- 1Forensic Toxicology and Chemistry Unit, CURML, Lausanne University Hospital, Geneva University Hospitals, Lausanne, Switzerland
- 2Faculty Unit of Toxicology, CURML, Faculty of Biology and Medicine, University of Lausanne, Lausanne, Switzerland
- 3Unit of Medicine and Traffic Psychology, CURML, Lausanne University Hospital, Geneva University Hospitals, Lausanne, Switzerland
- 4Center for Primary Care and Public Health (Unisanté), University of Lausanne, Lausanne, Switzerland
Driving under the influence of psychoactive substances is a major cause of motor vehicle crashes. The identification and quantification of substances most frequently involved in impaired-driving cases in a single analytic procedure could be an important asset in forensic toxicology. In this study, a highly sensitive and selective liquid chromatography (LC) approach hyphenated with Orbitrap high-resolution mass spectrometry (HRMS) was developed for the quantification of the main drugs present in the context of driving under the influence of drugs (DUID) using 100 μL of whole blood. This procedure involves a simple sample preparation and benefit from the selectivity brought by parallel reaction monitoring (PRM) allowing to solve most DUID cases using a single multi-analyte injection. The method was fully validated for the quantification of the major classes of psychoactive substances associated with impaired-driving (cannabinoids, cocaine and its metabolites, amphetamines, opiates and opioids, and the major benzodiazepines and z-drugs). The validation guidelines set by the “Société Française des Sciences et des Techniques Pharmaceutiques” (SFSTP) were respected for 22 psychoactive substances using 15 internal standards. Trueness was measured to be between 95.3 and 107.6% for all the tested concentrations. Precision represented by repeatability and intermediate precision was lower than 12% while recovery (RE) and matrix effect (ME) ranged from 49 to 105% and from −51 to 3%, respectively. The validated procedure provides an efficient approach for the simultaneous and simple quantification of the major drugs associated with impaired driving benefiting from the selectivity of PRM.
Introduction
Road crashes are a worldwide public health issue, causing a significant number of deaths and injuries each year. Indeed, 1.25 million people died and about 50 million were injured in road traffic crashes in 2015 according to the World Health Organization (2015). In addition, in Europe almost 25% of adults reported at least one instance of illicit drug consumption in their life (Liakoni et al., 2018). These two issues are closely linked, since one of the major causes of road crashes is the consumption of psychotropic substances, including drugs and alcohol, resulting in driving impairment (Elliott et al., 2009; Favretto et al., 2018). For instance, in Norway, at least 21% of traffic crashes were related to either alcohol or drug use between 2005 and 2015 (Valen et al., 2019). The total number of victims of fatal crashes has significantly decreased in the past years in Western countries thanks to efficient prevention. Yet, the use of medicinal or illicit drug and/or alcohol is an increasing phenomenon in Europe (Snenghi et al., 2018; Pelletti et al., 2019), and the percentage of fatal crashes due to the driver's impairment remain constant (between 17 and 22% from 1995 to 2017) in Switzerland [Office Fédéral de la Statistique (OFS), 2018].
Due to the large variety of drugs and pharmaceuticals with various psychoactive effects, there is a need for medical experts to establish solid statement on a potential driving-impairment and for official quantification of drugs and alcohol levels in blood (Martin et al., 2017). In Switzerland, a zero tolerance with technical cut-offs is implemented regarding classical drugs of abuse (DoA) toward drivers (1.5 ng/ml for THC and 15 ng/ml for morphine, cocaine, amphetamine, methamphetamines, MDEA, and MDMA) (Walsh et al., 2004; Steuer et al., 2016). The situation is more complex regarding the consumption of medicinal drugs and the toxicological interpretation of their concentration (Ravera et al., 2012). With respect to the law, the driving capability under pharmaceuticals is concomitantly determined by a “three pillars expertise” including police assessment, medical expertise, and toxicological analysis in blood, being the biological matrix of reference regarding toxicological interpretation (Steuer et al., 2014). The Swiss Federal Roads Office (FEDRO) defines a list of controlled substances that the laboratories must be able to quantify in the context of external quality controls (EQCs) in whole blood regarding driving under the influence of drugs (DUID). Those recommendations, associated with the knowledge of drug prevalence among suspected drivers, were used to establish a list of substances of interest in the present study.
Improvements regarding instrumentation, notably brought on by the developments of Orbitrap technology, offer new opportunities in terms of analytical strategies (Hoffman et al., 2018; Joye et al., 2019). Indeed, various Orbitrap-based parallel reaction monitoring (PRM) applications have been reported, especially in the field of proteomics (Domon and Gallien, 2015; Rauniyar, 2015; Bourmaud et al., 2016). In a PRM acquisition, a precursor selected by a quadrupole is fragmented in a higher-energy collisional dissociation (HCD) cell (Ronsein et al., 2015). Following this experiment, all product ions are simultaneously acquired in the high-resolution Orbitrap analyzer. Up to now, the use of triple-quadrupole (QQQ) using Selected Reaction Monitoring (SRM) has been the gold standard regarding targeted quantitative analyses (Hopfgartner et al., 2004; Rauniyar, 2015). However, SRM and PRM have comparable sensitivity with similar linearities, dynamic ranges, precision, and repeatability (Domon and Gallien, 2015; Joye et al., 2020). Yet Orbitrap-based PRM offers further advantages, since the acquisition of all selected precursors' fragments is performed, thereby limiting the a priori information required for method development. Indeed, the selection of quantifying ions is only necessary during the data processing step once the whole fragmentation spectra is acquired. Moreover, HRMS provides a higher specificity, allowing for the separation of the background ions from the targeted molecules (Ronsein et al., 2015).
Drug quantification can easily benefit from the PRM specificities that have been enlightened for proteomic applications. Even though this strategy is relatively recent regarding illicit drug and pharmaceuticals analyses in toxicology, it has received a growing interest. Indeed, PRM quantification has been reported for the quantitative analysis of abiraterone (Bhatnagar et al., 2018), beclabuvir (Jiang et al., 2017), anticoagulant rodenticides (Gao et al., 2018), and sterols (Schott et al., 2018). Regarding drugs of abuse, a first application has been described for the quantification of cannabinoids in whole blood (Joye et al., 2020).
