- 1School of Economics and Management, North University of China, Taiyuan, China
- 2School of Economics, Shanxi University of Finance and Economics, Taiyuan, China
Against the backdrop of global climate governance and China’s dual-carbon goals, low-carbon city construction has emerged as a critical strategy for balancing economic growth and environmental sustainability. This study evaluates the impact of low-carbon city construction on inclusive green growth in China from 2006 to 2021. Empirical results indicate that these policies contribute to an average increase of 0.005 units in inclusive green growth for the treatment group compared to the control group, with robustness assessed through placebo tests and alternative variable substitutions. Further analysis indicates that the policy effects are more pronounced in central cities, resource-based cities, and cities with high carbon abatement potential, driven strongly by urban technological innovation, industrial structure optimization, and enhanced government leadership. However, spatial effect analysis shows that, despite a significant positive spatial correlation between cities, the spillover effects of low-carbon policies are limited, primarily concentrated within the pilot cities. Therefore, it is recommended to strengthen cross-regional governance alliances, establish green technology transfer centers, promote the “R&D-manufacturing-recycling” industrial chain, and deploy tailored regional transformation incentives. Additionally, drawing on international models such as the EU’s carbon border mechanism and Germany’s industry-university-research framework, collaborative “regional green growth partnerships” should be developed to foster inclusive green growth.
1 Introduction
As cities have become the new engine of global economic growth in the post-industrial era, the rapid pace of urbanization has brought new challenges to global environmental governance (McGee and Mori, 2021). It is estimated that cities account for over 80% of the world’s gross domestic product (GDP) and over 75% of carbon dioxide emissions (CO2) (Shan et al., 2021). As the second largest economy in the world, China’s reform and opening up over the past 40 years has made remarkable achievements in economic growth and social development. According to the data released by the National Bureau of Statistics of China in the Statistical Bulletin of National Economic and Social Development of the People’s Republic of China for 2023, the country’s total GDP for the year reached 126,058.2 billion yuan, marking a growth of 5.2% compared to the previous year. By the end of the year, the urbanization rate of the national permanent resident population was 66.16%, an increase of 0.94% points from the end of the previous year (NBSC, 2024). However, the rough and reckless growth and the previous development strategy of “pollute first, treat later” have led to uncertainty in green growth and sustainable development (Cheng et al., 2016; Liu et al., 2025; Zhao et al., 2020). Since 2006, China has surpassed the United States to become the world’s largest CO2 emitter (Zeng et al., 2021). China’s rapid economic development, industrialization, and urbanization have led to a continuous increase in energy demand and carbon dioxide emissions. By 2021, China’s total energy consumption, electricity consumption, and carbon dioxide emissions reached 5,259 million tons of standard coal, 8,259 TWh, and 10,647 Mt, respectively, which is an increase of 258%, 559%, and 244% compared to the year 2000. This vigorous growth trend means that China must proactively abandon its traditional development models and path dependencies, integrating the concept of green growth into its new urbanization process, thereby fostering an inclusive green growth model that balances societal development and effective environmental management. In this context, this paper examines China as a unique case and research area, exploring the positive effects of implementing low-carbon urban development to address current environmental constraints and promote inclusive green growth. The findings of this study are also of significant relevance to global sustainable development.
The concept of inclusive green growth, first introduced by the World Bank in 2012 and elaborated through the UN Sustainable Development Goals (SDGs), establishes a dual mandate for sustainable development that integrates environmental efficiency (“greening”) with social equity (“inclusiveness”) (Berkhout et al., 2018; Zhang and Li, 2023). In China’s context, this paradigm aligns with the national strategy for high-quality development characterized by innovation, coordination, green transition, openness, and shared benefits (Said and Dindar, 2024). While empirical studies confirm an upward trajectory in China’s inclusive green growth, persistent regional disparities highlight systemic challenges. Key barriers include technological mismatches between innovation scale and green development needs (Liu et al., 2021), economic policy uncertainties (Gu et al., 2021), and institutional capacity deficits in urban governance (Fan et al., 2023). These domestic findings resonate with international evidence showing context-dependent sustainability outcomes. For instance, Shobande and Asongu (2023) demonstrate that while information and communication technologies (ICT) robustly enhance environmental sustainability in South Africa, their impacts in Nigeria remain ambiguous, underscoring the spatial heterogeneity of policy effectiveness and the critical role of infrastructure preparedness.
To address regional imbalances, China has implemented urban-level policy experiments, among which the Low-Carbon City Pilot Policy (LCCPP) serves as a cornerstone intervention (Xu et al., 2023; Xu et al., 202a). Extant research delineates LCCPP’s multidimensional impacts: economically, it stimulates green industrial upgrading through technological innovation (Peng et al., 2023; Wu et al., 2023; Yin and Guo, 2022); socially, it fosters behavioral shifts toward sustainable lifestyles (Liu and Xu, 2022); environmentally, it improves energy efficiency and enables synergistic governance of carbon and smog pollution (Li et al., 2022; Wang et al., 2023). These domestic observations find parallels in global policy analyses. Shobande et al. (2024) reveal that carbon trading systems in OECD countries achieve emission reductions primarily when coupled with renewable energy investments, whereas standalone carbon taxes may exacerbate growth-emission trade-offs. Furthermore, circular economy strategies integrated with inclusive growth mechanisms—such as lifecycle product management and workforce reskilling—prove effective in accelerating decarbonization across EU nations. Such international evidence complements China’s local experiments, suggesting that policy coherence between technological, economic, and social instruments is pivotal for achieving sustainable transitions (Shobande et al., 2025). Despite these advancements, critical knowledge gaps remain unresolved. First, while existing studies extensively document LCCPP’s isolated economic or environmental outcomes (Yang et al., 2023; Zeng et al., 2023), its holistic impact on inclusive green growth—a concept demanding simultaneous progress in both environmental efficiency and social equity—has yet to be systematically evaluated. Second, the transmission mechanisms require clarification: although technological innovation and behavioral change are frequently cited pathways (Liu and Xu, 2022; Yin and Guo, 2022), comprehensive analyses integrating technological, institutional, and spatial drivers remain lacking. Third, spatial dynamics are underexplored, particularly the potential for policy spillovers across regions. This omission contrasts with international findings demonstrating spatially heterogeneous impacts of sustainability policies, as evidenced by Shobande and Ogbeifun (2022) analysis of ICT’s differential environmental effects across OECD nations.
To bridge these gaps, this study posits three core research questions: (1) Can the LCCPP effectively promote inclusive green growth in Chinese cities? (2) Through which mechanisms—technological innovation, institutional restructuring, or behavioral change—does the LCCPP exert its influence? (3) Do these policy effects exhibit spatial spillovers or regional heterogeneity? By synthesizing methodological approaches from China-focused policy evaluations (Li et al., 2022; Wu et al., 2023) and global analytical frameworks on policy coherence (Shobande et al., 2025), our analysis provides novel insights into optimizing multiscale sustainability governance.
This study advances the literature on inclusive green growth and low-carbon policy through three original contributions. First, departing from existing measurement frameworks that often emphasize single dimensions of sustainability, we systematically construct a multidirectional index system spanning economic, social, and environmental inclusiveness—the three constitutive pillars of inclusive green growth—and apply it dynamically to 279 Chinese cities from 2006 to 2021. This novel metric not only quantifies urban green development trajectories but also establishes an analytical baseline for policy effect evaluation. Second, leveraging the quasi-experimental setting of China’s low-carbon city pilot policy, we employ a difference-in-differences (DID) approach augmented by rigorous robustness checks (parallel trend analysis, placebo tests, PSM-DID) to empirically isolate the policy’s causal impacts. The analysis further uncovers critical heterogeneity across city types, challenging the “one-size-fits-all” policy presumption and offering actionable insights for differentiated governance frameworks. Third, extending beyond conventional evaluation paradigms, we pioneer spatial econometric investigations that reveal limited intercity spillovers despite significant spatial autocorrelation—a counterintuitive finding that underscores the localized nature of low-carbon policy benefits and necessitates revised strategies for regional coordination. Collectively, these theoretical, methodological, and empirical advancements deepen academic understanding of sustainability policy mechanisms while informing precision governance in green transitions.
