is denoted as (see Equation 21):
It is subsequently passed through the fully connected layer fc1, with the formula for the first layer given as follows (see Equation 22):
where
Then, the LeakyReLU activation function is applied to the output
At this point, a second fully connected layer, fc2, is passed, mapping the output from the previous layer to a scalar value that indicates whether the image is a true HR image (see Equation 24). The second fully connected layer Eq:
where
2.4 Loss function
This research develops a composite loss strategy is developed by integrating multiple loss functions, aiming to enhance the perceptual quality of the generated images through simultaneous refinement of pixel-level accuracy and high-level visual features. Specifically, the loss functions employed include content loss, VGG feature loss, and adversarial loss. The synergistic combination of these loss functions helps to further enhance the image’s fine details and high-frequency components, improving upon pixel-level restoration, and thus boosting the realism and visual appeal of the generated image.
2.4.1 Loss of content
Content loss quantifies the pixel-level differences between the generated and real images. We employ the mean square error (MSE) loss function to calculate the content loss. This loss function aids the generator in progressively restoring image details by minimizing pixel-wise differences between the generated and high-resolution images. The formula for content loss is as follows (see Equation 25):
Here,
2.4.2 Perceived loss
To enhance the visual quality of the generated images, we incorporate the feature loss from the VGG19 model. VGG19 has demonstrated exceptional performance in capturing high-level semantic features of images, especially in applications like image classification and generation. In this study, we utilize the convolutional layers of VGG19 (excluding the final classification layer) to capture high-level features from an image and assess the discrepancy between the generated and real images within these feature spaces.
The VGG feature loss guides the generator by comparing the VGG features of the generated image to those of the real image, enabling the model to not only minimize pixel-level discrepancies but also capture the high-level structural and semantic content of the image. The formula for the VGG feature loss is (see Equation 26):
Here,
2.4.3 Adversarial loss
Adversarial loss is a key part of Generative Adversarial Networks (GANs), optimizing the generator to create more convincing images that can be progressively refined through feedback from the discriminator. In the GAN framework, the discriminator’s task is to determine whether an image originates from a real dataset or is a synthetic one created by the generator. Simultaneously, the generator strives to improve the quality of the images through adversarial learning, making it progressively harder for the discriminator to differentiate between real and generated images.
The expression for computing the adversarial loss is given by (see Equation 27):
where D(I) denotes the true probability output of the discriminator for image I. During the training process, the adversarial loss helps the generator to optimize the details and complexity of the image, especially the high-frequency part, through the feedback from the discriminator, thus making the image more natural and realistic. By minimizing the adversarial loss, the generator gradually improves the realism and visual finesse of the image, especially in the case of complex backgrounds or rich textures. Adversarial training motivates the generator to continuously improve the details, so that the realism and visualization of the image are improved, and the final generated image is more vivid in details and complexity.
To balance the influence of each loss function, we introduced hyperparameters λ1, λ2, and λ3 into the total loss, which control the contributions of content loss, VGG feature loss, and adversarial loss, respectively. In this study, the weight coefficients for content loss and VGG feature loss were both set to 0.5, while the weight coefficient for adversarial loss was set to 0.1. These hyperparameters were adjusted experimentally to ensure high-quality image reconstruction, effective guidance of high-level features, and enhanced visual realism.
3 Results
To assess the effectiveness of the proposed DEGAN method, experiments are conducted using publicly available remote sensing datasets.
3.1 Experimental setup
Our model was trained and evaluated on three remote sensing datasets: UC Merced, AID, and NEG-Scene. The UC Merced dataset consists of 21 different remote sensing scenes, such as farmland, airports, and buildings. Each scene category contains 100 images, each with a resolution of 256 × 256 pixels and a spatial resolution of 0.3 m per pixel. The dataset has clear feature boundaries, is suitable for remote sensing image super-resolution tasks, and is effective in testing high-resolution reduction performance. The AID dataset includes 30 distinct scenes, such as farmland, airports, and buildings. Each image in this dataset has a resolution of 600 × 600 pixels and a spatial resolution of 0.5 m per pixel. The dataset contains a variety of complex backgrounds and multiple feature types, making it suitable for testing the effectiveness of reconstructing a variety of complex scenes in remote sensing image super-resolution tasks. The NEG-Scene dataset consists of 1000 high-resolution images, each with a spatial resolution of 0.5 m and dimensions of 400 × 400 pixels. The dataset contains remotely sensed imagery from a variety of environments and geographic regions, providing a more complex test environment for super-resolution tasks. For these datasets, we consider the images as high-quality images and generate their corresponding low-quality versions by applying bicubic interpolation. Our objective is to recover the high-resolution images from their low-resolution versions. The experiments were carried out using the PyTorch framework, and the computations were performed on a single NVIDIA GeForce RTX 4090 GPU featuring 24 GB of memory. To ensure a fair comparison, each model was trained on the identical dataset and assessed individually through an end-to-end process. The evaluation metrics include the Peak Signal-to-Noise Ratio (PSNR) as well as the Structural Similarity Index (SSIM). PSNR quantifies pixel-level variations between two images, whereas SSIM assesses the similarity in structure. An increased PSNR value signifies superior image quality, while an SSIM score approaching 1 implies that the generated image closely resembles the original high-resolution version.
3.2 Evaluation indicators
We quantitatively assessed the effectiveness of our method in comparison to other approaches using two key metrics: PSNR and SSIM. The formulas and detailed descriptions of these metrics are provided in Table 1.
PSNR assesses image quality by measuring pixel-level variations, whereas SSIM evaluates the structural similarity between a super-resolved image and the original high-resolution version. In general, higher PSNR and SSIM scores indicate superior image quality.
