- 1Department of Pathology, Microbiology and Forensic Medicine, School of Medicine, The University of Jordan, Amman, Jordan
- 2Department of Clinical Laboratories and Forensic Medicine, Jordan University Hospital, Amman, Jordan
- 3Department of Translational Medicine, Faculty of Medicine, Lund University, Malmö, Sweden
- 4Sheikh Jaber Al-Ahmad Al-Sabah Hospital, Ministry of Health, Kuwait City, Kuwait
- 5School of Medicine, The University of Jordan, Amman, Jordan
- 6School of Science, The University of Jordan, Amman, Jordan
- 7College of Administration and Economics, Al-Iraqia University, Baghdad, Iraq
- 8Computer Techniques Engineering Department, Baghdad College of Economic Sciences University, Baghdad, Iraq
- 9Department of Clinical Pharmacy, Faculty of Pharmacy, Al-Baha University, Al-Baha, Saudi Arabia
- 10Department of Clinical Pharmacy, The National Hepatology and Tropical Medicine Research Institute, Cairo, Egypt
- 11Department of Business Technology, Al-Ahliyya Amman University, Amman, Jordan
Background: In the recent generative artificial intelligence (genAI) era, health sciences students (HSSs) are expected to face challenges regarding their future roles in healthcare. This multinational cross-sectional study aimed to confirm the validity of the novel FAME scale examining themes of Fear, Anxiety, Mistrust, and Ethical issues about genAI. The study also explored the extent of apprehension among HSSs regarding genAI integration into their future careers.
Methods: The study was based on a self-administered online questionnaire distributed using convenience sampling. The survey instrument was based on the FAME scale, while the apprehension toward genAI was assessed through a modified scale based on State-Trait Anxiety Inventory (STAI). Exploratory and confirmatory factor analyses were used to confirm the construct validity of the FAME scale.
Results: The final sample comprised 587 students mostly from Jordan (31.3%), Egypt (17.9%), Iraq (17.2%), Kuwait (14.7%), and Saudi Arabia (13.5%). Participants included students studying medicine (35.8%), pharmacy (34.2%), nursing (10.7%), dentistry (9.5%), medical laboratory (6.3%), and rehabilitation (3.4%). Factor analysis confirmed the validity and reliability of the FAME scale. Of the FAME scale constructs, Mistrust scored the highest, followed by Ethics. The participants showed a generally neutral apprehension toward genAI, with a mean score of 9.23 ± 3.60. In multivariate analysis, significant variations in genAI apprehension were observed based on previous ChatGPT use, faculty, and nationality, with pharmacy and medical laboratory students expressing the highest level of genAI apprehension, and Kuwaiti students the lowest. Previous use of ChatGPT was correlated with lower apprehension levels. Of the FAME constructs, higher agreement with the Fear, Anxiety, and Ethics constructs showed statistically significant associations with genAI apprehension.
Conclusion: The study revealed notable apprehension about genAI among Arab HSSs, which highlights the need for educational curricula that blend technological proficiency with ethical awareness. Educational strategies tailored to discipline and culture are needed to ensure job security and competitiveness for students in an AI-driven future.
1 Introduction
The adoption of generative artificial intelligence (genAI) into healthcare is inevitable with evidence pointing to its current wide applications in different healthcare settings (Yim et al., 2024). As genAI advances rapidly in its capabilities, it would fundamentally transform healthcare with subsequent revolution in operational efficiency with improved patient outcomes (Sallam, 2023; Verlingue et al., 2024; Sallam et al., 2025a). Nevertheless, the integration of genAI into healthcare practices is expected to introduce formidable challenges (Dave et al., 2023; Sallam, 2023). Central to these challenges is the expected profound implications on the structure and composition of the workforce in healthcare (Daniyal et al., 2024; Rony et al., 2024b).
On a positive note, the potential of genAI to streamline workflow in healthcare settings is hard to dispute (Mese et al., 2023; Fathima and Moulana, 2024). As stated in a commentary by Bongurala et al. (2024), AI assistants can decrease documentation time for healthcare professionals (HCPs) by as much as 70% which would enable a greater focus on direct patient care. To be more specific with examples, the improved efficiency provided by genAI can be achieved through automated transcription of patient encounters, data entry into electronic health records (EHRs), and improved patient communication as illustrated by Small et al. (2024), Tai-Seale et al. (2024), Badawy et al. (2025) and Sallam et al. (2025b).
On the other hand, alongside the aforementioned opportunities, genAI introduces complex challenges in healthcare where even minor errors can lead to grave consequences (Panagioti et al., 2019; Gupta et al., 2025). An urgent concern of genAI integration into healthcare is the fear of job displacement (Christian et al., 2024; Rony et al., 2024b; Sallam et al., 2024a). As genAI abilities to handles routine and complex tasks in healthcare is realized, the demand for human intervention may diminish, prompting shifts in job roles or even losses (Rawashdeh, 2023; Ramarajan et al., 2024). However, this genAI anticipated impact is not uniform and it could vary across healthcare specialties and cultural contexts. This variability demands careful study to identify determinants of attitude to genAI and devise strategies that maximize genAI benefits in healthcare while addressing critical concerns, including job security (Kim et al., 2025).
Research studies have already started to examine how health science students and HCPs perceive the genAI tools such as ChatGPT mostly in the context of Technology Acceptance Model (TAM) (Sallam et al., 2023; Abdaljaleel et al., 2024; Chen S.Y. et al., 2024). In the context of concerns of possible job displacement, (Rony et al., 2024b) reported that HCPs in Bangladesh expressed concerns about AI undermining roles traditionally occupied by humans. Their analysis highlighted several concerns such as threats to job security, moral questions regarding AI-driven decisions, impacts on patient-HCP relationships, and ethical challenges in automated care (Rony et al., 2024b). In Jordan, a study among medical students developed and validated the FAME scale to measure Fear, Anxiety, Mistrust, and Ethical concerns associated with genAI (Sallam et al., 2024a). This study revealed a range of concerns among medical students, highlighting notable apprehension regarding the impact of genAI on their future careers as physicians (Sallam et al., 2024a). Notably, mistrust and ethical issues predominated over fear and anxiety, illustrating the complicated emotional and cognitive reactions that are elicited by this inevitable novel technology (Sallam et al., 2024a).
From a broader perspective, Nicholas Caporusso introduced the term “Creative Displacement Anxiety” (CDA) to define a psychological state triggered by the perceived or actual infiltration of genAI on areas that required human creativity (Caporusso, 2023). The CDA reflects a complex range of emotional, cognitive, and behavioral responses to the expanding roles of genAI in areas traditionally dependent on human creativity (Caporusso, 2023). Caporusso argued that a thorough understanding genAI and its adoption could alleviate its negative psychological impacts, advocating for proactive engagement with this transformative technology (Caporusso, 2023).
Extending on the previous research on genAI apprehension in the context of healthcare, our study broadens the FAME scale’s validation to a diverse, multinational sample of health sciences students in order to offer a more comprehensive understanding of attitude to genAI in healthcare. Key to our inquiry was the delineation of “Apprehension” as a distinct state of reflective unease that differs fundamentally from the immediate, visceral responses associated with fear or anxiety based on Grillon (2008). Herein, Apprehension was defined as a measure to reflect the awareness and cautious consideration of genAI’s future implications rather than acute, present-focused threats.
Thus, our study objectives involved the assessment of student apprehension toward genAI integration in healthcare settings, with confirmatory validation of the FAME scale to ensure its reliability in measuring anxiety, fear, mistrust, and ethical concerns. Specifically, our study addressed the following major questions: First, what is the degree of apprehension toward genAI among health sciences students across various disciplines, including medicine, dentistry, pharmacy, nursing, rehabilitation, and medical laboratory sciences? Second, does the FAME scale effectively capture and measure the specific determinants underlying this apprehension? Finally, which demographic variables and FAME constructs are significantly associated with apprehension toward genAI among health students in Arab countries?
2 Methods
2.1 Study settings and participants
This study utilized a cross-sectional survey design targeting health sciences students, spanning fields of medicine, dentistry, pharmacy/doctor of pharmacy, nursing, rehabilitation, and medical laboratory sciences. The study group comprised students of Arab nationality enrolled in universities across the Arab region, as outlined in the survey’s introductory section.
Recruitment of the potential participants was based on snowball sampling convenient approach as outlined by Leighton et al. (2021). This approach depended on widely-used social media and messaging platforms, including Facebook, X (formerly Twitter), Instagram, LinkedIn, Messenger, and WhatsApp, starting with the authors’ networks across Egypt, Iraq, Jordan, Kuwait, and Saudi Arabia and encouraging further survey dissemination. Data collection started on October 27 and ended on November 5, 2024.
Adhering to the Declaration of Helsinki, the ethical approval was granted by the Institutional Review Board (IRB) at the Deanship of Scientific Research at Al-Ahliyya Amman University, Jordan. Participation was voluntary without monetary incentives, and all respondents provided electronic informed consent following an introduction of the survey that detailed study aims, procedures, and confidentiality issues.
Hosted on SurveyMonkey (SurveyMonkey Inc., San Mateo, CA, USA) in both Arabic and English, the survey access was limited to a single response per IP address to ensure data reliability. All items required mandatory responses for study inclusion, with rigorous quality checks to ensure data integrity. A minimum response time of 120 s was set, guided by a median pre-filtration response time of 222.5 s and a 5th percentile benchmark of 111.85 s. Additionally, responses were screened for contradictions: participants who selected “none” for genAI model use but indicated the use of specific genAI models were excluded for inconsistency.
Our study design adhered to Exploratory Factor Analysis (EFA) and Confirmatory Factor Analysis (CFA) guidelines which suggest a minimum of 200 participants for sufficient statistical power (Mundfrom et al., 2005). Considering the multinational scope, we targeted over 500 participants to robustly estimate apprehension to genAI across diverse populations.
2.2 Details of the survey instrument
Following informed consent, the survey began with demographic data collection including the following variables: age, sex, faculty, nationality, university location, institution type (public vs. private), and the latest grade point average (GPA). The second section inquired about the prior use of genAI, frequency of use, and the self-rated competency in using genAI tools.