Herein, we present a validated single multi-analyte procedure for the quantification of the main substances regarding DUID cases using 100 μL of whole blood. The quantified substances were selected based on the FEDRO list and the prevalence of substances consumed by the drivers in Switzerland (Augsburger and Rivier, 1997; Augsburger et al., 2005; Senna et al., 2010). The validated approach uses the advantages provided by HRMS and especially PRM for the simultaneous quantification of 22 DoA and pharmaceuticals alongside 15 internal standards (IS), enabling the solving of most DUID cases with a single injection and a simple sample preparation.
Materials and Methods
Standards and Reagents
Water, methanol, formic acid (FA), and ammonium formiate were furnished by Biosolve. Drugs standard were purchased from Cerilliant or Lipomed, at either 1 mg/ml or 100 μg/ml. External quality controls (ECQ) were purchased from Medidrug, ACQ Science, or Clincheck. Blank lyophilized whole blood was acquired from ACQ Science.
Solution Preparation
Standard solutions containing tetrahydrocannabinol (THC), 11-Nor-9-carboxy-THC (THC-COOH), alprazolam, amphetamine, methamphetamine, 3,4-methylendioxymethamphetamine (MDMA), 3,4-methylene dioxy-amphetamine (MDA), methylphenidate, cocaine, cocaethylene, lorazepam, bromazepam, zolpidem, benzoylecgonine, morphine, codeine, methadone, tramadol, O-desmethyltramadol, diazepam, nordiazepam, oxazepam were prepared for calibration curve and internal quality control (IQC) preparation. In parallel, solutions containing THC-D3, THC-COOH-D9, cocaine-D3, benzoylecgonine-D3, amphetamine-D8, MDMA-D5, methylphenidate-D10, morphine-D3, codeine-D3, methadone-D3, tramadol13C-D3, O-desmethyltramadol-D6, nordiazepam-D5, alprazolam-D5, and zolpidem-D6 were prepared as internal standard (IS) solutions.
Calibration samples were prepared by spiking lyophilized whole blood at 5 concentration levels (Table 1). IS were added to reach a final concentration of 10 (THC-D3), 100, or 1,000 ng/ml depending on the specific calibration range.
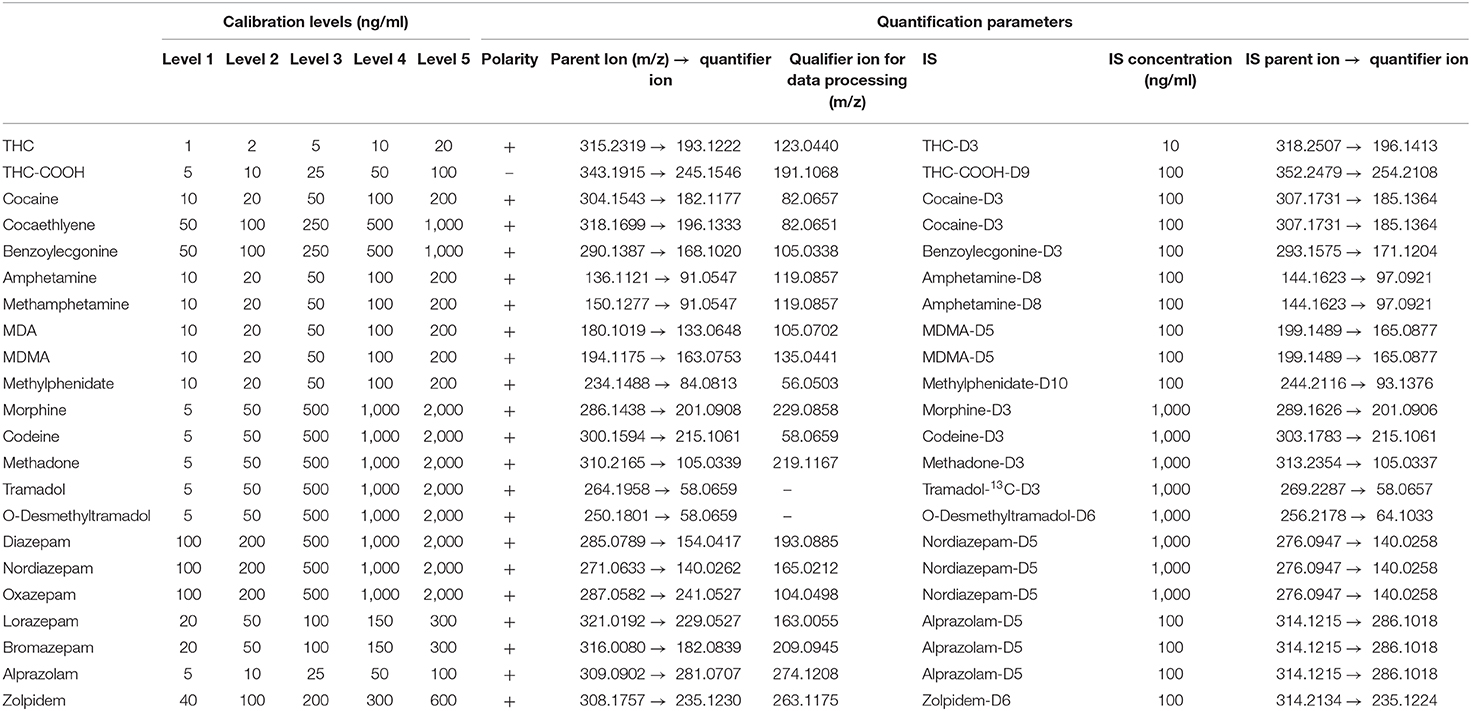
Table 1. Calibration levels and quantification parameters (IS, polarity, parent ion m/z, and quantifier ions) for the substances of interest. The calibration ranges are in adequacy with the legal thresholds and the therapeutic ranges.
Sample Pre-treatment
IS solutions were spiked in Eppendorfs and evaporated to dryness before adding 100 μL of whole blood. The extraction was then performed by protein precipitation using 300 μL of methanol. After centrifugation for 10 min at 14,000 rpm, the upper methanolic phase was transferred into a new Eppendorf and evaporated to dryness under a nitrogen flow. Reconstitution was performed using 100 μL of 1:9 methanol: water and 10 μL were injected into the LC-HRMS system (Supplemental Figure 1).
