- School of Internet Economics and Business, Fujian University of Technology, Fuzhou, China
Facing escalating climate challenges, the Chinese government has highlighted the importance of targeted industrial policy for driving low-carbon transformation within its Five-Year Plans, whose effectiveness warrants evaluation. This paper employs a fixed-effects model across provinces, industries, and years, adopting data from 36 sectors across 30 Chinese provinces from 1997 to 2019, to assess the impact of the targeted industrial policy on low-carbon transformation. We reveal that industries receiving targeted support exhibit a 9.2% higher low-carbon transformation index than those without. The effects of industrial policy on low-carbon transformation are more pronounced in the context of local government policy, recent Five-Year Plans, sectors with moderate to low technological complexity, and high-emission sectors. Mechanism analysis shows that industrial policy facilitates low-carbon transformation by structural effects and technological effects. These insights offer a foundation for refining industrial policy design and execution to achieve carbon neutrality goals and foster sustainable growth.
1 Introduction
Decarbonization has become a consensus direction in the global industrialization process and is the thematic essence of China’s new development philosophy (Stern and Xie, 2023). However, China’s industrial low-carbon transformation (LCT) still faces numerous difficulties and significant challenges (Lin and Guan, 2023). According to data from the China Carbon Emission Accounting Database (CEADs), China’s industrial sectors saw a 3-fold increase in carbon emissions during the past 20 years (Xu J. et al., 2024). As a result, the development model marked by “high investment, intensive energy use” domestically led to severe ecological crises (Zhao et al., 2016). Globally, as one of the world’s largest energy consumers and carbon emitters, China’s carbon dioxide emissions now account for one-third of the global total (IEA, 2021). Therefore, China’s promotion of the low-carbon transformation of its industry is an important link and a top priority for the world to deal with environmental pollution and climate change.
In the institutional context of China, to navigate the challenges of industrial low-carbon transformation effectively, a policy-driven approach is essential (Lin and Wang, 2023). Industrial policy (IP) generally aims to foster industrial development and efficiency (Juhász et al., 2024). As a burgeoning industrial power, industrial policy has significantly shaped China’s industrialization and structural shifts (Branstetter et al., 2023). Currently, facing environmental constraints and rising costs, China’s industrial growth is at a crossroads, where traditional factor-driven models fall short of the new era’s demands for “low-carbon, green, and sustainable” development (Yang M. et al., 2022). Given the limitations of marketization in China’s context, the transition to low-carbon industries still predominantly depends on government policy directives (Zhang, 2015; Chen et al., 2025). Among all, industrial policy plays a critical role in fostering resource efficiency and technological innovation, thus catalyzing the shift towards a low-carbon economy, whose effect is yet to be discovered and quantified (Wang and Wang, 2024).
While China’s Five-Year Plans (FYP) exemplify a centralized, strategic approach to driving industrial low-carbon transformation, other major economies adopt distinct mechanisms to achieve similar goals. In the European Union, the Clean Industrial Deal integrates affordable clean energy, demand for low-carbon products, and circular economy principles—targeting a 24% circular material use rate by 2030—within a comprehensive framework supported by over €100 billion funding and market-based tools like the Emissions Trading System (Veugelers et al., 2024). In the United States, the Department of Energy’s Industrial Decarbonization Roadmap promotes energy efficiency, electrification, low-carbon fuels, and carbon capture technologies to decarbonize industry (Cresko et al., 2022), while state-level policies also play a key role, such as prioritizing low-carbon industrial products through procurement strategies (Alliance, 2022). In contrast, China’s Five-Year Plans embed low-carbon objectives within a top-down national economic strategy, distinguishing them from the EU’s multilateral, market-driven approach and the U.S.‘s technology- and procurement-focused model. China’s experience in low carbon transition highlights the diversity of policy tools and governance structures in advancing industrial decarbonization, positioning its uniqueness and globally relevance.
In the literature, the industrial low-carbon transformation has become an important and emerging strand of literature, given its importance for achieving sustainable economic development and controlling climate change. From a government policy perspective, a large body of literature centers around dedicated, standalone environmental policies such as environmental regulation (Hou et al., 2023), carbon trading (Liu et al., 2023), innovation pilot (Zhou et al., 2023), energy conservation and emission reduction policy (Tao et al., 2024), and tax reform (Kong et al., 2024). While numerous studies have investigated the influence of environmental policies on fostering industrial low-carbon transformation and achieving sustainable development, the specific role of industrial policy in this context has received comparatively less attention.
Meanwhile, research closely aligned with this paper examines the effects of targeted industrial policy, as stipulated by the Five-Year Plans (Xu, 2022). However, a consensus on its environmental impacts remains elusive. Some researchers argue that industrial policy can simultaneously bolster industrial development and mitigate carbon emissions. For instance, industrial policy could decrease the carbon emission intensity of supported sectors. Specifically, emission reductions will occur in high-emission sectors supported by industrial policy, while low-emission sectors will expand in size (Yu et al., 2020). Conversely, industrial policy may also cause overinvestment (Zhou and Zhao, 2022), or as a result of economic growth orientation, bias technological innovation in favor of production-oriented technologies, thereby inhibiting green total factor productivity progress (Zhu et al., 2021).
The existing literature has yet to definitively answer how targeted industrial policy influences the industrial low-carbon transformation. Given the diverse nature of industries, a uniform policy application across all sectors and regions is impractical. This necessitates selective support for industries with greater potential for low-carbon transformation (Meckling, 2021). This study seeks to bridge this gap by exploring the impact of China’s targeted industrial policy, as deployed through the Five-Year Plans on the low-carbon transformation.
Starting from the practical demands for China’s industrial shift towards low-carbonization and the divergent views in the existing literature, this paper poses the following progressive questions: What has been the impact of industrial policy on the industrial low-carbon transformation? Under what circumstances are industrial policy more effective? What specific channels does industrial policy utilize to drive the low-carbon transformation? Utilizing specific policy data from the Five-Year Plan governmental documents, combined with the calculated indices of industrial low-carbon transformation, this paper develops a unique Year-Province-Industry panel dataset covering the period from 1997 to 2019 for China’s 36sectors in 30 provinces and applies a fixed-effects model to empirically analyze the correlation between industrial policy and industrial low-carbon transformation. The findings indicate that targeted industries experience a 9.2% increase in low-carbon transformation index compared to non-targeted counterparts. Additionally, the paper explores the varied impacts of industrial policy on low-carbon transformation from various perspectives. We find that: local government policy has the most significant effect, followed by those jointly implemented by central and local governments, with central government policy having a lesser impact. The effectiveness of industrial policy in enhancing low-carbon transformation grows with each successive Five-Year Plan. Furthermore, industrial policy is particularly effective in promoting low-carbon transformation in mid and low-tech industries and is more beneficial for high-emission industries than for those with lower emissions. Further, this paper identifies two primary mechanisms through which industrial policy facilitates low-carbon transformation: structural and technological effects. Structurally, it encourages a shift towards low-carbon industrial and energy structures. Technologically, it boosts low-carbon innovation and fosters the development of energy-saving technologies.
This paper contributes to the literature in three key ways. First, it examines how China’s industrial policy, particularly through the Five-Year Plans, fosters a “win-win” scenario by aligning industrial development with low-carbon transformation, shifting the focus from environmental regulations to national development strategies and introducing the concept of “development-driven transformation” (Li et al., 2020; Du and Yi, 2022). Second, this paper leverages a comprehensive dataset of industrial input-output, carbon emissions, and energy consumption across 36 manufacturing industries in 30 Chinese provinces, using the SBM-GML model to construct a provincial sub-sectoral low-carbon transformation index, offering a nuanced analysis of industry and regional heterogeneity (Bai et al., 2023; Zhao et al., 2023). Third, we employed an emission reduction decomposition framework and heterogeneity analyses to elucidate the structural and technological mechanisms of industrial policy (Song et al., 2024), clarifying variations in national versus local policy effects and industry-specific responses (Huwei et al., 2023).
The remainder of this paper is arranged as follows. Section 2 proposes the theoretical framework and research hypothesis. Section 3 introduces the research design, including the model, variables, and data. Sections 4 and 5 report the main result, heterogeneous analysis, and mechanism analysis. Section 6 discusses the conclusions and policy implications.