3.3 Experimental procedure
For our experiments, we have chosen three remote sensing image collections: the AID dataset for scene classification, the UC Merced dataset, and the NEG-Scene dataset. In this paper, we inspect and crop all remote sensing images used for training to remove color distortion. Our study concentrates on the super-resolution task with two levels of magnification: ×2 and ×4. The high-resolution images are sized at 256 × 256, while the low-resolution counterparts are generated by downsampling using double cubic interpolation.
All experiments were performed with PyTorch, and the models were trained on a single NVIDIA GeForce RTX 4090 GPU with 24 GB of memory. In this paper, we set the batch size to 16 to ensure that the model does not experience memory overflow during training. If a larger batch size is required, it can be adjusted according to the available GPU memory.
During training, we used the Adam optimizer with β1 initialized to 0.9 and β2 initialized to 0.999. The initial learning rate was set to 0.0001. To accelerate convergence, we used a learning rate decay strategy, where the learning rate was reduced to 10% of the original after 50 cycles of training. This strategy can fine-tune the model at a later stage to make the image reconstruction more stable and accurate.
3.4 Experiments with simulated image datasets
To evaluate the effectiveness of our proposed DEGAN model, we conducted comparative experiments using the double-cubic interpolation technique along with seven advanced super-resolution methods, including EFDN, DBPN, SRGAN, ESRGAN, HSENet, TransENet, and SwinIR. These models are highly valuable for tasks related to super-resolution of remote sensing images. We re-implemented each of these approaches using publicly available code and carried out evaluations under the same testing conditions. EFDN (Edge-enhanced Feature Distillation Network) is an innovative method presented at the 2022 IEEE Conference on Computer Vision and Pattern Recognition (CVPR), which leverages edge-enhanced feature distillation. HSENet is an efficient super-resolution model introduced in the 2021 issue of IEEE Transactions on Geoscience and Remote Sensing (TGRS), which focuses on enhancing image details through adaptive learning. TransENet and SwinIR are both Transformer-framed models. To ensure an unbiased evaluation, all models are trained and tested on the UCMerced dataset, with consistent batch sizes and the same evaluation metrics (PSNR and SSIM).
Table 2 presents the effectiveness of the DEGAN model alongside six other comparison models for x2 and x4 super-resolution reconstruction tasks on the UCMerced dataset. While many contemporary methods, such as SwinIR, EFDN, and DBPN, perform well in terms of PSNR and SSIM, DEGAN surpasses them in both metrics. Notably, at ×4 magnification, DEGAN achieves a PSNR of 28.901 and an SSIM of 0.7962, which significantly surpasses the other models.
The experimental results in Table 2 reveal that among all the methods, Bicubic interpolation yields the poorest performance, particularly at ×4 magnification, with a PSNR of 23.83 and SSIM of 0.6468. This suggests that it significantly lags behind deep learning-based methods in terms of detail recovery and high-quality reconstruction. In contrast, deep learning-based methods (such as SRGAN and ESRGAN) show some improvement at ×2 magnification. However, at ×4 magnification, their PSNR and SSIM values remain relatively low, highlighting the challenges these methods face when dealing with high-magnification images.
EFDN and DBPN are more advanced methods proposed in recent years with better edge recovery and global feature modeling capabilities, but at ×4 magnification, their PSNR values are 27.71 and 28.47, respectively, and their SSIM values fail to reach the desired level, especially in the detail recovery, which still has some room for improvement. HSENet, TransENet, and SwinIR achieve relatively high performance by adopting more convolutional layers and feature extraction strategies, especially SwinIR, which shows further improvement in detail and global information recovery by introducing the attention mechanism of Transformer, with a PSNR of 28.23 and SSIM of 0.7796.
However, despite the progress made by SwinIR and other methods, our proposed DEGAN model shows stronger advantages at both zoom factors (x2 and x4). Specifically, in the 4x zoom task, DEGAN reaches a PSNR of 28.901 and an SSIM of 0.7962, outperforming SwinIR (28.23/0.7796) by 0.67 dB in PSNR and 0.0166 in SSIM. This indicates a notable enhancement in image detail recovery and visual quality with our method. DEGAN achieves this objective through a carefully designed module, incorporating mechanisms such as attention and diffusion, which significantly improve the model’s performance to recover details and perform well in high-magnification scenarios. It also addresses the issue of detail loss that is common in existing methods when processing remote-sensing images.
We also compare the performance of the different algorithms on two evaluation metrics, namely, the number of parameters (Params) and the amount of floating-point operations (FLOPs).Params refers to the total number of weights and biases that need to be learned and stored in the model.The higher the Params, the more expressive the model is, but it may also lead to greater memory usage and risk of overfitting.FLOPs denotes the number of floating-point operations required for the model to perform one forward number of floating-point operations required for the model to perform one forward inference.The higher the value of FLOPs, the more computationally intensive the model is, and the inference may be slower and more demanding on hardware computational power. In the Params metric, EFDN and SRGAN have smaller Params, which helps to reduce memory occupation and improve computational efficiency. In the FLOPs metric, HSENet and ESRGAN have higher FLOPs and higher computational requirements. The improvement of our model DEGAN in these two metrics will be an important direction for future work.
