- 1Department of Gastroenterology, The First Affiliated Hospital of Zhengzhou University, Zhengzhou, China
- 2Department of Gastroenterology, The Second Affiliated Hospital of Zhengzhou University, Zhengzhou, China
- 3Department of Cardiology, The People’s Hospital of Jiawang District of Xuzhou, Xuzhou, China
Background: Intestinal health issues affect approximately 20% of the global population, yet the relationship between insulin resistance (IR) and intestinal health remains poorly understood. This study evaluated the discriminative ability of five IR surrogate indices—homeostatic model assessment for insulin resistance (HOMA-IR), triglyceride-glucose index (TyG), TyG adjusted for body mass index (TyG-BMI), triglyceride-to-high-density lipoprotein cholesterol ratio (TG/HDL-C), and estimated glucose disposal rate (eGDR)—for chronic diarrhea and constipation in adults.
Methods: Using data from the 2005–2010 National Health and Nutrition Examination Survey (NHANES), we analyzed associations between five IR surrogate indices and chronic diarrhea/constipation in adults. Key variables were selected via the Boruta algorithm and incorporated into weighted multivariate logistic regression models. Restricted cubic spline (RCS) analysis, threshold effect analysis, and receiver operating characteristic (ROC) curves were employed to assess these associations.
Results: Among 6,133 participants in this study, 7.5% had chronic diarrhea and 7.4% had chronic constipation. After adjusting for confounders, multivariate logistic regression revealed significant positive associations of HOMA-IR (OR: 1.02, 95% CI: 1.00–1.04), TyG (OR: 1.28, 95% CI: 1.05–1.55), and TyG-BMI (OR: 1.01, 95% CI: 1.00–1.01) with chronic diarrhea, while eGDR showed an inverse association (OR: 0.88, 95% CI: 0.80–0.96). No significant associations were observed between IR surrogate indices and chronic constipation. RCS and threshold effect analyses demonstrated a non-linear relationship between TG/HDL-C and chronic diarrhea: Each 1-unit increase in TG/HDL-C below the threshold of 7.33 elevated diarrhea risk by 11% (95% CI: 1.05–1.17). ROC analysis indicated that TyG-BMI (AUC: 0.656 vs. 0.644) and eGDR (AUC: 0.652 vs. 0.644) significantly improved the discriminative ability of the baseline model for chronic diarrhea, whereas HOMA-IR and TyG showed no statistically meaningful enhancements.
Conclusion: IR surrogate indices were significantly associated with chronic diarrhea but not chronic constipation, highlighting their potential as biomarkers for screening diarrhea in the general population.
1 Introduction
In recent years, there has been an increasing recognition of the importance of intestinal health, particularly regarding the prevalent issues of chronic diarrhea and constipation. Approximately 20% of adults are diagnosed with chronic diarrhea, while approximately 17% experience constipation in the general population (1, 2). These health challenges significantly impact individuals’ quality of life and contribute to both direct and indirect costs associated with treatment and reduced work productivity (3, 4). Individuals suffering from chronic diarrhea and constipation face heightened risks of metabolic and cardiovascular conditions and are also more likely to develop colorectal cancer and experience all-cause mortality (5, 6). Despite the serious implications of these disorders, timely interventions and effective management strategies are often neglected in clinical practice. Therefore, it is imperative to elevate awareness within primary care regarding the burden of these diseases and to advocate for early diagnosis, facilitating the formulation of targeted prevention and control measures.
The mechanisms behind chronic diarrhea and constipation are still not fully understood. Earlier research has revealed various factors that contribute to the development of chronic diarrhea and constipation, such as poor dietary habits (7), bacterial infections (8), intestinal dysbiosis (9, 10), and psychological issues such as anxiety and depression (9, 11). A growing amount of evidence shows that abnormal intestinal habits are closely linked to metabolic disorders. People who have diabetes (12), non-alcoholic fatty liver disease (5), metabolic syndrome (6), and obesity (13) are more vulnerable to experiencing chronic diarrhea and constipation. A shared pathological mechanism connecting these conditions is insulin resistance (IR). Lipopolysaccharides (LPSs) that originate from gut microbiota can trigger IR through the activation of Toll-like receptor 4 (TLR4) (14). In addition, substantial evidence supports a causal relationship between chronic diarrhea, constipation, and intestinal dysbiosis (9, 10). As a result, this study speculates an association between IR and the prevalence of chronic diarrhea and constipation.
The hyperinsulinemic-euglycemic clamp technique is widely recognized as the gold standard for assessing IR; however, its complex procedures and high costs significantly limit its widespread clinical application (15). Therefore, developing cost-effective and easily accessible surrogate markers for IR is of great practical significance. In recent years, several IR surrogate indices have been introduced into clinical practice, including homeostatic model assessment for insulin resistance (HOMA-IR), triglyceride-glucose index (TyG), TyG adjusted for body mass index (TyG-BMI), triglyceride-to-high-density lipoprotein cholesterol ratio (TG/HDL-C), and estimated glucose disposal rate (eGDR). These indices each have unique advantages: TyG and TyG-BMI excel in evaluating lipid metabolism-related IR, eGDR comprehensively reflects glucose disposal capacity, and TG/HDL-C integrates multiple metabolic parameters to provide a more comprehensive assessment (16–20). Nevertheless, existing research has primarily focused on the relationship between IR and chronic metabolic diseases such as diabetes, obesity, and cardiovascular diseases, while studies exploring the association between IR and intestinal health remain limited. This study was the first to systematically evaluate the correlation between these five IR surrogate indices and chronic diarrhea and constipation, aiming to identify novel biomarkers for early diagnosis and intervention of these conditions, thereby addressing a critical gap in the field of IR surrogates and intestinal health.
2 Methods
2.1 Study population
This study included 31,034 participants from the National Health and Nutrition Examination Survey (NHANES) conducted between 2005 and 2010. NHANES, approved by the Ethics Review Board of the National Center for Health Statistics, is designed to systematically investigate the nutritional and health status of U.S. citizens biennially. After providing informed consent, participants completed the questionnaires, physical examinations, and biological sample collection, with the assistance of trained technicians. Final examination reports were reviewed by staff, anonymized to protect participant privacy, and publicly released on the NHANES website. Figure 1 outlines the participant selection procedure for this research. Exclusion criteria included (1) individuals <20 years old (n = 13,722); (2) those lacking essential data for five IR surrogate indices calculations (n = 10,006); (3) individuals with missing Bristol Stool Form Scale (BSFS) data (n = 555); and (4) individuals with missing weight data or WTSAF2YR ≤ 0 (n = 618).