LC-HRMS Method
A Thermo Scientific Ultimate 3000 LC system with a Phenomenex 2.6 μm C18 (10 cm × 2.1 mm) maintained at 45°C was used for chromatographic separation. Mobile phases were composed of A, ammonium formate 10 mM at pH 3.3, and B, methanol with 0.1% FA. Phase B was ramped linearly from 2 to 98% over 7.5 min. The column was then washed at 98% of B for 3.5 min, followed by a 6 min re-equilibration at 2% of B at 300 μL/min for a total analysis run of 17 min. The LC was coupled to a Q Exactive Plus system (Thermo Scientific, Bremen, Germany) via a heated electro spray ionization (ESI) source (H-ESI II probe, Thermo Scientific). The ionization spray voltage was set to 3 kV, sheath gas flowrate was set to 40, and auxiliary gas flowrate to 10 (both in arbitrary units). The method functioned in PRM, using an inclusion list containing the exact mass of the parent ion and the retention time windows for the different analytes. A polarity switch in negative was performed at 7.5 min for the specific detection of THC-COOH with a switch back in positive polarity at 8.7 min for the detection of THC. Resolution was set to 17,500 for the HCD fragmentation performed using an NCE at 50 eV with an AGC target of 1e5 and a maximum IT of 100 ms.
Method Validation
The validation criteria used to evaluate the analytical process was based on the directives of the “Société Française des Sciences et des Techniques Pharmaceutiques” (SFSTP) regarding bioanalytical methods and adapted to our specific requirements (Boulanger et al., 2003; Peters et al., 2007; Lynch, 2016). Two product ions (one quantifier and one qualifier) were used for data processing (Table 1) and full MS/MS spectra were compared with the online advanced mass spectral database m/z cloud. The validation was performed over 3 non-consecutive days (p = 3). The trueness and precision were evaluated using a variance analysis-based statistical treatment (ANOVA). Calibration (Cal) was performed in duplicate at 5 different concentration levels (k = 5) (Table 1) while quality controls (QCs) were prepared in quadruplicate at the two lowest and highest concentration levels (k = 4). Using the acquired data, trueness, precision, accuracy, linearity, limits of detection (LOD), and quantification (LOQ) were determined. Six different blank bloods were analyzed for selectivity assessment investigating for potential interferences. The approach developed by Matuszweski et al. was used for recovery (RE) and matrix effect (ME) evaluation (Matuszewski et al., 2003). In this optic, three sample sets were prepared, including all the substances of interest at two concentration levels (low being level 2 and high being level 4 described in Table 1). Sample set 1 represented neat standards spiked after the extraction, while sample set 2 represented blank blood spiked after extraction. Sample set 3 represented blank blood spiked before extraction. The absence of interfering peaks at the established retention times (RT) for the analytes and the IS was used to ensure the specificity. The chemical stability of all analytes was evaluated under sample handling and storage conditions at low and high concentrations in five replicates. Benchtop (6 h, room temperature), autosampler (24 h, 5°C), three cycles of freeze-thaw (−20°C), and short term (1 week, −20°C) conditions were used for stability determination.
In order to evaluate the method, 8 different EQCs were analyzed in duplicates using the exact same procedure.
Results and Discussion
Method Development
In the present study, 22 analytes (15 IS) included in the main classes of drugs of abuse, as well as the major benzodiazepines, were analyzed using a single simultaneous multi-analyte quantitative approach. This list of substances was established based on the FEDRO recommendations and on the knowledge of the prevalence of psychoactive compounds among suspected impaired drivers. A nationwide study performed on 4,668 samples collected on suspected drivers in 2010 in Switzerland proved cannabinoids (48%), alcohol (35%), cocaine (25%), opiates (15%), amphetamines (7%), and benzodiazepines (6%) to be the most detected substances (Senna et al., 2010). The use of such multi-analyte approaches is challenging due to the various physico-chemical properties of the substances of interest and requires specific care during method development. To ensure a proper quantification, retention time windows were set for the acquisition ensuring the acquisition of a sufficient number of acquisition points (Figure 1). For good-quality integration and reproducible quantification, a minimum of 10–15 points is necessary to define exactly the peak start, peak apex, and peak end. The method was designed to resolve the wide majority of DUID cases using a single procedure and a limited amount of biological sample (Supplemental Figure 1) (Senna et al., 2010). The method allows the successful PRM-based quantification of cannabinoids, amphetamines, cocaine and its metabolites, opiates and opioids, and the major benzodiazepines at the sensitivity necessary for legal thresholds and therapeutic ranges (OOCCR-OFROU, 2008; Schulz et al., 2012).
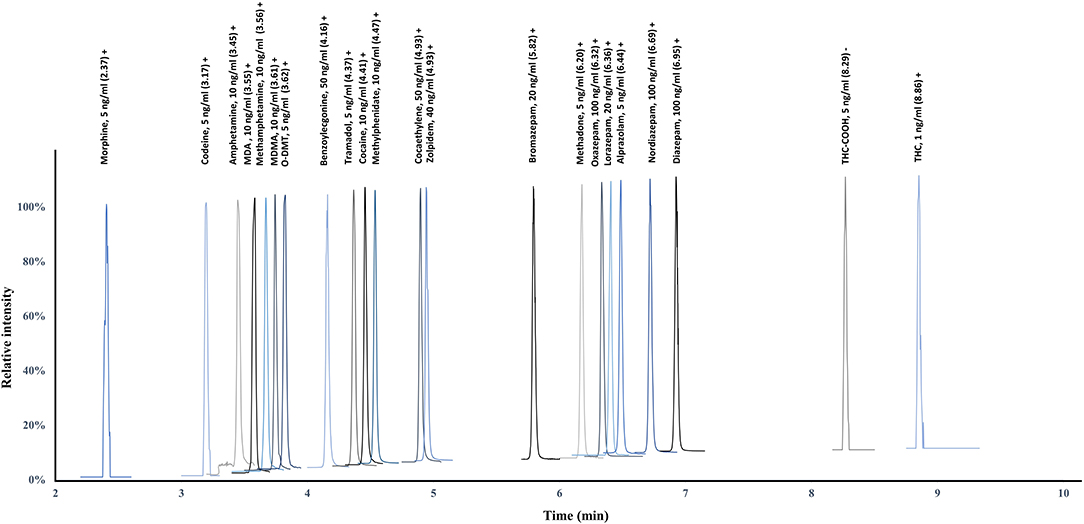
Figure 1. Chromatographic separation at LOQ (calibration level 1) using retention time windows to ensure a sufficient number of acquisition points for proper quantification.