Figures 4, 5 illustrate the 2× super-resolution reconstruction outputs of each algorithm on two remote-sensing images. The subfigures are arranged in a sequential order, starting from the upper-left corner and proceeding to the bottom-right, showing the reconstruction results for the HR reference image, double-cubic interpolation, SRGAN, EFDN, DBPN, HSENet, TransENet, SwinIR, and the DEGAN method introduced in this study. From the figures, it can be seen that the overall clarity of the reconstructed image using bicubic interpolation is insufficient, the loss of details is more serious and the edges are too smooth, presenting an obvious blurring effect; SRGAN results have improved the blurring problem to a certain extent, but it is still accompanied by some artifacts; the edges of the reconstructed image by EFDN are softer, but it is slightly insufficient in terms of sharpness, and at the same time there are fewer artifacts; DBPN excels in accurately reproducing both structural forms and intricate textures, whereas EFDN achieves even more impressive reconstruction outcomes. HSENet yields reconstructions with excellent clarity; however, minor artifacts are evident in regions featuring intricate backgrounds; TransENet is excellent in controlling the artifacts, but the texture performance in some areas still needs to be improved; Visually, the output of SwinIR resembles that of our proposed DEGAN approach; however, DEGAN’s reconstructions more faithfully capture the appearance of authentic high-resolution images. Overall, the outputs of SwinIR and our proposed DEGAN approach display similar visual quality; however, DEGAN’s reconstruction more effectively recovers the global structure and closely mimics the visual characteristics of the authentic high-resolution image.
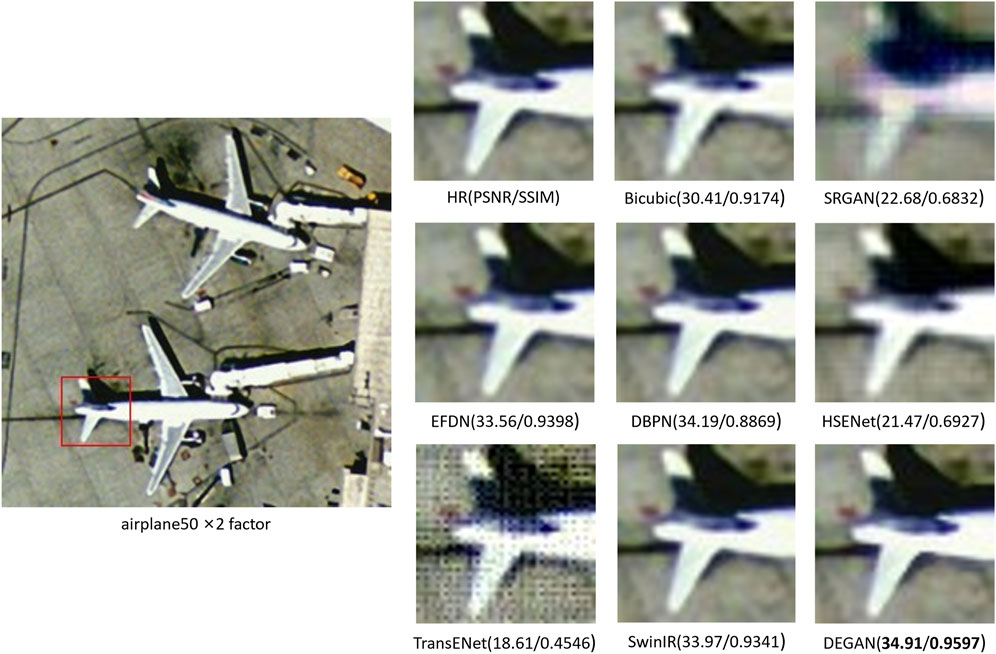
Figure 4. Comparison of 2x super-resolution reconstruction results of each algorithm on an analog image in the UCMerced dataset.
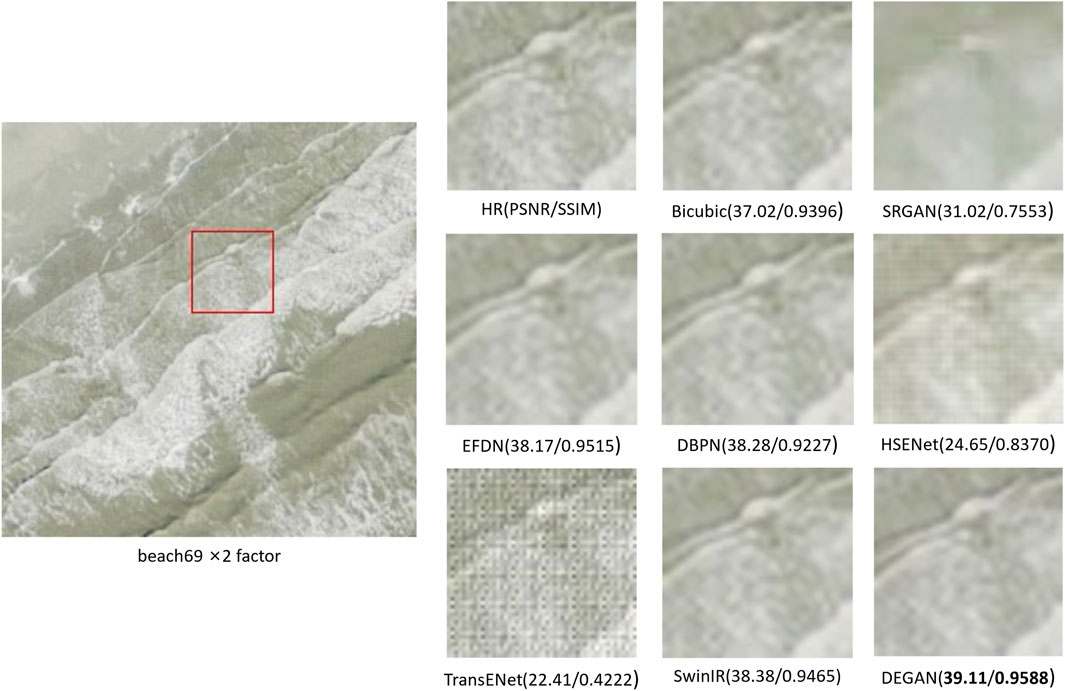
Figure 5. Comparison of 2x super-resolution reconstruction results of each algorithm on another simulated image in the UCMerced dataset.