The remaining structure of this paper is organized as follows: The Section 2 conducts a theoretical analysis and formulates research hypotheses. The Section 3 describes the methodology and data used in the study. Sections 4, 5 present the empirical results and the related testing procedures. The Section 6 offers the research conclusions and policy recommendations.
2 Theoretical analysis and hypotheses development
As shown in Figure 1, this study hypothesizes that LCCPP has a positive impact on Inclusive Green Growth. Specifically, LCCPP can promote urban technological innovation, optimize industrial structure, and enhance government leadership behavior, thereby advancing the level of Inclusive Green Growth. Therefore, this paper proposes three research hypotheses, which are discussed in detail below.
2.1 Urban technological innovation
In China, local governments have the authority to implement tailored pilot policies and policy innovations that tackle specific urban development challenges. Successful experiences obtained from pilot cities can subsequently be replicated elsewhere (Zou et al., 2022). Participating in these prestigious pilot initiatives encourages local governments to offer greater support for research and development in clean and green technologies, mitigating uncertainty and reducing the risks enterprises face in green investment, thus driving technological innovation capabilities (Peng and Bai, 2018). Furthermore, pilot cities establish clear carbon emission targets as well as platforms for carbon trading and registration, providing enterprises sustained economic incentives, such as through the sale of surplus carbon credits, to invest in green production technologies (Chen et al., 2021). Urban technological innovation fostered by these actions further promotes inclusive green growth. First, targeted and coordinated investment in green technological advancement improves technology coherence and helps avoid resource allocation redundancies, thus enhancing economic inclusiveness and sustainability (Sun et al., 2020; Zhou and Feng, 2017). Second, higher technological innovation efficiency strengthens resource use and production processes, facilitates new sustainable business models, and promotes transition in residents’ consumption and employment patterns, eventually realizing stable economic growth and environmental improvement simultaneously (Trapp and Kanbach, 2021; Ulucak, 2020). In recent years, the customized application of artificial intelligence in the environmental field has injected new momentum into technological innovation for low-carbon cities, supporting innovative green energy development and ecological protection solutions. Its comprehensive technological effects have been initially validated, and the enormous potential for educational promotion has continuously emerged, receiving initial research support from practical levels (Chen et al., 2024). At the same time, the direct technological dividends brought by digital transformation, which enhance production technology and economic efficiency, are particularly evident in high-pollution traditional enterprises. This helps improve overall departmental production efficiency and promotes the greening of industrial structures, thereby strengthening the mechanisms of policy transformation towards innovation-driven governance (Zhao et al., 2024). Thus, this study proposes:
H1:. Low-carbon urban construction promotes inclusive green growth through urban technological innovation.
2.2 Industrial structure optimization
Low-carbon city pilot policies leverage financial and policy support to promote a shift from high-emission industries towards green, service-oriented sectors, facilitating low-carbon travel and green consumption (Zeng et al., 2023). Such initiatives encourage market-driven elimination of non-transitionable high-emission industries, economic structure adjustment, stringent environmental standards, and early integration of sustainable principles in urban planning (Zheng et al., 2021). This further incentivizes low-carbon transportation, eco-friendly building practices, environmental protection, new energy, and other high-tech and emerging industries (Yang et al., 2019), alongside accelerated growth within low-carbon service sectors (Liu, 2023). Industrial structure upgrading enhances inclusive green growth in two key respects: first, it fosters the concentration of high-quality human capital, develops urban services, improves livelihood infrastructure, ultimately advancing economic inclusivity and welfare (Sun et al., 2022); second, boosting the proportion of modern services, advanced manufacturing, and digital economies maximizes economic output while minimizing energy consumption and emissions, thus supporting sustainable, green growth (Cheng et al., 2019; Ren et al., 2022). In the process of upgrading low-carbon industries, the construction of a multidimensional applicable evaluation benchmark management system is crucial for forming an institutional framework for industrial collaborative growth and accurately judging the adaptability of technical standards and environmental constraints. This aims to enhance the structural optimization level and precise management of new industrial sectors, enabling urban economies to achieve more inclusive green growth (Xu et al., 2024b). Accordingly, this research proposes:
H2:. Low-carbon urban construction promotes inclusive green growth through industrial structure optimization.
2.3 Government leadership behavior
The pilot policy for low-carbon cities, as a policy tool with Chinese characteristics, strengthens government leadership through dual institutional mechanisms. While the GDP-oriented development model may weaken local governments' incentives for environmental governance, the policy innovation demonstrated in low-carbon city construction creates a regional demonstration effect (Hui et al., 2016), serving as an institutional counterbalance to traditional growth priorities. This pilot system establishes a “signaling-commitment” framework: in contexts with weak environmental incentives, local governments utilize policy experimentation to showcase administrative capabilities, while the leadership activities in low-carbon development reciprocally enhance policy implementation capacity and public behavior regulation (Tie et al., 2020). The reinforced leadership facilitates inclusive green growth through three ways: first, by institutionalizing stricter environmental standards that internalize ecological costs into production systems; second, through fiscal reallocation that prioritizes green infrastructure investment; third, via regulatory innovation that transforms environmental governance from passive compliance to proactive prevention. These institutional upgrades ultimately translate policy innovation into sustainable growth outcomes through improved resource allocation efficiency and green technological spillovers. During the pilot process of low-carbon cities, a strict and coordinated government policy standard was established. By managing waste disposal and upgrading infrastructure ecologically, residents' awareness of green production and living can be effectively enhanced. The normative and proactive interventions demonstrated by the government in the exploration of ecological civilization construction have led to a comprehensive and continuous improvement in its ecological performance. This aligns with the motivational norms of local governments under policy and interest constraints and is also conducive to promoting innovations in green production and consumption (Ma et al., 2024). Therefore, this paper proposes the following research hypothesis:
H3:. Low-carbon urban construction promotes inclusive green growth by strengthening government leadership behavior.
2.4 Spatial spillover effects
The spatial spillover effects of low-carbon city pilot policies also constitute an important driving mechanism for inclusive green growth. As a vehicle for regional policy innovation, pilot cities establish networks for the flow of factors and channels for knowledge spillover across administrative boundaries, forming a spatial transmission mechanism characterized by the diffusion of clean technologies, the synergy of environmental regulations, and the sharing of green infrastructure. Geographically proximate cities achieve low-carbon technology transfer through industrial linkage effects, facilitate the optimal allocation of green production factors through interconnected transportation infrastructure, and promote the convergence of environmental regulation standards through policy learning effects. This spatial synergy effect not only breaks through resource misallocation caused by administrative barriers but also reduces marginal abatement costs through the economies of scale in regional environmental governance, ultimately achieving a balanced distribution of economic growth dividends and ecological improvement benefits on a larger geographical scale. In particular, it is worth mentioning that the policy experiences among emerging economic cities overseas have significant spillover and learning effects. However, in countries where barriers to energy transition are evident or where the monetary policy environment is relatively volatile, the uncertainty of policy expectations may lead to significant negative spillovers on the clean energy transition. This indicates that spatial spillovers do not always occur positively, and targeted localized experiments are needed to deepen particularly the policy mechanisms, promoting upgrades in long-term governance performance and collaboration (Liu et al., 2025). Therefore, this paper proposes the following research hypothesis:
H4:. The construction of low-carbon cities promotes inclusive green growth through spatial spillover effects.
3 Methodology and data
3.1 Model specification
This article constructs a difference-in-differences model to verify the impact of low-carbon city development on inclusive green growth, as shown in Equation 1.
