- School of Foreign Languages, Xiamen Institute of Technology, Xiamen, China
China’s rapid economic growth and the influx of foreign investment have greatly contributed to increased pollutant emissions. As a result, the country faces growing challenges in balancing environmental protection with green economic development. It is crucial to understand the spatial variation in foreign direct investment (FDI) and environmental pollution across cities, as well as the impact of pollution during periods of severe environmental degradation. This study analyzes the spatial distribution and changes over time of FDI and environmental pollution in Chinese cities from 2010 to 2019. It also explores the spatial effects of environmental pollution on FDI. Using a generalized differences-in-differences (DID) model, the research examines how China’s Atmospheric Key Control Zone (AKCZ) policy has affected FDI in cities and their neighboring areas. The results show that cities with higher pollution levels attract less FDI, and pollution in one city can deter investment in surrounding areas. On the other hand, better air quality in a city tends to encourage FDI in nearby regions. The AKCZ policy has a positive impact on FDI within the designated cities but causes a “siphoning” effect in neighboring areas. Well-designed environmental policies are essential for aligning environmental protection with the attraction of foreign investment, ultimately supporting the sustainable development of urban economies.
1 Introduction
Since China’s reform and opening-up, the country has laid a strong foundation for the entry of foreign direct investment (FDI). According to the National Bureau of Statistics of China, the country’s outbound FDI stock reached $3.66 trillion in 2023. FDI inflows amounted to $163.254 billion, accounting for 12.26% of global FDI flows—an increase of 177 times from $920 million in 1983. As the largest developing country, China continues to need significant capital to achieve domestic industrial upgrading and sustainable economic growth (Chen et al., 2023).
The rapid expansion of the economy has led to a sharp rise in China’s consumption of traditional fossil fuels, contributing to severe environmental degradation. This has placed immense pressure on China’s ecological protection efforts and green economic development initiatives, positioning the country as one of the world’s largest polluters, with carbon emissions accounting for 30% of global emissions (Shan et al., 2018; Lu et al., 2025). At the 27th Conference of the Parties to the Paris Agreement, the Chinese government formally committed to addressing environmental issues by implementing a series of measures, including energy transition, wastewater treatment, soil pollution control, and carbon sequestration (Zhang and Chen, 2022; Zhang et al., 2023; Lu et al., 2024; Cong et al., 2024). However, given the current state of environmental governance and industrial development, China must guide industries to attract high-quality FDI to fulfill its environmental commitments. Understanding how environmental policies attract FDI is key to achieving synergistic and sustainable economic development.
This paper empirically examines the spatial impact of environmental issues on FDI by selecting relevant environmental variables and comprehensive panel data from 253 Chinese cities, which are matched with city-level FDI data. The core explanatory variables, including SO2 emissions, industrial soot emissions, and PM2.5 levels, represent environmental challenges. These variables capture both emissions and pollution data, allowing for a thorough analysis of how environmental issues affect FDI in Chinese cities. Additionally, the study evaluates the spatial effects of China’s large-scale environmental policies on FDI and their influence on neighboring cities.
The key contribution of this paper is its focus on a significant gap in existing research on environmental issues and the impact of environmental policies on FDI. Expanding on previous studies, the paper explores the FDI landscape in Chinese cities and uses spatial modeling to investigate whether an environmental haven hypothesis exists in terms of these cities’ appeal to FDI. As the world’s largest energy consumer and second-largest economy, with a massive population and vast development potential, China faces challenges of uneven regional development. This study explores the combined effects of environmental problems and policies on FDI, offering a theoretical foundation for cities to develop environmental policies that align with FDI attraction and promote the green and sustainable growth of urban economies.
The structure of the paper is as follows: Section 2 presents the literature review, Section 3 outlines the research methods and data sources, and Section 4 discusses the results of the spatiotemporal analysis of the impact of environmental pollution and policies on FDI. Finally, the paper concludes with a summary of the research findings and policy recommendations related to environmental pollution and FDI attraction.
2 Literature review and theoretical framework
2.1 Literature review
Most empirical research has focused on the “pull” factors that attract FDI. Key factors identified in the literature include political stability, openness to trade, economic growth rate, market size, labor market, financial credit, infrastructure, taxation, and education. Table 1 summarizes several studies on the impact of FDI, providing an empirical analysis of how these and other factors influence FDI inflows.
2.2 Theoretical foundations
The pollution haven hypothesis is a widely studied and supported theory in research on environmental issues related to FDI. It suggests that polluting industries are likely to relocate to countries with weaker environmental regulations (Levinson, 2018; Singhania and Saini, 2021; He, 2006; Ren et al., 2014). For example, Yoon and Heshmati (2021) research indicates that South Korea’s manufacturing investment, including foreign investment, tends to favor countries with less stringent environmental regulations. In their study of FDI in Chinese provinces, Xiong and Chen (2022) found that environmental regulations have had a negative impact on FDI over the last 2 decades. JinRu et al. (2023) also support the pollution haven hypothesis with their analysis of FDI in Belt and Road countries from 1990 to 2020. On the other hand, Ge, Hu, and Ren (2020) argue that the impact of China’s environmental regulations on FDI depends on the technological level of foreign firms, with the pollution haven hypothesis being insignificant for high-tech firms. Similarly, Yang et al. (2018) examined the heterogeneity of environmental regulations’ impact on FDI, revealing that while environmental regulations generally have a negative effect, there is regional variation within China. Additionally, Gök et al. (2024) explored how different pollution levels affect FDI, noting that higher carbon emissions attract FDI in countries with lax environmental standards, while deterring FDI in countries with stricter regulations. Cheng et al. (2018) found that FDI and environmental regulations influence each other bidirectionally, with increased FDI strengthening regional environmental regulations, which weakens the pollution haven hypothesis. These studies suggest that while the pollution haven hypothesis is prevalent, its impact varies across regions and is influenced by a range of factors, often diminishing in areas with stricter regulations. This phenomenon is partly due to some countries prioritizing GDP growth over environmental issues, as seen in the case of China (Temurshoev, 2006). Xu et al. (2024) also argue that high economic growth targets can reduce public participation in environmental monitoring once foreign investors are introduced.
