- 1Division of Sustainable Development, College of Science and Engineering, Hamad Bin Khalifa University, Qatar Foundation, Doha, Qatar
- 2Department of Health Protection and Communicable Disease Control, Ministry of Public Health, Doha, Qatar
- 3Division of Information and Computing Technology, College of Science and Engineering, Hamad Bin Khalifa University, Qatar Foundation, Doha, Qatar
- 4Department of Electrical and Computer Engineering, Pak-Austria Fachhochschule Institute of Applied Sciences and Technology, Haripur, Pakistan
- 5Department of Microbiology and Immunology, Weill Cornell Medicine-Qatar, Cornell University, Qatar Foundation-Education City, Doha, Qatar
- 6Biological and Environmental Science and Engineering Division, King Abdullah University of Science and Technology, Thuwal, Saudi Arabia
Qatar is a peninsular country with predominantly hot and humid weather, with 88% of the total population being immigrants. As such, it leaves the country liable to the introduction and dissemination of vector-borne diseases, in part due to the presence of native arthropod vectors. Qatar's weather is expected to become warmer with the changing climatic conditions across the globe. Environmental factors such as humidity and temperature contribute to the breeding and distribution of different types of mosquito species in a given region. If proper and timely precautions are not taken, a high rate of particular mosquito species can result in the transmission of various vector-borne diseases. In this study, we analyzed the environmental impact on the probability of occurrence of different mosquito species collected from several different sites in Qatar. The Naive Bayes model was used to calculate the posterior probability for various mosquito species. Further, the resulting Naive Bayes predictions were used to define the favorable environmental circumstances for identified mosquito species. The findings of this study will help in the planning and implementation of an active surveillance system and preventive measures to curb the spread of mosquitoes in Qatar.
1. Introduction
Mosquito-borne infections are a major health concern, with more than half of the world's population currently estimated to be at risk (1). World Health Organization (WHO) and the US Centers for Disease Control and Prevention (CDC), along with other international health agencies, have endeavored to improve surveillance for mosquito-borne diseases such as malaria and dengue fever, among others (2). Despite the rising global importance of mosquito-borne diseases, there are, to date no effective vaccines available to hamper the spread of these infections (3, 4). However, recently, a tetravalent chimeric vaccine, Dengvaxia (CYD-TDV), has been licensed for individuals 9–45 years of age in over ten dengue-endemic countries (5). Consequently, this puts vulnerable populations at increased risk of contracting mosquito-borne infections, thus affecting their quality of life. Several factors affect mosquito and mosquito-borne pathogens' life cycles and their geographical spread, including rapid population expansion and urbanization, animal migrations and trade, climate change, etc. (6). The impact of global climate change and environmental factors on vector-borne diseases (VBDs) can cause substantial changes in the transmission of VBD pathogens from vectors to the hosts (animal and human) (6).
Qatar is a small country with a total land area of 11,600 sq. km and a population of 3 million in 2022, and the majority (~88%) are immigrants and reside in the urban area (99%) (7). The climate in Qatar is hot and humid, and the average annual temperature is 27.1°C, with an average annual precipitation of 72 mm (8). The large immigrant force, urban area residents, and favorable weather conditions make Qatar susceptible to VBDs (9–11). Presently, as a non-endemic country, Qatar's vector control and surveillance initiatives are still inadequate (12). To address this problem, Qatar needs to strengthen its technical capacities in the field of entomology, with a particular focus on active surveillance and vector detection. Extensive long-term surveys are required to assess the effectiveness of mitigation strategies, which is a costly and time-demanding investment.
The presence of a single occurrence of VBD poses a health threat of transmission within different communities, especially those at higher risk of contracting the infection. Recently, our longitudinal and snapshot surveys in Qatar revealed that the southern house mosquito, Culex quinquefasciatus, is the most ubiquitous and populous mosquito species, followed by Culex perexiguus, suggesting a risk of West Nile virus (WNV) transmission. Anopheles stephensi was also widely distributed including in urbanized areas in Qatar, implying a possibility of local malaria transmission (9, 10, 12–14). In addition, Aedes caspius, a wetland mosquito, is also common, posing a risk of Rift Valley Virus (RFV) transmission.
Several studies have reported the effect of different factors, such as temperature, habitat, precipitation, migration, and relative humidity, on the mosquitoes' growth and abundance (15–18). Temperature is one of the essential abiotic factors responsible for insects' physiology, behavior, ecology, and survival (19). Mosquitoes adapt strategies to maintain body temperature and adapt to different climatic conditions by synthesizing heat shock proteins, thermoregulation, or changing their behavioral activity (20, 21). Moreover, temperature influences the length and duration of the extrinsic incubation period (EIP) (22). Relative humidity work in tandem with temperature to influence mosquito desiccation resistance (23), oviposition rate, adult mortality, adult survival, fecundity, hatching rates, sex proportion, and longevity ratio between female and male mosquitos (24). For example, mosquitoes have a shorter life span when relative humidity is <60%, but they live longer with increasing relative humidity (25). For Aedes aegypti, the gonotrophic cycle becomes shorter at higher mean temperatures and is optimal between 26 and 30°C (26). In addition, the temperature was the highest predictor of malaria transmission via Anopheles species, reaching its peaks at between 27 and 28°C (24, 27, 28). It was found that the risk of clinical malaria increased exponentially above 60% relative humidity and was twice as high at 80% (25). The Culex mosquito is the most prevalent mosquito species on the planet and a vector of various medically important viruses such as WNV, St. Louis Encephalitis virus (SLEV), and Japanese Encephalitis virus (JEV) (29–31). The Culex quinquefasciatus larvae also grow faster as the temperature rises, favoring the adult population (32–34).