The primary outcome measure in the study was “Apprehension toward genAI” entailing assessment of the anticipatory unease about genAI’s future impact on healthcare. Apprehension was assessed through three items adapted and modified from the State-Trait Anxiety Inventory (STAI) (Spielberger et al., 1971; Spielberger and Reheiser, 2004). These items were: (1) I feel tense when thinking about the impact of generative AI like ChatGPT on my future in healthcare; (2) The idea of generative AI taking over aspects of patient care makes me nervous; and (3) I feel uneasy when I hear about new advances in generative AI for healthcare. The three items were assessed on a 5-point Likert scale from “agree,” “somewhat agree,” “neutral,” “somewhat disagree,” to “disagree.” Finally, the validated 12-item FAME scale was administered (Sallam et al., 2024a), measuring Fear, Anxiety, Mistrust, and Ethics, with each construct represented by three items rated on a 5-point Likert scale from “agree” to “disagree.” The full questionnaire is provided in Supplementary S1.
2.3 Statistical and data analysis
In the statistical and data analyses, IBM SPSS Statistics for Windows, Version 27.0, Armonk, NY: IBM Corp and JASP software (Version 0.19.0) were used (Jasp Team, 2024). Each construct score—Apprehension, Fear, Anxiety, Mistrust, and Ethics—was calculated by summing responses to the corresponding three items, where “agree” was assigned a score of 5, and “disagree” a score of 1, yielding higher scores for stronger agreement with each construct.
Data normality for these 5 scale variables was assessed via the Kolmogorov–Smirnov test, justifying subsequent use of the non-parametric tests (Mann Whitney U test [M-W] and Kruskal Wallis test [K-W]) for univariate associations based on non-normality of the five scales (p < 0.001 for all). Spearman’s rank-order correlation was used to assess the correlation between two scale variables by measuring the Spearman’s rank correlation coefficient (ρ).
In examining predictors of apprehension toward genAI, univariate analyses identified candidate variables for inclusion in multivariate analysis based on the p value threshold of 0.100. Analysis of Variance (ANOVA) was employed to confirm the linear regression model validity with multicollinearity diagnostics using the Variance Inflation Factor (VIF) to flag any potential multicollinearity issues, with VIF threshold of >5 (Kim, 2019). Statistical significance for all analyses was set at p < 0.050.
To validate the structure of the FAME scale, EFA was conducted with maximum likelihood estimation and Oblimin rotation and sampling adequacy checked through the Kaiser-Meyer-Olkin (KMO) measure, while the factorability was confirmed by Bartlett’s test of sphericity. Subsequent CFA was performed to confirm the FAME scale latent factor structure. Fit indices, including the Root Mean Square Error of Approximation (RMSEA), Standardized Root Mean Square Residual (SRMR), Goodness of Fit Index (GFI), and the Tucker-Lewis Index (TLI) were employed to evaluate model fit. Internal consistency across survey constructs was evaluated using Cronbach’s α, with a threshold of α ≥ 0.60 considered acceptable for reliability (Tavakol and Dennick, 2011; Taber, 2018).
3 Results
3.1 Description of the study sample following quality checks
As indicated in Figure 1, the final study sample comprised 587 students representing 72.6% of the participants who consented to participate and met the quality check criteria.
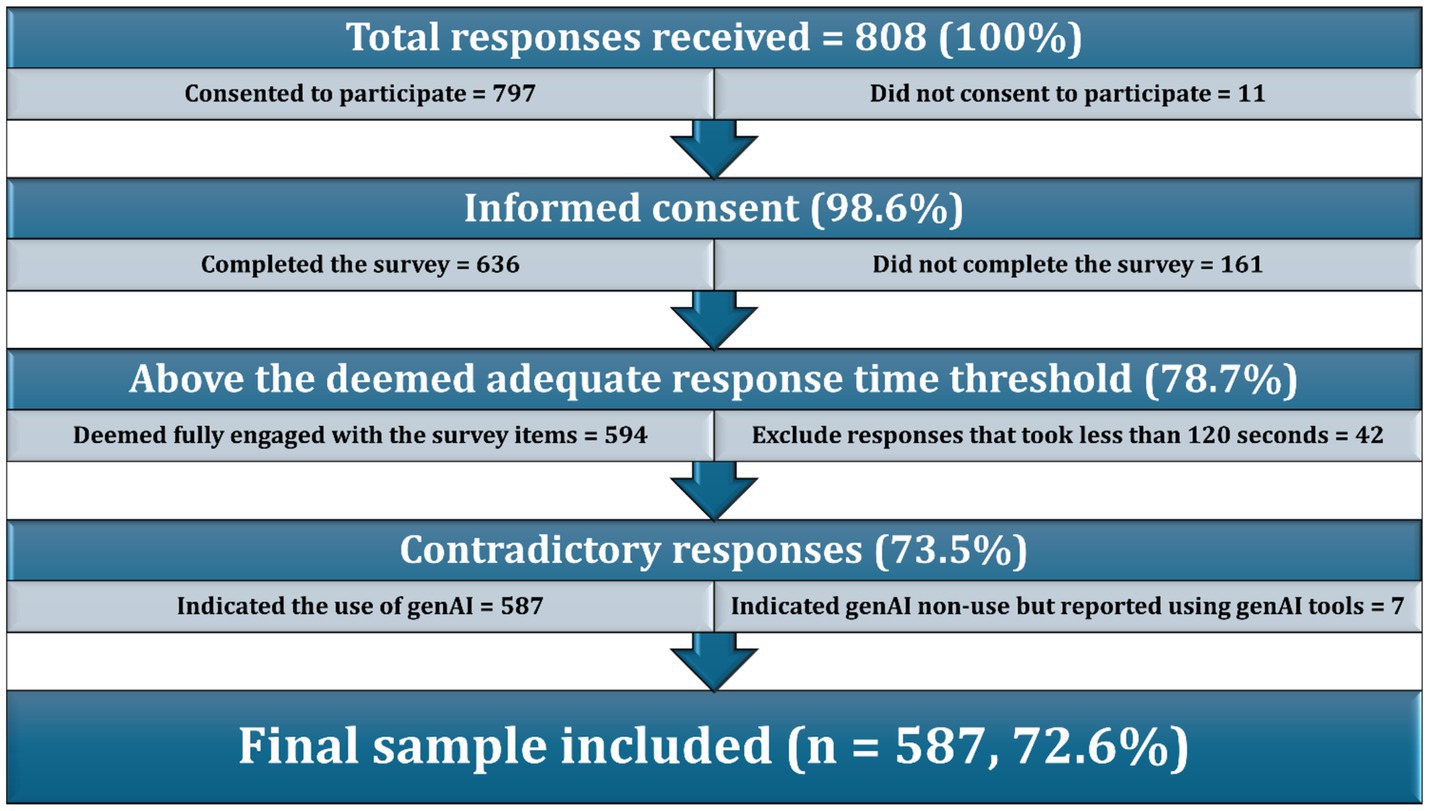
Figure 1. Flowchart of quality control for final study sample selection. genAI, generative artificial intelligence.
The final sample primarily consisted of students under 25 years (92.7%) and females (72.9%). Medicine (35.8%) and Pharmacy/PharmD (34.2%) were the most represented faculties. The most common nationality was Jordanian (31.3%), and a slight majority of participants were studying in Jordan (51.3%), with most attending public universities (59.1%). A significant portion indicated high academic performance, with 67.1% reporting either excellent or very good latest GPAs. Generative AI use was widespread, with 80.4% indicating previous use of ChatGPT, although other genAI tools were used less frequently. Regular genAI engagement was common, and 55.9% of participants reported being either competent or very competent (Table 1).
3.2 Confirmatory factor analysis of the FAME scale
The CFA for the FAME scale showed a good model fit across several fit indices. The chi-square difference test revealed a statistically significant model fit improvement for the hypothesized factor structure (χ2(48) = 194.455, p < 0.001) compared to the baseline model (χ2(66) = 4315.983), which suggested that the four-factor model captured the structure of the data. The CFI was 0.966 and the TLI was 0.953, both of which indicated a good model fit while the RMSEA was 0.072 indicating an acceptable model fit.
Bartlett’s test of sphericity (χ2(66) = 4,273.092, p < 0.001) and the KMO measure of sampling adequacy (0.872 overall) indicated that the data were appropriate for factor analysis (Table 2).