2 Institutional background, theoretical analysis, and hypothesis
2.1 Institutional background of industrial policy
The inception of China’s “Five-Year Plans” in 1953 marked a strategic approach to national development, with industrial policies playing a crucial role in guiding the evolution and modernization of China’s industrial sectors. Throughout each Five-Year Plan cycle, the Chinese government formulates a set of industrial policies to direct the developmental trajectory of industries over the coming 5 years, selecting key sectors for support and establishing specific development objectives for them. The government employs a comprehensive array of measures to support its prioritized sectors, thereby achieving the objectives set out in its industrial policy, including providing financial incentives such as subsidies and tax breaks, facilitating access to low-interest loans, creating favorable regulatory environments, investing in infrastructure development, fostering research and development through grants and partnerships, and implementing import and export controls to protect domestic industries.
Since the beginning of the 21st century, the Five-Year Plan industrial policy has increasingly focused on sustainable development goals (Stern and Xie, 2023). The 9th Five-Year Plan (1996-2000) was the first to set energy conservation and emission reduction targets for major pollutants such as SO2, emphasizing the importance of cleaner industrial production, marking a significant initiation of sustainable industrial development strategies. The 10th Five-Year Plan (2001-2005) introduced a quantitative SO2 emission reduction target of 10% and set environmental technology development goals for targeted industries. The 11th Five-Year Plan (2006-2010) marked a turning point by introducing a mandatory energy efficiency target—reducing energy intensity (energy consumption per unit GDP)—while encouraging clean energy adoption in industries (Yuan and Zuo, 2011).
Further advancing the commitment to sustainable development, the 12th Five-Year Plan explicitly set low-carbon transformation as a developmental direction, introducing specific goals such as significantly reducing the intensity of carbon dioxide emissions and energy consumption, adjusting industrial and energy structures, and promoting the research and application of low-carbon technologies, along with corresponding policy tools (Li and Wang, 2012). In this regard, a carbon emission intensity target (CO2 emissions per unit of energy consumption) was introduced for the first time. The 13th Five-Year Plan established the concept of “promoting transformation through development”, signifying the maturation of industrial policy’s strategic approach to industrial low-carbon transformation (Gosens et al., 2017).
Figure 1 displays the changes in the low-carbon transformation index—a composite measure of carbon efficiency and emission reduction (detailed in Section 3.2)—between industries supported by industrial policy (IP) and those not supported. Industries targeted by IP refer to sectors prioritized in China’s Five-Year Plans for development and decarbonization incentives, while non-targeted industries lack such policy support. As can be seen, before 2001, the difference in low-carbon transformation trends between the two categories of industries was not significant. Since 2001, the growth rate of low-carbon transformation in targeted industries has significantly accelerated, with the disparity continuing to widen. It was not until 2016 that the rates of low-carbon transformation in both categories of industries began to converge. However, the degree of transformation in targeted industries has consistently remained higher than in unsupported industries. This provides prima facie evidence of the low-carbon transformation effects of industrial policy.
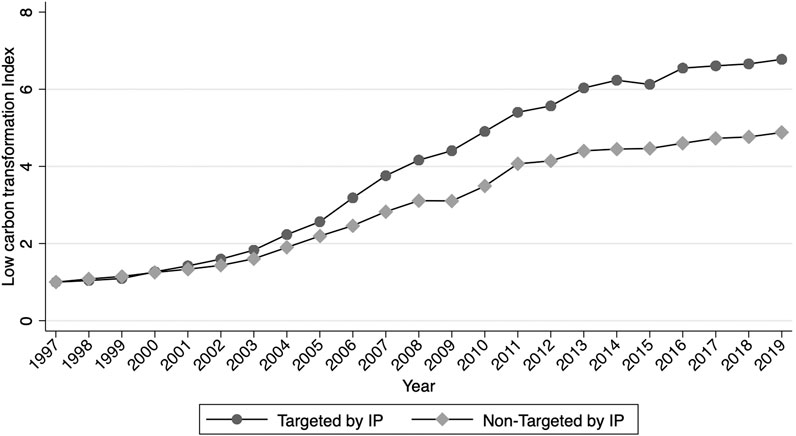
Figure 1. Industrial low-carbon transformation index under different industrial policy support scenarios. Note: Detailed variable construction and methodology elaborated in the research design Section 3.2.
2.2 Theoretical analysis and hypothesis
2.2.1 Industrial policy and low-carbon transformation
Overall, industrial policy can promote the low-carbon transformation of target industries through direct subsidies, goal-setting, and resource reallocation (Di Tommaso et al., 2020). Firstly, industrial policy provides direct subsidies to retrofit production lines in key industries, making the production process cleaner through energy-saving and emission-reduction modifications (Reijnders, 2003). Industrial policy strategically applies direct subsidies to encourage targeted industries to retrofit their production lines, focusing on energy efficiency and pollution reduction. This not only facilitates a cleaner production process by integrating advanced technologies but also significantly reduces operational costs in the long term (Zhang F. et al., 2020). The reduction in energy consumption and emissions directly contributes to environmental sustainability and aligns with global standards for green manufacturing.
Secondly, specific targets for energy saving and emission reduction are set, allowing companies that meet these criteria to enjoy additional support, thereby encouraging enterprises to undertake decarbonization-related costs (Du and Yi, 2022). Setting specific targets for energy saving and emission reduction serves as a powerful incentive for industries. Companies meeting or exceeding these targets receive additional governmental support, such as tax rebates or further subsidies. This not only rewards compliance but also motivates ongoing improvements, fostering a competitive environment where companies strive to innovate and enhance their environmental practices. By doing so, industrial policy can accelerate the adoption of end-of-pipe treatments and other pollution control technologies, enhancing overall industry compliance with environmental regulations (Wang et al., 2023).
Lastly, industrial policy introduces investments that steer resources towards high-productivity, low-resource consumption enterprises, allowing key industries to fully utilize factor resources to increase output, thereby reducing energy consumption and carbon emissions (Yang X. et al., 2022). By redirecting investments towards enterprises that exhibit high productivity and low resource consumption, industrial policy promotes an efficient allocation of capital. This encourages industries to optimize their use of resources, leading to increased output while minimizing input costs related to energy and raw materials (Usman and Balsalobre-Lorente, 2022). As a result, industries are able to lower the energy and carbon intensity of their production processes, contributing to a substantial decrease in overall industrial carbon footprints. This strategy not only supports sustainable industrial growth but also positions industries to be resilient and adaptive in a resource-constrained world, thereby ensuring long-term economic stability and environmental sustainability (Wu et al., 2021). Based on the institutional background outlined above, this paper proposes research hypothesis 1:
H1. Industrial policy can facilitate low-carbon transformation in targeted industries.
2.2.2 The structural effect of industrial policy in driving low-carbon transformation
From the institutional background, it is evident that the pathway through which industrial policy drives industrial low-carbon transformation primarily involves advancing upgrades in industrial and energy structures, encouraging green innovation, and energy-saving biased progress in low-carbon technologies (Zhang Y. et al., 2020).
In terms of structural effect, on one hand, industrial policy can promote the relative expansion of low-carbon emission industries while constraining the scale of highly polluting industries, thereby substituting high-emission sectors and achieving the decarbonization of industrial structures (Zhao et al., 2022). Industrial policy can facilitate the growth of industries with lower carbon footprints by providing financial incentives, regulatory support, and research and development funding, which encourage the scaling of these industries. Concurrently, governments also impose stricter regulations and higher costs on high-emission industries, effectively reducing their competitive edge and market share, which leads to a natural phasing out of less efficient and more polluting sectors (Zhang et al., 2022).
On the other hand, industrial policy can leverage various preferential measures to incentivize enterprises to replace fossil fuels with clean energy, thus achieving the purification of the energy structure (Xu et al., 2020). By promoting subsidies for renewable energy sources and penalizing the use of fossil fuels, industrial policy encourages enterprises to switch to cleaner energy alternatives. This not only reduces the carbon intensity per unit of production but also aligns with trends towards energy sustainability (Lee et al., 2023). Based on the above analysis, this paper proposes the following hypothesis 2:
H2. Industrial policy can drive low-carbon transformation through structural effects.