Trueness and Precision
Independent QC samples at 4 different calibration levels were injected in 4 replicates over 3 non-consecutive days for the determination of trueness and precision. Accuracy represents the total error and is divided into trueness (representing the “bias” or the systematic error) and precision (referring as the standard deviation or random errors) (Gonzalez et al., 2010). The trueness can be evaluated by calculating the percentage difference between the experimental and the expected theoretical values. In the present study, the systematic error varied from −4.7 to 7.6% (Table 2). Precision was divided into two parameters: the relative standard deviation (repeatability or RR.S.D.) and the inter-day variability (intermediate precision or IPR.S.D.). RR.S.D. represents the variability under similar conditions, meaning that the analyses are performed by the same operator using the same reagents and samples. On the other hand, IPR.S.D. represented the variability associated with the use of the same samples on different days with different reagents. Precision parameters were evaluated to be between 1.1 and 11.6% (Table 2). Accuracy profiles are visual representations combining both the trueness and the precision to represent the uncertainty measurement (Figure 2). Precision is represented by the calculated confidence limit at 95% at each concentration level. Accuracy profiles also include the representation of acceptance limits of ±20% at the LLOQ suggested for method validation (±15% at the other calibration levels). All analyzed QCs were within the acceptance limits.
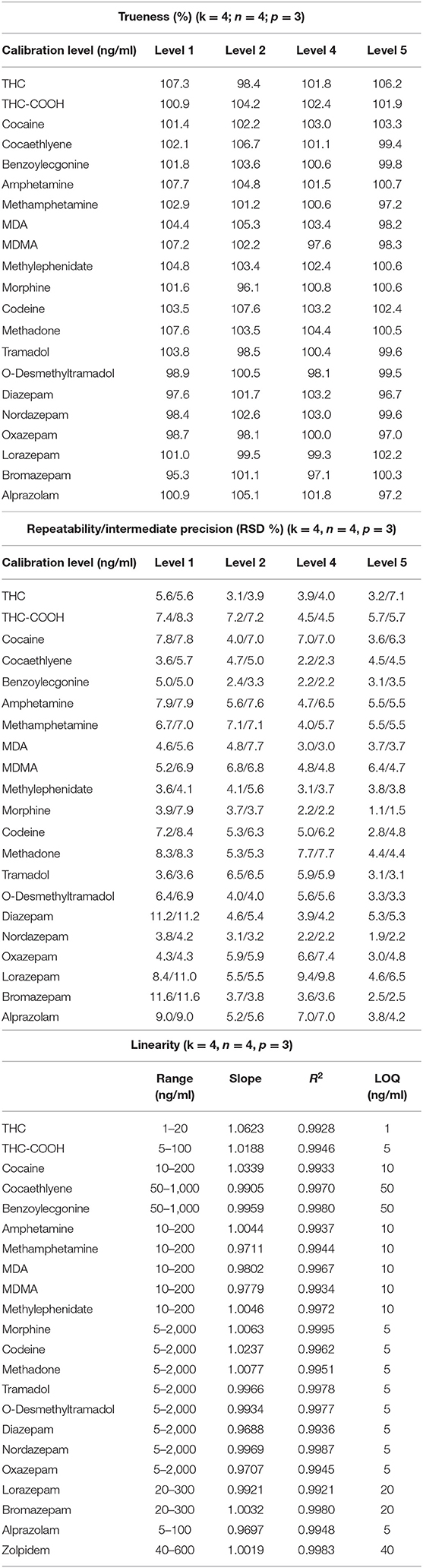
Table 2. Results for trueness, precision, and linearity (k is the number of concentration levels, n the number of repetitions by levels, and p the number of non-consecutive days).
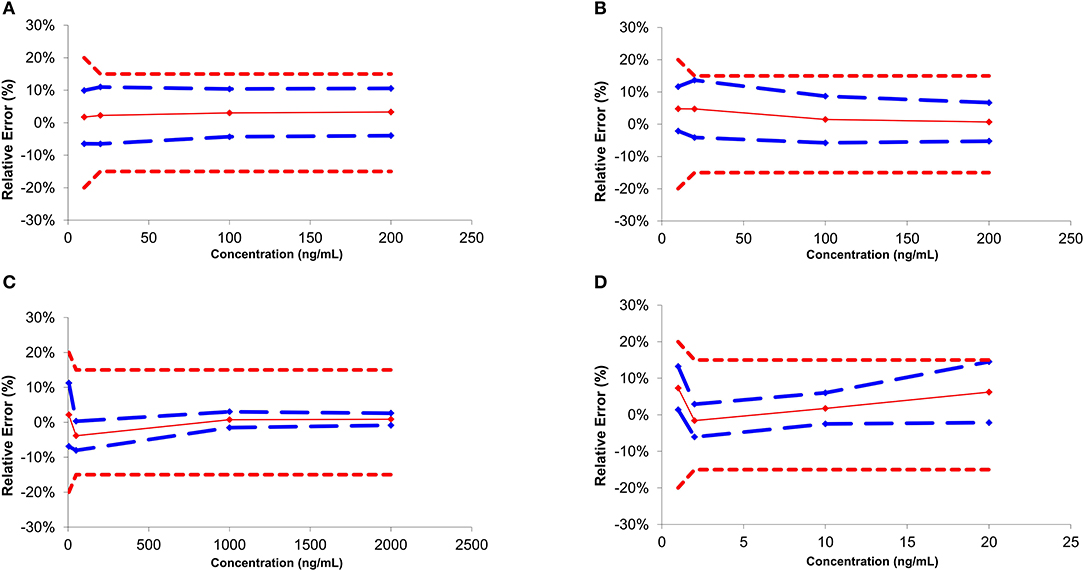
Figure 2. Accuracy profile for the main classes of drugs of abuse regulated by FEDRO [Cocaine (A), Amphetamine (B), Morphine (C), and THC (D)].
