Illuminating the Numbers: Integrating Mathematical Models to Optimize Photomedicine Dosimetry and Combination Therapies
- 1Translational Biophotonics Cluster, Northeastern University, Boston, MA, United States
- 2Department of Physics, Northeastern University, Boston, MA, United States
- 3Department of Bioengineering, Northeastern University, Boston, MA, United States
- 4Joint Department of Biomedical Engineering, University of North Carolina at Chapel Hill and North Carolina State University, Chapel Hill, NC, United States
- 5Lineberger Comprehensive Cancer Center, School of Medicine, University of North Carolina at Chapel Hill, Chapel Hill, NC, United States
- 6Department of Gynecologic Oncology, H. Lee Moffitt Cancer Center and Research Institute, Tampa, FL, United States
- 7Department of Immunology, H. Lee Moffitt Cancer Center and Research Institute, Tampa, FL, United States
- 8Wellman Center for Photomedicine, Massachusetts General Hospital and Harvard Medical School, Boston, MA, United States
- 9Division of Health Sciences and Technology, Harvard University and Massachusetts Institute of Technology, Cambridge, MA, United States
- 10Department of Diagnostic Imaging and Interventional Radiology, H. Lee Moffitt Cancer Center and Research Institute, Tampa, FL, United States
- 11Department of Integrated Mathematical Oncology, H. Lee Moffitt Cancer Center and Research Institute, Tampa, FL, United States
- 12Department of Radiation Oncology, H. Lee Moffitt Cancer Center and Research Institute, Tampa, FL, United States
Cancer photomedicine offers unique mechanisms for inducing local tumor damage with the potential to stimulate local and systemic anti-tumor immunity. Optically-active nanomedicine offers these features as well as spatiotemporal control of tumor-focused drug release to realize synergistic combination therapies. Achieving quantitative dosimetry is a major challenge, and dosimetry is fundamental to photomedicine for personalizing and tailoring therapeutic regimens to specific patients and anatomical locations. The challenge of dosimetry is perhaps greater for photomedicine than many standard therapies given the complexity of light delivery and light–tissue interactions as well as the resulting photochemistry responsible for tumor damage and drug-release, in addition to the usual intricacies of therapeutic agent delivery. An emerging multidisciplinary approach in oncology utilizes mathematical and computational models to iteratively and quantitively analyze complex dosimetry, and biological response parameters. These models are parameterized by preclinical and clinical observations and then tested against previously unseen data. Such calibrated and validated models can be deployed to simulate treatment doses, protocols, and combinations that have not yet been experimentally or clinically evaluated and can provide testable optimal treatment outcomes in a practical workflow. Here, we foresee the utility of these computational approaches to guide adaptive therapy, and how mathematical models might be further developed and integrated as a novel methodology to guide precision photomedicine.
Introduction
Photodynamic therapy (PDT) has been developed as a clinical therapy over the past century with origins dating back to ancient civilizations and applications for skin diseases using plant extracts and sunlight [1, 2]. Contemporary photodynamic chromophores for PDT, often termed photosensitizers (PS), feature enhanced photophysical properties for deep-tissue absorption of far-red laser light and for generating cytotoxic, activated chemical species (including reactive oxygen species). These modern PS are often engineered to rapidly clear from the skin and body to minimize the risk of severe sunburn—the major potential side-effect of otherwise non-toxic photomedicines [1, 2]. An example of clinical impact has been the use of PDT as a frontline therapy in ophthalmology for the wet form of age-related macular degeneration (wet AMD). PDT has benefited several 100,000 AMD patients worldwide since the early 2000s [3, 4]. In oncology applications, PDT offers unique modes of cell death induction [5–7] that bypass many mechanisms of classical drug resistance [8], and tumor specificity with low toxicity to off-target tissues and organs when light activation is confined to the tumor site [1, 2]. A number of molecular-targeted PDT agents (e.g., antibody-PS conjugates) are emerging with enhanced tumor selectivity in preclinical models [9–16] as well as optically-active nanomedicine [17–19] to facilitate spatiotemporal control of multi-drug combination therapies that damage various tumor compartments in concert (e.g., cancer cells, tumor-supportive stromal cells, and microvasculature) while suppressing metastatic escape [19]. These advances promise to impact oncology more substantially than the present use of unformulated PS agents.