2.2.3 The technological effect of industrial policy in driving low-carbon transformation
In terms of technological effects, on one hand, industrial policy can stimulate enterprises to innovate in low-carbon technologies thereby reducing the carbon emissions per unit of output, thus improving carbon productivity (Song et al., 2022). By providing innovation subsidies and access to green financing, governments incentivize companies to invest in low-carbon R&D (Lin and Xie, 2023). These financial instruments lower the risk and increase the potential return on investments in new technologies, which are essential for driving decarbonization-related innovation (Qi et al., 2022).
On the other hand, industrial policy can promote biased technological progress, i.e., encouraging enterprises to substitute capital for energy, thus achieving low-carbon transformation (Su and Fan, 2022). The implementation of renewable energy subsidies and other fiscal instruments encourages a shift in production techniques, from energy-intensive methods to those that are more capital and technology-intensive (Zhou et al., 2020). By increasing the relative cost of nonrenewable energy, particularly fossil fuels, industrial policy shifts the economic balance, making it more cost-effective for companies to invest in energy-saving technologies rather than continuing with traditional, less efficient tech practices (Shao and Wang, 2024). Based on the above theories, this paper proposes the following hypothesis 3:
H3. Industrial policy can drive the industrial low-carbon transformation through technological effects.
The hypotheses H1, H2, and H3 are interrelated rather than independent, forming a cohesive framework to assess industrial policy’s role in low-carbon transformation. H1 posits that industrial policy facilitates low-carbon transformation in targeted industries, serving as the foundational hypothesis and primary empirical focus of this paper. H2 and H3, in turn, delineate the mechanisms through which this effect manifests.
3 Research methodology
3.1 Model
This paper employs a model incorporating fixed effects for province, industry, and year to evaluate the influence of industrial policy on the low-carbon transformation (Wu et al., 2019). We adopted a fixed-effects model to assess the impact of targeted industrial policies on low-carbon transformation based on theoretical considerations and data characteristics (Angrist and Pischke, 2008; Clark and Linzer, 2015; Breuer and Dehaan, 2024). This approach controls for unobserved, time-invariant heterogeneity across provinces and industries—such as varying industrial bases or policy enforcement capacities—critical in the context of Five-Year Plans. A random-effects model, which assumes uncorrelated individual effects with explanatory variables, is less suitable here, as province-specific factors likely influence both policy implementation and LCT. As shown in Table 2 column (3), we conducted a Hausman test, which strongly favored the fixed-effects model, rejecting the null hypothesis of no systematic difference between specifications. Specifically, the model is set as following:
In the model, the subscript i represents the province, j represents the industry, and t represents the year.
3.2 Variables
3.2.1 The explained variable: industrial low-carbon transformation index
The dependent variable in this paper is the Industrial Low-Carbon Transformation Index (LCT), calculated at the sub-sector level for each province in China (Song and Zhou, 2021). This study conceptualizes industrial low-carbon transformation as a multifaceted process that integrates considerations of output growth, emission reduction, and energy efficiency. Drawing on established methodologies, we employ the super-efficiency Slack-based measure (SBM) model in conjunction with the Global Malmquist-Luenberger (GML) index decomposition approach to calculate the LCT (Meng and Qu, 2022).
The SBM and GML method are sequentially linked in constructing the low-carbon transformation (LCT) index. Initially, the SBM model (Equation 2) calculates the carbon emission efficiency for each industry k in period t, where ρ represents efficiency scores based on inputs (x), desirable outputs (y), and undesirable outputs (p), accounting for slacks (
First, the algorithm for the super-efficient SBM can be expressed as:
In model (Equation 2),
The term
3.2.2 Explanatory variable: industrial policy
The explanatory variable in this paper is derived from the industrial policy references within the Five-Year Plan (FYP) documents issued by both central and local governments (Barbieri et al., 2019; Chen et al., 2022). To operationalize industrial policy, we employ a text analysis approach, coding it as a dummy variable. Specifically, industries mentioned within the 9th FYP to the 13th FYP by central and provincial governments, and described with terms such as “key”, “priority”, and “pillar”, among other related terms, are identified as targeted by policy. For any given industry j in region i in year t that is highlighted as a key development sector in the FYP of the central or local government,
3.2.3 Control variables
To isolate the causal effect of industrial policy on low-carbon transformation, we include control variables informed by prior theoretical and empirical research (Bai et al., 2023; Pan et al., 2023; Shang and Lv, 2023; Zeng et al., 2023; Kong et al., 2024; Tan et al., 2024). On one hand, government environmental regulation represents the primary confounding factor. Additionally, industrial productivity and state capital control capacity are significant influences on the formulation of industrial policies. On the other hand, development economics posits that industry characteristics and endowments determine the potential for low-carbon transition. Accordingly, this study controls for typical industry features such as scale, profitability, and capital intensity. Given that existing studies provide detailed discussions on the theoretical basis for variable selection, we prioritize brevity here while summarizing their relevance and expected effects. (1) Environmental Regulation (ER), proxied by the ratio of environmental protection-related words in Government Working Reports, is expected to promote low-carbon transformation by incentivizing green innovation. (2) Per Capita Output (PROD), industrial value added per employee, may enhance low-carbon efficiency through productivity gains. (3) Government Intervention (GOV), the share of state and collective capital, may hinder low-carbon transformation due to inefficiencies associated with high state ownership. (4) Industry Scale (SIZE), logged enterprise units per sector, likely supports decarbonization through scale economies. (5) Profit Rate (PROFIT), profit-to-value-added ratio, is anticipated to facilitate low-carbon investments. (6) Average Firm Size (AVERFIRM), employees per enterprise, may improve emission reduction via innovation capacity. (7) Capital Intensity (CAPIN), capital stock per employee, is expected to drive sustainability through technological upgrades.
3.3 Data
Following the previous work on this dataset relatively unique dataset, this paper further applied this province-sectors-year panel data to identifies the casual relationship between industrial policy and the LCT (Zhou and Song, 2022). The data for calculating the industrial low-carbon transformation and control variables is derived from the China Industrial Economic Statistics Yearbook, the energy consumption and pollution emission data are acquired from the China Emission Accounts and Datasets. The industry policy data utilized in this paper is sourced from the National Economic and Social Development Five-Year Plans published by the Central People’s Government of the People’s Republic of China and provincial local governments. To ensure data quality and reliability, we implemented several preprocessing steps. First, missing values were addressed in three ways: (1) within-province industry gaps were filled using linear interpolation; (2) consecutive missing values for specific units were imputed with the average from adjacent provinces in the same industry and year; and (3) industries with severe missing data in certain provinces were excluded entirely. Second, to mitigate the impact of extreme values, all continuous variables underwent Winsorization at the 1st and 99th percentiles. Third, price deflation was applied to ensure comparability: industrial output (PROD) was deflated using the Producer Price Index from the China Price Statistical Yearbook, while capital stock (CAPIN) was adjusted with provincial Fixed Asset Investment Price Indices.
Table 1 demonstrates the descriptive statistics of the main variables.
4 Results and discussions
4.1 Baseline results
Table 2 presents the results for the baseline model. Column (1) is the OLS model result, column (2) includes all the control variables, and only provincial, and industrial fixed effects, and column (3) is the full model. The baseline results suggest that the industrial policy has significantly fostered the LCT of targeted industries. In Column (3), the coefficient for the IP is 0.312. Hence, in terms of economic significance for the regression, if an industry is prioritized by the industrial policy, the LCT would rise by 9.2% relative to the untargeted. Thus, the hypothesis H1 is verified.
Our analysis shows that targeted industrial policies increase the low-carbon transformation index by 9.2%, a statistically significant result with notable economic implications. Based on the data from IEA, China’s industrial sector contributed 10.15 billion tons of CO2 in 20201. A 9.2% reduction equates to approximately 933.8 million tons of CO2 avoided annually. Valued at China’s carbon market price of $14 per ton2, this translates to a cost saving of approximately $13.048 billion per year, reflecting the economic benefit of reduced emission allowances. However, low-carbon transformation encompasses more than emissions reduction; it also enhances production efficiency, a benefit not fully captured by current data. Consequently, our estimate likely understates the true economic impact of these policies, as efficiency gains—such as reduced energy costs or improved output—remain unquantified.
