- 1QIMP Team, Center for Medical Physics and Biomedical Engineering, Medical University Vienna, Vienna, Austria
- 2Department of Radiology, Children's Hospital of Michigan, The Detroit Medical Center, Wayne State University School of Medicine, Detroit, MI, United States
- 3MR Center of Excellence, Medical University of Vienna, Vienna, Austria
State-of-the-art patient management frequently requires the use of non-invasive imaging methods to assess the anatomy, function or molecular-biological conditions of patients or study subjects. Such imaging methods can be singular, providing either anatomical or molecular information, or they can be combined, thus, providing “anato-metabolic” information. Hybrid imaging denotes image acquisitions on systems that physically combine complementary imaging modalities for an improved diagnostic accuracy and confidence as well as for increased patient comfort. The physical combination of formerly independent imaging modalities was driven by leading innovators in the field of clinical research and benefited from technological advances that permitted the operation of PET and MR in close physical proximity, for example. This review covers milestones of the development of various hybrid imaging systems for use in clinical practice and small-animal research. Special attention is given to technological advances that helped the adoption of hybrid imaging, as well as to introducing methodological concepts that benefit from the availability of complementary anatomical and biological information, such as new types of image reconstruction and data correction schemes. The ultimate goal of hybrid imaging is to provide useful, complementary and quantitative information during patient work-up. Hybrid imaging also opens the door to multi-parametric assessment of diseases, which will help us better understand the causes of various diseases that currently contribute to a large fraction of healthcare costs.
Introduction
Since the discovery of X-rays by Wilhelm Conrad Roentgen in 1895 [1], non-invasive medical imaging has become a standard tool for the diagnosis and staging of numerous diseases. X-ray Computed Tomography (CT) and Magnetic Resonance (MR), first introduced in the early [1] and late 1970s [2, 3], respectively, are the most widely used tomographic imaging techniques for depicting morphological changes of the human anatomy [4–6]. Metabolic or functional changes, which may occur without a corresponding change of anatomy, can be depicted by functional imaging, which has proven to provide essential information for the diagnosis and staging of many diseases. The first tomographic functional imaging modality was Single Photon Emission Tomography (SPECT), introduced in the early 1960s [7], followed be the first Positron Emission Tomography (PET) system in 1972 [8] and the first MR system in 1977 [3].
By combining anatomical and functional imaging within a single, hybrid imaging system, complementary diagnostic information can be obtained in order to gather a comprehensive picture of the disease. First attempts for obtaining such “anato-metabolic images” [9] were based on sophisticated software techniques to co-register structural and functional information [10, 11]. In the late 1990s, imaging systems that combine two complementary imaging techniques within the same gantry (e.g., PET/CT, SPECT/CT) became available [12, 13]. This approach is known as “hardware fusion”, in contrast to the software fusion approaches mentioned above.
This review briefly describes the developmental paths of hardware fusion systems and discusses their future in clinical routine and research. In section Basic Concepts of Hybrid Imaging we describe the basic concepts of clinical and preclinical hybrid imaging systems. Section Hybrid Imaging Technology highlights technological advances, such as novel detectors for PET, SPECT, and CT, time-of-flight PET and organ-specific or total-body PET systems. In section Data handling we discuss the data handling in hybrid imaging systems, including data acquisition, data storage, image reconstruction and data correction techniques. Section Joint Data Exploration focuses on the potential of joint data exploration, and section Multi-Center Standardization summarizes ongoing efforts regarding the standardization of hybrid imaging systems. Finally, a summary of the state-of-art and an outlook on future trends in hybrid imaging is presented in section Outlook and Future Trends.
Basic concepts of hybrid imaging
Among the range of existing functional imaging techniques (functional magnetic resonance imaging—fMRI, perfusion MR imaging, magnetic particle imaging—MPI, Near-infrared spectroscopy—NIRS, etc.), PET and SPECT present as very sensitive methods for the non-invasive and quantitative investigation of physiological processes at a molecular level. Nevertheless, PET and SPECT provide mostly functional information that may not always be directly associated with well-defined anatomical structures. The lack of high-contrast anatomical information in either SPECT or PET image data (independent of the radiotracer) is a major limitation of these imaging techniques. In order to maximize the potential of PET or SPECT, it has been recognized that either nuclear medicine modality could be combined with a high-resolution anatomical imaging modality. In that regard, PET/CT and PET/MR were developed with the aim of aligning functional and anatomical information to improve the clinical outcome of these studies, while SPECT/CT was conceived primarily for the purpose of providing routinely CT-based attenuation and scatter correction of SPECT data [14–16]. Nonetheless, the adoption of CT-based attenuation and scatter correction has proven to yield similar benefits for SPECT and PET alike; these include shorter transmission times as well as higher-quality data for post-injection transmission imaging [17].
Clinical SPECT/CT, PET/CT and PET/MR systems
Figure 1 shows representative designs for SPECT/CT, PET/CT, and PET/MR systems available on the market today, and Table 1 summarizes their most important technical specifications.
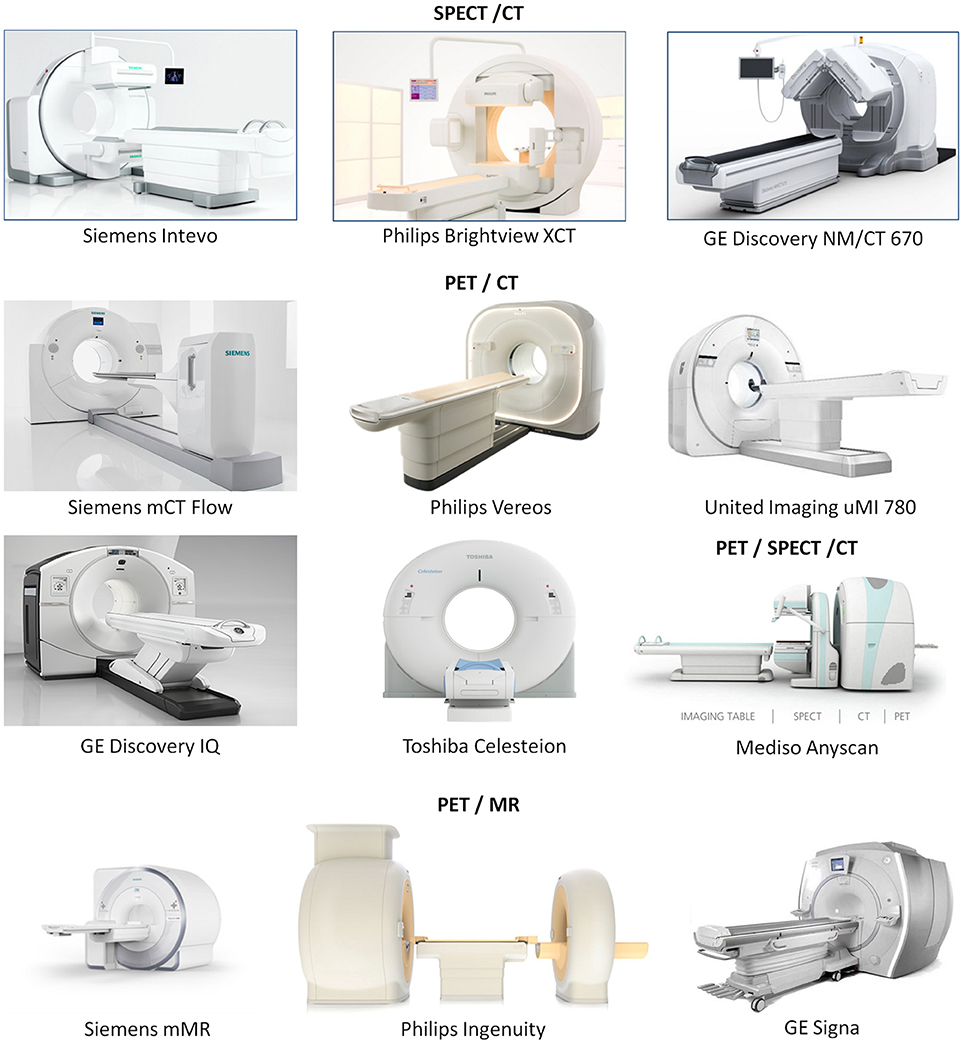
Figure 1. Images of commercially available hybrid imaging systems for clinical use. The figure shows three SPECT/CT, five PET/CT, three PET/MR and one triple modality SPECT/PET/CT systems. Systems include the first CTM-PET(/CT) system (Siemens mCT Flow, see section Organ-Specific System Design and Total-Body Systems); two SiPM-based PET systems (GE Signa, uMI 780), the first digital SiPM based PET system (Philips Vereos); a BGO-based system (GE Discovery IQ) and the first commercially available fully-integrated PET/MR (Siemens mMR). Images taken from vendor's web pages and published with the permission of the copyright holders (the respective vendors).
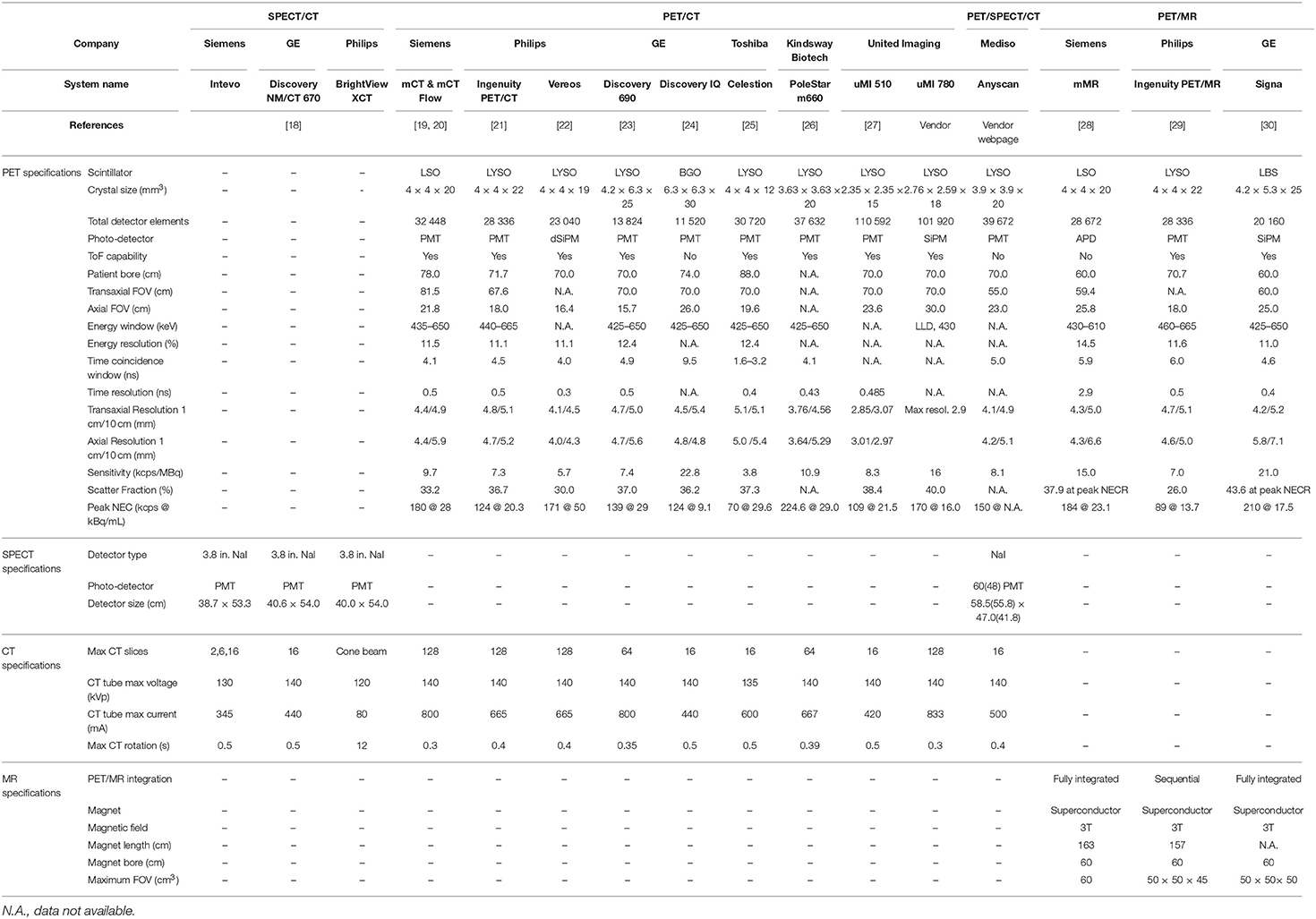
Table 1. PET, SPECT, CT, and MR specifications of selected dual and triple modality clinical systems commercially available.
SPECT/CT
The first combined SPECT/CT design was proposed by Mirshanov in 1987 [31]; however, it took a decade until SPECT/CT became commercially available following some key contributions by Blankespoor et al. [12]. Since then, SPECT/CT has advanced rapidly and several commercial system designs are available today.
Two facets of the design of the integrated CT components can be identified: first, SPECT/CT systems include fully-diagnostic CT systems with fast-rotation detectors that permit the simultaneous acquisition of 16 or 64 detector rows, while the X-ray tubes provide sufficient tube voltages together with high tube current and automatic exposure control (General Electric Discovery NM/CT 670 and the Siemens Symbia [32]). These systems also support advanced CT capabilities such as cardiac gating, calcium scoring and iterative reconstruction [18, 33].
On the other hand, SPECT/CT systems include rotating SPECT components that come with adapted rotation times and dose-optimized acquisition modes, while employing CT-type components with limited acquisition flexibility, such as lower tube voltages and currents (General Electric Hawkeye, Philips Brightview-XCT [32]). The Hungarian company Mediso, for example, offers a triple-modality SPECT/CT/PET system (Mediso AnyScan), which combines all three modalities within a single device (Figure 1).
Most clinical SPECT systems are based on planar detectors consisting of two-dimensional (2D) array of photomultiplier tubes (PMTs) attached to the back of the scintillation crystal with en-face collimators. The location of a photon interaction site is computed as the center-of-gravity of the position-dependent energy signals from the PMTs according to the so-called Anger logic [34]. In recent years, alternatives to the Anger logic have been proposed (see section Detector Technology for CT, SPECT, and PET). However, the comparably high costs involved, mainly from the use of solid state detectors, restricts adoption in smaller systems designed for special applications, such as cardiac [35], brain [36] or pre-clinical imaging [37].
The benefit of SPECT/CT has been proven for a wide range of clinical applications [38–41]. The main advantages of SPECT/CT include improved attenuation correction and accurate anatomical allocation of the SPECT/CT findings, both resulting in better diagnostic performance. Moreover, combined SPECT/CT imaging has demonstrated its value particularly in the clinical management of patients with cardiovascular disease [35].
PET/CT
The very first PET/CT prototype was proposed in 1984 at Gunma University in Japan [5]. The two tomographs were situated next to each other with the patient table moving sideways between the two units. The first whole-body PET/CT prototype was proposed by Townsend and colleagues in the late 1990's [13, 42]. Over the years, PET/CT designs from various medical imaging system vendors have been presented following the advances in CT and PET instrumentation. To date, several vendors worldwide offer a broad range of PET/CT designs.
The success of PET/CT imaging is based on several factors: first, an anatomical and functional whole-body survey in a single session is logistically efficient for both the patient and the healthcare provider. Second, due to the interplay of complementing data streams, the diagnostic information of a PET/CT scan is superior to that of PET or CT alone [43, 44]. A third advantage is the possibility to use CT information to correct PET data for photon attenuation and scatter or utilize it to correct for partial volume effects [16].
PET/MR
An interesting alternative to PET/CT is to combine PET with Magnetic Resonance Imaging (MR), since the range of MR examinations is complementary to that of PET. MR imaging reveals structural, functional and metabolic information through the interaction of three different magnetic fields (static, gradient, dynamic) with the protons present in the tissues [2, 3]. The wide variety of imaging sequences, along with better soft-tissue contrast compared to CT, renders MR an efficient diagnostic tool. The potential of reducing patient dose by employing MR in lieu of CT can be also considered an advantage compared to CT, mainly for pediatric examinations and cardiac imaging.
However, the physical combination of PET and MR represents a major technological challenge [45–48]. Conventional PET systems use PMTs to detect the scintillation light. These PMTs are highly sensitive to magnetic fields and, therefore, cannot be operated inside an MR magnet. One possible approach to overcome this issue is to spatially separate the PET and the MR system in combination with an active shielding of the PMTs against the magnetic field from the MR. This design was proposed in 2010 for the first whole-body PET/MR, the Philips Ingenuity TF PET/MR system [29]. Alternative approaches make use of the potential of solid state photo-detectors, such as APDs or SiPMs [49–52], see section Detector Technology for CT, SPECT, and PET for details. This technology enables the design of fully integrated PET/MR systems that permit the simultaneous acquisition of PET and MR data within the same axial field-of-view (aka co-planar FOV). Today, two integrated systems are available commercially: the Siemens Biograph mMR [28] and the GE Signa PET/MR [30].
Preclinical hybrid imaging
Animal models of human disease are the basis of many research efforts to understand disease processes and the development of new pharmaceuticals [53]. Similar to human imaging, the combination of molecular imaging techniques and CT or MR has been proven beneficial for small-animal imaging [54]. Therefore, a large variety of dedicated small-animal hybrid systems, has been developed since the 1990s, with all hybrid combinations (PET/CT, PET/MR, SPECT/CT, SPECT/MR, and PET/SPECT/MR) commercially available. Figure 2 shows a number of dual- and triple-modality systems for small animal imaging that are available from several vendors. Table 2 summarizes the main system specifications. A complete description of all the available preclinical hybrid systems is outside the focus of this review paper, please see [46, 61–65] for details.
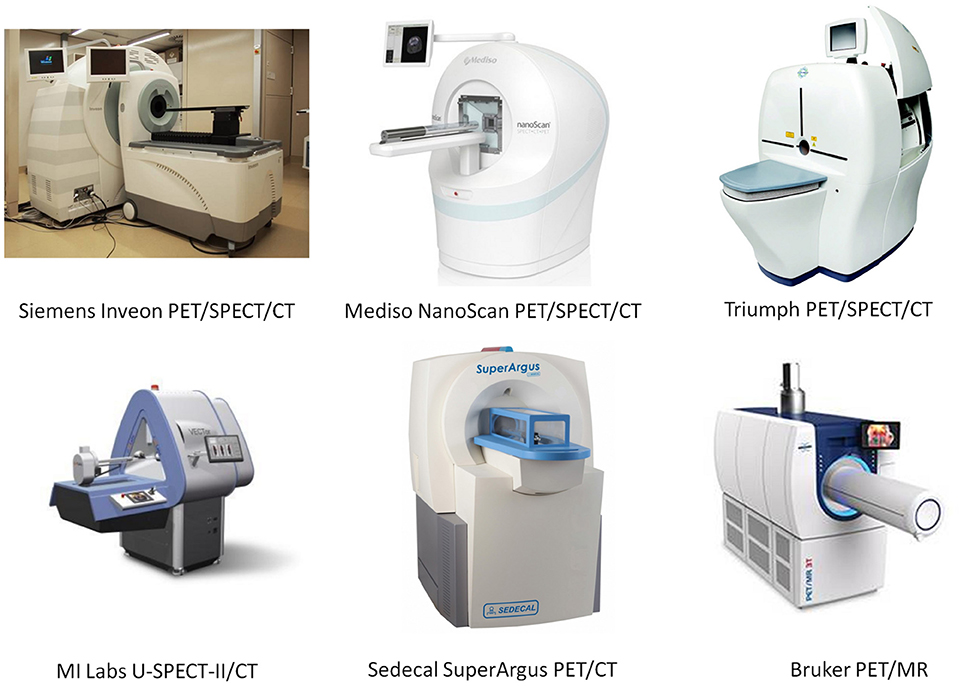
Figure 2. Selected commercially available, dual- and triple-modality preclinical systems. Images taken from vendor's web pages and published with the permission of the copyright holders (the respective vendors).