Another potential intrinsic advantage of photomedicine is that, rather than suppressing the immune system, it can stimulate acute inflammation, tumor-antigen exposure, and anti-tumor immunity [20]. A comprehensive review by Castano et al. summarizes the preclinical data demonstrating that PDT can be used to stimulate the host immune system [20]. For instance, it has been shown that pro-inflammatory cytokines are released following the administration of PDT in mouse models [20, 21]. In addition, PDT can increase the exposure of tumor-antigens to the host immune system. PDT-induced tumor necrosis facilitates the release of extra-cellular heat-shock protein (HSP) family members, such as HSP70 [20, 22]. HSP70 is a protein folding chaperone that stabilizes potential tumor-antigens and these HSP70–peptide tumor antigen complexes are thought to help stimulate an immune response through HSP binding to antigen-presenting cells, which in turn ultimately present the potential tumor antigens to CD8+ cytotoxic T cells [20]. However, PDT dosimetry is salient to maximizing anti-tumor immune stimulation vs. extensive tumor destruction that can counteract some of these mechanisms; e.g., high-dose PDT is more likely to interfere with immune cell trafficking in and out of the tumor by shutting down the tumor microvasculature. Gollnick et al. introduced an immune-enhancing PDT regime distinct from the conventional goal of maximal tumor damage [23]. Immune-enhancing PDT is performed at lower doses that promote acute inflammation and neutrophil infiltration into the tumor; and, a follow-up high-dose PDT regimen can be applied to reduce the tumor volume once the immune system is activated [23]. The use of low-dose and metronomic therapy is also being investigated for immune-enhancing effects for chemotherapy and radiotherapy [24, 25].
To the best of our knowledge, a comprehensive clinical study in humans of PDT-tumor immune modulation has not been performed although such a study is warranted. However, PDT-induced stimulation of the immune system is hypothesized as a candidate for clinical observations of increases in overall survival following PDT. A recent example is the finding that lung sparing surgery combined with intraoperative PDT of the chest cavity [26–28], followed by adjuvant chemotherapy, achieves remarkably extended survival for patients with malignant pleural mesothelioma compared to standard therapies [28]. The median overall survival of patients who received lung sparing surgery and intraoperative PDT is extended more than 2 years (3-year median overall survival) compared to patients treated by standard surgery with adjuvant chemotherapy (8-month median overall survival) [28]. The remarkable enhancement in survival extends ~2 years beyond the median time to disease recurrence (~1 year), which may suggest an anti-tumor immune-enhancing effect from PDT that also benefits from the sparing of lung tissue and the associated lymph nodes [28]. Inhibition of T cell egress from tumor-draining lymph nodes has recently been demonstrated to drastically limit antitumor immunity post radiation [29], spurring discussion for investigations into lymph node sparing in radiotherapy [30].
Like radiation therapy, PDT requires robust production of activated chemical species in order to illicit inflammation and cell death. PDT-based treatments involve the administration of a PS, which is non-toxic until it absorbs visible light, and the PS then facilitates the conversion of molecular oxygen into reactive oxygen species, among other photochemical mechanisms of producing activated reactive species, which are known to damage tumors by several mechanisms [2]. For instance, PDT can be applied to curtail the tumor microvasculature to starve tumors of nutrients [31], and it is also possible to produce direct tumor cell death via apoptosis, necrosis and other cell death pathways [7, 32], or a combination of tumor vascular and cellular effects [33]. Importantly, the PS-light interval can impact which compartment of the tumor receives the damage based on the pharmacokinetics of the PS [33]; and, the subcellular localization of the PS also determines the stimulation of specific cell death pathways and the interaction of PDT with other modes of therapy [7, 8, 34–36].