4.2 Endogeneity and robustness tests
4.2.1 Difference in differences model with propensity score matching
The non-randomness of the core explanatory variable, industrial policy, may cause selection bias. Due to potential systematic differences between industries that are supported and those that are not, the effect of industrial policy might stem from the characteristics of the industries rather than the actual impact of the policy. This could lead to a situation where the paper’s conclusions arise because industries with a higher degree of low-carbon transformation are more likely to be selected by governments.
To ensure that this critical endogeneity does not affect the identification of the empirical result, the paper uses changes in key industries between the successive Five-Year Plans as quasi-natural experiments. To address the challenges of conducting parallel trend tests due to the constant changes in industrial policy across different Five-Year Plan periods, this paper first uses control variables to calculate propensity scores and performs 1:1 matching between industries that are supported (treatment group) and those that are not supported (control group). Then, employing a difference-in-differences design, we test the causal impact of industrial policy on low-carbon transformation:
In Equation 4,
From Table 3, it is evident that conducting a difference-in-differences regression based on propensity score matching yields results that are still significantly positive. This further mitigates the concern of self-selection bias on the empirical results.
4.2.2 Heckman sample selection model
The effects of industrial policy implementation on the LCT might be biased by endogenous issues. For example, targeted industries are not randomly selected by the government, but depend on unobserved qualities. With non-random policy, researchers are unable to observe the counterfactual scenario of what would happen to the industrial low-carbon transformation if key industries had not received policy support. To address this concern, we employ the Heckman sample selection model to control for such endogeneity (Song et al., 2020). Specifically, based on the literature regarding the mechanisms of industrial policy formulation (Wu et al., 2019), we selected a set of variables potentially influencing government decisions to support certain industries3 to conduct a Probit regression and computed the Inverse Mill’s Ratio (IP_imr). This was then incorporated into the original regression model to correct for sample selection bias. The result is presented in Column (1) of Table 4. The significance of the Inverse Mill’s Ratio confirms the existence of sample selection bias. However, the direction and significance of the corrected regression coefficients remain unchanged, suggesting that the baseline model’s results would not be dampened by selection bias.
4.2.3 Panel data model with interactive fixed effects
Although the fixed-effect model adopted in this paper controls for endogeneity issues at the provincial, industrial, and year levels, potential biases may still exist. To mitigate endogeneity issues associated with unobservable factors, this paper further controls for three extra groups of interaction fixed effect: (1) Province × Industry; (2) Province × Year and Industry × Year; (3) Province × Five-Year Plan and Industry × Five-Year Plan. Columns (2) to (4) in Table 4 show that after incorporating these interactive fixed effects, the direction and significance of the regression results remained unchanged, indicating that the empirical results of the baseline are not easily influenced by omitted variables and the conclusions are robust.
4.2.4 Generalized method of moments estimation
When the random disturbance term in the baseline model exhibits heteroskedasticity and serial correlation, for instance, if the current value of the LCT depends on its previous value, the traditional static fixed-effect model might not yield consistent estimates. To address this, it becomes necessary to employ a dynamic panel model for verification. In response to this, we utilize the System Generalized Method of Moments estimator, incorporating the lagged term of the dependent variable into the baseline model. Column (5) of Table 4 presents the result. Upon including the lagged values of the dependent variable, the Sargan estimator indicates no over-identification of the instrumental variables; the AR (2) estimator is not significant, suggesting the absence of second-order autocorrelation in the error term. At this juncture, the regression coefficient of the industrial policy remains significantly positive, further substantiating the robustness of the results.
4.2.5 Anticipatory effect of industrial policy
Owing to the possibility that industrial policy might cause strategical behavior in an anticipatory manner, where firms might be impacted by the forthcoming industrial policy due to the advanced dissemination of targeted information, it becomes imperative to examine if such policy foresight could affect the result (Schulz and Rode, 2022). To this end, we incorporated the industrial policy from the previous Five-Year Plan into the regression model to disentangle the effects of policy anticipation by introducing a dummy variable for the industrial policy from the previous planning period (IP_prev). In column (6) of Table 4, the coefficient of the industrial policy remains significantly positive, indicating that the results are robust to the anticipatory effect.
4.3 The heterogeneous impact of industrial policy on the industrial low-carbon transformation
From existing literature, it's evident that there remains significant controversy regarding the effectiveness of industrial policy, the heterogeneity inherent in industrial policy itself is one of the primary reasons leading to inconsistent policy assessment outcomes. Therefore, the evaluation of the impact of industrial policy should not merely focus on their effectiveness. Instead, it should address the more pivotal question of under what conditions are industrial policy effective (Aiginger and Rodrik, 2020). In light of this, this paper further delves into the heterogeneous effects of industrial policy on the industrial low-carbon transformation from the perspective of policy origins, planning cycles, technological complexity, and pollution level.
4.3.1 Heterogeneous effect of central and local government industrial policy
To examine the varied impacts of industrial policies originating from different government levels, this study categorizes industrial policy into three distinct types: targeted by central-local jointly, central government-only targeted, and local government-only targeted. The classification leads to three dummy variables: IPcp indicates joint support (1 if supported, 0 otherwise), IPoc for central-government-only IP, and IPop for local-government-only IP.
Results in Table 5 show significant differences in how central versus local government policy affects industrial low-carbon transformation. Results suggest local government policy has the most substantial impact, followed by joint policy support, with central government policy having the least effect. This hierarchy remains consistent when comparing all policy types simultaneously in the regression, highlighting that locally supported industries outperform others in low-carbon transformation by 12.3% over the average, versus 9% for jointly supported and 7.1% for centrally supported industries. The effectiveness of local policy likely stems from local governments’ greater awareness of regional industry strengths and needs, enabling more targeted and efficacious policy formulation to foster low-carbon development (Pan et al., 2023).
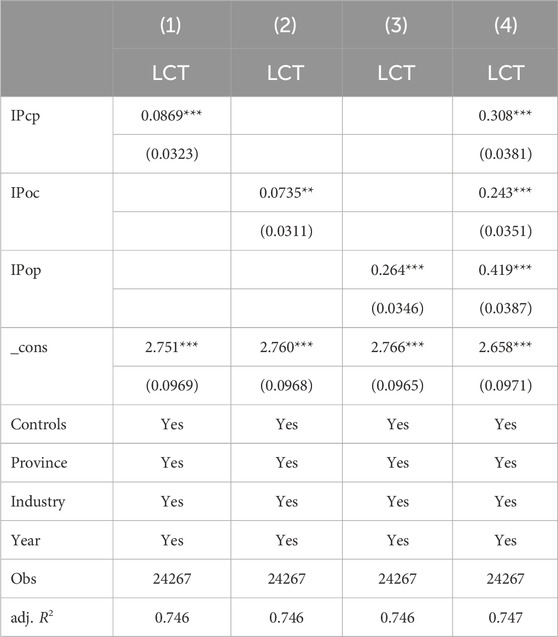
Table 5. The heterogeneous impact of central and local government industrial policy on the low-carbon transformation.
Local government policies outperform central policies due to decentralization advantages (Sun et al., 2023). Local authorities possess superior information about regional industrial structures and environmental conditions, enabling tailored interventions that align with specific needs (Song et al., 2025). This aligns with fiscal federalism, where proximity enhances policy efficacy.
4.3.2 Impact of industrial policy on the low-carbon transformation in different FYP cycles
It is essential to examine how evolving industrial policy has influenced low-carbon transformation in China. Given the continual improvement in policy implementation, we categorize the data by FYP periods: the 9th (1997-2000), 10th (2001-2005), 11th (2006-2010), 12th (2011-2015), and 13th (2016-2019). Findings in Table 6 highlight an escalating influence of industrial policy on low-carbon transformation across these periods. The 9th FYP saw negligible policy impact. However, from the 10th FYP onwards, as environmental targets became integral to national development goals, the government increasingly focused on low-carbon growth, thus enhancing the effectiveness of industrial policy from the 11th to the 13th FYPs.
4.3.3 Heterogeneity with different levels of technological complexity
This study continues to investigate whether sector-specific industrial policy can foster low-carbon transformation across industries with varied technological complexities. To do so, we classify two-digit industrial sectors into low, medium, and high technology groups according to established categorizations (Chen et al., 2021).
