- 1School of Agricultural Economics and Rural Development, Renmin University of China, Beijing, China
- 2Institute of Agricultural Economics and Development, Chinese Academy of Agricultural Sciences, Beijing, China
The advancement of the digital economy is vital for decreasing agricultural carbon emissions and fostering high-quality agricultural development. Using panel data from 31 Chinese provinces between 2000 and 2021, this paper employs a dual machine learning model for causal inference to analyze the impact of digital financial inclusion on agricultural carbon emissions intensity, its underlying mechanisms, and the characteristics of heterogeneity. The study finds that digital inclusive finance significantly reduces agricultural carbon intensity through two main channels: enhancing scientific and technological innovation and narrowing the urban-rural income gap. Additionally, the expansion of arable land management and the acceleration of economic structural transformation positively moderate these effects. These conclusions remain robust after a series of robustness tests. Further combining factors such as resource endowment, geographic location, economic concentration, and food production areas in the heterogeneity test, the study found that regional differences significantly influence the effect of financial inclusion on agricultural carbon intensity. Therefore, it is essential to enhance the development of inclusive finance, break down regional barriers to promote synergistic development, actively support economic transformation and large-scale operations, strengthen scientific and technological innovation, and narrow the urban-rural income gap to support China’s agricultural green transformation.
1 Introduction
Since the launch of economic reforms and the implementation of the opening-up policy, China has undergone more than 4 decades of sustained economic growth and significant increases in per capita income. Per capita GDP has risen sharply from RMB 385 in 1978 to RMB 85,698 in 2022, achieving an average annual growth rate of 13.07%1. Nevertheless, it is crucial to acknowledge that the simplistic ‘high input–high output’ model continues to dominate agricultural development in China. The intensive use of agricultural inputs has become a primary factor enabling farmers to increase their income and elevate production levels. In 2020, China’s fertilizer usage reached 506.11 kg/ha, approximately 2–3 times greater than that of developed countries. The application of pesticides totaled 1,456,000 tonnes, representing 2.8 to 4.7 times the amount used in developed countries,2China’s farmland irrigation water use efficiency coefficient is only 0.57, which is significantly lower than the 0.7–0.8 efficiency rate in developed countries, leaving substantial room for improvement (He and Song, 2021). The overuse of production materials, including agricultural films and fertilizers, results in significant resource consumption and elevated carbon emissions. To achieve the lofty objectives of carbon peaking by 2030 and carbon neutrality by 2060, the Chinese government is prioritizing the decrease of carbon emissions in the agriculture sector. The publication of the Action Plan for Energy Conservation and Carbon Reduction for 2024–2025, the Opinions on Accelerating Comprehensive Green Transformation of Economic and Social Development, and the Implementation Plan for Reducing Emissions and Sequestering Carbon in Agriculture and Rural Areas has distinctly defined the goals, tasks, and strategies for emission reduction and carbon sequestration in the agricultural and rural sectors. These policies aim to improve the quality and quantity of China’s agricultural emission reduction initiatives by decreasing the carbon intensity of the agricultural sector and increasing carbon output efficiency by one unit (Zhang and Mu, 2019). Concurrently, Digital financial products are steadily integrating into the agricultural sector, offering new avenues for innovation and growth. The China Digital Economy Development White Paper (2020) highlights that the core of the digital economy revolves around using digital information as a vital factor of production, digital technology functions as the central driving force, while contemporary information networks become the principal medium for diverse economic operations (Mu and Ma, 2021). Historically, academic research has predominantly focused on integration and growth driven by the digital economy within the secondary and tertiary sectors. However, with the release of key documents such as the Outline of the Digital Rural Development Strategy and the Action Plan for the Development of Digital Rural Areas (2022–2025), The digital economy in agriculture and rural areas has encountered significant development opportunities. By harnessing advancements in internet technology and financial innovation, digital inclusive finance presents the dual benefits of integrating information technology and reducing financial barriers. It also holds considerable potential for enhancing agricultural carbon production effectiveness (Lu et al., 2024; Geng and Pan, 2024).
Within the existing literature, studies on broader themes such as financial development and its connection to carbon emissions are more abundant. On one hand, some researchers assert that finance is essential for the optimal allocation of resources. They suggest that a robust financial sector can effectively reduce the costs associated with financing green development, and that green financial instruments play a crucial role in curbing carbon emissions (Zhao C. et al., 2024; Guo et al., 2024; Lv et al., 2024). Additionally, financial agglomeration contributes to the diminution in agricultural carbon emissions (Liu et al., 2024). On the other hand, several studies propose finance could lower carbon emission intensity by fostering technological innovation and strengthening innovation mechanisms (Huang, 2024; Ye and Ye, 2019; Sun, 2023). Furthermore, scholars identify industrial agglomeration, large-scale agricultural operations, and the advancement of farming specialization as critical factors affecting agricultural carbon emissions (Liu and Xiao, 2020; Liu et al., 2022; Fan, 2022). In summary, digital financial development can decrease carbon emission intensity and foster green development. By combining information technology with inclusive finance, digital inclusive finance boosts the efficiency and accessibility for rural finance services, lowers financing barriers and alleviates the misallocation of agricultural resources. It has the potential to decrease agricultural carbon emissions, thus providing a foundation for further exploration of the connection between digital inclusive finance and agricultural carbon emissions.
This work study offers novel contributions, firstly, the use of advanced algorithmic capabilities of dual machine learning models to efficiently handle high-dimensional variables and conduct non-parametric predictions, and applying them to assess the effectiveness of digital financial inclusion, we successfully tackle the challenges of the “curse of dimensionality” and model specification bias, frequently encountered in traditional econometric approaches. This methodology substantially improves the credibility and robustness of the study’s findings. Secondly, a new analytical framework has been created that center on digital financial inclusion, technological innovation, urban-rural income disparity, and agricultural carbon emissions. With technological innovation and urban-rural income disparity as the starting points, the framework analyzes the mediating transmission mechanism of digital financial inclusion on agricultural carbon emission intensity, while simultaneously examining the moderating effects of land management scale and the degree of economic structural transformation on digital financial inclusion. Thirdly, the disparities in resource endowment, geographical differences, economic concentration, and agricultural policy orientation are analyzed to explore the heterogeneous effects of digital financial inclusion across different regions, providing quantitative support for the achievement of regional coordinated development and agricultural carbon emission reduction. It also offers theoretical and practical insights for China and other developing countries in formulating region-specific development policies to reduce carbon emission intensity.
The remainder of this paper is structured as follows: Section 2 provides a theoretical analysis of digital financial inclusion and agricultural carbon emission intensity, followed by the formulation of research hypotheses. Section 3 discusses the principles of unbiasedness in dual machine learning, as well as data explanation and processing. Section 4 presents an in-depth discussion of the empirical analysis results, encompassing robustness tests, mechanism analysis, and an exploration of heterogeneity. Section 5 wraps up with the study’s conclusions and offers policy recommendations.