Given the intricacies of PDT dosimetry, an ongoing dilemma is the lack of robust PDT dosimetry tools. These technologies are still maturing, and cutting-edge advances have yet to be translated to the clinic. However, advanced imaging modalities report prognostic indicators of biological responses [37], and significant advances in developing both explicit and implicit metrics of the photodynamic dose deposited in target sites have yielded exciting data ripe for rigorous analysis [37–41]. However, very little attention is given in general to a priori optimization of dose, dose fractionation, treatment timing, and sequencing of synergistic treatments among oncologists and cancer researchers. An exhaustive preclinical and clinical evaluation of all possible doses and treatment combinations is impossible. Recently, progress in integrated mathematical oncology, a powerful approach that iteratively utilizes experimental and clinical data to build calibrated quantitative models to predict response to untested treatments and treatment combinations, makes such complex analysis approachable [42, 43]. Development, calibration, and validation of quantitative and predictive models are critical in order to focus experimental and clinical trial design on regimens that maximize efficacy and quality of life.
Integrated Mathematical Oncology
Mathematical modeling in cancer research has a long history [42–47]. Mathematical oncology approaches often incorporate first-order mechanistic principles of tumor growth laws [48, 49], ecology, and evolution [50–53], and increasingly interactions with the immune system [54–59] to simulate biological responses to a variety of therapies [60–66]. One class of mathematical models may follow a “bottom-up” approach, where mechanisms on specific biological or temporal scales (i.e., subcellular, or cellular dynamics) are described mathematically, and simulations reveal emergent properties on larger scales (cellular, tissue level, organ level). Vis-à-vis “bottom-up” is the top-down approach, where modeling the dynamics on a higher level (for example a tumor population) may provide insights on the underlying mechanisms of the system (for example, cellular properties, and cell-cell interactions). A detailed summary of the most appropriate mathematical techniques for the different biological, temporal, and spatial scales with illustrative examples can be found elsewhere [67].
One recent application of successful mathematical oncology has demonstrated the translation of the ecological and evolutionary principles of emerging cancer treatment resistance into a clinical trial of adaptive therapy of metastatic castrate-resistant prostate cancer [68]. Simulations of a simple evolutionary game of three competing cancer cell subtypes and their distinct susceptibilities to a single drug (abiraterone, an inhibitor of testosterone production used for androgen-deprivation therapy) suggest that, instead of aiming for an impossible cure with continuous treatment, adaptive intermittent therapy could control the tumor by maintaining competition among the three cancer cell subtypes. With this approach, the androgen-independent cell population is not able to proliferate and establish the treatment refractory, and ultimately lethal, abiraterone-resistant disease [68]. In the prospective clinical study of adaptive hormone treatment, the majority of patients maintained stable oscillations of tumor burden for at least 27 months (10 of 11 patients were still progression free with stable tumor burden at the time of publication) using only 47% of the normal cumulative drug dose, whereas the median time to progression is 16.5 months for standard dosing [68]. An alternative strategy to successfully combat resistance may be to not target individual cell populations, but to change the fitness and cost of their interactions with each other and their tissue environments [69].
A wealth of knowledge exists already in the literature regarding the intricacies of PS pharmacokinetics, PS cellular localization, and photodynamic dosimetry [2]; and, there is considerable preclinical data deciphering the complex spatiotemporal interplay of PDT with the tumor and the tumor microenvironment that may increase the likelihood of treatment success [2]. Many of the biological factors influencing the efficacy of PDT as well as molecular mechanisms of tumor response to PDT have been elucidated. While clinical trials for new therapies are plentiful (including PDT), with a few exceptions, most are based on limited pre-clinical studies with arbitrarily chosen treatment parameters. This motivates the development and application of mathematical and computational models that capture salient features from the preclinical and clinical knowledgebase to make reliable predictions by conducting the experiments in silico to identify a small set of potentially optimal protocols for experimental testing. Unexpected novel insights and breakthroughs may also be garnered from the simulations that are hard to anticipate due to the multiparameter nature of combination therapies and tumor biology—such as the hypothesis that adaptive therapy with intermittent dosing and drug holidays [53, 68, 70–76] can lead to stable tumor oscillations that has now shown remarkable promise in the clinic [68].