2.2 IR surrogate indices
This study incorporated five IR surrogate indices; the detailed calculation formulas for these indices are presented in Table 1 (16–20). Published studies have recommended stratifying eGDR using specific cutoff values (<4, 4–6, 6–8, and ≥8 mg/kg/min) (21, 22) as these categories have been shown to reflect significant differences in mortality rates among individuals with diabetes. Conversely, due to the lack of established diagnostic thresholds for the remaining four IR surrogate indices, these parameters were categorized via quartile-based stratification.
2.3 Assessment of chronic diarrhea and chronic constipation
In this study, chronic diarrhea and constipation were assessed using BSFS (Table 2) (23). Participants were classified as having chronic diarrhea if their usual or most common stool type was categorized as Type 6–7, or as having chronic constipation if their stool type was categorized as Type 1–2. All other stool types were considered normal.
2.4 Covariates
The covariates in this study included general participant information such as age, gender, race/ethnicity, education level, marital status (married or living with a partner, and unmarried or separated), and the poverty-to-income ratio (PIR) (<1.3, 1.3–3.5, ≥3.5) (24). BMI was calculated as weight in kilograms divided by height in meters squared (kg/m2) (25). Laboratory measurements included serum fasting blood glucose (FBG), which was assessed using the Roche/Hitachi Cobas C 501 Chemistry Analyzer (Roche Diagnostics, Indianapolis, IN, United States) before 2015, employing a hexokinase-mediated enzymatic reaction. In 2015, the laboratory instrumentation was updated, with the Roche C501 replaced by the Roche C311 Chemistry Analyzer (Roche Diagnostics, Indianapolis, IN, United States). A correction equation was applied to adjust glucose measurements between the two instruments, following official guidelines. Glycated hemoglobin (HbA1c) was measured using the Tosoh G8 Glycohemoglobin Analyzer (HLC-723G8), based on high-performance liquid chromatography (HPLC). Serum insulin concentrations were determined using the Tosoh AIA-900 Automated Immunoassay Analyzer (Tosoh Bioscience, Tokyo, Japan), employing an immunoenzymometric assay (IEMA). Liver function tests were conducted using the DxC800 system, a fully automated clinical chemistry analyzer developed by Beckman Coulter. High-density lipoprotein cholesterol (HDL-C), low-density lipoprotein cholesterol (LDL-C), triglycerides (TGs), and total cholesterol (TC) were measured using the Roche Cobas 6,000 Chemistry Analyzer (Roche Diagnostics, Indianapolis, IN, United States). Behavioral habits and comorbidities were assessed as follows: Smoking behavior was classified according to participants’ lifetime smoking patterns: non-smoker (fewer than 100 cigarettes ever smoked), former smoker (over 100 cigarettes smoked but abstinent for at least 1 year), and current smoker (over 100 cigarettes smoked and actively smoking) (26). Alcohol consumption was defined as having at least 12 drinks of any type of alcoholic beverage in the past year, where one drink was equivalent to 12 oz. of beer, 5 oz. of wine, or 1.5 oz. of liquor (24). Physical activity (PA) was categorized into four levels based on metabolic equivalent of task (MET) metrics: (1) no PA (0 MET·min/week); (2) low PA (<600 MET·min/week); (3) moderate PA (600–1,200 MET·min/week); and (4) high PA (>1,200 MET·min/week) (27).
Participants’ mental health status was determined using the Patient Health Questionnaire-9 (PHQ-9). PHQ-9 score ≥10 was considered indicative of depressive symptoms, while scores below 10 were classified as normal (28). Diabetes was defined as meeting one or more of the following criteria: (1) a previous diagnosis of diabetes by a healthcare professional or current use of insulin or other glucose-lowering medications; (2) FBG ≥ 7 mmol/L; and/or (3) HbA1c ≥ 6.5% (29). The definition of hypertension encompassed fulfillment of either diagnostic parameter: (1) self-reported physician-diagnosed hypertension or active pharmacological management with antihypertensive agents; (2) and sustained blood pressure elevation evidenced by triplicate measurements demonstrating systolic pressure ≥140 mmHg and/or diastolic values ≥90 mmHg, calculated through averaged readings (30).
2.5 Statistical analysis
Considering the complex, multi-stage sampling design of NHANES, sample weights were incorporated into the subsequent statistical analyses. Based on NHANES recommendations, the fasting subsample weight (WTSAF2YR) divided by the number of cycles was applied as the sample weight for this study (24). During data processing, participants with missing key variables were excluded, and the specific selection process is illustrated in Figure 1. For participants missing other covariates, multiple imputation using the random forest method was performed. The missing covariates and their proportions are detailed in Supplementary Table 1. Continuous variables were presented as mean ± standard deviation (SD), while categorical variables were expressed as proportions. The analysis of one-way ANOVA (for continuous variables) or chi-square tests (for categorical variables) was used to compare clinical data of participants grouped by intestinal health status. The Boruta algorithm, a supervised machine learning method based on random forests, was employed to identify covariates genuinely associated with intestinal health for inclusion in subsequent multivariate logistic regression models. Notably, to avoid the impact of multicollinearity, test indicators used to calculate independent variables were not individually included in the model. Furthermore, multicollinearity was assessed, and covariates with a variance inflation factor (VIF) > 5 were sequentially excluded to eliminate multicollinearity with independent variables. Multivariate logistic regression analysis was used to examine the associations between the five IR surrogate indices (analyzed as both continuous and categorical variables) and chronic diarrhea or chronic constipation. Model 1 was unadjusted for any variables. Model 2 was adjusted for age, gender, race/ethnicity, education level, marital status, and PIR. Model 3 was further adjusted for LDL-C, smoking status, alcohol consumption, diabetes, hypertension, mental health status, and PA based on Model 2.
Four-knot restricted cubic spline (RCS) plots were used to capture the dose–response relationships between the five IR surrogate indices and chronic diarrhea or constipation. If non-linear relationships were observed, threshold effect analysis was further conducted to examine the associations on either side of the inflection point. Receiver operating characteristic (ROC) curves were used to evaluate whether HOMA-IR, TyG, TyG-BMI, TG/HDL, and eGDR could improve the predictive ability of the baseline risk model for chronic diarrhea or chronic constipation. This study utilizes version R.4.3.0 for data analysis, with a two-tailed p-value less than 0.05 considered statistically significant.