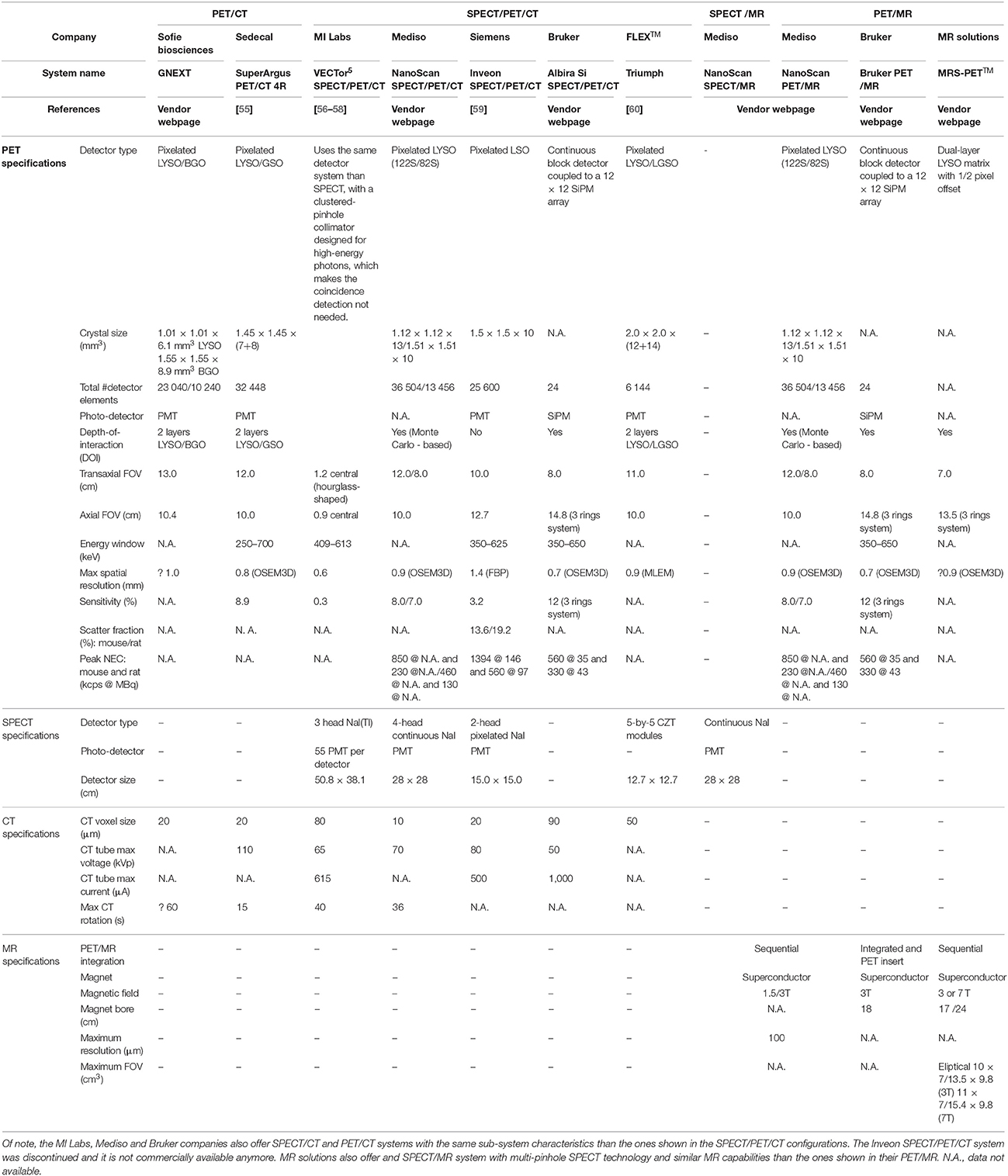
Table 2. PET, SPECT, CT and MR specifications of selected dual and triple modality preclinical systems.
Quantification in PET and SPECT
One of the most important advantages of tomographic nuclear imaging techniques is the ability to accurately quantify the amount of radio-labeled biomolecules (radiotracers) in vivo. In PET, this ability is based on the properties of the positron emission coincidence detection, which allows for correction of photon attenuation in the emission signal by using information gained from a separate transmission measurement [66]. As a result, emission images represent tracer concentration in units of Bq/mL. However, for the assessment of functional processes, the sole knowledge of tracer concentration is not sufficient. The tracer concentration in, for example, an organ depends on the amount of available radiotracer, which has led to the introduction of the standardized uptake value (SUV) in the clinical reports. The SUV is a semi-quantitative measure that normalizes the measured tracer concentration in tissue to a surrogate of the available tracer concentration in arterial blood / plasma. The most commonly used surrogate is the ratio of injected radiotracer to patient weight. The basic expression for SUV is [67]:
where AMeasured is the measured activity decay corrected to the time of injection (kBq/mL). AInjected is the activity injected into the patient (kBq/mL) and BW is the body weight of the patient (kg).
As of today, SUV remains a standard metric for PET-based diagnosis and therapy response assessment in oncology [68, 69]. However, the SUV represents only a simplified measure to describe a physiological process. For absolute quantification, i.e., the determination of physiological parameters (such as metabolic rate of glucose consumption in mmol/g/min), pharmaco-kinetic modeling is required [68]; this entails the knowledge of the time course of tracer concentration in arterial blood as well as in the tissue. From that, the pharmaco-kinetic parameters can be derived from a temporal relationship between these functions [70]. However, both the requirement of invasive arterial blood sampling and the complexity of kinetic modeling are significant limitations for the routine adoption of absolute quantification in clinical routine so far [71].
In contrast to PET imaging, and given the more challenging approaches toward attenuation and scatter correction, SPECT has not been considered a quantitative modality until recently. However, methodological advances have led to an increase in the number of papers reporting high quantitative accuracy with SPECT [18, 72].
Hybrid imaging technology
This section reviews the basic constituent technologies for hybrid imaging systems. We start with a discussion on radiation detectors used in CT, SPECT, and PET systems (section Detector Technology for CT, SPECT, and PET). Time-of-flight and continuous table motion PET are discussed in sections Time-of-Flight PET and Spiral PET, Continuous Table Motion, respectively, while in section Organ-Specific System Design and Total-Body Systems we discuss organ-specific and total body hybrid imaging systems. Finally, in section MR Technology we provide a brief overview of the technology used in MR systems.
Detector technology for CT, SPECT, and PET
The most commonly used radiation detectors for CT, SPECT, and PET imaging systems are based on scintillator crystals, because they are fast, they provide a high stopping power for photons across a range of emission energies and their cost-per-volume rate is far superior to other detector materials. A scintillation detector consist of a crystal that produces scintillation light after the interaction with radiation and a photo-detector that converts the scintillation light into an electrical signal [73], which is then processed by the electronic system.
There are several scintillating materials that are currently being used. They can be classified into organic or inorganic scintillators that come in solid, liquid or gaseous state. For medical imaging applications, solid inorganic scintillators are preferred, due to their higher density (i.e., stopping power), which is required for high sensitivity imaging systems. They are also characterized by the highest light output (i.e., number of photons emitted per unit of deposited energy), which is directly related to the energy resolution. Most PET and SPECT systems are composed of pixelated scintillators to assign the interaction position of the gamma photon [74], although blocks made of continuous crystal have been proposed as well [75, 76]. Table 3 lists some of the scintillators commonly used in CT, SPECT and PET detector systems together with their key properties [77–81]. New scintillator materials are being developed continuously. Some examples include scintillators based on cerium doping of lanthanide and transition metal elements, such as LuAP:Ce, CeBr3, LuBO3:Ce, and others based on lead (Pb), tungsten (W), and gadolinium (Gd) [82].
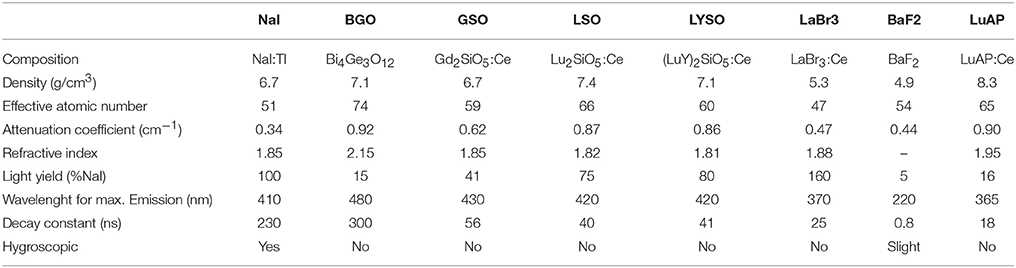
Table 3. Physical properties of some scintillators used in medical imaging applications (adapted from Lewellen [77]).
Photomultiplier tubes (PMTs) represent the most common mean to measure and detect the scintillation light emitted by the scintillator crystal. They consist of a vacuum enclosure within a thin photocathode layer at the entrance window and several electrodes, called dynodes, which amplify the electrical signal created in the photocathode by means of secondary electron emissions. A PMT produces an electric pulse with amplitude proportional to the number of scintillation photons that reach the photocathode, which, in turn, is proportional to the deposited energy.
The main advantage of PMTs as scintillation light detector is its high amplification capability (in the order to 106–107). On the other hand, their most significant drawback of PMTs is their sensitivity to magnetic fields, which makes them not suitable for use in combined PET/MR or SPECT/MR systems [83]. A valid alternative to PMTs are solid-state detectors such as Avalanche Photodiodes (APDs) or Silicon Photomultipliers (SiPMs). Solid-state detectors have several inherent advantages over PMTs (Table 4), such as high quantum efficiency, compact and flexible shape, ruggedness, demonstrated insensitivity to magnetic fields up to 9.4 T and potentially inexpensive mass production [49–51]. Solid-state detectors are semiconductor devices with a low-field depleted region where visible or near-UV photons can create electron-hole pairs by photoelectric effect. APDs exist as small discrete devices or as monolithic arrays, which can be used for individual or multiplexed crystal readouts. Silicon Photomultipliers (SiPMs) consists of a densely packed matrix of small APD cells biased to be operated above avalanche breakdown in the so-called Geiger mode. Since the Geiger-mode operation yields a high gain (105–106), a multi-cell structure can provide a proportional output for moderate photon flux by summing the signal of all cells that have been activated. In 2009, Philips Healthcare introduced the digital SiPMs, also known as Digital Photon Counter (DPC), which combines a conventional array of Geiger-mode photodiodes with a fully-digital electronic readout system, thus, allowing timing resolutions of up to 60 ps Full Width Half Maximum (FWHM) [84–87].
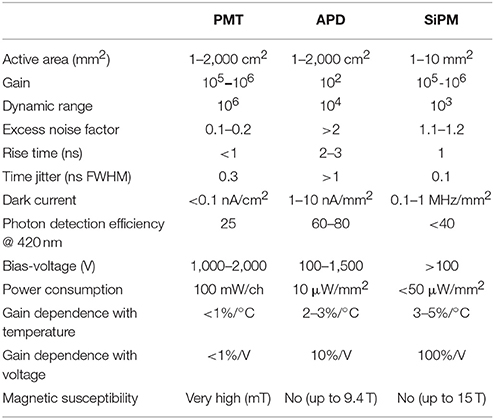
Table 4. Characteristics of photo-detectors used in medical imaging applications (adapted from Lecomte [79]).
Semiconductor detectors represent the main alternative to scintillator-based detectors in CT and SPECT imaging systems. In comparison to scintillators the main advantage of semiconductor detectors is the direct conversion from radiation to an electric pulse, which avoids the degrading effects associated with scintillation light production, propagation and conversion to an electrical signal in the photodetector. Their use in PET systems is much less frequent, due to their lower stopping power for the high-energy 511 keV annihilation photons and their higher costs.
To date, the most widely investigated semiconductors for nuclear medicine and CT imaging are CdTe and CdZnTe (CZT). Both offer a relatively high stopping power (similar to the one offered by NaI:Tl scintillator crystals) and they can be operated at room temperature. Furthermore, with the use of highly granulated detectors high spatial, energy and time resolution can be achieved [88]. Table 5 summarizes important properties of some semiconductor materials used for medical imaging applications [80].
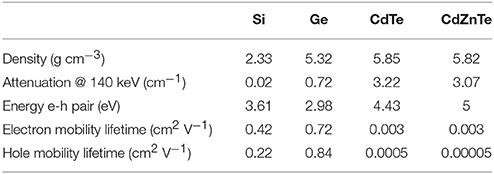
Table 5. Physical properties of some semiconductor detectors used in medical imaging applications (adapted from Peterson and Furenlid [80]).
Time-of-flight PET
Another important technological advance for PET systems, referred to as Time-of-Flight (ToF) PET, was made possible with the introduction of very fast detectors. The concept of ToF assumes that the annihilation point of two photons originating from a single positron annihilation can be calculated from their travel time differences [89–91].
State-of-the-art PET systems achieve time resolutions of about 500 ps FWHM (using photo multiplier tubes) [29, 19], down to about 300 ps FWHM (using SiPMs) [30, 22], which corresponds to an uncertainty of determining the origin of the annihilation along the Line Of Response (LOR) of about 15 and 9 cm, respectively. With this additional information, the SNR in the reconstructed PET images can be improved significantly [92]. Thanks to recent improvements in detector technology, new scintillator materials and fast digital SiPMs photodetectors [93], ToF resolution can be significantly improved; the latest developments in PET detector technology aim at timing resolutions of 100 ps FWHM, or less [92, 94].
Spiral PET, continuous table motion
Commercially available whole-body PET systems offer fixed axial FOVs between 15 cm and 25 cm. To cover larger axial areas, multiple bed positions are acquired in a step-and-shoot protocol [95]. An alternative to the traditional step-and-shoot approach is a continuous table motion (CTM) during the acquisition. This technique was first proposed in 1992 by Dahlbom et al. [95] aiming to increase the uniformity of the sensitivity across the examined axial area. First attempts to implement CTM in a clinical PET only system were made as early as 2001 [96] and followed by several implementations of CTM techniques in PET/CT systems a few years later [97, 98]. However, it took until 2013 to implement CTM into a commercially available system [19].
CTM offers two main advantages in comparison to step-and-shoot acquisitions: a uniform sensitivity across the entire axial FOV and the possibility to customize the axial scan range. While in step-and shoot acquisitions uniform sensitivity can also be achieved by an appropriate selection of bed overlap, the axial scan range is restricted to a discrete number of bed positions. Another advantage of CTM acquisitions is the possibility of new acquisition protocols that support whole-body parametric imaging [99]. Given the above benefits, CTM can be regarded a particular asset in combined PET/CT imaging.
Organ-specific system design and total-body systems
The development of state-of-the-art detectors allows the design of high-resolution organ-specific hybrid systems, as well as high-sensitivity total body PET/CT with extended axial field-of-view (aFOV).
Although most attempts toward organ-specific imaging were based on stand-alone PET or SPECT systems [100–103], significant efforts in fully-integrated, organ-specific imaging technologies were made in recent years. For example, Siemens Healthcare did propose a “Brain PET” system that was based on a PET ring insert for a 3 T MR system [104]. More recently, a SiPM-based PET insert has been developed for integration with any MR scanner [105]. Alternative efforts aim at developing MR-compatible PET inserts for breast imaging. Initial prototypes have been proposed [106] with promising results, and a breast hybrid PET/RF insert is being developed based on digital SiPMs (dSiPMs) for enhanced diagnosis of breast cancer (HYPMED 2016: http://www.hypmed.eu/). Similar approaches have been proposed for the diagnosis of prostate and pancreatic cancer, whereby PET and Ultrasound (US) components are combined in an endoscope device [107].
The concept of a large axial FOV (aFOV) whole-body PET was introduced by Crosetto in the 1990's [108]. Following the introduction of this concept and after several simulation studies, the EXPLORER (Extreme Performance Long Research scanner) consortium was set up with the task of building the world's first total-body PET/CT system—the EXPLORER PET/CT [109, 110]. The EXPLORER has a 200 cm aFOV based on mainstream PET detector technology. It will consist of a total of 400,000 crystals, thus, yielding about 100 times more LORs than a state-of-the-art whole-body PET(/CT) system.
The elongated axial coverage of the EXPLORER type system is expected to yield a 30–40 times increase in sensitivity over current generation PET systems. This translates to detecting over 40% of the counts emitted from a point source in air located at the center of the FOV [111]. This impressive increase in sensitivity provides a number of key advantages: imaging at very low radioactivity levels (~10 MBq) [112], increased throughput from shorter examination times and whole-body imaging that can be performed within a single breath hold. With the total-body coverage provided by the EXPLORER, it is also possible to perform whole-body dynamic PET imaging, thus, avoiding temporal gaps, which are present in current multi-bed, multi-pass imaging protocols [113, 114]. However, efficient image reconstruction and data handling schemes are required for the EXPLORER system. Zhang et al. [112] proposed a quantitative image reconstruction method that demonstrated a 7-fold increase in the signal-to-noise ratio in comparison to current PET systems.
Independent to the EXPLORER, a high-resolution 100 cm-aFOV PET system, called “PET20.0,” is currently being configured in cooperation between Ghent University and Vrije Universiteit Brussels [115]. In comparison to the PET EXPLORER, PET20.0 has a shorter aFOV of 100 cm. However, with monolithic detector crystals and improved positioning methods, the spatial resolution of PET20.0 (2.0 mm) is expected to be better than the spatial resolution of the EXPLORER (3.5 mm).
In contrast to the aforementioned PET systems, a lower-cost alternative based on resistive plate chamber (RPC) detectors has been proposed also. In addition to being economic and reliable, RPC-PET detectors offer good spatial-temporal resolution, high time-of-flight resolution and high energy sensitivity. Further, the RPC detectors allow for the accurate measurement of Depth-of-interaction (DOI), which renders RPC-PET parallax-error free. These properties make RPC-detectors an excellent option for an extended aFOV PET system [116].
MR technology
Currently over 60% of clinical MR systems operate at 1.5 Tesla for cost and safety reasons [3, 117]. To date, the conventional upper limit for the field strength of clinical MR is 3 Tesla. The growing adoption of MR systems with 7 Tesla (over 80 units installed worldwide) could be seen to represent the new frontier of MR imaging1. Of note, singular testing of MR systems with 10.5 T (University of Minnesota, USA) and 11.7 T (CEA, Saclay, France) is work-in-progress [3], but it is unlikely that these ultra-high-field MR systems will soon be employed in hybrid imaging.
For hybrid imaging of human subjects and patients, i.e., combined PET and MR, actively shielded 3 T magnets have been chosen by all manufacturers. In addition to the wide bore magnet, fast gradient coils (slew rate 200 mT/m/ms) and dedicated RF coils (proton frequency at 128 MHz) are required [3, 118]. Recently, maximum gradient strength of up to 300 mT/m were realized at 3 T [119], although state-of-the-art clinical systems operate with a gradient strength of 70 mT/m, which is sufficient for most routine pulse sequences prescribed. Dedicated RF-coil arrays are being developed to further improve sensitivity and speed and to avoid costly ultra-high field magnets, thus, rendering a suitable option for combined PET/MR systems [120] as well as for various parallel imaging techniques [121–123]. Parallel imaging enables more efficient data sampling to either increase acquisition speed or the amount of information collected per time interval.
Data handling
This section provides an overview of standard data acquisition and image reconstruction techniques for functional (SPECT, PET) and anatomical (MR, CT) imaging modalities (section Image Acquisition and Reconstruction in CT, PET, and SPECT to Quantitative Data Corrections in PET and SPECT). Advanced data processing methods are described in sections Anatomically-Driven PET/SPECT Image Reconstruction to Outlook and Future Trends, which make use of the anatomical information provided by the CT or MR images to improve the PET-SPECT quantification).
Image acquisition and reconstruction in CT, PET, and SPECT
A CT image represents the tissue-dependent attenuation of X-rays of the investigated object, thereby making use of an X-ray source of known source strength and an opposite detector array, both of which rotate at a fixed speed around the center of the FOV. Alternatively, SPECT and PET images represent the distribution of activity concentrations (per voxel) of a single photon (SPECT) or a positron (PET) emitter.
There are two main categories for Image Reconstruction (IR) algorithms in CT, SPECT and PET: analytical reconstructions and iterative reconstructions [124–126]. Analytical approaches are based on a closed-form equation that directly yields one solution based on the input (Figure 3A), while iterative techniques are based on a more accurate description of the imaging process, thereby resulting in a more complicated mathematical solution that requires multiple steps to achieve an image. Iterative methods model the data collection process in a tomographic system and search for the image that is most consistent with the measured data (Figure 3B).
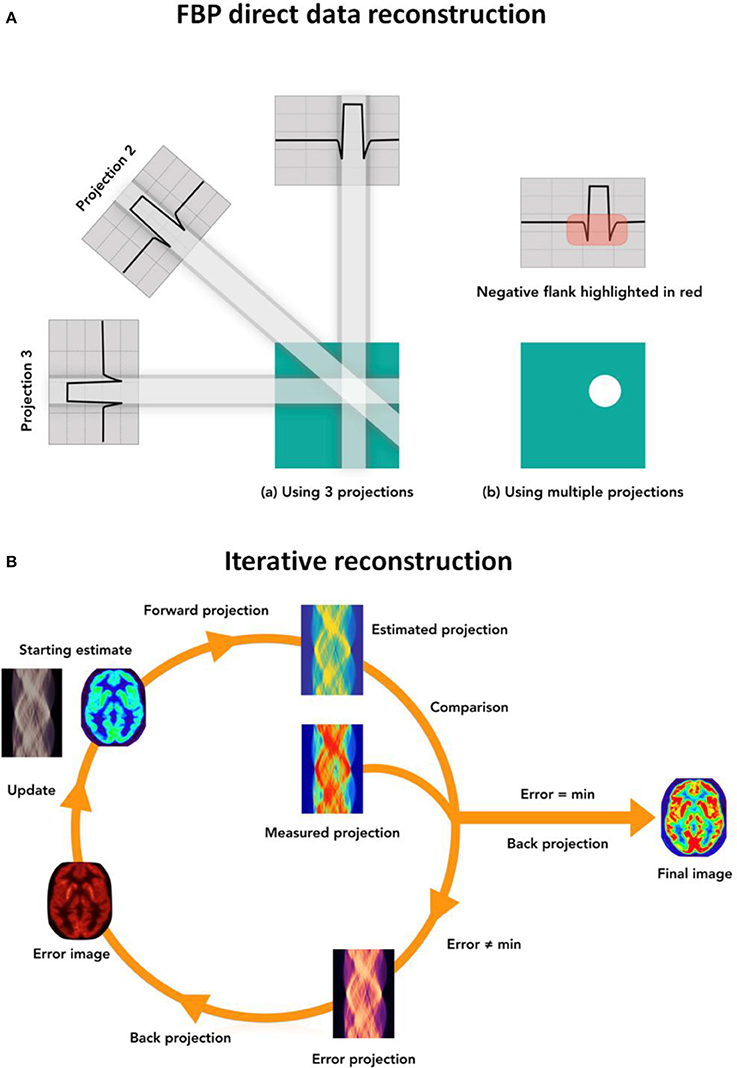
Figure 3. (A) Simplified view of the direct FBP image reconstruction technique: the projected data is filtered using different filter kernels and back-projected into the image domain. Multiple projections are required to obtain the final CT image. (B) Schematic view of the iterative reconstruction process: first, a forward projection of the initial estimated image is used to create the estimated projected data; then the estimated data is compared to the acquired raw data and a set of correction factors is derived. These correction factors are back-projected and used to update the initial estimated image. All three steps describe an iterative loop, which is repeated until a predefined condition is satisfied and the final image is generated. Similar reconstruction processes apply for SPECT and PET data.