This finding in Table 7 reveals significant variation in the effectiveness of industrial policy across technological complexity tiers. Notably, medium and low-tech industries have seen substantial benefits from IP, with the medium-tech sector experiencing the most significant enhancement in low-carbon transformation. Conversely, high-tech industries have not shown similar progress.
Medium- and low-tech industries benefit more than high-tech sectors from these policies (Yang et al., 2023). This may stem from their lower technological complexity and greater adaptability to standardized low-carbon measures, such as energy efficiency upgrades, which require less innovation capacity compared to high-tech industries reliant on cutting-edge R&D. While medium-low industries, with their complex developmental trajectories, benefit from policy guidance towards low-carbon paths, high-tech sectors pose a challenge. High-tech sectors, conversely, may face diminishing returns from broad policies due to their specialized needs (Xu P. et al., 2024). Despite their high innovation costs and risks that seemingly warrant policy support, the government’s limited knowledge and guidance capabilities fall short of promoting low-carbon advancements in these areas.
4.3.4 Heterogeneity with different pollution levels
Whether government industrial policy is fine-tuned to match the specific needs of different sectors, particularly emphasizing the pollution levels of industries is of great importance (Yu et al., 2020). To examine if the impact of industrial policy on low-carbon transformation differs by pollution levels, this study conducts a grouped regression analysis in two steps. Initially, industries are classified into heavily-polluted and non-heavily-polluted categories based on official environmental guidelines. Additionally, the sample is segmented into high, medium, and low emission intensity groups based on tertiles.
Findings in Table 8 reveal that industrial policy significantly fosters low-carbon transformation in heavily polluted industries more than in non-heavily polluting samples. Furthermore, the positive impact is most pronounced in the high-emission group, diminishing in the medium-emission group, and least in the low-emission group. This underscores that industrial policy is strategically directed at mitigating pollution in sectors with higher emission levels. Industries with higher pollution levels show amplified effects, reflecting their greater potential for emission reductions under stringent regulation (Lin and Zhang, 2023). Rigorous environmental regulations can drive innovation and efficiency gains, particularly in high-emission sectors with substantial room for improvement, making policy interventions more impactful by unlocking larger absolute reductions (Huang and Yi, 2023).
5 Mechanism analysis
In order to test the influence mechanism of industrial policy to promote the low-carbon transformation, the following mediation effect model is constructed:
Where
5.1 Structural effect
This paper verifies whether industrial policy can promote industrial low-carbon transformation through the decarbonization of industrial structures and the purification of energy structures.
The mediating variable of industrial structure decarbonization (UCE) is measured by the proportion of low-carbon industries in the total industrial output value of the province (Tian et al., 2019). A positive coefficient of UCE would suggest that industrial policy is facilitating this transformation by enhancing the share of low-carbon industries.
Another critical pathway is the shift toward cleaner energy structures, vital for reducing carbon emissions primarily sourced from fossil fuels (Xu et al., 2020). This paper measures energy structure upgrading (NF) through the proportion of renewable energy consumption to total energy consumption. A significantly positive coefficient will indicate that IP is effectively encouraging a move away from fossil fuels towards cleaner energy sources.
The mediation effect regression results of structural effects are presented in column (1)–(4) in Table 9. Column (1) shows that industrial policy significantly contributes to the expansion of low-emission industries. Column (2) further verified that industrial policy can promote LCT by expanding low-carbon industries. In column (3), supported industries demonstrate a 3.98% higher share of non-fossil energy consumption than unsupported ones. The coefficients for IP and NF are both significantly positive in column (4), confirming that IP can promote industrial low-carbon transformation by encouraging the use of renewable energy. According to the above results, the research hypothesis 2 was verified.
5.2 Technological effect
To explore how industrial policy facilitates low-carbon transformation via technological effect, this paper focuses on the mediating effect of low-carbon innovation and energy-saving technological progress. Due to the challenge of collecting accurate proxies for low-carbon innovation at a granular level (Zhu et al., 2019), we aggregate the number of low-carbon patent applications by all listed Chinese companies from 1997 to 2019 to the year-province-industry level, and then take natural logarithm to serve as the proxy for low-carbon innovation effect (LCIN). Additionally, the capital-to-energy input ratio (ZK) is used to represent energy-saving biased technological progress, highlighting the shift towards more energy-efficient technologies (Acemoglu et al., 2012; Dong et al., 2019). A higher value of ZK indicates greater substitution of energy inputs with capital inputs within the input structure, reflecting a technological inclination towards using capital rather than energy, thereby making the industry cleaner.
Table 9 column (5) reveals that industries supported by industrial policy see a 28.1% higher rate of low-carbon patent applications than their unsupported counterparts, demonstrating the policy’s role in spurring low-carbon technological innovation. Column (6) further verifies the channel through which IP promotes LCT by encouraging low-carbon innovation.
Column (7) shows that supported industries exhibit a significant shift towards energy-saving technological progress, evidenced by a relative increase in capital input compared to energy input. Finally, the results in column (8) confirm the impact pathway of energy-saving biased technological progress. Accordingly, this paper confirms the research hypothesis 3.
6 Conclusions and policy implications
6.1 Findings
Industrial policy serves as a critical mechanism by which governments can shape economic growth, steer the industry toward modernization and efficiency, and address market failures. Facing escalating environmental pressures and resource limitations, China urgently needs to pivot towards a low-carbon development model to sustain high-quality growth. This study examines the role of industrial policy in facilitating low-carbon transformation within China’s industrial sector. Employing a fixed-effects model that accounts for variations across regions, industries, and years, adopting data spanning 36 industries across 30 provinces from 1997 to 2019, this paper aims to quantify the effectiveness of the industrial policy in promoting low-carbon transformation.
The key findings are as follows. First, industrial policy has significantly promoted the low-carbon transformation. Compared to unsupported industries, the low-carbon transformation index of those supported by targeted industrial policy will see an increase of 9.2%. Second, in terms of heterogeneity, this paper finds that the effects of local government industrial policy, later Five-Year Plans, and policy in industries with medium or low technological complexity, and in heavily polluting and high-emission industries are more significant. Furthermore, industrial policy can promote low-carbon transformation through low-carbon structural upgrading and technological progress.
6.2 Policy implications
Based on the conclusions of this paper, the Chinese government should continue to implement effective industrial policy to drive the low-carbon transformation. China’s industrial policy, particularly those enacted by local governments under the Five-Year Plans have significantly contributed to the low-carbon industrial transformation, suggesting that sustainable industrial development can be promoted through the strategy of “development-driven transformation”. The increase in the low-carbon transformation index provides actionable insights for policymakers. To maximize this effect, we suggest: (1) increasing subsidies for green technologies, such as carbon capture and renewable energy, targeting high-emission sectors like steel and cement; and (2) promoting regional low-carbon industrial clusters to enhance scale and innovation spillovers. A notable real-world example is the Ordos Low-Carbon Industrial Park in Inner Mongolia, launched in 2021 (Li et al., 2023). This initiative integrates renewable energy with traditional industries, reducing CO2 emissions by an estimated 3.5 million tons annually while creating over 10,000 green jobs by 2023. Supported by the 14th Five-Year Plan, it showcases how policy-driven transformation can align economic growth with decarbonization.
To enhance this transformation further, it's essential to refine policy formulation and implementation, focusing on the distinct needs of central and local governance as well as industry-specific characteristics. The heterogeneity analysis of this paper underscores the need for tailored approaches to policy implementation. In terms of government functions, the central government should define clear policy goals and incentives, granting flexibility to local authorities. Local governments, in turn, should capitalize on regional strengths and prioritize sectors with substantial growth potential for low-carbon initiatives. For high-tech industries, the government needs to find effective incentives, provide special subsidies for high-tech, low-carbon production models and technology applications, and utilize market mechanisms such as carbon quotas and carbon pricing to encourage high-tech enterprises to implement low-carbon transformation. Moreover, designing policy for high-emission industries is crucial for fostering low-carbon transformation and preventing a lock-in of outdated low-carbon strategies. In addition to encouraging high-pollution industries to reduce carbon emissions themselves, industrial policy also needs to consider promoting the expansion of low-emission industries and facilitating regional or international industrial transfers to gradually replace high-pollution industries during policy formulation and implementation.