Climate change is expected to get worse within the gulf region, which will result in higher dry and wet bulb temperatures, lower rainfalls, and increased humidity, among other weather changes (35, 36). Furthermore, a warmer climate means higher energy consumption due to the increased expulsion of waste energy from condenser/cooling towers as heat, thus further raising temperature and humidity (37–39). Additionally, a sudden increase in population during the FIFA World Cup 2022, coupled with favorable climatic conditions, is likely to facilitate the distribution and proliferation of mosquito species. Therefore, it is essential to conduct further studies that correlate the propagation of mosquito species and mosquito-borne diseases with environmental conditions.
Machine learning-based classification has become an increasingly valuable tool in recent years and can handle nonlinear, high-dimensional data with complex computable operations (40, 41). Classification algorithms, such as Naive Bayes (NB), neural networks (NN), decision tree (DT), Linear Discriminant Analysis (LDA), Support Vector Machine (SVM), and deep learning (DL) algorithms, are significant types of machine learning approaches (42–45). Due to its computational simplicity and efficiency, NB is consistently being enhanced and is frequently employed in various applications (46). For instance, Genoud et al. (47) implemented machine learning methods such as DT, NB, LDA, and SVM to predict the mosquitoes' species using optical signals. Their findings show that using optical sensors in conjunction with machine learning can be a feasible alternative or complementary to traditional mosquito population monitoring methods. Similarly, the present work focuses on implementing machine learning technique to compute the mosquitoes' specie probability distribution. In addition, the dependency of ambient conditions (ambient temperature and humidity) on the mosquito species distribution in Qatar is analyzed and discussed.
2. Materials and methods
2.1. Study area and environmental data
A total of nine different sites of diverse habitats were selected, as listed in Table 1, and the mosquitoes' samples were collected from August 2017 to August 2018, and details have previously been described elsewhere (13). The ambient conditions have been approximated using the data from six different stations of the Qatar Meteorological Department (QMD). The collected data is used to program the machine-learning algorithm, i.e., NB, to predict the probability distribution of certain mosquitoes species under environmental conditions.
2.2. Sample collection and morphological characterization
To adjust for seasonal data, a set of repeated sampling sessions was conducted across the country to gather adult mosquito samples between August 2017 and August 2018. Farms, sewage basins, widam company and zoo were among the nine places chosen to represent various environmental sub-types that could influence mosquito breeding (Table 1). Over a thousand mosquitoes were collected and transported to the laboratory for morphological and molecular characterization, as reported previously elsewhere (13). Thousands of mosquitoes were collected, but the presence of considerable by-catches (attracted by the black light) and the poor quality of preservation did not allow all specimens to be properly sorted and identified. However, to obtain an estimate of sampling outcomes under our time constraints, we performed subsampling and analyzed one randomly chosen sample per month and per site. We analyzed 327 samples, yielding the detection of seven mosquito species or groups. The details are furnished in the previously published study (13).
2.3. Environmental conditions
