- 1Department of Gerontology, The First Affiliated Hospital of Ningbo University, Zhejiang, China
- 2Department of Anesthesiology, Beilun District People's Hospital, Ningbo, Zhejiang, China
- 3Meigu County People's Hospital, Liangshan Prefecture, Sichuan, China
- 4The Children's Hospital, Zhejiang University School of Medicine, National Clinical Research Center for Child Health, Hangzhou, China
- 5Department of Anesthesiology, The First Affiliated Hospital of Ningbo University, Zhejiang, China
- 6Department of Ultrasound in Medicine, The First Affiliated Hospital of Ningbo University, Ningbo, China
- 7The First People's Hospital of Yuexi County, Liangshan Prefecture, Sichuan, China
- 8School of Medicine, Zhejiang University, Hangzhou, Zhejiang, China
Background: The available data exhibit inconsistent findings regarding the association between obesity and sleep problems among older adults. The objective of this study was to assess the potential association between obesity and sleep disorders in the older population.
Methods: The data utilised in this cross-sectional investigation was obtained from the National Health and Nutritional Examination Survey (NHANES) conducted between 2005 and 2018. The study employed a multivariate logistic regression model and conducted subgroup analysis to evaluate the association between obesity and sleep disturbance.
Results: The investigation consisted of 2,570 older people who provided complete information, out of which 324 individuals satisfied the criteria for sleep disturbance. The findings from the multivariable adjusted logistic regression model indicate that individuals in the overweight and normal weight groups exhibited decreased odds of experiencing sleep disorder, as evidenced by the adjusted odds ratios (AOR) of 0.46 (95% confidence interval [CI] = 0.34–0.61) and 0.33 (95% CI = 0.22–0.47), respectively. These results were statistically significant (p < 0.001) when compared to individuals in the obese group. The investigation of age and gender subgroups demonstrated similar associations between various BMI categories and sleep disorders in the older population.
Conclusion: In summary, there exists a correlation between obesity and sleep disorders in the senior population. A significant association was observed between BMI and the likelihood of experiencing sleep disorders, indicating a dose–response relationship. Individuals with a higher BMI demonstrated a heightened likelihood of experiencing sleep disorders compared to those with a lower BMI.
Introduction
Obesity and sleep disturbances are prominent health issues that have received considerable attention due to their adverse impacts on personal welfare and the broader public health landscape (1–3). The global incidence of these illnesses has been progressively rising, placing a significant strain on healthcare systems and requiring a thorough comprehension of their correlation within distinct populations (4, 5). The aged population is particularly vulnerable due to age-related physiological changes, which render them more prone to a range of health issues (6, 7).
In recent years, a considerable body of research has been dedicated to investigating the correlation between obesity and sleep disturbances, emphasising the reciprocal nature of this connection (8, 9). Obesity, which is defined as the excessive buildup of adipose tissue in the body, has been associated with a wide range of detrimental health consequences, such as cardiovascular disorders, type 2 diabetes, and specific forms of cancer (10, 11). Moreover, obesity is widely acknowledged as a substantial risk factor in the emergence of sleep disorders, including obstructive sleep apnoea (OSA), insomnia, and restless leg syndrome (RLS) (12, 13). Sleep disturbances can significantly impact the quality of life and general health of individuals, resulting in symptoms such as daytime weariness, cognitive impairment, and increased morbidity.
The aged demographic constitutes a distinctive subset of individuals with discernible physiological and behavioural attributes. The process of ageing is frequently linked to alterations in body composition, hormone patterns, and sleep structure, all of which may contribute to the onset of obesity and sleep disorders (14). Furthermore, it is important to note that the aged demographic is especially susceptible to the negative consequences associated with these illnesses as a result of preexisting comorbidities and decreased functional capacities. The comprehension of the correlation between obesity and sleep disturbances in the senior population holds significant significance in the context of public health planning and the development of intervention methods.
This study aims to investigate the correlation between obesity and sleep disturbances in the older population by utilising data obtained from the National Health and Nutrition Examination Survey (NHANES) conducted during the period of 2005 to 2018. The National Health and Nutrition Examination Survey (NHANES) is a survey conducted in the US that aims to gather complete health-related data. This nationally representative survey collects information on several aspects, such as demographics, medical conditions, and sleep patterns. Through the examination of this comprehensive dataset, our objective is to examine the frequency of obesity and sleep disorders within the older demographic, research the probable variables that contribute to their simultaneous occurrence, and evaluate the effects of these illnesses on the overall health outcomes of this susceptible population.