The fundamental importance of photodynamic dosimetry for achieving local tumor control, drug-release, and synergistic interactions with other therapeutic modalities, has motivated the development of mathematic models of photochemistry and photophysics as well as dosimetry and dose deposition [39, 77–80]. Other modeling studies focused on identifying optimal oxygenation conditions for PDT effects [81, 82], and on information processing and cellular decision-making during PDT to predict cell survival [83]. In a number of recent studies, the effect of cell death through apoptosis and necrosis was quantitatively modeled [84, 85]. These studies suggest that PDT is most likely successful in tumors with high surface-to-volume ratios, and that PDT is unlikely to provide control in fast proliferating deep tumor tissues, which supports previous model results for low-penetrating red-light PDT [86]. As PDT and radiation therapy share similar biological responses and routine involvement of medical physics, modeling approaches for radiotherapy response may be readily translatable to identify optimal PDT protocols [59, 61, 63, 87–93]. In this Perspective, we suggest integrated mathematical oncology as a computational platform for developing quantitative models and simulations of PDT dosimetry to optimize local tumor control, tumor-focused drug release, spatiotemporal dynamics, and photodynamic priming of systemic modes of therapy. Based on the wealth of available mathematical models and increasingly emerging data on PDT, it is conceivable that established mathematical oncology workflows can be adapted to PDT. Integrating preclinical and clinical experience with computational models and simulation, in an iterative approach with continuous refinements based on observed results, will identify the most promising protocols for subsequent experimental and clinical validation (Figure 1). This computational model-guided approach to research and clinical oncology promises an efficient, cost-effective, and safe approach to synergize with experimental study design.
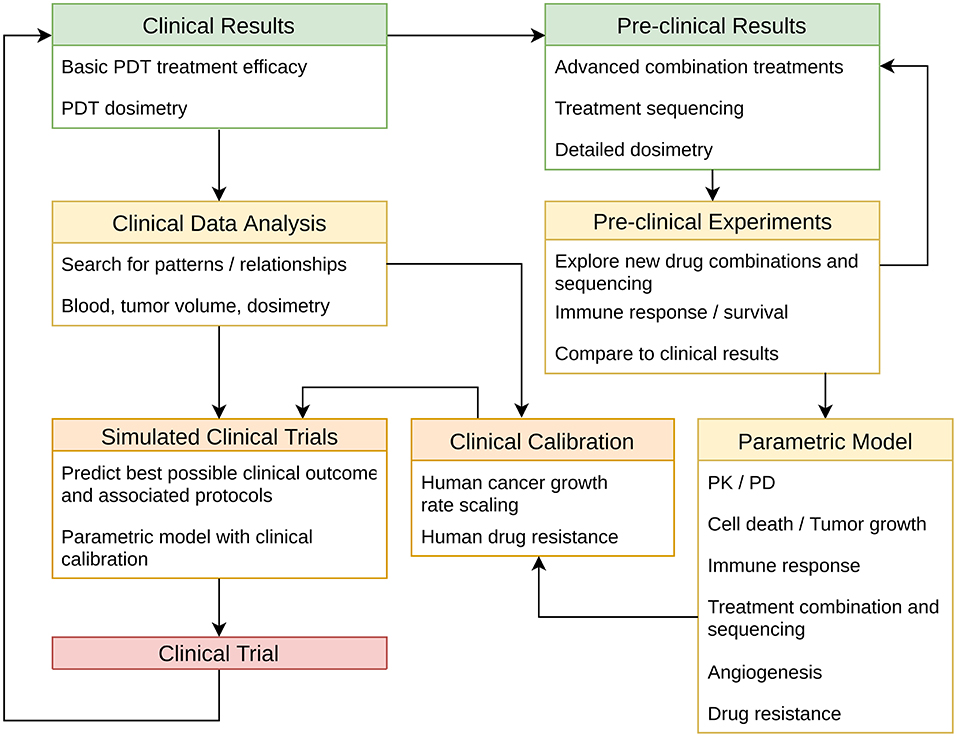
Figure 1. Proposed work flow for integrating pre-clinical and clinical data with quantitative models of PDT dosimetry, synergies with other therapies, and interactions with tumor biology. The model is calibrated to unify observations from human data and preclinical biological models, and applied to simulate many possible clinical treatment regimens. Optimal regimens are then identified and can be tested in preclinical and clinical studies. Further, results from biological models and human studies may then be used to further refine the computational model as needed in an iterative approach. Online simulation may also be used to guide personalized and adaptive therapy tailored to individual patients and tumor biology dynamics using feedback from clinical tests and imaging data throughout the course of the treatment. Color-coding suggests time-to-realization for these steps: green boxes indicate that a great deal of data is available in the existing literature; yellow boxes indicate experiments and models that can be immediately developed; orange boxes indicate steps that require preliminary work to be done first; and, the red box (Clinical Trial) represents the overarching goal for this workflow. PK, pharmacokinetics; PD, pharmacodynamics. Adapted from McGuire et al. [60].