3 Results
3.1 Clinical characteristics
A total of 6,133 participants were enrolled in this study, of whom 7.5% (n = 463) had chronic diarrhea and 7.4% (n = 450) had chronic constipation. The clinical characteristics of the participants, stratified by intestinal health status, are summarized in Table 3. The mean age of the participants was 49.37 ± 17.82 years, with 49.2% being male. Compared to the normal group, the chronic diarrhea group showed a lower proportion of male participants (44.1% vs. 51.4%), Mexican Americans (23.3% vs. 18.1%), individuals with less than a high school education (41.0% vs. 25.7%), lower income levels (37.4% vs. 28.2%), alcohol consumption (24.8% vs. 15.7%), and physical inactivity (36.9% vs. 29.3%). In addition, the chronic diarrhea group had a higher prevalence of hypertension (57.5% vs. 45.9%), diabetes (24.8% vs. 15.7%), and depressive symptoms (16.6% vs. 7%). Notably, the chronic diarrhea group demonstrated significantly elevated levels of HOMA-IR (4.57 ± 5.19 vs. 3.52 ± 4.33), TyG (8.81 ± 0.68 vs. 8.63 ± 0.67), TyG-BMI (269.82 ± 70.26 vs. 250.50 ± 64.06), and TG/HDL (3.25 ± 2.90 vs. 2.99 ± 5.14), alongside reduced eGDR levels (6.71 ± 2.77 vs. 7.55 ± 2.63), compared to the normal group (p < 0.001).
Similarly, the chronic constipation group exhibited a lower prevalence of male participants (28.2% vs. 51.4%), Mexican Americans (21.1% vs. 18.1%), individuals with less than a high school education (31.3% vs. 25.7%), lower income levels (36.4% vs. 28.2%), physical inactivity (35.1% vs. 29.3%), and depressive symptoms (11.3% vs. 7%). The chronic constipation group exhibited lower levels of HOMA-IR (3.38 ± 3.93 vs. 3.52 ± 4.33), TyG-BMI (245.24 ± 66.18 vs. 250.50 ± 64.06), and TG/HDL (2.71 ± 2.79 vs. 2.99 ± 5.14), while eGDR (6.71 ± 2.77 vs. 7.55 ± 2.63) levels were higher (p < 0.001).
3.2 Feature selection
The feature selection results based on the Boruta algorithm are presented in Figure 2. After 500 iterations, the top 10 variables most closely associated with chronic diarrhea (ranked by z-score, excluding IR surrogate indices) were identified as age, hypertension, depressive symptoms, race/ethnicity, education level, diabetes, LDL-C, gender, PIR, and smoking status. Similarly, the top 10 parameters most strongly associated with chronic constipation included age, gender, alcohol consumption, hypertension, diabetes, smoking status, marital status, LDL-C, PA, depression and PIR. Based on the feature selection results from the Boruta algorithm and previous research findings, the covariates included in the final logistic regression models were as follows: Model 1 was unadjusted; Model 2 adjusted for age, gender, race/ethnicity, education level, marital status, and PIR; Model 3 further adjusted for LDL-C, smoking status, alcohol consumption, diabetes, hypertension, depressive symptoms, and PA based on Model 2.
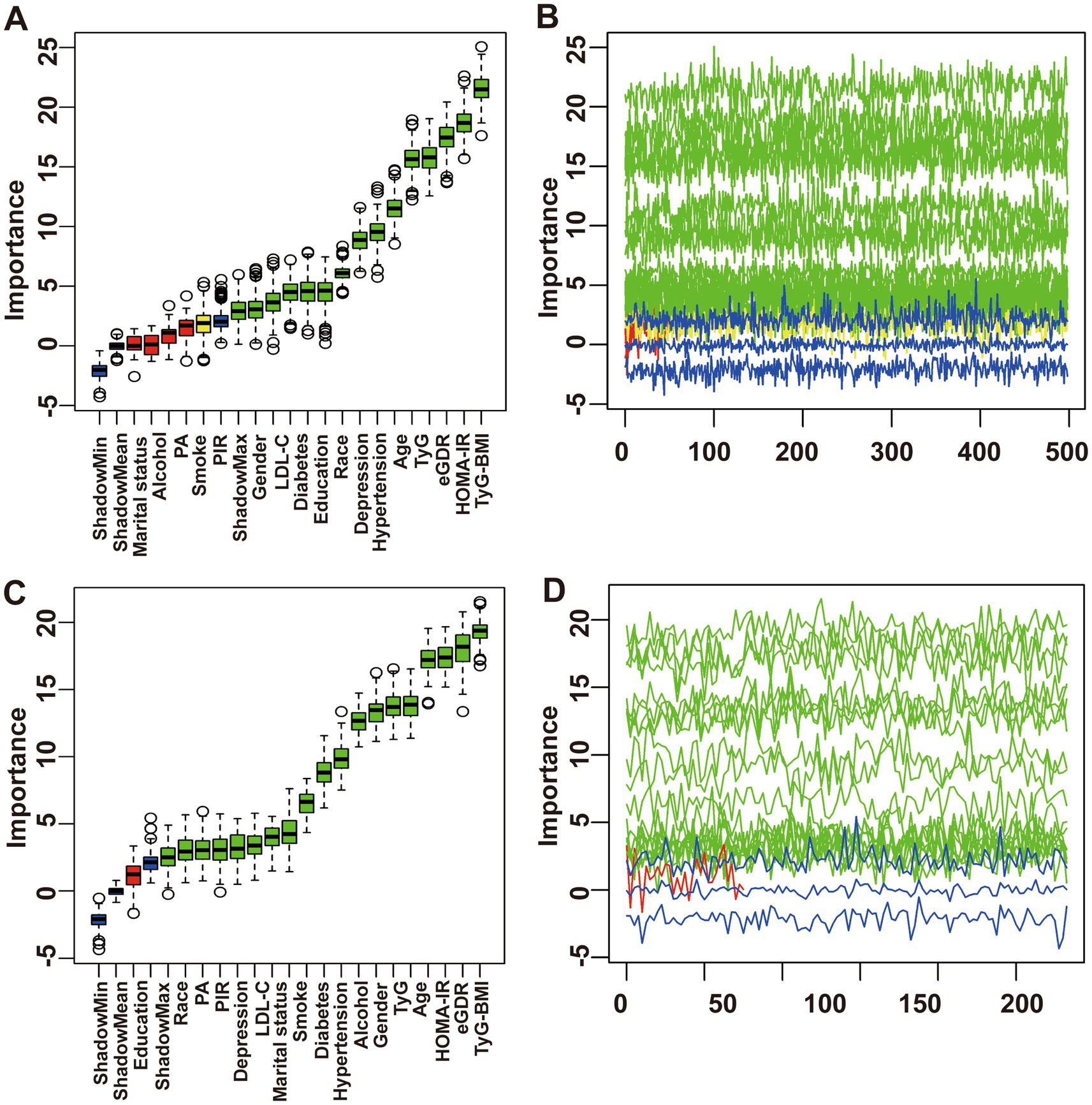
Figure 2. Feature selection process for chronic diarrhea based on Boruta’s algorithm (A) and the value evolution of Z-score in the screening process (B). Feature selection process for chronic constipation based on Boruta’s algorithm (C) and the value evolution of Z-score in the screening process (D).