Linearity and LOQ
The definition of linearity stands as the method capacity to provide a result proportional to the actual sample concentration. To determine this parameter, a linear regression model based on the least square method was applied on the fit of the obtained concentration as a function of the theorical concentration. Slopes values were comprised between 0.9688 and 1.0623 with coefficients of determination above 0.9921 for all the compounds confirming the method linearity within the concentration ranges of interest (Table 2). LOQs were fixed according to the lowest point of the calibration curve (Table 1).
Selectivity, Recovery, and Matrix Effect
Selectivity is defined as the ability to differentiate the analyte of interest from potential interferences. To assess the good selectivity of the method, six different blank blood samples were analyzed using the complete extraction procedure. No compounds impairing the detection and quantification of the analytes of interest were observed. HRMS technology offers a high selectivity due to its resolving power, therefore reducing the number of potential interferences (Chindarkar et al., 2014). However, ME, including ion suppression or enhancement, are often associated with the use of ESI as ion source challenging the method selectivity. The determination of such ME is therefore crucial to ensure a proper detection and quantification of the substances of interest. ME ranged from −51% (15% CV) of ion suppression for THC at low concentration and 3% (13% CV) of ion enhancement for lorazepam, being consistent with the existing literature (Table 3) (Simonsen et al., 2010; Fernandez Mdel et al., 2013; Montenarh et al., 2014; Steuer et al., 2014; Vaiano et al., 2016; De Boeck et al., 2017). All values concerning RE and ME are summarized in Table 3. To compensate for those undesirable ME, isotopically-labeled internal standards was used for normalization.
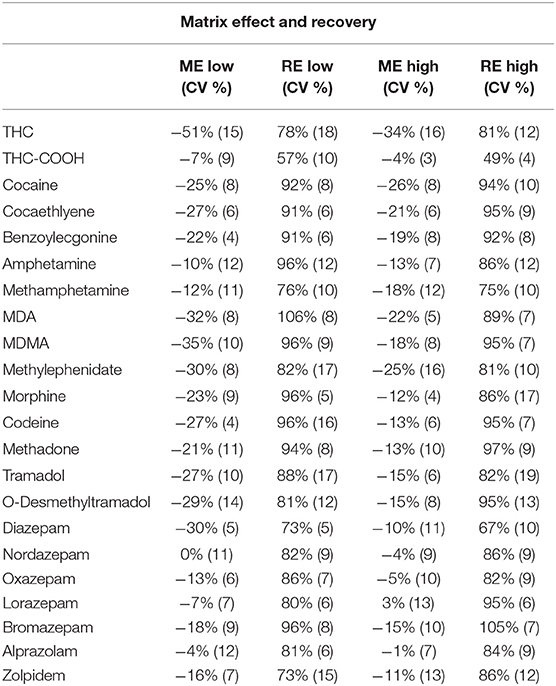
Table 3. Results for Recovery and Matrix Effect performed at low- and high-quality control concentrations.
Stability
Results regarding analytes' stability are listed in Table 4. Overall, stability ranged between 86 and 115%, assuring that the samples were stables within the tested conditions (auto-sampler, bench-top, 3 cycles of freeze-thaw and short-term stability.
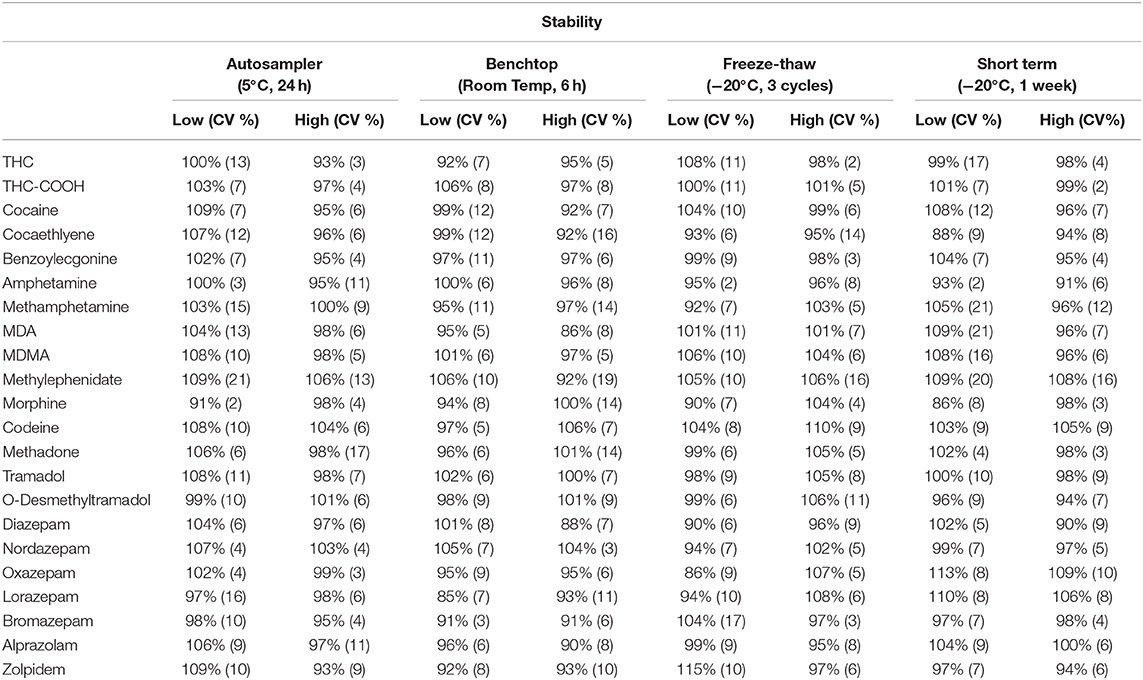
Table 4. Three cycles of freeze-thaw (−20°C), benchtop (6 h, room temperature), autosampler (24 h, 5°C), and short-term (1 week, −20°C) conditions were performed in this stability assay at low- and high-quality control concentrations.
External Quality Control Analysis
Eight commercial EQCs were analyzed in duplicates to ensure the robustness of the developed method and procedure. In total, the quantification was performed on 28 samples of amphetamines, 24 samples of cocaine and its metabolites, 16 samples of cannabinoids, 36 samples of opioids and opiates, and 28 samples of benzodiazepines and z-drugs. Results comparison between the described method and the expected EQCs values are represented in Figure 3 for the different classes of molecules involved in DUID cases. A good correlation was observed between the expected and the obtained values. The relative standard deviation was lower than 20% for all tested substances, confirming the efficiency of the PRM quantitative acquisition mode for toxicological analyses. This method confirms the potential of PRM as a solid alternative to classical MRM approaches (Li et al., 2016; Lv et al., 2018; Joye et al., 2020).