Analytical IR
The filtered-backprojection (FBP) reconstruction [127] method is the standard method for image reconstruction of CT data. The FBP method combines a back-projection operation, which describes the propagation of the measured projection data into the image domain, with a filter component that compensates for the low-pass blurring inherent to the back-projection approach. With the advances in CT technology, different adaptations of the FBP algorithm (interpolation methods, 3D-reconstruction methods: Feldkamp algorithm, etc. [128–132]) have been proposed to compensate for fan-beam and cone-beam geometries. Likewise, exact [128, 133] and approximate [134] 3D reconstruction methods have been proposed for helical cone beam reconstruction, which are being used in most commercial CT systems due to their flexibility and computational efficiency [125].
Iterative IR
Iterative methods offer improvements over the analytical approach because they allow the noise structure of the data to be accounted for, thus, incorporating a more realistic model of the system. All iterative reconstruction methods consist of three steps that are iterated until a convergence condition is met (Figure 3B). First, an estimated raw data set is created from an estimated initial image using a realistic model of the tomographic system. Second, the artificial raw data is compared with the real data acquired by the system in order to obtain a correction term for each bin in the projected data. Finally, these correction terms are back-projected and used to update the image estimate.
Iterative reconstruction methods can be classified in deterministic and statistical approaches [135]. The most frequently used deterministic approaches are the Algebraic Reconstruction Technique (ART) [136], the simultaneous ART (SART) [137], and the ordered-subsets Simultaneous Iterative Reconstruction technique (OS-SIRT) [138]. Statistical methods incorporate counting statistics of the detected photons into the reconstruction process. The most well-known methods are the Maximum likelihood expectation-maximization (ML-EM) [139], its accelerated version by means of the ordered-subsets expectation-maximization (OSEM) [140], and the model-based iterative reconstruction (MBIR) [141], which is used predominantly in multi-slice helical CT.
Image reconstruction methods in SPECT and PET are similar to the methods employed in CT although the physical nature of the acquired data is different. These differences relate mainly to the geometrical model (e.g., CT—fan-beam, cone-beam or helical vs. PET—cylindrical rings of detectors vs. SPECT—rotating flat detector panels plus collimators), and, thus, to the level of mandatory a priori data corrections.
Statistical IRs have been used extensively in SPECT and PET due to their benefits with regards to noise reduction and improved accuracy in the reconstructed images [124]. However, these approaches are not yet widely adopted in clinical CT. This is due to the numerous pre-processing and calibration steps in CT, which change the statistical properties of the measured data; the high spatial resolution in CT, which requires the application of edge-preserving regularization techniques; and the large amount of data that requires long reconstruction times. Nonetheless, the exponential growth of computer technology and the recent introduction of IR methods that can be implemented on a Graphic Processing Unit (GPU, [142]) render the use of iterative reconstruction in CT more and more a clinical commodity.
Image acquisition and reconstruction in MR
MR imaging is a non-invasive tomographic imaging technique that acquires data from the inside of an object using radio-frequency (RF) excitation of protons that subsequently retransmit the absorbed energy, depending on the biological environment. Nuclear induction of RFs is performed using coils or coil arrays, with a wavelength larger or similar to the object dimensions (at 3 T/128 MHz the proton wavelength in biological tissue is about 30–50 cm). In order to allow spatial encoding of RF emission from bulk tissue, gradient coils need to be used that limit the resonance frequency to a small portion of image space. This is commonly performed using three independent linear gradient fields, although other approaches, using, e.g., radial or spiral gradients, exist. Following a combination of excitations and measurements, imaging and contrast modules can be selected independently, thus, making MR a highly versatile and flexible imaging technique with excellent soft tissue contrast [143]. The imaging module defines the details (i.e., matrix size, 2D/3D) of the data collection from an object of a given size in a given time (i.e., measurement time). Anatomical MR data acquisition is performed for the purpose of high-resolution imaging (i.e., matrix size 256 × 256 up to 512 × 512) in a matter of a few minutes. For functional data, reduced spatial resolution is acceptable (i.e., 64 × 64 up to 128 × 128) in view of acquiring 3D frames within 10–50 ms and with short repetition times, TR, of 100–2,000 ms over the course of several minutes.
Given the variety of pulse sequences and sequence parameters (e.g., TR, TE, MA, FOV, etc.) data processing and analysis pipelines, particularly in research, can be rather complex. In addition, differences in pulse sequences and processing software can be observed between manufacturers [118], often depending on hardware performance or Intellectual Property (IP) issues. By combining short (ms) magnetic pulses produced by an RF-coil with orthogonal magnetic gradients in x, y, z; voxel localization in 2D or 3D is enabled and the voltage induced in the receive coil can be detected. This voltage is converted into numbers (12-bit to 16-bit digital resolution) and visualized on a computer screen as gray scale values/images of tissue specific image contrast.
In a mathematical framework, a Fourier transformation of the detected signal is stored in k-space (2D-space consisting of all frequencies and phases detected in the measured RF signal) and then transformed into image space (x,y) via the inverse Fourier transformation. The result is a spatially resolved magnetization vector M(r).
Quantitative data corrections in PET and SPECT
In order to obtain quantitative and artifact-free PET or SPECT images, several corrections must be applied to the acquired data, which include, for example: decay correction, photon attenuation correction (AC), scatter correction, normalization, dead time correction and randoms subtraction (only in PET). Here we will discuss the most widely methods used for quantitative data corrections in PET and SPECT images, and their application in PET/CT and SPECT/CT systems. In PET/MR, as the MR image information is not related to the attenuation properties of the material, novel methodologies for AC need to be developed, and are discussed in detail in section Novel MR-Based Attenuation Correction (AC) Methods for PET/MR. For a general overview on the quantitative correction methods for PET we refer the reader to Zaidi [144], while for SPECT we suggest [72, 145].
Attenuation and scatter corrections in PET/CT
The emitted photons in PET and SPECT are subject to attenuation as they travel through the patient. As consequence, the number of detected photons in each LOR will be reduced. The most widely used method for AC in PET/CT was proposed by Kinahan et al. [17, 146] and it is known as “bilinear” segmentation-scaling method. Here, the PET attenuation image is estimated by first using a threshold to separate the bone component from the soft tissues of the CT image, and then using separate scaling factors for the bone and non-bone component. Alternatively, the emitted photons may be scattered in the patient body or in the detector itself, suffering a deviation of its trajectory. As a consequence, the LOR of a scattered event will not be longer aligned with the emission point. The most extensively used method for scatter correction in PET/CT (and PET/MR) is the Single Scatter Simulation (SSS) method [147, 148], where only a single scatter event is considered and multiple or out of the FoV scatter contributions are included as scaling factors.
Attenuation and scatter corrections in SPECT/CT
Similar to in PET/CT, the bilinear method proposed for CT-based AC can be used in combined SPECT/CT systems [18, 149]. On the other hand, the most common approach for estimating scatter events in SPECT/CT is the measure of counts in additional energy windows adjacent to the photopeak window. The most common examples are the Dual Energy Window (DEW) approach that neglects upper scatter or the Triple Energy Window (TEW) [150–152]. More complex solutions, based on Monte Carlo estimations of the scattered events, have been also proposed [153, 154] and are expected to be widely used in the near future.
Randoms subtraction in PET
Random events occur when two uncorrelated photons, from two different decays, are detected in coincidence within the timing window. The randoms events can be estimated by using a delayed coincidence window [155].
Normalization in PET and SPECT
Because of variations in the gain of PMTs, inaccuracies/tolerances in detector block building, physical variation of scintillator efficiency, etc., the detection sensitivity varies from detector to detector. Information on these variations, often known as normalization [145, 156], is required for the reconstruction of quantitative and artifact-free images.
Anatomically-driven PET/SPECT image reconstruction
Iterative IR methods for PET and SPECT are challenged in two ways. First, PET, and SPECT images have an inherently limited spatial resolution due to the physical process that leads to the image formation. And, second, noisy images are obtained from over-iterated reconstructions, given the lack of an objective stopping rule in the iterative reconstruction. The latter problem is addressed in clinical routine by using a Gaussian post-reconstruction filter, which further degrades the perceived resolution and contrast of the images in return for lower noise levels.
Bayesian methods attempt to improve the quality of the reconstructed image by taking advantage of prior knowledge [157], that can be obtained, for example, from a co-registered anatomical image (CT or MR). This information is known a priori and is often incorporated into a maximum a posteriori (MAP) objective function [158]. These reconstruction methods lead to improvements of contrast and noise properties of the reconstructed SPECT and PET images [159–161]. This a-priori known anatomical information can be introduced into the reconstruction algorithm by using probabilistic image models [162] or similarity measures between the anatomical and the functional images [163].
Partial volume correction in PET and SPECT
The partial volume effect (PVE) is related to the limited spatial resolution of the nuclear imaging system and affects the quantitative accuracy of the images, particularly for small lesions and brain structures (Figure 4). PVEs are caused by two different phenomena: the limited spatial resolution of the imaging system (Figure 4A) and the discrete image sampling of a continuous 3D activity distribution (Figure 4B). PVE results in smearing of the reconstructed activity levels and, thus, has a significant impact on their qualitative and quantitative assessment.
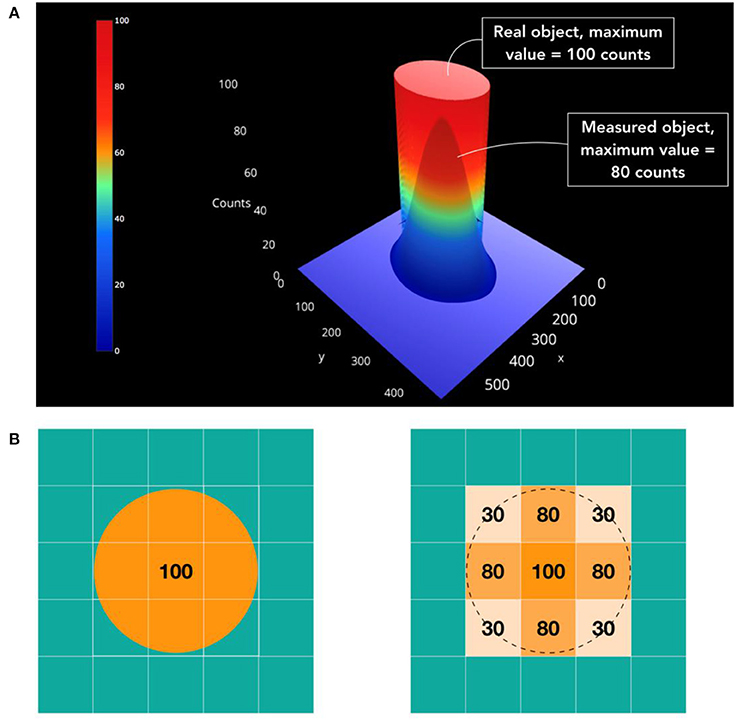
Figure 4. Illustration of the partial volume effect (PVE) in medical imaging. (A) A circular source of uniform activity in a warm background region yields a measured image in which part of signal emanating from the source is observed outside (spill-out) while part of the background signal is observed within the lesion (spill-in). (B) The tracer distribution is sampled on a voxel grid and the contours of the voxels do not match the actual contours of the tracer distribution (Tissue-fraction).
A wide variety of partial volume correction (PVC) methods have been developed in the past, most of them employing high-resolution anatomical information from a co-registered CT or MR image as reference [164–167]. PVC methods can be grouped into two main categories: post-reconstruction and during-reconstruction methods. Post-reconstruction methods include the region-based methods, such as the recovery-coefficient correction [168] and the geometric transfer-matrix (GTM) method [169]. Other post-reconstruction corrections consist of voxel-based methods, such as the popular Mueller-Gaertner method (MGM) [170], image deconvolution [171], and the “region-based voxel-wise correction” (RBV) [172]. Alternative approaches for PVC operate in sinogram space, where statistical noise is spatially uncorrelated and easier to incorporate in the PVC procedure [173–176]. Reconstruction-based methods enhance the spatial resolution by using the implicit PVC compensation through system-response modeling [177]. More advanced PVC methods make use of anatomical priors during the reconstruction process [159, 161], as described in the previous section.
Despite the large number of PVC methodologies proposed in the literature, explicit corrections for PVE are still far from being fully utilized in clinical routine [178]. This is due to several challenges associated with their automated implementation. First, the accurate delineation of the tissues of interest from the morphological images is a challenging task, especially in cases where the metabolic tissue boundaries do not correspond well to the morphologic boundaries. Second, some of the PVC methods require segmentation of tissues into different VOIs that span the entire cross-sectional extent of the subject, which can be computationally intensive.
Motion compensation
The hybrid imaging protocols for SPECT/CT, PET/CT, and PET/MR systems often span acquisition times of several minutes and, thus, cover multiple breath-holds or cardiac contractions. Therefore, these hybrid images are subject to involuntary patient motion during the acquisition [179]. The induced respiratory motion as well as cardiac contraction introduce motion blur in the acquired images (Figure 5), which may affect the clinical interpretation of the acquired images [182].
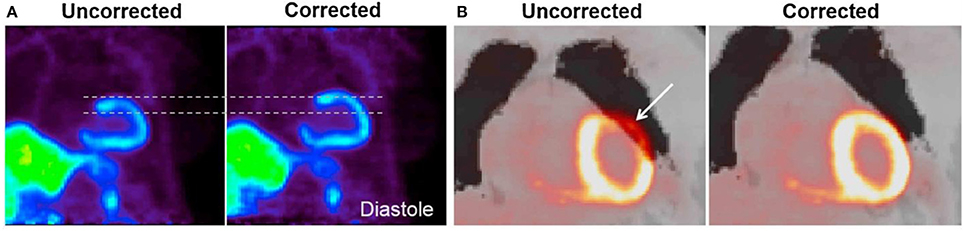
Figure 5. Effect of respiratory and cardiac motion on PET image quality. (A) Blurring effect due to cardiac motion in a patient scanned with 13N-NH3. (B) Misalignment artifact between the PET-emission data and the corresponding MR-AC map. Misalignment artifacts like these are common in cardiac PET-imaging, where they are known to cause false-positive findings in up to 40% of all studies [180]. Reprinted from Rausch et al. [181] with permission from Elsevier.
Patient motion has traditionally been detected through the use of external markers, such as ECG-electrodes for cardiac motion or respiratory belts [183] as well as infrared/optical systems [184] for respiration-induced motion. Recently, the use of data-driven methods has gained substantial interest for both stand-alone and multi-modality imaging systems [185–188]. Continuous motion tracking can be used for motion compensation of PET and MR images [189, 190]. In thoracic PET imaging, several motion detection techniques have been proposed, through built-in readout of the respiratory position and special radial-self gating sequences, respectively [186, 187, 191]. Cardiac motion is most commonly estimated by using cine-MR imaging and tagged MR imaging [192].
Approaches toward respiratory and/or cardiac gating are commonly used to reduce motion-induced blurring in PET and SPECT images, whereby the emission data is divided into gates that correspond to different respiratory or cardiac phases [185]. However, a more preferable solution is to perform motion compensation (MoCo) using the full data-acquisition, both to reduce acquisition times and to improve the signal-to-noise ratios in the PET or SPECT images. Therefore, different MoCo techniques have been proposed: MoCo before image reconstruction (projection-based methods) [193], during PET-image reconstruction—motion compensation during image reconstruction (MCIR) [194], and after PET-image reconstruction [195]—Reconstruction Transform Average (RTA). Recent studies have been performed to evaluate the performance of these three methodologies [196, 197]. More advanced methods, that use the synergistic information derived from the simultaneous PET and MR data acquisition, have been proposed to estimate [198] and to correct for motion in both PET and MR images [189]. Other attempts focused on the implementation of joint MoCo and PVC approaches to further improve the PET quantification of small lesions in regions that are susceptible to motion [199, 200].
In addition to respiratory and cardiac motion, non-periodic and unpredictable motion can also occur during the scan. Organ specific motion detection techniques have been developed for head and neck examinations, through the use of frequent 3D-MR navigators, which can be employed to apply motion compensation (MoCo) to the PET images [201].
While most of the aforementioned references were related to PET/CT and PET/MR imaging, it is important to note that most of these techniques are applicable also to SPECT [202], while standard data-driven motion detection techniques for PET do not work well for SPECT [203]. Nevertheless, the more advanced Principal Component Analysis (PCA) and Laplacian Eigenmaps (LE) methods for data-driven respiratory motion detection have demonstrated good results for both PET and SPECT imaging [203, 204].
Novel MR-based attenuation correction (AC) methods for PET/MR
In PET/MR examinations the anatomical information used for attenuation correction (AC) originates from the complementary MR image information, which is not related to the attenuation properties of the material [205]. Moreover, the imaging of cortical bone is a challenge in MR given the fast relaxation times of solid materials [206]. As a consequence, it is not possible to distinguish between cortical bone and air in most MR sequences [207]. To address this issue, new AC methods had to be developed, that can be categorized into three basic concepts [181] (Figure 6): (i) segmentation-based methods, which are based on a segmentation of MR images into different tissue classes; (ii) atlas- or model-based approaches, which incorporate a-priory knowledge of attenuation properties of the investigated subject from data bases; and (iii) reconstruction-based methods, which are based on a direct reconstruction of the attenuation and activity values from the emission data.
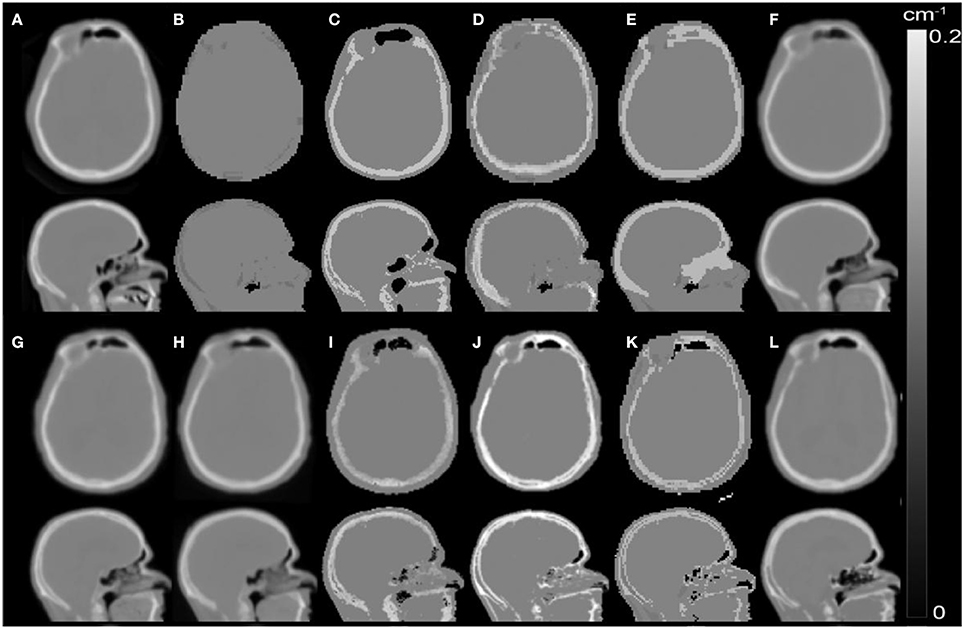
Figure 6. Illustration of popular MR-based AC methods using the same axial slice of AC maps of a patient processed with different AC methods: (A) Reference CT-based AC; Standard methods: (B) standard DIXON-based MR-AC implemented in the Siemens mMR system (SW v. VB20), (C) UTE-based standard MR-AC for brain examinations implemented in the Siemens mMR system (SW v. VB20); Template-based methods: (D) Koesters et al. [208], (E) Anazodo et al. [209], (F) Izquierdo-Garcia et al. [210], (G) Burgos et al. [211], (H) Merida et al. [212]; MLAA-based methods: (I) Benoit et al. [213]; Segmentation-based methods: (J) Cabello et al. [214], (K) Juttukonda et al [215], (L) Ladefoged et al. [216]. Figure adapted from Ladefoged et al. [217] (Courtesy of Claes N. Ladefoged, Rigshospitalet Copenhagen, Denmark; Modified from the original image published under the creative commons license http://creativecommons.org/licenses/by-nc-nd/4.0/). Figure published with the permission of the copyright holders (Claes N. Ladefoged).
At present, standard AC approaches for whole-body imaging in clinical systems rely on segmentation-based methods [218, 219], recently also in combination with atlas-based approaches incorporating the major bone structures into the AC [220, 221]. In general, these approaches perform reasonably well, although for specific application their accuracy may not be sufficient [222]. Thus, several advanced AC methods have been explored in conjunction with various types of PET/MR examinations [223, 224, 217]. Most of the developed AC methods are tailored to brain examinations and provide acceptable accuracies (with deviations below 5% respect to CT-based AC) [217]. A selection of available MRI based AC methods for the head is given in Figure 6. A detailed comparison of these methods can be found in Ladefoged et al. [217]. However, for AC of body parts other than the brain as well as for AC of non-rigid hardware components (e.g., flexible surface coils), challenges still persist [225, 226], such as body truncation, metal and respiratory artifacts, MR coils attenuation, etc. Some promising solutions for whole-body AC are currently under development, for further details we refer the reader to Leynes et al. [223], Mehranian et al. [225], Heußer et a. [227].