The dependent variable
3.2 Variables
3.2.1 Dependent variable
Inclusive green growth (Igg). We refer to Zhang and Li (2023) and Fan et al. (2023) and to construct a comprehensive evaluation index system from the three dimensions of economic inclusiveness, social inclusiveness, and environmental inclusiveness. Specifically, economic inclusiveness is measured by per capita GDP, GDP growth rate, the ratio of per capita disposable income between urban and rural residents, and the end-of-year balance of urban and rural residents' savings; social inclusiveness is assessed through the number of hospital beds per 10,000 people, books per 10,000 people in public libraries, the number of theaters and cinemas per 10,000 people, the number of participants in basic pension insurance for urban workers, basic medical insurance, and unemployment insurance; environmental inclusiveness is gauged by industrial sulfur dioxide emissions, industrial wastewater discharge, industrial particulate emissions, comprehensive utilization rate of general industrial solid waste, harmless treatment rate of domestic garbage, and centralized treatment rate of wastewater treatment plants. In this paper, we refer to the practice of Zou et al. (2023) to calculate the inclusive green growth index by using the entropy weight TOPSIS method. The entropy weight TOPSIS method is divided into two stages, firstly, the entropy weight method calculates the weight of each evaluation index, and multiplies the evaluation index data with the weight to get the weight matrix, and then the weight matrix is calculated by the TOPSIS method to get the value of
(1) We first obtain the initial matrix
(2) The standardisation matrix
(3) Determine the information entropy (
In Equation 5,
(4) Determine the weight of evaluation indicator
(5) The weighted decision matrix for the indicators in year t is shown in Equation 7.
TOPSIS method is a multi-criteria decision-making technique (Li et al., 2018). The method selects the optimal solution by constructing ideal solutions for and against the evaluation object, calculating the relative proximity of each solution to the ideal solution, and ranking the solutions. In this study, based on the matrix
The positive ideal scenario
The Euclidean distance was used to calculate the distance between the impact indicators and the positive and negative ideal solutions for each province (Chen et al., 2022). The distances
Finally, the inclusive green growth index
3.2.2 Independent variable
Low-Carbon City Pilot Policy (Lcc_pilot). This paper matches the lists of the three batches of low-carbon city pilots announced by the National Development and Reform Commission with the data from each city, combining the timing of the pilot city designations to construct a dummy variable for the low-carbon city pilot policy (Lcc_pilot). It should be noted that once a city is designated as a pilot city for low-carbon development in a given year, it is considered a low-carbon city pilot for all subsequent years.
3.2.3 Control variables
In this study, based on the existing literature and theoretical framework, population size (Pop), urbanisation level (Urb), information technology level (Info), and consumption level of residents (Con) are selected as control variables, covering economic, social and technological factors. The selection of these control variables not only captures the impact of low-carbon city building on inclusive green growth more comprehensively, but also effectively reduces omitted variable bias. Specifically, (1) Population size (Pop). Large-scale population agglomeration usually increases resource consumption and carbon emissions, thus posing challenges to the sustainable development of cities (Jones and Kammen, 2011). Therefore, when studying the impacts of low-carbon city building, it is necessary to include population size as a control variable to avoid the interference of population size changes on the results. In addition, population size is also closely related to various aspects such as economic growth and infrastructure development (Golley and Zheng, 2015). (2) Urbanisation level (Urb). The level of urbanisation is an important indicator of the urban development process, which broadly affects resource use efficiency and carbon emission levels. It has been shown that as the urbanisation process advances, land use efficiency and resource integration capacity increase, but it is also accompanied by an increase in energy consumption and environmental pressure (Liu et al., 2016). Including the level of urbanisation as a control variable helps to remove the potential impact of urbanisation process on inclusive green growth, thus better identifying the role of low-carbon urban policies. (3) Informatisation level (Info). Informatisation level reflects the city’s development in information and communication technology (ICT). Information technology plays a key role in promoting the application of green technology and improving the efficiency of resource utilisation (Li et al., 2023). The improvement of information technology can not only reduce carbon emissions in traditional industries, but also promote the innovation and application of new energy technologies, which has an important impact on the green development of the city. (4) Consumption level of residents (Con). The consumption level of residents is an important indicator reflecting the living standard and consumption ability of urban residents, which has a significant correlation with energy demand and carbon emissions, and the consumption level also reflects the demand of urban residents for green products and services.
3.2.4 Mechanism variables
First, for H1, the number of patents applied is chosen as a proxy variable for measuring technological innovation in the city (Urban_tecinno). Secondly, for H2, the proportion of added value of tertiary industry to GDP was used as a proxy variable to measure the rationalisation level of the city’s industrial structure (Indust_strura). Finally, for H3, the number of times’environmental protection’appears in the government work report is calculated as a proxy variable for government leadership behaviour (Gover_leadbe), and this variable data comes from the annual work report of each city government online, we use python based on jieba thesaurus for keyword extraction and word frequency statistics. This is because if the government sets strong and clear goals for what needs to be achieved in local environmental governance, the expression of this willpower will be released through authoritative channels, such as public government documents such as annual programme reports (Tie et al., 2020).
3.3 Data sources and descriptive statistical analysis
The data used in this article are derived from the “China City Statistical Yearbook,” the China Economic and Social Big Data Research Platform, and the EPS database. Missing data were supplemented through the statistical yearbooks of various prefecture-level cities and linear interpolation. Considering that the years 2006–2021 encompass three crucial developmental phases in China—namely the Eleventh, Twelfth, and Thirteenth Five-Year Plans—this study collected balanced panel data for 279 prefecture-level cities from 2006 to 2021. The research sample excludes the regions of Hong Kong, Macau, Taiwan, the Tibet Autonomous Region, and cities with severe data deficiencies. Additionally, logarithmic transformations were applied to the dependent variables, control variables, and mechanism variables to maintain model simplicity and interpretative power, while also avoiding the issues of multicollinearity and data non-stationarity that can arise from excessive complexity. Table 1 reports the descriptive analysis results of the main variables.
4 Results
4.1 Benchmark regression
The baseline regression results are presented in Table 2. Models (1) to (5) conducted regressions by progressively adding control variables to validate the policy effects of low-carbon city development on inclusive green growth. It was observed that the regression coefficient for
4.2 Sensitivity analysis
To ensure the reliability of the benchmark regression conclusions, this study conducts sensitivity analyses from multiple dimensions to eliminate the interference of random factors. First, the results of the parallel trend test indicate that prior to the implementation of the LCCPP, the levels of inclusive green growth in pilot cities and non-pilot cities met the common trend assumption, with significant differences only emerging after the policy was enacted (Figure 3). This provides a prerequisite guarantee for the applicability of the difference-in-differences method. Secondly, a placebo test constructed with 500 virtual policy time points reveals that the pseudo-estimated coefficients are densely distributed around zero, with significance levels far lower than the actual estimates (Figure 4), confirming the statistical significance of the policy effects as non-random. Furthermore, considering the potential non-random selection bias in the choice of pilot cities, this paper re-estimates using the propensity score matching difference-in-differences method (PSM-DID). The results show that after controlling for covariates such as urban economic scale, industrial structure, and resource endowment, the policy effect remains robust (Table 3). Additionally, by excluding special samples such as municipalities directly under the central government and special economic zones, and by removing the overlapping effects of “smart city” pilot policies within the same period, it was found that the direction and significance level of the core explanatory variable coefficients did not undergo substantial changes, as shown in Table 4. The consistency of the series of test results indicates that the mechanism through which the low carbon city policy promotes inclusive green growth is highly robust, and the benchmark regression conclusions are not affected by model specification bias, sample selection bias, or exogenous policy shocks.
4.2.1 Parallel trends test
Prior to the implementation of the low-carbon city pilot policy, the levels of inclusive green growth in both pilot and non-pilot cities should exhibit similar trends. Therefore, according to Equation 12, the specific regression model setup is as follows:
The model adopts a dynamic distributed lag design, systematically evaluating the time-varying effects of the low-carbon city pilot policy (Lcc_pilot) on inclusive green growth (Igg) by introducing 15 annual dummy variables (from 3 years before to 11 years after the policy implementation) and interaction terms with the treatment group dummy variable. The coefficients for the first three periods before the policy (t = −3 to t = −1) are used to validate the parallel trend assumption, while the coefficients for the policy period (t = 0) and the subsequent 11 periods (t = 1 to t = 11) reveal the dynamic evolution path of the policy effects. The control variable set (
4.2.2 Placebo test
In order to rigorously rule out the influence of potentially unobservable confounding factors, we conducted placebo tests to verify if the increase in inclusive green growth is indeed driven by low-carbon city construction rather than by random chance. Specifically, we randomly reassigned the policy implementation years within our sample cities and re-estimated the baseline regression model 500 times. Figure 3 visually summarizes the results of these placebo tests through the probability density distributions of estimated coefficients and their P-values. The kernel density plot for these placebo regression coefficients closely approximates a normal distribution centered around zero, implying no substantial false positive tendency. The majority of the placebo-derived coefficients are statistically insignificant (with their P-values greater than 10%), suggesting minimal risk of identifying spurious policy effects. Importantly, none of these coefficient estimates exceeds the actual policy effect size obtained from our benchmark regression (0.005), as highlighted by the vertical dashed reference line. Overall, these findings confirm that the previously identified significant baseline results are robust and not merely attributable to random factors, lending further credibility to our empirical conclusions.
