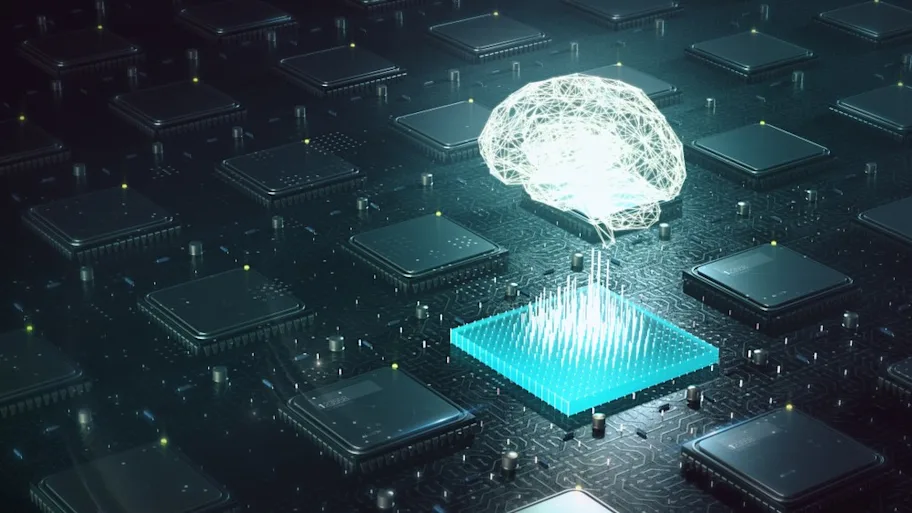
- Science News
- Featured news
- Artificial intelligence tricked by optical illusion, just like humans
Artificial intelligence tricked by optical illusion, just like humans
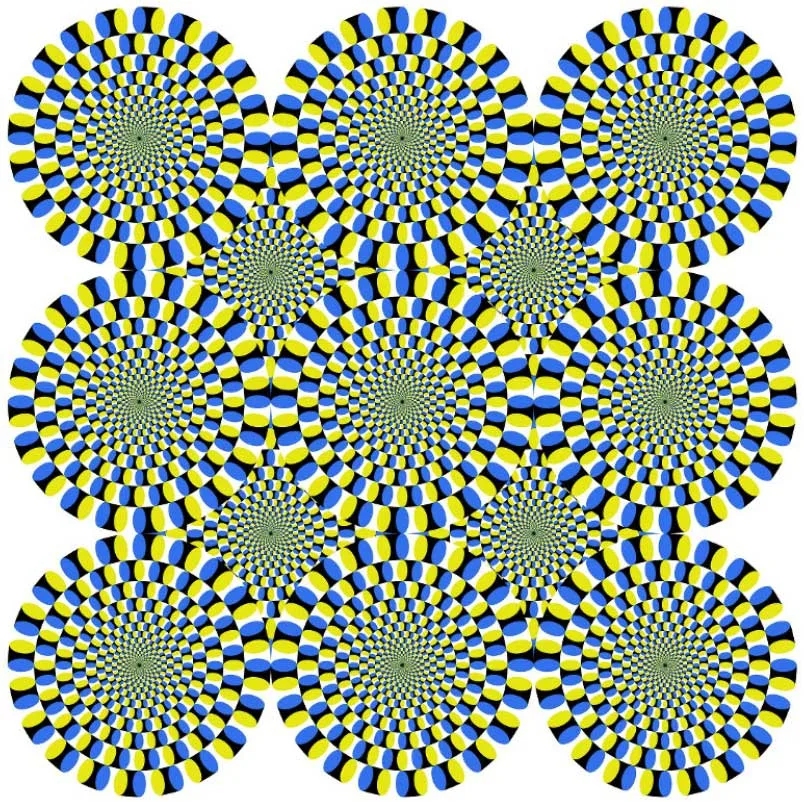
The stationary “rotating snake illusion” image, where the circles are mistakenly perceived as rotating by the human brain. Photo courtesy of Professor Akiyoshi Kitaoka, Ritsumeikan University, Japan
The study suggests that predictive coding theory is the basis of illusory motion
— By National Institutes of Natural Sciences, Japan
Artificial intelligence (AI) is tricked by optical illusions, find researchers working on deep neural networks (DNNs). The study is published in Frontiers in Psychology.
Developed with reference to the network structures and the operational algorithms of the brain, DNNs have achieved notable success in a broad range of fields — including computer vision, in which they have produced results comparable to, and in some cases superior to, human experts.
DNNs are based on predictive coding theory. This assumes that the internal models of the brain predict the visual world at all times and that errors between the prediction and the actual sensory input further refine the internal models. If the theory substantially reproduces the visual information processing of the brain, then the DNNs can be expected to represent the human visual perception of motion.
Related news: Researchers find algorithm for large-scale brain simulations
In this research, the DNNs were trained with natural-scene videos of motion from the point of view of the viewer. The motion prediction ability of the obtained computer model was then verified using a rotating propeller in unlearned videos and the “Rotating Snake Illusion” (image above).
The computer model accurately predicted the magnitude and direction of motion of the rotating propeller in the unlearned videos. Surprisingly, it also represented the rotational motion for the illusion images that were not physically moving — much like human visual perception. While the trained network accurately reproduced the direction of illusory rotation, it did not detect motion components in negative control pictures wherein people do not perceive illusory motion.
Associate professor Eiji Watanabe of the National Institute for Basic Biology, who led the research team, said: “This research supports the exciting idea that the mechanism assumed by the predictive coding theory is a basis of motion illusion generation. Using sensory illusions as indicators of human perception, deep neural networks are expected to contribute significantly to the development of brain research.”
Original article: Illusory Motion Reproduced by Deep Neural Networks Trained for Prediction
Corresponding author: Eiji Watanabe
REPUBLISHING GUIDELINES: Open access and sharing research is part of Frontier’s mission. Unless otherwise noted, you can republish articles posted in the Frontiers news blog — as long as you include a link back to the original research. Selling the articles is not allowed.