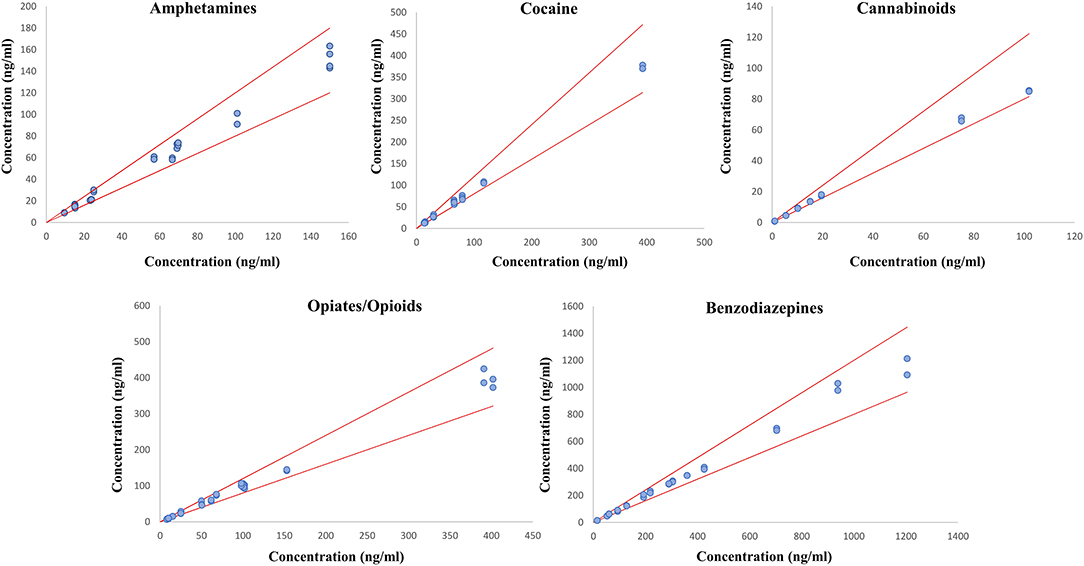
Figure 3. Method evaluation by comparing measured values and targeted commercial EQCs values. PRM measured concentrations are plotted as a function of the expected EQCs concentrations. The red lines represent the ±20% tolerance limits.
Conclusion
A quick and efficient multi-analyte procedure was successfully developed in whole blood for the simultaneous quantification of 37 substances of interest in DUID cases. PRM represents an interesting alternative to classical MRM quantitative analyses, with the capability of precisely quantifying a large panel of substances with similar performance in terms of linearity, dynamic range, precision, and repeatability (Rauniyar, 2015). PRM quantification does not require a priori selection of the fragments of interest, leading to a simplified method development and better control over the quantification experiment, especially regarding multi-analyte approaches. The quantitative PRM procedure presented herein benefits the increased selectivity and sensitivity brought by HRMS, offering a clear alternative for quantitative toxicological analyses.
Data Availability Statement
The raw data supporting the conclusions of this article will be made available by the authors, without undue reservation.
Author Contributions
TJ supervised the project, participated in the design of the study, did several analyses, and wrote the manuscript. KR did most of the analytical work and participated to the reflexion toward the project. JD paritcipated in the strategic choices toward the study and its design and critically revised the manuscript. JS participated in the supervision and design of the project and critically revised the manuscript. BF participated in the design of the study and critically revised the manuscript. MA participated in the strategic choices toward the study and its design and critically revised the manuscript. AT is the main contributor to the study design, supervised the project, and critically revised the manuscript. All authors contributed to the article and approved the submitted version.
Funding
The authors acknowledge financial support from the Swiss Federal Office of Public Health (contract no. 14.013792.204.0001/1300).
Conflict of Interest
The authors declare that the research was conducted in the absence of any commercial or financial relationships that could be construed as a potential conflict of interest.
Supplementary Material
The Supplementary Material for this article can be found online at: https://www.frontiersin.org/articles/10.3389/fchem.2020.00626/full#supplementary-material
References
Augsburger, M., Donze, N., Menetrey, A., Brossard, C., Sporkert, F., Giroud, C., et al. (2005). Concentration of drugs in blood of suspected impaired drivers. Forensic Sci. Int. 153, 11–15. doi: 10.1016/j.forsciint.2005.04.025
Augsburger, M., and Rivier, L. (1997). Drugs and alcohol among suspected impaired drivers in Canton de Vaud (Switzerland). Forensic Sci. Int. 85, 95–104. doi: 10.1016/S0379-0738(96)02084-1
Bhatnagar, A., McKay, M. J., Crumbaker, M., Ahire, K., Karuso, P., Gurney, H., et al. (2018). Quantitation of the anticancer drug abiraterone and its metabolite Delta(4)-abiraterone in human plasma using high-resolution mass spectrometry. J. Pharm. Biomed. Anal. 154, 66–74. doi: 10.1016/j.jpba.2018.03.012
Boulanger, B., Chiap, P., Dewe, W., Crommen, J., and Hubert, P. (2003). An analysis of the SFSTP guide on validation of chromatographic bioanalytical methods: progress and limitations. J. Pharm. Biomed. Anal. 32, 753–765. doi: 10.1016/S0731-7085(03)00182-1
Bourmaud, A., Gallien, S., and Domon, B. (2016). Parallel reaction monitoring using quadrupole-Orbitrap mass spectrometer: principle and applications. Proteomics 16, 2146–2159. doi: 10.1002/pmic.201500543
Chindarkar, N. S., Wakefield, M. R., Stone, J. A., and Fitzgerald, R. L. (2014). Liquid chromatography high-resolution TOF analysis: investigation of MSE for broad-spectrum drug screening. Clin. Chem. 60, 1115–1125. doi: 10.1373/clinchem.2014.222976
De Boeck, M., Missotten, S., Dehaen, W., Tytgat, J., and Cuypers, E. (2017). Development and validation of a fast ionic liquid-based dispersive liquid-liquid microextraction procedure combined with LC-MS/MS analysis for the quantification of benzodiazepines and benzodiazepine-like hypnotics in whole blood. Forensic Sci. Int. 274, 44–54. doi: 10.1016/j.forsciint.2016.12.026
Domon, B., and Gallien, S. (2015). Recent advances in targeted proteomics for clinical applications. Proteomics Clin. Appl. 9, 423–431. doi: 10.1002/prca.201400136