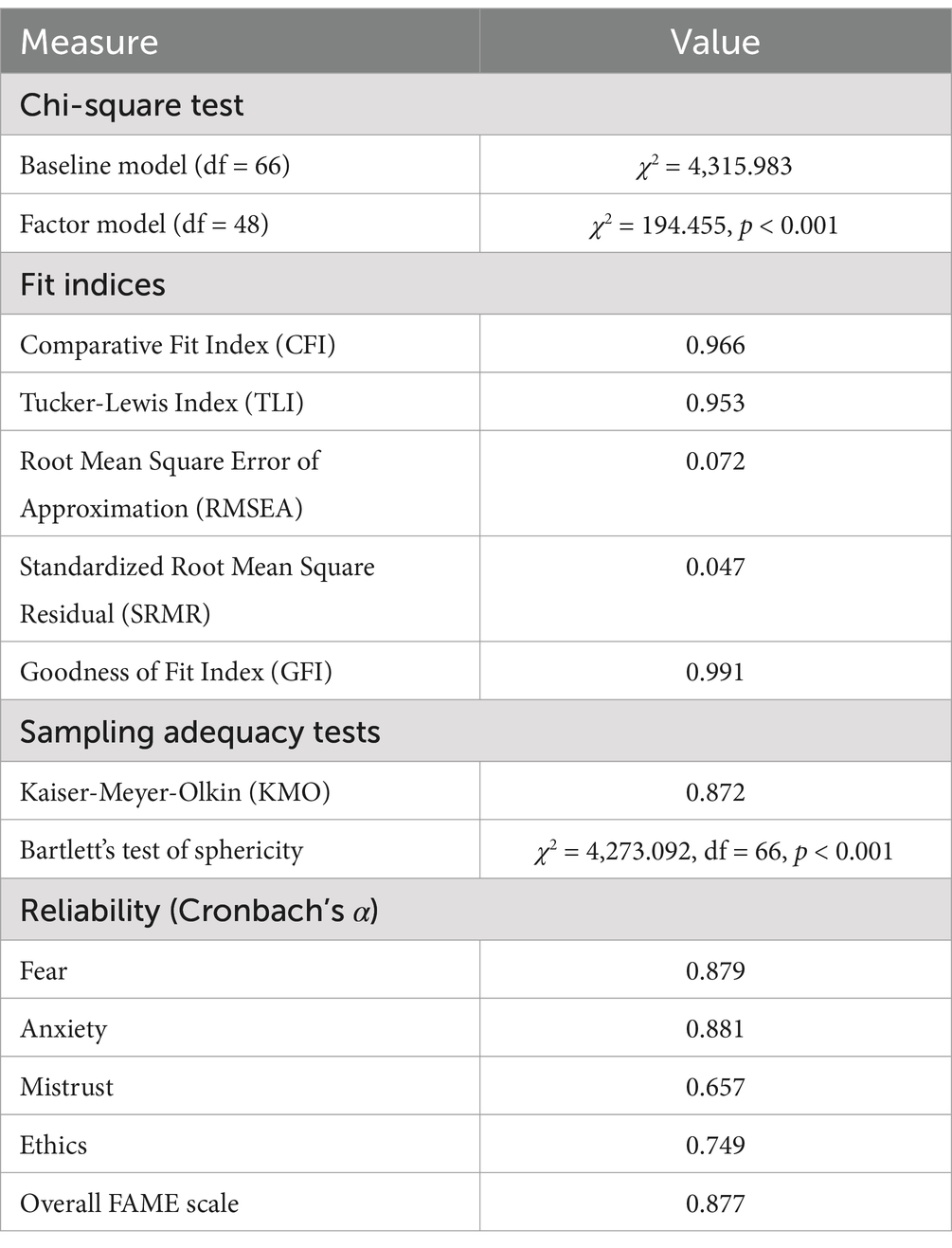
Table 2. Confirmatory factor analysis fit indices, reliability, and sampling adequacy of the FAME scale.
Figure 2 presents the CFA model for the FAME scale, evaluating constructs related to Fear, Anxiety, Mistrust, and Ethics as factors influencing health science students’ perceptions of genAI in healthcare.
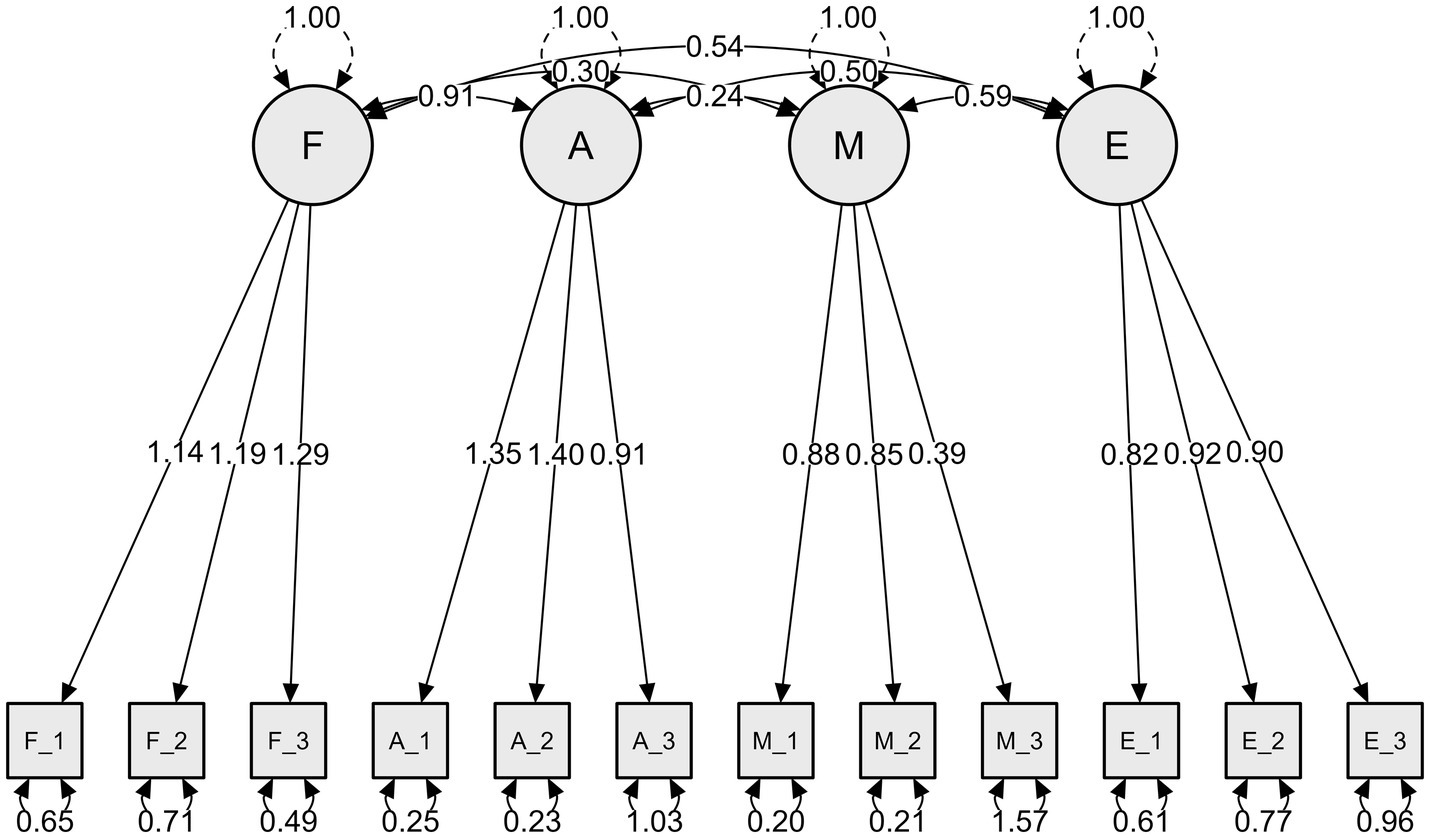
Figure 2. Confirmatory factor analysis (CFA) model of the FAME scale. F, Fear; A, Anxiety; M, Mistrust; E, Ethics.
Each factor demonstrated strong factor loadings for its respective indicators, suggesting adequate construct validity within the model. Factor loadings ranged from 0.65 to 1.40 across items, indicating robust relationships between observed variables and their underlying latent constructs.
The inter-factor correlations revealed significant relationships between Fear and Anxiety (0.30), Fear and Mistrust (0.24), Anxiety and Mistrust (0.50), and Anxiety and Ethics (0.54), while Mistrust and Ethics showed a correlation of 0.59. The results highlighted the structural validity of the FAME scale, suggesting that Fear, Anxiety, Mistrust, and Ethics can be reliably measured as distinct yet related factors in understanding health students’ attitude toward genAI role in healthcare.
3.3 Apprehension to genAI in the study sample
Apprehension toward genAI, as measured by a 3-item scale that showed an acceptable internal consistency with a Cronbach’s α of 0.850, yielded a mean score of 9.23 ± 3.60, indicating a neutral attitude with a tendency toward agreement.
Significant variations in apprehension were observed across several study variables. Faculty showed the highest apprehension in Medical Laboratory (11.08 ± 3.29) and Pharmacy/Doctor of Pharmacy (10.11 ± 3.49) students, contrasting with lower scores in Medicine (8.00 ± 3.33; p < 0.001, Figure 3).
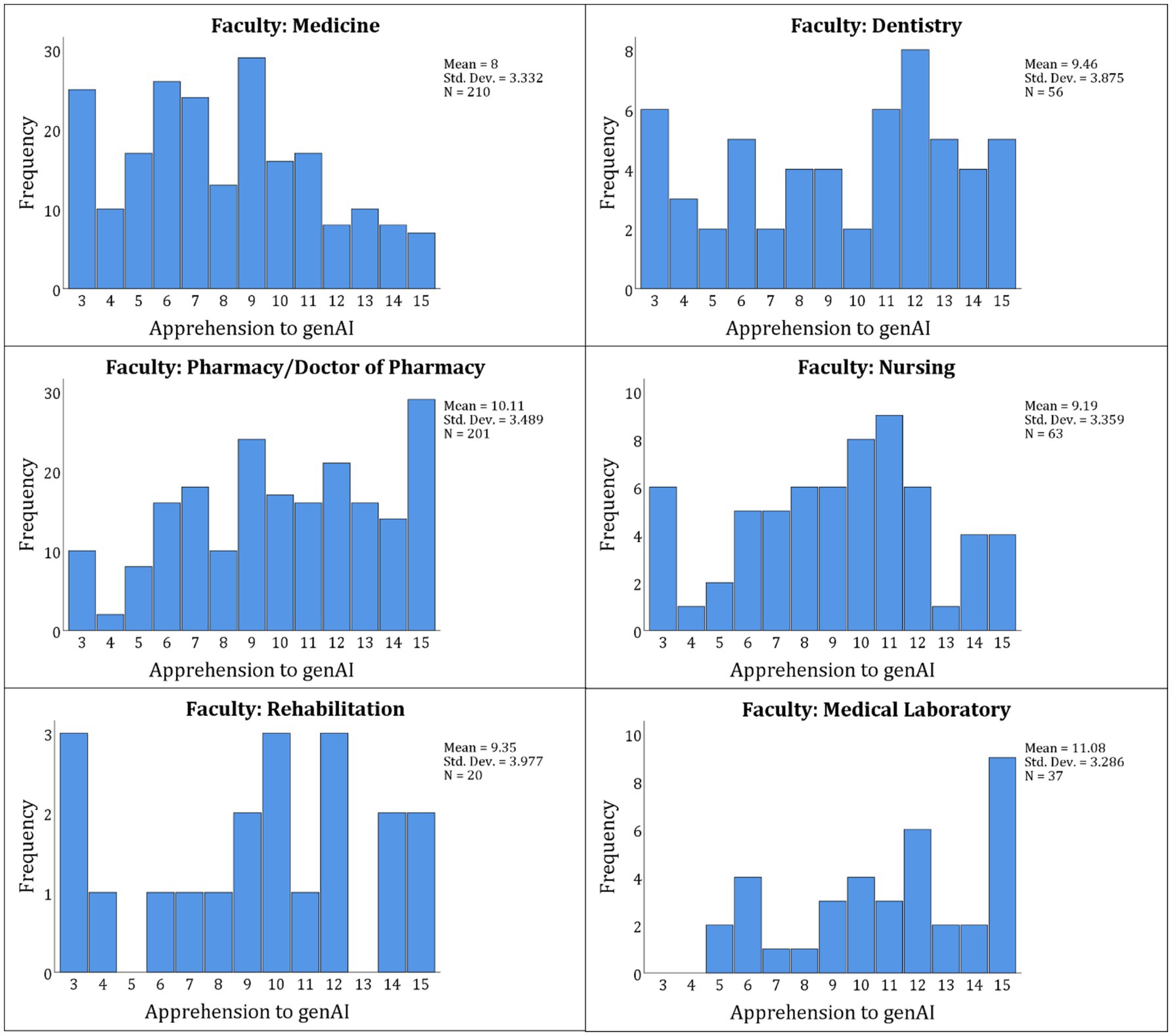
Figure 3. The distribution of apprehension to genAI in the study sample stratified per faculty. genAI, generative Artificial Intelligence.