3.3 Relationship between the IR surrogate indices and chronic diarrhea or chronic constipation
Three logistic regression models were constructed to examine the independent associations between five IR surrogate indices and the risks of chronic diarrhea and constipation (Tables 4, 5). When analyzed as continuous variables, each 1-unit increase in HOMA-IR, TyG, and TyG-BMI was associated with a 2% (95% CI: 1.00–1.04), 28% (95% CI: 1.05–1.55), and 1% (95% CI: 1.00–1.01) increased risk of chronic diarrhea, respectively, after full adjustment for covariates. Conversely, each 1-unit increase in eGDR was associated with a 12% reduction in the risk of chronic diarrhea (95% CI: 0.80–0.96). Similarly, when analyzed as categorical variables, participants in the highest quartile (Q4) of HOMA-IR, TyG, and TyG-BMI exhibited a 50% (95% CI: 1.04–2.17), 65% (95% CI: 1.13–2.42), and 90% (95% CI: 1.28–2.81) increased risk of chronic diarrhea, respectively, compared to those in the lowest quartile (Q1). In contrast, participants in the highest quartile (Q4) of eGDR showed a 44% reduction in the risk of chronic diarrhea (95% CI: 1.13–2.42). However, no significant associations were observed between HOMA-IR, TyG, TyG-BMI, TG/HDL, and eGDR and the risk of chronic constipation.
3.4 The detection of non-linear relationships
The RCS results are illustrated in Figure 3. Except for TG/HDL, which exhibited a non-linear association with chronic diarrhea (P for non-linear = 0.001), TyG, eGDR, HOMA-IR, and TyG-BMI all demonstrated linear relationships with chronic diarrhea (P for non-linear > 0.05). Further threshold effect analysis revealed that each 1-unit increase in TG/HDL-C below the threshold of 7.33 elevated diarrhea risk by 11% (95% CI: 1.05–1.17) (Table 6).
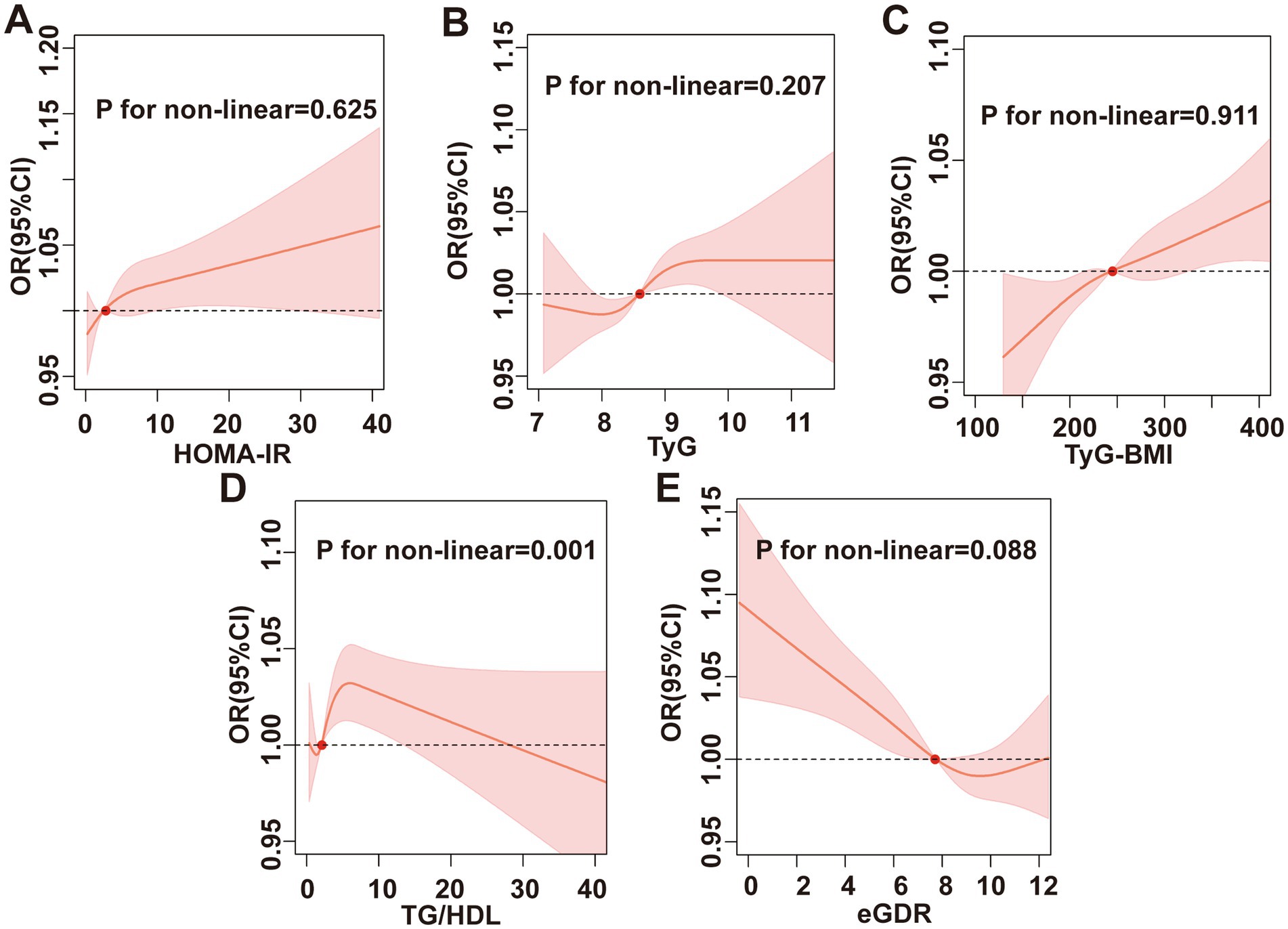
Figure 3. RCS analysis. Association between (A) HOMA-IR, (B) TyG index, (C) TyG-BMI, (D) TG/HDL, (E) eGDR, and chronic diarrhea.
3.5 ROC curve analysis
This study incorporated covariates from logistic Model 3 to establish a baseline risk model, aiming to evaluate the improvement in the discriminative ability of the model for diarrhea by adding IR surrogate indices. The results are illustrated in Figure 4. Although the inclusion of five IR surrogate indices enhanced the discriminative ability of the model for diarrhea, only the addition of TyG-BMI (AUC: 0.656 vs. 0.644, p < 0.01) and eGDR (AUC: 0.652 vs. 0.644, p = 0.03) demonstrated statistically significant improvements.