Joint data exploration
This section describes main efforts toward a fully-synergistic use of the anatomical and functional information available from hybrid imaging examinations. In section Kinetic Modeling and Image Derived Input Function (IDIF) we discuss the use of image-derived input functions in the context of kinetic modeling, in section Multi-Parametric Imaging (MPI) we provide an overview of the multi-parametric imaging studies performed using SPECT/CT, PET/CT, and PET/MR systems. Finally, in sections In Vivo Disease Characterization and Image-Derived Prediction Models we explore recent efforts in obtaining in vivo disease characterization and image-derived prediction models.
Kinetic modeling and image derived input function (IDIF)
As discussed in section Quantification in PET and SPECT, absolute PET quantification requires the measurement of an input function, which is typically done by means of an arterial blood sampling. The measurement of an arterial input function (AIF) is invasive, laborious and stressful for the patient. Obtaining an image-derived input function (IDIF) is a non-invasive alternative, whereby the input function (IF) can be directly obtained by defining a volume-of-interest (VOI) in the PET images and using anatomical information from the MR or CT images to segment the tissue of interest to obtain the IDIF (Figure 7A).
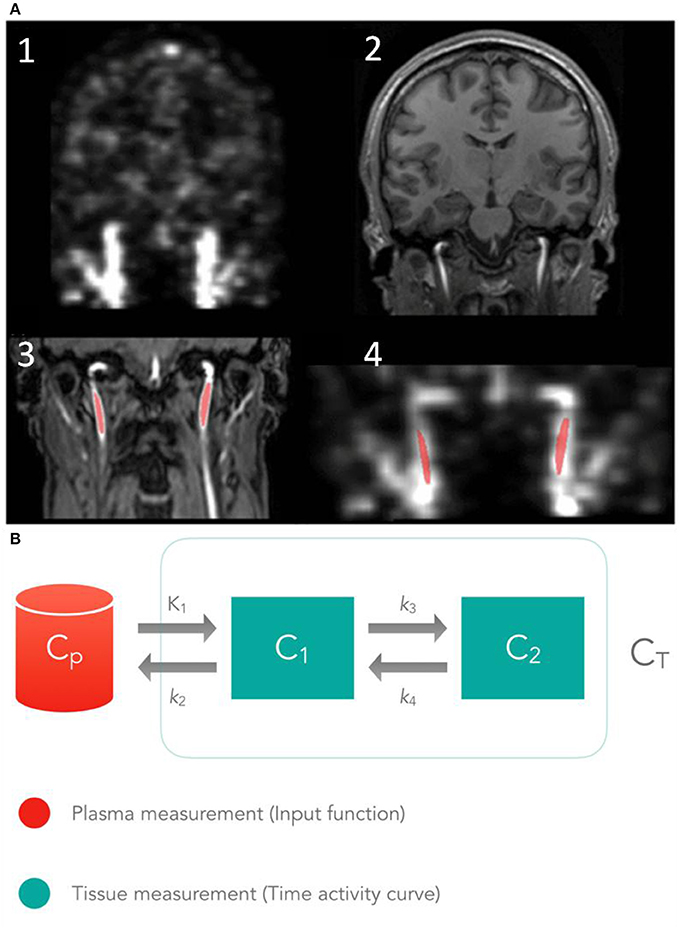
Figure 7. Schematic view of the IDIF concept. (A) 1- PET coronal view showing the carotid arteries, 2- T1-MPRAGE image, 3- TOF MR angiography image of neck region used to segment the carotid arteries, 4- PET coronal view co-registered with the segmented carotid arteries. (B) Illustration of the 2-tissue compartment model commonly used for FDG tracer kinetics. Here, CP represents the blood activity and C1, C2 the activity concentration of each tissue over time t. K1, k2, etc., are the rate constants that define the rate of tracer movement between compartments. This figure was adapted from Sari et al. [228], with permission of SAGE Publications, Ltd.
The concept of an IDIF has been successfully implemented in clinical routine examinations for cardiovascular studies, due to the availability of large blood pools (i.e., left ventricle) in the PET FOV [229, 230]. However in PET studies of the brain, calculation of the IDIF is challenged by two factors: PVE [231, 232] due to the small size of the blood pools, and subject motion [232, 233]. Various approaches have been proposed to extract an accurate IDIF that can be classified into PET-only based methods [232, 234–244], stand-alone PET and MR-based methods [245, 228, 246], and fully-integrated PET/MR-based methods [247–249]. Combined PET/MR can potentially allow researchers to address the aforementioned challenges (PVE and subject motion) in an automatic way [228, 248, 249], in addition to bearing a logistic advantage. It should be noted that various corrections (delay, dispersion, metabolite correction) may be required to convert the measured blood IDIF to plasma IDIF.
An IF can be employed together with a physiological model in order to calculate physiological parameters, such as metabolic rate of glucose (umol/100 g/min) or blood flow (ml/100 g/min) [250]. The creation of such parametric images can be divided into three different methods: (i) graphical analysis, (ii) compartment model, and (iii) reference tissue model. The first two methods require information on the non-bound tracer-activity available in the blood.
Graphical analyses methods include the Gjedde-Patlak equation [251, 252], which describes irreversibly bound tracers, such as [18F]FDG and the Logan-plot, which can be used to describe the reversibly bound tracers [253]. Tissue-compartment models have been proposed by Kety and Smith in 1948 for the calculation of the blood-flow in the brain using nitric oxide [254, 255]. Figure 7B represents a 2-tissue compartment model, which is the most appropriate to evaluate the tracer dynamics of FDG. Here, K1 is the influx-constant (amount of tracer moving from the blood to the tissue), k2 the efflux-rate (amount of tracer that returns to blood), CP the blood activity and CT the tissue concentration over time t. Compartment 1 (C1) represents the un-metabolized tracer and compartment 2 (C2) the metabolized tracer (where k3 represents phosphorylation by hexokinase and k4 dephosphorylation) [256]. Reference tissue models are primarily employed for neurological studies where the cerebellum or the pons is often used as reference tissues.
Multi-parametric imaging (MPI)
Multi-parametric imaging (MPI) has been of interest since the adoption of standalone PET, CT, and MR imaging, but gained further attention following the availability of combined imaging systems [257]. Combined, or dual-modality imaging systems facilitate multi-faceted and complex evaluations of tumor phenotypes, thus, promising an improved characterization of lesions and pathophysiology [258].
In addition to the anatomical information provided by CT-images, quantitative assessment of physiological parameters extracted from CT-images in combination with functional PET-images increase the diagnostic value [259]. For example, texture analyses (TA) of the tumor heterogeneity obtained from CT-images is frequently used in combination with functional PET data [259]. However, the TA varies significantly with the quality of the underlying image data, thus, limiting the reproducibility of the procedure, particularly in the context of PET imaging [260].
Fully-integrated PET/MR systems permit the quasi-simultaneous acquisition and evaluation of functional and structural information of patients, and, thus, support the multi-parametric assessment of these subjects, as shown for the patients with neurological disorders [261]. Specifically, the use of parametric imaging [section Kinetic Modeling and Image Derived Input Function (IDIF)] of data from PET/MR systems can help to shed new light on the mechanisms that underlie structural changes in the brain through the use of targeted radio-labeled tracers in combination with gadolinium-based contrast enhancement of MR [262]. Likewise, the combination of PET and MR images has proven to add prognostic value for assessments of myocardial examinations [189, 263, 264], while the value of the multi-parametric images obtained for oncological studies is still under evaluation [265].
in vivo disease characterization
Hybrid imaging plays an important role in the characterization of several diseases [4]. Thanks to hybrid imaging lesion detection, tissue delineation and therapy monitoring have become more accurate [266]. Hybrid imaging has been key to cancer patient management [267], thanks also to its ability to depict tumor heterogeneity in a morphological and functional context [260]. Given that conventional indices, such as standard uptake value (SUV), maximum or metabolic tumor volume (MTV) are insufficient to characterize malignancies [268], several groups have started to investigate the feasibility of in vivo tumor characterization in the context of textural evaluation [269]. As a result of this initiative, first reports have appeared that point at promising results of a quantitative assessment of tumor heterogeneity in light of therapy response prediction [270, 271], disease-specific survival [272] as well as prognostic stratification [273]. Meanwhile, challenges using textural features remain, since such calculations are highly sensitive to acquisition, reconstruction and sample size variations [274–276]. Overall, the need of reproducibility evaluation as well as standardization of textural features is being acknowledged in the field [274].
The approach that considers medical images as data mining sources for large-scale in vivo feature evaluations is called radiomics [277]. It is expected that with the introduction of hybrid imaging, radiomics holds the potential to lead to automated tumor characterization [278–280] and personalized treatment regiments in the future [281]. For more details, we refer to the companion review by Papp et al.2 in this journal issue.
Image-derived prediction models
Machine learning (ML) algorithms have the ability to identify key patterns and characteristics of large scale datasets [282]. While the concept of ML is not new, it has only recently become popular thanks to recent advances in computational power and capacities that made it feasible to handle large datasets. ML holds also a great potential for dealing with “big data” generated by hybrid imaging methods [280]. For example, ML has been successfully applied in hybrid imaging to predict survival [283], treatment outcome [284], and tumor grading [285]. Furthermore, unsupervised ML allows the identification of breast cancer subtypes [286]. For more details, we refer to the companion review by Papp et al.4 in this journal issue.
Multi-centre standardization
The performance of a SPECT or PET system is related to factors as detector type and geometry, the properties of the built in electronics and the implemented data processing. To characterize the system performance, standardized procedures are published by the National Electrical Manufacturers Association (NEMA) [287–289]. While these standards are a good example how to set up a basic set of measurements for the purpose of system characterization and comparison, their results are generally not suitable to predict the quantitative accuracy of a clinical examination. Quantification in nuclear medicine examinations is not only a matter of proper imaging system performance. The outcome of a study is influenced also by various factors related to imaging technology, biology and imaging physics [290]. In PET/CT operations, for example, a significant variability of system performance, examination protocols, and quantitative reporting across different centers has been described [291]. A similar variability in workflow has been observed in SPECT/CT operations [292]. Although in SPECT/CT most readings are based on a qualitative assessment of the tracer distribution, significant variation in quantitative evaluations of SPECT/CT examination can be expected from the use of CT-AC.
This variability in quantification between systems and across sites is a major barrier to the utilization of quantitative nuclear medicine and hybrid imaging in larger cohort and multi-center studies [293]. To gain reproducible and statistically significant results, e.g., for the evaluation of a new drug or the usability of an imaging modality to assess treatment response, a sufficient number of examinations has to be collected. Since this is usually not possible within a single institution, data from several centers need to be pooled following the harmonization of imaging protocols [293]. Several guidelines have been put forward [294–296] and specific programs, namely the accreditation programs of the EANM or the ACR, have been launched to sensitize the imaging community and to help address this challenge toward pooled data evaluation.
In general, most of the factors influencing simple quantitative readings, as SUV, are understood and—in many cases—corrections and workarounds exist [290]. However, with the availability of more advanced image evaluation techniques, such as ML-based approaches employing textural parameters, new challenges for a standardized evaluation arise. Textural parameters are strongly influenced by the choice of image reconstruction, voxel size and post processing steps (e.g., Gaussian filtering). Furthermore, the choice and definitions of the textural analysis metrics vary widely and the evaluation techniques differ substantially, thus, rendering a comparison between studies almost impossible [260, 297]. The need for standardization efforts in the field of textural analyses has been highlighted previously [260, 298, 299], and efforts are currently under way to address this challenge [260, 300–302].
Outlook and future trends
Open research data, sharing knowledge
Clinical research is an essential building block for the concept of efficient patient management. Research studies are generally complex and the resulting data are valuable, not only to the principal investigator but to society as a whole [303]. Although today's ubiquitous multi-modality imaging protocols produce an abundance of qualitatively diverse data sets, there is a lack of genuinely integrative analysis schemes that could provide added value to a pure additive analysis.
Moreover, many researchers remain reluctant to share their data with an expert audience [304, 305] beyond describing them as part of peer-reviewed publications. In contrast, sharing research data in a structured and tangible way has been shown to yield benefits for both, the principal investigators of the study who generated the data as well as other experts [306] in the field who may re-use the data with alternative evaluation algorithms to extract new information that may subsequently benefit patient management [303]. Obviously, a reliable anonymization or even pseudo-anonymization of the relevant data must precede any type of data accrual and accessibility as part of public data repositories. Frequently, the challenges associated with prior data handling alone render such repositories a mighty wish rather than a useful reality.
Still, after data are properly anonymized and made available under an open data policy, the quality of such public Supplementary Material collections of published studies, at least, is frequently variable, and many times the re-use of these data is not possible [307]. The same holds true for the quality of alternative public data archives that were shown to contain incomplete data and data archived only partially in over 56% cases that prevented re-use [305].
Nevertheless, future trends in multi-modality imaging point toward the emergence of advanced database structures that include interactive 3D visualization tools and powerful data mining applications. The leading candidate for generating such flexible database structures is the XML (eXtensible Markup Language) model [308] due to its flexibility and scalability. The flexibility of XML database structures allows the combination of patient data with similar disease within a common reference frame, allowing meta-analysis of data patterns distributed over many modalities. An example of such an overarching effort is the National Database for Autism Research (NDAR, http://data-archive.nimh.nih.gov/#NDAR-anchor), an NIH-funded data repository that aims to accelerate progress in autism spectrum disorder (ASD) research through data sharing, data harmonization, and the reporting of research results.
Multi-tracer PET and SPECT imaging
Another interesting application is the multi-tracer SPECT or PET imaging, which aims to image two or more tracers in a single scan, simultaneously characterizing multiple aspects of physiology and function without the need for repeat imaging visits [309]. Tracer separation in multi-tracer SPECT takes advantage of the differences in the spectro-temporal properties (differences in the gamma emission energies and dynamic behavior) of the radionuclides to identify the tracer distributions [310, 311].
Multi-tracer PET must rely on differences in the kinetics and/or spatial distributions of the tracers, because the energy of annihilation photons of all tracers is 511 keV. The different techniques to separate multiple-tracer PET may be based on the analysis of the different radioactive half-lives of the radionuclides [312, 313], multi-tracer compartment models [314] or generalized methods that make use of PCA to separate the tracers [315]. A recent development in multi-tracer PET is the combination of a pure and a non-pure positron emitter [316]. This technique relies upon detecting the auxiliary prompt gamma in coincidence with an annihilation event in order to measure triple-coincidence events originating from only one of the tracers, and use the spatial distribution of the triple coincidences to disentangle the coincidences originating from each tracer [317, 318].
Dual-modality tracers/contrasts
Contrast-enhanced CT data as part of a combined PET/CT examination provides additional, helpful information in comparison to non-enhanced PET/CT studies [319–321]. Here, the main benefit relates to a more precise anatomical localization of pathologies by differentiating lesions from surrounding structures. On the other hand, MR provides highly-detailed non-invasive anatomical image information and excellent soft tissue contrast [322], even in comparison to contrast-enhanced CT.
Efforts are under way to develop dual-modality contrast in PET/MR based on nanoparticle-based probes. Currently, super paramagnetic iron oxide nanoparticles (SPIONs) are used that incorporate positron emitters (e.g., 52-Mn, 59-Fe, and 124-I) and contain small surface molecules (peptides, organic molecules) that bind to tumor tissue [323, 324]. Although the physiological functionality of the PET probe is limited by its attachment to a large nanoparticle, the combination provides high spatial resolution (MR) with high sensitivity (PET).
With the advent of hybrid microPET/SPECT/CT systems, efforts to develop multi-tracer PET-SPECT studies have been made. Although the scattered photons from PET preclude to obtain useful information in SPECT with simultaneously injected PET/SPECT tracers [325, 326], sequential studies where the SPECT radiotracer is injected and imaged first and the PET radiotracer is injected and imaged in the presence of the SPECT tracer have shown promising results [326].
Hybrid imaging and therapy (planning, follow-up)
One of the major developments in radiotherapy in the coming years is the use of hybrid imaging for individualized biology-guided radiation therapy (RT) [327]. Given the information on the tumors provided by PET/CT and PET/MR imaging, dual-modality PET-based imaging represents an optimal basis for RT individualization, especially in the context of intensity-modulated radiotherapy (IMRT). The combination of IMRT and hybrid imaging enables the modulation of radiation dose distribution according to local phenotypic or micro-environmental variations in an individual tumor (aka “dose painting”) [328, 329]. The goal of the dose painting process is to select and delineate target volumes (and organs at risk) on the basis of complementary diagnostic information, thus, aiding in heterogeneous delivery of radiation within the tumor volume by targeting radio-resistant areas. This approach is particularly promising in treatment monitoring, by taking into account the individual patient response and dynamically adjusting the treatment plan based on current outcome. Given the broad selection of established PET tracers, hybrid imaging provides RT planning with patho-physiological information pertaining to various molecular pathways of the tumor, including metabolism, proliferation, oxygen delivery and consumption as well as receptor or gene expression. Examples are [18F]FDG [330] and [18F]/[11C]choline [331–333] as surrogates for tumor burden, [18F]FLT as a surrogate for proliferation (or cellular growth fraction) [334], and hypoxia sensitive tracers such as [18F]FMISO [335] and [64Cu]ATSM [336] as surrogates of cellular hypoxia.
On other hand, PET monitoring is a popular method for range verification of particle therapy: proton or hadron therapy [337]. Positron emitters are generated inside the patient during treatment, and therefore, by using PET imaging the delivered dose can be indirectly measured [338, 339]. This can be done during the irradiation (on-line or in-beam PET), which is beneficial when imaging short-lived emitters [340], or after the treatment in an off-site system (off-line), which can provide better counting statistics for relatively long-lived radionuclides [341]. In on-line systems, the integration of PET imaging into the treatment environment poses geometric constraints, conditioning the imaging performance. In off-line imaging, full-ring scanners are used, offering higher detection efficiency. However, transferring the patient to a separate system may cause alignment errors or isotope washout [337].
Future trends
In our opinion, future trends in the field of hybrid imaging relate to:
1. The development of new advanced imaging technologies that allow for more precise and accurate information, such as the use of faster ToF detectors, the design of total-body systems with very high sensitivity, the introduction of organ-specific systems with very high spatial resolution and new probes for contrast-enhanced PET/CT and PET/MR.
2. The development of advanced quantitative corrections for hybrid imaging data. This encloses accurate techniques for attenuation and scatter correction of PET and SPECT data in hybrid systems, more efficient multi-modality techniques for patient motion correction, as well as advanced and efficient methodologies for PVC of the lower resolution functional images.
3. The development of efficient and clinically viable multi-modality protocols and computational frameworks that can handle various large data cohorts. An essential feature of such integration efforts is the quantitative description of data properties, so that relationships in anatomical and functional domains between complementing modalities can be expressed in a mathematically concise way. Thus, taking advantage of high-level integration schemes, quantitative results are combined into data structures that provide a consistent framework for the application of both machine learning and advanced data mining techniques.
Conclusion
Hybrid imaging is the physical combination of complementary anato-metabolic imaging modalities, and, to date, relates mainly to combined SPECT/CT, PET/CT, and PET/MR imaging, which are available for clinical use and small-animal research. Hybrid imaging is supported by continuous cross-specialty efforts on integrating hardware components and leveraging complementary signals for improved image quality and quantitative accuracy of non-invasive assessments of the patients and subjects.
To date, multiple evidence exists for the benefits of hybrid imaging that extend from increased diagnostic accuracy, faster examinations to cross-fertilization of know-how from formerly distinct professional groups.
Hybrid imaging is able to provide unique information on diseases that—when pooled in larger-scale studies—can be utilized in a “big data” approach to design automated, computer-based models for disease and therapy response prediction, both of which benefit also from ongoing technological and methodological improvements of hybrid imaging systems.
Hybrid imaging invites stakeholders from a variety of scientific disciplines in an effort to provide diagnostic means that help us understand the complex biological processes involved in a disease better and, thus, help patients today and in the years to come.
Author contributions
JC-G collected the data and wrote sections Introduction, Basic Concepts of Hybrid Imaging, Detector Technology for CT, SPECT and PET, Image Acquisition and Reconstruction in CT, PET, and SPECT; Quantitative Data Corrections in PET and SPECT; Anatomically-Driven PET/SPECT Image Reconstruction; Partial Volume Correction in PET and SPECT; and Multi-Tracer PET and SPECT Imaging. He also merged and revised all the individual contributions from the other authors and prepared the full version of the manuscript with all figures and tables. IR wrote sections Time-of-Flight PET; Spiral PET, Continuous Table Motion; Novel MR-Based Attenuation Correction (AC) Methods for PET/MR; and Multi-Center Standardization. LS wrote sections Organ-Specific System Design and Total-Body Systems; Kinetic Modeling and Image Derived Input Function (IDIF); and prepared all the figures of the manuscript. ML wrote sections Motion Compensation and Multi- Parametric Imaging (MPI). OM wrote section Outlook and Future Trends. EM wrote sections MR Technology, Image Acquisition and Reconstruction in MR, and contributed to others. LP wrote sections In vivo Disease Characterization and Image-Derived Prediction Models. TB conceived the topic, prepared the abstract and conclusions from the full version of the manuscript and revised all the individual contributions. All authors participated in manuscript review.
Conflict of interest statement
The authors declare that the research was conducted in the absence of any commercial or financial relationships that could be construed as a potential conflict of interest.
Acknowledgments
The authors would like to thank Miklos Kovacs for the useful discussions about SPECT systems and configurations, Claes N. Ladefoged for kindly providing us with the image data for Figure 6 and Jun Bao for providing us with the technical specifications of the uMI PET/CT systems.
Footnotes
1. ^ Siemens Healthineers (Erlangen, Germany) obtained both, CE labeling and FDA approval for their 7 T Terra system in 2017.