The Porter hypothesis offers an alternative view, suggesting that stringent environmental regulations can increase a city’s competitiveness and attract FDI in the long run (Leeuwen and Mohnen, 2017; Porter, 1991). Empirical studies have provided support for this hypothesis. For instance, Wu et al. (2022) show that greater environmental information disclosure encourages FDI. Additionally, strong environmental regulations contribute to both increased FDI inflows and the sustainable development of host regions (Ferrara et al., 2014). Huang and Li (2023) find that environmental taxation in China has greatly boosted FDI following legal reforms. Li and Xiao (2024) highlight that green business environments improve the quality of regional FDI, while Wang and Zhang (2022) demonstrate that enhanced environmental regulations have increased FDI in China’s industrial sector. Liu et al. (2023) emphasize that well-designed environmental regulations can mediate the relationship between FDI and carbon emissions, with FDI reducing regional carbon emissions under effective regulatory frameworks.
While both the pollution haven and Porter hypotheses have been widely explored, their conclusions vary based on regional contexts and the stringency of policies. Some studies confirm that lax environmental standards attract pollution-intensive industries, while others argue that stringent regulations enhance the quality of FDI. However, research on the spatial effects of environmental pollution and policies on FDI remains limited, especially given China’s rapid urbanization and uneven regional development. Many existing studies overlook multidimensional environmental variables and fail to consider the spillover effects on neighboring regions. This study addresses these gaps by using various environmental indicators and policy variables to comprehensively assess the spatial impacts of environmental issues on FDI. It further investigates how large-scale environmental policies, such as China’s AKCZ, influence FDI in pilot cities and their neighboring areas. By integrating spatial econometric models and generalized DID approaches, this research aims to provide a detailed understanding of how environmental challenges and policies shape FDI dynamics. Based on the Porter hypothesis, this study proposes the following hypothesis: China’s environmental policies, by improving environmental quality and signaling a green transition, strongly promote FDI inflows into pilot cities.
3 Methods and data
3.1 Spatial and temporal characteristics of population movements
3.1.1 Standard deviation ellipse modeling
The research analyzes the distribution direction, spatial dispersion, and migration patterns of FDI’s center of gravity across Chinese cities by calculating the parameters of the ellipse’s center, its major and minor axes, and the azimuth angle using the standard ellipse model (Bachi, 1963). Key parameters of the model include the standard deviation along both the long and short axes as shown in Equations 1– 5:
Ellipse Center:
Azimuth angle:
3.1.2 Spatial weighting matrices
The spatial weight matrix forms the foundation of spatial correlation analysis, reflecting the degree of association between two regions. In this study, three types of spatial matrices are constructed: the spatial distance matrix (W1), which measures the degree of association between cities based on their physical distance; the spatial adjacency matrix (W2), which assesses the association between cities based on their proximity; and the economic distance matrix (W3), which evaluates the degree of correlation between cities based on their economic relationships. The general form of these matrices is as shown in Equation 6:
The spatial adjacency matrix is constructed using a binary adjacency matrix if city i and city j are adjacent or connected (Equation 7):
If city i and city j are not neighboring or connected (Equation 8).
In this study, the geospatial distance matrix is constructed primarily based on the latitude and longitude of the cities. The general form of this matrix is as shown in Equation 9:
The economic distance matrix combines both geographical distance and economic factors to create a spatial economic distance weight matrix. This method takes into account both the spatial characteristics of geographic distance and the economic relationships between cities. The general form of this matrix is expressed as shown in Equation 10:
where
3.1.3 Spatial autocorrelation test
Before applying spatial econometric models to analyze the spatial effects of environmental pollution on city FDI, spatial autocorrelation tests are conducted to confirm the presence of spatial autocorrelation and assess the feasibility of using spatial econometric modeling. In this study, the global Moran’s I statistic is used to evaluate the spatial autocorrelation of FDI and selected air pollution indicators. The formula is as shown in Equation 11:
where
(1) FDI and environmental pollution indicators exhibit no spatial autocorrelation (Moran’s I = 0).
(2) There is no significant spatial clustering of high-FDI or high-pollution cities.
3.2 Measurement models
3.2.1 Generalized differences-in-differences model
In this study, the generalized DID model is employed to examine the spatial and temporal effects of China’s Atmospheric Key Control Zone (AKCZ) policy on FDI in cities. This model also assesses the implementation effects of the AKCZ policy. To address potential issues of causality and endogeneity arising from the exogenous policy, fixed effects are included to enhance the robustness of the generalized DID model (Beck et al., 2010; Wang, 2013). Additionally, several commonly used control variables that influence FDI are incorporated into the model (Tanna et al., 2018; Lin and Ye, 2017; Zhao et al., 2024; Nguyen and Le, 2021; Liargovas and Skandalis, 2012; Asiedu, 2006; Othman et al., 2018; Wang et al., 2021; Kolstad and Villanger, 2008), resulting in the following econometric regression model (Equation 12):
where i represents the city and t denotes the year. The explanatory variable
The driving effect of the AKCZ policy on FDI in cities is examined by constructing a time heterogeneity model, as shown in Equation 13:
where
A spatial generalized DID model is established to study the spatial effect of AKCZ policy on FDI in neighboring cities, as shown in Equation 14:
Equation 14 introduces a new set of control variables,
In this paper, the PSM-DID method was further employed to conduct a robustness test. When the PSM-DID method was applied, a Logit regression was first performed on the control variables to estimate the propensity score values, and the treatment group and control group were then matched. After matching was completed, the DID method was used to compare the results before and after the pilot implementation.