The dry-bulb (ambient) temperature and relative humidity observations from August 2017 to August 2018 were collected from the six meteorological stations of the Qatar Meteorological Department (QMD), Civil Aviation Authority. The locations of QMD stations are shown in Figure 1 in black dots. The Qatar Civil Aviation Authority stated that meteorological observations had undergone rigorous appropriate methodology to ensure homogeneity and quality. Furthermore, the six stations have had no systematic changes in their location or measurement techniques in the last three decades. The red dots represent the collection sites and habitats for mosquito collection. The temperature and humidity conditions of the nine sites considered in this work were derived from the data gathered from QMD stations. The inverse distance weighted (IDW) analysis tool within the geographic information system (GIS) was employed to estimate the weather conditions using the coordinates of sites (listed in Table 1) (48). The IDW is a widely used deterministic interpolation tool in the spatiotemporal characterization of climatic parameters. The IDW can be extended by reducing and extending methods to solve interpolation problems. It does not require any subjective assumption to select the semi-variogram model. Instead, it directly depends on the adjacent measured values or specific mathematical formulations for defining the smoothness of the surface. By definition, the IDW considers the measured points adjacent to the undetermined point, which is compared with the distant points. The general formula for the IDW is expressed as follows:
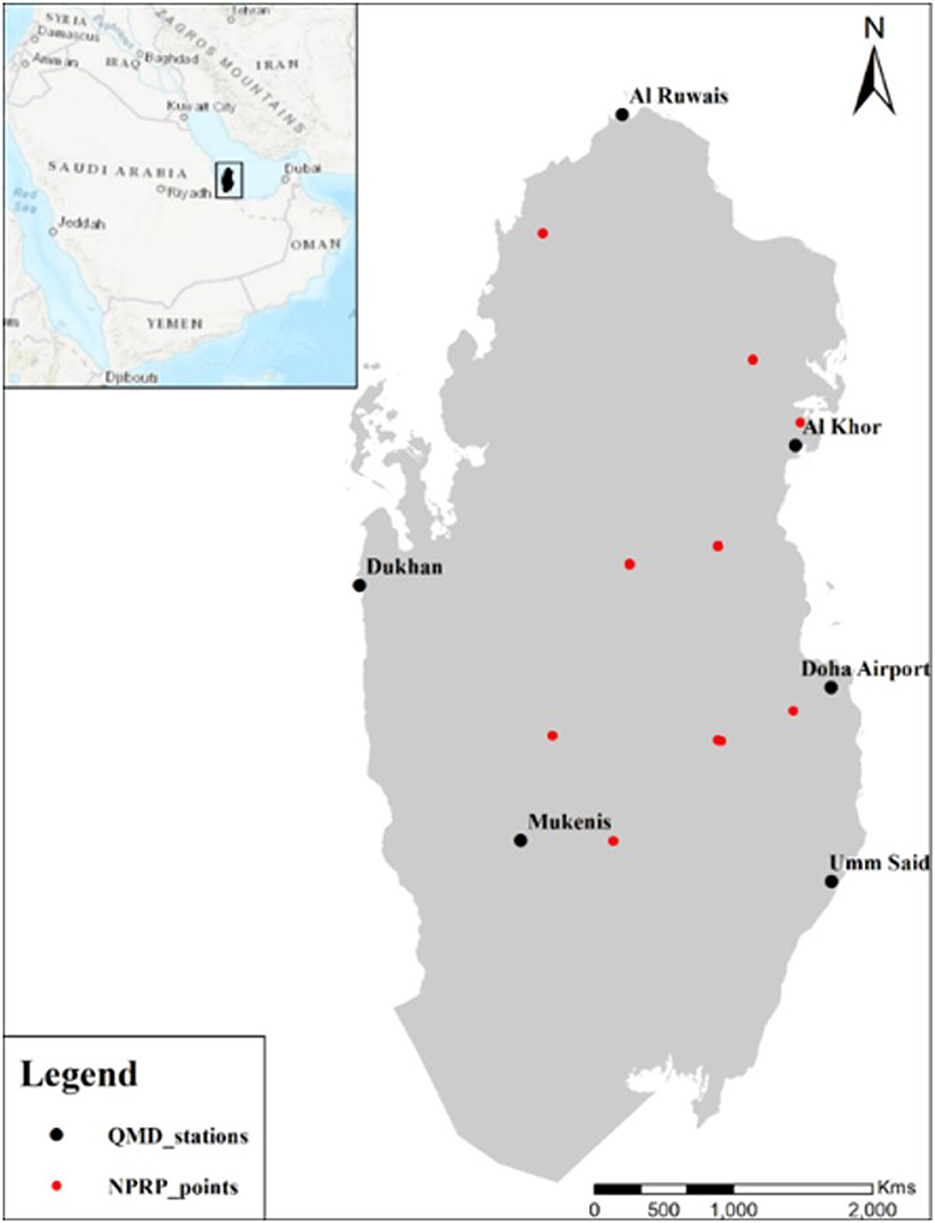
Figure 1. The location map, including Qatar Meteorological Department (QMD) stations in black and the studied nine sites in red color, respectively.
Where Z(si) is the measured/determined value at the ith location; λi is the undetermined weight for the measured value at the ith location, which depends totally on the distance to the prediction location; s0 is the prediction location, and N is the number of measured values. For more information about the IDW tool, the readers can refer to (49).
2.4. Naïve Bayes (NB) algorithm
Since its creation, Naive Bayes (NB) has been effectively used in numerous applications. The Bayes' theorem (also known as Bayes' law or Bayes' rule), which carries Thomas Bayes' name, predicts the likelihood of an event based on knowledge of circumstances that may be associated with it in probability theory and statistics (50). Its ability to forecast future probabilities of events is quite effective. A family of straightforward “probabilistic classifiers” known as NB classifiers is based on using the Bayes theorem with strong (naive) independence assumptions between the features. NB is a conditional probability model that assigns probabilities to each of C possible outcomes or classes given a problem instance to be classified, represented by a vector X encoding some n attributes. Based on the observed data, it works by estimating the posterior probabilities of a given instance belonging to each conceivable class.
This study's goal was to forecast the existence of a certain mosquito species at a particular location under particular environmental circumstances, not to categorize various mosquito species. Therefore, in this study, instead of using the NB classifier, the posterior probabilities calculated by the NB were utilized to estimate the production of different mosquito species with variations in temperature and humidity in the state of Qatar. Due to its straightforward and easy implementation, the NB model has been chosen for this study, and previously this model has been used in several similar applications (47, 51).
Suppose a data D = {Xi, yi}, where represents an instance with n features, yi represents one of class c = 1, 2, …, C associated with an instance Xi, and i = 1, 2, …N is the total N observations in D. For a new observation , NB assigns a label ŷ, which is predicted as:
where the posterior probability P is calculated by the Bayes theorem as:
Based on the features independence supposition, the joint probability is expressed as:
This indicates that the conditional distribution over the class variable c under the aforesaid independence assumptions is as follows:
where is a scaling contact depending only on the feature variables which are known.
The NB classifier calculates the posterior probabilities as defined in Equation (5) and classifies an observation as belonging to one of the classes as defined in Equation (2). In this study, the posterior probabilities calculated by the NB were utilized to estimate the production of different mosquito species with variations in temperature and humidity in the state of Qatar. Table 2 enlists the details of the arguments for the NB algorithm. Furthermore, the numerical experimentation was carried out in MATLAB R2019 on a 2.9 GHz 6-Core Intel Core i9 Mac-Book Pro.