2. ^ Papp et al. Personalizing medicine through hybrid imaging and medical big data analysis.
References
1. Friedland GW, Thurber BD. The birth of CT. AJR Am J Roentgenol. (1996) 167:1365–1370. doi: 10.2214/ajr.167.6.8956560
2. Rinck PA. Magnetic Resonance in Medicine: The Basic Textbook of the European Magnetic Resonance Forum. 12th revised and enlarged edition. Hoboken, NJ: Blackwell Scientific Publications (2018). Available online at: www.magnetic-resonance.org
3. Moser E, Laistler E, Schmitt F, Kontaxis G. Ultra-high field NMR and MRI—the role of magnet technology to increase sensitivity and specificity. Front Phys. (2017) 5:33. doi: 10.3389/fphy.2017.00033
4. Beyer T, Freudenberg LS, Townsend DW, Czernin J. The future of hybrid imaging—part 1: hybrid imaging technologies and SPECT/CT. Insights Imaging (2011) 2:161–9. doi: 10.1007/s13244-010-0063-2
5. Beyer T, Townsend DW, Czernin J, Freudenberg LS. The future of hybrid imaging—part 2: PET/CT. Insights Imaging. (2011) 2:225–34. doi: 10.1007/s13244-011-0069-4
6. Beyer T, Freundenberg LS, Czernin J, Townsend DW. The future of hybrid imaging-part 3: Pet/mr, small-animal imaging and beyond. Insights Imaging (2011) 3:189. doi: 10.1007/s13244-011-0136-x
7. Jaszczak RJ. The early years of single photon emission computed tomography (SPECT): an anthology of selected reminiscences. Phys Med Biol. (2006) 51:R99–115. doi: 10.1088/0031-9155/51/13/R07
8. Nutt R. The history of positron emission tomography. Mol Imaging Biol. (2002) 4:11–26. doi: 10.1016/S1095-0397(00)00051-0
9. Wahl RL, Quint LE, Cieslak RD, Aisen AM, Koeppe RA, Meyer CR. “Anatometabolic” tumor imaging: fusion of FDG PET with CT or MRI to localize foci of increased activity. J Nucl Med. (1993) 34:1190–7.
10. Hutton BF, Braun M. Software for image registration: algorithms, accuracy, efficacy. Semin Nucl Med. (2003) 33:180–92. doi: 10.1053/snuc.2003.127309
11. Slomka PJ, Baum RP. Multimodality image registration with software: state-of-the-art. Eur J Nucl Med Mol Imaging (2009) 36:44–55. doi: 10.1007/s00259-008-0941-8.
12. Blankespoor SC, Wu X, Kalki K, Brown JK, Cann CE, Hasegawa BH. Attenuation correction of SPECT using X-ray CT on an emission-transmission CT system: myocardial perfusion assessment. In: IEEE Nuclear Science Symposium and Medical Imaging Conference Record. Vol 2. IEEE (1995). p. 1126–30.
13. Beyer T, Townsend DW, Brun T, Kinahan PE, Charron M, Roddy R. A combined PET/CT scanner for clinical oncology. J Nucl Med. (2000) 41:1369–79.
14. Lang TF, Hasegawa BH, Liew SC, Brown JK, Blankespoor SC, Reilly SM. Description of a prototype emission-transmission computed tomography imaging system. J Nucl Med. (1992) 33:1881–7.
15. LaCroix KJ, Tsui BMW, Hasegawa BH, Brown JK. Investigation of the use of X-ray CT images for attenuation compensation in SPECT. IEEE Trans Nucl Sci. (1994) 41:2793–9. doi: 10.1109/23.340649
16. Townsend DW. Multimodality imaging of structure and function. Phys Med Biol. (2008) 53:R1–39. doi: 10.1088/0031-9155/53/4/R01
17. Kinahan PE, Hasegawa BH, Beyer T. X-ray-based attenuation correction for positron emission tomography/computed tomography scanners. Semin Nucl Med. (2003) 33:166–79. doi: 10.1053/snuc.2003.127307
18. Ritt P, Sanders J, Kuwert T. SPECT/CT technology. Clin Transl Imaging (2014) 2:445–57. doi: 10.1007/s40336-014-0086-7
19. Rausch I, Cal-González J, Dapra D, Gallowitsch HJ, Lind P, Beyer T. Performance evaluation of the Biograph mCT Flow PET/CT system according to the NEMA NU2-2012 standard. EJNMMI Phys. (2015) 2:26. doi: 10.1186/s40658-015-0132-1
20. Jakoby BW, Bercier Y, Conti M, Casey ME, Bendriem B, Townsend DW. Physical and clinical performance of the mCT time-of-flight PET/CT scanner. Phys Med Biol. (2011) 56:2375–89. doi: 10.1088/0031-9155/56/8/004
21. Kolthammer JA, Su K-H, Grover A, Narayanan M, Jordan DW, Muzic RF. Performance evaluation of the Ingenuity TF PET/CT scanner with a focus on high count-rate conditions. Phys Med Biol. (2014) 59:3843–59. doi: 10.1088/0031-9155/59/14/3843
22. Miller M, Zhang J, Binzel K, Griesmer J, Laurence T, Narayanan M, et al. Characterization of the vereos digital photon counting PET system. J Nucl Med. (2015) 56(Suppl. 3):434.
23. Bettinardi V, Presotto L, Rapisarda E, Picchio M, Gianolli L, Gilardi MC. Physical performance of the new hybrid PET/CT Discovery-690. Med Phys. (2011) 38:5394. doi: 10.1118/1.3635220
24. Reynés-Llompart G, Gámez-Cenzano C, Romero-Zayas I, Rodríguez-Bel L, Vercher-Conejero JL, Martí-Climent JM. Performance characteristics of the whole-body discovery IQ PET/CT system. J Nucl Med. (2017) 58:1155–61. doi: 10.2967/jnumed.116.185561
25. Kaneta T, Ogawa M, Motomura N, Iizuka H, Arisawa T, Hino-Shishikura A. Initial evaluation of the Celesteion large-bore PET/CT scanner in accordance with the NEMA NU2-2012 standard and the Japanese guideline for oncology FDG PET/CT data acquisition protocol version 2.0. EJNMMI Res. (2017) 7:83. doi: 10.1186/s13550-017-0331-y
26. Montgomery DW, Amira A, Zaidi H. Performance evaluation of a new high-sensitivity TOF clinical PET/CT system. J Nucl Med. (2015) 56:433–3.
27. Xu B, Liu C, Dong Y, Tang R, Liu Y, Yang H, et al. Performance evaluation of a high-resolution TOF clinical PET/CT. J Nucl Med. (2016) 57:202.
28. Delso G, Fürst S, Jakoby B, Ladebeck R, Ganter C, Nekolla SG. Performance measurements of the siemens mMR integrated whole-body PET/MR scanner. J Nucl Med. (2011) 52:1914–22. doi: 10.2967/jnumed.111.092726
29. Zaidi H, Ojha N, Morich M, Griesmer J, Hu Z, Maniawski P. Design and performance evaluation of a whole-body Ingenuity TF PET-MRI system. Phys Med Biol. (2011) 56:3091–106. doi: 10.1088/0031-9155/56/10/013
30. Grant AM, Deller TW, Khalighi MM, Maramraju SH, Delso G, Levin CS. NEMA NU 2-2012 performance studies for the SiPM-based ToF-PET component of the GE SIGNA PET/MR system. Med Phys. (2016) 43:2334–43. doi: 10.1118/1.4945416
31. Mirshanov DM. Transmission-Emission Computer Tomograph (1987). USSR Patent No. 621.386:616-07320.01.87-SU-181935.
32. Seret A, Nguyen D, Bernard C. Quantitative capabilities of four state-of-the-art SPECT-CT cameras. EJNMMI Res. (2012) 2:45. doi: 10.1186/2191-219X-2-45
33. Buck AK, Nekolla S, Ziegler S, Beer A, Krause BJ, Herrmann K, et al. SPECT/CT. J Nucl Med. (2008) 49:1305–19. doi: 10.2967/jnumed.107.050195
35. Slomka PJ, Berman DS, Germano G. New cardiac cameras: single-photon emission CT and PET. Semin Nucl Med. (2014) 44:232–51. doi: 10.1053/j.semnuclmed.2014.04.003
36. Suzuki A, Takeuchi W, Ishitsu T, Tsuchiya K, Morimoto Y, Ueno Y. High-sensitivity brain SPECT system using cadmium telluride (CdTe) semiconductor detector and 4-pixel matched collimator. Phys Med Biol. (2013) 58:7715–31. doi: 10.1088/0031-9155/58/21/7715
37. Tan J-W, Cai L, Meng L-J. A prototype of the MRI-compatible ultra-high resolution SPECT for in vivo mice brain imaging. In: 2009 IEEE Nuclear Science Symposium Conference Record (NSS/MIC). IEEE (2009). p. 2800–5.
38. Mariani G, Bruselli L, Kuwert T, Kim EE, Flotats A, Israel O. A review on the clinical uses of SPECT/CT. Eur J Nucl Med Mol Imaging (2010) 37:1959–85. doi: 10.1007/s00259-010-1390-8
39. Bhargava P, He G, Samarghandi A, Delpassand ES. Pictorial review of SPECT/CT imaging applications in clinical nuclear medicine. Am J Nucl Med Mol Imaging (2012) 2:221–31.
40. Ahmadzadehfar H, Biersack H (eds.). Clinical Applications of SPECT-CT. Berlin; Heidelberg: Springer-Verlag (2014).
41. Ljungberg M, Pretorius PH. SPECT/CT: an update on technological developments and clinical applications. Br J Radiol. (2018) 91:20160402. doi: 10.1259/bjr.20160402
42. Townsend DW, Beyer T, Blodgett TM. PET/CT scanners: a hardware approach to image fusion. Semin Nucl Med. (2003) 33:193–204. doi: 10.1053/snuc.2003.127314
43. Czernin J, Allen-Auerbach M, Schelbert HR. Improvements in cancer staging with PET/CT: literature-based evidence as of September 2006. J Nucl Med. (2007) 48(Suppl 1):78S−88S.
44. Walrand S, Hesse M, Jamar F. Update on novel trends in PET/CT technology and its clinical applications. Br J Radiol. (2018) 91:20160534. doi: 10.1259/bjr.20160534
45. Herzog H, Lerche C. Advances in clinical PET/MRI instrumentation. PET Clin. (2016) 11:95–103. doi: 10.1016/j.cpet.2015.09.001
46. Vandenberghe S, Marsden PK. PET-MRI: a review of challenges and solutions in the development of integrated multimodality imaging. Phys Med Biol. (2015) 60:R115–54. doi: 10.1088/0031-9155/60/4/R115
47. Zaidi H, Del Guerra A. An outlook on future design of hybrid PET/MRI systems. Med Phys. (2011) 38:5667–89. doi: 10.1118/1.3633909
48. Cabello J, Ziegler SI. Advances in PET/MR instrumentation and image reconstruction. Br J Radiol. (2018) 91:20160363. doi: 10.1259/bjr.20160363
49. Pichler BJ, Judenhofer MS, Catana C, Walton JH, Kneilling M, Nutt RE. Performance test of an LSO-APD detector in a 7-T MRI scanner for simultaneous PET/MRI. J Nucl Med. (2006) 47:639–47.
50. Spanoudaki VC, Mann AB, Otte AN, Konorov I, Torres-Espallardo I, Paul S, et al. Use of single photon counting detector arrays in combined PET/MR: characterization of LYSO-SiPM detector modules and comparison with a LSO-APD detector. J Instrum. (2007) 2:P12002. doi: 10.1088/1748-0221/2/12/P12002
51. Judenhofer MS, Wehrl HF, Newport DF, Catana C, Siegel SB, Becker M. Simultaneous PET-MRI: a new approach for functional and morphological imaging. Nat Med. (2008) 14:459–65. doi: 10.1038/nm1700
52. Spanoudaki VC, Levin CS. Photo-detectors for time of flight positron emission tomography (ToF-PET). Sensors (2010) 10:10484–505. doi: 10.3390/s101110484
53. de Jong M, Essers J, van Weerden WM. Imaging preclinical tumour models: improving translational power. Nat Rev Cancer. (2014) 14:481–93. doi: 10.1038/nrc3751
54. Meikle SR, Kench P, Kassiou M, Banati RB. Small animal SPECT and its place in the matrix of molecular imaging technologies. Phys Med Biol. (2005) 50:R45–61. doi: 10.1088/0031-9155/50/22/R01
55. Lee T-S, Rittenbach A, Fernandez CG, Lopez-Longas J, Arco JM, Tsui BMW. Initial evaluation of a state-of-the-art commercial preclinical PET/CT scanner. In: 2016 IEEE Nuclear Science Symposium, Medical Imaging Conference and Room-Temperature Semiconductor Detector Workshop (NSS/MIC/RTSD). Strasbourg: IEEE (2016). p. 1–4.
56. van der Have F, Vastenhouw B, Ramakers RM, Branderhorst W, Krah JO, Ji C. U-SPECT-II: an ultra-high-resolution device for molecular small-animal imaging. J Nucl Med. (2009) 50:599–605. doi: 10.2967/jnumed.108.056606
57. Goorden MC, van der Have F, Kreuger R, Ramakers RM, Vastenhouw B, Burbach JP. VECTor: a preclinical imaging system for simultaneous submillimeter SPECT and PET. J Nucl Med. (2013) 54:306–12. doi: 10.2967/jnumed.112.109538
58. Walker MD, Goorden MC, Dinelle K, Ramakers RM, Blinder S, Shirmohammad M. Performance assessment of a preclinical PET scanner with pinhole collimation by comparison to a coincidence-based small-animal PET scanner. J Nucl Med. (2014) 55:1368–74. doi: 10.2967/jnumed.113.136663
59. Magota K, Kubo N, Kuge Y, Nishijima K-I, Zhao S, Tamaki N. Performance characterization of the Inveon preclinical small-animal PET/SPECT/CT system for multimodality imaging. Eur J Nucl Med Mol Imaging. (2011) 38:742–752. doi: 10.1007/s00259-010-1683-y
60. Larsson Åkerman L. A Technical Validation of The PET/SPECT/CT (Triumph) Scanner. (2011). Available online at: https://uu.diva-portal.org/smash/get/diva2:407708/FULLTEXT01.pdf.
61. Kuntner C, Stout D. Quantitative preclinical PET imaging: opportunities and challenges. Front Phys. (2014) 2:12. doi: 10.3389/fphy.2014.00012
62. Binderup T, El-Ali H, Ambrosini V, Skovgaard D, Jensen MM, Li F, et al. Molecular imaging with small animal PET/CT. Curr Med Imaging Rev. (2011) 7:234–7. doi: 10.2174/157340511796411221
63. Goertzen AL, Bao Q, Bergeron M, Blankemeyer E, Blinder S, Cañadas M. NEMA NU 4-2008 comparison of preclinical pet imaging systems. J Nucl Med. (2012) 53:1300–9. doi: 10.2967/jnumed.111.099382
64. Khalil MM, Tremoleda JL, Bayomy TB, Gsell W. Molecular SPECT imaging: an overview. Int J Mol Imaging (2011) 2011:1–15. doi: 10.1155/2011/796025
65. Wehrl HF, Judenhofer MS, Wiehr S, Pichler BJ. Pre-clinical PET/MR: technological advances and new perspectives in biomedical research. Eur J Nucl Med Mol Imaging (2009) 36:56–68. doi: 10.1007/s00259-009-1078-0
66. Huang SC, Hoffman EJ, Phelps ME, Kuhl DE. Quantitation in positron emission computed tomography: 2. Effects of inaccurate attenuation correction. J Comput Assist Tomogr. (1979) 3:804–14. doi: 10.1097/00004728-197903060-00018
67. Fletcher JW, Kinahan PE. PET/CT Standardized uptake values (SUVs) in clinical practice and assessing response to therapy. NIH Public Access. (2010) 31:496–505. doi: 10.1053/j.sult.2010.10.001.PET/CT
68. Wahl RL, Jacene H, Kasamon Y, Lodge MA. From RECIST to PERCIST: evolving considerations for PET response criteria in solid tumors. J Nucl Med. (2009) 50(Suppl. 1):122S−50S. doi: 10.2967/jnumed.108.057307
69. Yankeelov TE, Abramson RG, Quarles CC. Quantitative multimodality imaging in cancer research and therapy. Nat Rev Clin Oncol. (2014) 11:670–80. doi: 10.1038/nrclinonc.2014.134
70. Kotasidis FA, Tsoumpas C, Rahmim A. Advanced kinetic modelling strategies: towards adoption in clinical PET imaging. Clin Transl Imaging (2014) 2:219–37. doi: 10.1007/s40336-014-0069-8
71. Lammertsma AA. Forward to the past: the case for quantitative PET imaging. J Nucl Med. (2017) 58:1019–24. doi: 10.2967/jnumed.116.188029
72. Bailey DL, Willowson KP. An evidence-based review of quantitative SPECT imaging and potential clinical applications. J Nucl Med. (2013) 54:83–9. doi: 10.2967/jnumed.112.111476
73. Wernick MN, Aarsvold JN. Emission Tomography : The Fundamentals of PET and SPECT. Elsevier Academic Press (2004). doi: 10.1016/B978-0-12-744482-6.50030-2
74. Casey ME, Nutt R. A Multicrystal two dimensional BGO detector system for positron emission tomography. IEEE Trans Nucl Sci. (1986) 33:460–3. doi: 10.1109/TNS.1986.4337143
75. Joung J, Miyaoka RS, Lewellen TK. cMiCE:a high resolution animal PET using continuous LSO with a statistics based positioning scheme. In: IEEE Nuclear Science Symposium Conference Record (Cat. No.01CH37310). IEEE (2001). p. 1137–41.
76. Carles M, Lerche CW, Sánchez F, Mora F, Benlloch JM. Position correction with depth of interaction information for a small animal PET system. Nucl Instruments Methods Phys Res Sect A Accel Spectrometers, Detect Assoc Equip. (2011) 648:S176–80. doi: 10.1016/j.nima.2010.11.192
77. Lewellen TK. Recent developments in PET detector technology. Phys Med Biol. (2008) 53:R287–317. doi: 10.1088/0031-9155/53/17/R01
78. Madsen MT. Recent advances in SPECT imaging. J Nucl Med. (2007) 48:661–73. doi: 10.2967/jnumed.106.032680
79. Lecomte R. Novel detector technology for clinical PET. Eur J Nucl Med Mol Imaging (2009) 36:69–85. doi: 10.1007/s00259-008-1054-0
80. Peterson TE, Furenlid LR. SPECT detectors: the Anger Camera and beyond. Phys Med Biol. (2011) 56:R145–82. doi: 10.1088/0031-9155/56/17/R01
81. Shefer E, Altman A, Behling R, Goshen R, Gregorian L, Roterman Y, et al. State of the art of CT detectors and sources: a literature review. Curr Radiol Rep. (2013) 1:76–91. doi: 10.1007/s40134-012-0006-4
82. Lecoq P. Development of new scintillators for medical applications. Nucl Instruments Methods Phys Res Sect A Accel Spectrometers Detect Assoc Equip. (2016) 809:130–9. doi: 10.1016/j.nima.2015.08.041
83. Vaquero JJ, Sánchez JJ, Udías JM, Cal-González J, Desco M. MRI compatibility of position-sensitive photomultiplier depth-of-interaction PET detectors modules for in-line multimodality preclinical studies. Nucl Instruments Methods Phys Res Sect A Accel Spectrometers Detect Assoc Equip. (2013) 702:83–7. doi: 10.1016/j.nima.2012.08.046
84. Degenhardt C, Prescher G, Frach T, Thon A, de Gruyter R, Schmitz A, et al. The digital silicon photomultiplier - a novel sensor for the detection of scintillation light. In: IEEE Nuclear Science Symposium Conference Record. Orlando, FL (2009). p. 2383–6.
85. Frach T, Prescher G, Degenhardt C, De Gruyter R, Schmitz A, Ballizany R. The digital silicon photomultiplier - principle of operation and intrinsic detector performance. IEEE Nucl Sci Symp Conf Rec. (2009). p.1959–65.
86. Degenhardt C, Zwaans B, Frach T, de Gruyter R. Arrays of digital Silicon Photomultipliers—intrinsic performance and application to scintillator readout. In: IEEE Nuclear Science Symposuim and Medical Imaging Conference. Knowville, TN: IEEE (2010). p. 1954–6.