3.2.2 Spatial measurement models
To estimate the impact of pollution on FDI, a baseline regression model is first applied, assuming a relationship between city pollution and FDI from 2010 to 2019. It also includes socio-economic control variables for the same period. The model is expressed as shown in Equation 15:
where
Relying solely on the benchmark regression analysis may underestimate the spillover effect of carbon emissions on population migration. To address this, the study uses spatial lag models (SLM), spatial error models (SEM), and spatial Durbin models (SDM) to analyze the impact of pollution on FDI. The SLM model incorporates endogenous interactions between variables and focuses on the spatial correlation of subregional explanatory variables (Cliff and Ord, 1982). This model includes a spatial lag term for the dependent variable to explicitly capture the impact of FDI in neighboring cities on FDI in the city under study. The formula for the model is as shown in Equation 16:
The SEM model accounts for the interaction of spatial error terms, but it does not directly analyze the spatial effects of explanatory variables. The model is formulated as shown in Equation 17:
The SDM model combines the spatial lag terms of both the independent and dependent variables, providing a more comprehensive analysis of spatial interactions (Lee and Yu, 2010; Durbin, 1960; Anselin, 1988). The model is as shown in Equation 18:
In Equations 16–18, δ, ρ, and θ denote the coefficients of
(1) Spatial autocorrelation of FDI is absent (
(2) Environmental pollution has no direct effect on FDI (
(3) Pollution spillovers to neighboring cities are non-existent (
3.3 Data sources
The AKCZ policy was implemented in 2013, focusing on key regions such as Beijing-Tianjin-Hebei, the Yangtze River Delta, and the Pearl River Delta, covering 47 cities (as shown in Figure 1). The policy aims to reduce SO2 and nitrogen oxide emissions in pilot cities, lower urban PM2.5 concentrations, and increase the number of days with favorable air quality. For example, in the Beijing-Tianjin-Hebei AKCZ pilot region, the average PM2.5 concentration decreased from about 106 μg/m3 in 2013 to 44 μg/m3 by 2020, a nearly 60% reduction. Similarly, the Yangtze River Delta pilot region saw a reduction of approximately 40% over the same period. Based on these developments, this study uses SO2 emissions, industrial soot emissions, and PM2.5 concentrations as core explanatory variables, analyzing their combined impact on FDI alongside environmental policy variables. Since the period after 2020 saw significant pandemic-related disruptions, leading to major deviations in environmental pollutant emissions and FDI and increased data heterogeneity, this research focuses on the 2010–2019 period for regression analysis. Treating the AKCZ implementation as a quasi-natural experiment, the study designates pilot cities as the experimental group and non-pilot cities as the control group, using a generalized DID model to evaluate the policy’s effect on promoting FDI in these urban areas.
The data used in this study consist of a comprehensive panel dataset from 253 prefecture-level cities in China, covering the period from 2010 to 2019. These data were sourced from the China City Statistical Yearbook, Local Statistical Yearbook, China Environmental Statistical Yearbook, and the Atmospheric Composition Analysis Group. Some variables have been transformed into their natural logarithmic form for empirical analysis. The descriptive statistics of these variables are presented in Table 2.
4 Results and discussion
4.1 Spatio-temporal characterization
4.1.1 Trends in the spatial and temporal evolution of FDI
Due to the significant gaps in FDI data for cities in western China, the standard deviation ellipse may be distorted. As a result, only data from eastern China are selected for this study. Six key time points in 2010, 2013, 2015, 2016, 2018, and 2019 are used to plot the standard deviation ellipse and the centroid of FDI in Chinese cities (Figure 2). The results reveal that from 2010 to 2019, the standard deviation ellipse generally contracted inward in the north. Both the ellipse and the center of gravity show a clear shift from 2016 to 2019, with the center of gravity moving notably southward. At the same time, the standard deviation ellipse shows noticeable inward contraction in the northeast. This suggests that the increase in FDI was more pronounced in southern cities compared to northern cities, with the FDI gap between southern and northern cities becoming more pronounced over time.
4.1.2 Spatial autocorrelation analysis
Table 3 presents the global Moran’s indices for FDI and pollution variables from 2010 to 2019, based on matrix W1. The global Moran’s index for each variable is positive and passes the significance test. The results indicate that FDI in Chinese cities exhibits significant spatial correlation. Similarly, PM2.5, SO2 emissions, and industrial soot emissions also show spatial correlation. These findings support the appropriateness of using spatial econometric models.
To better understand the spatial agglomeration characteristics and local spatial correlations of FDI and environmental pollution in cities, we calculated the Moran index for FDI across 253 Chinese cities and plotted the Moran scatterplot for 2019. As shown in Figure 3, most cities in China’s FDI and environmental pollution data fall in the first and third quadrants of the Moran scatterplot. This suggests a strong spatial clustering of both FDI and environmental pollution. The first quadrant shows that cities with high foreign investment are surrounded by other cities with similarly high FDI, such as those in the Yangtze River Delta and the Pearl River Delta. The third quadrant, on the other hand, represents cities with low FDI that are adjacent to other low-FDI regions. The Moran scatterplot for city pollution indicators reveals that cities with high pollutant emissions are located near other cities with high pollution levels, while cities with low emissions are clustered with other low-emission cities. Both FDI and city pollution exhibit high-high and low-low aggregation patterns.
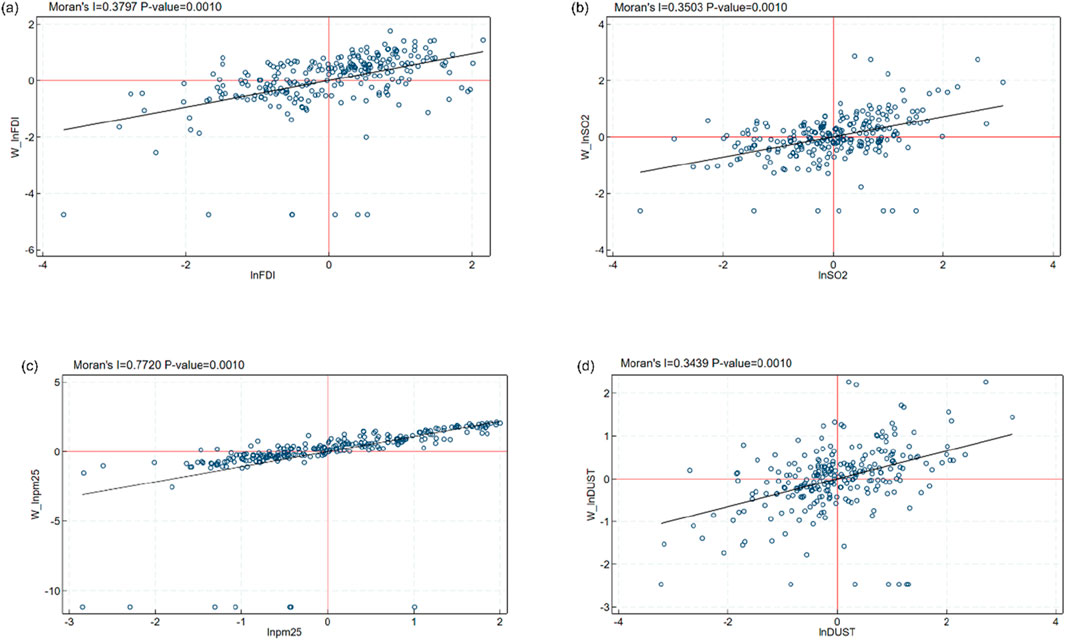
Figure 3. Moran scatterplot for 2019 (a): FDI, (b) SO2 emissions per capita, (c) average PM2.5 for the year, (d) industrial soot emissions per capita).