Methods
Study population
The National Health and Nutrition Examination study (NHANES) is a comprehensive and representative study conducted in the US. It gathers extensive data on the nutrition and health status of the general population. The survey data collected by NHANES are made publically accessible, allowing researchers and data users to access and analyse the information. The National Centre for Health Statistics (NCHS) collects its statistics in biennial cycles (15). In order to get substantial samples for analytical purposes, we aggregated seven cycles of the continuous National Health and Nutrition Examination Survey (NHANES) data spanning from 2005 to 2018. The survey protocol was approved by the NCHS Research Ethics Review Board, and all participants provided signed informed permission. Additional information regarding the National Health and Nutrition Examination Survey (NHANES) can be accessed through the official website of the Centres for Disease Control and Prevention (CDC).1
Sleep disorders are more common in older people. Therefore, it is of special clinical significance to identify the factors affecting sleep disorders in the elderly population (14, 16). Out of a total of 70,190 participants obtained from the NHANES database, we eliminated individuals who were younger than 60 years old (n = 56,710), those who had incomplete data on sleep problem (n = 4,071), and those who were lacking information on other variables (n = 6,839). Ultimately, a comprehensive total of 2,570 individuals were incorporated into the scope of this research endeavour. Figure 1 illustrates the flowchart depicting the systematic selection procedure.
Exposure variates
Body mass index (BMI) was employed as a marker of obesity. BMI, serving as an indicator of total body fat, was determined by dividing the weight (in kilogrammes) by the square of the height (in metres). It was then categorised into four groups: underweight (< 18.5 kg/m2), normal weight (18.5–25 kg/m2), overweight (25–30 kg/m2), and obese (≥ 30 kg/m2) (17).
Covariates
Covariates considered include several demographic characteristics: Age, gender, race, education level, marital status, depression, poverty income ratio (PIR), smoking status, alcohol intake, total daily calories and caffeine intake, and recreational physical activity. Education level was assessed by the question “What is the highest grade or level of school you have completed or the highest degree you have received?” [less than high school/high school graduate or above]. The participants were categorised into two groups based on their marital status: those who had a spouse and those who did not. The assessment of depressive symptoms was conducted utilising the 9-item Patient Health Questionnaire (PHQ-9) depression scale. This scale has nine questions that are derived from the symptoms of depression outlined in the Diagnostic and Statistical Manual of Mental Disorders, Fourth Edition (DSM-IV). The scoring system for each of the nine items ranges from 0 (not at all) to 3 (nearly every day). By summing the scores of these nine things, a total score within the range of 0 to 27 can be obtained (18). In the present investigation, those who obtained PHQ-9 total scores equal to or greater than 10 were classified as exhibiting clinically significant depression (CRD) (19). The study sample was stratified into four distinct groups based on their alcohol consumption patterns: non-drinker, 1 to <5 drinks/month, 5 to <10 drinks/month, or 10+ drinks/month. The participants were queried regarding their lifetime consumption of 100 cigarettes and their present smoking habits in order to ascertain the presence of both current and former smokers. The individuals included in the study were classified as former smokers if they were not now engaged in smoking behaviour, but had previously consumed a minimum of 100 cigarettes in their lifetime. In addition, we incorporated the variable of total daily caloric intake as a potential covariate. Medical professionals conducted diagnoses of various diseases, such as diabetes and stroke, by inquiring participants with the question, “Have you ever been told by a doctor or health professional that you have __?.” The individuals reported several comorbid conditions of interest, namely congestive heart failure, chronic obstructive lung disease (including emphysema and/or chronic bronchitis), coronary artery disease, malignancy, and hypertension.
Outcome variable
The dependent variable in this study was the presence or absence of a sleep problem among the participants. An individual was classified as having a sleep disorder if they responded affirmatively to the query, “Have you been diagnosed with a sleep disorder?”