2 Theoretical mechanisms and research hypothesis
In the agricultural sector, inefficient and carbon-intensive farming practices amplify negative externalities and exacerbate carbon emissions, whereas science-based, precision-oriented production strategies create positive externalities and contribute to reducing the intensity of agricultural carbon emissions (Bai and Jia, 2024; Wei et al., 2024). Digital inclusive finance enhances resource utilization efficiency and promotes green total factor productivity, thereby directly contributing to the reduction of agricultural carbon emissions (Zhao et al., 2023). In addition, digital inclusive finance can reduce transaction costs and lower financial service thresholds in four ways: overcoming time and space constraints, improving the efficiency of rural financial services, increasing expected returns, and lowering the threshold for agricultural financing (Zhang G. et al., 2024; Shen et al., 2024; Li et al., 2024). This, in turn, enhances resource allocation efficiency in agricultural production. Furthermore, digital inclusive finance can expand farmers’ financing options through its digital capabilities (Chen et al., 2023), and can alleviate the “long-tail” challenge faced by rural residents (Zhou and Zhou, 2024). Facilitating the shift toward a greener and more sustainable production model, advocating for sustainable techniques and minimizing carbon emissions in agricultural operations, thereby lowering the intensity of agricultural carbon emissions. Based on the analyses discussed, this study introduces Hypothesis 1.
H1. The development of digital inclusive finance can reduce agricultural carbon emission intensity.
Enabled by mobile communication and information integration technologies, digital inclusive finance can fully harness the advantages of green finance, thereby promoting the adoption and implementation of low-carbon technologies in agriculture (Sun and Zhang, 2023). On the one hand, the traditional financial sector has long neglected the financial demands of rural communities and farmers, which has impeded agricultural technological innovation and the introduction and development of new crop varieties (Zhao X. et al., 2024). Moreover, advancements in agricultural technology not only improve regional carbon emission reduction efficiency but also generate demonstration effects that encourage neighboring regions to adopt advanced mitigation technologies, thereby contributing to a broader reduction in agricultural carbon emission intensity (Yang H. et al., 2022). On the other hand, digital financial inclusion can promote clean agricultural production by facilitating the adoption of green technologies, thereby contributing to carbon emission reduction (Yang Y. et al., 2022). Specifically, it can drive technological innovation, optimize the allocation of agricultural resources, reshape the energy structure, and enhance the efficiency of carbon-intensive inputs such as pesticides, fertilizers, and agricultural films (Hu et al., 2024; Liu et al., 2021). Simultaneously, it facilitates the structural transformation of the agricultural sector by reducing the proportion of high-carbon emission production (Shen and Zhang, 2024) and enhances carbon production efficiency per unit. Building on the analyses presented above, this paper puts forward Hypothesis 2.
H2. Financial inclusion reduces agricultural carbon intensity through regional technological and scientific innovations.
Agricultural production is characterized by long growth cycles, high climate dependency, slow capital turnover, and low return on investment. As a result, agricultural development requires not only special fiscal subsidies but also needs corresponding financial policy support to address persistent financing constraints (Zhang X. et al., 2022; Zhang Y. et al., 2024). Profit-oriented traditional financial institutions often marginalize vulnerable groups, including farmers, which decreases their access to financial services (Zhang and Chen, 2022). Financing constraints are common in rural areas, posing additional barriers to the transition of agricultural production methods toward low-carbon practices (Lin and Zhang, 2019). With financial backing, farmers can lower agricultural carbon emission intensity associated with agriculture by advancing the mechanization of agricultural production, optimizing crop cultivation patterns, and realizing economies of scale by shifting from fragmented to integrated operations (Zhang and Lu, 2023). Compared with the conventional financial system, digital inclusive finance supported by information technology is highly inclusive and convenient, significantly reducing the cost of financial services and rapidly expanding the service area. This facilitates access to financial resources for rural residents (He and Yang, 2021), it promotes economic development, decreases the urban-rural income gap, reduces financial risks for farmers adopting green production technologies, all while lowering agricultural carbon emission intensity. On the basis of the above analyses, this paper presents Hypothesis 3.
H3. Inclusive finance reduces agricultural carbon intensity by narrowing the rural-urban income gap.
Variations in regional economic development act as critical contextual factors that influence the effectiveness of financial inclusion. Regions undergoing substantial economic transformation typically experience a more significant influence of digital financial inclusion in minimizing agricultural carbon emissions (Gao et al., 2024). Digital inclusive finance plays a pivotal role promoting the development of digital villages, providing widespread coverage, high utilization, and advanced digitization in regions with greater economic development. In contrast, this impact is less pronounced in areas with diminished economic growth (Wen et al., 2024; Chen M. et al., 2024; Chen et al., 2024b; Chen et al., 2024c). Moreover, economic transformation promotes the growth of novel technology and managerial frameworks, enhances the efficiency of resource integration and utilization, and decreases carbon emission intensity (Zhang and Li, 2023). Furthermore, some studies have identified a notable spatial correlation between digital financial inclusion and the scale of land operations (Chen et al., 2023). The decentralized and small-scale characteristics of agricultural production remain significant constraints on fully leveraging the potential of digital financial inclusion. In contrast, moderate-scale operations can amplify the impact of digital financial inclusion in agricultural services (Wang and Qiu, 2024). These operations enhance productivity, enhances unit carbon productivity, and advance the green development of agriculture (Chen et al., 2023; Luo and Wei, 2023). Building on the above discussion, the following hypotheses are proposed.
H4. Both the degree of economic transformation and the scale of land operation enhance the effectiveness of financial inclusion in reducing the carbon intensity of agriculture.
Based on the analysis of the above theoretical mechanisms, it can be concluded that the inclusiveness of digital inclusive finance is the integration of traditional financial services and modern technology, by leveraging China’s advanced network infrastructure and mobile payment systems, improving the efficiency of rural financial services, reducing the cost of agricultural financing, optimizes resource allocation, and directly reduces the intensity of agricultural carbon emissions. Simultaneously, it mitigates carbon emission intensity by fostering scientific and technological innovation and narrowing the income gap between urban and rural areas. Furthermore, the degree of economic transformation and the scale of land management positively moderate the reduction of agricultural carbon emission intensity. The detailed mechanism of this influence is illustrated in Figure 1.
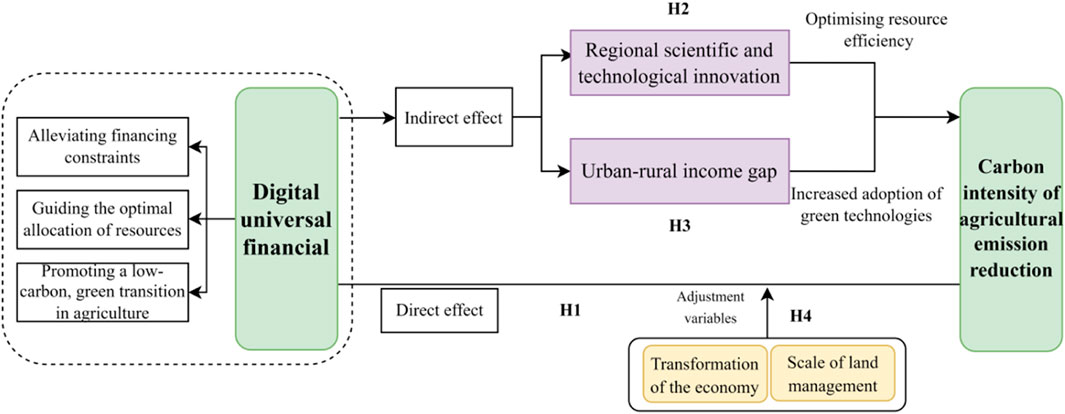
Figure 1. Theoretical framework for the impact of digital financial inclusion on agricultural carbon emissions.