Kuwaiti students had the lowest apprehension (7.92 ± 3.46; p = 0.006), with students studying in Kuwait also reporting a lower apprehension (7.21 ± 3.48; p = 0.004). Public university students exhibited less apprehension (8.61 ± 3.55) than those in private universities (10.13 ± 3.47; p < 0.001).
Previous ChatGPT users reported lower apprehension (8.94 ± 3.5) than non-users (10.43 ± 3.75; p < 0.001), and daily users of genAI had lower apprehension (8.16 ± 3.49) compared to less frequent users (p < 0.001). Competency in genAI use was inversely related to apprehension, with “not competent” individuals scoring higher (10.9 ± 3.66) than those self-rated as “very competent” (8.63 ± 3.66; p = 0.006, Table 3).
3.4 The FAME scale scores in the study sample
The mean scores for the FAME constructs indicated varying distribution with Mistrust scoring the highest at 12.46 ± 2.54, followed by Ethics at 11.10 ± 3.06, Fear at 9.96 ± 3.88, and Anxiety at 9.18 ± 3.85 (Figure 4).
A Spearman’s rank-order correlation was conducted to assess the relationship between apprehension toward genAI and the FEAR four constructs. The analysis revealed a statistically significant positive correlation between the Fear and apprehension constructs, ρ = 0.653, p < 0.001; the Anxiety and apprehension constructs, ρ = 0.638, p < 0.001; a weak yet statistically significant positive correlation with the Mistrust score, ρ = 0.100; p = 0.016, a moderate, statistically significant positive correlation with the Ethics construct, ρ = 0.440, <0.001 (Figure 5).
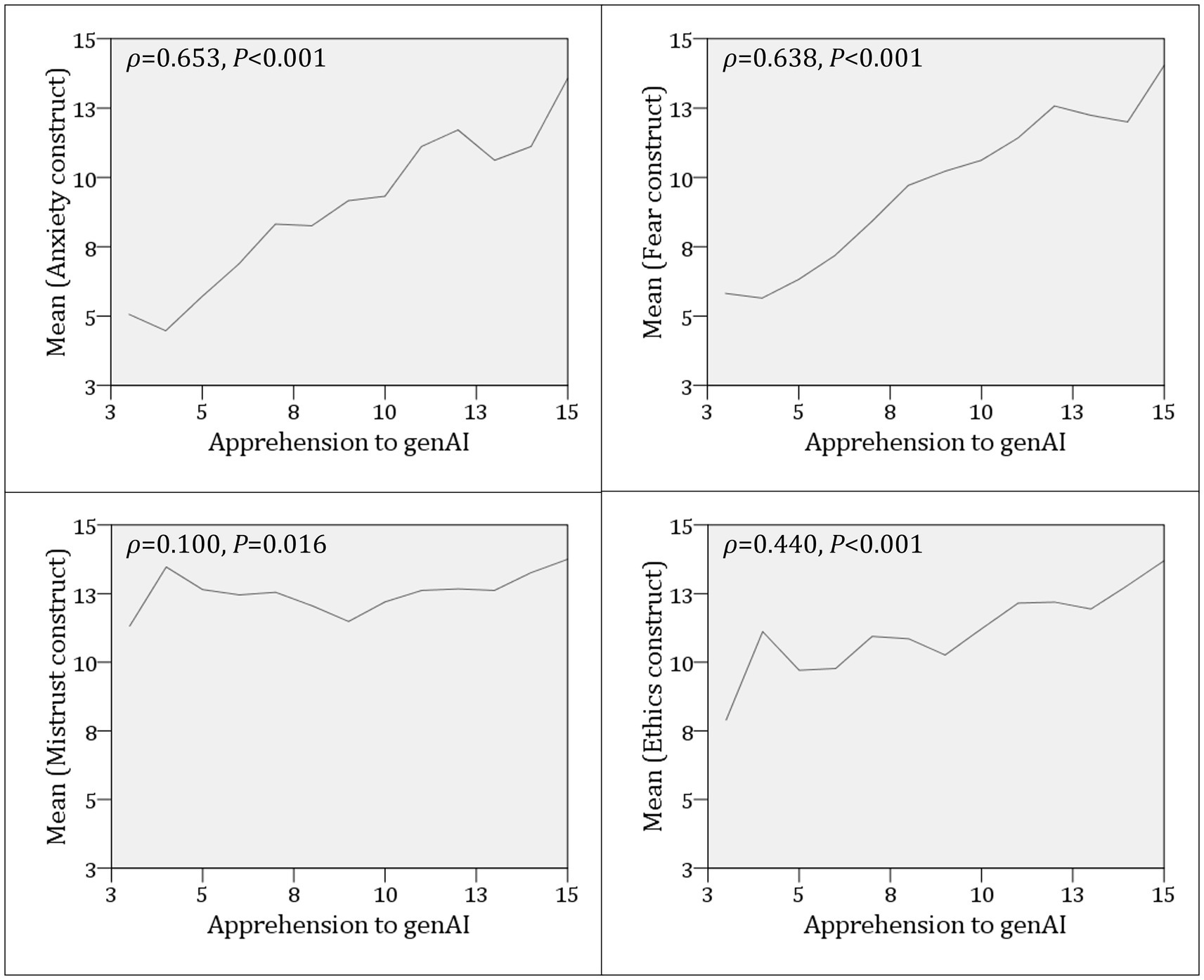
Figure 5. The correlation between the apprehension to genAI scores and the four FAME constructs scores. genAI, generative artificial intelligence; ρ, Spearman’s rank correlation coefficient.
3.5 Multivariate analysis for the factors associated with apprehension to genAI
The regression analysis explained a substantial variance, with R2 of 0.511, indicating that 51.1% of the variance in apprehension toward genAI was accounted for by the included predictors in the model. The regression model demonstrated statistical significance with an F-value of 54.720 and a p < 0.001 by ANOVA confirming that the whole model was a significant predictor of apprehension toward genAI.
The regression model examining predictors of apprehension toward genAI showed that faculty affiliation (B = 0.209, p = 0.010) and ChatGPT non-use prior to the study (B = −0.635, p = 0.027) were both significantly associated with apprehension, with faculty having a positive effect and non-ChatGPT use having a negative effect.
Nationality (B = −0.180, p = 0.034) and the country where the university is located (B = 0.183, p = 0.036) also demonstrated significant associations with apprehension levels. Among the psychological constructs, Fear (B = 0.302, p < 0.001), Anxiety (B = 0.251, p < 0.001), and Ethics (B = 0.212, p < 0.001) all showed strong positive associations with apprehension, suggesting that higher agreement with these constructs were linked with greater apprehension toward genAI (Table 4). In terms of multicollinearity, the VIF values indicated no severe multicollinearity concerns, as all are below 5. However, the Fear (VIF = 3.105) and Anxiety constructs (VIF = 3.118) were higher relative to other variables, suggesting moderate correlation with other predictors.
4 Discussion
In our study, we investigated the apprehension toward genAI models among health sciences students mainly in five Arab countries. The results pointed to a slight inclination toward apprehension about genAI, albeit the level of apprehension being close to neutral. Nevertheless, the level of genAI apprehension varied with notable disparities found in different demographic and educational contexts (e.g., nationality, faculty). The results suggested that while the participating students were not overwhelmingly apprehensive regarding genAI, they did harbor some apprehension about the implications of genAI in their future careers. This was manifested as a cautious acceptance of genAI rather than outright enthusiasm or rejection for this novel and inevitable technology.
The validity of our results is supported by the following factors. First, the rigorous quality check for responses received included ensuring the receipt of a single response per IP address, checking for contradictory responses, and setting a threshold for acceptable time to complete the survey to avoid common potential caveats in survey studies as listed by Nur et al. (2024). Second, the robust statistical analyses including EFA and CFA conducted helped to confirm the structural reliability of the FAME scale utilized in our assessment. Third, the diverse study sample primarily involving five different Arab countries provided acceptable credibility and generalizability to the study findings.
In this study, a substantial majority of the participants (87.4%) reported using at least one genAI tool, with a predominant use of ChatGPT by 80.4% of respondents. This result could highlight a trend hinting to the normalization of genAI tools’ use among health sciences students in Arab countries. In turn, this could reflect a broader genAI acceptance and integration into the students’ academic and potential professional careers.