3. Results and discussion
3.1. Morphological identification and characterization of vector species
Among the various species of mosquitoes identified, the most abundant were Culex quinquefasciatus and Cx. (Cux.) perexiguus, (which are often difficult to distinguish from dried—and frequently damaged—adults), found at all sites. The only Anopheles species found was (Cellia) stephensi at seven sites (excluding site 1: Al Khor—Sewage basins and site 6: Al Shamal—Farm). Moreover, Culex quinquefasciatus was prevalent throughout the year, while An. stephensi was only moderately abundant between October and November and then between June and July. Aedes (Ochlerotatus) caspius, was observed at six different sites and at various times throughout the year but was found in small numbers. Our entomological survey collected samples from farms, garden centers, industrial regions, sewage lakes and treatment plants, urban building areas, wetlands, worker housing, and a zoo to account for diverse elements affecting mosquito reproductive capabilities and distributions.
Furthermore, every inspected area contained one or more mosquito species, with the southern house mosquito species Culex quinquefasciatus having the most widespread geographical range due to the high degree of adaptability to their environment. Additionally, our findings were consistent with the species' known preferences and posed a risk to public health through disease transmission (52). For generalization, the mosquitoes were grouped into Culex, Anopheles, and Aedes, as shown in Figure 2. Most of the mosquitoes' samples were collected from Doha and Al-Rayyan municipalities. This data was used in combination with the weather dataset to predict the mosquitoes' probability distribution. To appropriately assess the risk of mosquito-borne diseases, routine field monitoring and analyses are required to address research gaps in terms of breeding, distribution, and biting inclinations of various mosquito species presently found in Qatar.
3.2. Environmental conditions
Figure 3 exhibits the monthly average ambient temperature and relative humidity distributions for selected sites from August 2017 to August 2018. The lowest monthly average temperature recorded in January ranged from 16.9 to 18.8°C. The temperatures steadily rose as the summer months approached. For the period from June to August, the monthly average temperature reached 35 to 37°C. However, the maximum temperature during summer can potentially reach well beyond 45°C.
Moreover, the monthly average temperature variation was small between different sites, and the maximum variation in temperature was <2°C. The monthly average temperature at site 8 was found to be slightly higher than at the other sites. The monthly average relative humidity variation for different sites is shown in Figure 4. The humidity index in Qatar typically tends to be high, averaging in the range of 45–65% during the half of summer (August and September), fall, and most of the winter season until the following February. Afterward, the average monthly humidity decreased to the lowest at 26% (June) and then increased again during the summer, thus continuing the cycle. In contrast with the temperature profile, the variation of relative humidity (12–22%) among different sites was statistically significant. In summary, site 8 was relatively warmer and less humid, and site 6 exhibited lower monthly average temperature and higher relative humidity than other sites, respectively.
3.3. Probability distribution
At first, the accuracy of the NB model is evaluated by conducting a 10-fold cross-validation, and the observed model's accuracy was found to be 81.34%. The model's accuracy can be further improved by improving the class imbalance problem of the collected dataset. As, many samples in this dataset belong to Culex class only, therefore the classifier learning can be biased toward this particular class. Culex families of mosquitoes were found to be more abundant in Qatar; hence, the probabilities were computed only for Anopheles and Aedes mosquitoes. The results presented in sections Morphological identification and characterization of vector species and Environmental conditions were used to train the NB algorithm to predict the distribution of mosquito species. Figure 5 shows the probability distribution of Anopheles (malaria vector) throughout the year for the different collection sites. It was observed that the probability of the presence of Anopheles mosquitoes is lowest during the winter season (≤ 0.05) and increases during summer, reaching its peak in June, given that they thrive in warmer temperatures. However, it varies when compared between different sites as the probability of Anopheles mosquito at site 6 is just 0.162 and at site 8 is 0.538. This significant difference could be due to site 8 (Doha—Widam Company) being much warmer and less humid than site 6 (Al Shamal—Farm), which has lower ambient temperature and higher humidity compared to the other sites. Figure 6 represents the Aedes mosquito probability distribution from August 2017 to August 2018 for the different collection sites. It follows a similar trend to that of Anopheles mosquito distribution, i.e., lower presence in the winter (December to February) and higher in the summer season, respectively. However, in the summer season, the probability of presence of Aedes mosquitoes is lower than that of Anopheles mosquitoes. Like Anopheles mosquito probability distribution, the probability for Aedes mosquito tends to decrease at higher humidity. Thus, temperatures around 35°C with humidity levels of 35–45 % are suitable environmental conditions for the abundance of both species, i.e., Anopheles and Aedes.
These conditions, compounded by a largely immigrant population of Qatar who travels to and from VBD endemic countries (9), have the potential to further propagate Anopheles and Aedes species of mosquitoes.
Figure 7 shows the comparative distribution probability of mosquitoes with respect to temperature and humidity. For both species (Anopheles and Aedes), the likelihood increased at higher temperatures and lower humidity. However, for Aedes mosquito, the probability increased with the declining humidity levels to a certain point before it started to decrease again. The suitable environment for Aedes mosquitoes was observed to be in the range of 35–40°C temperature and 35–45 % relative humidity. Furthermore, our findings align with the studies (28, 53, 54). Although machine-learning models are good at predicting the mosquitoes' specie probability distribution, the only drawback these models have is the requirement of labeled data for model learning. It is often difficult to collect and label different mosquito species, especially the species those are rarely found at specific sites. Therefore, it might not be feasible to apply such models in scenarios where the labeled data is not available. However, if the labeled data is available to train machine-learning models, these models can easily predict the presence of certain species at certain sites in advance, and therefore, precautionary measures could be taken in advance to control the production of mosquitos on those sites, if necessary.