3 Research design
3.1 Double machine learning model specification
This paper aims to examine the impact of the financial inclusion on carbon emission intensity of agricultural. Traditional econometric models for causal inference present various limitations. For instance, the synthetic control method can be used to create a counterfactual control group, but it is ineffective if the treatment group contains large ‘outliers’ or extreme value characteristics. Although regression discontinuity design (RDD) addresses control group issues more effectively, its robustness diminishes substantially as bandwidth increases. To overcome these challenges, a growing number of researchers have begun integrating machine learning techniques into causal inference within the field of economics (Chernozhukov et al., 2018; Athey et al., 2019; Knittel and Stolper, 2021).
Current international academic research on machine learning primarily falls into two categories. The first focuses on the application of dual machine learning models, with an emphasis on analyzing causal mechanisms, mediating effects, and robustness across various economic contexts (Zhang Y. et al., 2022; Yang et al., 2020; Farbmacher et al., 2022). The second examines the theoretical foundations and algorithmic principles of dual machine learning algorithms. Some scholars have improved the estimation of robust standard errors in dual clustering by employing multidirectional cross-fitting, refining the dual machine learning method for application to multidirectional clustered data and significantly enhancing the robustness and validity of estimations (Chiang et al., 2022). In addition, some researchers have innovatively integrated the dual machine learning model with dynamic analysis in an innovative manner, using weighted estimation to calculate the dynamic disposition effect, offering important theoretical support for panel data analysis (Bodory et al., 2022). Domestic research on dual machine learning remains in its early stages, with limited large-scale empirical studies. Notable studies include those by Mayra and Xie (2024) and Zhou et al. (2024), whose findings underscore the model’s practicality and advantages.
As a cutting-edge methodological approach, dual machine learning effectively addresses key challenges such as high-dimensional variable control and model specification bias, and it consistently outperforms traditional econometric models in terms of model fitting and analytical capacity. Agricultural carbon intensity is a multifaceted variable influenced by various determinants, such as government policy intervention, low-carbon technology adoption costs, and shifts in industrial structure. Controlling for the influence of these confounding factors is essential. Traditional models struggle with handling a large number of predictor variables and complex functional forms (Gu et al., 2020), and are prone to multicollinearity and weak out-of-sample predictive ability when dealing with high-dimensional control variables. By employing various machine learning algorithms and regularization techniques to effectively manage (Chen, Y., et al., 2022), extract and analyze data features, and uncover hidden relationships in large, high-dimensional, and complex economic data (Leippold et al., 2022). This not only addresses the ‘curse of dimensionality’ resulting from variable redundancy but also reduces estimation bias stemming from an insufficient number of key control variables (Tao and Guo, 2023). Furthermore, in the process of economic structural transformation and growth, non-linear relationships frequently arise (Tao and Guo, 2023; Liu, J. et al., 2023), and the strength of dual machine learning in managing non-linear data can effectively mitigate the issue of misspecification in traditional econometric models and enhance the robustness of the results (Yang et al., 2020). Moreover, dual machine learning features superior Neyman orthogonality, which ensures the unbiasedness of the treatment effect estimate in limited sample sizes. On this foundation, the partially linear dual machine learning model operates as follows:
Equation 1 the main regression equation,
The process is as follows: first, the auxiliary equation
Similarly, Equation 4 can again be approximated as:
Where,
3.2 Variable setting and selection
3.2.1 Dependent variable
This study focuses on the plantation sector, with the agricultural carbon emission intensity calculated based on the methodology proposed by Ji et al. (2023). Two primary criteria are used to evaluate the intensity of agricultural carbon emissions: (1) carbon emissions from agricultural input consumption, with specific carbon sources detailed in Table 1; and (2) the correlation coefficients related to agricultural carbon emissions from machine harvesting, land turning, and irrigation operations during crop growth. These coefficients are primarily drawn from the research conducted by the School of Biological and Agricultural Engineering at China Agricultural University and from Norse (2012).
In light of this, the following Equation 5 is used to determine the study’s carbon emission intensity:
Where,
The carbon intensity of the agricultural sector in Province
3.2.2 Core explanatory variable
Digital Inclusive Finance (lnif): This denotes the creation of a financial service system that leverages digital technology and mobile internet to improve underprivileged and underserved communities’ access to financial services. The advancement of this model is assessed through a comprehensive evaluation of the breadth of coverage (Lncb), depth of use (Lnud), and the level of digitalization (Lndl) in financial services. The dataset, which was released jointly by Ant Group and Peking University’s Financial Research Centre, covers the years 2011–2021. However, no detailed analysis or measurement is provided for periods available beyond this timeframe. In their foundational study on the compilation of the digital inclusive finance index, Guo et al. (2024) explore the essential features of digital inclusive finance. They argue that the core indicators of the breadth of digital financial coverage lie in the number of payment platforms and the degree to which banks enable card binding. The scale of financial institutions’ expansion affects residents’ access to third-party payment platforms and the convenience of bank card bundling, which are closely associated with the depth dimension of digital inclusive financial development. The degree of digital development is primarily determined by the prevalence of mobile payments, which in turn depends on Internet and telephone penetration. Therefore, this variable is a reasonable proxy for the digital development of digital inclusive finance between 2000 and 2010. The depth of digital financial inclusion consists mainly of payment and credit services, which are primarily realized through deposits and loans in financial institutions. Thus, changes in deposits and loans are appropriate proxies for the depth of digital financial inclusion during this period, capturing the relevant characteristics of its evolution. To extend the study period, this paper uses three proxies—Internet and telephone penetration rates, the quantity of financial institutions and the extent of financial growth (assessed by the ratio of loans and deposits to local GDP), serves as indicators of the degree of digital development, as well as the scope and depth of financial inclusion in each province during the period from 2000 to 2010. The data for each province and city for all years is completed using the same methodology as that applied for the 2011–2021 period, this method enables the creation of a comprehensive dataset to assess the degree of digital financial inclusion.
To further examine the spatiotemporal dynamics changes in carbon emission intensity and digital financial inclusion across space and time in China’s provinces, ArcGIS 10.8 software was used to apply the breakpoint method. China’s digital financial inclusion index and agricultural carbon emission intensity were classified into five levels, as visualized in Figure 2. Based on the four time points selected between 2000 and 2021, it is observed that annual agricultural carbon emission intensity is negatively correlated with digital financial inclusion. As digital financial inclusion advances, overall carbon emission intensity demonstrates a noticeable downward trend. From a spatial perspective, agricultural carbon emission intensity and digital financial inclusion exhibit strong spatial heterogeneity. This is because China’s coastal areas, through industrial and technological upgrading, have significantly increased output value while reducing carbon emission intensity. With the continuous expansion of digital financial inclusion, its influence has gradually extended from the coast to the inland regions. From a temporal perspective, the carbon emission intensity across provinces has declined at varying rates, a change that aligns with the development trajectory of the digital economy.