4.2.3 PSM-DID
Considering that there may still be some selection bias in practical operations, namely, that the government’s selection of pilot cities may involve a “special list,” the assumption of random grouping may not be strictly satisfied. Directly comparing differences between pilot and non-pilot cities could involve selection bias. Therefore, this paper employs the propensity score matching (PSM) method proposed by Heckman et al. (1997) to address this issue. Specifically, to obtain each city’s propensity score, a logit regression is conducted on the binary variable indicating whether a city is a low-carbon city construction pilot (
4.2.4 Robustness tests on special samples and other policy influences
(1) Excluding Special Cases. To further verify the robustness of the research conclusions, two strategies were employed to eliminate special cases: Strategy 1 considers the administrative level and economic development of Beijing, Tianjin, Shanghai, and Chongqing, which are significantly higher than other cities, thus samples from these four municipalities were excluded. Strategy 2 involves trimming 1% of the tails from both the dependent variable and control variables before regression to eliminate the impact of extreme values. As shown in columns (1)–(2) of Table 4, excluding these special cases did not significantly alter the conclusions of this paper.
(2) Considering Other Concurrent Policies. In assessing the policy effects of constructing low-carbon cities on inclusive green growth, it is necessary to exclude the influence of other concurrent pilot policies. China implemented pilot programs for “Smart Cities,” “Broadband China,” and “National Big Data Comprehensive Experimental Zones” in 2012, 2014, and 2015 respectively. For this purpose, dummy variables for “Broadband China Pilot (Bc_pilot),” “Smart City Pilot (Sc_pilot),” and “National Big Data Comprehensive Experimental Zone Pilot (Bd_pilot)” were established and included in the regression equations. Results from Model (3)–(6) in Table 4 indicate that after removing the influence of concurrent policies, the baseline regression conclusions remain robust.
5 Discussion
5.1 Heterogeneity test
Table 5 demonstrates the heterogeneous impacts of low-carbon city building in different types of cities, revealing that policy effects vary according to city characteristics. These differences reflect the complexity and diversity of low-carbon transitions in different economic and social structures and provide important insights into the effectiveness of policies.
5.1.1 Heterogeneity analyses based on the administrative level of cities
The policy effects of low-carbon city building on inclusive green growth are all characterised by significant heterogeneity, which necessitates an in-depth discussion. In this paper, municipalities directly under the central government, sub-provincial cities and provincial capitals are classified as central cities, and other prefectural cities are classified as non-central cities, in order to reveal the impacts of resource and policy tilting, and the comparative results are shown in Table 5 model (1)–(2). Centre cities are usually the areas where economic and policy resources are concentrated, and low carbon city building produces a significant positive effect in these cities. The administrative level of cities serves as an effective proxy for policy effect heterogeneity because it institutionally determines resource allocation priorities and policy implementation capacities in China’s governance system. Central cities (municipalities, sub-provincial cities, and provincial capitals) inherently possess superior political status that grants them preferential access to fiscal transfers, innovative talent pools, and pilot policy authorizations from central/provincial governments. This hierarchical advantage enables them to secure stronger financial backing, accelerate technological adoption, and implement low-carbon initiatives through streamlined administrative coordination factors critically reflected in their enhanced inclusive green growth outcomes. Conversely, non-central cities face structural constraints in resource acquisition and policy flexibility due to their lower administrative ranking, resulting in delayed infrastructure upgrades and fragmented industrial transitions despite identical policy mandates. The administrative hierarchy thus fundamentally shapes cities' institutional capacity to convert low-carbon policies into tangible green growth outcomes through its embedded mechanisms of resource concentration and governance efficiency differentiation.
5.1.2 Heterogeneity analysis based on urban resource endowment
Based on the National Sustainable Development Plan for Resource-Based Cities (2013–2020) issued by the State Council, the sample cities are divided into resource-based cities and non-resource-based cities, and the comparison results are shown in Table 5 Models (3)–(4). It can be found that resource-based cities rely on traditional high-carbon emission industries, such as coal and oil, and therefore face greater structural challenges in promoting green transformation. However, the data in the table suggests that resource-based cities show a significant increase in green growth driven by low-carbon policies. This may be due to the fact that these cities have accelerated their industrial restructuring under the pressure of low-carbon policies, and have taken the initiative to reduce their reliance on highly polluting industries in favour of greener alternatives, such as renewable energy and energy-saving technologies. The remarkable results of resource-based cities in building low-carbon cities reflect the huge potential for policy transformation in these ‘high-emission towns’. In contrast, non-resource cities, which rely less on traditional industries with high carbon emissions, have seen a relatively small marginal effect of green transformation, which may indicate that these cities already have a certain foundation for green development and that the additional effect of low-carbon policies is relatively limited.
5.1.3 Heterogeneity analysis based on the carbon emission reduction potential of cities
We measure the carbon emission reduction potential of a city based on the ratio of carbon dioxide emissions to GDP. If this ratio in a given year exceeds the national median, it indicates relatively low carbon emission efficiency for that city, and hence it is classified as having high carbon emission reduction potential. Otherwise, the city is categorized as having low carbon emission reduction potential. Estimation results reported in Table 5 [Models (5)–(6)] present the heterogeneous impacts of city-specific characteristics on the marginal effects from low-carbon policies. As expected, the policy effects are more pronounced in cities assessed as having high carbon emission reduction potential. Typically, these cities are facing greater pressures to control emissions and thus can realize more significant carbon reductions and substantial economic restructuring when transitioning into low-carbon cities. Consequently, introduction of policies promoting low-carbon city construction has provided strong transformative motivation to these cities, leading to a notable increase in inclusive green growth. By contrast, the policy impact is comparatively moderate in cities classified as having low carbon emission reduction potential, although still positive. This is likely because these cities already possess a relatively solid foundation in emission efficiency and green growth. Therefore, the incremental stimulus provided by additional policy intervention tends to be smaller. For these cities, low-carbon policies primarily serve to reinforce and sustain gains from their previously established green developments, rather than significantly transforming their current economic structures.
The heterogeneity analysis indicates that the effectiveness of low-carbon city policies varies significantly depending on cities’ emission intensity, economic structures, and resource endowments. Generally, policy effects are found to be stronger in high-emission, resource-based or central cities, suggesting that these cities pose greater potential and need greater policy-driven transformation in industrial and technical structures to achieve inclusive green growth targets. By contrast, lower-emission, non-resource-based or peripheral cities, which already offer more favourable baseline conditions and existing green infrastructure, tend to benefit modestly, mainly through policy guidance and demonstration effects that consolidate their advantages. These differentiated patterns underscore that policymakers must carefully consider each city’s unique context, tailoring policies and resource allocations accordingly. By precisely targeting policies in accordance with urban heterogeneity criteria, authorities may optimize the effectiveness of low-carbon policies, contributing to the achievement of coordinated regional green development and long-term sustainability. Similar to recent integrated multidimensional assessments [such as Peng et al. (2024)], our results emphasize the necessity of evaluating sustainability-related issues from multiple perspectives to more precisely implement urban sustainable policies.
5.2 Mechanism test
The empirical results confirm that advancing the construction of low-carbon cities can significantly promote inclusive green growth. Further discussion will be made on the transmission mechanism of this policy effect. This paper draws on the mediation effect model proposed by Jiang (2022) to construct the following test equation. The test results are presented in Table 6.
