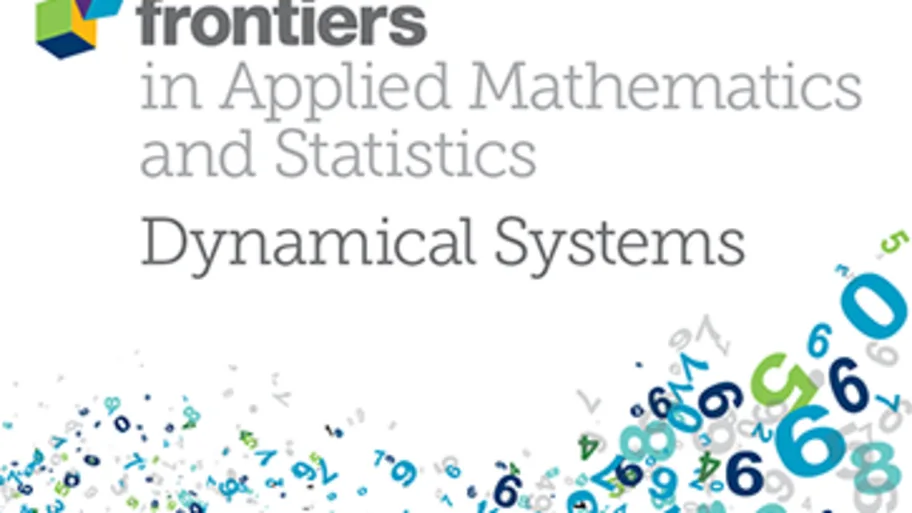
- Science News
- Engineering
- Complexity of the unexpected
Complexity of the unexpected
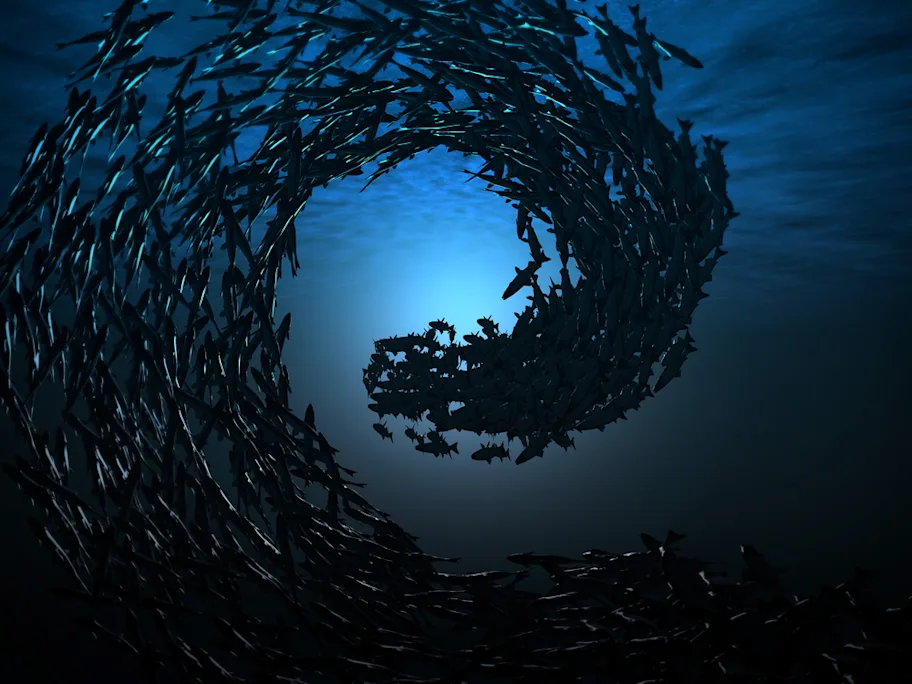
by Mikhail Prokopenko, Centre for Complex Systems, University of Sydney
Complex systems is a new approach to science, engineering and management that studies how relationships between parts give rise to the collective behaviors of the entire system, and how the system interacts with its environment. Dynamics of a complex system cannot be predicted, or explained, as a linear aggregation of the individual dynamics of its components, and the interactions among the many constituent microscopic parts bring about macroscopic phenomena that cannot be understood by considering any single part alone (“the whole is more than the sum of the parts”).
Complex systems are often confused with complicated systems which may also comprise a large number of components and interactions. This is not surprising: after all, both concepts express a notion opposite to being simple or straightforward. The two terms also share a common Latin origin: complexus originates from complectī (“to entwine or encircle”), derived in turn from com- (“together”) and plectere (“to weave”), while complicātus is a form of complicāre (“to fold together”) which augments com- (“together”) with plecāre (“to fold”). So how significant is the difference between weaving and folding some parts together?