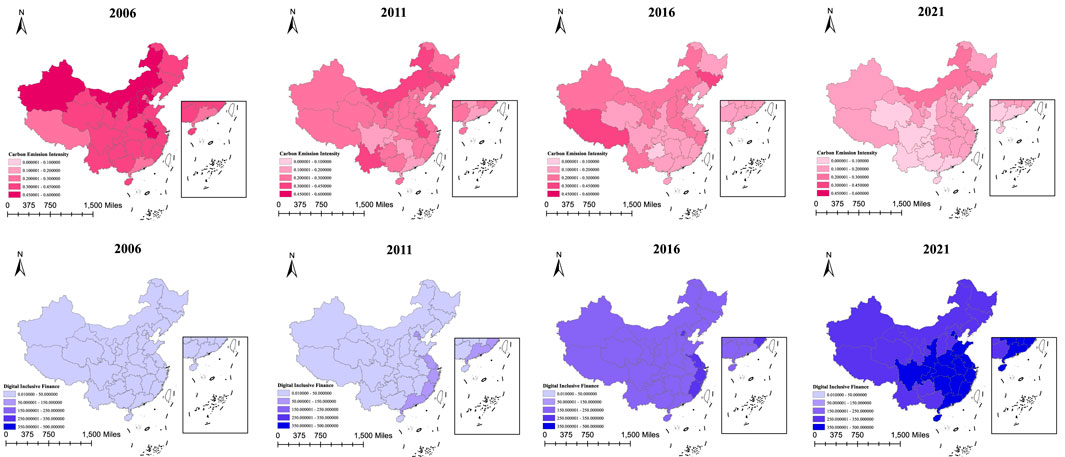
Figure 2. Spatial and temporal trends in agricultural carbon emission intensity and digital financial inclusion.
3.2.3 Control variables
Agricultural carbon emission intensity is affected by a wide range of factors. Traditional research has primarily focuses on economic volume (Shen and Li, 2023), environmental inputs (Fan, 2022), and the educational background of agricultural practitioners (Cheng et al., 2016). While these control variables can partially mitigate the impact of related factors, they are often insufficient for comprehensive analysis. To more accurately account for the determinants of agricultural carbon emission intensity, this study incorporates control variables across seven key dimensions: climate, economic scale, mechanization degree, industrial institutions, urban-rural gap, human resource level, and financial inputs, to analyze the factors influencing agricultural carbon emission intensity more accurately. Considering the research objectives and data availability, the detailed selection and definitions of control variables are presented below.
Agricultural industry structure (is) is defined as the ratio of the output value of the plantation industry to the total output value of the agriculture, forestry, animal husbandry, and fishery industries. Rural education level (edu) is expressed as the percentage of the rural labor force with high school education and above. The level of agricultural economic development (el) is measured by per capita agricultural output at comparable prices. Natural disaster level (dl) is expressed as the incidence rate of natural disasters affecting crops. The level of public investment in agriculture (gl) is measured by the proportion of local government expenditure on agriculture, forestry, and water conservancy relative to total fiscal expenditure. The degree of agricultural openness (ow) is expressed as the ratio of total agricultural imports (adjusted by the RMB-USD exchange rate) to total agricultural output. The degree of development of agricultural modernization (kw) is measured by the level of agricultural machinery power per unit of planting area. Rural labor outflow (lnwl) is represented by the difference between the total number of people employed in the countryside and the number of people employed in the primary industry (in 10,000 people). Consumption level (lnconsumption) is represented by local per capita consumption expenditure. Industrial structure (fir_ratio) is expressed as the percentage added of the primary industry as a proportion of the gross regional product (GRP). Agricultural industry aggregation (agglomeration) is represented by the ratio of local agricultural GDP to local GDP, relative to the national ratio of agricultural GDP to national GDP. Environmental regulation (envir_finance) is represented by environmental protection expenditure as a share of total budgetary expenditure. All quadratic terms of the control variables in this study are constructed by squaring of each individual variable.
3.2.4 Mechanism variables
Mediating variable: Development of science, technology, and innovation (lnip). The level of scientific and technological development in each province and city is measured by the number of granted invention patents. The urban-rural income gap is calculated using Theil’s index (Theil), following the method outlined by Theil (1967), with the calculation process shown in Equation 7.
Where
Moderating variables: Economic transformation (Economic_trans), measured as the ratio of the total value of the tertiary sector to the total value of the secondary sector. The scale of agricultural operations (scale), measured as the ratio of the area sown to the agricultural labor force.
3.3 Data
The data used to quantify agricultural carbon emissions in this study were obtained by the China Statistical Yearbook (2001–2022), China Agricultural Statistics (2001–2022), China Agricultural Yearbook (2001–2022). The financial inclusion data come from Provincial Digital Financial Inclusion Index (2011–2021). Data on invention patents are compiled from the Annual Report of the State Intellectual Property Office. The number of migrant workers is calculated using data from the China Population Statistical Yearbook and the China Rural Statistical Yearbook. Other control variables are sourced from the National Bureau of Statistics, the Provincial Statistical Yearbook, and the China Rural Management Statistical Yearbook. Linear interpolation was employed to rectify missing data, ensuring the sample’s consistency and the study’s temporal scope. The descriptive statistics for all variables are shown in Table 2.
4 Empirical analysis
4.1 Baseline analysis
This study adopts a double machine learning model partitioning the samples in a 1:4 ratio, and applies the support vector machine (SVM) algorithm for estimation. Is employed to cross-validate the predictive solutions for both the main and auxiliary equations. The full sample regression results are shown in Table 3, Columns (1)–(3) correspond to the inclusion of the primary control variable, adjustments for time and spatial effects, and the secondary controlled variable, correspondingly. The findings show that the regression coefficients are both negative and statistically significant at the 1% level, suggesting which the advancement of digital inclusive finance significantly reduces agricultural carbon emission intensity. These findings provide empirical support for Hypothesis 1. Building on model (1), the explanatory variables are replaced with two mechanism variables: the level of regional innovation (Lnip) and the urban-rural income disparity (LnTheil), when accounting for the linear and quadratic components of the other variables, correspondingly The regression results are presented in (4)–(7), which indicate that inclusive finance fosters regional innovation and contributes to reducing the urban-rural income gap, and all regression coefficients being significant at the 5% level.
4.2 Robustness check
In verifying the robustness of the findings, this paper examines two dimensions: the sample and the machine learning algorithm,. Sample-based robustness is tested by reconfiguring the sample space, removing outliers, and controlling for contemporaneous policy effects. Robustness of the machine learning algorithm is assessed by adjusting the cross-validation folds and substituting the algorithm. These procedures help mitigate the influence of sample and algorithm variations during the machine learning process on the study results and ensure the robustness of the findings.
4.2.1 Adjust sample interval
Given the substantial discrepancies in data collection among Chinese provinces and municipalities, including all provinces for regression analyses could introduce estimation bias. Therefore, the sample sizes of some explanatory variables varies due to missing data. To ensure the consistency key explanatory variables, the analyses use time horizons of 2005–2021 and 2011–2021 to further validate the robustness of the results under the sub-samples. Specifically, the results in column (1) of Table 4 show that after adjusting the time range of some samples, the financial inclusion index remains significantly negatively correlated with agricultural carbon emission intensity at the 1% significance level, indicating that the development of financial inclusion continues to reduce the intensity of agricultural carbon emissions and verifies the robustness of the results. Furthermore, the results in the table show that the regression coefficient increases in absolute value from −0.078 to −0.145 in post 2010 sample, suggesting that the effect of financial inclusion on agricultural carbon emissions has become more pronounced as China transitions to high-quality economic development.
4.2.2 Remove outlier effects
In this study, to ensure the robustness of the results and minimize the impact of outliers on model estimation, extreme values in the year and province variables were treated by excluding data points outside the 1st and 99th percentiles, as well as the 5th and 95th percentiles. After this robustness treatment, the dual machine learning analysis was rerun to further verify the model’s robustness. This approach not only enhances the credibility of the findings, but also provides a solid foundation for future research to more accurately assess variable relationships and draw reliable conclusions. The detailed regression results, as presented in Table 4 (2), reveal that after removing some provincial outliers, the results from the double machine learning analysis stays in considerably negative at the 1%, showing minimal deviation compared to the baseline results. The removal of outliers does not substantially influence the findings of this article, thereby affirming robustness and reliability of the outcomes.