In Equation 13,
5.2.1 The mediating role of urban technological innovation
From the perspective of urban technological innovation, the results in the column (1) indicate that the construction of low-carbon cities significantly promotes urban technological innovation. Low-carbon city pilot projects not only advance the implementation of environmental protection policies but also enhance the overall innovation capacity of cities by encouraging technological research and application. This aligns with the spatial resonance of the policy pilot diffusion theory proposed by Zou et al. (2022), which suggests that local governments transform the construction of low-carbon cities into a competitive arena for technological innovation through an internal championship mechanism. Urban technological innovation is the core driving force behind low-carbon transformation, especially in the context of intensified global technological competition and increasing demands for environmental protection. The enhancement of urban technological innovation provides strong momentum for the transition to a green economy.
5.2.2 The mediating role of industrial structure optimization
Columns (2) confirm that optimizing industrial structure is another key pathway to achieving inclusive green growth in the construction of low-carbon cities. The results indicate that the development of low-carbon cities significantly promotes the upgrading and optimization of industrial structures. By reducing reliance on traditional high-pollution and high-energy-consuming industries, low-carbon policies accelerate the emergence of green industries and foster the development of emerging sectors such as clean energy and green manufacturing. The pathway through which industrial structure upgrading drives the development of green industries aligns closely with the research by Zeng et al. (2023). Furthermore, empirical studies by Yang et al. (2019) demonstrate that the construction of low-carbon cities facilitates economic green transformation through the cultivation of emerging high-tech industries, such as environmental protection and new energy sectors. This finding complements the analysis in column (3) regarding the mechanism by which the rise of emerging industries enhances resource utilization efficiency. Additionally, the dual approach proposed by Zheng et al. (2021)—eliminating outdated capacity through market mechanisms and embedding low-carbon standards in the planning phase—has been concretized in the policy effects of this study. Local governments are not only compelled to transform traditional industries through carbon trading mechanisms but also raise the entry thresholds for heavily polluting industries at the urban planning level, reflecting the connection between theory and policy practice. The optimization of industrial structure has a very significant impact on inclusive green growth, indicating that the transformation and upgrading of industrial structures are one of the key driving factors of green growth. This may be attributed to the fact that, on one hand, optimizing industrial structure promotes high-quality population agglomeration while accelerating the development of smart industries and the digital economy Ren et al. (2022).
5.2.3 The mediating role of government leadership behavior
Finally, government leadership plays a crucial role in promoting the construction of low-carbon cities and inclusive green growth. Columns (3) confirm that the development of low-carbon cities significantly influences government leadership behavior, while active government participation and guidance notably foster inclusive green growth. This aligns closely with the logic revealed by Hui et al. (2016) regarding “low-carbon pilot projects triggering a competition for policy innovation among local governments” —even under the pressure of GDP assessments, local governments demonstrate their ability to innovate beyond traditional paths through practical actions such as setting industry access thresholds and investing in green infrastructure. Additionally, government policy signals are key in managing market expectations and creating a stable policy environment for green economic activities. The result also supports the direct impact of government leadership on inclusive green growth, indicating that in the implementation of low-carbon policies, the government serves not only as a policymaker but also as a guarantor of policy effectiveness. This echoes Tie et al. (2020), who argue that low-carbon pilot policies compel local governments to shift from being “passive responders” to “active innovators,” ultimately advancing green transformation by enhancing their environmental regulatory capabilities and guiding the public and market.
5.3 Spatial effects test
To capture the multidimensional spatial spillover effects of Low-Carbon City Pilot policies (LCCPP) on Inclusive Green Growth (Igg), this study employs a tripartite spatial econometric framework incorporating three model specifications and three spatial weight matrices. The Spatial Error Model (SEM) accounts for unobserved spatial correlation in residuals, the Spatial Lag Model (SLM) examines spillovers through endogenous interaction effects, and the Spatial Durbin Model (SDM) simultaneously considers both endogenous interaction and exogenous spillovers from explanatory variables. These models are estimated using: (1) an inverse distance matrix reflecting geographic proximity decay, where spatial dependence decreases with physical distance; (2) an economic distance matrix calibrated by per capita GDP disparities, capturing core-periphery economic linkages; and (3) an economic-geographic nested matrix combining both dimensions to identify synergistic spatial effects. Through comparative analysis across these model-matrix combinations, the research systematically verifies the existence, direction, and transmission channels of LCCPP’s spatial spillovers while controlling for spatial autocorrelation biases. The results reveal how technological diffusion and regulatory convergence in pilot cities generate cross-border growth externalities through geographically bounded knowledge spillovers and economically driven factor mobility, providing empirical foundations for coordinated regional decarbonization strategies.
5.3.1 Spatial correlation results
In order to comprehensively assess the impact of low-carbon city building on inclusive green growth, it is first necessary to test whether there is a spatial correlation of inclusive green growth between regions. Spatial correlation implies that a city’s inclusive green growth is not only influenced by its own economic, technological and policy factors, but may also have some correlation with the level of economic development and policy implementation of neighbouring cities. In other words, inclusive green growth among cities may not occur in isolation, but rather spatial dependence through interregional interactions and linkages. The results are shown in Table 7.
It can be seen that the Moran’s I under all three weight matrices is positive and the p-value is less than 0.001 in all years, indicating that there is a significant positive spatial correlation of the inclusive green growth index in all years and under all matrices. This spatial positive correlation implies that there is a linkage effect between a city’s level of inclusive green growth and its neighbouring cities. Specifically, if a city achieves a high level of inclusive green growth in a given year, its neighbouring cities tend to show similar growth trends.
The Moran’s I (column 1) of the inverse distance matrix is relatively small, usually ranging from 0.046 to 0.059, reflecting the fact that the spatial positive correlation of inclusive green growth between regions weakens as distance increases. This result is consistent with the classical theory of spatial economics that the closer the geographical distance, the closer the economic activities, policy diffusion and technology sharing between cities. Thus, under the inverse distance matrix, while the correlation exists, this spatial dependence is more limited, suggesting that spatial interactions occur mainly between relatively close regions.
The economic distance matrix (Column 3) shows a relatively high Moran’s I, with values typically ranging from 0.238 to 0.332. This suggests that the higher the economic similarity between cities, the stronger the spatial dependence of their inclusive green growth performance. That is, cities with similar economic conditions are more likely to influence each other on their green development paths. This may be due to the fact that these cities face similar economic structures and development needs, and are therefore more likely to achieve shared green growth through policy coordination, industrial cooperation and technology diffusion. This higher Moran index highlights the important role of economic linkages in promoting green development between regions, suggesting that policy influence may not be limited to geographic proximity, but is also closely related to similarity in economic structure.
The Moran’s I for the economic-geographical nested matrix (column 5) falls somewhere in between, usually between 0.069 and 0.084. This result reflects the interaction of the dual dimensions of economy and geography, taking into account both geographic proximity and economic linkages. This matrix reveals that the spatial relevance of inclusive green growth is stronger in groups of cities that are both economically and geographically interconnected. It can be understood that such cities are not only relatively close geographically, but also have stronger linkages in terms of economic interactions, policy implementation and resource sharing. Thus, economic and geographic nesting effects combine to drive synergies on inclusive green growth in these cities.
Figure 4 illustrates the spatial correlation of the inclusive green growth index in 2006 and 2021 under three different weighting matrices. Overall, the inverse distance matrix, the economic distance matrix and the economic-geographical nested matrix reveal the linkage effects of inclusive green growth between cities under geographical, economic and combined factors, respectively. Under the inverse distance matrix, the Moran’s I is relatively low in 2006 and 2021, reflecting some positive correlation between geographically neighbouring cities, but this correlation is weak. The scatter is concentrated near the origin, suggesting that most cities have more similar levels of growth to their neighbours, but the strength of spatial interaction is lower. In contrast, the economic distance matrix shows a higher Moran’s I, suggesting a stronger green growth linkage effect between cities with similar economic conditions. Cities with similar economies are more likely to show consistent green development trends, both in 2006 and 2021, which may be due to the fact that similar industrial structures, policy orientations, and levels of economic development allow these cities to show greater synergies in the implementation of low-carbon policies. However, the linkage effect between economically similar cities is still significant, even though the Moran’s I of the economic distance matrix decreases in 2021 compared to 2006. The economic-geographical nested matrix, on the other hand, combines economic and geographical factors to reflect the dual spatial and economic linkages between cities. Under this matrix, the spatial correlation exhibits a moderate level and is relatively stable, suggesting that economically and geographically similar cities play complementary roles in promoting green development. Overall, these scatter plots reveal the dependence of inclusive green growth on the spatial dimension, i.e., the green growth of cities is influenced not only by their own policies and economic conditions, but also by their neighbouring cities, especially those that are more economically similar.