4 Discussion
This study comprehensively investigated the associations between five IR surrogate indices and chronic diarrhea and chronic constipation among U.S. adults. The results revealed that HOMA-IR, TyG, TyG-BMI, TG/HDL, and eGDR were significantly associated with chronic diarrhea in adults, and these associations were independent of diabetes, depressive symptoms, and PA levels. Furthermore, TyG-BMI and eGDR significantly improved the discriminative ability of the baseline model for chronic diarrhea. However, no evidence was found to support an association between these five IR surrogate indices and chronic constipation. These findings highlight the potential of IR surrogate indices as early predictive markers for chronic diarrhea, providing a novel theoretical foundation for the prevention and treatment of this condition.
IR is defined by diminished cellular sensitivity to insulin, leading to impaired glucose uptake, dysregulated hepatic glucose production, and altered lipid metabolism (31). Research indicates that IR surrogate indices hold significant clinical value in primary healthcare and have the potential for widespread application (32). However, studies investigating the relationship between IR surrogate indices and intestinal health remain limited. Existing evidence suggests that intestinal health is closely associated with factors such as gut microbiota, dietary habits, infections, and psychological states (33). Specifically, gut microbiota dysbiosis can compromise the mucosal immune barrier, leading to inflammatory responses, oxidative stress, and IR-related pathological processes (34). Animal studies further demonstrate that probiotics and Lactobacillus plantarum can modulate gut microbiota composition, improve glucose and lipid metabolism, and enhance insulin sensitivity (35). Clinical research has also shown that individuals with metabolic disorders, including diabetes (36), non-alcoholic fatty liver disease (37), metabolic syndrome (6), and obesity (13), are more susceptible to intestinal dysfunction. These findings suggest that IR may play a significant role in the development of abnormal intestinal health. Notably, a recent study reported a positive correlation between the TyG index and chronic diarrhea but found no significant association with chronic constipation (38). Building on this, our study further explored the relationship between multiple IR surrogate indices and intestinal health. The results revealed that several IR surrogate indices were significantly associated with chronic diarrhea but not with chronic constipation, consistent with previous findings. Currently, the relationship between IR and chronic constipation remains controversial, with no conclusive evidence supporting a direct link. This may be due to the study population not reaching the critical threshold for related risks. Future prospective studies are needed to clarify the causal relationship between IR and chronic constipation.
This study utilized RCS to elucidate the complex associations between IR surrogate indices and chronic diarrhea risk. Although multivariate logistic regression showed no significant correlation between TG/HDL ratio and chronic diarrhea, RCS analysis revealed a non-linear association, and threshold effect analysis further quantified this relationship: Each 1-unit increase in TG/HDL below 7.33 elevated chronic diarrhea risk by 11%. HOMA-IR, TyG, TyG-BMI, and eGDR exhibited linear dose–response relationships. Integrating IR surrogate indices into primary care practices can improve the accuracy of diagnoses for chronic diarrhea, thus promoting the creation of more tailored and effective management approaches. Specifically, populations showing heightened levels of IR surrogate indices can be selected for focused strategies to enhance intestinal health, which may involve more stringent monitoring, alterations in lifestyle, and strong pharmacological treatments to reduce their risk of chronic diarrhea. The intricacy of dietary questionnaires, along with the financial implications and discomfort linked to testing intestinal flora, presents considerable obstacles to their widespread use in primary care settings. In contrast, IR surrogate indices are easily accessible via standard blood tests and physical assessments, rendering them especially advantageous for primary care contexts.
This study demonstrated that only TyG-BMI and eGDR improved the discriminative ability of the baseline risk model for chronic diarrhea. Compared to HOMA-IR and TyG, the TyG-BMI and eGDR also incorporate BMI, effectively capturing the abnormal state of visceral fat accumulation in the body. Furthermore, individuals with diabetes and obesity are at a higher risk of experiencing chronic diarrhea (39, 40). Notably, pro-inflammatory cytokines released by visceral adipose tissue have been shown to impair intestinal barrier function and exacerbate chronic diarrhea (41, 42). Meanwhile, eGDR dynamically assesses insulin sensitivity and peripheral glucose disposal efficiency, offering a more comprehensive reflection of metabolic disturbances on intestinal energy metabolism and immune microenvironment (43). Although multivariate logistic regression revealed significant correlations between IR surrogate indices and chronic diarrhea, the discriminative capability of the ROC model was still limited, as indicated by an AUC below 0.75. Notably, previous studies on chronic diarrhea have also reported suboptimal AUC values. For instance, Yinda et al. found that the body roundness index (BRI) achieved an AUC of 0.606 for discriminating chronic diarrhea, outperforming both BMI (AUC: 0.569) and waist circumference (AUC: 0.572) (44). Due to data constraints, the current model did not incorporate gut microbiota-derived metabolites and dietary profiles. Future prospective studies should develop multidimensional predictive models by integrating metabolomic, microbiomic, and immunomic biomarkers to systematically unravel the IR-gut microenvironment interaction network, thereby optimizing risk stratification efficacy.
The precise pathological mechanisms underlying the relationship between IR surrogate indices and intestinal health remain incompletely understood but likely involve multiple interconnected pathways. First, IR-driven dysregulation of glucose and lipid metabolism compromises energy supply to intestinal smooth muscle, impairing its contractile function. Elevated blood glucose and insulin levels alter the excitability of intestinal nerves and musculature, resulting in motility disturbances that may present as constipation or diarrhea (45, 46). Second, systemic low-grade inflammation associated with IR elevates pro-inflammatory cytokines, which degrade intestinal barrier integrity and disrupt enteric nervous system signaling, further exacerbating motility dysfunction (47, 48). Third, IR is closely linked to gut microbiota dysbiosis. Such dysbiosis reduces short-chain fatty acid production, weakens the intestinal barrier, and facilitates endotoxin translocation, aggravating IR and intestinal impairment (49, 50). Fourth, IR may dysregulate gut–brain axis communication, perturbing the secretion of neurotransmitters (serotonin) and hormones (Glucagon-like peptide-1), which modulates intestinal motility and secretory activity (50–52). Finally, IR-related oxidative stress damages intestinal epithelial cells and neurons, impairing motility and barrier function, possibly contributing to constipation or diarrhea (53, 54). These interwoven mechanisms form a complex pathophysiological network that collectively drives chronic diarrhea or constipation.
To the best of our understanding, this research represents the initial attempt to analyze the relationships among IR surrogate indices and intestinal health. The robust quality control protocols and sophisticated sampling design implemented by NHANES facilitated the evaluation of correlations across various adult populations within the United States. In addition, the utilization of multivariate weighted logistic regression along with RCS analysis markedly improved the strength and dependability of our results. Notably, this investigation considered several confounding factors, such as socioeconomic status, social aspects, depression, PA levels, diabetes, and BMI, indicating that the findings of our study can be applied broadly.