4.2.3 Control for parallel policy effects
Minimizing the influence of concurrent environmental policies and ensuring accurate estimation of policy effects are crucial when assessing the relationship between financial inclusion and carbon intensity. After 2015, the State Council launched the Comprehensive Reform Programme for the Ecological Civilization System to explore new avenues for green development and promote the construction of a green ecological civilization in China. Subsequently, beginning in 2018, environmental protection tax policies were gradually introduced to guide the environmental transformation of China’s economy. In response, this study constructs policy dummy variables for ‘ecological civilization’ (Tech) and ‘environmental protection tax’ (envir_tax) for model analysis. The results, excluding the impact of other policies, are presented in Table 4 (3). The findings indicate that after controlling for the effects for one or two parallel policies during the same timeframe, the financial inclusion regression coefficient remains at −0.052 and is statistically significant relationship at 1% level, which is closely aligned with the baseline regression outcomes.
4.2.4 Reset double machine learning model
The robustness of the findings in this study was comprehensively evaluated by varying the number of cross-validation folds and applying multiple machine learning algorithms. This approach reduces dependence on specific algorithmic configurations while enhancing the study’s reliability and generalizability. Adjusting the sample splitting ratio directly affects the model’s generalization capability and predictive accuracy, while increasing the number of sample folds helps reduce sensitivity to particular training sets and improves prediction performance on new data. Furthermore, comparing the performance of different algorithms on the same dataset. Further validates the robustness of the findings from multiple perspectives, assesses the effectiveness of algorithms in addressing a specific problem, and guides further adjustments or optimizations. This multi-faceted approach not only minimizes overreliance on a single algorithmic setup but also strengthens the credibility and validity of the research findings through validation from multiple perspectives. Thus, using different folds for cross-fitting can significantly impact the machine learning analysis results. By changing the ratio from the previous 1:4 to 1:2, 1:3, 1:5, and 1:6, resulting in Kfolds = 3, Kfolds = 4, Kfolds = 6, and Kfolds = 7, respectively, the analysis results, as presented in Table 5, indicate that the coefficient for the digital financial inclusion index remains significantly negative at the 1% level, consistently demonstrating its substantial mitigating effect on agricultural carbon emission intensity.
Avoiding computational bias from a single machine learning algorithm in the regression results, the support vector machine algorithm was replaced with gradient descent (GradBoost), Random forest (Rf), and integrated machine learning (Ensemble learning), which combines the three algorithms for validation. Respectively. The new machine learning algorithms were used to conduct residual cross-validation of the main and auxiliary equations. Table 6 outlines the relationship between financial inclusion and the intensity of agriculture carbon emissions. Study’s findings point to a strong negative relationship observed across all three machine learning approaches at the 1% level, almost indistinguishable from the benchmark regression findings, thereby reinforcing the robustness and credibility of the model estimates.
4.3 Mechanism check
4.3.1 Mediation effect check
This section employs the Bootstrap-Sgmediation2 method (Preacher and Hayes, 2008) command to conduct a causal mediation effect analysis, with bootstrap replications configured to 500 and 1,000 to ensure the robustness of the results.
4.3.1.1 Enhance regional technological innovation
To examine how inclusive finance influences regional scientific and technological innovation, subsequently reducing agricultural carbon emission intensity. This present research utilizes the count of innovative inventions per province as a key metric to assess the level of scientific and technological innovation across different regions. The results show that both direct and indirect effects are negative and statistically significant at the 1% level, indicating that financial inclusion reduces agricultural carbon emission intensity through the regional scientific and technological innovation channel. This provides strong empirical support for Hypothesis H2.
4.3.1.2 Reduce urban-rural income gap
This study further explores the mechanism through which inclusive finance reduces agricultural carbon emission intensity by addressing the urban-rural income disparity. To quantify this gap, the Thiel index is calculated, incorporating variables such as urban-rural income, population, and other relevant factors. The regression results, presented in Table 7, indicate that both the indirect and direct effects of financial inclusion mediated by the urban-rural income gap on agricultural carbon emissions are significantly negative at the 1% level. These findings suggest that urban-rural income gap serves as a transmission mechanism, thus confirming Hypothesis H3.
4.3.2 Moderation effect check
4.3.2.1 Analysis of the moderating effect of economic transition degree
To evaluate the moderating effect of economic transformation on financial inclusion. An interaction term between economic transformation and financial inclusion is constructed, and the results from three machine learning models, presented in Table 8 (1), indicate that all moderating effects are substantial and unfavorable at the 1% level. This implies that economic transformation amplifies the impact of financial inclusion in reducing agricultural carbon emission intensity, thereby validating Hypothesis H4.
4.3.2.2 Analysis of the moderating effect of land management scale
Analyzing the moderating effect of land operation scale on the relationship between financial inclusion and agricultural carbon emissions. This proxy is used to construct an interaction term between land management scale and financial inclusion, which is subsequently integrated into the regression analysis, producing the findings displayed in Table 8 (2). Analysis of data utilizing three machine learning methods indicates that the interaction term between land scale and financial inclusion is strongly negative at the 1% level. This finding provides strong empirical support for Hypothesis H4, confirming that land operation scale enhances the carbon-reducing impact of financial inclusion.
4.4 Heterogeneity analysis
4.4.1 Resource endowment
The “Hu Huanyong Line,” proposed by Chinese geographer Hu Huanyong in 1935, delineates to outline the demographic landscape of China. It also approximately corresponds to the 400 mm precipitation isohyet that demarcates the semi-humid and semi-arid regions of the country. The group regression results presented in Table 9 indicate which the regression coefficient for the regions located east of the Hu Huanyong Line is negative and statistically significant at the 1% level. In unequivocal words, this coefficient exceeds the entire sample regression values, signifying that the effect of financial inclusion on reducing agricultural carbon emission intensity is more pronounced in regions situated east of the Hu Huanyong Line.
4.4.2 Geographic location
Due to the high share of maritime transport in international trade, and China’s reform and opening-up policy, which progressed from the coast to the rivers and then to the interior, the coastal region possesses unique distinct geographic advantages3. The coastal heterogeneity study denotes that this coefficients for coastal regions are notably negative at the 1% level, as shown in Table 10, Findings suggest that financial inclusion in coastal locations exerts a more significant influence on diminishing agricultural carbon emission intensity, as evidenced by a larger absolute value compared to the full-sample analysis. Similarly, the regression results for non-coastal regions are significantly negative at the 1% level, indicating that financial inclusion in these areas also results in a reduction of agricultural carbon emission intensity.
4.4.3 Economic concentration
Stimulating regional economic development, China has considered the unique characteristics of each region, forming five major economic zones: Guangdong-Hong Kong-Macao, Beijing-Tianjin-Hebei, the central Yangtze River region, the Yangtze River Delta, and Chengdu-Chongqing, each customized to local circumstances4, the region exhibits a high degree of integration in financial resources, information infrastructure, and modern transportation systems. Thus, a heterogeneity analysis was performed based on whether the sample was located within an economic zone. The regression results in Table 11 shows the regression results for samples within economic zones demonstrated significance at the 1% level, with the absolute value notably exceeding those of the non-economic zone samples.