Statistical analysis
Continuous variables are shown as medians [interquartile ranges (IQRs)]; categorical variables are presented as total numbers and percentages. Comparisons between groups were made using the χ2 test for categorical variables and the Mann–Whitney U test for continuous variables, as appropriate. We divided the blood lead concentration into four categories. The study employed multivariate logistic regression analysis to investigate the association between BMI and the presence of sleep disorders. Sleep disorder-related factors were identified by referencing existing literature and utilising data from the NHANES database. Subsequently, factors with a significance level of p < 0.05 in inter-group single factor analysis were recognised as confounders for inclusion in subsequent multivariate regression analysis. Model 1 in its initial form did not account for any potential confounding variables. In Model 2, adjustments were made for gender and age. In addition to the variables adjusted by Model 2, there are other potential confounding factors that need to be considered. To evaluate the potential nonlinear association of sleep disorder with BMI, the restricted cubic spline (RCS) analysis was performed in multivariable-adjusted models. RCS fits the predictor variable by segmenting it into a series of polynomial functions, with each segment being fitted using a low-order polynomial. The study conducted subgroup analysis, stratifying the data by age and gender. The statistical analyses were conducted using R software (version 4.1.1), and a significance level of p < 0.05 was employed to determine statistical significance.
Results
Description of the study population
This study comprised a sample of 2,570 adults ranging in age from 60 to 85 years who had available data on sleep disorders. Among the people included in the study, 49.5% (n = 1,272) fell within the age range of 60–69 years. This was followed by individuals aged 70–79 years, accounting for 28.0% (n = 720) of the sample. Lastly, those aged 80 years and above constituted 22.5% (n = 578) of the participants. The gender distribution exhibited a reasonably similar proportion. A subset of the study population, comprising 12.6%, consisted of individuals who reported experiencing sleep difficulties. There were significant differences seen in age, gender, obesity, depression, alcohol intake, diabetes, stroke, and comorbidity index between the sleep disorder group and the non-sleep disorder group (p < 0.05; Table 1). Individuals diagnosed with sleep disorders were more likely to be aged 60–69 years, male, have higher rates of obesity, increased prevalence of depression, a medical history that includes diabetes and stroke, and a higher comorbidity index.
Baseline comparison of different BMI groups
Of the participants included in the study, 653 participants were between 18.5 and 25 kg/m2 (Normal weight group), 914 participants were between 25 and 30 kg/m2 (Overweight group), and 1,003 patients were ≥ 30 kg/m2 (Obese group). Table 1 lists the demographic features of all participants between different BMI groups. In different groups of BMI, age, gender, race, marital status, rates of depression, PIR, alcohol intake, smoking status, recreational physical activity, diabetes, comorbidity index, and rates of sleep disorder are significantly different (p < 0.05; Table 2).
Association of BMI with sleep disorder
The incidence of sleep disorder was significantly higher among patients with Obese group (19.6%; 197 of 1,003), compared with patients with Overweight group (9.4%; 86 of 914), and Normal weight group (6.3%; 41 of 653; p < 0.001; Table 2). We have used three multivariate logistic regression models to show the relationship between BMI with sleep disorder in Table 3: model 1, no covariate was adjusted; model 2, age, and gender were adjusted; model 3, age, gender, race, marital status, rates of depression, PIR, alcohol intake, smoking status, recreational physical activity, diabetes, comorbidity index, and rates of sleep disorder were adjusted. We found a significantly positive association between BMI with the incidence of sleep disorder in the unadjusted model (Model 1). In model 2, the ORs (95% CIs) after adjusting for age and gender for incidence of sleep disorder in participants with Overweight weight group and Normal group compared with those with Obese group were 0.40 (0.31–0.53), 0.27 (0.19–0.38), respectively (Table 3, p < 0.001). In model 3, the ORs (95% CIs) after adjusting for related indexes for incidence of sleep disorder in participants with Overweight weight group and Normal group compared with those with Obese group were 0.46 (0.34–0.61), 0.33 (0.22–0.47), respectively (Table 3, p < 0.001). For sensitivity analysis, different BMI levels group were transformed into a categorical variable, and the p value for the trend of different BMI levels group with categorical variables was consistent with the result of different BMI levels group as a continuous variable in the different models (Table 3, P trend < 0.001).
The nonlinear analyses of the association between continuous BMI and sleep disorder
Spline models with fully adjusted covariates were constructed to profile a more direct relationship between BMI and sleep disorder. A J-shaped association was observed between BMI and sleep disorder (p < 0.001, and nonlinear p = 0.186; Figure 2).