While the results of this research provide novel insights into the early monitoring of chronic diarrhea and chronic constipation, several limitations warrant consideration. First, the cross-sectional design of the NHANES dataset restricts the ability to establish causal relationships between IR surrogate indices and intestinal health. Future studies should adopt a prospective design to validate these causal links. Second, the temporal scope of the data is confined to NHANES surveys conducted from 2005 to 2010, which limits external validation. While this study employs rigorous analytical methods, excluding residual confounding factors remains challenging. For instance, the gut microbiota, diet composition components, and medication usage may also influence the risk of diarrhea and constipation. In addition, the study population predominantly originates from the United States, which may restrict the external validity of the findings. Variations in dietary habits and lifestyles across different countries and regions could impact the applicability of the results to other populations. Therefore, further verification of the research findings in diverse geographical and cultural contexts is necessary to ascertain their universal applicability. Finally, due to the constraints of the NHANES dataset, more detailed clinical information required to apply the Rome IV criteria is not available; our assessment of intestinal health status relies solely on BSFS. Identifying chronic diarrhea and constipation through self-reported questionnaires may result in the potential misclassification. The limited scope of the intestinal health questionnaire hinders the collection of comprehensive data regarding various aspects of intestinal health, such as stool frequency and consistency, biochemical and/or microbial status, and duration and medication history for diarrhea. We agree that future research should incorporate both stool form and duration of symptoms to more accurately classify diarrhea subtypes.
5 Conclusion
This study identified significant associations between five IR surrogate indices and an elevated risk of chronic diarrhea. These findings suggest that IR surrogate markers hold promise as cost-effective, simple, and accessible early predictors for chronic diarrhea in high-risk populations.
Data availability statement
The original contributions presented in the study are included in the article/Supplementary material, further inquiries can be directed to the corresponding author.
Ethics statement
This study was conducted in accordance with the principles of the Declaration of Helsinki. The National Center for Health Statistics, alongside the Ethics Review Board, sanctioned the protocol for NHANES, with mandatory written informed consent documentation secured from all study enrollees prior to data collection.
Author contributions
DZ: Conceptualization, Data curation, Formal analysis, Investigation, Methodology, Resources, Software, Supervision, Validation, Visualization, Writing – original draft, Writing – review & editing. MZ: Conceptualization, Data curation, Formal analysis, Investigation, Methodology, Software, Validation, Writing – original draft. BG: Conceptualization, Data curation, Formal analysis, Investigation, Software, Writing – original draft. HL: Data curation, Formal analysis, Investigation, Methodology, Software, Writing – original draft.
Funding
The author(s) declare that no financial support was received for the research and/or publication of this article.
Conflict of interest
The authors declare that the research was conducted in the absence of any commercial or financial relationships that could be construed as a potential conflict of interest.
Generative AI statement
The author(s) declare that no Gen AI was used in the creation of this manuscript.
Publisher’s note
All claims expressed in this article are solely those of the authors and do not necessarily represent those of their affiliated organizations, or those of the publisher, the editors and the reviewers. Any product that may be evaluated in this article, or claim that may be made by its manufacturer, is not guaranteed or endorsed by the publisher.
Supplementary material
The Supplementary material for this article can be found online at: https://www.frontiersin.org/articles/10.3389/fnut.2025.1602922/full#supplementary-material
References
1. Zhao, Y, Guo, X, Zhang, Z, Ma, X, Wang, R, Yan, X, et al. Epidemiology of functional diarrhea and comparison with diarrhea-predominant irritable bowel syndrome: a population-based survey in China. PLoS One. (2012) 7:e43749. doi: 10.1371/journal.pone.0043749
2. Choung, R, Locke, G, Schleck, C, Zinsmeister, A, and Talley, N. Cumulative incidence of chronic constipation: a population-based study 1988-2003. Aliment Pharmacol Ther. (2007) 26:1521–8. doi: 10.1111/j.1365-2036.2007.03540.x
3. Gupta, A, Nshuti, L, Grewal, U, Sedhom, R, Check, D, Parsons, H, et al. Financial burden of drugs prescribed for Cancer-associated symptoms. JCO Oncol Pract. (2022) 18:140–7. doi: 10.1200/OP.21.00466
4. Bielefeldt, K. Cost of constipation: a new LookWith a focus on new medications. J Clin Gastroenterol. (2022) 56:249–56. doi: 10.1097/MCG.0000000000001514
5. Shin, A, Xu, H, and Imperiale, T. Associations of chronic diarrhoea with non-alcoholic fatty liver disease and obesity-related disorders among US adults. BMJ Open Gastroenterol. (2019) 6:e322:e000322. doi: 10.1136/bmjgast-2019-000322
6. Peng, Y, Liu, F, Qiao, Y, Wang, P, Ma, B, Li, L, et al. Association of abnormal bowel health with major chronic diseases and risk of mortality. Ann Epidemiol. (2022) 75:39–46. doi: 10.1016/j.annepidem.2022.09.002
7. Losno, E, Sieferle, K, Perez-Cueto, F, and Ritz, C. Vegan diet and the gut microbiota composition in healthy adults. Nutrients. (2021) 13:2402. doi: 10.3390/nu13072402
8. Ravel, A, Mircher, C, Rebillat, A, Cieuta-Walti, C, and Megarbane, A. Feeding problems and gastrointestinal diseases in down syndrome. Arch Pediatr. (2020) 27:53–60. doi: 10.1016/j.arcped.2019.11.008