5 Research findings and policy recommendations
This study focuses on digital inclusive finance and assesses agricultural carbon emission intensity in 31 provinces and cities in China from 2000 to 2021. An empirical analysis employing a dual machine learning framework indicates that inclusive finance significantly reduces agricultural carbon emission intensity and mitigates it through two mediating channels: regional scientific and technological innovation and the urban-rural income gap. In addition, the degree of economic transformation and the scale of land management are found to positively moderate the core explanatory variables. Further analysis reveals significant heterogeneity in resource endowment, geographic location, economic concentration, and production area positioning. Economically advantaged regions are more capable of mobilizing capital, talent, and technology to leverage financial inclusion and reduce agricultural carbon emissions. Conversely, disadvantaged regions face limitations in the effectiveness of financial inclusion due to resource constraints and other factors, which can even negate its impact.
Drawing from the study’s conclusions, the following policy recommendations are proposed. Firstly, efforts should be made to accelerate the development of digital financial inclusion, promoting expansion of financial institutions into more remote and less developed rural areas. Collaborate with local governments to streamline the financing approval process, facilitating farmers’ access to agricultural production capital more efficiently. The state should incentivize local governments to strategically plan and invest in broadband network infrastructure and provide targeted policy preferences for central and western regions, toward advanced digital platforms, including 5G, big data, and cloud computing. Given the disparities between eastern and western China, network infrastructure development should align with local application needs to ensure that farmers can quickly grasp market demand for green agricultural products and enhance the efficiency of digital financial inclusion. Additionally, the central government should emphasize the significance of rural finance through policy incentives and top-level design, establishing a well-functioning cycle of rural and inclusive finance to help reduce the intensity of agricultural carbon emissions intensity.
Secondly, local governments should enhance support for technological innovation by strengthening policy guidance and improving incentives mechanisms. This will accelerate the formation of technological innovation clusters and achieve high-quality agricultural development through technological advancements. Pursuing a low-carbon and green agricultural development path requires both strengthening agricultural technology development and optimizing the industrial structure to establish a systematic emission reduction framework. In this process, local government should actively formulate policies to promote technological progress while focusing on the efficient allocation of green agricultural technology resources. Moreover, integrating green agricultural technology with structural adjustments will ensure the complementary advantages of various emission reduction pathways. By leveraging local resource endowments, the government can contribute to building a comprehensive and sustainable emission reduction system, fostering sustainable agricultural development.
Thirdly, to reduce the income gap between urban and rural areas, urban employment service agencies and enterprises should prioritize professional skills training for farmers, establish platforms to promote urban-rural employment collaboration, and enhance farmers’ capacity to adopt new technologies and ideas. At the same time, addressing regional disparities in digital financial infrastructure and expanding the reach of digital inclusive finance can support the integrated development of rural industries will effectively cultivate and enhance farmers’ digital financial literacy. Additionally, improving the efficiency of digital inclusive financial services in rural areas will help narrow both the urban-rural gap and the ‘digital divide’ by alleviating spatial mismatches in regional digital economic development. This approach can also will also prevent excessive rural labor outflows, which could results in increased dependence on chemical fertilizers and other inputs. By improving local capacity and retaining labor, digital inclusive finance can play a vital role in supporting agricultural carbon emission reduction efforts.
Fourthly, economic transformation and large-scale land management should be strategically promoted according to local conditions to support low-carbon agricultural development. On the one hand, local governments should advance economic transformation by increasing investment in tertiary digital infrastructure, expanding Internet access in rural areas, and reducing digital connectivity costs to enhance farmers’ access to information. These efforts will help reduce the carbon intensity agriculture in the context of digital financial inclusion. On the other hand, promoting large-scale agricultural operations and optimizing the allocation of agricultural inputs are crucial for sustainable development. Strengthening the land transfer management systems and increasing agricultural land transfer subsidies will ensure institutional guarantees for scaling up operations. Furthermore, improving the dissemination and adoption of advanced agricultural technologies will maximize their efficiency benefits and carbon reduction potential. Integrating these technologies with the training of new-generation professional farmers will further contribute to achieving the “double carbon” goal.
Data availability statement
The raw data supporting the conclusions of this article will be made available by the authors, without undue reservation.
Author contributions
FZ: Conceptualization, Funding acquisition, Supervision, Writing – review and editing. SC: Project administration, Validation, Writing – original draft. XW: Data curation, Formal Analysis, Writing – original draft.
Funding
The author(s) declare that financial support was received for the research and/or publication of this article. This work is part of the research accomplishments of the project supported by the National Natural Science Foundation of China (72373146), the General Project of the National Social Science Foundation of China (21BJY131), the 2023 Bidding Project of the Institute of Planning and Design, Ministry of Agriculture and Rural Affairs (XCZYZDKT-20230101), the Fundamental Research Funds for Central Public Welfare Research Institutes (16100520240016), and the Science and Technology Innovation Project Grant of Chinese Academy of Agricultural Sciences (10-IAED-01–2024).
Conflict of interest
The authors declare that the research was conducted in the absence of any commercial or financial relationships that could be construed as a potential conflict of interest.
Generative AI statement
The author(s) declare that no Generative AI was used in the creation of this manuscript.
Publisher’s note
All claims expressed in this article are solely those of the authors and do not necessarily represent those of their affiliated organizations, or those of the publisher, the editors and the reviewers. Any product that may be evaluated in this article, or claim that may be made by its manufacturer, is not guaranteed or endorsed by the publisher.
Footnotes
1Data calculated by the National Statistical Office, https://data.stats.gov.cn/easyquery.htm?cn=C01.
2Data from the National Bureau of Statistics of China and the Food and Agriculture Organisation of the United Nations (FAO), https://data.stats.gov.cn/easyquery.htm?cn=C01和https://www.fao.org/faostat/zh/#data/RFM.
3Coastal provinces were among the first to benefit from China’s reform and opening-up policies. Their strategic geographic location facilitated the formation of a locational advantage. The coastal regions were also the first in China to participate in the international division of labor and manufacturing relocation, giving them a first-mover advantage.
4Covering an area of 1,138,100 square kilometers and housing a population of 703 million, these economic regions account for 62.57% of the national economy, despite comprising only 11.86% of the country’s total land area. The five economic circles are prominent in science and technology innovation, hosting 107 double first-class universities, which represent 71.8% of the national total. Beijing, Shanghai, Shenzhen, and Hangzhou collectively represent 82% of the total unicorns in the country.