Subgroup analyses
Figure 3 presents subgroup analyses pertaining to the connection between various levels of BMI and the occurrence of sleep disorders. The individuals involved in the study were categorised into subgroups based on their age and gender. The findings of the study indicate a consistent and significant relationship between various BMI levels and the occurrence of sleep disorders across different subgroups (Figure 3, P trend < 0.05).
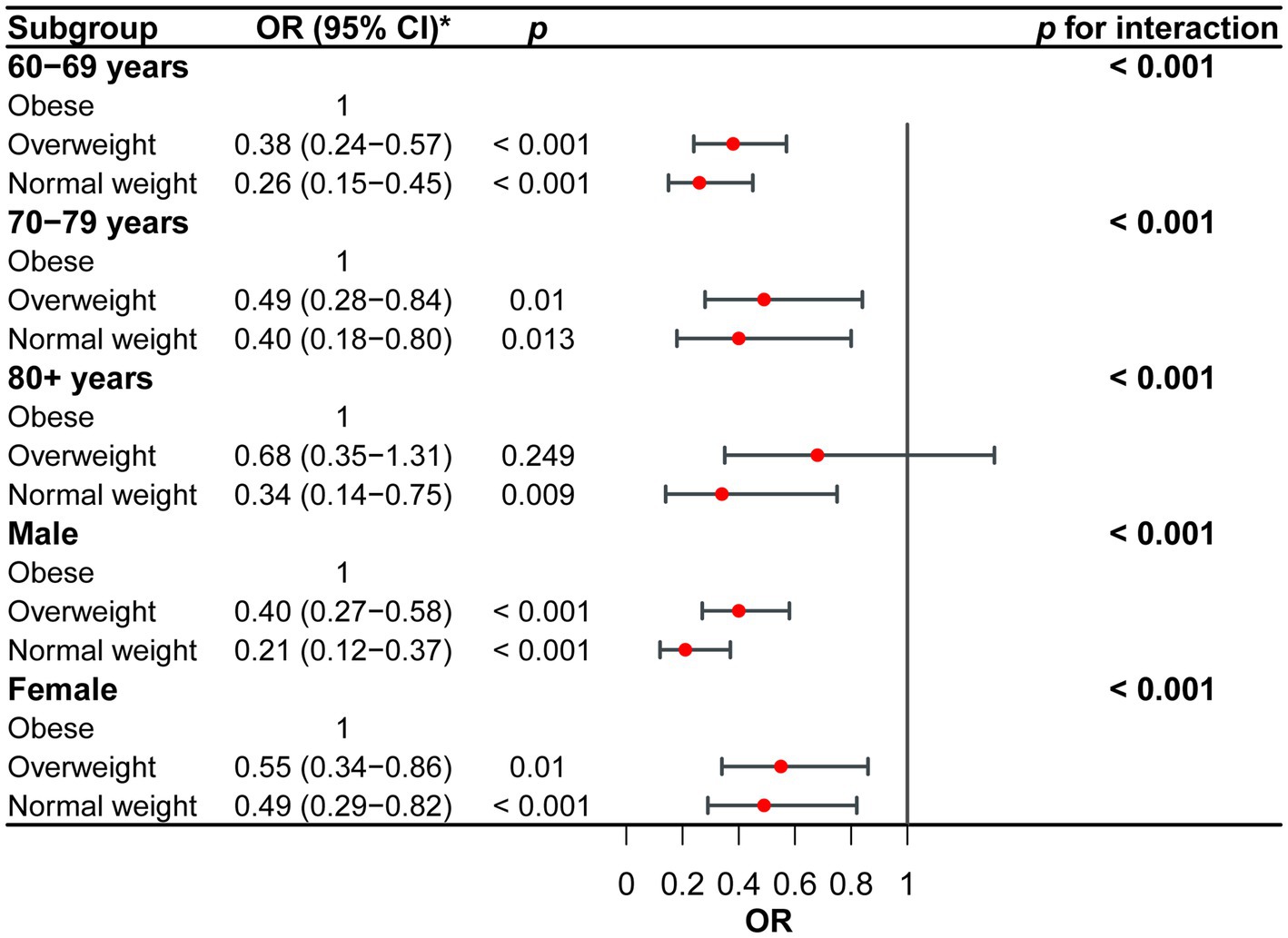
Figure 3. Subgroup analyses for the correlation between different BMI levels and incidence of sleep disorder. *Adjusted for age, sex, race, marital status, depression, PIR, alcohol intake, smoking status, recreational physical activity, diabetes, and comorbidity index.