The widespread use of ChatGPT specifically hints to its dominant presence and popularity compared to other genAI tools. As shown by the results of this study, lesser engagement with other genAI tools such as My AI On Snapchat (16.4%), Copilot (12.9%), and Gemini (10.6%) may indicate a disparity in functionality, user experience, or perhaps availability of different genAI tools, which suggests the ChatGPT position as the pioneering genAI tool. The pattern of genAI tool preference aligned with findings from other regional studies, such as that conducted by Sallam et al. (2024a), which also noted a variability of genAI use among medical students in Jordan, with ChatGPT leading significantly.
The dominant use of genAI tools, particularly ChatGPT, among university students, which was revealed in our study, hints to an emerging norm among university students in Arab countries as also shown in a recent study in the United Arab Emirates (Sallam et al., 2024b). This finding was reported internationally, as evidenced by Ibrahim et al. (2023) in a large multinational study that was conducted in Brazil, India, Japan, the United Kingdom, and the United States. The aforementioned study highlighted a strong tendency among students to employ ChatGPT in university assignments as shown in other studies as well (Ibrahim et al., 2023; Strzelecki, 2023; Mansour and Wong, 2024; Strzelecki, 2024). Taken together, the observed rise of genAI models’ use in higher education demands an immediate and thorough examination by educational institutions and educators alike (Masters et al., 2025).
Specifically, this scrutiny must assess how genAI models could influence learning outcomes and academic integrity as reported in a recent scoping review by Xia et al. (2024). Such an evaluation is essential to ensure that the integration of genAI models in higher education does not compromise the foundational principles of educational fairness and integrity, but rather enhances them, maintaining a balance between innovation and traditional academic values (Yusuf et al., 2024).
The major finding of our study was the demonstration of a mean apprehension score of 9.23 regarding genAI among health sciences students in Arab countries. This result suggests a level of readiness among those future HCPs to engage with genAI tools, albeit with an underlying caution. Particularly pronounced was the Mistrust expressed in the FAME scale, where the Mistrust construct achieved the highest mean of 12.46 of the four constructs. This high score denoted an agreement among the participating students on the view of genAI inability to replicate essential human attributes required in healthcare such as empathy and personal insight. Such skepticism likely derives from concerns that genAI, for all its analytical capabilities, cannot fulfill the demands of empathetic patient care, which remains a cornerstone of high-quality healthcare and patients’ satisfaction as shown by Moya-Salazar et al. (2023). Nevertheless, this view has already been refuted in several studies that showed the empathetic capabilities of genAI at least to an acceptable extent (Ayers et al., 2023; Chen D. et al., 2024; Hindelang et al., 2024).
Additionally, ethical concerns among the participating students in this study were notable. This was illustrated by a mean score for the Ethics construct of 11.10, highlighting the anticipated ethical ramifications of genAI deployment in healthcare which were extensively investigated in recent literature (Oniani et al., 2023; Sallam, 2023; Wang et al., 2023; Haltaufderheide and Ranisch, 2024; Ning et al., 2024). In this study, the students voiced substantial concerns over potential ethical breaches, including fears of compromised patient privacy and exacerbated healthcare inequities which are among the most feared and anticipated concerns of genAI use in healthcare (Khan et al., 2023). Thus, there is a necessity for robust ethical guidelines and regulatory frameworks to ensure that genAI applications are deployed responsibly, safeguarding both equity and confidentiality in patient care (Wang et al., 2023; Ning et al., 2024).
In this study, the Fear construct showed a mean score of 9.96. This result could signal a cautiously neutral yet discernibly fearful stance among health science students about the implications of genAI for job security and the relevance of human roles in the future healthcare. Such fear likely stems from concerns that genAI efficiency and accuracy could overshadow the human roles in healthcare. Subsequently, this can lead to job redundancies and a transformative shift in the professional healthcare settings. This result was in line with fears expressed in a recent studies among HCPs in Bangladesh (Rony et al., 2024a; Rony et al., 2024b). Additionally, the Anxiety construct, with a score of 9.18, may suggest that the traditional healthcare curricula may not be fully preparing health science students for an AI-driven healthcare settings in the near future (Gantwerker et al., 2020). This suggests an urgent need to bridge the gap between current educational programs and the futuristic demands of a technology-driven healthcare sector as reviewed by Charow et al. (2021).
The nuanced patterns of genAI apprehension identified in this study should not be interpreted in isolation. Rather, these observations likely reflect a confluence of contextual and demographic factors. These factors include the students’ academic backgrounds, levels of exposure to digital health technologies, and the broader socio-economic conditions surrounding healthcare education. The observed association between prior ChatGPT use and lower levels of genAI apprehension is particularly revealing. It suggests that familiarity with genAI tools can foster digital confidence, thereby reducing uncertainty and fear as shown in various contexts (Lambert et al., 2023; Abou Hashish and Alnajjar, 2024; Hur, 2025). In contrast, students with little or no exposure to such AI technologies may form their views based on unfamiliarity or secondhand perceptions, which can heighten skepticism as reported by García-Alonso et al. (2024). These insights highlight the importance of future research that moves beyond surface-level statistics to explore how educational, cultural, and psychological influences interact in shaping perceptions of genAI in healthcare education.
In regression analysis, the primary determinants of apprehension to genAI in this study included academic faculty, nationality, and the country in which the university is located. Additionally, statistically significant factors correlated with apprehension to genAI included the previous ChatGPT use and three out of the four constructs from the FAME scale namely Fear, Anxiety, and Ethics.
Specifically, the regression coefficients indicated distinct apprehension among pharmacy/doctor of pharmacy and medical laboratory students. This result could be seen as a rational response to the feared devaluation of the specialized skills and traditional roles of pharmacists and medical technologists by genAI (Chalasani et al., 2023). Additionally, the heightened apprehension toward genAI among pharmacy and medical laboratory students, relative to their peers in other health disciplines, can be attributed to the specific vulnerabilities of their fields to AI integration (Antonios et al., 2021; Hou et al., 2024). Pharmacy students may perceive a direct threat to their roles in medication management and patient counseling, as genAI promises to streamline treatment personalization, potentially diminishing the pharmacist involvement in direct patient care (Roosan et al., 2024).
Similarly, medical laboratory students face the prospect of AI automating complex diagnostic processes, potentially reducing their participation in critical decision-making and analytical reasoning (Dadzie Ephraim et al., 2024). On the other hand, medical students in this study showed a relatively lower apprehension toward genAI. This may stem from the perception that their roles involve a broader range of responsibilities and skills that are harder to automate and the many options of specialization they have. The practice of medicine involves complex decision-making, direct patient interactions, and nuanced clinical judgment, areas where AI is seen as a support tool rather than a replacement (Bragazzi and Garbarino, 2024). Nursing and dental students, like their medical counterparts in this study, exhibited relatively lower apprehension toward genAI likely due to the hands-on and interpersonal nature of their disciplines, which are perceived as less susceptible to automation.
An interesting result of the study was the variability in apprehension toward genAI among health sciences students from different Arab countries. Specifically, heightened apprehensions to genAI were found among student from Iraq, Jordan, and Egypt, contrasted with the significantly lower apprehension in Kuwait. This result can be explained through several socio-economic, educational, and cultural perspectives. Such an observation could potentially reflect a broader socio-economic uncertainties and disparities in technological integration within healthcare systems in Iraq, Jordan, and Egypt. These countries, while rich in educational history, face economic challenges that could affect the employment rates and resulting in healthcare resource constraints (Lai et al., 2016; Katoue et al., 2022). In such conditions, the introduction of genAI might be viewed more as a competitive threat than a supportive tool, exacerbating fears of job displacement amidst already competitive job markets (Kim et al., 2025).
The higher apprehension observed in these countries is likely compounded by concerns over the ethical use of AI in settings where regulatory frameworks might be perceived as underdeveloped or inadequately enforced. Conversely, Kuwaiti students’ lower levels of apprehension can be attributed to several factors. Economically more stable and with substantial investments in healthcare and education, Kuwait among other Gulf Cooperation Council (GCC) countries offers a more optimistic outlook on technological advancements (Shamsuddinova et al., 2024). Subsequently, the integration of genAI into healthcare would be seen as an enhancement to professional capabilities rather than a threat. Nevertheless, these cross-group differences warrant cautious interpretation. The current study did not adjust for potential confounding factors such as variation in educational curricula, differential exposure to genAI models, or culturally embedded attitudes toward automation in healthcare. In addition, the lack of measurement invariance testing precluded reaching definitive conclusions regarding the FAME scale performance across sub-groups. Thus, the observed differences in genAI apprehension may, in part, reflect measurement bias rather than genuine underlying perceptual divergence. Future studies employing qualitative or mixed-method designs are needed to more precisely delineate the contextual and cognitive factors underlying these variations in genAI apprehension.
Finally, the pronounced apprehension toward genAI among students exhibiting higher scores in the Fear, Anxiety, and Ethics constructs of the FAME scale, as well as among those who had not previously used ChatGPT should be dissected through a psychological perspective. Students scoring higher in Fear and Anxiety constructs likely perceive genAI not merely as a technological tool, but as a profound disruption. Fear often stems from the perceived threat of job displacement which is a sentiment deeply in-built in the collective psyche of individuals entering competitive fields like healthcare (Reichert et al., 2015; Kurniasari et al., 2020; Zirar et al., 2023).
Anxiety, closely tied to fear as reveled in factor analysis, might be amplified by the uncertainty of coping with rapidly evolving genAI technologies that could alter the whole healthcare future settings (Zirar et al., 2023). On the other hand, the higher scores in Ethics construct in association with higher genAI apprehension suggested the role of ethical implications of integrating genAI in healthcare. Based on the items included in the Ethics construct, the students were likely worried about patient privacy, the integrity of data handling by genAI, and the equitable distribution of AI-enhanced healthcare services which are plausible issue as discussed extensively in recent literature (Oniani et al., 2023; Bala et al., 2024; Ning et al., 2024; Williamson and Prybutok, 2024). The heightened apprehension among students who had not previously used ChatGPT before the study can be attributed to a lack of familiarity and understanding of genAI capabilities and limitations.