Mechanism analysis highlights that structure upgrading and technological progress are pivotal in driving low-carbon transformation. China’s central and local governments should guide traditional and high-pollution industries towards modern, eco-friendly practices, encouraging the growth of low-carbon sectors. To this end, prioritizing the development of a cleaner energy infrastructure and shifting financial incentives from fossil fuels to sustainable energy sources are vital steps. To stimulate industrial low-carbon transformation effectively, policy should focus on supporting breakthroughs in essential technologies and increase funding for energy-efficient innovations. Encouraging the adoption of green technologies through subsidies and emphasizing energy-saving targets in R&D can maximize the technological advancements’ benefits for low carbon transformation.
Implementing these recommendations, however, faces feasibility challenges and potential obstacles. Ensuring local governments can effectively execute policies requires addressing capacity disparities, as some regions lack technical expertise or administrative resources (Tu et al., 2024). This can be mitigated through targeted training programs and central government-led pilot projects to build local competency. Resource allocation poses another difficulty, particularly for subsidies in high-tech and low-emission industries, given fiscal constraints in less-developed provinces. A solution is to leverage public-private partnerships (PPPs) and international funding, such as green bonds, to supplement local budgets. For high-tech industries, the complexity of designing effective incentives may encounter resistance from firms prioritizing short-term profits over long-term sustainability. Introducing phased carbon pricing and performance-based subsidies can align market incentives with policy goals (Chen and Wang, 2023). High-emission industry transitions risk economic disruption or job losses, which could be addressed by phased industrial transfer plans and retraining programs for affected workers. These strategies enhance feasibility while overcoming implementation barriers, ensuring policies translate into tangible low-carbon outcomes.
6.3 Limitation and future perspectives
While this paper offers robust evidence on the role of industrial policy in driving low-carbon transformation, certain limitations should be acknowledged. First, the data primarily cover China’s industrial sector within a specific timeframe, potentially limiting generalizability to other regions or periods (especially after COVID-19) with differing industrial and policy contexts. Second, despite using fixed-effects models and control variables to address confounding factors, unobserved heterogeneity or reverse causality—such as low-carbon progress shaping policy design—may still affect causal inference. Third, the analysis does not account for the varying support intensity of Five-Year Plans across industries, which may influence the effectiveness of policies in promoting low-carbon transitions in different sectors.
These limitations highlight directions for future research. Expanding the dataset to include cross-national comparisons could assess the applicability of our findings across diverse governance and industrial settings. Developing more precise variable approaches to measure industrial policy intensity—such as industry-specific investment allocations or policy enforcement metrics—would better capture the differential impacts of Five-Year Plans. Additionally, investigating the interplay between industrial policy and emerging factors, such as carbon markets, could further elucidate low-carbon transformation dynamics.
Data availability statement
The raw data supporting the conclusions of this article will be made available by the authors, without undue reservation.
Author contributions
LS: Formal Analysis, Writing – original draft, Writing – review and editing. JY: Data curation, Investigation, Software, Writing – original draft. TL: Resources, Supervision, Validation, Visualization, Writing – original draft. JZ: Funding acquisition, Project administration, Supervision, Writing – review and editing.
Funding
The author(s) declare that financial support was received for the research and/or publication of this article. This work was supported by The National Social Science Fund of China (23BJY017).
Conflict of interest
The authors declare that the research was conducted in the absence of any commercial or financial relationships that could be construed as a potential conflict of interest.
Generative AI statement
The author(s) declare that no Generative AI was used in the creation of this manuscript.
Publisher’s note
All claims expressed in this article are solely those of the authors and do not necessarily represent those of their affiliated organizations, or those of the publisher, the editors and the reviewers. Any product that may be evaluated in this article, or claim that may be made by its manufacturer, is not guaranteed or endorsed by the publisher.
Footnotes
1See the IEA Data Explorer: https://www.iea.org/data-and-statistics/data-tools/greenhouse-gas-emissions-from-energy-data-explorer.
2See the International Carbon Action Partnership websites: https://icapcarbonaction.com/en/ets-prices.
3According to the literature, we include the following variables: total industrial output, capital input, labor input, total factor productivity, output share, government intervention, industry size, industry profit, and average firm size (Wu et al., 2019).
References
Acemoglu, D., Aghion, P., Bursztyn, L., and Hemous, D. (2012). The environment and directed technical change. Am. Econ. Rev. 102, 131–166. doi:10.1257/aer.102.1.131
Aiginger, K., and Rodrik, D. (2020). Rebirth of industrial policy and an agenda for the twenty-first century. J. Ind. Compet. Trade 20, 189–207. doi:10.1007/s10842-019-00322-3
Alliance, U. C. (2022). Enabling industrial decarbonization: a policy guidebook for us states. U. S. Clim. Alliance.
Angrist, J. D., and Pischke, J.-S. (2008). “Chapter 5. Parallel worlds: fixed effects, differences-in-differences, and panel data,” in Chapter 5. Parallel worlds: fixed effects, differences-in-differences, and panel data (Princeton University Press), 221–248. doi:10.1515/9781400829828-010
Bai, T., Qi, Y., Li, Z., and Xu, D. (2023). Digital economy, industrial transformation and upgrading, and spatial transfer of carbon emissions: the paths for low-carbon transformation of Chinese cities. J. Environ. Manag. 344, 118528. doi:10.1016/j.jenvman.2023.118528
Barbieri, E., Di Tommaso, M. R., Tassinari, M., and Marozzi, M. (2019). Selective industrial policies in China: investigating the choice of pillar industries. Int. J. Emerg. Mark. 16, 264–282. doi:10.1108/IJOEM-02-2018-0105
Branstetter, L. G., Li, G., and Ren, M. (2023). Picking winners? Government subsidies and firm productivity in China. J. Comp. Econ. 51, 1186–1199. doi:10.1016/j.jce.2023.06.004
Breuer, M., and Dehaan, E. (2024). Using and interpreting fixed effects models. J. Account. Res. 62, 1183–1226. doi:10.1111/1475-679X.12559
Chen, J., Feng, H., and Zhou, H. (2022). Local industrial policy and productivity: evidence from China. Contemp. Econ. Policy 40, 138–161. doi:10.1111/coep.12555
Chen, M., and Wang, K. (2023). The combining and cooperative effects of carbon price and technological innovation on carbon emission reduction: evidence from China’s industrial enterprises. J. Environ. Manag. 343, 118188. doi:10.1016/j.jenvman.2023.118188
Chen, R., Zhang, Q., and Wang, J. (2025). Impact assessment of green finance reform on low-carbon energy transition: evidence from China’s pilot zones. Environ. Impact Assess. Rev. 110, 107654. doi:10.1016/j.eiar.2024.107654
Chen, Z., Zhang, X., and Chen, F. (2021). Do carbon emission trading schemes stimulate green innovation in enterprises? Evidence from China. Technol. Forecast. Soc. Change 168, 120744. doi:10.1016/j.techfore.2021.120744