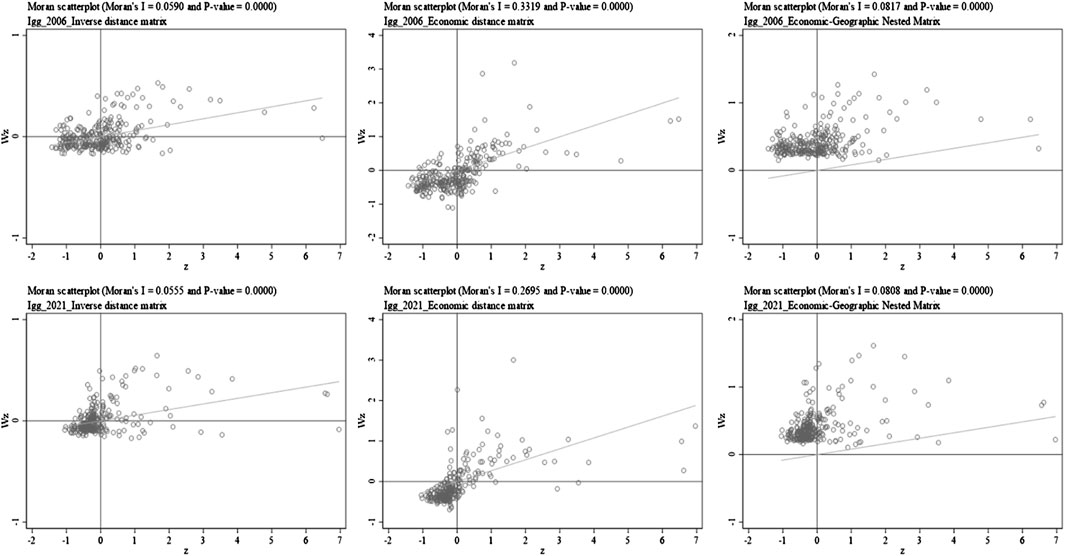
Figure 4. Scatterplot of localised Moran’s I under three weighting matrices (2006, 2021). Note: The horizontal axis (z) denotes the standardized value of the dependent variable after standardization. The vertical axis (Wz) represents the standardized spatial lagged variable value of the dependent variable, which is calculated from the given spatial weight matrix.
5.3.2 Spatial effects results
Table 8 shows the regression results of low carbon city building on inclusive green growth index under three different weight matrices, namely, inverse distance matrix, economic distance matrix, and economic-geographical nested matrix, and three spatial models, namely, spatial error model (SEM), spatial lag model (SLM), and spatial Durbin model (SDM), which reveal the direct and spatial effects of low carbon city building on inclusive green growth. From the results, no matter which matrices and models are chosen, the direct effect of low-carbon city construction on inclusive green growth is always significant, and the main effect coefficient is positive and significant in all models, about 0.005, indicating that low-carbon policy effectively promotes inclusive green growth in the areas where the policy is implemented.
However, the results show a more mixed case when discussing spatial effects. First, while there is spatial dependence, particularly in the spatial error and lag models, and the spatial coefficients are positive and significant under all matrices, suggesting that there is indeed some spatial correlation in the inclusive green growth index between cities, this correlation is more in the form of growth synergies between similar cities than spillover effects through policy diffusion. Specifically, the spatial lag term under the inverse distance matrix and the economic-geographic nested matrix is not significant in the spatial Durbin model, and even presents a negative value under the inverse distance matrix, implying the failure to form significant positive policy interactions between neighbouring cities. The spillover effect under the economic distance matrix, although positive in the SDM model, is still insignificant, suggesting that the diffusion effect of low-carbon policies between cities fails to be significant even if the economic structures are similar. The total effect (LR_Total) is more significant in the spatial lag model, especially under the inverse distance and economic distance matrices, which shows that the total effect includes a strong direct effect, but the indirect effect is more limited, and the spatial spillover effect does not appear. The positive effects of low-carbon policies are mainly reflected in the areas where the policies are implemented, and are not effectively transmitted to neighbouring areas, indicating that even under conditions of geographical proximity or economic similarity, the cross-regional effects of the policies are limited by the differences in resources, policy implementation capacity and economic structure between cities. This result reveals the limitations of low-carbon policies, which, despite their contribution to green growth in individual cities, lack significant regional diffusion effects, suggesting that future policy design may need to focus more on interregional synergies in order to achieve broader policy spillovers and cross-regional development effects.
6 Conclusion and policy recommendations
This study constructs a comprehensive evaluation system for inclusive green growth and reveals the driving effect of China’s low-carbon city pilot policy on inclusive green growth and its mechanisms based on a multi-period difference-in-differences (DID) approach. The research findings are as follows: (1) The construction of low-carbon cities significantly promotes inclusive green growth. This conclusion remains robust under various sensitivity analyses. (2) Low-carbon city construction enhances the level of inclusive green growth in pilot cities through three pathways: stimulating urban technological innovation, optimizing industrial structure, and strengthening government leadership behavior. This effect is particularly pronounced in central cities, resource-based cities, and high carbon abatement potential cities. (3) Furthermore, although spatial econometric tests indicate a positive spatial correlation in green growth among cities, the policy effects are primarily localized, showing no significant spillover effects to neighboring cities.
To improve the practicality of policy recommendations, we distinctively propose strategies targeted towards national governments, local governments, and the private sector, respectively. At the national level, policymakers can establish provincial-level “Low-Carbon Governance Alliances” inspired by the EU’s cross-regional cooperation on carbon adjustment mechanisms, facilitating unified carbon emission trading and ecological compensation across jurisdictions. At the local-government level, cities can collaborate to form regional green technology transfer centers modeled after the Fraunhofer Institute approach in Germany, accelerating innovation diffusion through patent sharing pools and risk-sharing mechanisms. Moreover, regional governments could draw lessons from Kitakyushu Eco-Town in Japan and collectively develop “Green Industry Maps,” cultivating intercity networks based on integrated “R&D–Manufacturing–Recycling” chains. At the private-sector level, businesses in high-emission and resource-based cities can be incentivized by tailored financing programs (e.g., capacity replacement funding) and expanded green-certificate trading schemes. Together, these tiered interventions support the formation of replicable Regional Green Growth Partnerships that enhance inclusive green development nationwide.
This paper has some limitations that need to be addressed. First, while the control variables were carefully selected based on theoretical frameworks and data availability, the complexity of green growth determinants suggests the potential value of incorporating additional socio-technical factors. Future studies could expand the analytical framework by integrating cross-disciplinary datasets, particularly in areas such as educational attainment gradients and transportation infrastructure spatial networks. Second, considering data availability and completeness, this study only selected key indicators to measure the main characteristics of inclusive green growth. Future research could incorporate more indicators to supplement and improve the current evaluation system. Third, this study simplified the mediation effect model process and attempted to use new testing methods. Future research could consider applying more advanced mediation effect testing models combined with machine learning techniques to improve the robustness of the mechanism analysis conclusions.
Data availability statement
The original contributions presented in the study are included in the article/supplementary material, further inquiries can be directed to the corresponding author/s.
Author contributions
XM: Conceptualization, Data curation, Formal Analysis, Investigation, Methodology, Writing – original draft, Writing – review and editing. TZ: Conceptualization, Methodology, Software, Validation, Writing – review and editing.