87. Düppenbecker PM, Weissler B, Gebhardt P, Schug D, Wehner J, Marsden PK. Development of an MRI-compatible digital SiPM detector stack for simultaneous PET/MRI. Biomed Phys Eng Express. (2016) 2:15010. doi: 10.1088/2057-1976/2/1/015010
88. Chmeissani M, Kolstein M, Gabriel Macias-Montero J, Puigdengoles C, García J, Prats X. First results of a highly granulated 3D CdTe detector module for PET. Phys Med Biol. (2018) 63:25032. doi: 10.1088/1361-6560/aaa44c
89. Conti M. Focus on time-of-flight PET: the benefits of improved time resolution. Eur J Nucl Med Mol Imaging (2011) 38:1147–57. doi: 10.1007/s00259-010-1711-y
90. Surti S, Karp JS. Advances in time-of-flight PET. Phys Med. (2016) 32:12–22. doi: 10.1016/j.ejmp.2015.12.007
91. Vandenberghe S, Mikhaylova E, D'Hoe E, Mollet P, Karp JS. Recent developments in time-of-flight PET. EJNMMI Phys. (2016) 3:3. doi: 10.1186/s40658-016-0138-3
92. Lecoq P. Pushing the limits in time-of-flight PET imaging. IEEE Trans Radiat Plasma Med Sci. (2017) 1:473–85. doi: 10.1109/TRPMS.2017.2756674
93. Gundacker S, Acerbi F, Auffray E, Ferri A, Gola A, Nemallapudi MV, et al. State of the art timing in TOF-PET detectors with LuAG, GAGG and L(Y)SO scintillators of various sizes coupled to FBK-SiPMs. J Instrum. (2016) 11:P08008. doi: 10.1088/1748-0221/11/08/P08008
94. Nemallapudi MV, Gundacker S, Lecoq P, Auffray E, Ferri A, Gola A. Sub-100 ps coincidence time resolution for positron emission tomography with LSO:Ce codoped with Ca. Phys Med Biol. (2015) 60:4635–49. doi: 10.1088/0031-9155/60/12/4635
95. Dahlbom M, Yu D-C, Cherry SR, Chatziioannou A, Hoffman EJ. Methods for improving image quality in whole body PET scanning. IEEE Trans Nucl Sci. (1992) 39:1079–83. doi: 10.1109/23.159763
96. Dahlbom M, Reed J, Young J. Implementation of true continuous bed motion in 2-D and 3-D whole-body PET scanning. IEEE Trans Nucl Sci. (2001) 48:1465–9. doi: 10.1109/23.958381
97. Brasse D, Newport D, Carney JP, Yap J, Reynolds C, Reed J, et al. Continuous bed motion acquisition on a whole body combined PET/CT system. In: EEE Nuclear Science Symposium Conference Record. Vol 2. Norfolk, VA: IEEE (2002). p. 951–5.
98. Townsend DW, Reed J, Newport DF, Carney JPJ, Tolbert SH, Newby D, et al. Continuous bed motion acquisition for an LSO PET/CT scanner. In: IEEE Symposium Conference Record Nuclear Science. Vol 4. Rome: IEEE (2004). p. 2383–7.
99. Karakatsanis NA, Zhou Y, Lodge MA, Casey ME, Wahl RL, Zaidi H. Generalized whole-body Patlak parametric imaging for enhanced quantification in clinical PET. Phys Med Biol. (2015) 60:8643–73. doi: 10.1088/0031-9155/60/22/8643
100. Wienhard K, Schmand M, Casey ME, Baker K, Bao J, Eriksson L, et al. The ECAT HRRT: performance and first clinical application of the new high resolution research tomograph. IEEE Trans Nucl Sci. (2002) 49:104. doi: 10.1109/TNS.2002.998689
101. Majewski S, Proffitt J, Brefczynski-Lewis J, Stolin A, Weisenberger AG, Xi W, et al. HelmetPET: a silicon photomultiplier based wearable brain imager. In: IEEE Nuclear Science Symposium Conference Record. Valencia (2011). p. 4030–4.
102. Yamamoto S, Honda M, Oohashi T, Shimizu K, Senda M. Development of a brain PET system, PET-Hat: a wearable PET system for brain research. IEEE Trans Nucl Sci. (2011) 58(3 Pt 1):668–73. doi: 10.1109/TNS.2011.2105502
103. Tashima H, Yamaya T. Proposed helmet PET geometries with add-on detectors for high sensitivity brain imaging. Phys Med Biol. (2016) 61:7205–20. doi: 10.1088/0031-9155/61/19/7205
104. Kolb A, Wehrl HF, Hofmann M, Judenhofer MS, Eriksson L, Ladebeck R. Technical performance evaluation of a human brain PET/MRI system. Eur Radiol. (2012) 22:1776–88. doi: 10.1007/s00330-012-2415-4
105. González AJ, Majewski S, Sánchez F, Aussenhofer S, Aguilar A, Conde P, et al. The MINDView brain PET detector, feasibility study based on SiPM arrays. Nucl Instruments Methods Phys Res Sect A Accel Spectrometers Detect Assoc Equip. (2016) 818:82–90. doi: 10.1016/j.nima.2016.02.046
106. Ravindranath B, Junnarkar SS, Purschke ML, Maramraju S, Hong X, Tomasi D, et al. Results from prototype II of the BNL simultaneous PET-MRI dedicated breast scanner. In: IEEE Nuclear Science Symposium Conference Record. Orlando, FL (2009). p. 3315–7. doi: 10.1109/NSSMIC.2009.5401742
107. Varela J. EndoTOFPET-US: multi-modal endoscope for ultrasound and time of flight PET. In: 2014 IEEE Nuclear Science Symposium and Medical Imaging Conference, NSS/MIC 2014. Seattle, WA (2016).
108. Crosetto DB. A modular VME or IBM PC based data acquisition system for multi-modality PET/CT scanners of different sizes and detector types. Nucl Sci Symp Conf Rec 2000 IEEE. (2000) 2:12/78–12/97. doi: 10.1109/NSSMIC.2000.949946
109. Cherry SR, Badawi RD, Karp JS, Moses WW, Price P, Jones T. Total-body imaging: transforming the role of positron emission tomography. Sci Transl Med. (2017) 9:eaaf6169. doi: 10.1126/scitranslmed.aaf6169
110. Cherry SR, Jones T, Karp JS, Qi J, Moses W, Badawi R. Total-body PET: maximizing sensitivity to create new opportunities for clinical research and patient care. J Nucl Med. (2018) 59:3–12. doi: 10.2967/jnumed.116.184028
111. Eriksson L, Townsend D, Conti M, Eriksson M, Rothfuss H, Schmand M, et al. An investigation of sensitivity limits in PET scanners. Nucl Instrum Methods Phys Res Sect A Accel Spectrometers Detect Assoc Equip. (2007) 580:836–42. doi: 10.1016/j.nima.2007.06.112
112. Zhang X, Zhou J, Cherry SR, Badawi RD, Qi J. Quantitative image reconstruction for total-body PET imaging using the 2-meter long EXPLORER scanner. Phys Med Biol. (2017) 62:2465–85. doi: 10.1088/1361-6560/aa5e46
113. Karakatsanis NA, Garibotto V, Rager O, Zaidi H. Continuous bed motion Vs. step-and-shoot acquisition on clinical whole-body dynamic and parametric PET imaging. In: 2015 IEEE Nuclear Science Symposium and Medical Imaging Conference, NSS/MIC 2015. San Diego, CA (2016).
114. Karakatsanis NA, Lodge MA, Tahari AK, Zhou Y, Wahl RL, Rahmim A. Dynamic whole-body PET parametric imaging: I. Concept, acquisition protocol optimization and clinical application. Phys Med Biol. (2013) 58:7391–418. doi: 10.1088/0031-9155/58/20/7391
115. Randall I. Total Body PET Scanner Targets Paediatric Patients. MedicalPhysicsWeb. Available online at http://medicalphysicsweb.org/cws/article/research/69657 (Accessed November 14, 2017).
116. Martins PM, Crespo P, Couceiro M, Ferreira NC, Marques RF, Seco J, et al. Fast full-body reconstruction for a functional human RPC-PET imaging system using list-mode simulated data and its applicability to radiation oncology and radiology. arXiv. 2017:1706.07075.
117. Moser E, Stahlberg F, Ladd ME, Trattnig S. 7-T MR-from research to clinical applications? NMR Biomed. (2012) 25:695–716. doi: 10.1002/nbm.1794
118. Moser E, Stadlbauer A, Windischberger C, Quick HH, Ladd ME. Magnetic resonance imaging methodology. Eur J Nucl Med Mol Imaging (2009) 36:30–41. doi: 10.1007/s00259-008-0938-3
119. Le Bihan D, Mangin JF, Poupon C, Clark CA, Pappata S, Molko N. Diffusion tensor imaging: concepts and applications. J Magn Reson Imaging (2001) 13:534–46. doi: 10.1002/jmri.1076
120. Sander CY, Keil B, Chonde DB, Rosen BR, Catana C, Wald LL. A 31-channel MR brain array coil compatible with positron emission tomography. Magn Reson Med. (2015) 73:2363–75. doi: 10.1002/mrm.25335
121. Pruessmann KP, Weiger M, Scheidegger MB, Boesiger P. SENSE: sensitivity encoding for fast MRI. Magn Reson Med. (1999) 42:952–62.
122. Griswold MA, Jakob PM, Heidemann RM, Nittka M, Jellus V, Wang J. Generalized autocalibrating partially parallel acquisitions (GRAPPA). Magn Reson Med. (2002) 47:1202–10. doi: 10.1002/mrm.10171
123. Larkman DJ, Nunes RG. Parallel magnetic resonance imaging. Phys Med Biol. (2007) 52:R15–55. doi: 10.1088/0031-9155/52/7/R01
124. Alessio A, Kinahan P. PET image reconstruction. Nucl Med. (2006) 1:1–22. doi: 10.1088/0031-9155/54/12/007.Iterative
125. Hsieh J, Nett B, Yu Z, Sauer K, Thibault J-B, Bouman CA. Recent advances in CT image reconstruction. Curr Radiol Rep. (2013) 1:39–51. doi: 10.1007/s40134-012-0003-7
126. Geyer LL, Schoepf UJ, Meinel FG, Nance JW, Bastarrika G, Leipsic JA. State of the art: iterative CT reconstruction techniques. Radiology. (2015) 276:339–57. doi: 10.1148/radiol.2015132766
128. Feldkamp LA, Davis LC, Kress JW. Practical cone-beam algorithm. J Opt Soc Am A. (1984) 1:612–9. doi: 10.1364/JOSAA.1.000612
129. Katsevich A. A general scheme for constructing inversion algorithms for cone beam CT. Int J Math Math Sci. (2003) 2003:1305–21. doi: 10.1155/S0161171203209315
130. Zhuang T, Leng S, Nett BE, Chen G-H. Fan-beam and cone-beam image reconstruction via filtering the backprojection image of differentiated projection data. Phys Med Biol. (2004) 49:5489–503. doi: 10.1088/0031-9155/49/24/007
131. Pack JD, Noo F, Clackdoyle R. Cone-beam reconstruction using the backprojection of locally filtered projections. IEEE Trans Med Imaging (2005) 24:70–85. doi: 10.1109/TMI.2004.837794
132. Grimmer R, Oelhafen M, Elstrøm U, Kachelrieß M. Cone-beam CT image reconstruction with extended z range. Med Phys. (2009) 36:3363–70. doi: 10.1118/1.3148560
133. Katsevich A. Theoretically exact filtered backprojection-type inversion algorithm for spiral CT. SIAM J Appl Math. (2002) 62:2012–26. doi: 10.1137/S0036139901387186
134. Tang X, Hsieh J, Nilsen RA, Dutta S, Samsonov D, Hagiwara A. A three-dimensional-weighted cone beam filtered backprojection (CB-FBP) algorithm for image reconstruction in volumetric CT—helical scanning. Phys Med Biol. (2006) 51:855–74. doi: 10.1088/0031-9155/51/4/007
135. Beister M, Kolditz D, Kalender WA. Iterative reconstruction methods in X-ray CT. Phys Medica. (2012) 28:94–108. doi: 10.1016/j.ejmp.2012.01.003
136. Gordon R, Bender R, Herman GT. Algebraic reconstruction techniques (ART) for three-dimensional electron microscopy and x-ray photography. J Theor Biol. (1970) 29:471–81. doi: 10.1016/0022-5193(70)90109-8
137. Andersen AH, Kak AC. Simultaneous algebraic reconstruction technique (SART): a superior implementation of the art algorithm. Ultrason Imaging (1984) 6:81–94. doi: 10.1177/016173468400600107
138. Gilbert P. Iterative methods for the three-dimensional reconstruction of an object from projections. J Theor Biol. (1972) 36:105–17. doi: 10.1016/0022-5193(72)90180-4
139. Shepp LA, Vardi Y. Maximum likelihood reconstruction for emission tomography. IEEE Trans Med Imaging (1982) 1:113–22. doi: 10.1109/TMI.1982.4307558
140. Hudson HM, Larkin RS. Accelerated image reconstruction using ordered subsets of projection data. IEEE Trans Med Imaging (1994) 13:601–9. doi: 10.1109/42.363108
141. Thibault J-B, Sauer KD, Bouman CA, Hsieh J. A three-dimensional statistical approach to improved image quality for multislice helical CT. Med Phys. (2007) 34:4526–44. doi: 10.1118/1.2789499
142. Xu F, Xu W, Jones M, Keszthelyi B, Sedat J, Agard D. On the efficiency of iterative ordered subset reconstruction algorithms for acceleration on GPUs. Comput Methods Programs Biomed. (2010) 98:261–70. doi: 10.1016/j.cmpb.2009.09.003
143. Liang Z-P, Lauterbur PC. Principles of Magnetic Resonance Imaging : A Signal Processing Perspective. New York, NY: SPIE Optical Engineering Press (2000).
145. Willowson K, Bailey DL, Baldock C. Quantitative SPECT reconstruction using CT-derived corrections. Phys Med Biol. (2008) 53:3099–12. doi: 10.1088/0031-9155/53/12/002
146. Kinahan PE, Townsend DW, Beyer T, Sashin D. Attenuation correction for a combined 3D PET/CT scanner. Med Phys. (1998) 25:2046–53. doi: 10.1118/1.598392
147. Watson CC. New, faster, image-based scatter correction for 3D PET. IEEE Trans Nucl Sci. (2000) 47:1587–94. doi: 10.1109/23.873020
148. Watson CC, Casey ME, Michel C, Bendriem B. Advances in scatter correction for 3D PET/CT. IEEE Symp Conf Rec Nucl Sci 2004. (2004) 5:3–7. doi: 10.1109/NSSMIC.2004.1466317
149. Patton JA, Turkington TG. SPECT/CT physical principles and attenuation correction. J Nucl Med Technol. (2008) 36:1–10. doi: 10.2967/jnmt.107.046839
150. Jaszczak RJ, Greer KL, Floyd CE, Harris CC, Coleman RE. Improved SPECT quantification using compensation for scattered photons. J Nucl Med. (1984) 25:893–900.
151. Ogawa K, Harata Y, Ichihara T, Kubo A, Hashimoto S. A practical method for position-dependent Compton-scatter correction in single photon emission CT. IEEE Trans Med Imaging. (1991) 10:408–12. doi: 10.1109/42.97591
152. Zaidi H, Koral KF. Scatter modelling and compensation in emission tomography. Eur J Nucl Med Mol Imaging (2004) 31:761–82. doi: 10.1007/s00259-004-1495-z
153. Peterson M, Gustafsson J, Ljungberg M. Monte Carlo-based quantitative pinhole SPECT reconstruction using a ray-tracing back-projector. EJNMMI Phys. (2017) 4:32. doi: 10.1186/s40658-017-0198-z
154. Elschot M, Lam MGEH, van den Bosch MAAJ, Viergever MA, de Jong HWAM. Quantitative Monte Carlo-based 90Y SPECT reconstruction. J Nucl Med. (2013) 54:1557–63. doi: 10.2967/jnumed.112.119131
156. Badawi RD, Marsden PK. Developments in component-based normalization for 3D PET. Phys Med Biol. (1999) 44:571–94. doi: 10.1088/0031-9155/44/2/020
157. Guobao W, Jinyi Q. Penalized likelihood PET image reconstruction using patch-based edge-preserving regularization. IEEE Trans Med Imaging. (2012) 31:2194–204. doi: 10.1109/TMI.2012.2211378
158. Green PJ. Bayesian reconstructions from emission tomography data using a modified EM algorithm. IEEE Trans Med Imaging (1990) 9:84–93. doi: 10.1109/42.52985
159. Alessio AM, Kinahan PE. Improved quantitation for PET/CT image reconstruction with system modeling and anatomical priors. Med Phys. (2006) 33:4095–103. doi: 10.1118/1.2358198
160. Chun SY. The use of anatomical information for molecular image reconstruction algorithms: attenuation/scatter correction, motion compensation, and noise reduction. Nucl Med Mol Imaging (2010). (2016) 50:13–23. doi: 10.1007/s13139-016-0399-8
161. Bai B, Li Q, Leahy RM. Magnetic resonance-guided positron emission tomography image reconstruction. Semin Nucl Med. (2013) 43:30–44. doi: 10.1053/j.semnuclmed.2012.08.006
162. Hebert T, Leahy R. A generalized EM algorithm for 3-D Bayesian reconstruction from Poisson data using Gibbs priors. IEEE Trans Med Imaging. (1989) 8:194–202. doi: 10.1109/42.24868
163. Ardekani BA, Braun M, Hutton BF, Kanno I, Iida H. Minimum cross-entropy reconstruction of PET images using prior anatomical information. Phys Med Biol. (1996) 41:2497–517. doi: 10.1088/0031-9155/41/11/018
164. Soret M, Bacharach SL, Buvat I. Partial-volume effect in PET tumor imaging. J Nucl Med. (2007) 48:932–45. doi: 10.2967/jnumed.106.035774
165. Rousset O, Rahmim A, Alavi A, Zaidi H. Partial volume correction strategies in PET. PET Clin. (2007) 2:235–49. doi: 10.1016/j.cpet.2007.10.005
166. Erlandsson K, Buvat I, Pretorius PH, Thomas BA, Hutton BF. A review of partial volume correction techniques for emission tomography and their applications in neurology, cardiology and oncology. Phys Med Biol. (2012) 57:R119–59. doi: 10.1088/0031-9155/57/21/R119
167. Erlandsson K, Dickson J, Arridge S, Atkinson D, Ourselin S, Hutton BF. MR imaging–guided partial volume correction of PET data in PET/MR imaging. PET Clin. (2015) 11:161–77. doi: 10.1016/j.cpet.2015.09.002
168. Hoffman EJ, Huang SC, Phelps ME. Quantitation in positron emission computed tomography: 1. Effect of object size. J Comput Assist Tomogr. (1979) 3:299–308. doi: 10.1097/00004728-197906000-00001
169. Rousset OG, Ma Y, Evans AC. Correction for partial volume effects in PET: principle and validation. J Nucl Med. (1998) 39:904–11.
170. Müller-Gärtner HW, Links JM, Prince JL, Bryan RN, McVeigh E, Leal JP. Measurement of radiotracer concentration in brain gray matter using positron emission tomography: MRI-based correction for partial volume effects. J Cereb Blood Flow Metab. (1992) 12:571–83. doi: 10.1038/jcbfm.1992.81
171. Teo BK, Seo Y, Bacharach SL, Carrasquillo JA, Libutti SK, Shukla H. Partial-volume correction in PET: validation of an iterative postreconstruction method with phantom and patient data. J Nucl Med. (2007) 48:802–10. doi: 10.2967/jnumed.106.035576
172. Thomas BA, Erlandsson K, Modat M, Thurfjell L, Vandenberghe R, Ourselin S. The importance of appropriate partial volume correction for PET quantification in Alzheimer's disease. Eur J Nucl Med Mol Imaging. (2011) 38:1104–19.
173. Moore SC, Southekal S, Park MA, McQuaid SJ, Kijewski MF, Muller SP. Improved regional activity quantitation in nuclear medicine using a new approach to correct for tissue partial volume and spillover effects. IEEE Trans Med Imaging. (2012) 31:405–16. doi: 10.1109/TMI.2011.2169981
174. Southekal S, McQuaid SJ, Kijewski MF, Moore SC. Evaluation of a method for projection-based tissue-activity estimation within small volumes of interest. Phys Med Biol. (2012) 57:685–701. doi: 10.1088/0031-9155/57/3/685
175. Cal-González J, Moore SC, Park MA, Herraiz JL, Vaquero JJ, Desco M. Improved quantification for local regions of interest in preclinical PET imaging. Phys Med Biol. (2015) 60:7127–49. doi: 10.1088/0031-9155/60/18/7127
176. Cal-Gonzalez J, Li X, Heber D, Rausch I, Moore SC, Schäfers K. Partial volume correction for improved PET quantification in 18F-NaF imaging of atherosclerotic plaques. J Nucl Cardiol. (2017). doi: 10.1007/s12350-017-0778-2. [Epub ahead of print].