Discussion
The primary objective of this study was to examine the correlation between obesity and sleep disturbances among older individuals. The data utilised for this investigation was obtained from the NHANES conducted throughout the period of 2005 to 2018. The results of our study offer significant contributions to the understanding of the correlation between these two crucial health issues among the aged population. This research provides essential knowledge that can inform public health strategies and guide treatment approaches.
The existing data demonstrate inconclusive findings regarding the correlation between obesity and sleep disorders. A cross-sectional population-based study conducted in the U.S. revealed a significant association between short sleep duration and all categories of abnormal body weight, including underweight, overweight, and obesity (20). However, a longitudinal observation within a large national cohort of Thai adults did not find any significant association between short sleep duration and underweight (21). Another study involving longevity Chinese individuals found no association between BMI and sleep quality (22). These inconsistencies in findings may stem from variations in ethnicity, characteristics of the study population, and the presence of other confounding factors.
The principal finding of our study reveals a statistically significant positive correlation between obesity and sleep disturbances among the senior population. The study revealed a significant association between sleep problems and obesity, indicating that individuals with sleep disorders exhibit a considerably greater incidence of obesity as compared to those without such illnesses. The aforementioned observation aligns with prior studies conducted on individuals from both younger and older age groups, highlighting the significance of obesity as a contributing factor to the onset and worsening of sleep disruptions (23–26).
The precise mechanisms that connect BMI and sleep disorders remain incompletely comprehended; however, various viable hypotheses can be posited. There exists a correlation between the buildup of visceral fat in the abdominal region and an augmented secretion of inflammatory cytokines and adipokines. This physiological response has been found to have adverse effects on sleep patterns, leading to a deterioration in the overall quality of sleep (27–29). Metabolic abnormalities, such as insulin resistance, dyslipidemia, and inflammation, are commonly observed in individuals with central obesity. These abnormalities have been found to potentially disrupt the regular sleep–wake cycle and contribute to sleep disorders (30). Furthermore, one additional plausible mechanism that may explain the correlation between obesity and sleep disturbances is the existence of obstructive sleep apnoea (OSA). OSA is a prevalent sleep disease characterised by the partial or total obstruction of the upper airway during sleep. This obstruction results in recurrent interruptions in breathing and the disruption of sleep patterns. OSA exhibits a robust correlation with obesity, particularly among older individuals. This association is attributed to age-related alterations in the upper airway, which can intensify the collapse of the airway during sleep (31, 32). Furthermore, it is worth noting that inflammation associated with obesity and changes in adipose tissue could potentially play a role in the disturbance of sleep patterns and the emergence of various sleep disorders, including insomnia and restless leg syndrome (RLS) (33–35).
The utilisation of multivariate logistic regression analysis in our study enabled us to account for any confounding variables, hence enhancing the reliability of our evaluation of the association between obesity and sleep disturbances among the older population. The findings of the study revealed that older adults who were classified as overweight or normal weight had significantly reduced likelihood of suffering sleep disorders in comparison to persons classified as obese. The results of this study indicate the presence of a dose–response correlation, wherein greater BMI levels are linked to a heightened susceptibility to sleep disruptions. In our subgroup analysis, following adjustment for confounding factors, we observed consistent findings across various age and gender cohorts. Weight management therapies targeting obesity in the senior population may offer potential benefits in terms of enhancing sleep quality and mitigating the likelihood of sleep disturbances. Our study highlights the significance of addressing weight management in the senior population as a means to improve healthy sleep patterns and general health, given that obesity is a risk factor that can be modified. Lifestyle treatments, encompassing dietary and exercise programmes, customised to address the unique requirements and constraints of older individuals, have the potential to significantly alleviate the adverse effects of obesity on the quality of sleep.