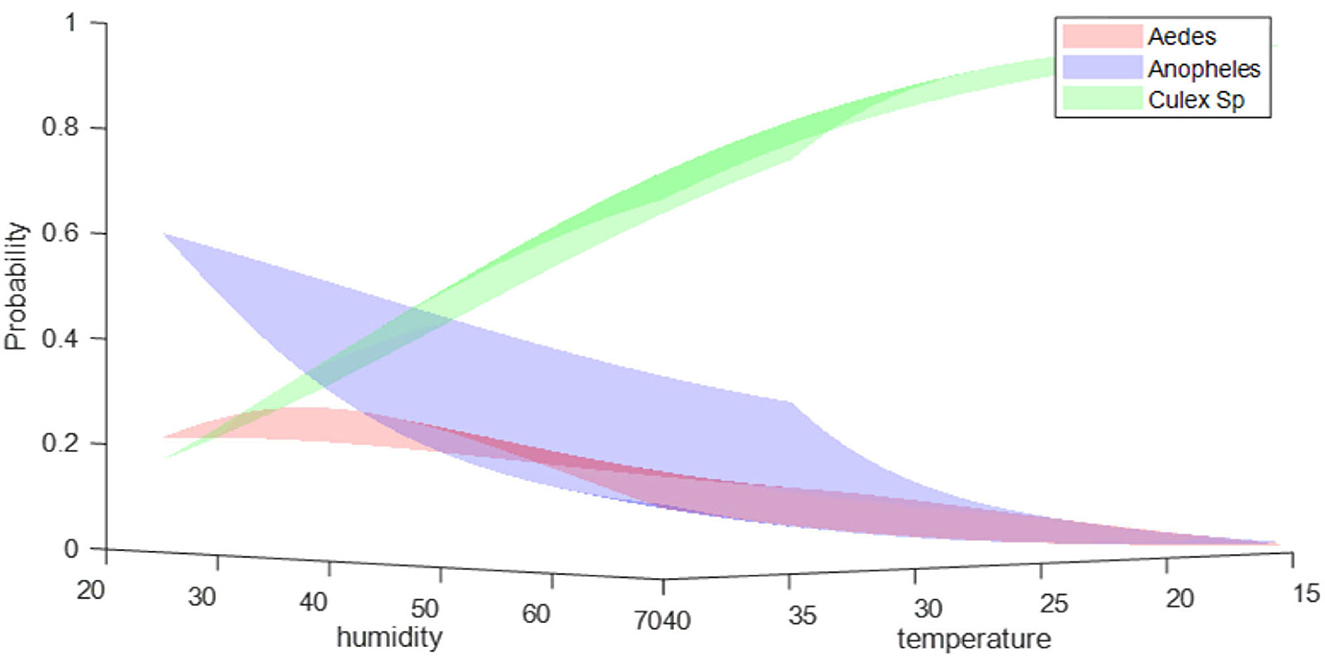
Figure 7. Predicted probability distribution of mosquito species with respect to environmental conditions (ambient temperature and humidity).
4. Conclusion and recommendations
Climate change affects the environment and humans, and also has social and economic implications. The increased greenhouse gas emissions on the part of human activities contribute to warming temperatures on Earth. This will, in turn, engender further dissemination of infectious diseases via vectors of concern and floods—all of which are threats to human health. Such events propel human beings beyond the limits to which they can adapt; therefore, death rates increase. Depending on the area that a population is living in, the relationship between temperature and mortality varies. The changes in temperature and other climate variables control how vectors are redistributed. Such changes contribute to the burden of VBDs and the deterioration of human health.
This study conducted mosquitoes species identification and propagation at nine different sites of various habitats in Qatar using the Naive Bayes algorithm. It was found that the Culex mosquitoes are the most abundant, followed by the Anopheles and Aedes types. The Anopheles and Aedes types were observed at seven and six collection sites, respectively. Results show that higher temperatures and lesser humidity enhance the chance of both species (Anopheles and Aedes). However, the risk of an Aedes mosquito increases as the humidity decreases, but only to a certain point and then drops. Temperatures between 35 and 40°C and relative humidity levels between 35 and 45 % are ideal for Aedes mosquitoes. Our results reaffirm the need for a robust surveillance system working in combination with the environmental sector and an extensive, multivariate dataset to provide a clearer outlook of the potential distribution and abundance of mosquito species.
A resilient healthcare structure to protect against mosquito-borne infection requires (1) continuous monitoring and surveillance and (2) a large dataset to provide a clearer picture of the likelihood of mosquito species abundance and distribution. In addition, there is an increased risk of VBD transmission during FIFA World Cup 2022 as Qatar prepares to host the event, with over a million attendees expected to arrive in the country. Therefore, policy development and implementation are vital for adequate emergency preparedness and response for VBDs.
Data availability statement
The raw data supporting the conclusions of this article will be made available by the authors, without undue reservation.
Author contributions
Conceptualization: FT, DB, AR, and SA-G. Methodology: FT, DB, AR, and SB. Software: FT, SA, and AR. Formal analysis: FT and DB. Investigation: DB, HA-R, and MA-T. Resources: EF, AS, and SA-G. Data curation and visualization: FT and AR. Writing—original draft preparation: FT, DB, and AR. Writing—review and editing: SS, AS, EF, and SA-G. Supervision: SA-G, AS, and EF. Project administration: AS and SA-G. Funding acquisition: SA-G, AS, EF, and DB. All authors contributed to the article and approved the submitted version.