Elliott, S., Woolacott, H., and Braithwaite, R. (2009). The prevalence of drugs and alcohol found in road traffic fatalities: a comparative study of victims. Sci. Justice 49, 19–23. doi: 10.1016/j.scijus.2008.06.001
Favretto, D., Visentin, S., Stocchero, G., Vogliardi, S., Snenghi, R., and Montisci, M. (2018). Driving under the influence of drugs: prevalence in road traffic accidents in Italy and considerations on per se limits legislation. Traffic Inj. Prev. 19, 786–793. doi: 10.1080/15389588.2018.1500018
Fernandez Mdel, M., Wille, S. M., Kummer, N., Di Fazio, V., Ruyssinckx, E., and Samyn, N. (2013). Quantitative analysis of 26 opioids, cocaine, and their metabolites in human blood by ultra performance liquid chromatography-tandem mass spectrometry. Ther. Drug Monit. 35, 510–521. doi: 10.1097/FTD.0b013e31828e7e6b
Gao, X., Li, H., Li, H., Dong, S., Chu, J., Guo, H., et al. (2018). Sensitive determination of nine anticoagulant rodenticides in blood by high resolution mass spectrometry with supported liquid extraction pretreatment. Forensic Sci. Int. 292, 39–44. doi: 10.1016/j.forsciint.2018.09.009
Gonzalez, A. G., Herrador, M. A., and Asuero, A. G. (2010). Intra-laboratory assessment of method accuracy (trueness and precision) by using validation standards. Talanta 82, 1995–1998. doi: 10.1016/j.talanta.2010.07.071
Hoffman, M. A., Fang, B., Haura, E. B., Rix, U., and Koomen, J. M. (2018). Comparison of quantitative mass spectrometry platforms for monitoring kinase ATP probe uptake in lung cancer. J. Proteome Res. 17, 63–75. doi: 10.1021/acs.jproteome.7b00329
Hopfgartner, G., Varesio, E., Tschappat, V., Grivet, C., Bourgogne, E., and Leuthold, L. A. (2004). Triple quadrupole linear ion trap mass spectrometer for the analysis of small molecules and macromolecules. J. Mass Spectr. 39, 845–855. doi: 10.1002/jms.659
Jiang, H., Titsch, C., Zeng, J., Jones, B., Joyce, P., Gandhi, Y., et al. (2017). Overcoming interference with the detection of a stable isotopically labeled microtracer in the evaluation of beclabuvir absolute bioavailability using a concomitant microtracer approach. J. Pharm. Biomed. Anal. 143, 9–16. doi: 10.1016/j.jpba.2017.04.030
Joye, T., Sidibe, J., Deglon, J., Karmime, A., Sporkert, F., Widmer, C., et al. (2019). Liquid chromatography-high resolution mass spectrometry for broad-spectrum drug screening of dried blood spot as microsampling procedure. Anal. Chim. Acta 1063, 110–116. doi: 10.1016/j.aca.2019.02.011
Joye, T., Widmer, C., Favrat, B., Augsburger, M., and Thomas, A. (2020). Parallel reaction monitoring-based quantification of cannabinoids in whole blood. J. Anal. Toxicol. 44, 541–548. doi: 10.1093/jat/bkz113
Li, Y., Zhang, J., Jin, Y., Wang, L., Zhao, W., Zhang, W., et al. (2016). Hybrid quadrupole-orbitrap mass spectrometry analysis with accurate-mass database and parallel reaction monitoring for high-throughput screening and quantification of multi-xenobiotics in honey. J. Chromatogr. A 1429, 119–126. doi: 10.1016/j.chroma.2015.11.075
Liakoni, E., Yates, C., Dines, A. M., Dargan, P. I., Heyerdahl, F., Hovda, K. E., et al. (2018). Acute recreational drug toxicity: comparison of self-reports and results of immunoassay and additional analytical methods in a multicenter European case series. Medicine 97:e9784. doi: 10.1097/MD.0000000000009784
Lv, X. J., Sun, Z., Wang, P. L., Yang, J., Xu, T. Y., Jia, Q. Q., et al. (2018). Chemical profiling and quantification of Dan-Deng-Tong-Nao-capsule using ultra high performance liquid chromatography coupled with high resolution hybrid quadruple-orbitrap mass spectrometry. J. Pharm. Biomed. Anal. 148, 189–204. doi: 10.1016/j.jpba.2017.09.034
Lynch, K. L. (2016). CLSI C62-A: a new standard for clinical mass spectrometry. Clin. Chem. 62, 24–29. doi: 10.1373/clinchem.2015.238626
Martin, J. L., Gadegbeku, B., Wu, D., Viallon, V., and Laumon, B. (2017). Cannabis, alcohol and fatal road accidents. PLoS ONE 12:e0187320. doi: 10.1371/journal.pone.0187320
Matuszewski, B. K., Constanzer, M. L., and Chavez-Eng, C. M. (2003). Strategies for the assessment of matrix effect in quantitative bioanalytical methods based on HPLC-MS/MS. Anal. Chem. 75, 3019–3030. doi: 10.1021/ac020361s
Montenarh, D., Hopf, M., Maurer, H. H., Schmidt, P., and Ewald, A. H. (2014). Detection and quantification of benzodiazepines and Z-drugs in human whole blood, plasma, and serum samples as part of a comprehensive multi-analyte LC-MS/MS approach. Anal. Bioanal. Chem. 406, 803–818. doi: 10.1007/s00216-013-7513-x
OOCCR-OFROU (2008). Ordonnance de l'OFROU concernant l'ordonnance sur le contrôle de la circulation routière (art. 34). Available online at: https://www.admin.ch/opc/fr/classified-compilation/20080078/index.html#id-6-6 (accessed May 27, 2020).