However, we have to admit that our study has several shortcomings that need to be noted and addressed in further research: (1) NHANES is a well-representative survey, and in theory these results may be generalizable to the overall US population. However, there may be selection bias in the NHANES sampling process, which may result in a sample that is not fully representative of the entire US population; (2) Due to the cross-sectional design of the study, it is not possible to establish a causal relationship between obesity and sleep disturbances. There is a need for longitudinal studies to investigate the temporal association between these variables and to evaluate the potential of weight reduction therapies in enhancing sleep outcomes among older adults. (2) While we have made efforts to account for numerous covariates to mitigate confounding bias, sleep disorders are complex conditions influenced by various genetic and environmental factors. Consequently, there may be unidentified confounders not documented in the NHANES database that could contribute to the development of sleep disorders; (3) the utilisation of self-reported sleep problems may give rise to recall bias and subsequent misclassification. Self-reported measures are inherently subjective and may be influenced by various factors such as recall bias, individual perception of symptoms, and social desirability bias. Participants may underreport or overreport symptoms, leading to inaccuracies in the data. Despite these limitations, self-reported measures remain valuable tools in epidemiological research due to their practicality and cost-effectiveness. The integration of objective sleep measures, such as polysomnography, into future research endeavours has the potential to enhance the comprehensiveness and precision of evaluating sleep disruptions among older adults.
In summary, our research presents persuasive findings that substantiate the correlation between obesity and sleep disturbances among the senior demographic. The aforementioned discovery highlights the significance of treating obesity as a potential risk factor for sleep disturbances among the older population. It also emphasises the necessity for focused interventions that focus on promoting weight control and enhancing sleep health in this vulnerable demographic. Through the use of these measures, it is possible to augment the holistic welfare and standard of living of senior adults, hence potentially mitigating the impact of sleep-related problems on public health. Additional research and longterm studies are required to substantiate these findings and to go into the underlying processes of this association in more depth.
Data availability statement
Publicly available datasets were analysed in this study. This data can be found at: https://www.cdc.gov/nchs/nhanes/index.htm.
Author contributions
WW: Formal analysis, Writing – original draft. ZC: Methodology, Resources, Software, Writing – review & editing. WZ: Writing – review & editing, Validation, Visualization. RY: Methodology, Resources, Writing – review & editing. YS: Data curation, Formal analysis, Software, Writing – review & editing. QY: Software, Methodology, Resources, Visualization, Writing – review & editing. JL: Investigation, Project administration, Writing – review & editing. JZ: Investigation, Project administration, Conceptualization, Funding acquisition, Methodology, Writing – original draft, Writing – review & editing.
Funding
The author(s) declare that financial support was received for the research, authorship, and/or publication of this article. JZ received support from the Natural science foundation project of Ningbo city, Zhejiang province (No. 2023 J134), the Zhejiang medical and health science and technology programme (No. 2019KY572 and 2022RC245), and the Zhejiang Provincial Natural Science Foundation of China (No. LGF21H150002).
Conflict of interest
The authors declare that the research was conducted in the absence of any commercial or financial relationships that could be construed as a potential conflict of interest.
Publisher’s note