Figures 6, 7 display the 4x upscaled outputs produced by various methods when applied to two simulated images. In these figures, the subfigures arranged row-wise from the top-left to the bottom-right, each sequentially illustrating the reconstruction outcomes of the HR reference image, bicubic interpolation, SRGAN, EFDN, DBPN, ESRGAN, HSENet, TransENet, SwinIR, and the proposed DEGAN algorithm. The reconstruction results of SRGAN are slightly blurred in texture rendering, and the reconstruction of EFDN is smooth, but the texture details are not sufficiently detailed, and the artifacts are slightly visible in the high-contrast region. The reconstruction results of EFDN are smooth, and although the artifacts are effectively controlled, the texture details are insufficient and lack realism; DBPN has excellent overall clarity, but artifacts can still be observed in some high-contrast areas; the reconstruction results of ESRGAN and HSENet are more balanced, and only a small number of artifacts are detected in local areas; the reconstructed image of TransENet has fewer artifacts but the texture hierarchy is insufficient; the reconstruction results of SwinIR and DEGAN are less blurred in the texture rendering. The TransENet reconstructed image has fewer artifacts, but the texture level is insufficient; the SwinIR reconstructed image shows high clarity, although there are still artifacts in some areas. By contrast, our DEGAN approach significantly improves the restoration of the overall structure and true textures while preserving image clarity, resulting in a visual output that more accurately reflects the authentic high-resolution image.
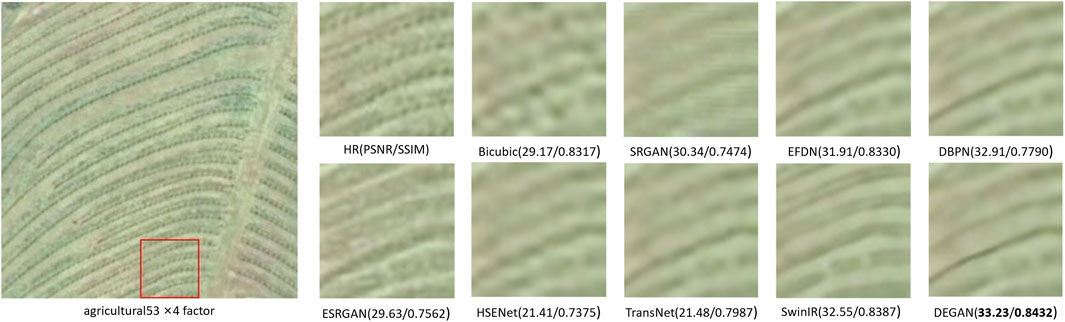
Figure 6. Comparison of 4-fold super-resolution reconstruction results of each algorithm on a simulated image in the UCMerced dataset.
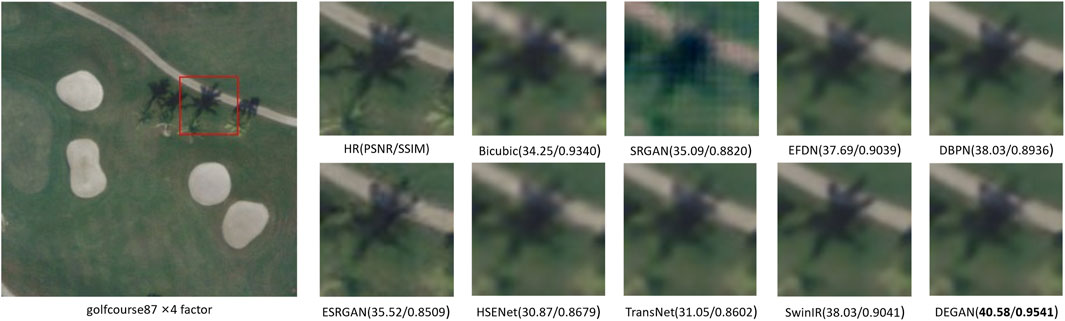
Figure 7. Comparison of the results of 4-fold super-resolution reconstruction of each algorithm on another simulated image in the UCMerced dataset.
By comparing the reconstruction results of different methods, the experiments show that our proposed DEGAN method performs superiorly in the super-resolution task of remote sensing images. Compared with other methods, DEGAN is able to recover the global structure more efficiently and capture the visual features of real high-resolution images, especially in complex background and detail recovery. Although other methods like SwinIR also perform well in terms of visual quality, DEGAN is more stable in image recovery and can maintain high-precision reconstruction at different scales. This suggests that DEGAN has a strong potential for application in the field of remote sensing image super-resolution, especially when dealing with remote sensing images with complex backgrounds.
3.5 Ablation experiment
In this part of the research, we perform a series of ablation experiments to assess the influence of attention mechanisms and diffusion modules on super-resolution performance.
3.5.1 Effectiveness of attention mechanisms
To assess the effect of the attention mechanism (CBAM) on super-resolution performance, we performed an ablation study with a 4x scaling factor, comparing the effects of various attention modules, including SE, CAM, SAM, and the integrated CBAM. We evaluated the performance improvements brought by each attentional mechanism in the super-resolution model and compared them with a baseline model that lacks any attention mechanism. The findings from these experiments are presented in Table 3.