177. Panin VY, Kehren F, Michel C, Casey M. Fully 3-D PET reconstruction with system matrix derived from point source measurements. IEEE Trans Med Imaging (2006) 25:907–21. doi: 10.1109/TMI.2006.876171
178. Cysouw MCF, Kramer GM, Schoonmade LJ, Boellaard R, de Vet HCW, Hoekstra OS. Impact of partial-volume correction in oncological PET studies: a systematic review and meta-analysis. Eur J Nucl Med Mol Imaging (2017) 44:2105–16. doi: 10.1007/s00259-017-3775-4
179. Nehmeh SA, Erdi YE. Respiratory motion in positron emission tomography/computed tomography: a review. Semin Nucl Med. (2008) 38:167–76. doi: 10.1053/j.semnuclmed.2008.01.002
180. Gould KL, Pan T, Loghin C, Johnson NP, Guha A, Sdringola S. Frequent diagnostic errors in cardiac PET/CT due to misregistration of CT attenuation and emission PET images: a definitive analysis of causes, consequences, and corrections. J Nucl Med. (2007) 48:1112–21. doi: 10.2967/jnumed.107.039792
181. Rausch I, Quick HH, Cal-Gonzalez J, Sattler B, Boellaard R, Beyer T. Technical and instrumentational foundations of PET/MRI. Eur J Radiol. (2017) 94:A3–13. doi: 10.1016/j.ejrad.2017.04.004
182. Kesner AL, Chung JH, Lind KE, Kwak JJ, Lynch D, Burckhardt D. Validation of software gating: a practical technology for respiratory motion correction in PET. Radiology. (2016) 281:239–48. doi: 10.1148/radiol.2016152105
183. Lassen ML, Rasmussen T, Christensen TE, Kjær A, Hasbak P. Respiratory gating in cardiac PET: effects of adenosine and dipyridamole. J Nucl Cardiol. (2016) 24:1941–9. doi: 10.1007/s12350-016-0631-z
184. Olesen OV, Paulsen RR, Højgaard L, Roed B, Larsen R. Motion tracking for medical imaging: a nonvisible structured light tracking approach. IEEE Trans Med Imaging. (2012) 31:79–87. doi: 10.1109/TMI.2011.2165157
185. Kesner AL, Schleyer PJ, Büther F, Walter MA, Schäfers KP, Koo PJ. On transcending the impasse of respiratory motion correction applications in routine clinical imaging – a consideration of a fully automated data driven motion control framework. EJNMMI Phys. (2014) 1:8. doi: 10.1186/2197-7364-1-8
186. Grimm R, Fürst S, Souvatzoglou M, Forman C, Hutter J, Dregely I. Self-gated MRI motion modeling for respiratory motion compensation in integrated PET/MRI. Med Image Anal. (2014) 19:110–20. doi: 10.1016/j.media.2014.08.003
187. Munoz C, Kolbitsch C, Reader AJ, Marsden P, Schaeffter T, Prieto C. MR-based cardiac and respiratory motion-compensation techniques for PET-MR imaging. PET Clin. (2016) 11:179–91. doi: 10.1016/j.cpet.2015.09.004
188. Kesner AL. The relevance of data driven motion correction in diagnostic PET. Eur J Nucl Med Mol Imaging 44:2326–7. doi: 10.1007/s00259-017-3794-1
189. Kolbitsch C, Ahlman MA, Davies-Venn C, Evers R, Hansen M, Peressutti D. Cardiac and respiratory motion correction for simultaneous cardiac PET - MR. J Nucl Med. (2017) 58:846–52. doi: 10.2967/jnumed.115.171728
190. Munoz C, Neji R, Cruz G, Mallia A, Jeljeli S, Reader AJ. Motion-corrected simultaneous cardiac positron emission tomography and coronary MR angiography with high acquisition efficiency. Magn Reson Med. (2017) 79:339–50. doi: 10.1002/mrm.26690
191. Gillman A, Rose S, Smith J, Thomas P, Dowson N. PET motion correction in context of integrated PET/MR: current techniques, limitations, and future projections. Med Phys. (2017). 44:e430–45. doi: 10.1002/mp.12577.
192. Huang C, Petibon Y, Ouyang J, Reese TG, Ahlman MA, Bluemke DA. Accelerated acquisition of tagged MRI for cardiac motion correction in simultaneous PET-MR: phantom and patient studies. Med Phys. (2015) 42:1087–97. doi: 10.1118/1.4906247
193. Livieratos L, Stegger L, Bloomfield PM, Schafers K, Bailey DL, Camici PG. Rigid-body transformation of list-mode projection data for respiratory motion correction in cardiac PET. Phys Med Biol. (2005) 50:3313–22. doi: 10.1088/0031-9155/50/14/008
194. Rahmim A, Tang J, Zaidi H. Four-Dimensional image reconstruction strategies in cardiac-gated and respiratory-gated PET imaging. PET Clin. (2013) 8:51–67. doi: 10.1016/j.cpet.2012.10.005
195. Picard Y, Thompson CJ. Motion correction of PET images using multiple acquisition frames. IEEE Trans Med Imaging (1997) 16:137–44. doi: 10.1109/42.563659
196. Feng T, Wang J, Fung G, Tsui B. Non-rigid dual respiratory and cardiac motion correction methods after, during, and before image reconstruction for 4D cardiac PET. Phys Med Biol. (2016) 61:151–68. doi: 10.1088/0031-9155/61/1/151
197. Polycarpou I, Tsoumpas C, Marsden PK. Analysis and comparison of two methods for motion correction in PET imaging. Med Phys. (2012) 39:6474. doi: 10.1118/1.4754586
198. Fieseler M, Gigengack F, Jiang X, Schäfers KP. Motion correction of whole-body PET data with a joint PET-MRI registration functional. Biomed Eng Online. (2014) 13(Suppl. 1):S2. doi: 10.1186/1475-925X-13-S1-S2
199. Petibon Y, Guehl NJ, Reese TG, Ebrahimi B, Normandin MD, Shoup TM. Impact of motion and partial volume effects correction on PET myocardial perfusion imaging using simultaneous PET-MR. Phys Med Biol. (2017) 62:326–43. doi: 10.1088/1361-6560/aa5087
200. Cal-González J, Tsoumpas C, Lassen ML, Rasul S, Koller L, Hacker M. Impact of motion compensation and partial volume correction for 18F-NaF PET/CT imaging of coronary plaque. Phys Med Biol. (2017) 63:015005. doi: 10.1088/1361-6560/aa97c8
201. Keller SH, Hansen C, Hansen C, Andersen FL, Ladefoged C, Svarer C. Sparsely sampled MR navigators as a practical tool for quality control and correction of head motion in simultaneous PET/MR. EJNMMI Phys. (2015) 1(Suppl. 1):A36. doi: 10.1186/2197-7364-1-S1-A36
202. Rahmim A, Zaidi H. PET versus SPECT: strengths, limitations and challenges. Nucl Med Commun. (2008) 29:193–207. doi: 10.1097/MNM.0b013e3282f3a515
203. Sanders JC, Ritt P, Kuwert T, Vija AH, Maier AK. Fully automated data-driven respiratory signal extraction from SPECT images using laplacian eigenmaps. IEEE Trans Med Imaging. (2016) 35:2425–35. doi: 10.1109/TMI.2016.2576899
204. Thielemans K, Schleyer P, Marsden PK, Manjeshwar RM, Wollenweber S, Ganin A. Comparison of different methods for data-driven respiratory gating of PET data. In: 2013 IEEE Nuclear Science Symposium and Medical Imaging Conference (2013 NSS/MIC). Seoul: IEEE (2013), p. 1–4.
205. Beyer T, Moser E. MR/PET or PET/MRI: does it matter? Magn Reson Mater Phys Biol Med. (2013) 26:1–4. doi: 10.1007/s10334-012-0365-0
206. Träber F, Block W, Layer G, Bräucker G, Gieseke J, Kretzer S, et al. Determination of H relaxation times of water in human bone marrow by fat-suppressed turbo spin echo in comparison to MR spectroscopic methods. J Magn Reson Imaging (1996) 6:541–8. doi: 10.1002/jmri.1880060318
207. Samarin A, Burger C, Wollenweber SD, Crook DW, Burger IA, Schmid DT. PET/MR imaging of bone lesions - Implications for PET quantification from imperfect attenuation correction. Eur J Nucl Med Mol Imaging. (2012) 39:1154–60. doi: 10.1007/s00259-012-2113-0
208. Koesters T, Friedman KP, Fenchel M, Zhan Y, Hermosillo G, Babb J. Dixon sequence with superimposed model-based bone compartment provides highly accurate PET/MR attenuation correction of the brain. J Nucl Med. (2016) 57:918–24. doi: 10.2967/jnumed.115.166967
209. Anazodo UC, Thiessen JD, Ssali T, Mandel J, Günther M, Butler J. Feasibility of simultaneous whole-brain imaging on an integrated PET-MRI system using an enhanced 2-point Dixon attenuation correction method. Front Neurosci. (2015) 8:434. doi: 10.3389/fnins.2014.00434
210. Izquierdo-Garcia D, Hansen AE, Förster S, Benoit D, Schachoff S, Fürst S. An SPM8-based approach for attenuation correction combining segmentation and nonrigid template formation: application to simultaneous PET/MR brain imaging. J Nucl Med. (2014) 55:1825–30. doi: 10.2967/jnumed.113.136341
211. Burgos N, Cardoso MJ, Thielemans K, Modat M, Pedemonte S, Dickson J. Attenuation correction synthesis for hybrid PET-MR scanners: application to brain studies. IEEE Trans Med Imaging (2014) 33:2332–41. doi: 10.1109/TMI.2014.2340135
212. Merida I, Costes N, Heckemann R, Hammers A. Pseudo-CT generation in brain MR-PET attenuation correction: comparison of several multi-atlas methods. EJNMMI Phys. (2015) 2(Suppl. 1):A29. doi: 10.1186/2197-7364-2-S1-A29
213. Benoit D, Ladefoged CN, Rezaei A, Keller SH, Andersen FL, Højgaard L. Optimized MLAA for quantitative non-TOF PET/MR of the brain. Phys Med Biol. (2016) 61:8854–74. doi: 10.1088/1361-6560/61/24/8854
214. Cabello J, Lukas M, Forster S, Pyka T, Nekolla SG, Ziegler SI. MR-Based attenuation correction using ultrashort-echo-time pulse sequences in dementia patients. J Nucl Med. (2015) 56:423–9. doi: 10.2967/jnumed.114.146308
215. Juttukonda MR, Mersereau BG, Chen Y, Su Y, Rubin BG, Benzinger TLS. MR-based attenuation correction for PET/MRI neurological studies with continuous-valued attenuation coefficients for bone through a conversion from R2* to CT-Hounsfield units. Neuroimage. (2015) 112:160–8. doi: 10.1016/j.neuroimage.2015.03.009
216. Ladefoged CN, Benoit D, Law I, Holm S, Kjær A, Højgaard L. Region specific optimization of continuous linear attenuation coefficients based on UTE (RESOLUTE): application to PET/MR brain imaging. Phys Med Biol. (2015) 60:8047–65. doi: 10.1088/0031-9155/60/20/8047
217. Ladefoged CN, Law I, Anazodo U, St Lawrence K, Izquierdo-Garcia D, Catana C. A multi-centre evaluation of eleven clinically feasible brain PET/MRI attenuation correction techniques using a large cohort of patients. Neuroimage (2017) 147:346–59. doi: 10.1016/j.neuroimage.2016.12.010
218. Martinez-Möller A, Souvatzoglou M, Delso G, Bundschuh RA, Chefd'hotel C, Ziegler SI. Tissue classification as a potential approach for attenuation correction in whole-body PET/MRI: evaluation with PET/CT data. J Nucl Med. (2009) 50:520–6. doi: 10.2967/jnumed.108.054726
219. Rausch I, Rust P, DiFranco MD, Lassen M, Stadlbauer A, Mayerhoefer ME. Reproducibility of MRI dixon-based attenuation correction in combined PET/MR with applications for lean body mass estimation. J Nucl Med. (2016) 57:1096–101. doi: 10.2967/jnumed.115.168294
220. Sekine T, Buck A, Delso G, Ter Voert EE, Huellner M, Veit-Haibach P. Evaluation of atlas-based attenuation correction for integrated PET/MR in human brain: application of a head atlas and comparison to true CT-based attenuation correction. J Nucl Med. (2016) 57:215–220. doi: 10.2967/jnumed.115.159228
221. Paulus DH, Quick HH, Geppert C, Fenchel M, Zhan Y, Hermosillo G. Whole-body PET/MR imaging: quantitative evaluation of a novel model-based MR attenuation correction method including bone. J Nucl Med. (2015) 56:1061–6. doi: 10.2967/jnumed.115.156000
222. Rausch I, Rischka L, Ladefoged CN, Furtner J, Fenchel M, Hahn A. PET/MRI for oncologic brain imaging: a comparison of standard MR-based attenuation corrections with a model-based approach for the siemens mMR PET/MR system. J Nucl Med. (2017) 58:1519–25. doi: 10.2967/jnumed.116.186148
223. Leynes AP, Yang J, Wiesinger F, Kaushik S, Shanbhag DD, Seo Y, et al. Direct PseudoCT generation for pelvis PET/MRI attenuation correction using deep convolutional neural networks with multi-parametric MRI: zero echo-time and dixon deep pseudoCT (ZeDD-CT). J Nucl Med. (2018). 59:852–8. doi: 10.2967/jnumed.117.198051
224. Arabi H, Zaidi H. Whole-body bone segmentation from MRI for PET/MRI attenuation correction using shape-based averaging. Med Phys. (2016) 43:5848–61. doi: 10.1118/1.4963809
225. Mehranian A, Arabi H, Zaidi H. Vision 20/20: magnetic resonance imaging-guided attenuation correction in PET/MRI: challenges, solutions, and opportunities. Med Phys. (2016) 43:1130–55. doi: 10.1118/1.4941014
226. Olin A, Ladefoged CN, Langer NH, Keller SH, Löfgren JO, Hansen AE. Reproducibility of MR-based attenuation maps in PET/MRI and the impact on PET quantification in lung cancer. J Nucl Med. (2017). doi: 10.2967/jnumed.117.198853. [Epub ahead of print].
227. Heußer T, Rank CM, Berker Y, Freitag MT, Kachelrieß M. MLAA-based attenuation correction of flexible hardware components in hybrid PET/MR imaging. EJNMMI Phys. (2017) 4:12. doi: 10.1186/s40658-017-0177-4
228. Sari H, Erlandsson K, Law I, Larsson HB, Ourselin S, Arridge S. Estimation of an image derived input function with MR-defined carotid arteries in FDG-PET human studies using a novel partial volume correction method. J Cereb Blood Flow Metab. (2017) 37:1398–409. doi: 10.1177/0271678X16656197
229. Iida H, Rhodes CG, de Silva R, Yamamoto Y, Araujo LI, Maseri A. Myocardial tissue fraction–correction for partial volume effects and measure of tissue viability. J Nucl Med. (1991) 32:2169–2175.
230. Iida H, Rhodes CG, de Silva R, Araujo LI, Bloomfield PM, Lammertsma AA. Use of the left ventricular time-activity curve as a noninvasive input function in dynamic oxygen-15-water positron emission tomography. J Nucl Med. (1992) 33:1669–77.
231. Zanotti-Fregonara P, Chen K, Liow J-S, Fujita M, Innis RB. Image-derived input function for brain PET studies: many challenges and few opportunities. J Cereb Blood Flow Metab. (2011) 31:1986–98. doi: 10.1038/jcbfm.2011.107
232. Zanotti-Fregonara P, Liow JS, Comtat C, Zoghbi SS, Zhang Y, Pike VW. Image-derived input function in PET brain studies. Nucl Med Commun. (2012) 33:982–9. doi: 10.1097/MNM.0b013e328356185c
233. Mourik JEM, Lubberink M, Lammertsma AA, Boellaard R. Image derived input functions: effects of motion on tracer kinetic analyses. Mol Imaging Biol. (2011) 13:25–31. doi: 10.1007/s11307-010-0301-5
234. Mourik JE, Lubberink M, Schuitemaker A, Tolboom N, van Berckel BN, Lammertsma AA. Image-derived input functions for PET brain studies. Eur J Nucl Med Mol Imaging (2009) 36:463–71. doi: 10.1007/s00259-008-0986-8
235. Mourik JEM, Lubberink M, Klumpers UMH, Comans EF, Lammertsma AA, Boellaard R. Partial volume corrected image derived input functions for dynamic PET brain studies: methodology and validation for [11C]flumazenil. Neuroimage. (2008) 39:1041–50. doi: 10.1016/j.neuroimage.2007.10.022
236. Zhou S, Chen K, Reiman EM, Li D, Shan B. A method for generating image-derived input function in quantitative 18F-FDG PET study based on the monotonicity of the input and output function curve. Nucl Med Commun. (2012) 33:362–70. doi: 10.1097/MNM.0b013e32834f262e
237. Wahl LM, Asselin MC, Nahmias C. Regions of interest in the venous sinuses as input functions for quantitative PET. J Nucl Med. (1999) 40:1666–75.