The study findings highlight the need for a systematic revision of the current healthcare curricula to address apprehensions about genAI and prepare future HCPs for careers soon to be heavily influenced by AI technologies (Tursunbayeva and Renkema, 2023). To address genAI apprehension and enhance proficiency, curricular developments should include AI literacy courses to explore AI functionalities and ethical dimensions, tailored to each healthcare discipline given the current lack of such curricular as revealed by Busch et al. (2024).
Ethics modules in healthcare education, specifically dealing with AI, should dissect real-world scenarios and ethical dilemmas (Naik et al., 2022). Additionally, the curriculum can encourage research and critical analysis projects that assess genAI impact on healthcare outcomes and patient satisfaction. Workshops aimed at hands-on training in genAI tools can help diminish fear of redundancy by illustrating how genAI augments rather than replaces human expertise (Giannakos et al., 2024). These initiatives can collectively culminate in successful incorporation of AI into educational frameworks, fostering a generation of HCPs who are both technically confident and ethically prepared.
The current study methodological rigor and multinational scope provided a strong foundation for its findings; nevertheless, despite its strengths, our study was not without limitations. First, the use of a cross-sectional survey design precluded the ability to establish causal relationships between the study variables, and longitudinal future studies are recommended to assess the trends of changing attitude to genAI and causality. Second, recruitment of the potential participants was based on a convenience and snowball sampling approach, which could have introduced bias by over-representing certain groups within the network of the initial participants and under-representing others outside of these networks. Third, although the total sample size was adequate for psychometric analyses, the distribution across countries was uneven, which could limit the interpretability of country-specific comparisons and reduce the cross-national generalizability of findings. Fourth, while the FAME scale demonstrated strong psychometric properties in our overall Arab sample, we did not conduct formal measurement invariance testing across countries or academic sub-groups. Thus, the observed differences in this study may reflect potential measurement bias rather than true variation in apprehension toward genAI. This underscores the need for future studies to evaluate configural, metric, and scalar invariance to ensure cross-group comparability. Finally, the study relied on self-reported data (e.g., latest GPA, genAI use, etc.), which can be subject to response biases such as social desirability or recall biases. While self-reporting is a practical and widely used approach in survey research (Demetriou et al., 2015), these limitations may affect the accuracy and consistency of the responses (Brenner and DeLamater, 2016).
To enhance the generalizability and contextual depth of future research, we recommend the adoption of stratified or probability-based sampling methods to ensure more representative and balanced participant recruitment across diverse academic and national contexts. Additionally, while the FAME scale offers a robust framework for quantifying genAI-related apprehension, future studies should consider complementing it with qualitative approaches or expanded item sets that capture the more nuanced psychological and contextual dimensions of fear, anxiety, and mistrust toward genAI in healthcare. These strategies will support a more comprehensive understanding of how educational and cultural factors would shape attitudes toward emerging technologies among future healthcare professionals.
5 Conclusion
In this multinational survey, Arab health sciences students exhibited a predominantly neutral yet cautiously optimistic attitude toward genAI, as evidenced by a mean apprehension score that leaned slightly toward agreement. This perception varied notably by discipline and nationality as pharmacy and medical laboratory students expressed the highest apprehension, likely due to the perceived potential disruption of genAI in their specialized fields. On the other hand, Kuwaiti students showed the lowest genAI apprehension, potentially reflecting national policies favoring technological adoption and integration into educational systems or underlying job security. Significant associations were found between apprehension and three constructs of the FAME scale—fear, anxiety, and ethics—highlighting deep-seated concerns that call for targeted educational strategies to address genAI apprehension. However, given the limitations in sampling methods and lack of measurement invariance testing, these cross-national differences should be interpreted with caution and regarded as exploratory. As genAI tools advance, it is crucial for healthcare education to evolve accordingly, ensuring that future HCPs are not only technologically proficient but also well-prepared to address ethical issues introduced by genAI. Integrating genAI into healthcare curricula must be done strategically and ethically, to prepare the students to effectively manage both the technological and ethical challenges posed by AI, thereby enhancing their readiness to address fears of job displacement and ethical dilemmas.
Data availability statement
The datasets presented in this study can be found in online repositories. The names of the repository/repositories and accession number(s) can be found in the article/Supplementary material.
Ethics statement
The studies involving humans were approved by The Institutional Review Board (IRB) of the Deanship of Scientific Research at Al-Ahliyya Amman University, Jordan. The studies were conducted in accordance with the local legislation and institutional requirements. The participants provided their written informed consent to participate in this study.
Author contributions
MaS: Conceptualization, Data curation, Formal analysis, Investigation, Methodology, Project administration, Resources, Software, Supervision, Validation, Visualization, Writing – original draft, Writing – review & editing. KA-M: Data curation, Investigation, Methodology, Writing – review & editing. HA: Data curation, Investigation, Methodology, Writing – review & editing. NAlb: Data curation, Investigation, Methodology, Writing – review & editing. ShA: Data curation, Investigation, Methodology, Writing – review & editing. FA: Data curation, Investigation, Methodology, Writing – review & editing. AA: Data curation, Investigation, Methodology, Writing – review & editing. SaA: Data curation, Investigation, Methodology, Writing – review & editing. NAlh: Data curation, Investigation, Methodology, Writing – review & editing. DA-Z: Data curation, Investigation, Methodology, Writing – review & editing. FS: Data curation, Investigation, Methodology, Writing – review & editing. MoS: Data curation, Investigation, Methodology, Writing – review & editing. AS: Data curation, Investigation, Methodology, Writing – review & editing. MM: Data curation, Investigation, Methodology, Writing – review & editing. DA: Data curation, Investigation, Methodology, Writing – review & editing. AA-A: Data curation, Investigation, Methodology, Supervision, Validation, Writing – review & editing.
Funding
The author(s) declare that no financial support was received for the research and/or publication of this article.
Conflict of interest
The authors declare that the research was conducted in the absence of any commercial or financial relationships that could be construed as a potential conflict of interest.
The author(s) declared that they were an editorial board member of Frontiers, at the time of submission. This had no impact on the peer review process and the final decision.
Generative AI statement
The authors declare that no Gen AI was used in the creation of this manuscript.
Publisher’s note
All claims expressed in this article are solely those of the authors and do not necessarily represent those of their affiliated organizations, or those of the publisher, the editors and the reviewers. Any product that may be evaluated in this article, or claim that may be made by its manufacturer, is not guaranteed or endorsed by the publisher.
Supplementary material
The Supplementary material for this article can be found online at: https://www.frontiersin.org/articles/10.3389/feduc.2025.1542769/full#supplementary-material
References
Abdaljaleel, M., Barakat, M., Alsanafi, M., Salim, N. A., Abazid, H., Malaeb, D., et al. (2024). A multinational study on the factors influencing university students' attitudes and usage of ChatGPT. Sci. Rep. 14:1983. doi: 10.1038/s41598-024-52549-8
Abou Hashish, E. A., and Alnajjar, H. (2024). Digital proficiency: assessing knowledge, attitudes, and skills in digital transformation, health literacy, and artificial intelligence among university nursing students. BMC Med. Educ. 24:508. doi: 10.1186/s12909-024-05482-3
Antonios, K., Croxatto, A., and Culbreath, K. (2021). Current state of laboratory automation in clinical microbiology laboratory. Clin. Chem. 68, 99–114. doi: 10.1093/clinchem/hvab242
Ayers, J. W., Poliak, A., Dredze, M., Leas, E. C., Zhu, Z., Kelley, J. B., et al. (2023). Comparing physician and artificial intelligence Chatbot responses to patient questions posted to a public social media forum. JAMA Intern. Med. 183, 589–596. doi: 10.1001/jamainternmed.2023.1838
Badawy, M. K., Khamwan, K., and Carrion, D. (2025). A pilot study of generative AI video for patient communication in radiology and nuclear medicine. Heal. Technol. 15, 395–404. doi: 10.1007/s12553-025-00945-z
Bala, I., Pindoo, I., Mijwil, M., Abotaleb, M., and Yundong, W. (2024). Ensuring security and privacy in healthcare systems: a review exploring challenges, solutions, future trends, and the practical applications of artificial intelligence. Jordan Med. J. 58. doi: 10.35516/jmj.v58i2.2527
Bongurala, A. R., Save, D., Virmani, A., and Kashyap, R. (2024). Transforming health care with artificial intelligence: redefining medical documentation. Mayo Clinic Proc. Digit. Health 2, 342–347. doi: 10.1016/j.mcpdig.2024.05.006
Bragazzi, N. L., and Garbarino, S. (2024). Toward clinical generative AI: conceptual framework. JMIR AI 3:e55957. doi: 10.2196/55957
Brenner, P. S., and DeLamater, J. (2016). Lies, damned lies, and survey self-reports? Identity as a cause of measurement Bias. Soc. Psychol. Q. 79, 333–354. doi: 10.1177/0190272516628298
Busch, F., Hoffmann, L., Truhn, D., Ortiz-Prado, E., Makowski, M. R., Bressem, K. K., et al. (2024). Global cross-sectional student survey on AI in medical, dental, and veterinary education and practice at 192 faculties. BMC Med. Educ. 24:1066. doi: 10.1186/s12909-024-06035-4
Caporusso, N. (2023). Generative artificial intelligence and the emergence of creative displacement anxiety: review. Res. Directs Psychol. Behav. 3. doi: 10.53520/rdpb2023.10795
Chalasani, S. H., Syed, J., Ramesh, M., Patil, V., and Pramod Kumar, T. M. (2023). Artificial intelligence in the field of pharmacy practice: a literature review. Explor. Res. Clin. Soc. Pharm. 12:100346. doi: 10.1016/j.rcsop.2023.100346
Charow, R., Jeyakumar, T., Younus, S., Dolatabadi, E., Salhia, M., Al-Mouaswas, D., et al. (2021). Artificial intelligence education programs for health care professionals: scoping review. JMIR Med. Educ. 7:e31043. doi: 10.2196/31043
Chen, S. Y., Kuo, H. Y., and Chang, S. H. (2024). Perceptions of ChatGPT in healthcare: usefulness, trust, and risk. Front. Public Health 12:1457131. doi: 10.3389/fpubh.2024.1457131
Chen, D., Parsa, R., Hope, A., Hannon, B., Mak, E., Eng, L., et al. (2024). Physician and artificial intelligence Chatbot responses to cancer questions from social media. JAMA Oncol. 10, 956–960. doi: 10.1001/jamaoncol.2024.0836
Christian, M, Pardede, R, Gularso, K, Wibowo, S, and Muzammil, OM. (2024). Sunarno evaluating AI-induced anxiety and technical blindness in healthcare professionals. In: 2024 3rd international conference on creative communication and innovative technology (ICCIT). pp. 1–6.