Pelletti, G., Verstraete, A. G., Reyns, T., Barone, R., Rossi, F., Garagnani, M., et al. (2019). Prevalence of therapeutic drugs in blood of drivers involved in traffic crashes in the area of Bologna, Italy. Forensic Sci. Int. 302:109914. doi: 10.1016/j.forsciint.2019.109914
Peters, F. T., Drummer, O. H., and Musshoff, F. (2007). Validation of new methods. Forensic Sci. Int. 165, 216–224. doi: 10.1016/j.forsciint.2006.05.021
Rauniyar, N. (2015). Parallel reaction monitoring: a targeted experiment performed using high resolution and high mass accuracy mass spectrometry. Int. J. Mol. Sci. 16, 28566–28581. doi: 10.3390/ijms161226120
Ravera, S., Monteiro, S. P., de Gier, J. J., van der Linden, T., Gomez-Talegon, T., Alvarez, F. J., et al. (2012). A European approach to categorizing medicines for fitness to drive: outcomes of the DRUID project. Br. J. Clin. Pharmacol. 74, 920–931. doi: 10.1111/j.1365-2125.2012.04279.x
Ronsein, G. E., Pamir, N., von Haller, P. D., Kim, D. S., Oda, M. N., Jarvik, G. P., et al. (2015). Parallel reaction monitoring (PRM) and selected reaction monitoring (SRM) exhibit comparable linearity, dynamic range and precision for targeted quantitative HDL proteomics. J. Proteomics 113, 388–399. doi: 10.1016/j.jprot.2014.10.017
Schott, H. F., Krautbauer, S., Horing, M., Liebisch, G., and Matysik, S. (2018). A validated, fast method for quantification of sterols and gut microbiome derived 5alpha/beta-stanols in human feces by isotope dilution LC-high-resolution MS. Anal. Chem. 90, 8487–8494. doi: 10.1021/acs.analchem.8b01278
Schulz, M., Iwersen-Bergmann, S., Andresen, H., and Schmoldt, A. (2012). Therapeutic and toxic blood concentrations of nearly 1,000 drugs and other xenobiotics. Crit Care 16:R136. doi: 10.1186/cc11441
Senna, M. C., Augsburger, M., Aebi, B., Briellmann, T. A., Donze, N., Dubugnon, J. L., et al. (2010). First nationwide study on driving under the influence of drugs in Switzerland. Forensic Sci. Int. 198, 11–16. doi: 10.1016/j.forsciint.2010.02.014
Simonsen, K. W., Hermansson, S., Steentoft, A., and Linnet, K. (2010). A validated method for simultaneous screening and quantification of twenty-three benzodiazepines and metabolites plus zopiclone and zaleplone in whole blood by liquid-liquid extraction and ultra-performance liquid chromatography- tandem mass spectrometry. J. Anal. Toxicol. 34, 332–341. doi: 10.1093/jat/34.6.332
Snenghi, R., Pelletti, G., Frigo, A. C., Forza, G., Nalesso, A., Montisci, M., et al. (2018). The dangerous pattern of concurrent use of alcohol and cocaine among drunk-drivers of Northeast Italy. Alcohol Alcohol 53, 735–741. doi: 10.1093/alcalc/agy050
Steuer, A. E., Eisenbeiss, L., and Kraemer, T. (2016). Blood alcohol analysis alone versus comprehensive toxicological analysis - systematic investigation of missed co-ingested other drugs in suspected alcohol-impaired drivers. Forensic Sci. Int. 267, 52–59. doi: 10.1016/j.forsciint.2016.08.009
Steuer, A. E., Forss, A. M., Dally, A. M., and Kraemer, T. (2014). Method development and validation for simultaneous quantification of 15 drugs of abuse and prescription drugs and 7 of their metabolites in whole blood relevant in the context of driving under the influence of drugs–usefulness of multi-analyte calibration. Forensic Sci. Int. 244, 92–101. doi: 10.1016/j.forsciint.2014.08.022
Vaiano, F., Busardo, F. P., Palumbo, D., Kyriakou, C., Fioravanti, A., Catalani, V., et al. (2016). A novel screening method for 64 new psychoactive substances and 5 amphetamines in blood by LC-MS/MS and application to real cases. J. Pharm. Biomed. Anal. 129, 441–449. doi: 10.1016/j.jpba.2016.07.009
Valen, A., Bogstrand, S. T., Vindenes, V., Frost, J., Larsson, M., Holtan, A., et al. (2019). Driver-related risk factors of fatal road traffic crashes associated with alcohol or drug impairment. Accid. Anal. Prev. 131, 191–199. doi: 10.1016/j.aap.2019.06.014
Walsh, J. M., de Gier, J. J., Christopherson, A. S., and Verstraete, A. G. (2004). Drugs and driving. Traffic Inj. Prev. 5, 241–253. doi: 10.1080/15389580490465292
World Health Organization (2015). Global Status Report on Road Safety. Geneva: World Health Organization. Available online at: https://www.who.int/violence_injury_prevention/road_safety_status/2015/en/ (accessed May 27, 2020).
Keywords: parallel reaction monitoring, quantitative analysis, whole blood, driving under the influence of drugs, multi-analyte
Citation: Joye T, Rocher K, Déglon J, Sidibé J, Favrat B, Augsburger M and Thomas A (2020) Driving Under the Influence of Drugs: A Single Parallel Monitoring-Based Quantification Approach on Whole Blood. Front. Chem. 8:626. doi: 10.3389/fchem.2020.00626
Received: 15 April 2020; Accepted: 15 June 2020;
Published: 26 August 2020.
Edited by:
Ana de-Castro-Ríos, University of Santiago de Compostela, SpainReviewed by:
Sarah M. R. Wille, National Institute for Criminalistics and Criminology (NICC), BelgiumMarilyn A. Huestis, Thomas Jefferson University, United States
Copyright © 2020 Joye, Rocher, Déglon, Sidibé, Favrat, Augsburger and Thomas. This is an open-access article distributed under the terms of the Creative Commons Attribution License (CC BY). The use, distribution or reproduction in other forums is permitted, provided the original author(s) and the copyright owner(s) are credited and that the original publication in this journal is cited, in accordance with accepted academic practice. No use, distribution or reproduction is permitted which does not comply with these terms.
*Correspondence: Aurélien Thomas, YXVyZWxpZW4udGhvbWFzQGNodXYuY2g=