The results of the experiments show that incorporating the CBAM module leads to a 0.2 dB improvement in PSNR and a slight improvement in SSIM (by 0.002). This suggests that the CBAM module provides a small but significant gain in the image feature extraction process, which is especially helpful in recovering the edge details of the image. Our further analysis reveals that the CBAM module, especially in low-contrast regions and regions with more details, optimizes the ability of detail reconstruction. Compared to other attention mechanisms, CBAM can better balance channel and spatial attention, thus improving the overall reconstruction quality.
In addition, we conducted comparison experiments with various attention mechanisms. The results indicate that although the SE module has some improvement on the super-resolution task, its effect is slightly inferior compared to CBAM. In terms of performance, the CBAM module outperforms other types of attentional mechanisms in both PSNR and SSIM, and shows superior visual effects. Especially in detail recovery and edge sharpness, the CBAM module shows a clear advantage.
In this experiment, the CBAM module contains two main parts: the Channel Attention Module (CAM) and the Spatial Attention Module (SAM). CAM enhances important features mainly by adaptively adjusting the inter-channel weights, while SAM further improves the model’s ability to capture the spatial structure of the image. The combination of the two makes CBAM more efficient than a single module in processing complex image details.
3.5.2 Effectiveness of diffusion modules
To assess the impact of the diffusion module (Diffusion) on the image super-resolution task, we compare a model incorporating this module with a baseline model that excludes it. Table 4 presents the PSNR and SSIM values for each model at a 4x scaling factor.
Based on the data in Table 4, integrating the diffusion module leads to an increase in PSNR by 0.446 dB. Although the change in SSIM is minimal, the significant boost in PSNR highlights the crucial role of the diffusion module in improving the image’s structural clarity. The diffusion module effectively restores the high-frequency details in the image by gradually removing noise and artifacts. In the reconstruction process, the module utilizes the noise level as the diffusion step t. By mapping through the fully connected layer and incorporating the bottleneck features as residuals, the network can dynamically adjust the denoising intensity according to varying noise levels, thereby improving the accuracy of texture and edge restoration. Second, the introduction of the Transformer encoder in the bottleneck layer enables self-attentive modeling of the global features, enabling the network to capture long-range relationships within the image, which is crucial for restoring fine details. It is the effective integration of fine-grained details and abstract semantics that enhances the realism of the reconstructed image in terms of both structure and details, thereby demonstrating a clear advantage in the PSNR metric.
Furthermore, we investigate how various U-Net architectures affect super-resolution outcomes by comparing the standard U-Net with a variant that incorporates a Transformer structure in its bottleneck layer. The experimental results (see Table 5) show that the structure after the introduction of the Transformer has certain advantages in global feature modeling, especially when dealing with complex texture and edge information, which can further improve the ability to recover image details and thus achieve finer reconstruction results.
Based on the ablation experiments discussed above, the diffusion module demonstrates notable advantages in merging low-level and high-level features while also strengthening the restoration of high-frequency details. Its significant improvement in PSNR is mainly due to the following reasons: on the one hand, by accurately modeling the noise level and dynamically adjusting the denoising strength, the diffusion module can retain the detailed information more efficiently; on the other hand, the introduction of the Transformer in the bottleneck layer further enhances the ability to model the global information, allowing it to capture long-range dependencies and, as a result, more effectively restore complex textures and edge details. The results of different U-Net variants also show that a reasonable design of the network structure can significantly improve the image reconstruction quality while maintaining computational efficiency. These results provide valuable references and improvement directions for further optimization of super-resolution networks.
4 Discussion
4.1 Comparison with previous methods
In this study, a novel super-resolution reconstruction method for remote sensing images is proposed, which improves the quality of super-resolution reconstruction of remote sensing images by embedding the diffusion module and attention mechanism into the generative adversarial network. And certain improvements are made to address the problems of insufficient detail information and adaptability to complex environments. Numerous experimental results show that the DEGAN model proposed in this study performs well in the task of super-resolution reconstruction of remote sensing images, surpassing many existing methods in detail recovery and global structure reconstruction.
Compared with the SEG-ESRGAN model, DEGAN is able to better adapt to complex scenes and is clearer in the recovery and reconstruction of edge information (Salgueiro et al., 2022). Compared with the MBGPIN model, DEGAN improves its reconstruction quality in high-frequency texture by introducing the attention mechanism, which makes the texture details in the generated image more realistic and natural (Sharifuzzaman et al., 2024). Compared with the TBMRA model, DEGAN further improves the image quality by introducing the diffusion module, which enables the model to obtain more detailed information in complex scenes, showing similar advantages to the TBMRA model (Patnaik et al., 2024). Compared with the MRENet model, DEGAN captures the detail information more effectively through adversarial training and better handles the edge information in the image, thus improving the reconstruction quality of remote sensing images (Safarov et al., 2025).
Therefore, DEGAN not only has unique advantages in detail recovery of remote sensing images, but also provides an important reference in improving the quality of super-resolution reconstruction and enhancing the detail information.
4.2 Limitations and future work
The DEGAN model proposed in this paper performs well in most of the remote sensing image super-resolution tasks, but still has some limitations. First, in some cases where high noise exists, extreme noise and complex scenes may affect the reconstruction results of the model. In addition, the model may suffer from excessive smoothing or artifacts on image edges and detail information in some specific scenes. Future work will focus on optimizing the denoising process, enhancing the robustness to extreme noise, and improving the reconstruction results in complex scenes.