All claims expressed in this article are solely those of the authors and do not necessarily represent those of their affiliated organizations, or those of the publisher, the editors and the reviewers. Any product that may be evaluated in this article, or claim that may be made by its manufacturer, is not guaranteed or endorsed by the publisher.
Footnotes
References
1. Wong, MCS, Huang, J, Wang, J, Chan, PSF, Lok, V, Chen, X, et al. Global, regional and time-trend prevalence of central obesity: a systematic review and meta-analysis of 13.2 million subjects. Eur J Epidemiol. (2020) 35:673–83. doi: 10.1007/s10654-020-00650-3
2. Ford, ND, Patel, SA, and Narayan, KMV. Obesity in low- and middle-income countries: burden, drivers, and emerging challenges. Annu Rev Public Health. (2017) 38:145–64. doi: 10.1146/annurev-publhealth-031816-044604
3. Pavlova M, K, and Latreille, V. Sleep Disorders. Am J Med. (2019) 132:292–9. doi: 10.1016/j.amjmed.2018.09.021
4. Nixon, JP, Mavanji, V, Butterick, TA, Billington, CJ, Kotz, CM, and Teske, JA. Sleep disorders, obesity, and aging: the role of orexin. Ageing Res Rev. (2015) 20:63–73. doi: 10.1016/j.arr.2014.11.001
5. Theorell-Haglöw, J, and Lindberg, E. Sleep duration and obesity in adults: what are the connections? Curr Obes Rep. (2016) 5:333–43. doi: 10.1007/s13679-016-0225-8
6. Ou, M-Y, Zhang, H, Tan, P-C, Zhou, S-B, and Li, Q-F. Adipose tissue aging: mechanisms and therapeutic implications. Cell Death Dis. (2022) 13:300. doi: 10.1038/s41419-022-04752-6
7. Mutz, J, Hoppen, TH, Fabbri, C, and Lewis, CM. Anxiety disorders and age-related changes in physiology. Br J Psychiatry. (2022) 221:528–37. doi: 10.1192/bjp.2021.189
8. Hur, S, Oh, B, Kim, H, and Kwon, O. Associations of diet quality and sleep quality with obesity. Nutrients. (2021) 13:3181. doi: 10.3390/nu13093181
9. Ning, X, Lv, J, Guo, Y, Bian, Z, Tan, Y, Pei, P, et al. Association of Sleep Duration with weight gain and general and central obesity risk in Chinese adults: a prospective study. Obesity (Silver Spring). (2020) 28:468–74. doi: 10.1002/oby.22713
10. Piché, M-E, Tchernof, A, and Després, J-P. Obesity phenotypes, diabetes, and cardiovascular diseases. Circ Res. (2020) 126:1477–500. doi: 10.1161/CIRCRESAHA.120.316101
11. Avgerinos, KI, Spyrou, N, Mantzoros, CS, and Dalamaga, M. Obesity and cancer risk: emerging biological mechanisms and perspectives. Metabolism. (2019) 92:121–35. doi: 10.1016/j.metabol.2018.11.001
12. Carneiro, G, and Zanella, MT. Obesity metabolic and hormonal disorders associated with obstructive sleep apnea and their impact on the risk of cardiovascular events. Metabolism. (2018) 84:76–84. doi: 10.1016/j.metabol.2018.03.008
13. Lin, S, Zhang, H, Gao, T, Zhong, F, Sun, Y, Cai, J, et al. The association between obesity and restless legs syndrome: a systemic review and meta-analysis of observational studies. J Affect Disord. (2018) 235:384–91. doi: 10.1016/j.jad.2018.04.042
14. Yaremchuk, K. Sleep disorders in the elderly. Clin Geriatr Med. (2018) 34:205–16. doi: 10.1016/j.cger.2018.01.008
15. Chen, T-C, Clark, J, Riddles, MK, Mohadjer, LK, and THI, F. National Health and nutrition examination survey, 2015-2018: sample design and estimation procedures. Vital Health Stat. (2020) 2:184.
16. Liu, X, Li, Y, Chai, Y, Zhang, Y, Zhang, L, and Zhang, H. Thyroid function and thyroid homeostasis parameters are associated with increased urinary albumin excretion in euthyroid individuals over 60 years old from NHANES. Front Endocrinol. (2023) 14:1285249. doi: 10.3389/fendo.2023.1285249
17. Obesity: preventing and managing the global epidemic. Report of a WHO consultation. World Health Organ Tech Rep Ser. (2000) 894:i–xii.
18. Kroenke, K, Spitzer, RL, and Williams, JB. The PHQ-9: validity of a brief depression severity measure. J Gen Intern Med. (2001) 16:606–13. doi: 10.1046/j.1525-1497.2001.016009606.x
19. Manea, L, Gilbody, S, and McMillan, D. Optimal cut-off score for diagnosing depression with the patient health questionnaire (PHQ-9): a meta-analysis. CMAJ: Canadian Med Assoc J = Journal de L'Association Medicale Canadienne. (2012) 184:E191–6. doi: 10.1503/cmaj.110829
20. Krueger, PM, and Friedman, EM. Sleep duration in the United States: a cross-sectional population-based study. Am J Epidemiol. (2009) 169:1052–63. doi: 10.1093/aje/kwp023