Dadzie Ephraim, R. K., Kotam, G. P., Duah, E., Ghartey, F. N., Mathebula, E. M., and Mashamba-Thompson, T. P. (2024). Application of medical artificial intelligence technology in sub-Saharan Africa: prospects for medical laboratories. Smart Health 33:100505. doi: 10.1016/j.smhl.2024.100505
Daniyal, M., Qureshi, M., Marzo, R. R., Aljuaid, M., and Shahid, D. (2024). Exploring clinical specialists’ perspectives on the future role of AI: evaluating replacement perceptions, benefits, and drawbacks. BMC Health Serv. Res. 24:587. doi: 10.1186/s12913-024-10928-x
Dave, T., Athaluri, S. A., and Singh, S. (2023). ChatGPT in medicine: an overview of its applications, advantages, limitations, future prospects, and ethical considerations. Front. Artif. Intell. 6:1169595. doi: 10.3389/frai.2023.1169595
Demetriou, C., Ozer, B. U., and Essau, C. A. (2015). “Self-report questionnaires” in The encyclopedia of clinical psychology. eds. R. L. Cautin and S. O. Lilienfeld (Wiley Online Library), 1–6.
Fathima, M., and Moulana, M. (2024). Revolutionizing breast cancer care: AI-enhanced diagnosis and patient history. Comput. Methods Biomech. Biomed. Eng. 28, 642–654. doi: 10.1080/10255842.2023.2300681
Gantwerker, E., Allen, L. M., and Hay, M. (2020). “Future of health professions education curricula” in Clinical education for the health professions: theory and practice. eds. D. Nestel, G. Reedy, L. McKenna, and S. Gough (Singapore: Springer Nature), 1–22.
García-Alonso, E. M., León-Mejía, A. C., Sánchez-Cabrero, R., and Guzmán-Ordaz, R. (2024). Training and technology acceptance of ChatGPT in university students of social sciences: a netcoincidental analysis. Behav. Sci. (Basel) 14:612. doi: 10.3390/bs14070612
Giannakos, M., Azevedo, R., Brusilovsky, P., Cukurova, M., Dimitriadis, Y., Hernandez-Leo, D., et al. (2024). The promise and challenges of generative AI in education. Behav. Inform. Technol., 1–27. doi: 10.1080/0144929X.2024.2394886
Grillon, C. (2008). Models and mechanisms of anxiety: evidence from startle studies. Psychopharmacology 199, 421–437. doi: 10.1007/s00213-007-1019-1
Gupta, R., Tiwari, S., and Chaudhary, P. (2025). “Generative AI techniques and models” in Generative AI: techniques, models and applications. eds. R. Gupta, S. Tiwari, and P. Chaudhary (Switzerland, Cham: Springer Nature), 45–64.
Haltaufderheide, J., and Ranisch, R. (2024). The ethics of ChatGPT in medicine and healthcare: a systematic review on large language models (LLMs). npj Digit. Med. 7:183. doi: 10.1038/s41746-024-01157-x
Hindelang, M., Sitaru, S., and Zink, A. (2024). Transforming health care through Chatbots for medical history-taking and future directions: comprehensive systematic review. JMIR Med. Inform. 12:e56628. doi: 10.2196/56628
Hou, H., Zhang, R., and Li, J. (2024). Artificial intelligence in the clinical laboratory. Clin. Chim. Acta 559:119724. doi: 10.1016/j.cca.2024.119724
Hur, J. W. (2025). Fostering AI literacy: overcoming concerns and nurturing confidence among preservice teachers. Inf. Learn. Sci. 126, 56–74. doi: 10.1108/ILS-11-2023-0170
Ibrahim, H., Liu, F., Asim, R., Battu, B., Benabderrahmane, S., Alhafni, B., et al. (2023). Perception, performance, and detectability of conversational artificial intelligence across 32 university courses. Sci. Rep. 13:12187. doi: 10.1038/s41598-023-38964-3
Jasp Team (2024). JASP (Version 0.19.0) [Computer Software]. Available online at: https://jasp-stats.org/ (Accessed November 9, 2024).
Katoue, M. G., Cerda, A. A., García, L. Y., and Jakovljevic, M. (2022). Healthcare system development in the Middle East and North Africa region: challenges, endeavors and prospective opportunities. Front. Public Health 10:1045739. doi: 10.3389/fpubh.2022.1045739
Khan, B., Fatima, H., Qureshi, A., Kumar, S., Hanan, A., Hussain, J., et al. (2023). Drawbacks of artificial intelligence and their potential solutions in the healthcare sector. Biomed. Mater. Devices, 1–8. doi: 10.1007/s44174-023-00063-2
Kim, J. H. (2019). Multicollinearity and misleading statistical results. Korean J. Anesthesiol. 72, 558–569. doi: 10.4097/kja.19087
Kim, J. J. H., Soh, J., Kadkol, S., Solomon, I., Yeh, H., Srivatsa, A. V., et al. (2025). AI anxiety: a comprehensive analysis of psychological factors and interventions. AI Ethics. doi: 10.1007/s43681-025-00686-9
Kurniasari, L., Suhariadi, F., and Handoyo, S. (2020). Are young physicians have problem in job insecurity? The impact of health systems' changes. J. Educ. Health Commun. Psychol. 9. doi: 10.12928/jehcp.v9i1.15660
Lai, Y, Ahmad, A, and Wan, CD (2016) Higher education in the Middle East and North Africa: exploring regional and country specific potentials.
Lambert, S. I., Madi, M., Sopka, S., Lenes, A., Stange, H., Buszello, C. P., et al. (2023). An integrative review on the acceptance of artificial intelligence among healthcare professionals in hospitals. NPJ Digit. Med. 6:111. doi: 10.1038/s41746-023-00852-5
Leighton, K., Kardong-Edgren, S., Schneidereith, T., and Foisy-Doll, C. (2021). Using social media and snowball sampling as an alternative recruitment strategy for research. Clin. Simul. Nurs. 55, 37–42. doi: 10.1016/j.ecns.2021.03.006
Mansour, T., and Wong, J. (2024). Enhancing fieldwork readiness in occupational therapy students with generative AI. Front. Med. (Lausanne) 11:1485325. doi: 10.3389/fmed.2024.1485325
Masters, K., Heather, M., Jennifer, B., Tamara, C., Kataryna, N., Sofia, V.-A., et al. (2025). Artificial intelligence in health professions education assessment: AMEE guide no. 178. Med. Teach., 1–15. doi: 10.1080/0142159X.2024.2445037
Mese, I., Taslicay, C. A., and Sivrioglu, A. K. (2023). Improving radiology workflow using ChatGPT and artificial intelligence. Clin. Imaging 103:109993. doi: 10.1016/j.clinimag.2023.109993
Moya-Salazar, J., Goicochea-Palomino, E. A., Porras-Guillermo, J., Cañari, B., Jaime-Quispe, A., Zuñiga, N., et al. (2023). Assessing empathy in healthcare services: a systematic review of South American healthcare workers' and patients' perceptions. Front. Psych. 14:1249620. doi: 10.3389/fpsyt.2023.1249620
Mundfrom, D. J., Shaw, D. G., and Ke, T. L. (2005). Minimum sample size recommendations for conducting factor analyses. Int. J. Test. 5, 159–168. doi: 10.1207/s15327574ijt0502_4
Naik, N., Hameed, B. M. Z., Shetty, D. K., Swain, D., Shah, M., Paul, R., et al. (2022). Legal and ethical consideration in artificial intelligence in healthcare: who takes responsibility? Front. Surg. 9:862322. doi: 10.3389/fsurg.2022.862322
Ning, Y., Teixayavong, S., Shang, Y., Savulescu, J., Nagaraj, V., Miao, D., et al. (2024). Generative artificial intelligence and ethical considerations in health care: a scoping review and ethics checklist. Lancet Digit. Health 6, e848–e856. doi: 10.1016/s2589-7500(24)00143-2
Nur, A. A., Leibbrand, C., Curran, S. R., Votruba-Drzal, E., and Gibson-Davis, C. (2024). Managing and minimizing online survey questionnaire fraud: lessons from the triple C project. Int. J. Soc. Res. Methodol. 27, 613–619. doi: 10.1080/13645579.2023.2229651
Oniani, D., Hilsman, J., Peng, Y., Poropatich, R. K., Pamplin, J. C., Legault, G. L., et al. (2023). Adopting and expanding ethical principles for generative artificial intelligence from military to healthcare. npj Digit. Med. 6:225. doi: 10.1038/s41746-023-00965-x
Panagioti, M., Khan, K., Keers, R. N., Abuzour, A., Phipps, D., Kontopantelis, E., et al. (2019). Prevalence, severity, and nature of preventable patient harm across medical care settings: systematic review and meta-analysis. BMJ 366:l4185. doi: 10.1136/bmj.l4185
Ramarajan, M., Dinesh, A., Muthuraman, C., Rajini, J., Anand, T., and Segar, B. (2024). “AI-driven job displacement and economic impacts: ethics and strategies for implementation” in Cases on AI ethics in business. eds. K. L. Tennin, S. Ray, and J. M. Sorg (Hershey, PA, USA: IGI Global), 216–238.