Toward a Mathematical Model of Immune-Enhancing PDT
Cancer therapy success may be a combination of the direct lethal effect on cancer cells and, possibly more importantly, the subsequent indirect effect of stimulating a potent antitumor immune response. Tumors grow in a complex ecosystem that is the result of co-evolution of the tumor with its host environment. A contribution of the complex tumor microenvironment to treatment outcome is increasingly appreciated. Focal therapies like PDT and radiation can potentially induce a robust antitumor immune response that provides a second wave of cell kill and tumor regression [94, 95]. Functional immunity is comprised of two main conceptual components: (1) immune effector populations that act to regress the tumor including natural killer (NK) cells, N1 neutrophils, CD4+ helper T (Th) cells, CD8+ cytotoxic (CTL) T cells, M1 macrophages and mature dendritic cells (DC); and (2), immune suppressor cells that facilitate tumor escape, including N2 neutrophils, regulatory T (Treg) cells, myeloid-derived suppressor cells (MDSC), M2 macrophages, and tolerogenic DC [96]. After immune effector populations become activated against a pathogen or, as in cancer, against cells presenting abnormal antigens, immune suppression is a natural response to prevent autoimmune diseases. As an example, Tregs can suppress antitumor immunity through a variety of mechanisms, including inhibition of DC maturation and function, release of inhibitory cytokines such as TGFβ, and high expression of the IL-2 co-receptor CD25 that deprives the environment of IL-2 and thereby disrupts CD8+ T cell proliferation and granzyme A and B-dependent effector T-cell cytolysis [97]. Our present understanding of this network of immune effectors vs. immune suppressors can in principle be modeled to guide the development of therapies that can help shift the local tumor environment away from immunosuppression by bypassing or reducing checkpoints to effector immune cell activity.
Despite recent advancements in cancer immunotherapy, mainstay cancer therapies have not yet specifically focused on enhancing the immune response to tumor antigens to help eliminate or control tumors. Radiation therapy, for example, increases the mutational burden and induces cell stress as well as immunogenic cell death, thereby exposing an array of otherwise hidden and de novo tumor-associated antigens, stress proteins, and danger-associated molecular patterns to the immune system [98, 99]. Yet standard daily radiation over many weeks may be detrimental to antitumor immunity, as CD8+ T cells are generally very radiation sensitive [100]. Tumor infiltrating lymphocyte (TIL) enrichment after neoadjuvant radiation (RT before surgery) was previously assessed for 40 rectal cancer patients. The densities of CD3+ and CD8+ T lymphocytes significantly increased from pre-treatment biopsy specimens to post-treatment surgically resected specimens [101]. Neoadjuvant RT for early stage breast cancer significantly improves disease-free survival compared to radiation after surgery, arguably due to induction of robust antitumor immunity and immune memory [102]. Numerous clinical trials are currently underway exploring different radiation dose and dose fractionation protocols for clinical efficacy against the targeted tumor and induction of systemic antitumor immunity. However, the large number of possible treatment protocols make a trial-and-error approach unlikely to be successful, and the quest for optimal treatment protocols and treatment combinations may need to include mathematical modeling [30]. As recently demonstrated for radiation-induced local and systemic antitumor immunity, mathematical models may be parameterized by preclinical and clinical data to simulate an array of possible treatment regimens with varying therapeutic modalities, drug dosages, and treatment sequences [44]. Model predictions of optimal treatments can be tested in pre-clinical studies and ultimately prospective clinical trials.
Enhanced selectivity of PDT presents a great opportunity to overcome the limitations of chemotherapy and radiotherapy to potentially impact the efficacy of immune checkpoint inhibitors, and guidance from mathematical modeling may be readily available. The numerous models on tumor-immune interactions studies [55–57, 103] and radiation response modeling studies [59, 89, 104–106] may translate directly to PDT, albeit with different model parameters. These can be obtained from relatively simple and well thought-out in vitro and in vivo studies (Figure 1).