Funding
This publication was made possible by NPRP-Standard (NPRP-S) Twelfth (12th) Cycle grant NPRP12S-0212-190073 from the Qatar National Research Fund (a member of Qatar Foundation). DB and EF benefit from an NPRP grant (NPRP12S-0310-190284) from the QNRF. The findings herein reflect the work and are solely the responsibility of the authors.
Acknowledgments
We express our gratitude and appreciation to MoPH for the support with staff and funding of the field studies. We also thank the Friends of Environment Center for their support in sample collection, as well as colleagues of Doha and Al Rayyan pest control units for assisting in the fieldwork.
Conflict of interest
The authors declare that the research was conducted in the absence of any commercial or financial relationships that could be construed as a potential conflict of interest.
Publisher's note
All claims expressed in this article are solely those of the authors and do not necessarily represent those of their affiliated organizations, or those of the publisher, the editors and the reviewers. Any product that may be evaluated in this article, or claim that may be made by its manufacturer, is not guaranteed or endorsed by the publisher.
Author disclaimer
Any opinions, findings, conclusions, or recommendations expressed in this work are those of the authors and do not necessarily reflect the views of HBKU, MoPH, and QNRF.
Supplementary material
The Supplementary Material for this article can be found online at: https://www.frontiersin.org/articles/10.3389/fpubh.2022.970694/full#supplementary-material
References
1. World Health Organization. A Global Brief on Vector-Borne Diseases. (2014). Geneva, Switzerland: World Health Organization
2. Thacker SB. The President's Malaria initiative. US: United States Agency for International Development Centers for Disease Control and Prevention (US), Department of Health and Human Services (US) (2018).
3. Manguin S, Dev V. Towards Malaria Elimination - A Leap Forward. Manguin S, Dev V, editors InTech (2018).
4. Valenzuela JG, Aksoy S. Impact of vector biology research on old and emerging neglected tropical diseases. PLoS Negl Trop Dis. (2018) 12:e0006365. doi: 10.1371/journal.pntd.0006365
5. Thomas SJ, Yoon I-K, A. review of Dengvaxia®: development to deployment. Hum Vaccin Immunother. (2019) 15:2295–314. doi: 10.1080/21645515.2019.1658503
6. Mathieu K, Karmali M. Vector-borne diseases, climate change and healthy urban living: Next steps. Canada Commun Dis Rep. (2016) 42:220–1. doi: 10.14745/ccdr.v42i10a13
7. Qatar. Index Mundi. Available from: https://www.indexmundi.com/facts/qatar/#Environment (accessed on 2022 September 29).
8. Climate: Qatar. Climate-Data. Available from: https://en.climate-data.org/asia/qatar-183/ (accessed on 2022 September 29).
9. Farag E, Bansal D, Chehab MAH, Al-Dahshan A, Bala M, Ganesan N, et al. Epidemiology of Malaria in the State of Qatar, 2008-2015. Mediterr J Hematol Infect Dis. (2018) 10:e2018050. doi: 10.4084/mjhid.2018.050
10. Schaffner F, Bansal D, Mardini K, Al-Marri SA, Al-Thani MHJ, Al-Romaihi H, et al. Vectors and vector-borne diseases in Qatar: current status, key challenges and future prospects. J Eur Mosq Control Assoc. (2021) 39:3–13. doi: 10.52004/JEMCA2021.x001
11. Tahir F, Ajjur SB, Serdar MZ, Al-Humaiqani MMA, Kim D, Al-Thani SK, et al. Qatar Climate Change Conference 2021: A platform for addressing key climate change topics facing Qatar and the world. Al-Ghamdi SG, Al-Thani SK, editors. Doha, Qatar: Hamad bin Khalifa University Press (HBKU Press) (2021), 1–38 p.
12. Schaffner F, Bansal D, Al-Thani MHJ, Al-Romaihi H, Farag EABA. Preventing vector-borne diseases at major sport events: Addressing the challenges for FIFA 22 in Qatar. PLoS Negl Trop Dis. (2021) 15:e0009135. doi: 10.1371/journal.pntd.0009135
13. Farag EABA, Bansal D, Mardini K, Sultan AA, Al-Thani MHJ, Al-Marri SA, et al. Identification and characterisation of mosquitoes from different locations in Qatar in 2017-2019. Parasite. (2021) 28:84. doi: 10.1051/parasite/2021079
14. Farag EA, Jaffrey S, Daraan F, Al-Shamali MHMA, Khan FY, Coyle P V, et al. Dengue Epidemiology in Qatar from 2013–2021: A Retrospective Study. Trop Med Infect Dis. (2022) 7:329. doi: 10.3390/tropicalmed7110329
15. Thu HM, Aye KM, Thein S. The effect of temperature and humidity on dengue virus propagation in Aedes aegypti mosquitos. Southeast Asian J Trop Med Public Health. (1998) 29:280–4.