238. Guo H, Renaut R, Chen K, Reiman E. Clustering huge data sets for parametric PET imaging. BioSystems. (2003) 71:81–92. doi: 10.1016/S0303-2647(03)00112-6
239. Fang Y-H, Kao T, Liu R-S, Wu L-C. Estimating the input function non-invasively for FDG-PET quantification with multiple linear regression analysis: simulation and verification with in vivo data. Eur J Nucl Med Mol Imaging (2004) 31:692–702. doi: 10.1007/s00259-003-1412-x
240. Liptrot M, Adams KH, Martiny L, Pinborg LH, Lonsdale MN, Olsen NV. Cluster analysis in kinetic modelling of the brain: a noninvasive alternative to arterial sampling. Neuroimage (2004) 21:483–93. doi: 10.1016/j.neuroimage.2003.09.058
241. Naganawa M, Kimura Y, Ishii K, Oda K, Ishiwata K, Matani A. Extraction of a plasma time-activity curve from dynamic brain PET images based on independent component analysis. IEEE Trans Biomed Eng. (2005) 52:201–10. doi: 10.1109/TBME.2004.840193
242. Su Y, Shoghi KI. Single-input–dual-output modeling of image-based input function estimation. Mol Imaging Biol. (2010) 12:286–94. doi: 10.1007/s11307-009-0273-5
243. Lyoo CH, Zanotti-Fregonara P, Zoghbi SS, Liow J-S, Xu R, Pike VW, et al. Image-derived input function derived from a supervised clustering algorithm: methodology and validation in a clinical protocol using [11C](R)-rolipram. PLoS ONE (2014) 9:e89101. doi: 10.1371/journal.pone.0089101
244. Simončič U, Zanotti-Fregonara P. Image-derived input function with factor analysis and a-priori information. Nucl Med Commun. (2015) 36:187–93. doi: 10.1097/MNM.0000000000000231
245. Litton JE. Input function in PET brain studies using MR-defined arteries. J Comput Assist Tomogr. (1997) 21:907–9. doi: 10.1097/00004728-199711000-00012
246. Fung EK, Carson RE. Cerebral blood flow with [15O]water PET studies using an image-derived input function and MR-defined carotid centerlines. Phys Med Biol. (2013) 58:1903–23. doi: 10.1088/0031-9155/58/6/1903
247. Da Silva NA, Herzog H, Weirich C, Tellmann L, Rota Kops E, Hautzel H, et al. Image-derived input function obtained in a 3TMR-brainPET. Nucl Instruments Methods Phys Res Sect A Accel Spectrometers Detect Assoc Equip. (2013) 702:22–5. doi: 10.1016/j.nima.2012.08.030
248. Jochimsen TH, Zeisig V, Schulz J, Werner P, Patt M, Patt J. Fully automated calculation of image-derived input function in simultaneous PET/MRI in a sheep model. EJNMMI Phys. (2016) 3:2. doi: 10.1186/s40658-016-0139-2
249. Khalighi MM, Deller TW, Fan AP, Gulaka PK, Shen B, Singh P. Image-derived input function estimation on a TOF-enabled PET/MR for cerebral blood flow mapping. J Cereb Blood Flow Metab. (2018) 38:126–35. doi: 10.1177/0271678X17691784
250. van Assema DM, Lubberink M, Bauer M, van der Flier WM, Schuit RC, Windhorst AD. Blood-brain barrier P-glycoprotein function in Alzheimer's disease. Brain. (2012) 135:181–9. doi: 10.1093/brain/awr298
251. Gjedde A. Calculation of cerebral glucose phosphorylation from brain uptake of glucose analogs in vivo: a re-examination. Brain Res Rev. (1982) 4:237–74. doi: 10.1016/0165-0173(82)90018-2
252. Patlak CS, Blasberg RG, Fenstermacher JD. Graphical evaluation of blood-to-brain transfer constants from multiple-time uptake data. J Cereb Blood Flow Metab. (1983) 3:1–7. doi: 10.1038/jcbfm.1983.1
253. Logan J, Fowler JS, Volkow ND, Wolf AP, Dewey SL, Schlyer DJ, et al. Graphical analysis of reversible radioligand binding from time-activity measurements applied to [N-11C-methyl]-(-)-cocaine PET studies in human subjects. J Cereb Blood Flow Metab. (1990) 10:740–7. doi: 10.1038/jcbfm.1990.127
254. Kety SS, Schmidt CF. The determination of cerebral blood flow in man by the use of nitrous oxide in low concentrations. Am J Physiol Content. (1945) 143:53–66. doi: 10.1152/ajplegacy.1945.143.1.53
255. Kety SS, Schmidt CF. The nitrous oxide method for the quantitative determination of cerebral blood flow in man: theory, procedure and normal values. J Clin Invest. (1948) 27:476–83. doi: 10.1172/JCI101994
256. Mintun MA, Raichle ME, Kilbourn MR, Wooten GF, Welch MJ. A quantitative model for the in vivo assessment of drug binding sites with positron emission tomography. Ann Neurol. (1984) 15:217–27. doi: 10.1002/ana.410150302
257. Bailey DL, Pichler BJ, Gückel B, Barthel H, Beer AJ, Bremerich J. Combined PET/MRI: multi-modality multi-parametric imaging is here. Mol Imaging Biol. (2015) 17:595–608. doi: 10.1007/s11307-015-0886-9
258. Padhani AR, Miles KA. Multiparametric imaging of tumor response to therapy 1. Radiology (2010) 256:348–64. doi: 10.1148/radiol.10091760
259. Singh D, Miles K. Multiparametric PET/CT in oncology. Cancer Imaging. (2012) 12:336–44. doi: 10.1102/1470-7330.2012.9007
260. Hatt M, Tixier F, Pierce L, Kinahan PE, Le Rest CC, Visvikis D. Characterization of PET/CT images using texture analysis: the past, the present… any future? Eur J Nucl Med Mol Imaging (2017) 44:151–65. doi: 10.1007/s00259-016-3427-0
261. Heiss W-D. Hybrid PET/MR imaging in neurology: present applications and prospects for the future. J Nucl Med. (2016) 57:993–5. doi: 10.2967/jnumed.116.175208
262. Puttick S, Bell C, Dowson N, Rose S, Fay M. PET, MRI, and simultaneous PET/MRI in the development of diagnostic and therapeutic strategies for glioma. Drug Discov Today (2015) 20:306–17. doi: 10.1016/j.drudis.2014.10.016
263. Nensa F, Poeppel TD, Tezgah E, Heusch P, Nassenstein K, Forsting M, et al. Integrated assessment of cardiac PET/MRI: co-registered PET and MRI polar plots by mutual mr-based segmentation of the left ventricular myocardium. World J Cardiovasc Dis (2017) 7:91–104. doi: 10.4236/wjcd.2017.74010
264. Rischpler C, Nekolla SG, Dregely I, Schwaiger M. Hybrid PET/MR imaging of the heart: potential, initial experiences, and future prospects. J Nucl Med. (2013) 54:402–15. doi: 10.2967/jnumed.112.105353
265. Spick C, Herrmann K, Czernin J. 18F-FDG PET/CT and PET/MRI perform equally well in cancer: evidence from studies on more than 2,300 patients. J Nucl Med. (2016) 57:420–30. doi: 10.2967/jnumed.115.158808
266. Even-Sapir E, Keidar Z, Bar-Shalom R. Hybrid imaging (SPECT/CT and PET/CT)-improving the diagnostic accuracy of functional/metabolic and anatomic imaging. Semin Nucl Med. (2009) 39:264–75. doi: 10.1053/j.semnuclmed.2009.03.004
267. Kjaer A. Hybrid imaging with PET/CT and PET/MR. Cancer Imaging (2014) 14(Suppl. 1):O32. doi: 10.1186/1470-7330-14-S1-O32
268. Visvikis D, Hatt M, Tixier F, Le Rest CC. The age of reason for FDG PET image-derived indices. Eur J Nucl Med Mol Imaging (2012) 39:1670–2. doi: 10.1007/s00259-012-2239-0
269. Haralick RM, Shanmugam K, Dinstein I. Textural features for image classification. Syst Man Cybern. (1973) 3:610–21. doi: 10.1109/TSMC.1973.4309314
270. Tixier F, Le Rest CC, Hatt M, Albarghach N, Pradier O, Metges JP. Intratumor heterogeneity characterized by textural features on baseline 18F-FDG PET images predicts response to concomitant radiochemotherapy in esophageal cancer. J Nucl Med. (2011) 52:369–78. doi: 10.2967/jnumed.110.082404
271. Bundschuh RA, Dinges J, Neumann L, Seyfried M, Zsótér N, Papp L, et al. Textural parameters of tumor heterogeneity in 18F-FDG PET/CT for therapy response assessment and prognosis in patients with locally advanced rectal cancer. J Nucl Med. (2014) 55:891–7. doi: 10.2967/jnumed.113.127340
272. Pyka T, Bundschuh RA, Andratschke N, Mayer B, Specht HM, Papp L. Textural features in pre-treatment [F18]-FDG-PET/CT are correlated with risk of local recurrence and disease-specific survival in early stage NSCLC patients receiving primary stereotactic radiation therapy. Radiat Oncol. (2015) 10:100. doi: 10.1186/s13014-015-0407-7
273. Desseroit MC, Visvikis D, Tixier F, Majdoub M, Perdrisot R, Guillevin R. Development of a nomogram combining clinical staging with 18F-FDG PET/CT image features in non-small-cell lung cancer stage I-III. Eur J Nucl Med Mol Imaging (2016) 43:1477–85. doi: 10.1007/s00259-016-3325-5
274. Brooks FJ, Grigsby PW. The effect of small tumor volumes on studies of intratumoral heterogeneity of tracer uptake. J Nucl Med. (2014) 55:37–42. doi: 10.2967/jnumed.112.116715
275. Hatt M, Majdoub M, Vallières M, Tixier F, Le Rest CC, Groheux D. 18F-FDG PET uptake characterization through texture analysis: investigating the complementary nature of heterogeneity and functional tumor volume in a multi-cancer site patient cohort. J Nucl Med. (2015) 56:38–44. doi: 10.2967/jnumed.114.144055
276. Zhao B, Tan Y, Tsai WY, Qi J, Xie C, Lu L. Reproducibility of radiomics for deciphering tumor phenotype with imaging. Sci Rep. (2016) 6:23428. doi: 10.1038/srep23428
277. Gillies RJ, Kinahan PE, Hricak H. Radiomics: images are more than pictures, they are data. Radiology. (2015) 278:151169. doi: 10.1148/radiol.2015151169
278. Aerts HJ, Velazquez ER, Leijenaar RT, Parmar C, Grossmann P, Carvalho S. Decoding tumour phenotype by noninvasive imaging using a quantitative radiomics approach. Nat Commun. (2014) 5:4006. doi: 10.1038/ncomms5006
279. Parmar C, Leijenaar RT, Grossmann P, Rios Velazquez E, Bussink J, Rietveld D. Radiomic feature clusters and Prognostic Signatures specific for Lung and Head and Neck cancer. Sci Rep. (2015) 5:1–10. doi: 10.1038/srep11044
280. Gillies RJ, Beyer T. PET and MRI: is the whole greater than the sum of its parts? Cancer Res. (2016) 76:6163–6. doi: 10.1158/0008-5472.CAN-16-2121
281. Court LE, Fave X, Mackin D, Lee J, Yang J, Zhang L. Computational resources for radiomics. Transl Cancer Res. (2016) 5:340–8. doi: 10.21037/tcr.2016.06.17
282. Kourou K, Exarchos TP, Exarchos KP, Karamouzis M V. Fotiadis DI. Machine learning applications in cancer prognosis and prediction. Comput Struct Biotechnol J. (2015) 13:8–17. doi: 10.1016/j.csbj.2014.11.005
283. Kickingereder P, Burth S, Wick A, Götz M, Eidel O, Schlemmer HP. Radiomic profiling of glioblastoma: identifying an imaging predictor of patient survival with improved performance over established clinical and radiologic risk models. Radiology (2016) 280:880–9. doi: 10.1148/radiol.2016160845
284. Ypsilantis PP, Siddique M, Sohn HM, Davies A, Cook G, Goh V. Predicting response to neoadjuvant chemotherapy with PET imaging using convolutional neural networks. PLoS ONE (2015) 10:e0137036. doi: 10.1371/journal.pone.0137036
285. Wu W, Parmar C, Grossmann P, Quackenbush J, Lambin P, Bussink J. Exploratory study to identify radiomics classifiers for lung cancer histology. Front Oncol. (2016) 6:71. doi: 10.3389/fonc.2016.00071
286. Wu J, Cui Y, Sun X, Cao G, Li B, Ikeda DM. Unsupervised clustering of quantitative image phenotypes reveals breast cancer subtypes with distinct prognoses and molecular pathways. Clin Cancer Res. (2017) 23:3334–2. doi: 10.1158/1078-0432.CCR-16-2415
287. National Electrical Manufacturers Association. NEMA Standards Publication NU 4 - 2008 Performance Measurements of Small Animal Positron Emission Tomographs. Rosslyn, VA (2008).
288. National Electrical Manufacturers Association. NEMA Standards Publication NU-2 2012 Performance Measurements of Positron Emission Tomographs. Rosslyn, VA (2012).
289. National Electrical Manufacturers Association. NEMA Standards Publication NU-1 2012 Performance Measurements of Gamma Cameras. Rosslyn, VA (2012).
290. Boellaard R. Standards for PET image acquisition and quantitative data analysis. J Nucl Med. (2008) 50(Suppl. 1):11S−20S. doi: 10.2967/jnumed.108.057182
291. Beyer T, Czernin J, Freudenberg LS. Variations in clinical PET/CT operations: results of an international survey of active PET/CT users. J Nucl Med. (2011) 52:303–10. doi: 10.2967/jnumed.110.079624
292. Wieder H, Freudenberg LS, Czernin J, Navar BN, Israel O, Beyer T. Variations of clinical SPECT/CT operations. Nuklearmedizin (2012) 51:154–60. doi: 10.3413/Nukmed-0467-12-01
293. Chauvie S, Bergesio F. The strategies to homogenize PET/CT metrics: the case of onco-haematological clinical trials. Biomedicines. (2016) 4:26. doi: 10.3390/biomedicines4040026
294. Boellaard R, Delgado-Bolton R, Oyen WJ, Giammarile F, Tatsch K, Eschner W. FDG PET/CT: EANM procedure guidelines for tumour imaging: version 2.0. Eur J Nucl Med Mol Imaging (2014) 42:328–54. doi: 10.1007/s00259-014-2961-x
295. Varrone A, Asenbaum S, Vander Borght T, Booij J, Nobili F, Någren K, et al. EANM procedure guidelines for PET brain imaging using [18F]FDG, version 2. Eur J Nucl Med Mol Imaging. (2009) 36:2103–10. doi: 10.1007/s00259-009-1264-0
296. Flotats A, Knuuti J, Gutberlet M, Marcassa C, Bengel FM, Kaufmann PA. Hybrid cardiac imaging: SPECT/CT and PET/CT. A joint position statement by the European Association of Nuclear Medicine (EANM), the European Society of Cardiac Radiology (ESCR) and the European Council of Nuclear Cardiology (ECNC). Eur J Nucl Med Mol Imaging. (2011) 38:201–12. doi: 10.1007/s00259-010-1586-y
297. Chalkidou A, O'Doherty MJ, Marsden PK. False discovery rates in PET and CT studies with texture features: a systematic review. PLoS ONE (2015) 10:e0124165. doi: 10.1371/journal.pone.0124165
298. Molina D, Pérez-Beteta J, Martínez-González A, Martino J, Velasquez C, Arana E. Lack of robustness of textural measures obtained from 3D brain tumor MRIs impose a need for standardization. PLoS ONE (2017) 12:e0178843. doi: 10.1371/journal.pone.0178843
299. Nyflot MJ, Yang F, Byrd D, Bowen SR, Sandison GA, Kinahan PE. Quantitative radiomics: impact of stochastic effects on textural feature analysis implies the need for standards. J Med Imaging. (2015) 2:41002. doi: 10.1117/1.JMI.2.4.041002
300. van Velden FH, Kramer GM, Frings V, Nissen IA, Mulder ER, de Langen AJ. Repeatability of radiomic features in non-small-cell lung cancer [18F]FDG-PET/CT studies: impact of reconstruction and delineation. Mol Imaging Biol. (2016) 18:788–95. doi: 10.1007/s11307-016-0940-2
301. Tixier F, Hatt M, Le Rest CC, Le Pogam A, Corcos L, Visvikis D. Reproducibility of tumor uptake heterogeneity characterization through textural feature analysis in 18F-FDG PET. J Nucl Med. (2012) 53:693–700. doi: 10.2967/jnumed.111.099127
302. Leijenaar RT, Carvalho S, Velazquez ER, van Elmpt WJ, Parmar C, Hoekstra OS. Stability of FDG-PET radiomics features: AN integrated analysis of test-retest and inter-observer variability. Acta Oncol (Madr) (2013) 52:1391–7. doi: 10.3109/0284186X.2013.812798
303. Costello MJ. Motivating online publication of data. Bioscience (2009) 59:418–27. doi: 10.1525/bio.2009.59.5.9
304. Wicherts JM, Bakker M, Molenaar D. Willingness to share research data is related to the strength of the evidence and the quality of reporting of statistical results. PLoS ONE (2011) 6:e26828. doi: 10.1371/journal.pone.0026828
305. Roche DG, Kruuk LEB, Lanfear R, Binning SA. Public data archiving in ecology and evolution: how well are we doing? PLoS Biol. (2015) 13:e1002295. doi: 10.1371/journal.pbio.1002295
306. Tenopir C, Allard S, Douglass K, Aydinoglu AU, Wu L, Read E. Data sharing by scientists: practices and perceptions. PLoS ONE (2011) 6:e21101. doi: 10.1371/journal.pone.0021101
307. Santos C, Blake J, States DJ. Supplementary data need to be kept in public repositories. Nature (2005) 438:738. doi: 10.1038/438738a
308. Bray T, Paoli J, Sperberg-McQueen CM, Maler E, Yergeau F. Extensible markup language (XML). World Wide Web J. (1997) 2:27–66.
309. Kadrmas DJ, Hoffman JM. Methodology for quantitative rapid multi-tracer PET tumor characterizations. Theranostics (2013) 3:757–73. doi: 10.7150/thno.5201
310. El Fakhri G, Moore SC, Maksud P, Aurengo A, Kijewski MF. Absolute activity quantitation in simultaneous 123I/99mTc brain SPECT. J Nucl Med. (2001) 42:300–8.
311. El Fakhri G, Sitek A, Zimmerman RE, Ouyang J. Generalized five-dimensional dynamic and spectral factor analysis. Med Phys. (2006) 33:1016–24. doi: 10.1118/1.2179168
312. Verhaeghe J, D'Asseler Y, Staelens S, Lemahieu I. Noise properties of simultaneous dual tracer PET imaging. In: IEEE Nuclear Science Symposium Conference Record, Vol 5. Fajardo: IEEE (2005). p. 2611–4.
313. Figueiras FP, Jiménez X, Pareto D, Gómez V, Llop J, Herance R. Simultaneous dual-tracer PET imaging of the rat brain and its application in the study of cerebral ischemia. Mol Imaging Biol. (2011) 13:500–10. doi: 10.1007/s11307-010-0370-5
314. Koeppe RA, Ficaro EP, Raffel DM, Minoshima S, Kilbourn MR. Temporally overlapping dual-tracer PET studies. In: Carson RE, Daube-Witherspoon ME, Herscovitch P, editors. Quantitative Functional Brain Imaging with Positron Emission Tomography. San Diego, CA: Elsevier (1998). p. 359–66.
315. Kadrmas DJ, Rust TC. Feasibility of rapid multitracer PET tumor imaging. IEEE Trans Nucl Sci. (2005) 52:1341–7. doi: 10.1109/TNS.2005.858230
316. Andreyev A, Celler A. Dual-isotope PET using positron-gamma emitters. Phys Med Biol. (2011) 56:4539–56. doi: 10.1088/0031-9155/56/14/020
317. Lage E, Herraiz JL, Parot V. Multiplexable Emission Tomography. (2013). Available online at: https://patents.google.com/patent/WO2013188011A1/en.
318. Cal-González J, Lage E, Herranz E, Vicente E, Udias JM, Moore SC. Simulation of triple coincidences in PET. Phys Med Biol. (2015) 60:117–36. doi: 10.1088/0031-9155/60/1/117
319. Strobel K, Heinrich S, Bhure U, Soyka J, Veit-Haibach P, Pestalozzi BC, et al. Contrast-enhanced 18 F-FDG PET/CT: 1-stop- shop imaging for assessing the resectability of pancreatic cancer. J Nucl Med. (2008) 49:1408–13. doi: 10.2967/jnumed.108.051466
320. Badiee S, Franc BL, Webb EM, Chu B, Hawkins RA, Coakley F. Role of IV iodinated contrast material in 18 F-FDG PET/CT of liver metastases. Am J Roentgenol. (2008) 191:1436–9. doi: 10.2214/AJR.07.3750
321. Asagi A, Ohta K, Nasu J, Tanada M, Nadano S, Nishimura R. Utility of contrast-enhanced FDG-PET/CT in the clinical management of pancreatic cancer. Pancreas (2013) 42:11–19. doi: 10.1097/MPA.0b013e3182550d77
322. Kuhn FP, Hullner M, Mader CE, Kastrinidis N, Huber GF, von Schulthess GK, et al. Contrast-enhanced PET/MR imaging versus contrast-enhanced PET/CT in head and neck cancer: how much mr information is needed? J Nucl Med. (2014) 55:551–8. doi: 10.2967/jnumed.113.125443
323. Thakor AS, Gambhir SS. Nanooncology: the future of cancer diagnosis and therapy. CA Cancer J Clin. (2013) 63:395–418. doi: 10.3322/caac.21199
324. Yen SK, Padmanabhan P, Selvan ST. Multifunctional iron oxide nanoparticles for diagnostics, therapy and macromolecule delivery. Theranostics (2013) 3:986–1003. doi: 10.7150/thno.4827
325. Fakhri G El. Ready for prime time? Dual tracer PET and SPECT imaging. Am J Nucl Med Mol Imaging (2012) 2:415–7.
326. Chapman SE, Diener JM, Sasser TA, Correcher C, González AJ, Avermaete TV. Dual tracer imaging of SPECT and PET probes in living mice using a sequential protocol. Am J Nucl Med Mol Imaging. (2012) 2:405–14.
327. Shi X, Meng X, Sun X, Xing L, Yu J. PET/CT imaging-guided dose painting in radiation therapy. Cancer Lett. (2014) 355:169–75. doi: 10.1016/j.canlet.2014.07.042
328. Thomas HM, Kinahan PE, Samuel JJE, Bowen SR. Impact of tumour motion compensation and delineation methods on FDG PET-based dose painting plan quality for NSCLC radiation therapy. J Med Imaging Radiat Oncol. (2018) 62:81–90. doi: 10.1111/1754-9485.12693
329. Daniel M, Andrzejewski P, Sturdza A, Majercakova K, Baltzer P, Pinker K. Impact of hybrid PET/MR technology on multiparametric imaging and treatment response assessment of cervix cancer. Radiother Oncol. (2017) 125:420–5. doi: 10.1016/j.radonc.2017.10.036
330. Bradley J, Thorstad WL, Mutic S, Miller TR, Dehdashti F, Siegel BA. Impact of FDG-PET on radiation therapy volume delineation in non–small-cell lung cancer. Int J Radiat Oncol. (2004) 59:78–86. doi: 10.1016/j.ijrobp.2003.10.044
331. Souvatzoglou M, Krause BJ, Pürschel A, Thamm R, Schuster T, Buck AK, et al. Influence of 11C-choline PET/CT on the treatment planning for salvage radiation therapy in patients with biochemical recurrence of prostate cancer. Radiother Oncol. (2011) 99:193–200. doi: 10.1016/j.radonc.2011.05.005
332. Pasqualetti F, Panichi M, Sainato A, Matteucci F, Galli L, Cocuzza P. [(18)F]Choline PET/CT and stereotactic body radiotherapy on treatment decision making of oligometastatic prostate cancer patients: preliminary results. Radiat Oncol. (2016) 11:9. doi: 10.1186/s13014-016-0586-x
333. Alongi F, Fersino S, Giaj Levra N, Mazzola R, Ricchetti F, Fiorentino A. Impact of 18F-choline PET/CT in the decision-making strategy of treatment volumes in definitive prostate cancer volumetric modulated radiation therapy. Clin Nucl Med. (2015) 40:e496–500. doi: 10.1097/RLU.0000000000000841
334. McKinley ET, Ayers GD, Smith RA, Saleh SA, Zhao P, Washington MK, et al. Limits of [18F]-FLT PET as a biomarker of proliferation in oncology. PLoS ONE (2013) 8:e58938. doi: 10.1371/journal.pone.0058938
335. Segard T, Robins PD, Yusoff IF, Ee H, Morandeau L, Campbell EM, et al. Detection of hypoxia with 18F-fluoromisonidazole (18F-FMISO) PET/CT in suspected or proven pancreatic cancer. Clin Nucl Med. (2013) 38:1–6. doi: 10.1097/RLU.0b013e3182708777
336. Lapi SE, Lewis JS, Dehdashti F. Evaluation of hypoxia with copper-labeled diacetyl-bis(N-methylthiosemicarbazone). Semin Nucl Med. (2015) 45:177–85. doi: 10.1053/j.semnuclmed.2014.10.003
337. Knopf A-C, Lomax A. In vivo proton range verification: a review. Phys Med Biol. (2013) 58:R131–60. doi: 10.1088/0031-9155/58/15/R131
338. Sportelli G, Belcari N, Camarlinghi N, Cirrone GA, Cuttone G, Ferretti S. First full-beam PET acquisitions in proton therapy with a modular dual-head dedicated system. Phys Med Biol. (2014) 59:43–60. doi: 10.1088/0031-9155/59/1/43
339. Shao Y, Sun X, Lou K, Zhu XR, Mirkovic D, Poenisch F. In-beam PET imaging for on-line adaptive proton therapy: an initial phantom study. Phys Med Biol. (2014) 59:3373–88. doi: 10.1088/0031-9155/59/13/3373
340. Ferrero V, Fiorina E, Morrocchi M, Pennazio F, Baroni G, Battistoni G. Online proton therapy monitoring: clinical test of a Silicon-photodetector-based in-beam PET. Sci Rep. (2018) 8:4100. doi: 10.1038/s41598-018-22325-6
Keywords: hybrid imaging, combined imaging, instrumentation, nuclear medicine, data processing, data corrections
Citation: Cal-Gonzalez J, Rausch I, Shiyam Sundar LK, Lassen ML, Muzik O, Moser E, Papp L and Beyer T (2018) Hybrid Imaging: Instrumentation and Data Processing. Front. Phys. 6:47. doi: 10.3389/fphy.2018.00047
Received: 28 December 2017; Accepted: 30 April 2018;
Published: 18 May 2018.
Edited by:
Claudia Kuntner, Austrian Institute of Technology, AustriaReviewed by:
Jose M. Perez, Centro de Investigaciones Energéticas, Medioambientales y Tecnológicas, SpainMarc Huisman, Medical Center, VU University Amsterdam, Netherlands
Copyright © 2018 Cal-Gonzalez, Rausch, Shiyam Sundar, Lassen, Muzik, Moser, Papp and Beyer. This is an open-access article distributed under the terms of the Creative Commons Attribution License (CC BY). The use, distribution or reproduction in other forums is permitted, provided the original author(s) and the copyright owner are credited and that the original publication in this journal is cited, in accordance with accepted academic practice. No use, distribution or reproduction is permitted which does not comply with these terms.
*Correspondence: Jacobo Cal-Gonzalez, amFjb2JvLmNhbGdvbnphbGV6QG1lZHVuaXdpZW4uYWMuYXQ=