Although the model in this paper has successfully processed remote sensing images with a maximum resolution of 600 × 600, high-resolution remote sensing images usually contain more detailed information, so in future research we will consider extending the model to support higher resolution image processing. At the same time this brings higher computational complexity and memory requirements. To address this issue, future work will focus on optimizing the network structure and improving the computational efficiency of the model so that it can process images with larger resolution. In addition, we will explore methods such as self-supervised learning to improve the robustness and efficiency of the model so that it is better adapted to the task of super-resolution reconstruction of high-resolution images.
To further boost the model’s efficiency and resilience, subsequent enhancements may be implemented across three distinct areas: network structure optimization, self-supervised learning and pre-training strategies, and software and hardware co-optimization for computational complexity. First, in network structure optimization, multi-scale feature fusion technology can be adopted to effectively fuse feature information of different resolutions by designing multi-scale branching or pyramid structure, which not only improves the detail recovery ability, but also helps to minimize the computational complexity; in addition, the use of techniques such as depth-separable convolution, pruning, or quantization to construct a lightweight network architecture, which can reduce the model’s parameter size and computational requirements while preserving reconstruction performance. Secondly, in terms of self-supervised learning and pre-training strategies, designing self-supervised pre-training tasks based on contrast learning or occlusion recovery, and utilizing a large dataset of unlabeled remote sensing images for pre-training to capture additional latent image features and enhance the robustness of super-resolution reconstruction; at the same time, by adopting multi-task joint learning and integrating tasks such as image segmentation and object detection, the model can share underlying features, enhancing its generalization capability and improving performance in detail recovery across various remote sensing scenarios. Finally, for the computational complexity, we can also start from software and hardware co-optimization, study the efficient inference mechanism of the model on embedded devices or GPUs, and further shorten the inference time through the combination of algorithm optimization and hardware acceleration; at the same time. With the help of distributed training and model compression techniques, the performance and flexibility of large-scale data processing can be improved while maintaining reconstruction quality. In summary, although DEGAN has shown considerable advancements in the super-resolution reconstruction of remote sensing images, future work should address the challenges related to computational complexity and detail processing. This will help enhance the model’s efficiency and robustness, enabling broader practical applications in remote sensing image processing.
5 Conclusion
In this study, we propose an improved method for super-resolution reconstruction of remote sensing images, DEGAN. By incorporating the diffusion module and the attention mechanism into the generator, the model improves the adaptability to complex remote sensing scenarios while enhancing the detail restoration and improving the image realism. The diffusion module further improves image details and suppresses blurring through denoising, thus significantly enhancing the visual effect of the generated results. Through adversarial training, our model is optimized in terms of pixel accuracy and perception, thus enhancing the super-resolution reconstruction quality of remote sensing images. Through a large number of experimental results, it is shown that DEGAN outperforms the traditional super-resolution algorithm and other existing state-of-the-art SR models, and significantly improves the super-resolution reconstruction performance of remote sensing images.
Data availability statement
Publicly available datasets were analyzed in this study. This data can be found here: http://weegee.vision.ucmerced.edu/datasets/landuse.html.
Author contributions
RL: Conceptualization, Methodology, Resources, Software, Visualization, Writing – original draft, Writing – review and editing. LW: Data curation, Validation, Visualization, Writing – review and editing. SS: Formal Analysis, Software, Visualization, Writing – review and editing. MY: Conceptualization, Funding acquisition, Methodology, Supervision, Writing – original draft, Writing – review and editing. LM: Investigation, Writing – review and editing.
Funding
The author(s) declare that financial support was received for the research and/or publication of this article. This work was supported by the Inner Mongolia Autonomous Region’s unveiling and leadership project (2024JBGS0014).
Acknowledgments
We also appreciate the helpful feedback from our colleagues during the internal review process.
Conflict of interest
The authors declare that the research was conducted in the absence of any commercial or financial relationships that could be construed as a potential conflict of interest.
Generative AI statement
The author(s) declare that no Generative AI was used in the creation of this manuscript.
Publisher’s note
All claims expressed in this article are solely those of the authors and do not necessarily represent those of their affiliated organizations, or those of the publisher, the editors and the reviewers. Any product that may be evaluated in this article, or claim that may be made by its manufacturer, is not guaranteed or endorsed by the publisher.