References
Athey, S., Tibshirani, J., and Wager, S. (2019). Generalized random forests. Ann. Stat. 47 (2), 1148–1178. doi:10.1214/18-aos1709
Bai, Z., and Jia, H. (2024). Research on the policy configuration of ‘win-win’ between carbon peak and digital economy development. Nankai Econ. Res. (5), 177–197. doi:10.14116/j.nkes.2024.05.011
Bodory, H., Huber, M., and Lafférs, L. (2022). Evaluating (weighted) dynamic treatment effects by double machine learning. Econ. J. 25 (3), 628–648. doi:10.1093/ectj/utac018
Chen, M., Zhang, T., and Chu, Q. (2024a). Research on the regional collaborative development mechanism and path of digital inclusive finance in China: based on the perspective of eight comprehensive economic regions. Collect. Essays Finance Econ. (5), 60–70. doi:10.13762/j.cnki.cjlc.2024.05.009
Chen, Y., Wang, S., and Lu, S. (2024b). The impact of digital inclusive finance on agricultural scale operation: an empirical analysis based on spatial panel and threshold models. Contemp. Econ. Manag. 46 (3), 87–96. doi:10.13253/j.cnki.ddjjgl.2024.03.009
Chen, Y., Zhou, J., and Peng, J. (2024c). The motives behind dividends of Chinese listed companies: evidence from machine learning. China Ind. Econ. (5), 155–173. doi:10.19581/j.cnki.ciejournal.2024.05.009
Chen, Y., Zou, Y., and Li, X. (2023). The impact of digital inclusive finance on the urban-rural income gap: an analysis based on the moderating effect of the digital divide. Macroecon. Res. (10), 4–22. doi:10.16304/j.cnki.11-3952/f.2023.10.007
Chen, Z., Tang, C., Liu, B., Liu, P., and Zhang, X. (2022). Can socialized services reduce agricultural carbon emissions in the context of appropriate scale land management? Front. Environ. Sci. 10, 1039760. doi:10.3389/fenvs.2022.1039760
Cheng, L., Zhang, J., and Tian, Y. (2016). Spatial differentiation characteristics and dependence effects of agricultural carbon productivity in Chinese provinces. Resour. Sci. 38 (2), 276–289. doi:10.18402/resci.2016.02.10
Chernozhukov, V., Chetverikov, D., Demirer, M., Duflo, E., Hansen, C., Newey, W., et al. (2018). Double/debiased machine learning for treatment and structural parameters. Econ. J. 21 (1), C1–C68. doi:10.3386/w23564
Chiang, H. D., Kato, K., Ma, Y., and Sasaki, Y. (2022). Multiway cluster robust double/debiased machine learning. J. Bus. Econ. Stat., 40 (3), 1046–1056.
Fan, D. (2022). Agricultural technological progress, agricultural structure rationalisation and agricultural carbon emission intensity. Stat. Decis. Mak. 38 (20), 154–158. doi:10.13546/j.cnki.tjyjc.2022.20.030
Farbmacher, H., Huber, M., Lafférs, L., Langen, H., and Spindler, M. (2022). Causal mediation analysis with double machine learning. Economet. J., 25, (2), 277–300. doi:10.48550/arXiv.2002.12710
Gao, G., Wang, Q., and Zhi, H. (2024). An analysis of the impact of digital inclusive finance on agricultural carbon emission intensity. Econ. Issues (1), 57–65. doi:10.16011/j.cnki.jjwt.2024.01.010
Geng, N., and Pan, J. (2024). How does agricultural production trusteeship affect farmers' green technology adoption? China popul.-resour.-environ. 34 (4), 210–220. doi:10.12062/cpre.20231213
Gu, S., Kelly, B., and Xiu, D. (2020). Empirical asset pricing via machine learning. Rev. Financ. Stud. 33 (5), 2223–2273. doi:10.1093/rfs/hhaa009
Guo, X., Yang, J., Shen, Y., and Zhang, X. (2024). Impact on green finance and environmental regulation on carbon emissions: evidence from China. Front. Environ. Sci. 12, 1307313. doi:10.3389/fenvs.2024.1307313
He, K., and Song, H. (2021). China's food security under resource and environmental constraints: connotation, challenges and policy orientation. J. Nanjing Agric. Univ. Soc. Sci. Ed. 21 (3), 45–57. doi:10.19714/j.cnki.1671-7465.2021.0036
He, M., and Yang, X. (2021). Digital inclusive finance, carbon emissions, and total factor productivity. Financ. Forum 26 (2), 18–25. doi:10.16529/j.cnki.11-4613/f.2021.02.004
Hu, Y., Liu, J., Zhang, S., Liu, Y., Xu, H., and Liu, P. (2024). New mechanisms for increasing agricultural total factor productivity: analysis of the regional effects of the digital economy. Econ. Anal. Policy 83, 766–785. doi:10.1016/j.eap.2024.07.017
Huang, J., He, W., Dong, X., Wang, Q., and Wu, J. (2024). How does green finance reduce China's carbon emissions by fostering green technology innovation? Energy 298, 131266. doi:10.1016/j.energy.2024.131266
Ji, X., Cui, Y., Zhang, S., Sun, H., Yuan, M., and Zhang, Y. (2023). Spatial effects and mechanisms of farmland transfer on agricultural carbon emission intensity. China Environ. Sci., 43 (12), 6611–6624. doi:10.19674/j.cnki.issn1000-6923.20230906.003
Knittel, C. R., and Stolper, S. (2021). Machine learning about treatment effect heterogeneity: the case of household energy use. AEA Pap. Proc. 111, 440–444. doi:10.1257/pandp.20211090
Leippold, M., Wang, Q., and Zhou, W. (2022). Machine learning in the Chinese stock market. J. Financ. Econ. 145 (2), 64–82. doi:10.1016/j.jfineco.2021.08.017
Li, M., Wang, Z., and He, G. (2024). Banking financial technology innovation, structural inclusive effects, and entrepreneurial vitality. Manag. World 40 (6), 195–216. doi:10.3969/j.issn.1002-5502.2024.06.011
Lin, M., and Zhang, Z. (2019). Promoting green production of enterprises with “blockchain + production”: new thoughts on the government's role. Econ. Dyn. (5), 42–56.
Liu, D., Zhu, X., and Wang, Y. (2021). China's agricultural green total factor productivity based on carbon emission: an analysis of evolution trend and influencing factors. J. Clean. Prod. 278, 123692. doi:10.1016/j.jclepro.2020.123692
Liu, H., Wen, S., and Wang, Z. (2022). Agricultural production agglomeration and total factor carbon productivity: based on NDDF–MML index analysis. China Agric. Econ. Rev. 14 (4), 709–740. doi:10.1108/caer-02-2022-0035
Liu, J., Zheng, C., and Hong, Y. (2023). How can machine learning empower management research? A review of domestic and international frontiers and future prospects. Manag. World 39 (9), 191–216. doi:10.19744/j.cnki.11-1235/f.2023.0106
Liu, L., Zhang, L., Li, B., Wang, Y., and Wang, M. (2024). Can financial agglomeration curb carbon emissions reduction from agricultural sector in China? Analyzing the role of industrial structure and digital finance. J. Clean. Prod. 440, 140862. doi:10.1016/j.jclepro.2024.140862
Liu, Q., and Xiao, H. (2020). The impact of farmland management scale and fiscal policy for supporting agriculture on agricultural carbon emission. Resour. Sci. 42 (6), 1063–1073. doi:10.18402/resci.2020.06.05
Lu, H., Ding, Y., and Wu, W. (2024). Effectiveness assessment of agricultural green development advance zone policy: an empirical study based on a sample of counties in Zhejiang Province. Res. Dev. Manag. 36 (4), 24–34. doi:10.13581/j.cnki.rdm.20231575
Luo, M., and Wei, B. (2023). The carbon reduction effect of agricultural productive services: effects and mechanisms. Econ. Geogr. 40 (4), 58–68. doi:10.15931/j.cnki.2023.04.001
Lv, C., Jiang, Y., and He, J. (2024). Carbon emission reduction effect of green financial policies: practice from green financial reform and innovation pilot zone. China Manag. Sci., 1–24. doi:10.16381/j.cnki.issn1003-207x.2023.0888
Mayra, M., and Xie, Y. (2024). The impact of agricultural productive services on high-quality agricultural development: based on a double machine learning model. Res. World, 1–12. doi:10.13778/j.cnki.11-3705/c.2024.06.002
Mu, J., and Ma, L. (2021). Measurement of digital economy development index and regional differences in rural agriculture in China. J. South China Agric. Univ. Soc. Sci. Ed. 20 (4), 90–98. doi:10.7671/j.issn.1672-0202.2021.04.009
Norse, D. (2012). Low carbon agriculture: Objectives and policy pathways. Environ. Dev., 1 (1), 25–39.