16. Couret J, Benedict MQ, A. meta-analysis of the factors influencing development rate variation in Aedes aegypti (Diptera: Culicidae). BMC Ecol. (2014) 14:3. doi: 10.1186/1472-6785-14-3
17. Reinhold J, Lazzari C, Lahondère C. Effects of the environmental temperature on aedes aegypti and aedes albopictus mosquitoes: a review. Insects. (2018) 9:158. doi: 10.3390/insects9040158
18. Ryan SJ, Carlson CJ, Tesla B, Bonds MH, Ngonghala CN, Mordecai EA, et al. Warming temperatures could expose more than 1.3 billion new people to Zika virus risk by 2050. Glob Chang Biol. (2021) 27:84–93. doi: 10.1111/gcb.15384
19. Denlinger DL, Yocum GD. “Physiology of heat sensitivity,” in Temperature Sensitivity in Insects and Application in Integrated Pest Management. Boca Raton, FL: CRC Press (2019), 7–53. Available from: https://www.taylorfrancis.com/books/9781000242430/chapters/10.1201/9780429308581-2 doi: 10.1201/9780429308581-2
20. Benoit JB, Lopez-Martinez G, Patrick KR, Phillips ZP, Krause TB, Denlinger DL. Drinking a hot blood meal elicits a protective heat shock response in mosquitoes. Proc Natl Acad Sci. (2011) 108:8026–9. doi: 10.1073/pnas.1105195108
21. Lahondère C, Lazzari CR. Mosquitoes cool down during blood feeding to avoid overheating. Curr Biol. (2012) 22:40–5. doi: 10.1016/j.cub.2011.11.029
22. Paaijmans KP, Read AF, Thomas MB. Understanding the link between malaria risk and climate. Proc Natl Acad Sci. (2009) 106:13844–9. doi: 10.1073/pnas.0903423106
23. Mogi M, Miyagi I, Abadi K. Inter- and Intraspecific Variation in Resistance to Desiccation by Adult Aedes (Stegomyia) spp. (Diptera: Culicidae) from Indonesia. J Med Entomol. (1996) 33:53–7. doi: 10.1093/jmedent/33.1.53
24. Costa EAP de A, Santos EM de M, Correia JC, Albuquerque CMR de. Impact of small variations in temperature and humidity on the reproductive activity and survival of Aedes aegypti (Diptera, Culicidae). Rev Bras Entomol. (2010) 54:488–93. doi: 10.1590/S0085-56262010000300021
25. Yé Y, Louis VR, Simboro S, Sauerborn R. Effect of meteorological factors on clinical malaria risk among children: an assessment using village-based meteorological stations and community-based parasitological survey. BMC Public Health. (2007) 7:101. doi: 10.1186/1471-2458-7-101
26. Carrington LB, Armijos MV, Lambrechts L, Barker CM, Scott TW. Effects of fluctuating daily temperatures at critical thermal etremes on aedes aegypti life-history traits. PLoS ONE. (2013) 8:e58824. doi: 10.1371/journal.pone.0058824
27. Beck-Johnson LM, Nelson WA, Paaijmans KP, Read AF, Thomas MB, Bjørnstad ON. The Effect of Temperature on Anopheles Mosquito Population Dynamics and the Potential for Malaria Transmission. PLoS ONE. (2013) 8:e79276. doi: 10.1371/journal.pone.0079276
28. Yan G, Githeko A, Zhou G, Minakawa N, Omukunda E. Malaria vector productivity in relation to the highland environment in Kenya. Am J Trop Med Hyg. (2006) 75:448–53. doi: 10.4269/ajtmh.2006.75.448
29. Weaver SC, Reisen WK. Present and future arboviral threats. Antiviral Res. (2010) 85:328–45. doi: 10.1016/j.antiviral.2009.10.008
30. Daep CA, Muñoz-Jordán JL, Eugenin EA. Flaviviruses, an expanding threat in public health: focus on dengue, West Nile, and Japanese encephalitis virus. J Neurovirol. (2014) 20:539–60. doi: 10.1007/s13365-014-0285-z
31. Diaz-Badillo A, Bolling BG, Perez-Ramirez G, Moore CG, Martinez-Munoz JP, Padilla-Viveros AA, et al. The distribution of potential West Nile virus vectors, Culex pipiens pipiens and Culex pipiens quinquefasciatus (Diptera: Culicidae), in Mexico City. Parasit Vectors. (2011) 4:70. doi: 10.1186/1756-3305-4-70
32. Seah A, Aik J, Ng L-C. Effect of meteorological factors on Culex mosquitoes in Singapore: a time series analysis. Int J Biometeorol. (2021) 65:963–5. doi: 10.1007/s00484-020-02059-9
33. Soh S, Aik J. The abundance of Culex mosquito vectors for West Nile Virus and other flaviviruses: A time-series analysis of rainfall and temperature dependence in Singapore. Sci Total Environ. (2021) 754:142420. doi: 10.1016/j.scitotenv.2020.142420
34. Reisen WK, Fang Y, Martinez VM. Effects of Temperature on the Transmission of West Nile Virus by Culex tarsalis (Diptera: Culicidae). J Med Entomol. (2006) 43:309–17. doi: 10.1093/jmedent/43.2.309
35. Pal JS, Eltahir EAB. Future temperature in southwest Asia projected to exceed a threshold for human adaptability. Nat Clim Chang. (2016) 6:197–200. doi: 10.1038/nclimate2833
36. Kang S, Tuel A, Eltahir E ~A. ~B. “Projections of high resolution climate change for Northwest Africa using MIT-Regional Climate model,” in AGU Fall Meeting Abstracts. (2018). p. A21L-2896.