9. Pilla, R, and Suchodolski, J. The role of the canine gut microbiome and metabolome in health and gastrointestinal disease. Front Vet Sci. (2019) 6:498. doi: 10.3389/fvets.2019.00498
10. Feng, C, Gao, G, Wu, K, and Weng, X. Causal relationship between gut microbiota and constipation: a bidirectional Mendelian randomization study. Front Microbiol. (2024) 15:1438778. doi: 10.3389/fmicb.2024.1438778
11. Singh, P, Mitsuhashi, S, Ballou, S, Rangan, V, Sommers, T, Cheng, V, et al. Demographic and dietary associations of chronic diarrhea in a representative sample of adults in the United States. Am J Gastroenterol. (2018) 113:593–600. doi: 10.1038/ajg.2018.24
12. Sommers, T, Mitsuhashi, S, Singh, P, Hirsch, W, Katon, J, Ballou, S, et al. Prevalence of chronic constipation and chronic diarrhea in diabetic individuals in the United States. Am J Gastroenterol. (2019) 114:135–42. doi: 10.1038/s41395-018-0418-8
13. Han, K, Wang, X, Niu, X, Li, T, and Linghu, E. Prevalence and associated factors of chronic diarrhea among adults with obesity in the United States: evidence from the National Health and nutrition examination survey 2005 to 2010. Obes Res Clin Pract. (2024) 18:328–35. doi: 10.1016/j.orcp.2024.11.002
14. He, F, and Li, Y. Role of gut microbiota in the development of insulin resistance and the mechanism underlying polycystic ovary syndrome: a review. J Ovarian Res. (2020) 13:73. doi: 10.1186/s13048-020-00670-3
15. Minh, H, Tien, H, Sinh, C, Thang, D, Chen, C, Tay, J, et al. Assessment of preferred methods to measure insulin resistance in Asian patients with hypertension. J Clin Hypertens. (2021) 23:529–37. doi: 10.1111/jch.14155
16. Liang, X, Lai, K, Li, X, Ren, D, Gui, S, Xing, Z, et al. Association between estimated glucose disposal rate and future cardiovascular disease risk across glucose metabolism status: a prospective cohort study. Diabetol Metab Syndr. (2025) 17:131. doi: 10.1186/s13098-025-01697-6
17. Guerrero-Romero, F, Simental-Mendia, L, Gonzalez-Ortiz, M, Martinez-Abundis, E, Ramos-Zavala, M, Hernandez-Gonzalez, S, et al. The product of triglycerides and glucose, a simple measure of insulin sensitivity. Comparison with the euglycemic-hyperinsulinemic clamp. J Clin Endocrinol Metab. (2010) 95:3347–51. doi: 10.1210/jc.2010-0288
18. Simental-Mendia, L, Rodriguez-Moran, M, and Guerrero-Romero, F. The product of fasting glucose and triglycerides as surrogate for identifying insulin resistance in apparently healthy subjects. Metab Syndr Relat Disord. (2008) 6:299–304. doi: 10.1089/met.2008.0034
19. Er, L, Wu, S, Chou, H, Hsu, LA, Teng, M, Sun, YC, et al. Triglyceride glucose-body mass index is a simple and clinically useful surrogate marker for insulin resistance in nondiabetic individuals. PLoS One. (2016) 11:e149731:e0149731. doi: 10.1371/journal.pone.0149731
20. Oliveri, A, Rebernick, R, Kuppa, A, Pant, A, Chen, Y, Du, X, et al. Comprehensive genetic study of the insulin resistance marker TG: HDL-C in the UK biobank. Nat Genet. (2024) 56:212–21. doi: 10.1038/s41588-023-01625-2
21. Zabala, A, Darsalia, V, Lind, M, Svensson, A, Franzen, S, Eliasson, B, et al. Estimated glucose disposal rate and risk of stroke and mortality in type 2 diabetes: a nationwide cohort study. Cardiovasc Diabetol. (2021) 20:202. doi: 10.1186/s12933-021-01394-4
22. Nystrom, T, Holzmann, M, Eliasson, B, Svensson, A, and Sartipy, U. Estimated glucose disposal rate predicts mortality in adults with type 1 diabetes. Diabetes Obes Metab. (2018) 20:556–63. doi: 10.1111/dom.13110
23. Ballou, S, Katon, J, Singh, P, Rangan, V, Lee, H, McMahon, C, et al. Chronic diarrhea and constipation are more common in depressed individuals. Clin Gastroenterol Hepatol. (2019) 17:2696–703. doi: 10.1016/j.cgh.2019.03.046
24. Johnson, C, Paulose-Ram, R, Ogden, C, Carroll, M, Kruszon-Moran, D, Dohrmann, S, et al. National health and nutrition examination survey: analytic guidelines, 1999-2010. Vital Health Stat. (2013) 2:1–24.
25. Zorn, S, de Groot, C, Brandt-Heunemann, S, von Schnurbein, J, Abawi, O, Bounds, R, et al. Early childhood height, weight, and bmi development in children with monogenic obesity: a european multicentre, retrospective, observational study. Lancet Child Adolesc Health. (2025) 9:297–305. doi: 10.1016/S2352-4642(25)00065-3
26. He, H, Xie, Y, Chen, Q, Li, Y, Li, X, Mu, Y, et al. The additive effect of the triglyceride-glucose index and estimated glucose disposal rate on long-term mortality among individuals with and without diabetes: a population-based study. Cardiovasc Diabetol. (2024) 23:307. doi: 10.1186/s12933-024-02396-8
27. Piercy, K, Troiano, R, Ballard, R, Carlson, S, Fulton, J, Galuska, D, et al. The physical activity guidelines for Americans. JAMA. (2018) 320:2020–8. doi: 10.1001/jama.2018.14854
28. Kroenke, K, Spitzer, R, and Williams, J. The PHQ-9: validity of a brief depression severity measure. J Gen Intern Med. (2001) 16:606–13. doi: 10.1046/j.1525-1497.2001.016009606.x
29. Qiu, Z, Geng, T, Wan, Z, Lu, Q, Guo, J, Liu, L, et al. Serum selenium concentrations and risk of all-cause and heart disease mortality among individuals with type 2 diabetes. Am J Clin Nutr. (2022) 115:53–60. doi: 10.1093/ajcn/nqab241
30. Duan, M, Zhao, X, Li, S, Miao, G, Bai, L, Zhang, Q, et al. Metabolic score for insulin resistance (METS-IR) predicts all-cause and cardiovascular mortality in the general population: evidence from NHANES 2001-2018. Cardiovasc Diabetol. (2024) 23:243. doi: 10.1186/s12933-024-02334-8
31. Sun, R, Wang, J, Li, M, Li, J, Pan, Y, Liu, B, et al. Association of insulin resistance with cardiovascular disease and all-cause mortality in type 1 diabetes: systematic review and meta-analysis. Diabetes Care. (2024) 47:2266–74. doi: 10.2337/dc24-0475
32. Tahapary, D, Pratisthita, L, Fitri, N, Marcella, C, Wafa, S, Kurniawan, F, et al. Challenges in the diagnosis of insulin resistance: focusing on the role of HOMA-IR and Tryglyceride/glucose index. Diabetes Metab Syndr. (2022) 16:102581. doi: 10.1016/j.dsx.2022.102581
33. Schiller, L, Pardi, D, Spiller, R, Semrad, C, Surawicz, C, Giannella, R, et al. Gastro 2013 APDW/WCOG Shanghai working party report: chronic diarrhea: definition, classification, diagnosis. J J Gastroenterol Hepatol. (2014) 29:6–25. doi: 10.1111/jgh.12392