Preacher, K. J., and Hayes, A. F. (2008). Asymptotic and resampling strategies for assessing and comparing indirect effects in multiple mediator models. Behav. Res. Methods 40 (3), 879–891. doi:10.3758/brm.40.3.879
Shen, Y., Fu, Y., and Song, M. (2024). Digital finance promotes sustainable total factor eco-efficiency: evidence from China. Appl. Econ. 56 (36), 4389–4403. doi:10.1080/00036846.2023.2211337
Shen, Y., and Li, J. (2023). The co-prosperity effect and spatial differentiation of digital inclusive finance in promoting the integrated development of rural industries. J. South China Agric. Univ. Soc. Sci. Ed. 22 (4), 82–95. doi:10.7671/j.issn.1672-0202.2023.04.008
Shen, Y., and Zhang, S. (2024). Digital inclusive finance empowering the construction of modern agricultural strong counties: theory and empirical evidence. Collect. Essays Finance Econ. (9), 57–68. doi:10.13762/j.cnki.cjlc.20240709.001
Sun, C. (2023). How are green finance, carbon emissions, and energy resources related in Asian sub-regions? Resour. Policy 83, 103648. doi:10.1016/j.resourpol.2023.103648
Sun, L., and Zhang, Q. (2023). Research on the impact of digital inclusive finance on corporate carbon emission intensity. Jiangxi Soc. Sci. 43 (11), 90–101.
Tao, X., and Guo, F. (2023). Heterogeneous policy effects evaluation and machine learning methods: research progress and future directions. Manag. World 39 (11), 216–237. doi:10.19744/j.cnki.11-1235/f.2023.0127
Wang, H., and Qiu, T. (2024). The effects of farmer cooperatives on agricultural carbon emissions reduction: evidence from rural China. J. Clean. Prod. 450, 141881. doi:10.1016/j.jclepro.2024.141881
Wei, Z., Sun, G., and Yao, Z. (2024). Dual benefits and influencing factors of marine carbon sink trading under voluntary emission reduction mechanism. China popul.-resour.-environ. 34 (4), 48–59. doi:10.12062/cpre.20230402
Wen, T., Zhao, X., and Zhang, L. (2024). Can digital inclusive finance promote the development of digital villages? J. Guizhou Univ. Finance Econ. (3), 1–10. doi:10.3969/j.issn.1003-6636.2024.03.001
Yang, H., Wang, X., and Bin, P. (2022a). Agriculture carbon-emission reduction and changing factors behind agricultural eco-efficiency growth in China. J. Clean. Prod. 334, 130193. doi:10.1016/j.jclepro.2021.130193
Yang, J., Chuang, H., and Kuan, C. (2020). Double machine learning with gradient boosting and its application to the Big N audit quality effect. J. Econom. 216 (1), 268–283. doi:10.1016/j.jeconom.2020.01.018
Yang, Y., Wu, L., and Zhang, Q. (2022b). The impact of digital inclusive finance on green agricultural growth: also discussing the moderating role of rural human capital investment. Inq. Econ. Issues (6), 165–180.
Ye, C., and Ye, Q. (2019). Is financial structure irrelevant to carbon emissions? Based on the perspective of financial supply-side structural reform. Econ. Theory Econ. Manag. (10), 31–44.
Zhang, G., Liang, Z., and Wu, Z. (2024a). Analysis of the evolution characteristics of digital inclusive finance in Chinese urban agglomerations and its effect on high-quality development. Geogr. Res. 43 (3), 621–639. doi:10.11821/dlyj020230426
Zhang, T., and Li, J. (2023). Network infrastructure, inclusive green growth, and regional disparities: causal inference based on double machine learning. Quant. Econ. Tech. Econ. Res. 40 (4), 113–135. doi:10.13653/j.cnki.jqte.20230310.005
Zhang, W., and Lu, Y. (2023). Research on the impact of digital inclusive finance on household property income. Financ. Dev. Res. (3), 79–86. doi:10.19647/j.cnki.37-1462/f.2023.03.011
Zhang, X., Zhang, X., and Gao, S. (2022a). Analysis of the potential willingness for farmland mortgage loans under the ‘three rights separation’ system: based on a survey of 916 farmers in Shandong Province. Dongyue Trib. 43 (4), 148–157. doi:10.15981/j.cnki.dongyueluncong.2022.04.018
Zhang, Y., Li, H., and Ren, G. (2022b). Quantifying the social impacts of the London Night Tube with a double/debiased machine learning-based difference-in-differences approach. Transp. Res. Part A Policy Pract. 163, 288–303. doi:10.1016/j.tra.2022.07.015
Zhang, Y., Wang, W., and Zhao, Y. (2024b). Disaster shocks, inclusive credit, and agricultural production efficiency loss: the mediating roles of financial exclusion and factor crowding. Financ. Forum 29 (6), 14–25. doi:10.16529/j.cnki.11-4613/f.2024.06.004
Zhang, Z., and Chen, X. (2022). Does digital finance increase the enrollment rate of ordinary high schools in rural areas? Res. Financ. Issues (6), 101–110. doi:10.19654/j.cnki.cjwtyj.2022.06.010
Zhang, Z., and Mu, Y. (2019). Can industrial agglomeration increase agricultural carbon productivity? China popul.-resour.-environ. 29 (7), 57–65. doi:10.12062/cpre.2019.01.04
Zhao, C., Zhong, C., Liu, C., and Xing, P. (2024a). How the digital economy is empowering green strategies for breaking carbon lock-in. J. Environ. Manag. 365, 121670. doi:10.1016/j.jenvman.2024.1216702024.121670
Zhao, J., Taghizadeh-Hesary, F., Dong, K., and Dong, X. (2023). How green growth affects carbon emissions in China: the role of green finance. Econ. Res.-Ekon. Istraž. 36 (1), 2090–2111. doi:10.1080/1331677x.2022.20955222022.2095522
Zhao, X., Benkraiem, R., Abedin, M. Z., and Zhou, S. (2024b). The charm of green finance: can green finance reduce corporate carbon emissions? Energy Econ. 134, 107574. doi:10.1016/j.eneco.2024.1075742024.107574
Zhou, J., Xu, W., and Mi, Z. (2024). The impact of the digital economy on urban ESG development. Geogr. Res. 43 (6), 1407–1424. doi:10.11821/dlyj.2023.09.23
Keywords: digital financial inclusion, double machine learning (DML), carbon emission intensity (CEI), impact mechanisms, green transformation
Citation: Zheng F, Chen S and Wang X (2025) How the impact and mechanisms of digital financial inclusion on agricultural carbon emission intensity: new evidence from a double machine learning model. Front. Environ. Sci. 13:1549623. doi: 10.3389/fenvs.2025.1549623
Received: 21 December 2024; Accepted: 17 April 2025;
Published: 28 April 2025.
Edited by:
Fuyou Guo, Qufu Normal University, ChinaCopyright © 2025 Zheng, Chen and Wang. This is an open-access article distributed under the terms of the Creative Commons Attribution License (CC BY). The use, distribution or reproduction in other forums is permitted, provided the original author(s) and the copyright owner(s) are credited and that the original publication in this journal is cited, in accordance with accepted academic practice. No use, distribution or reproduction is permitted which does not comply with these terms.
*Correspondence: Xizhao Wang, ODIxMDEyMjI0OTVAY2Fhcy5jbg==