Supplementary material
The Supplementary Material for this article can be found online at: https://www.frontiersin.org/articles/10.3389/feart.2025.1578321/full#supplementary-material
References
Chen, Z., Zhang, Y., Gu, J., Kong, L., Yang, X., Yu, F., et al. (2023). “Dual aggregation transformer for image super-resolution,” in Proceedings of the IEEE/CVF international conference on computer vision, 12312–12321. doi:10.48550/arXiv.2308.03364
Dong, C., Loy, C. C., He, K., and Tang, X. (2014). “Learning a deep convolutional network for image super-resolution,”. Computer vision – ECCV 2014, lecture notes in computer science. Editors D. Fleet, T. Pajdla, B. Schiele, and T. Tuytelaars (Cham: Springer), 8692, 184–199. doi:10.1007/978-3-319-10593-2_13
Dong, X., Wang, L., Sun, X., Jia, X., Gao, L., and Zhang, B. (2020). Remote sensing image super-resolution using second-order multi-scale networks. IEEE Trans. Geoscience Remote Sens. 59 (4), 3473–3485. doi:10.1109/TGRS.2020.3019660
Esser, P., Rombach, R., and Ommer, B. (2021). “Taming transformers for high-resolution image synthesis,” in Proceedings of the IEEE/CVF conference on computer vision and pattern recognition, 12873–12883. doi:10.48550/arXiv.2012.09841
Haut, J. M., Paoletti, M. E., Fernández-Beltran, R., Plaza, J., Plaza, A., and Li, J. (2019). Remote sensing single-image super-resolution based on a deep compendium model. IEEE Geoscience Remote Sens. Lett. 16 (9), 1432–1436. doi:10.1109/LGRS.2019.2899576
Ho, J., Jain, A., and Abbeel, P. (2020). Denoising diffusion probabilistic models. Adv. Neural Inf. Process. Syst. 33, 6840–6851. doi:10.48550/arXiv.2006.11239
Hu, W., Ju, L., Du, Y., and Li, Y. (2024). A super-resolution reconstruction model for remote sensing image based on generative adversarial networks. Remote Sens. 16 (8), 1460. doi:10.3390/rs16081460
Huan, H., Li, P., Zou, N., Wang, C., Xie, Y., Xie, Y., et al. (2021). End-to-end super-resolution for remote-sensing images using an improved multi-scale residual network. Remote Sens. 13 (4), 666. doi:10.3390/RS13040666
Jain, R., and Vatsavai, R. R. (2024). “Deep super resolution techniques for remote sensing big data: a comparative study,” in 2024 IEEE international conference on big data (BigData) (IEEE), 6011–6020. doi:10.1109/BigData62323.2024.10825964
Keshk, H. M., and Yin, X. C. (2021). Obtaining super-resolution satellites images based on enhancement deep convolutional neural network. Int. J. Aeronautical Space Sci. 22, 195–202. doi:10.1007/s42405-020-00297-0
Lei, S., and Shi, Z. (2021). Hybrid-scale self-similarity exploitation for remote sensing image super-resolution. IEEE Trans. Geoscience Remote Sens. 60, 1–10. doi:10.1109/TGRS.2021.3069889
Lei, S., Shi, Z., and Mo, W. (2021). Transformer-based multistage enhancement for remote sensing image super-resolution. IEEE Trans. Geoscience Remote Sens. 60, 1–11. doi:10.1109/TGRS.2021.3136190
Lei, S., Shi, Z., and Zou, Z. (2017). Super-resolution for remote sensing images via local–global combined network. IEEE Geoscience Remote Sens. Lett. 14 (8), 1243–1247. doi:10.1109/lgrs.2017.2704122
Li, H., Yang, Y., Chang, M., Chen, S., Feng, H., Xu, Z., et al. (2022). Srdiff: single image super-resolution with diffusion probabilistic models. Neurocomputing 479, 47–59. doi:10.1016/j.neucom.2022.01.029
Li, X., and Ren, Y. (2023). Diffusion models for image restoration and enhancement--A comprehensive survey. arXiv Prepr. arXiv:2308.09388. doi:10.48550/arXiv.2308.09388
Liang, J., Cao, J., Sun, G., Zhang, K., Van Gool, L., Timofte, R., et al. (2021). “Swinir: image restoration using swin transformer,” in Proceedings of the IEEE/CVF international conference on computer vision, 1833–1844. doi:10.48550/arXiv.2108.10257
Liebel, L., and Körner, M. (2016). Single-image super resolution for multispectral remote sensing data using convolutional neural networks. Int. Archives Photogrammetry, Remote Sens. Spatial Inf. Sci. 41, 883–890. doi:10.5194/isprs-archives-XLI-B3-883-2016
Lim, B., Son, S., Kim, H., Nah, S., and Lee, K. M. (2017). “Enhanced deep residual networks for single image super-resolution,” in Proceedings of the IEEE conference on computer vision and pattern recognition workshops, 136–144. doi:10.48550/arXiv.1707.02921
Lin, X., He, J., Chen, Z., Lyu, Z., Dai, B., Yu, F., et al. (2024). “Diffbir: toward blind image restoration with generative diffusion prior,” in European conference on computer vision. Cham: Springer, 430–448. doi:10.1007/978-3-031-73202-7_25
Liu, Z., Lin, Y., Cao, Y., Hu, H., Wei, Y., Zhang, Z., et al. (2021). “Swin transformer: hierarchical vision transformer using shifted windows,” in Proceedings of the IEEE/CVF international conference on computer vision, 10012–10022. doi:10.48550/arXiv.2103.14030
Pan, Z., Ma, W., Guo, J., and Lei, B. (2019). Super-resolution of single remote sensing image based on residual dense back projection networks. IEEE Trans. Geoscience Remote Sens. 57 (10), 7918–7933. doi:10.1109/TGRS.2019.2917427
Patnaik, A., Bhuyan, M. K., and MacDorman, K. F. (2024). A two-branch multiscale residual attention network for single image super-resolution in remote sensing imagery. IEEE J. Sel. Top. Appl. Earth Observations Remote Sens. 17, 6003–6013. doi:10.1109/JSTARS.2024.3371710
Rombach, R., Blattmann, A., Lorenz, D., Esser, P., and Ommer, B. (2022). “High-resolution image synthesis with latent diffusion models,” in Proceedings of the IEEE/CVF conference on computer vision and pattern recognition, 10684–10695. doi:10.48550/arXiv.2112.10752