This study uses the LM test and the robust LM test to assess spatial correlation. The results in Table 4 show that the model exhibits spatial correlation at the 1% significance level. Both the Wald test and the LR test are significant at the 1% level, confirming that the model does not degenerate into either the SLM or SEM. Therefore, the SDM is selected as the primary model. Additionally, the SLM and SEM are used for robustness checks to verify the consistency of the findings.
4.2 Spatial measurement model
4.2.1 Spatial measurement model results
Table 5 presents the regression results for the baseline model with year and city fixed effects. The results show that the SO2 emission coefficient is negative and statistically significant at the 5% level, with an estimated value of −0.101. This suggests that an increase in SO2 emissions significantly discourages foreign investment inflows. As a major atmospheric pollutant, SO2 is a precursor to environmental acidification, and its excessive emissions may act as a deterrent to investment by multinational companies (Malik and Kumar 2021; Zhang et al., 2021). The estimated coefficient for industrial soot emissions, another air pollutant, is also negative. Unlike SO2, industrial soot is primarily respiratory in nature and a major source of respirable particulate matter in the air. PM2.5, a direct indicator of air pollution, is negative and significant at the 1% level, serving as a direct explanatory variable for environmental pollution while reflecting the current state of air quality in the city. These results indicate that environmental issues hinder FDI inflows, with foreign enterprises preferring to invest in cities with better environmental quality and governance.
This study estimates the spatial effects of each environmental factor on FDI in Chinese cities from 2010 to 2019 using the SDM model with double fixed effects for year and city, and compares the results from SEM and SLM to enhance the robustness of the model. The SDM estimation results align with those from the baseline regression model, with the effects of the three environmental pollution variables on FDI remaining negative and statistically significant at the 1% level. The coefficients for lnPM25, lnDUST, and lnSO2 are −1.605, −0.069, and −0.096, respectively. The spatial spillover effect of annual average PM2.5 and pollutant emissions from cities to neighboring cities yields opposite results. The coefficient for lnPM25 is significantly positive at the 1% level, with a spillover effect coefficient of 1.187. This suggests that deteriorating air quality in a city can lead foreign-invested companies to invest in geographically adjacent cities. However, poor air quality in the city encourages them to choose neighboring cities instead. Nevertheless, pollutant emissions are generally controlled by regional environmental policies, and city emissions often affect the environment in surrounding areas. As a result, cities with high pollutant emissions substantially reduce the ability of neighboring cities to attract foreign investment.
According to Lesage and Pace’s theory (Lesage and Pace, 2008), the main regression coefficients may not accurately reflect the marginal effect of environmental pollution variables on FDI when the spatial lag term
The regression results show that the direct effect coefficients for industrial soot emissions and SO2 emissions on FDI are substantially negative, with coefficients of −0.091 and −0.118, respectively. This indicates that each 1-unit increase in lnDUST and lnSO2 in a neighboring city can reduce lnFDI in the city by 0.308 and 0.318, respectively. These findings suggest that industrial soot and SO2 emissions in a city can greatly diminish the ability of both that city and its neighboring cities to attract foreign investment.
4.2.2 Robustness tests
In addition to using the adjacency matrix for spatial regression analysis, this study also applies the geographical distance matrix (W2) and the economic distance matrix (W3) to conduct robustness checks. The detailed regression results are presented in Table 7.
Based on the results in Table 7, the findings remain robust after replacing the spatial weight matrix, consistently aligning with the outcomes of the spatial model using the W1 matrix. The direct, indirect, and total effects of SO2 and industrial soot emissions on FDI are all negative, indicating that SO2 and industrial soot emissions in urban areas reduce the ability of both the city and its neighboring regions to attract foreign investment. In contrast, the direct effect of PM2.5 on FDI is negative, while its indirect effect is positive. This suggests that an increase in air pollutants in adjacent areas paradoxically boosts FDI inflows into the local city.
4.2.3 Heterogeneity analysis
The previous analysis shows that the spatial model results for the three pollution explanatory variables remain robust even after substituting the spatial weight matrix. However, due to geographical differences, cities vary in resource endowments and economic conditions, which could result in disparities in how pollution impacts urban FDI. Therefore, further exploration of this heterogeneity is needed. The spatial analysis in Section 4.1 indicates that the center of gravity for FDI in China is located in the south, with a noticeable southward shift, and southern cities tend to have higher FDI levels than their northern counterparts. This study divides Chinese cities into northern and southern regions using the Qinling-Huaihe Line. The specific regression results are shown in Table 8.
The results in Table 8 show that the direct suppressive effect of PM2.5 on FDI is significantly stronger in northern cities than in southern ones. In northern cities, both industrial soot and SO2 emissions have a pronounced negative impact on FDI, while in southern cities, only industrial soot emissions exhibit a marginal inhibitory effect. This disparity may be due to the prevalence of heavy industry in northern cities, which results in higher pollutant emissions and, consequently, greater sensitivity to air quality among foreign enterprises. These findings are consistent with the conclusions of Yang et al. (2018) on regional heterogeneity in China’s environmental regulations.
The results also indicate that an increase in local PM2.5 concentration markedly promotes FDI inflows into neighboring cities. This phenomenon may arise because PM2.5, as a direct indicator of urban air quality, often triggers coordinated regional governance. Neighboring cities attract environmentally sensitive foreign investment by developing ecological industrial parks or clean energy networks, creating a synergistic effect through regional pollution pressures and green upgrading. A rise in PM2.5 signals the urgency of regional environmental governance, prompting foreign investors to focus on the green technology strategies of neighboring cities and invest proactively. In contrast, SO2 emissions and industrial soot have a significant negative impact on FDI in both the local city and its surrounding areas. This difference stems from the type of pollution and its industrial associations; SO2 and industrial soot are typically linked to heavy industry, and their emissions not only worsen environmental degradation but also signal a high-energy-consumption industrial structure. Foreign enterprises are likely to avoid such regions to reduce risks to their production environment and corporate image. Furthermore, SO2 emissions from heavy industrial cities may spill over to neighboring provinces, creating a “pollution chain effect” that reduces the region’s attractiveness, a pattern that is particularly noticeable in northern cities dominated by heavy industry.