Conclusions
In summary, PDT offers unique selectivity and mechanisms of action for eliciting tumor damage, overcoming classical drug-resistance, and enhancing anti-tumor immunity with a toxicity profile distinct from standard radiation and chemotherapy. To fully harness the benefits of PDT requires complex dosing and scheduling with concurrent treatments. Even arguably simple mathematical models can provide deep insights, and we forecast that the power and utility of integrated models will grow as the molecular underpinnings and multi-scale behavior of the complex tumor ecosystems is further elucidated and incorporated into these models. The rapid advancement of bioinformatics to characterize tumor genetic, transcriptome, and epigenetic heterogeneity as well as molecular mechanisms of interactions with the host microenvironment and immune system will greatly advance current phenomenological models—with the ultimate goal to create a multi-scale computational platform that integrates molecular-level detail, evolutionary principles, and systems biology.
Author Contributions
BS, HE, RL, and EK prepared the manuscript. All authors contributed to editing the final manuscript.
Conflict of Interest Statement
RW reports being a research fund recipient, consultant, advisor, steering committee or DSMB member, or speaker for Tesaro, Clovis, Genentech, Merck, Mersana, Oncomed, Ovation Diagnostics, Janseen, Amgen, and Tapimmune.
The remaining authors declare that the research was conducted in the absence of any commercial or financial relationships that could be construed as a potential conflict of interest.
Acknowledgments
This work was supported by National Institutes of Health Grants K22-CA181611 (to BS) and R00-CA175292 (to IR); and the Richard, and Susan Smith Family Foundation (Newton, MA) Smith Family Award for Excellence in Biomedical Research (to BS).
References
1. Dolmans D, Fukumura D, Jain RK. Photodynamic therapy for cancer. Nat Rev Cancer. (2003) 3:nrc1071. doi: 10.1038/nrc1071
2. Celli JP, Spring BQ, Rizvi I, Evans CL, Samkoe KS, Verma S, et al. Imaging and photodynamic therapy: mechanisms, monitoring, and optimization. Chem Rev. (2010) 110:2795–838. doi: 10.1021/cr900300p
3. Schmidt-Erfurth U, Hasan T. Mechanisms of action of photodynamic therapy with verteporfin for the treatment of age-related macular degeneration. Surv Ophthalmol. (2000) 45:195–214. doi: 10.1016/S0039-6257(00)00158-2
5. Kessel D, Luo Y. Photodynamic therapy: a mitochondrial inducer of apoptosis. Cell Death Differ. (1999) 6:28–35. doi: 10.1038/sj.cdd.4400446
6. Kessel D. Death pathways associated with photodynamic therapy. Med Laser Appl. (2006) 21:219–24. doi: 10.1016/j.mla.2006.05.006
7. Kessel D. Apoptosis, paraptosis and autophagy: death and survival pathways associated with photodynamic therapy. Photochem Photobiol. (2018) 95:119–25. doi: 10.1111/php.12952
8. Spring BQ, Rizvi I, Xu N, Hasan T. The role of photodynamic therapy in overcoming cancer drug resistance. Photochem Photobiol Sci. (2015) 14:1476–91. doi: 10.1039/C4PP00495G
9. Mew D, Wat C-K, Towers G, Levy J. Photoimmunotherapy: treatment of animal tumors with tumor-specific monoclonal antibody-hematoporphyrin conjugates. J Immunol. (1983) 130:1473–7.