Clark, T. S., and Linzer, D. A. (2015). Should I use fixed or random effects? Political Sci. Res. Methods 3, 399–408. doi:10.1017/psrm.2014.32
Cresko, J., Rightor, E., Carpenter, A., Peretti, K., Elliott, N., Nimbalkar, S., et al. (2022). U.S. Department of Energy’s industrial decarbonization Roadmap. USDOE Office Energy Effic. Renew. Energy (EERE). doi:10.2172/1961393
Di Tommaso, M. R., Tassinari, M., Barbieri, E., and Marozzi, M. (2020). Selective industrial policy and ‘sustainable’structural change. Discussing the political economy of sectoral priorities in the US. Struct. change Econ. Dyn. 54, 309–323. doi:10.1016/j.strueco.2020.05.005
Dong, Y., Shao, S., and Zhang, Y. (2019). Does FDI have energy-saving spillover effect in China? A perspective of energy-biased technical change. J. Clean. Prod. 234, 436–450. doi:10.1016/j.jclepro.2019.06.133
Du, J., and Yi, H. (2022). Target-setting, political incentives, and the tricky trade-off between economic development and environmental protection. Public Adm. 100, 923–941. doi:10.1111/padm.12768
Gosens, J., Kåberger, T., and Wang, Y. (2017). China’s next renewable energy revolution: goals and mechanisms in the 13th Five Year Plan for energy. Energy Sci. and Eng. 5, 141–155. doi:10.1002/ese3.161
Hou, S., Yu, K., and Fei, R. (2023). How does environmental regulation affect carbon productivity? The role of green technology progress and pollution transfer. J. Environ. Manag. 345, 118587. doi:10.1016/j.jenvman.2023.118587
Huang, H., and Yi, M. (2023). Impacts and mechanisms of heterogeneous environmental regulations on carbon emissions: an empirical research based on DID method. Environ. Impact Assess. Rev. 99, 107039. doi:10.1016/j.eiar.2023.107039
Huwei, W., Shuai, C., and Chien-Chiang, L. (2023). Impact of low-carbon city construction on financing, investment, and total factor productivity of energy-intensive enterprises. Energy J. 44, 79–102. doi:10.5547/01956574.44.2.hwen
Juhász, R., Lane, N., and Rodrik, D. (2024). The new economics of industrial policy. Annu. Rev. Econ. 16, 213–242. doi:10.1146/annurev-economics-081023-024638
Kong, L., Li, Z., Liu, B., and Xu, K. (2024). The impact of environmental protection tax reform on low-carbon total factor productivity: evidence from China’s fee-to-tax reform. Energy 290, 130216. doi:10.1016/j.energy.2023.130216
Lee, C.-C., Zhang, J., and Hou, S. (2023). The impact of regional renewable energy development on environmental sustainability in China. Resour. Policy 80, 103245. doi:10.1016/j.resourpol.2022.103245
Li, J., Shi, X., Wu, H., and Liu, L. (2020). Trade-off between economic development and environmental governance in China: an analysis based on the effect of river chief system. China Econ. Rev. 60, 101403. doi:10.1016/j.chieco.2019.101403
Li, J., and Wang, X. (2012). Energy and climate policy in China’s twelfth five-year plan: a paradigm shift. Energy Policy 41, 519–528. doi:10.1016/j.enpol.2011.11.012
Lin, B., and Guan, C. (2023). Evaluation and determinants of total unified efficiency of China’s manufacturing sector under the carbon neutrality target. Energy Econ. 119, 106539. doi:10.1016/j.eneco.2023.106539
Lin, B., and Wang, C. (2023). Does industrial relocation affect regional carbon intensity? Evidence from China’s secondary industry. Energy Policy 173, 113339. doi:10.1016/j.enpol.2022.113339
Lin, B., and Xie, Y. (2023). Positive or negative? R&D subsidies and green technology innovation: evidence from China’s renewable energy industry. Renew. Energy 213, 148–156. doi:10.1016/j.renene.2023.06.011
Lin, B., and Zhang, A. (2023). Can government environmental regulation promote low-carbon development in heavy polluting industries? Evidence from China’s new environmental protection law. Environ. Impact Assess. Rev. 99, 106991. doi:10.1016/j.eiar.2022.106991
Liu, B., Ding, C. J., Hu, J., Su, Y., and Qin, C. (2023). Carbon trading and regional carbon productivity. J. Clean. Prod. 420, 138395. doi:10.1016/j.jclepro.2023.138395
Meckling, J. (2021). Making industrial policy work for decarbonization. Glob. Environ. Polit. 21, 134–147. doi:10.1162/glep_a_00624
Meng, M., and Qu, D. (2022). Understanding the green energy efficiencies of provinces in China: a Super-SBM and GML analysis. Energy 239, 121912. doi:10.1016/j.energy.2021.121912
Pan, X., Wang, M., and Li, M. (2023). Low-carbon policy and industrial structure upgrading: based on the perspective of strategic interaction among local governments. Energy Policy 183, 113794. doi:10.1016/j.enpol.2023.113794
Qi, X., Guo, Y., Guo, P., Yao, X., and Liu, X. (2022). Do subsidies and R&D investment boost energy transition performance? Evidence from Chinese renewable energy firms. Energy Policy 164, 112909. doi:10.1016/j.enpol.2022.112909
Reijnders, L. (2003). Policies influencing cleaner production: the role of prices and regulation. J. Clean. Prod. 11, 333–338. doi:10.1016/s0959-6526(02)00027-6
Schulz, F., and Rode, J. (2022). Public charging infrastructure and electric vehicles in Norway. Energy Policy 160, 112660. doi:10.1016/j.enpol.2021.112660
Shang, W.-L., and Lv, Z. (2023). Low carbon technology for carbon neutrality in sustainable cities: a survey. Sustain. Cities Soc. 92, 104489. doi:10.1016/j.scs.2023.104489
Shao, J., and Wang, L. (2024). The role of different paths of technological progress in improving China’s energy efficiency. Energy and Environ. 35, 2008–2030. doi:10.1177/0958305X221148284
Song, L., Yuan, J., and Li, T. (2024). Harmonizing digital trade for sustainable stride: unveiling the industrial pollution reduction effect of China’s cross-border E-commerce comprehensive pilot zones. J. Environ. Manag. 370, 122834. doi:10.1016/j.jenvman.2024.122834
Song, L., Yuan, J., and Li, T. (2025). The impact of decentralization on carbon productivity in Chinese manufacturing: the perspective of industrial policy. Pol. J. Environ. Stud. doi:10.15244/pjoes/188596
Song, L., and Zhou, X. (2021). How does industrial policy affect manufacturing carbon emission? Evidence from Chinese provincial sub-sectoral data. Environ. Sci. Pollut. Res. 28, 61608–61622. doi:10.1007/s11356-021-15107-7
Song, M., Peng, L., Shang, Y., and Zhao, X. (2022). Green technology progress and total factor productivity of resource-based enterprises: a perspective of technical compensation of environmental regulation. Technol. Forecast. Soc. Change 174, 121276. doi:10.1016/j.techfore.2021.121276
Song, Q., Qin, M., Wang, R., and Qi, Y. (2020). How does the nested structure affect policy innovation empirical research on China’s low carbon pilot cities. Energy Policy 144, 111695. doi:10.1016/j.enpol.2020.111695
Stern, N., and Xie, C. (2023). China’s new growth story: linking the 14 th Five-Year Plan with the 2060 carbon neutrality pledge. J. Chin. Econ. Bus. Stud. 21, 5–25. doi:10.1080/14765284.2022.2073172
Su, Y., and Fan, Q. (2022). Renewable energy technology innovation, industrial structure upgrading and green development from the perspective of China’s provinces. Technol. Forecast. Soc. Change 180, 121727. doi:10.1016/j.techfore.2022.121727
Sun, Y., Gao, P., and Razzaq, A. (2023). How does fiscal decentralization lead to renewable energy transition and a sustainable environment? Evidence from highly decentralized economies. Renew. Energy 206, 1064–1074. doi:10.1016/j.renene.2023.02.069
Tan, L., Yang, Z., Irfan, M., Ding, C. J., Hu, M., and Hu, J. (2024). Toward low-carbon sustainable development: exploring the impact of digital economy development and industrial restructuring. Bus. Strat. Env. 33, 2159–2172. doi:10.1002/bse.3584
Tao, M., Wen, L., Sheng, M. S., and Poletti, S. (2024). Appraising the role of energy conservation and emission reduction policy for eco-friendly productivity improvements: an entropy-balancing DID approach. Energy Econ. 132, 107422. doi:10.1016/j.eneco.2024.107422