4.3 Generalized DID modeling results
4.3.1 Generalized DID model benchmark regression
The strength of the generalized DID model lies in its ability to create a quasi-natural experiment through an exogenous policy shock (the AKCZ pilot), effectively controlling for the complex spatiotemporal factors that influence the policy. Table 9 presents the results of the baseline regression for the generalized DID model with year and city fixed effects. Model (1) includes only the policy variable (DID), while model (2) incorporates all control variables. The estimation results of model (1) show that the AKCZ policy has a significant positive impact on city FDI, with a coefficient of 0.276 and a significance level of 1%. After including the control variables in model (2), the coefficient for DID remains significantly positive at the 1% level, confirming that the implementation of the AKCZ policy has substantially increased the capacity of pilot cities to attract FDI.
4.3.2 Tests for spatio-temporal heterogeneity
The time heterogeneity analysis of the FDI in cities following the AKCZ policy is conducted using Equation 13, with Figure 4 illustrating the changes in the FDI before and after the policy’s implementation at the 95% confidence level. The year 2013, marking the beginning of China’s AKCZ policy and the establishment of the pilot cities, shows a significant increase in the cities' ability to attract foreign investment in the early stages. There was a notable boost in FDI attraction right after the policy was introduced, with little lag in FDI growth. This can be attributed to foreign enterprises considering the city’s new policies as part of their investment decision-making process, focusing on evaluating the effects of these new policies.
Figure 5 shows the trend of FDI in relation to spatial distance, with a 95% confidence level, based on the estimation results of Equation 14. The results indicate that the siphoning effect of the AKCZ policy on the FDI of neighboring cities is more pronounced. Specifically, FDI in cities located within 150 km of the pilot city is not greatly affected due to the siphoning effect. This effect weakens as the distance from the pilot city exceeds 150 km, and FDI becomes significant again beyond 300 km, where the siphoning effect is no longer present. Within 150 km of the pilot city, FDI shows a decreasing trend with increasing distance. This may be due to the positive environmental impact of the AKCZ policy on neighboring cities, which weakens the siphoning effect, leading to a less significant decrease in FDI. As the distance increases, the influence of the AKCZ policy on the environment diminishes, and the siphoning effect becomes more pronounced. Overall, the trend of the AKCZ policy’s impact on population migration in surrounding areas first decreases and then stabilizes, with FDI in cities beyond the 150 km zone showing significant changes. This aligns with the general pattern of the siphoning effect.
4.3.3 Robustness tests
The use of the generalized DID model was tested in the previous section through the parallel trend test (Figure 3). A key assumption for the model is that there is no significant difference between the FDI of cities after the establishment of the AKCZ (treatment group) and before its establishment (control group). The results show that the variables before the establishment of the AKCZ are statistically insignificant, indicating that there is no significant difference in FDI between the cities in the treatment and control groups before the AKCZ was implemented.
Another important consideration is whether FDI is influenced by other regional environmental and economic policies. As key environmental control areas, the AKCZ pilot cities are often subject to additional environmental policies. To isolate the impact of the AKCZ policy, it is important to note that 27 of the AKCZ pilot cities in the sample are also low-carbon pilot cities, so the effect of the low-carbon city policy must be excluded. In addition to environmental policies, regional economic policies may also affect FDI. One such policy is the social credit system, which overlaps temporally with the AKCZ policy and aims to enhance financial system efficiency and unlock the positive effects of long-term credit, potentially influencing foreign investment decisions. To account for the influence of these additional policies on FDI, a DID model incorporating the AKCZ policy, low-carbon city policy, and social credit system policy is constructed based on Equation 12. This model fixes both year and city effects. In Model (2), the core variable DID is replaced with the low-carbon city policy variable, while in Model (3), it is replaced with the social credit system policy variable. Model (4) evaluates the combined impact of all three policy variables on FDI. The analysis results are presented in Table 10.
In the model, aside from the AKCZ policy, the effects of other policies on driving FDI are not significant. The effect of the AKCZ policy remains significant even after accounting for the additional policy variables, indicating that the AKCZ policy’s contribution to urban FDI is not driven by other economic or environmental policies. Although the low-carbon city policy is also an environmental policy, it differs in focus from the AKCZ policy. The low-carbon city policy primarily limits carbon emissions in the city, without addressing other air pollutants, while the AKCZ policy sets specific standards for major pollutants and targets implementation at the enterprise level. This focus on major pollutants has attracted significant investment from environmentally conscious foreign enterprises.
To increase the stability of the model, fixed effects and relevant control variables influencing city FDI were included. However, some other economic and demographic factors of cities may change over time and influence FDI. To test whether these factors affect the analysis results, an indirect placebo test was performed (Chetty et al., 2009; Ferrara et al., 2012). The results of the placebo test are shown in Figure 6. The results, after being randomized 1,000 times, are distributed around zero and follow a normal distribution, as expected in a placebo test.
To mitigate potential sample selection bias, this paper employs the PSM-DID method for robustness testing. In this approach, logit regression is conducted on the observable indicators using the dummy variable indicating whether a city is an AKCZ pilot. The propensity score matching value is then obtained. The city with the closest matching propensity score is paired with the AKCZ pilot city. Nearest neighbor matching, radius matching, and kernel matching methods were used to estimate the PSM-DID, and the results are presented in Table 11. The estimated coefficient for the pilot policy dummy variable remains significantly positive, indicating that the AKCZ policy has a positive effect on FDI. These results demonstrate the robustness of the findings.
The study shows that the AKCZ policy has a positive impact on FDI inflows to pilot cities, aligning with the Porter hypothesis, which argues that stringent environmental regulations can improve regional competitiveness and attract high-quality foreign investment (Huang and Li, 2023). These findings are in line with the research of Wang and Zhang (2022), which suggests that stronger environmental policies promote FDI growth in Chinese cities. This research also adds a new layer of understanding by highlighting the spatial differences in the policy’s effects, particularly the siphoning effect observed in cities within a 150-km radius of the pilot cities, where FDI decreases. This supports previous studies on the spillover effects of environmental policies (Cheng et al., 2018), emphasizing the need for coordinated regional governance to manage FDI dynamics between both local and neighboring areas under such policies.