10. Goff Hermanto U, Rumbaugh J, Blake J, Bamberg M, Hasan T. Photoimmunotherapy and biodistribution with an OC125-chlorin immunoconjugate in an in vivo murine ovarian cancer model. Brit J Cancer. (1994) 70:474–80. doi: 10.1038/bjc.1994.330
11. Molpus KL, Hamblin MR, Rizvi I, Hasan T. Intraperitoneal photoimmunotherapy of ovarian carcinoma xenografts in nude mice using charged photoimmunoconjugates. Gynecol Oncol. (2000) 76:397–404. doi: 10.1006/gyno.1999.5705
12. Savellano MD, Hasan T. Targeting cells that overexpress the epidermal growth factor receptor with polyethylene glycolated BPD verteporfin photosensitizer immunoconjugates. Photochem Photobiol. (2003) 77:431–9. doi: 10.1562/0031-8655(2003)0770431tctote2.0.co2
13. van Dongen GA, Visser GW, Vrouenraets M. Photosensitizer-antibody conjugates for detection and therapy of cancer. Adv Drug Deliv Rev. (2004) 56:31–52. doi: 10.1016/j.addr.2003.09.003
14. Savellano MD, Hasan T. Photochemical targeting of epidermal growth factor receptor: a mechanistic study. Clin Cancer Res. (2005) 11:1658–68. doi: 10.1158/1078-0432.CCR-04-1902
15. Mitsunaga M, Ogawa M, Kosaka N, Rosenblum LT, Choyke PL, Kobayashi H. Cancer cell–selective in vivo near infrared photoimmunotherapy targeting specific membrane molecules. Nat Med. (2011) 17:1685–91. doi: 10.1038/nm.2554
16. Spring BQ, Abu-Yousif AO, Palanisami A, Rizvi I, Zheng X, Mai Z, et al. Selective treatment and monitoring of disseminated cancer micrometastases in vivo using dual-function, activatable immunoconjugates. Proc Natl Acad Sci USA. (2014) 111:E933–42. doi: 10.1073/pnas.1319493111
17. Lovell JF, Jin CS, Huynh E, Jin H, Kim C, Rubinstein JL, et al. Porphysome nanovesicles generated by porphyrin bilayers for use as multimodal biophotonic contrast agents. Nat Mater. (2011) 10:324. doi: 10.1038/nmat2986
18. Carter KA, Shao S, Hoopes MI, Luo D, Ahsan B, Grigoryants VM, et al. Porphyrin–phospholipid liposomes permeabilized by near-infrared light. Nat Commun. (2014) 5:3546. doi: 10.1038/ncomms4546
19. Spring BQ, Sears BR, Zheng L, Mai Z, Watanabe R, Sherwood ME, et al. A photoactivable multi-inhibitor nanoliposome for tumour control and simultaneous inhibition of treatment escape pathways. Nat Nanotechnol. (2016) 11:378–87. doi: 10.1038/nnano.2015.311
20. Castano AP, Mroz P, Hamblin MR. Photodynamic therapy and anti-tumour immunity. Nat Rev Cancer. (2006) 6:535–45. doi: 10.1038/nrc1894
21. Gollnick S, Evans S, Baumann H, Owczarczak B, Maier P, Vaughan L, et al. Role of cytokines in photodynamic therapy-induced local and systemic inflammation. Brit J Cancer. (2003) 88:1772–9. doi: 10.1038/sj.bjc.6600864
22. Mitra S, Goren EM, Frelinger JG, Foster TH. Activation of heat shock protein 70 promoter with meso-tetrahydroxyphenyl chlorin photodynamic therapy reported by green fluorescent protein in vitro and in vivo. Photochem Photobiol. (2003) 78:615–22. doi: 10.1562/0031-8655.(2003)0780615AOHSPP2.0.CO2
23. Shams M, Owczarczak B, Manderscheid-Kern P, Bellnier DA, Gollnick SO. Development of photodynamic therapy regimens that control primary tumor growth and inhibit secondary disease. Cancer Immunol Immunother. (2015) 64:287–97. doi: 10.1007/s00262-014-1633-9
24. Emens LA, Middleton G. The interplay of immunotherapy and chemotherapy: harnessing potential synergies. Cancer Immunol Res. (2015) 3:436–43. doi: 10.1158/2326-6066.cir-15-0064
25. Wu J, Waxman DJ. Immunogenic chemotherapy: dose and schedule dependence and combination with immunotherapy. Cancer Lett. (2018) 419:210–21. doi: 10.1016/j.canlet.2018.01.050