Funding
The author(s) declare that financial support was received for the research and/or publication of this article. Thanks to the support from the National Social Science Foundation of China (20BJL080); National Natural Science Foundation of China (71874119); Shanxi Province Science and Technology Strategic Research Special Project (202304031401016); Research on the Development Path of New Quality Productivity Based on the Theory of Productive Forces in “Capital” (2024ZZ012); Research on the Cultivation and Development of New Quality Productivity in Shanxi Province Based on Local Conditions (2024ZD020).
Conflict of interest
The authors declare that the research was conducted in the absence of any commercial or financial relationships that could be construed as a potential conflict of interest.
Generative AI statement
The author(s) declare that no Generative AI was used in the creation of this manuscript.
Publisher’s note
All claims expressed in this article are solely those of the authors and do not necessarily represent those of their affiliated organizations, or those of the publisher, the editors and the reviewers. Any product that may be evaluated in this article, or claim that may be made by its manufacturer, is not guaranteed or endorsed by the publisher.
References
Berkhout, E., Bouma, J., Terzidis, N., and Voors, M. (2018). Supporting local institutions for inclusive green growth: developing an Evidence Gap Map. J. Life Sc. 84, 51–71. doi:10.1016/j.njas.2017.10.001
Chen, H., Guo, W., Feng, X., Wei, W., Liu, H., Feng, Y., et al. (2021). The impact of low-carbon city pilot policy on the total factor productivity of listed enterprises in China. Resour. Conservation Recycl. 169, 105457. doi:10.1016/j.resconrec.2021.105457
Chen, Y., Li, Q., and Liu, J. (2024). Innovating sustainability: VQA-based AI for carbon neutrality challenges. J. Organ. End. User Com. 36 (1), 337606. doi:10.4018/JOEUC.337606
Chen, Y. L., Shen, S. L., and Zhou, A. (2022). Assessment of red tide risk by integrating CRITIC weight method, TOPSIS-ASSETS method, and Monte Carlo simulation. Environ. Pollut. 314, 120254. doi:10.1016/j.envpol.2022.120254
Cheng, J., Dai, S., and Ye, X. (2016). Spatiotemporal heterogeneity of industrial pollution in China. China Econ. Rev. 40, 179–191. doi:10.1016/j.chieco.2016.07.001
Cheng, J., Yi, J., Dai, S., and Xiong, Y. (2019). Can low-carbon city construction facilitate green growth? Evidence from China's pilot low-carbon city initiative. J. Clean. Prod. 231, 1158–1170. doi:10.1016/j.jclepro.2019.05.327
Fan, S., Huang, H., Mbanyele, W., Guo, Z., and Zhang, C. (2023). Inclusive green growth for sustainable development of cities in China: spatiotemporal differences and influencing factors. Environ. Sci. Pollut. R. 30 (4), 11025–11045. doi:10.1007/s11356-022-22697-3
Golley, J., and Zheng, W. (2015). Population dynamics and economic growth in China. China Econ. Rev. 35, 15–32. doi:10.1016/j.chieco.2015.05.005
Gu, K., Dong, F., Sun, H., and Zhou, Y. (2021). How economic policy uncertainty processes impact on inclusive green growth in emerging industrialized countries: a case study of China. J. Clean. Prod. 322, 128963. doi:10.1016/j.jclepro.2021.128963
Heckman, J. J., Ichimura, H., and Todd, P. E. (1997). Matching as an econometric evaluation estimator: evidence from evaluating a job training programme. Rev. Econ. Stud. 64 (4), 605–654. doi:10.2307/2971733
Hui, Z., Xufeng, Z., and Ye, Q. (2016). Fostering local entrepreneurship through regional environmental pilot schemes: the low-carbon development path of China. China Int. J. 14 (3), 107–130. doi:10.1353/chn.2016.0026
Jiang, T. (2022). The mediating and moderating effects in empirical research on causal inference. China Ind. Econ. (05). doi:10.19581/j.cnki.ciejournal.2022.05.005
Jones, C. M., and Kammen, D. M. (2011). Quantifying carbon footprint reduction opportunities for US households and communities. Environ. Sci. Technol. 45 (9), 4088–4095. doi:10.1021/es102221h
Li, G., Gao, D., and Shi, X. X. (2023). How does information and communication technology affect carbon efficiency? Evidence at China's city level. Energ. Environ. 35, 4272–4293. doi:10.1177/0958305X231156405
Li, Z., Bai, T., and Tang, C. (2022). How does the low-carbon city pilot policy affect the synergistic governance efficiency of carbon and smog? Quasi-experimental evidence from China. J. Clean. Prod. 373, 133809. doi:10.1016/j.jclepro.2022.133809
Li, Z., Yang, T., Huang, C.-S., Xu, C.-Y., Shao, Q., Shi, P., et al. (2018). An improved approach for water quality evaluation: TOPSIS-based informative weighting and ranking (TIWR) approach. Ecol. Indic. 89, 356–364. doi:10.1016/j.ecolind.2018.02.014
Liu, F. (2023). The impact of China’s low-carbon city pilot policy on carbon emissions: based on the multi-period DID model. Environ. Sci. Pollut. R. 30 (34), 81745–81759. doi:10.1007/s11356-022-20188-z
Liu, K., Luo, J., Faridi, M. Z., Nazar, R., and Ali, S. (2025). Green shoots in uncertain times: decoding the asymmetric nexus between monetary policy uncertainty and renewable energy. Energ. Environ. doi:10.1177/0958305X241310198
Liu, X., and Xu, H. (2022). Does low-carbon pilot city policy induce low-carbon choices in residents' living: holistic and single dual perspective. J. Environ. Manage. 324, 116353. doi:10.1016/j.jenvman.2022.116353
Liu, Y., Yan, B., and Zhou, Y. (2016). Urbanization, economic growth, and carbon dioxide emissions in China: a panel cointegration and causality analysis. J. Geogr. Sci. 26, 131–152. doi:10.1007/s11442-016-1259-2
Liu, Z., Li, R., Zhang, X. T., Shen, Y., Yang, L., and Zhang, X. (2021). Inclusive green growth and regional disparities: evidence from China. Sustainability 13 (21), 11651. doi:10.3390/su132111651
Ma, Q., Zhang, Y., Hu, F., and Zhou, H. (2024). Can the energy conservation and emission reduction demonstration city policy enhance urban domestic waste control? Evidence from 283 cities in China. Cities 154, 105323. doi:10.1016/j.cities.2024.105323
McGee, T. G., and Mori, K. (2021). The management of urbanization, development, and environmental change in the megacities of Asia in the twenty-first century. Living megacity Towards Sustain. urban Environ., 17–33. doi:10.1007/978-4-431-56901-5_2
NBSC (2024). Statistical Bulletin on national economic and social development of the People's Republic of China 2023. Available online at: https://www.stats.gov.cn/sj/zxfb/202402/t20240228_1947915.html.