Tian, X., Bai, F., Jia, J., Liu, Y., and Shi, F. (2019). Realizing low-carbon development in a developing and industrializing region: impacts of industrial structure change on CO2 emissions in southwest China. J. Environ. Manag. 233, 728–738. doi:10.1016/j.jenvman.2018.11.078
Tu, C., Liang, Y., and Fu, Y. (2024). How does the environmental attention of local governments affect regional green development? Empirical evidence from local governments in China. Humanit Soc. Sci. Commun. 11 (1): 1–14. doi:10.1057/s41599-024-02887-9
Usman, M., and Balsalobre-Lorente, D. (2022). Environmental concern in the era of industrialization: can financial development, renewable energy and natural resources alleviate some load? Energy Policy 162, 112780. doi:10.1016/j.enpol.2022.112780
Veugelers, R., Tagliapietra, S., and Trasi, C. (2024). Green industrial policy in europe: past, present, and prospects. J. Ind. Compet. Trade 24, 4. doi:10.1007/s10842-024-00418-5
Wang, X., Su, Z., and Mao, J. (2023). How does haze pollution affect green technology innovation? A tale of the government economic and environmental target constraints. J. Environ. Manag. 334, 117473. doi:10.1016/j.jenvman.2023.117473
Wang, Y., and Wang, W. (2024). How green industrial policy affects the constancy of green technology innovation: a fresh proof from the innovation motivation perspective. Environ. Res. Commun. 6, 035027. doi:10.1088/2515-7620/ad35ac
Wu, L., Sun, L., Qi, P., Ren, X., and Sun, X. (2021). Energy endowment, industrial structure upgrading, and CO2 emissions in China: revisiting resource curse in the context of carbon emissions. Resour. Policy 74, 102329. doi:10.1016/j.resourpol.2021.102329
Wu, Y., Zhu, X., and Groenewold, N. (2019). The determinants and effectiveness of industrial policy in China: a study based on Five-Year Plans. China Econ. Rev. 53, 225–242. doi:10.1016/j.chieco.2018.09.010
Xu, G., Schwarz, P., and Yang, H. (2020). Adjusting energy consumption structure to achieve China’s CO2 emissions peak. Renew. Sustain. Energy Rev. 122, 109737. doi:10.1016/j.rser.2020.109737
Xu, J., Guan, Y., Oldfield, J., Guan, D., and Shan, Y. (2024a). China carbon emission accounts 2020-2021. Appl. Energy 360, 122837. doi:10.1016/j.apenergy.2024.122837
Xu, L. (2022). Towards green innovation by China’s industrial policy: evidence from made in China 2025. Front. Environ. Sci. 10. doi:10.3389/fenvs.2022.924250
Xu, P., Adebayo, T. S., Ahmad Khan, K., Özkan, O., and Khajimuratov Shukurullaevich, N. (2024b). United States’ 2050 carbon neutrality: myth or reality? Evaluating the impact of high-tech industries and green electricity. J. Clean. Prod. 440, 140855. doi:10.1016/j.jclepro.2024.140855
Yang, G., Nie, Y., Li, H., and Wang, H. (2023). Digital transformation and low-carbon technology innovation in manufacturing firms: the mediating role of dynamic capabilities. Int. J. Prod. Econ. 263, 108969. doi:10.1016/j.ijpe.2023.108969
Yang, M., Hong, Y., and Yang, F. (2022a). The effects of Mandatory Energy Efficiency Policy on resource allocation efficiency: evidence from Chinese industrial sector. Econ. Analysis Policy 73, 513–524. doi:10.1016/j.eap.2021.11.012
Yang, X., Nielsen, C. P., Song, S., and McElroy, M. B. (2022b). Breaking the hard-to-abate bottleneck in China’s path to carbon neutrality with clean hydrogen. Nat. Energy 7, 955–965. doi:10.1038/s41560-022-01114-6
Yu, Z., Chen, J., and Dong, J. (2020). The road to a low-carbon economy: the perspective of industrial plans. Econ. Res. J. 55, 116–132.
Yuan, X., and Zuo, J. (2011). Transition to low carbon energy policies in China—from the Five-Year Plan perspective. Energy policy 39, 3855–3859. doi:10.1016/j.enpol.2011.04.017
Zeng, S., Jin, G., Tan, K., and Liu, X. (2023). Can low-carbon city construction reduce carbon intensity?Empirical evidence from low-carbon city pilot policy in China. J. Environ. Manag. 332, 117363. doi:10.1016/j.jenvman.2023.117363
Zhang, A., Deng, R., and Wu, Y. (2022). Does the green credit policy reduce the carbon emission intensity of heavily polluting industries evidence from China’s industrial sectors. J. Environ. Manag. 311, 114815. doi:10.1016/j.jenvman.2022.114815
Zhang, F., Deng, X., Phillips, F., Fang, C., and Wang, C. (2020a). Impacts of industrial structure and technical progress on carbon emission intensity: evidence from 281 cities in China. Technol. Forecast. Soc. Change 154, 119949. doi:10.1016/j.techfore.2020.119949
Zhang, Y. (2015). Reformulating the low-carbon green growth strategy in China. Clim. Policy 15, S40–S59. doi:10.1080/14693062.2015.1094726
Zhang, Y., Li, X., Jiang, F., Song, Y., and Xu, M. (2020b). Industrial policy, energy and environment efficiency: evidence from Chinese firm-level data. J. Environ. Manag. 260, 110123. doi:10.1016/j.jenvman.2020.110123
Zhao, J., Jiang, Q., Dong, X., Dong, K., and Jiang, H. (2022). How does industrial structure adjustment reduce CO2 emissions? Spatial and mediation effects analysis for China. Energy Econ. 105, 105704. doi:10.1016/j.eneco.2021.105704
Zhao, S., Zhang, L., An, H., Peng, L., Zhou, H., and Hu, F. (2023). Has China’s low-carbon strategy pushed forward the digital transformation of manufacturing enterprises? Evidence from the low-carbon city pilot policy. Environ. Impact Assess. Rev. 102, 107184. doi:10.1016/j.eiar.2023.107184
Zhao, X., Zhang, X., and Shao, S. (2016). Decoupling CO2 emissions and industrial growth in China over 1993–2013: the role of investment. Energy Econ. 60, 275–292. doi:10.1016/j.eneco.2016.10.008
Zhou, B., and Zhao, S. (2022). Industrial policy and corporate investment efficiency. J. Asian Econ. 78, 101406. doi:10.1016/j.asieco.2021.101406
Zhou, T., Huang, X., and Zhang, N. (2023). The effect of innovation pilot on carbon total factor productivity: quasi-experimental evidence from China. Energy Econ. 125, 106895. doi:10.1016/j.eneco.2023.106895
Zhou, X., Pan, Z., Shahbaz, M., and Song, M. (2020). Directed technological progress driven by diversified industrial structural change. Struct. Change Econ. Dyn. 54, 112–129. doi:10.1016/j.strueco.2020.04.013
Zhou, X. L., and Song, L. (2022). Low-carbon transformation of China’s industry: realistic analysis and policy thinking. J. Quantitative 39, 22. doi:10.13653/j.cnki.jqte.2022.08.001
Zhu, J., Fan, Y., Deng, X., and Xue, L. (2019). Low-carbon innovation induced by emissions trading in China. Nat. Commun. 10, 4088. doi:10.1038/s41467-019-12213-6
Zhu, W., Zhu, Y., Lin, H., and Yu, Y. (2021). Technology progress bias, industrial structure adjustment, and regional industrial economic growth motivation —— research on regional industrial transformation and upgrading based on the effect of learning by doing. Technol. Forecast. Soc. Change 170, 120928. doi:10.1016/j.techfore.2021.120928
Keywords: targeted industrial policy, five-year plan, manufacturing, low-carbon transformation, SBM-GML JEL classification codes: O13; O25; Q01; Q56
Citation: Song L, Yuan J, Li T and Zhang J (2025) Can targeted industrial policy drive low-carbon transformation? evidence from China’s Five-Year Plans. Front. Environ. Sci. 13:1564489. doi: 10.3389/fenvs.2025.1564489
Received: 21 January 2025; Accepted: 24 March 2025;
Published: 25 April 2025.
Edited by:
Jinyu Chen, Central South University, ChinaReviewed by:
Xufeng Cui, Zhongnan University of Economics and Law, ChinaWenjuan Dong, Tsinghua University, China
Copyright © 2025 Song, Yuan, Li and Zhang. This is an open-access article distributed under the terms of the Creative Commons Attribution License (CC BY). The use, distribution or reproduction in other forums is permitted, provided the original author(s) and the copyright owner(s) are credited and that the original publication in this journal is cited, in accordance with accepted academic practice. No use, distribution or reproduction is permitted which does not comply with these terms.
*Correspondence: Jianzhong Zhang, NDA0MDY4NjgxQHFxLmNvbQ==