37. Andrić I, Le Corre O, Lacarrière B, Ferrão P, Al-Ghamdi SG. Initial approximation of the implications for architecture due to climate change. Adv Build Energy Res. (2021) 15:337–67. doi: 10.1080/17512549.2018.1562980
38. Andric I, Kamal A, Al-Ghamdi SG. Efficiency of green roofs and green walls as climate change mitigation measures in extremely hot and dry climate: Case study of Qatar. Energy Reports. (2020) 6:2476–89. doi: 10.1016/j.egyr.2020.09.006
39. Salimi M, Al-Ghamdi SG. Climate change impacts on critical urban infrastructure and urban resiliency strategies for the Middle East. Sustain Cities Soc. (2020) 54:101948. doi: 10.1016/j.scs.2019.101948
40. Rehman AU, Belhaouari SB, Ijaz M, Bermak A, Hamdi M. Multi-classifier tree with transient features for drift compensation in electronic nose. IEEE Sens J. (2021) 21:6564–74. doi: 10.1109/JSEN.2020.3041949
41. Thessen A. Adoption of machine learning techniques in ecology and earth science. One Ecosyst. (2016) 1:e8621. doi: 10.3897/oneeco.1.e8621
42. Chickering M, Heckerman D, Meek C. Large-sample learning of Bayesian networks is NP-hard. J Mach Learn Res. (2004) 5:1287–330. Available online at: https://www.jmlr.org/papers/volume5/chickering04a/chickering04a.pdf (accessed August 25, 2022).
43. Kohonen T. An introduction to neural computing. Neural Netw. (1988) 1:3–16. doi: 10.1016/0893-6080(88)90020-2
44. Hinton GE, Osindero S, Teh Y-W. A fast learning algorithm for deep belief nets. Neural Comput. (2006) 18:1527–54. doi: 10.1162/neco.2006.18.7.1527
45. Quinlan JR. Decision trees and decision-making. IEEE Trans Syst Man Cybern. (1990) 20:339–46. doi: 10.1109/21.52545
46. Xue Z, Wei J, Guo W, A. Real-time naive bayes classifier accelerator on FPGA. IEEE Access. (2020) 8:40755–66. doi: 10.1109/ACCESS.2020.2976879
47. Genoud AP, Gao Y, Williams GM, Thomas BP, A. comparison of supervised machine learning algorithms for mosquito identification from backscattered optical signals. Ecol Inform. (2020) 58:101090. doi: 10.1016/j.ecoinf.2020.101090
48. Ajjur S, Baalousha H. Sustainable development of qatar aquifers under global warming impact. Int J Glob Warm. (2021) 25:1. doi: 10.1504/IJGW.2021.10039988
49. Li L. “Constraint databases and data interpolation,” in: Encyclopedia of GIS (2008) (Boston, MA: Springer US), 144–53. doi: 10.1007/978-0-387-35973-1_188
50. Rish I, et al. “An empirical study of the naive Bayes classifier,” in IJCAI 2001 Workshop on Empirical Methods in Artificial Intelligence (2001), 41–6.
51. Kasinathan T, Singaraju D, Uyyala SR. Insect classification and detection in field crops using modern machine learning techniques. Inf Process Agric. (2021) 8:446–57. doi: 10.1016/j.inpa.2020.09.006
52. Becker N, Petric D, Zgomba M, Boase C, Madon M, Dahl C, et al. Mosquitoes and their control. Spr Sci. Bus. Media. (2010). doi: 10.1007/978-3-540-92874-4
53. Portilla Cabrera CV, Selvaraj JJ. Geographic shifts in the bioclimatic suitability for Aedes aegypti under climate change scenarios in Colombia. Heliyon. (2020) 6:e03101. doi: 10.1016/j.heliyon.2019.e03101
Keywords: climate change, environment, malaria, mosquitoes, Naive Bayes, Qatar, vector-borne disease (VBD)
Citation: Tahir F, Bansal D, Rehman Au, Ajjur SB, Skariah S, Belhaouari SB, Al-Romaihi H, Al-Thani MHJ, Farag E, Sultan AA and Al-Ghamdi SG (2023) Assessing the impact of climate conditions on the distribution of mosquito species in Qatar. Front. Public Health 10:970694. doi: 10.3389/fpubh.2022.970694
Received: 02 November 2022; Accepted: 28 December 2022;
Published: 16 January 2023.
Edited by:
Sheng-Qun Deng, Anhui Medical University, ChinaReviewed by:
Qiang Huang, Sun Yat-sen University, ChinaDevanathan Sukumaran, Defence Research and Development Establishment (DRDE), India
Yong Wei, Shenzhen Qianhai Shekou Free Trade Zone Hospital, China
Copyright © 2023 Tahir, Bansal, Rehman, Ajjur, Skariah, Belhaouari, Al-Romaihi, Al-Thani, Farag, Sultan and Al-Ghamdi. This is an open-access article distributed under the terms of the Creative Commons Attribution License (CC BY). The use, distribution or reproduction in other forums is permitted, provided the original author(s) and the copyright owner(s) are credited and that the original publication in this journal is cited, in accordance with accepted academic practice. No use, distribution or reproduction is permitted which does not comply with these terms.
*Correspondence: Sami G. Al-Ghamdi, c2FtaS5hbGdoYW1kaUBrYXVzdC5lZHUuc2E=
†ORCID: Sami G. Al-Ghamdi orcid.org/0000-0002-7416-5153