Peng, L., Yan, X., Jiang, Z., Yan, Z., and Xu, J. (2023). From pilots to demonstrations: the green economic development effect of low-carbon city pilot policies. Environ. Sci. Pollut. R. 30 (22), 62376–62396. doi:10.1007/s11356-023-26402-w
Peng, Q., He, W., Kong, Y., Shen, J., Yuan, L., and Stephen Ramsey, T. (2024). Spatio-temporal analysis of water sustainability of cities in the Yangtze River Economic Belt based on the perspectives of quantity-quality-benefit. Ecol. Indic. 160, 111909. doi:10.1016/j.ecolind.2024.111909
Peng, Y., and Bai, X. (2018). Experimenting towards a low-carbon city: policy evolution and nested structure of innovation. J. Clean. Prod. 174, 201–212. doi:10.1016/j.jclepro.2017.10.116
Ren, S., Li, L., Han, Y., Hao, Y., and Wu, H. (2022). The emerging driving force of inclusive green growth: does digital economy agglomeration work? Bus. Strateg. Environ. 31 (4), 1656–1678. doi:10.1002/bse.2975
Said, Z. M., and Dindar, S. (2024). Key challenges and strategies in the evaluation of sustainable urban regeneration projects: insights from a systematic literature review. Sustainabilit 16 (22), 9903. doi:10.3390/su16229903
Shan, Y., Fang, S., Cai, B., Zhou, Y., Li, D., Feng, K., et al. (2021). Chinese cities exhibit varying degrees of decoupling of economic growth and CO2 emissions between 2005 and 2015. One Earth 4 (1), 124–134. doi:10.1016/j.oneear.2020.12.004
Shobande, O. A., and Asongu, S. A. (2023). Searching for sustainable footprints: does ICT increase CO2 emissions? Environ. Model. Assess. 28 (1), 133–143. doi:10.1007/s10666-022-09859-w
Shobande, O. A., and Ogbeifun, L. (2022). Has information and communication technology improved environmental quality in the OECD? -a dynamic panel analysis. Int. J. Sust. Dev. World 29 (1), 39–49. doi:10.1080/13504509.2021.1909172
Shobande, O. A., Ogbeifun, L., and Tiwari, A. K. (2024). Extricating the impacts of emissions trading system and energy transition on carbon intensity. Appl. Energ. 357, 122461. doi:10.1016/j.apenergy.2023.122461
Shobande, O. A., Ogbeifun, L., and Tiwari, A. K. (2025). Carbon neutrality: synergy for energy transition, circular economy and inclusive green growth. J. Environ. Manage. 374, 124114. doi:10.1016/j.jenvman.2025.124114
Sun, H., Mao, W., Dang, Y., and Luo, D. (2022). What inhibits regional inclusive green growth? Empirical evidence from China. Environ. Sci. Pollut. R. 29 (26), 39790–39806. doi:10.1007/s11356-021-17250-7
Sun, Y. H., Ding, W. W., Yang, Z. Y., Yang, G. C., and Du, J. T. (2020). Measuring China's regional inclusive green growth. Sci. Total Environ. 713, 136367. doi:10.1016/j.scitotenv.2019.136367
Tie, M., Qin, M., Song, Q., and Qi, Y. (2020). Why does the behavior of local government leaders in low-carbon city pilots influence policy innovation? Resour. Conservation Recycl. 152, 104483. doi:10.1016/j.resconrec.2019.104483
Trapp, C. T., and Kanbach, D. K. (2021). Green entrepreneurship and business models: deriving green technology business model archetypes. J. Clean. Prod. 297, 126694. doi:10.1016/j.jclepro.2021.126694
Ulucak, R. (2020). How do environmental technologies affect green growth? Evidence from BRICS economies. Sci. Total Environ. 712, 136504. doi:10.1016/j.scitotenv.2020.136504
Wang, L., Shao, J., and Ma, Y. (2023). Does China's low-carbon city pilot policy improve energy efficiency? Energy 283, 129048. doi:10.1016/j.energy.2023.129048
Wang, Y., Zhao, H., Duan, F., and Wang, Y. (2018). Initial provincial allocation and equity evaluation of China’s carbon emission rights—based on the improved TOPSIS method. Sustainability 10 (4), 982. doi:10.3390/su10040982
Wu, J., Lin, K., and Sun, J. (2023). Pressure or motivation? The effects of low-carbon city pilot policy on China’s smart manufacturing. Comput. Ind. Eng. 183, 109512. doi:10.1016/j.cie.2023.109512
Xu, A., Wang, W., and Zhu, Y. (2023). Does smart city pilot policy reduce CO2 emissions from industrial firms? Insights from China. J. Innovation and Knowl. 8 (3), 100367. doi:10.1016/j.jik.2023.100367
Xu, P., Jin, Z., and Wu, X. (2024a). Effect of green trade barriers on export enterprise green technological innovation from the perspective of the low-carbon city pilot policy. Front. Env. Sci-switz. 12, 1486855. doi:10.3389/fenvs.2024.1486855
Xu, X., Liu, Z., Liu, W., Pei, C., Wu, X., and Nie, Z. (2024b). A sustainable development benchmarking framework for energy companies based on topic mining and knowledge graph: the case of oil and gas industry. Renew. Sust. Energ. Rev. 196, 114350. doi:10.1016/j.rser.2024.114350
Yang, F., Shi, B., Xu, M., and Feng, C. (2019). Can reducing carbon emissions improve economic performance–evidence from China. Economics 13 (1), 20190047. doi:10.5018/economics-ejournal.ja.2019-47
Yang, X., Yang, X., Zhu, J., Jiang, P., Lin, H., Cai, Z., et al. (2023). Achieving co-benefits by implementing the low-carbon city pilot policy in China: effectiveness and efficiency. Environ. Technol. Inno. 30, 103137. doi:10.1016/j.eti.2023.103137
Yin, J., and Guo, J. (2022). Ecological effect assessment of low-carbon city construction in China. Int. J. Env. Res. Pub. He. 19 (21), 14467. doi:10.3390/ijerph192114467
Zeng, S., Jin, G., Tan, K., and Liu, X. (2023). Can low-carbon city construction reduce carbon intensity? Empirical evidence from low-carbon city pilot policy in China. J. Environ. Manage. 332, 117363. doi:10.1016/j.jenvman.2023.117363
Zeng, S., Su, B., Zhang, M., Gao, Y., Liu, J., Luo, S., et al. (2021). Analysis and forecast of China's energy consumption structure. Energ. Policy 159, 112630. doi:10.1016/j.enpol.2021.112630
Zhang, T., and Li, J. (2023). Network Infrastructure,Inclusive green Growth,and regional inequality:from CausalInference of Double machine learning. J. Quantitative and Technol. Econ. 40 (04), 113–135. doi:10.13653/j.cnki.jqte.20230310.005
Zhao, S., Zhang, L., Peng, L., Zhou, H., and Hu, F. (2024). Enterprise pollution reduction through digital transformation? Evidence from Chinese manufacturing enterprises. Technol. Soc. 77, 102520. doi:10.1016/j.techsoc.2024.102520
Zhao, X., Meng, X., Zhou, Y., and Li, P. (2020). Policy inducement effect in energy efficiency: an empirical analysis of China. Energy 211, 118726. doi:10.1016/j.energy.2020.118726
Zheng, J., Shao, X., Liu, W., Kong, J., and Zuo, G. (2021). The impact of the pilot program on industrial structure upgrading in low-carbon cities. J. Clean. Prod. 290, 125868. doi:10.1016/j.jclepro.2021.125868
Zhou, X., and Feng, C. (2017). The impact of environmental regulation on fossil energy consumption in China: direct and indirect effects. J. Clean. Prod. 142, 3174–3183. doi:10.1016/j.jclepro.2016.10.152
Zou, C., Huang, Y., Wu, S., and Hu, S. (2022). Does “low-carbon city” accelerate urban innovation? Evidence from China. Sustain. Cities Soc. 83, 103954. doi:10.1016/j.scs.2022.103954
Keywords: low-carbon city construction, inclusive green growth, difference-in-differences method, double machine learning, energy policy evaluation
Citation: Mu X and Zou T (2025) Policy mechanisms and spatial dynamics of low-carbon city construction in promoting inclusive green growth in China. Front. Environ. Sci. 13:1559528. doi: 10.3389/fenvs.2025.1559528
Received: 13 January 2025; Accepted: 14 April 2025;
Published: 28 April 2025.
Edited by:
Yuanjun Zhao, Nanjing Audit University, ChinaReviewed by:
Serdar Dindar, Yıldırım Beyazıt University, TürkiyeLiang Yuan, China Three Gorges University, China
Ying Zhu, Xi’an University of Architecture and Technology, China
Olatunji Shobande, University of Aberdeen, United Kingdom
Xianghua Yue, Xiangnan University, China
Guangyao Deng, Lanzhou University of Finance and Economics, China
Copyright © 2025 Mu and Zou. This is an open-access article distributed under the terms of the Creative Commons Attribution License (CC BY). The use, distribution or reproduction in other forums is permitted, provided the original author(s) and the copyright owner(s) are credited and that the original publication in this journal is cited, in accordance with accepted academic practice. No use, distribution or reproduction is permitted which does not comply with these terms.
*Correspondence: Tong Zou, em91dG9uZ3N0dWR5QDE2My5jb20=