5 Conclusion and policy implications
5.1 Conclusion
This paper examines 253 cities in China, analyzing the relationship between FDI and environmental issues. It estimates the direct and indirect effects of environmental pollution on FDI using spatial matrices and econometric models, and evaluates how environmental policies impact FDI in both the cities and their neighboring areas. The key findings are as follows:
(1) From 2010 to 2019, the distribution of FDI in eastern China shifted southward. Southern cities showed a stronger ability to attract foreign investment compared to their northern counterparts. The analysis also reveals a strong correlation between FDI and environmental pollution, with cities that attract more FDI often being surrounded by others with similar levels of investment. In contrast, cities with higher pollution tend to be located near other polluted areas.
(2) Environmental pollution greatly reduces FDI inflows. Not only does it affect the city experiencing high pollution, but it also impacts neighboring areas. However, higher annual average PM2.5 levels were found to encourage FDI in nearby cities, indicating a complex relationship between air quality and investment flows.
(3) The generalized DID model reveals that the AKCZ policy has a positive and significant effect on FDI in the pilot cities. After ruling out the impact of the low-carbon city policy, the results support the Porter hypothesis, which suggests that stringent environmental regulations can enhance regional competitiveness and attract high-quality foreign investment.
(4) The spatio-temporal analysis from the generalized DID model shows significant differences in FDI before and after the AKCZ policy was implemented. The siphoning effect of the policy on neighboring cities is most prominent within a 150 km radius, diminishing with increasing distance.
Through these analyses, the study highlights that foreign investors in Chinese cities tend to favor locations with strong environmental policies and robust regulatory frameworks for pollution control. While the research primarily focuses on air pollution, it acknowledges that other types of pollution, such as water and soil contamination, may also influence FDI decisions. Future research could explore the differential impacts of various pollution types on FDI. Additionally, cross-country studies could help determine if these findings hold in other developing countries, considering differences in environmental regulations and economic structures. Future studies could also utilize natural experiments and machine learning techniques to better understand the nonlinear relationships between environmental pollution and FDI.
5.2 Policy implications
Based on the findings, the following policy recommendations are proposed to align environmental governance with FDI attraction:
(1) Strengthen environmental regulation and transparency. Cities with high pollutant emissions should implement stricter emission policies and adopt advanced pollution control technologies. The success of the AKCZ policy demonstrates that clear and rational urban environmental policies send positive signals of environmental responsibility to foreign investors, enhancing FDI attractiveness.
(2) Develop joint environmental governance frameworks between pilot cities and their neighboring regions to address the negative spillover effects of pollution and environmental policies on FDI. For instance, establishing regional green industrial zones could transform potential “siphoning effects” into “synergistic effects,” enabling neighboring cities to benefit from the policy.
(3) Tailor environmental policies to regional differences, considering the shifting center of FDI. Policies should be customized based on regional characteristics. For example, northern cities with lower FDI competitiveness could prioritize air quality improvements through subsidies for renewable energy adoption, while southern cities, which experience higher FDI inflows, could sustain FDI by incentivizing enterprises to develop green technologies.
Data availability statement
The original contributions presented in the study are included in the article/supplementary material, further inquiries can be directed to the corresponding author/.
Author contributions
RS: Writing – original draft, Writing – review and editing.
Funding
The author(s) declare that financial support was received for the research and/or publication of this article. This research was funded by Xiamen Social Science Research Project funded by Xiamen Federation of Social Sciences and Xiamen Academy of Social Sciences, grant number: XM2025QND01
Conflict of interest
The author declares that the research was conducted in the absence of any commercial or financial relationships that could be construed as a potential conflict of interest.
Generative AI statement
The author(s) declare that no Generative AI was used in the creation of this manuscript.
Publisher’s note
All claims expressed in this article are solely those of the authors and do not necessarily represent those of their affiliated organizations, or those of the publisher, the editors and the reviewers. Any product that may be evaluated in this article, or claim that may be made by its manufacturer, is not guaranteed or endorsed by the publisher.
Abbreviations
FDI, Foreign direct investment; DID, differences-in-differences; AKCZ, Atmospheric Key Control Zone; SLM, Spatial lag model; SEM, Spatial error model; SDM, Spatial Durbin model; PSM, Propensity score matching; VIF, Variance inflation factor; LM test, Lagrange Multiplier test; LR test, Likelihood Ratio test; PM2.5, Particulate matter 2.5; SO2, Sulfur dioxide; CO2, Carbon dioxide.