34. Abildinova, G, Benberin, V, Vochshenkova, T, Afshar, A, Mussin, N, Kaliyev, A, et al. Global trends and collaborative networks in gut microbiota-insulin resistance research: a comprehensive bibliometric analysis (2000-2024). Front Med (Lausanne). (2024) 11:1452227. doi: 10.3389/fmed.2024.1452227
35. Liu, T, Chen, G, Lin, C, Tsai, T, and Cheng, M. Lactobacillus plantarum twk10 relieves loperamide-induced constipation in rats fed a high-fat diet via modulating enteric neurotransmitters, short-chain fatty acids and gut microbiota. Food Funct. (2025) 16:181–94. doi: 10.1039/d4fo02270j
36. Koloski, N, Jones, M, Walker, M, Horowitz, M, Holtmann, G, and Talley, N. Diabetes mellitus is an independent risk factor for a greater frequency of early satiation and diarrhea at one and three years: two prospective longitudinal population-based studies. Neurogastroenterol Motil. (2023) 35:e14471. doi: 10.1111/nmo.14471
37. Appleby, R, Moghul, I, Khan, S, Yee, M, Manousou, P, Neal, T, et al. Non-alcoholic fatty liver disease is associated with dysregulated bile acid synthesis and diarrhea: a prospective observational study. PLoS One. (2019) 14:e211348:e0211348. doi: 10.1371/journal.pone.0211348
38. Zhu, J, Liu, M, and Sun, C. Assessment of the triglyceride glucose index in adult patients with chronic diarrhea and constipation. World J Clin Cases. (2024) 12:1094–103. doi: 10.12998/wjcc.v12.i6.1094
39. Alshaikh, A, Alshehri, A, Alshehri, A, Alobaid, A, Mohammed, A, Saeed, F, et al. Prevalence of gastrointestinal manifestations among diabetic patients in the aseer region: a cross-sectional study. Medicine (Baltimore). (2024) 103:e39895. doi: 10.1097/MD.0000000000039895
40. Han, K, Wang, X, Wang, Y, Niu, X, Xiang, J, Ru, N, et al. Prevalence of chronic diarrhea and its association with obesity in a Chinese community-based population. Chin Med J-Peking. (2024) 4:12. doi: 10.1097/CM9.0000000000003190
41. Yang, X, Wang, M, Ren, L, Shon, K, Cui, G, Cheng, Y, et al. Association between visceral adiposity index and bowel habits and inflammatory bowel disease: a cross-sectional study. Sci Rep. (2024) 14:23923. doi: 10.1038/s41598-024-73864-0
42. Zhuang, Y, Li, L, Sun, J, Zhang, Y, and Dai, F. Association of body roundness index with chronic diarrhea and constipation, nhanes 2005-2010. J Health Popul Nutr. (2025) 44:50. doi: 10.1186/s41043-025-00793-7
43. Williams, K, Erbey, J, Becker, D, Arslanian, S, and Orchard, T. Can clinical factors estimate insulin resistance in type 1 diabetes? Diabetes. (2000) 49:626–32. doi: 10.2337/diabetes.49.4.626
44. Wang, Y, Chen, F, Zhang, B, and Song, Z. Association of the body roundness index with chronic diarrhea and chronic constipation: findings based on the National Health and nutrition examination survey 2005-2010 data. Lipids Health Dis. (2025) 24:33. doi: 10.1186/s12944-025-02451-7
45. Wei, L, Ji, L, Miao, Y, Han, X, Li, Y, Wang, Z, et al. Constipation in DM are associated with both poor glycemic control and diabetic complications: current status and future directions. Biomed Pharmacother. (2023) 165:115202. doi: 10.1016/j.biopha.2023.115202
46. Wittmann, T. Neuropathy in the gut. Gastrointestinal motility disorders in patients with diabetes mellitus. Orv Hetil. (2012) 153:607–14. doi: 10.1556/OH.2012.29360
47. Jiao, P, and Xu, H. Adipose inflammation: cause or consequence of obesity-related insulin resistance. Diabet Metab Synd Obes. (2008) 1:25–31. doi: 10.2147/dmso.s4180
48. Tysoe, O. Pdl1 reduces adipose inflammation in obesity. Nat Rev Endocrinol. (2022) 18:334. doi: 10.1038/s41574-022-00668-5
49. Tremaroli, V, and Backhed, F. Functional interactions between the gut microbiota and host metabolism. Nature. (2012) 489:242–9. doi: 10.1038/nature11552
50. Mithieux, G. Gut microbiota and host metabolism: what relationship. Neuroendocrinology. (2018) 106:352–6. doi: 10.1159/000484526
51. Abildinova, G, Benberin, V, Vochshenkova, T, Afshar, A, Mussin, N, Kaliyev, A, et al. The gut-brain-metabolic axis: exploring the role of microbiota in insulin resistance and cognitive function. Front Microbiol. (2024) 15:1463958. doi: 10.3389/fmicb.2024.1463958
52. De, P, Da, S, Mendonca, I, De Souza, J, and Peixoto, C. Semaglutide attenuates anxious and depressive-like behaviors and reverses the cognitive impairment in a type 2 diabetes mellitus mouse model via the microbiota-gut-brain Axis. J Neuroimmune Pharm. (2024) 19:36. doi: 10.1007/s11481-024-10142-w
53. Mostafavi, A, and Zhou, J. Gut Microbiota Dysbiosis, oxidative stress, inflammation, and epigenetic alterations in metabolic diseases. Antioxidants-Basel. (2024) 13:985. doi: 10.3390/antiox13080985
54. Siqueira, F, Rodrigues, F, Ribeiro, S, Veras, H, Ferreira, F, Siqueira, R, et al. Induced acute hyperglycemia modifies the barrier function of the intestinal epithelium by tissue inflammation and tight junction disruption resulting in hydroelectrolytic secretion in an animal model. Braz J Med Biol Res. (2024) 57:e13309. doi: 10.1590/1414-431X2024e13309
Keywords: intestinal health, chronic diarrhea, chronic constipation, insulin resistance surrogate indices, NHANES (National Health and Nutrition Examination Survey)
Citation: Zhao D, Zhao M, Gao B and Lu H (2025) Evaluating the role of insulin resistance in chronic intestinal health issues: NHANES study findings. Front. Nutr. 12:1602922. doi: 10.3389/fnut.2025.1602922
Edited by:
Rashu Barua, New York University, United StatesReviewed by:
Md Hasif Sinha, University of Louisville, United StatesMohammad Abul Hasnat, Shahjalal University of Science and Technology, Bangladesh
Copyright © 2025 Zhao, Zhao, Gao and Lu. This is an open-access article distributed under the terms of the Creative Commons Attribution License (CC BY). The use, distribution or reproduction in other forums is permitted, provided the original author(s) and the copyright owner(s) are credited and that the original publication in this journal is cited, in accordance with accepted academic practice. No use, distribution or reproduction is permitted which does not comply with these terms.
*Correspondence: Dongyao Zhao, enp1emR5QDE2My5jb20=