Naively, this subtle distinction reflects on different design approaches: one flexibly weaves and interconnects the elements, revealing elastic and resilient emergent forms; while the other rigidly folds the components and reduces their interaction potential, following a prescribed procedure towards a planned, if often brittle, structure with predictable behavior.
This divergence becomes even more apparent when one compares natural organisms which have evolved their adaptive and self-organizing responses, on the one hand, with artificial machines which conform to precise blueprints and operate under predefined protocols, on the other. As noted by a well-known biologist, Carl Woese: “Machines are stable and accurate because they are designed and built to be so. The stability of an organism lies in resilience, the homeostatic capacity to reestablish itself.”
One striking example of biological complexity is a swarming behavior exhibited by schools of fish, herds of wildebeest, and flocks of birds. In response to a predator, many schools of fish display complex collective patterns of spatial aggregation, so that small perturbations can quickly cascade through an entire swarm in a wave-like manner transferring the survival- critical information.
While complex self-organizing systems adaptively process information in creating and exploiting emergent non-deterministic patterns, our engineering and management practice is driven by data, producing complicated designs and predictable deterministic regimes that prove brittle to unexpected malfunctions over and over again.
As modern day infrastructure is growing more interconnected, the breakdown of a single transformer in a small substation can lead to massive cascading failures in a continent-wide electrical power grid, triggering further interruptions to traffic and communication systems; the emergence of a new pathogen in a remote village can give rise to a devastating global epidemic; the introduction of an exotic new species can eventually contribute to a chain of food-web disruptions and wide ecosystem collapses.
Humans are typically inclined to use reductionist logic and analyse a system through a series of short, discrete scenarios, expecting a “correct response” to each scenario. However, not all scenarios have clear endings or known, correct answers. Modern power grids, communication and transport networks, mega-projects, and diverse social systems exhibit critical phenomena, characterized by phase transitions and tipping points, when a small change triggers a strong or even catastrophic response in the overall dynamics. How can we predict the behavior of systems that are too complex for our typical reductionist reasoning? The answers to this question are not intuitive or trivial, requiring a specific skill set which must be developed within educational programs explicitly dedicated to Complex Systems. Complexity, as a field of study, has shaped beyond the confines of physics, biology, mathematics, computer science and other disciplines which strongly contributed to its inception, and is on a verge of a rapid expansion within educational programs worldwide.
Professionals educated in science, engineering and management of Complex Systems will quantify the impact of unexpected events, design and analyse resilient socio-technological systems, and develop robust strategies for crisis forecasting and management. They will operate across discipline boundaries, in environments outside the experience of most professionals, providing key modelling and policy-informing inputs and insights to resolution of recurrent challenges across the globe.
The University of Sydney’s postgraduate program in Complex Systems, including a Master of Complex Systems (MCXS) offered from 2017, is unique in the Southern Hemisphere. It leverages the research strengths of its newly created Centre for Complex Systems and is aimed at an exclusive cohort of high-achieving individuals.
MCXS provides strong comprehensive skills in computational analysis, modelling and simulation of collective and dynamic emergent phenomena, while engaging quantitative social and health sciences. The core units of study include large-scale networks, agent-based modelling, complex civil systems, self-organisation and criticality, statistics, stability analysis, and visualization. The program also offers several internship opportunities, leading to specializations in engineering, biosecurity, ecology, transport, and research methods, covering disaster management, computational epidemiology, nonlinear dynamics, smart grids, control theory, resilient supply chains and quantitative logistics.
It is expected that a number of MCXS graduates will continue on a pathway to a research career, advancing the field of Complex Systems in the 21st century, and harnessing the power of complexity in real-world applications. A recent research topic in Frontiers of Robotics and AI has been devoted to theory and applications of Guided Self-Organisation (GSO) in real and synthetic dynamical systems. The goal of GSO is precisely to leverage the strengths of complex systems (adaptability, robustness, scalability) while directing the intrinsic self-organisation to constructive outcomes . This research topic investigated GSO across several domains, ranging from information processing in biological systems, to quantification of synergy in complex networks, to learning new robotic behaviors.
More likely than not, the scope of Complex Systems research will keep expanding as we continue to explore our interconnected world: as pointed out by a physicist Heinz R. Pagels several decades ago, “Science has explored the microcosmos and the macrocosmos; we have a good sense of the lay of the land. The great unexplored frontier is complexity.”
The 8th International Workshop on Guided Self-Organization is a satellite Workshop at the The Fifteenth International Conference on the Synthesis and Simulation of Living Systems. It takes place in Cancun, Mexico, 4 to 8 July 2016 at the International Convention Center (CANCUN ICC).
GSO-2016 is organized by The International Association for Guided Self-Organization (TIA-GSO).