References
Alam, Md, Tahir, M., and Omar, N. (2023). Do credit risks deter FDI? Empirical evidence from the SAARC countries. J. Econ. Finance Adm. Sci. 29, 42–56. doi:10.1108/JEFAS-09-2021-0191
Albahouth, A. A., and Muhammad, T. (2024). The relationship between trade openness and FDI inflows: evidence-based Insights from ASEAN region. Economies 12 (8), 208. doi:10.3390/economies12080208
Asiedu, E. (2006). “Foreign direct investment in Africa: the role of natural resources, market size, government policy, Institutions and political Instability.” World Econ. 29 (1): 63–77. doi:10.1111/j.1467-9701.2006.00758.x
Bachi, R. (1963). Standard distance measures and related methods for spatial analysis. Pap. Regional Sci. Assoc. 10 (1), 83–132. doi:10.1111/j.1435-5597.1962.tb00872.x
Beck, T., Levine, R., and Levkov, A. (2010). Big Bad Banks? The Winners and Losers from bank Deregulation in the United States. J. Finance 65 (5), 1637–1667. doi:10.1111/j.1540-6261.2010.01589.x
Bobenič Hintošová, A., Sudzina, F., and Barlašová, T. (2021). Direct and indirect effects of investment Incentives in Slovakia. J. Risk Financial Manag. 14 (2), 56. doi:10.3390/jrfm14020056
Chen, L., Wang, N., Li, Q., and Zhou, W. (2023). Environmental regulation, foreign direct investment and China’s economic development under the new normal: restrain or promote? Environ. Dev. Sustain. A Multidiscip. Approach Theory Pract. Sustain. Dev. 25 (5), 4195–4216. doi:10.1007/s10668-022-02239-0
Cheng, Z., Li, L., and Liu, J. (2018). The spatial correlation and interaction between environmental regulation and foreign direct investment. J. Regul. Econ. 54 (2), 124–146. doi:10.1007/s11149-018-9366-x
Chetty, R., Looney, A., and Kroft, K. (2009). Salience and taxation: theory and evidence. Am. Econ. Rev. 99 (4), 1145–1177. doi:10.1257/aer.99.4.1145
Cliff, A. D., and Keith Ord, J. (1982). Spatial processes: models and Applications. Q. Rev. Biol. doi:10.1111/j.1467-985X.2010.00681_13.x
Cong, L., Lu, S., Jiang, P., Zheng, T., Yu, Z., and Lü, X. (2024). CO2 sequestration and soil improvement in enhanced rock weathering: a review from an experimental perspective. Greenh. Gases Sci. Technol. 14, 1122–1138. doi:10.1002/ghg.2313
Durbin, J. (1960). The Fitting of time-series models. Revue de l'Institut Int. de Statistique/Rev. Int. Stat. Inst. 28 (3), 233–244. doi:10.2307/1401322
Ferrara, I., Missios, P., and Yildiz, H. M. (2014). Pollution havens, endogenous environmental policy and foreign direct investment. South. Econ. J., 140515071903005. doi:10.4284/0038-4038-2013.034
Ferrara, La, Eliana, A. C., and Duryea, S. (2012). Soap Operas and Fertility: evidence from Brazil. Am. Econ. J. Appl. Econ. 4 (4), 1–31. doi:10.1257/app.4.4.1
Gao, H., Chen, W., Li, J., and Zhou, T. (2024). Economic policy uncertainty and foreign direct investment: evidence from China. Struct. Change Econ. Dyn. 70, 178–191. doi:10.1016/j.strueco.2024.01.014
Ge, Y., Hu, Y., and Ren, S. (2020). Environmental regulation and foreign direct investment: evidence from China’s Eleventh and Twelfth Five-year Plans. Sustainability 12 (6), 2528. doi:10.3390/su12062528
Giner, J. M., and Giner, G. (2004). An interpretative model of foreign direct investment in China: an economic policy approach. China Econ. Rev. 15 (3), 268–280. doi:10.1016/j.chieco.2003.07.002
Gök, A., Ashraf, A., and Jasinska, E. (2024). The role of carbon emissions on inward foreign direct investment: a nonlinear dynamic panel data analysis. Sustainability 16 (13), 5550. doi:10.3390/su16135550
He, J. (2006). “Pollution haven hypothesis and environmental impacts of foreign direct investment: the case of industrial emission of sulfur dioxide (SO2) in Chinese provinces.” Ecol. Econ. 60 (1): 228–245. doi:10.1016/j.ecolecon.2005.12.008
Huang, J., and Li, Y. (2023). China’s environmental “fee-to-tax” and foreign direct investment—an empirical study based on intensity difference-in-differences. Front. Environ. Sci. 11. doi:10.3389/fenvs.2023.978388
Iamsiraroj, S. (2016). The foreign direct investment–economic growth nexus. Int. Rev. Econ. and Finance 42, 116–133. doi:10.1016/j.iref.2015.10.044
JinRu, L., Qamruzzaman, Md., Hangyu, Wu, and Kler, R. (2023). Corrigendum: do environmental quality, financial inclusion and good governance ensure the FDI sustainably in belt and road countries? Evidence from an application of CS-ARDL and NARDL. Front. Environ. Sci. 11. doi:10.3389/fenvs.2023.1154295
Kiptoo, J. (2024). The influence of political stability on foreign direct investment (FDI). Int. J. Dev. Country Stud. 6, 74–86. doi:10.47941/ijdcs.2165
Kolstad, I., and Villanger, E. (2008). “Determinants of foreign direct investment in services.” Eur. J. Political Econ. 24 (2): 518–533. doi:10.1016/j.ejpoleco.2007.09.001
Lee, L.-fei, and Yu, J. (2010). “Estimation of spatial autoregressive panel data models with fixed effects.” J. Econ. 154 (2): 165–185. doi:10.1016/j.jeconom.2009.08.001
Leeuwen, G. V., and Mohnen, P. (2017). Revisiting the Porter hypothesis: an empirical analysis of Green innovation for The Netherlands. Econ. Innovation New Technol. 26, 63–77. doi:10.1080/10438599.2016.1202521
Levinson, A. (2018). “Pollution haven hypothesis,” in The new Palgrave Dictionary of economics (London: Palgrave Macmillan UK), 10449–10454.
Li, X., and Xiao, L. (2024). “The impact of urban green business environment on FDI quality and its driving mechanism: evidence from China.” World Dev. 175: 106494. doi:10.1016/j.worlddev.2023.106494
Liargovas, P. G., and Skandalis, K. S. (2012). Foreign direct investment and trade openness: the case of developing economies. Soc. Indic. Res. 106 (2), 323–331. doi:10.1007/s11205-011-9806-9
Lin, S., and Ye, H. (2017). Foreign direct investment, trade credit, and Transmission of global Liquidity shocks: evidence from Chinese manufacturing firms. Rev. Financial Stud. 31 (1), 206–238. doi:10.1093/rfs/hhx087
Liu, H., Lei, H., and Zhao, S. (2023). The impact of FDI on industrial structure upgrading and carbon emission under the constraints of environmental regulation. Environ. Technol. 45, 5483–5500. doi:10.1080/09593330.2023.2295830
Lu, S., Yu, Z., Zhang, Y., and Xu, T. (2024). Review of non-isothermal processes in CCUS from a geomechanical perspective. Earth-Science Rev. 255, 104848. doi:10.1016/j.earscirev.2024.104848
Lu, S. Y., Jiang, P., Cong, L. H., and Lü, X. S. (2025). Research Progress on CO2 Geological Storage Reservoir and Caprock Mechanics: methods and Status. Greenh. GASES-SCIENCE Technol. doi:10.1002/ghg.2328
Malik, M. K., and Kumar, S. (2021). “Chapter 30 - sulfur dioxide: risk assessment, environmental, and health hazard,” in Hazardous Gases. Editors J. Singh, R. D. Kaushik, and M. Chawla (Academic Press), 375–389.