Rawashdeh, A. (2023). The consequences of artificial intelligence: an investigation into the impact of AI on job displacement in accounting. J. Sci. Technol. Policy Manag. 16, 506–535. doi: 10.1108/JSTPM-02-2023-0030
Reichert, A. R., Augurzky, B., and Tauchmann, H. (2015). Self-perceived job insecurity and the demand for medical rehabilitation: does fear of unemployment reduce health care utilization? Health Econ. 24, 8–25. doi: 10.1002/hec.2995
Rony, M. K. K., Numan, S. M., Johra, F. T., Akter, K., Akter, F., Debnath, M., et al. (2024a). Perceptions and attitudes of nurse practitioners toward artificial intelligence adoption in health care. Health Sci. Rep. 7:e70006. doi: 10.1002/hsr2.70006
Rony, M. K. K., Parvin, M. R., Wahiduzzaman, M., Debnath, M., Bala, S. D., and Kayesh, I. (2024b). "I wonder if my years of training and expertise will be devalued by machines": concerns about the replacement of medical professionals by artificial intelligence. SAGE Open Nurs. 10:23779608241245220. doi: 10.1177/23779608241245220
Roosan, D., Padua, P., Khan, R., Khan, H., Verzosa, C., and Wu, Y. (2024). Effectiveness of ChatGPT in clinical pharmacy and the role of artificial intelligence in medication therapy management. J. Am. Pharm. Assoc. 64, 422–428.e8. doi: 10.1016/j.japh.2023.11.023
Sallam, M. (2023). ChatGPT utility in healthcare education, research, and practice: systematic review on the promising perspectives and valid concerns. Healthcare (Basel) 11:887. doi: 10.3390/healthcare11060887
Sallam, M., Alasfoor, I. M., Khalid, S. W., Al-Mulla, R. I., Al-Farajat, A., Mijwil, M. M., et al. (2025b). Chinese generative AI models (DeepSeek and Qwen) rival ChatGPT-4 in ophthalmology queries with excellent performance in Arabic and English. Narra J. 5:e2371. doi: 10.52225/narra.v5i1.2371
Sallam, M., Al-Mahzoum, K., Almutairi, Y. M., Alaqeel, O., Abu Salami, A., Almutairi, Z. E., et al. (2024a). Anxiety among medical students regarding generative artificial intelligence models: a pilot descriptive study. Int. Med. Educ. 3, 406–425. doi: 10.3390/ime3040031
Sallam, M., Al-Mahzoum, K., Sallam, M., and Mijwil, M. M. (2025a). DeepSeek: is it the end of generative AI monopoly or the mark of the impending doomsday? Mesopotamian J. Big Data 2025, 26–34. doi: 10.58496/MJBD/2025/002
Sallam, M., Elsayed, W., Al-Shorbagy, M., Barakat, M., El Khatib, S., Ghach, W., et al. (2024b). ChatGPT usage and attitudes are driven by perceptions of usefulness, ease of use, risks, and psycho-social impact: a study among university students in the UAE. Front. Educ. 9:1414758. doi: 10.3389/feduc.2024.1414758
Sallam, M., Salim, N. A., Barakat, M., Al-Mahzoum, K., Al-Tammemi, A. B., Malaeb, D., et al. (2023). Assessing health students' attitudes and usage of ChatGPT in Jordan: validation study. JMIR Med. Educ. 9:e48254. doi: 10.2196/48254
Shamsuddinova, S., Heryani, P., and Naval, M. A. (2024). Evolution to revolution: critical exploration of educators’ perceptions of the impact of artificial intelligence (AI) on the teaching and learning process in the GCC region. Int. J. Educ. Res. 125:102326. doi: 10.1016/j.ijer.2024.102326
Small, W. R., Wiesenfeld, B., Brandfield-Harvey, B., Jonassen, Z., Mandal, S., Stevens, E. R., et al. (2024). Large language model-based responses to patients' in-basket messages. JAMA Netw. Open 7:e2422399. doi: 10.1001/jamanetworkopen.2024.22399
Spielberger, C. D., Gonzalez-Reigosa, F., Martinez-Urrutia, A., Natalicio, L. F., and Natalicio, D. S. (1971). The state-trait anxiety inventory. Revista Interamericana de Psicologia/Interam. J. Psychol. 5.
Spielberger, C. D., and Reheiser, E. C. (2004). “Measuring anxiety, anger, depression, and curiosity as emotional states and personality traits with the STAI, STAXI and STPI” in Comprehensive handbook of psychological assessment, Vol. 2: personality assessment (Hoboken, NJ, US: John Wiley & Sons, Inc.), 70–86.
Strzelecki, A. (2023). To use or not to use ChatGPT in higher education? A study of students’ acceptance and use of technology. Interact. Learn. Environ. 32, 5142–5155. doi: 10.1080/10494820.2023.2209881
Strzelecki, A. (2024). Students’ acceptance of ChatGPT in higher education: an extended unified theory of acceptance and use of technology. Innov. High. Educ. 49, 223–245. doi: 10.1007/s10755-023-09686-1
Taber, K. S. (2018). The use of Cronbach’s alpha when developing and reporting research instruments in science education. Res. Sci. Educ. 48, 1273–1296. doi: 10.1007/s11165-016-9602-2
Tai-Seale, M., Baxter, S. L., Vaida, F., Walker, A., Sitapati, A. M., Osborne, C., et al. (2024). AI-generated draft replies integrated into health records and physicians' electronic communication. JAMA Netw. Open 7:e246565. doi: 10.1001/jamanetworkopen.2024.6565
Tavakol, M., and Dennick, R. (2011). Making sense of Cronbach's alpha. Int. J. Med. Educ. 2, 53–55. doi: 10.5116/ijme.4dfb.8dfd
Tursunbayeva, A., and Renkema, M. (2023). Artificial intelligence in health-care: implications for the job design of healthcare professionals. Asia Pac. J. Hum. Resour. 61, 845–887. doi: 10.1111/1744-7941.12325
Verlingue, L., Boyer, C., Olgiati, L., Brutti Mairesse, C., Morel, D., and Blay, J. Y. (2024). Artificial intelligence in oncology: ensuring safe and effective integration of language models in clinical practice. Lancet Reg. Health Eur. 46:101064. doi: 10.1016/j.lanepe.2024.101064
Wang, C., Liu, S., Yang, H., Guo, J., Wu, Y., and Liu, J. (2023). Ethical considerations of using ChatGPT in health care. J. Med. Internet Res. 25:e48009. doi: 10.2196/48009
Williamson, S. M., and Prybutok, V. (2024). Balancing privacy and progress: a review of privacy challenges, systemic oversight, and patient perceptions in AI-driven healthcare. Appl. Sci. 14:675. doi: 10.3390/app14020675
Xia, Q., Weng, X., Ouyang, F., Lin, T. J., and Chiu, T. K. F. (2024). A scoping review on how generative artificial intelligence transforms assessment in higher education. Int. J. Educ. Technol. High. Educ. 21:40. doi: 10.1186/s41239-024-00468-z
Yim, D., Khuntia, J., Parameswaran, V., and Meyers, A. (2024). Preliminary evidence of the use of generative AI in health care clinical services: systematic narrative review. JMIR Med. Inform. 12:e52073. doi: 10.2196/52073
Yusuf, A., Pervin, N., and Román-González, M. (2024). Generative AI and the future of higher education: a threat to academic integrity or reformation? Evidence from multicultural perspectives. Int. J. Educ. Technol. High. Educ. 21:21. doi: 10.1186/s41239-024-00453-6
Zirar, A., Ali, S. I., and Islam, N. (2023). Worker and workplace artificial intelligence (AI) coexistence: emerging themes and research agenda. Technovation 124:102747. doi: 10.1016/j.technovation.2023.102747
Glossary
AI - Artificial intelligence
ANOVA - Analysis of Variance
CDA - Creative Displacement Anxiety
CFA - Confirmatory Factor Analysis
EFA - Exploratory Factor Analysis
EHRs - Electronic health records
FAME - Fear, Anxiety, Mistrust, and Ethics
GCC - Gulf Cooperation Council
genAI - Generative artificial intelligence
GFI - Goodness of Fit Index
GPA - Grade point average
HCPs - Healthcare professionals
HSSs - Health sciences students
KMO - Kaiser-Meyer-Olkin
K-W - Kruskal Wallis test
M-W - Mann Whitney U test
RMSEA - Root Mean Square Error of Approximation
SD - Standard deviation
SRMR - Standardized Root Mean Square Residual
STAI - State-Trait Anxiety Inventory
TAM - Technology Acceptance Model
TLI - Tucker-Lewis Index
VIF - Variance Inflation Factor
Keywords: technophobia, anxiety, ChatGPT, artificial intelligence, Chatbots, higher education, health education, psychology in education
Citation: Sallam M, Al-Mahzoum K, Alaraji H, Albayati N, Alenzei S, AlFarhan F, Alkandari A, Alkhaldi S, Alhaider N, Al-Zubaidi D, Shammari F, Salahaldeen M, Slehat AS, Mijwil MM, Abdelaziz DH and Al-Adwan AS (2025) Apprehension toward generative artificial intelligence in healthcare: a multinational study among health sciences students. Front. Educ. 10:1542769. doi: 10.3389/feduc.2025.1542769
Edited by:
Alyse Jordan, Indiana State University, United StatesReviewed by:
Edwin Ramirez-Asis, National University Santiago Antunez de Mayolo, PeruTara Mansour, MGH Institute of Health Professions, United States
Copyright © 2025 Sallam, Al-Mahzoum, Alaraji, Albayati, Alenzei, AlFarhan, Alkandari, Alkhaldi, Alhaider, Al-Zubaidi, Shammari, Salahaldeen, Slehat, Mijwil, Abdelaziz and Al-Adwan. This is an open-access article distributed under the terms of the Creative Commons Attribution License (CC BY). The use, distribution or reproduction in other forums is permitted, provided the original author(s) and the copyright owner(s) are credited and that the original publication in this journal is cited, in accordance with accepted academic practice. No use, distribution or reproduction is permitted which does not comply with these terms.
*Correspondence: Malik Sallam, bWFsaWsuc2FsbGFtQGp1LmVkdS5qbw==