21. Yiengprugsawan, V, Banwell, C, Seubsman, SA, and Sleigh, AC. Short sleep and obesity in a large national cohort of Thai adults. BMJ Open. (2012) 2:e000561. doi: 10.1136/bmjopen-2011-000561
22. Yan, Z, Chang-Quan, H, Zhen-Chan, L, and Bi-Rong, D. Association between sleep quality and body mass index among Chinese nonagenarians/centenarians. Age (Dordr). (2012) 34:527–37. doi: 10.1007/s11357-011-9251-3
23. Geva, N, Pinhas-Hamiel, O, Frenkel, H, Shina, A, Derazne, E, Tzur, D, et al. Twig G: obesity and sleep disorders: a nationwide study of 1.3 million Israeli adolescents. Obes Res Clin Pract. (2020) 14:542–7. doi: 10.1016/j.orcp.2020.10.008
24. Lizończyk, I, and Jośko-Ochojska, J. Relationship between overweight, obesity and sleep disorders in adolescents from selected cities of upper Silesia. Poland Ann Agric Environ Med. (2021) 28:193–7. doi: 10.26444/aaem/118706
25. van den Berg, JF, Knvistingh Neven, A, Tulen, JHM, Hofman, A, Witteman, JCM, Miedema, HME, et al. Actigraphic sleep duration and fragmentation are related to obesity in the elderly: the Rotterdam study. Int J Obes. (2008) 32:1083–90. doi: 10.1038/ijo.2008.57
26. Yuan, Y, Heizhati, M, Wang, L, Li, M, Lin, M, Gan, L, et al. Poor sleep quality is associated with new-onset hypertension in a diverse young and middle-aged population. Sleep Med. (2021) 88:189–96. doi: 10.1016/j.sleep.2021.10.021
27. Bhatt, SP, Guleria, R, and Kabra, SK. Metabolic alterations and systemic inflammation in overweight/obese children with obstructive sleep apnea. PLoS One. (2021) 16:e0252353. doi: 10.1371/journal.pone.0252353
28. Venancio, DP, and Suchecki, D. Prolonged REM sleep restriction induces metabolic syndrome-related changes: mediation by pro-inflammatory cytokines. Brain Behav Immun. (2015) 47:109–17. doi: 10.1016/j.bbi.2014.12.002
29. Kritikou, I, Basta, M, Vgontzas, AN, Pejovic, S, Liao, D, Tsaoussoglou, M, et al. Sleep apnoea, sleepiness, inflammation and insulin resistance in middle-aged males and females. Eur Respir J. (2014) 43:145–55. doi: 10.1183/09031936.00126712
30. Duan, D, Kim, LJ, Jun, JC, and Polotsky, VY. Connecting insufficient sleep and insomnia with metabolic dysfunction. Ann N Y Acad Sci. (2023) 1519:94–117. doi: 10.1111/nyas.14926
31. Ryan, S, Crinion, SJ, and McNicholas, WT. Obesity and sleep-disordered breathing--when two 'bad guys' meet. QJM. (2014) 107:949–54. doi: 10.1093/qjmed/hcu029
32. Rissanen, M, Oksenberg, A, Töyräs, J, Myllymaa, S, and Leppänen, T. Total durations of respiratory events are modulated within REM and NREM sleep by sleeping position and obesity in OSA patients. Sleep Med. (2021) 81:394–400. doi: 10.1016/j.sleep.2021.02.020
33. Akram, U, Barclay, N, Milkins, B, Stevenson, J, and Gardani, M. Sleep-related attentional and interpretive-bias in insomnia: a systematic review and meta-analysis. Sleep Med Rev. (2023) 67:101713. doi: 10.1016/j.smrv.2022.101713
34. Scherer, JS, Combs, SA, and Brennan, F. Sleep disorders, restless legs syndrome, and uremic pruritus: diagnosis and treatment of common symptoms in Dialysis patients. American J Kidney Dis: Official J Nat Kidney Foundation. (2017) 69:117–28. doi: 10.1053/j.ajkd.2016.07.031
Keywords: body mass index, obesity, sleep disorder, NHANES, elderly
Citation: Wang W, Chen Z, Zhang W, Yuan R, Sun Y, Yao Q, Lu J and Zheng J (2024) Association between obesity and sleep disorder in the elderly: evidence from NHANES 2005–2018. Front. Nutr. 11:1401477. doi: 10.3389/fnut.2024.1401477
Edited by:
James Harper, Sam Houston State University, United StatesReviewed by:
Jing Hong, Hypertension Center of People’s Hospital of Xinjiang Uygur Autonomous Region, ChinaJIanchun Yu, Peking Union Medical College Hospital (CAMS), China
Copyright © 2024 Wang, Chen, Zhang, Yuan, Sun, Yao, Lu and Zheng. This is an open-access article distributed under the terms of the Creative Commons Attribution License (CC BY). The use, distribution or reproduction in other forums is permitted, provided the original author(s) and the copyright owner(s) are credited and that the original publication in this journal is cited, in accordance with accepted academic practice. No use, distribution or reproduction is permitted which does not comply with these terms.
*Correspondence: Jungang Zheng, YjIwMTgwNjRAemp1LmVkdS5jbg==
†These authors have contributed equally to this work