Martins, R., Cerdeira, J., Fonseca, M., and Mohamed, B. (2021). Foreign direct investment determinants in Mano River Union countries: Micro and macro evidence. South Afr. J. Econ. 90 (3), 408–424. doi:10.1111/saje.12301
Nguyen, C. Q., and Le, T.-Ha (2021). “The nexus between trade openness and foreign direct investment amid structural economic Vulnerability in developing countries,” in Globalization, Deglobalization, and new Paradigms in business. Editors J. Paul, and S. Dhir (Cham: Springer International Publishing), 61–86.
Nketiah-Amponsah, E., and Bernard, S. (2020). Ease of doing business and foreign direct investment: case of Sub-Saharan Africa. Int. Adv. Econ. Res. 26 (3), 209–223. doi:10.1007/s11294-020-09798-w
Oliveira, P., and Rosa, F. (2021). Labour market flexibility and FDI attraction: a macroeconomic analysis. Panoeconomicus 68 (3), 267–291. doi:10.2298/pan180116030o
Othman, N., Andaman, G., Yusop, Z., and Ismail, M. (2018). Impact of public expenditures on FDI inflows into developing countries. Pertanika J. Soc. Sci. Humanit. 26, 751–768.
Ren, S., Yuan, B., Ma, X., and Chen, X. (2014). “International trade, FDI (foreign direct investment) and embodied CO2 emissions: a case study of Chinas industrial sectors.” China Econ. Rev. 28: 123–134. doi:10.1016/j.chieco.2014.01.003
Shahbaz, M., Mateev, M., Abosedra, S., Ali Nasir, M., and Jiao, Z. (2020). Determinants of FDI in France: role of transport infrastructure, education, financial development and energy consumption. Int. J. Finance and Econ. 26 (1), 1351–1374. doi:10.1002/ijfe.1853
Shan, Y., Guan, D., Zheng, H., Ou, J., Yuan, Li, Meng, J., et al. (2018). China CO2 emission accounts 1997–2015. Sci. Data 5 (1), 170201. doi:10.1038/sdata.2017.201
Singhania, M., and Saini, N. (2021). “Demystifying pollution haven hypothesis: role of FDI.” J. Bus. Res. 123: 516–528. doi:10.1016/j.jbusres.2020.10.007
Tanna, S., Li, C., and Vita, G.De (2018). “The role of external debt in the foreign direct investment–growth relationship.” Int. J. Finance and Econ. 23 (4): 393–412. doi:10.1002/ijfe.1628
Temurshoev, U. (2006). “Pollution haven hypothesis or factor endowment hypothesis: theory and empirical Examination for the US and China.”
Wang, C., and Zhang, Y.-J. (2022). The effect of environmental regulation and skill premium on the inflow of FDI:Evidence from Chinese industrial sectors. Int. Rev. Econ. and Finance 81, 227–242. doi:10.1016/j.iref.2022.05.011
Wang, J. (2013). The economic impact of special economic zones: evidence from Chinese municipalities. J. Dev. Econ. 101, 133–147. doi:10.1016/j.jdeveco.2012.10.009
Wang, K.-L., He, S., and Zhang, F.-Q. (2021). Relationship between FDI, fiscal expenditure and green total-factor productivity in China: from the perspective of spatial spillover. PLOS ONE 16 (4), e0250798. doi:10.1371/journal.pone.0250798
Wu, C., Wu, X., Chen, X., and Xu, X. (2022). Research on the impact of environmental information disclosure on foreign direct investment based on the “new normal” in China. China Revista Internacional de Contaminaciónn Ambiental. doi:10.20937/rica.54429
Xiong, J., and Chen, L. (2022). Does industrial up-gradation, environment regulations, and resource allocation impact on foreign direct investment: empirical evidence from China. Front. Psychol. 13, 999953. doi:10.3389/fpsyg.2022.999953
Xu, Y., Du, Z., Kong, L., and Xu, K. (2024). Research on the impact of public environmental participation on foreign direct investment: evidence from China. Environ. Res. Commun. 6 (2), 025019. doi:10.1088/2515-7620/ad2a8f
Yang, Y., Niu, G., Tang, D., and Zhu, M. (2018). Does environmental regulation affect the introduction of foreign direct investment in China? --Empirical research based on the spatial Durbin model. Pol. J. Environ. Stud. 28 (1), 415–424. doi:10.15244/pjoes/83692
Yoon, H., and Heshmati, A. (2021). Do environmental regulations affect FDI decisions? The pollution haven hypothesis revisited. Sci. Public Policy 48 (1), 122–131. doi:10.1093/scipol/scaa060
Zhang, C., Quan, B., Tang, J., Cheng, K., Tang, Y., Shen, W., et al. (2023). “China's wastewater treatment: status quo and sustainability perspectives.” J. Water Process Eng. 53: 103708. doi:10.1016/j.jwpe.2023.103708
Zhang, S., and Chen, W. (2022). “China’s energy transition Pathway in a carbon Neutral Vision.” Engineering 14: 64–76. doi:10.1016/j.eng.2021.09.004
Zhang, X., Wang, Z., Cheng, M., Wu, X., Zhan, N., and Xu, J. (2021). “Long-term ambient SO2 concentration and its exposure risk across China inferred from OMI observations from 2005 to 2018.” Atmos. Res. 247: 105150. doi:10.1016/j.atmosres.2020.105150
Keywords: air pollution, environmental policy, Chinese cities, spatial effects, environmental economics
Citation: Su R (2025) Spatial effects of environmental factors on foreign direct investment: evidence from Chinese cities. Front. Environ. Sci. 13:1577657. doi: 10.3389/fenvs.2025.1577657
Received: 16 February 2025; Accepted: 02 April 2025;
Published: 30 April 2025.
Edited by:
Mohammad Haseeb, Wuhan University, ChinaReviewed by:
Naila Erum, MARA University of Technology, MalaysiaMohammad Subhan, Marwadi University, India
Samia Khalid, Xi’an Jiaotong University, China
Esmatullah Karimi, Wuhan University, China
Copyright © 2025 Su. This is an open-access article distributed under the terms of the Creative Commons Attribution License (CC BY). The use, distribution or reproduction in other forums is permitted, provided the original author(s) and the copyright owner(s) are credited and that the original publication in this journal is cited, in accordance with accepted academic practice. No use, distribution or reproduction is permitted which does not comply with these terms.
*Correspondence: Ruiqian Su, cnFzdTEyQDEyNi5jb20=