Rossi, L., Bernuzzi, V., Fontanini, T., Bertozzi, M., and Prati, A. (2025). Swin2-MoSE: a new single image supersolution model for remote sensing. IET Image Process. 19 (1), e13303. doi:10.1049/ipr2.13303
Safarov, F., Khojamuratova, U., Komoliddin, M., Bolikulov, F., Muksimova, S., and Cho, Y.-I. (2025). MBGPIN: multi-branch generative prior integration network for super-resolution satellite imagery. Remote Sens. 17 (5), 805. doi:10.3390/rs17050805
Saharia, C., Ho, J., Chan, W., Salimans, T., Fleet, D. J., and Norouzi, M. (2022). Image super-resolution via iterative refinement. IEEE Trans. Pattern Analysis Mach. Intell. 45 (4), 4713–4726. doi:10.1109/TPAMI.2022.3204461
Salgueiro, L., Marcello, J., and Vilaplana, V. (2022). SEG-ESRGAN: a multi-task network for super-resolution and semantic segmentation of remote sensing images. Remote Sens. 14 (22), 5862. doi:10.3390/rs14225862
Salgueiro Romero, L., Marcello, J., and Vilaplana, V. (2020). Super-resolution of Sentinel-2 imagery using generative adversarial networks. Remote Sens. 12 (15), 2424. doi:10.3390/rs12152424
Shang, J., Gao, M., Li, Q., Pan, J., Zou, G., and Jeon, G. (2023). Hybrid-scale hierarchical transformer for remote sensing image super-resolution. Remote Sens. 15 (13), 3442. doi:10.3390/RS15133442
Sharifi, A., and Safari, M. M. (2025). Enhancing the spatial resolution of Sentinel-2 images through super-resolution using transformer-based deep-learning models. IEEE J. Sel. Top. Appl. Earth Observations Remote Sens. 18, 4805–4820. doi:10.1109/JSTARS.2025.3526260
Sharifuzzaman, S. A. S. M., Tanveer, J., Chen, Y., Chan, J. H., Kim, H. S., Kallu, K. D., et al. (2024). Bayes R-CNN: an uncertainty-aware bayesian approach to object detection in remote sensing imagery for enhanced scene interpretation. Remote Sens. 16 (13), 2405. doi:10.3390/rs16132405
Tu, J., Mei, G., Ma, Z., and Piccialli, F. (2022). SWCGAN: generative adversarial network combining swin transformer and CNN for remote sensing image super-resolution. IEEE J. Sel. Top. Appl. Earth Observations Remote Sens. 15, 5662–5673. doi:10.1109/JSTARS.2022.3190322
Wang, C., Mu, X., Yu, S., Liu, Y., Liu, J., Shi, H., et al. (2025). Super-resolution reconstruction of remote sensing images based on deep learning. Proc. Sixth Int. Conf. Geoscience Remote Sens. Map (GRSM 2024) 13506, 641–646. doi:10.1117/12.3057490
Wang, J., Yue, Z., Zhou, S., Chan, K. C. K., and Loy, C. C. (2024). Exploiting diffusion prior for real-world image super-resolution. Int. J. Comput. Vis. 132, 5929–5949. doi:10.1007/S11263-024-02168-7
Wang, S., Zhou, T., Lu, Y., and Di, H. (2021). Contextual transformation network for lightweight remote-sensing image super-resolution. IEEE Trans. Geoscience Remote Sens. 60, 1–13. doi:10.1109/TGRS.2021.3132093
Wang, Z., Li, L., Xue, Y., Jiang, C., Wang, J., Sun, K., et al. (2022a). FeNet: feature enhancement network for lightweight remote-sensing image super-resolution. IEEE Trans. Geoscience Remote Sens. 60, 1–12. doi:10.1109/TGRS.2022.3168787
Wang, Z., Zheng, H., He, P., Chen, W., and Zhou, M. (2022b). Diffusion-gan: training GANs with diffusion. arXiv Prepr. arXiv:2206.02262. doi:10.48550/arXiv.2206.02262
Yang, L., Zhang, Z., Song, Y., Hong, S., Xu, R., Zhao, Y., et al. (2023). Diffusion models: a comprehensive survey of methods and applications. ACM Comput. Surv. 56 (4), 1–39. doi:10.1145/3626235
Yang, T., Wu, R., Ren, P., Xie, X., and Zhang, L. (2024). “Pixel-aware stable diffusion for realistic image super-resolution and personalized stylization,” in European conference on computer vision. Cham: Springer, 74–91. doi:10.1007/978-3-031-73247-8_5
Yang, W., Feng, J., Yang, J., Zhao, F., Liu, J., Guo, Z., et al. (2017). Deep edge guided recurrent residual learning for image super-resolution. IEEE Trans. Image Process. 26 (12), 5895–5907. doi:10.1109/TIP.2017.2750403
Zhang, L., Rao, A., and Agrawala, M. (2023). “Adding conditional control to text-to-image diffusion models,” in Proceedings of the IEEE/CVF international conference on computer vision, 3836–3847. doi:10.48550/arXiv.2302.05543
Keywords: super-resolution, diffusion model, generative adversarial network, remote sensing image reconstruction, attention mechanism
Citation: Li R, Wen L, Shao S, Yu M and Mohaisen L (2025) A novel generative adversarial network framework for super-resolution reconstruction of remote sensing. Front. Earth Sci. 13:1578321. doi: 10.3389/feart.2025.1578321
Received: 17 February 2025; Accepted: 23 April 2025;
Published: 08 May 2025.
Edited by:
Zhiheng Liu, Xidian University, ChinaReviewed by:
Tariq Hussain, Zhejiang Gongshang University, ChinaRuba Al-Hussien, Jordan University of Science and Technology, Jordan
Jing Wang, Civil Aviation University of China, China
Zhongqiang Cui, Hebei GEO University, China
Mohamed Atef, University of Menoufia, Egypt
Copyright © 2025 Li, Wen, Shao, Yu and Mohaisen. This is an open-access article distributed under the terms of the Creative Commons Attribution License (CC BY). The use, distribution or reproduction in other forums is permitted, provided the original author(s) and the copyright owner(s) are credited and that the original publication in this journal is cited, in accordance with accepted academic practice. No use, distribution or reproduction is permitted which does not comply with these terms.
*Correspondence: Ming Yu, eXVtaW5nQG5lZnUuZWR1LmNu