26. Friedberg JS, Mick R, Stevenson JP, Zhu T, Busch TM, Shin D, et al. Phase II trial of pleural photodynamic therapy and surgery for patients with non-small-cell lung cancer with pleural spread. J Clin Oncol. (2004) 22:2192–201. doi: 10.1200/JCO.2004.07.097
27. Friedberg JS, Culligan MJ, Mick R, Stevenson J, Hahn SM, Sterman D, et al. Radical pleurectomy and intraoperative photodynamic therapy for malignant pleural mesothelioma. Ann Thor Surg. (2012) 93:1658–67. doi: 10.1016/j.athoracsur.2012.02.009
28. Friedberg JS, Simone CB, Culligan MJ, Barsky AR, Doucette A, McNulty S, et al. Extended pleurectomy-decortication–based treatment for advanced stage epithelial mesothelioma yielding a median survival of nearly three years. Ann Thor Surg. (2017) 103:912–9. doi: 10.1016/j.athoracsur.2016.08.071
29. Zhang X, Niedermann G. Abscopal effects with hypofractionated schedules extending into the effector phase of the tumor-specific T-cell response. Int J Radiat Oncol Biol Phys. (2018) 101:63–73. doi: 10.1016/j.ijrobp.2018.01.094
30. Enderling H, Kim S, Pilon-Thomas S. The accelerating quest for optimal radiation and immunotherapy combinations for local and systemic tumor control. Ther Radiology Oncol. (2018) 2:1–3. doi: 10.21037/tro.2018.08.04
32. Oleinick NL, Morris RL, Belichenko I. The role of apoptosis in response to photodynamic therapy: what, where, why, and how. Photochem Photobiol. (2002) 1:1–21. doi: 10.1039/b108586g
33. Chen B, Pogue BW, Hoopes JP, Hasan T. Combining vascular and cellular targeting regimens enhances the efficacy of photodynamic therapy. Int J Radiat Oncol Biol Phys. (2005) 61:1216–26. doi: 10.1016/j.ijrobp.2004.08.006
34. Zuluaga M-F, Lange N. Combination of photodynamic therapy with anti-cancer agents. Curr Med Chem. (2008) 15:1655–73. doi: 10.2174/092986708784872401
35. Rizvi I, Celli JP, Evans CL, Abu-Yousif AO, Muzikansky A, Pogue BW, et al. Synergistic enhancement of carboplatin efficacy with photodynamic therapy in a three-dimensional model for micrometastatic ovarian cancer. Cancer Res. (2010) 70:9319–28. doi: 10.1158/0008-5472.can-10-1783
36. Rizvi I, Obaid G, Bano S, Hasan T, Kessel D. Photodynamic therapy: promoting in vitro efficacy of photodynamic therapy by liposomal formulations of a photosensitizing agent. Laser Surg Med. (2018) 50:499–505. doi: 10.1002/lsm.22813
37. Mallidi S, Watanabe K, Timerman D, Schoenfeld D, Hasan T. Prediction of tumor recurrence and therapy monitoring using ultrasound-guided photoacoustic imaging. Theranostics. (2015) 5:289–301. doi: 10.7150/thno.10155
38. Wilson BC, Patterson MS. The physics, biophysics and technology of photodynamic therapy. Phys Med Biol. (2008) 53:R61. doi: 10.1088/0031-9155/53/9/R01
39. Wang K, Finlay JC, Busch TM, Hahn SM, Zhu TC. Explicit dosimetry for photodynamic therapy: macroscopic singlet oxygen modeling. J Biophot. (2010) 3:304–18. doi: 10.1002/jbio.200900101
40. Zhu TC, Liang X, Kim MM, Finlay JC, Dimofte A, Rodriguez C, et al. An IR navigation system for pleural PDT. Front Phys. (2015) 3:9. doi: 10.3389/fphy.2015.00009
41. Pogue BW, Elliott JT, Kanick SC, Davis SC, Samkoe KS, Maytin EV, et al. Revisiting photodynamic therapy dosimetry: reductionist & surrogate approaches to facilitate clinical success. Phys Med Biol. (2016) 61:R57. doi: 10.1088/0031-9155/61/7/R57
43. Barendsen G. Dose fractionation, dose rate and iso-effect relationships for normal tissue responses. Int J Radiat Oncol Biol Phys. (1982) 8:1981–97. doi: 10.1016/0360-3016(82)90459-x
44. Lea D, Catcheside D. The mechanism of the induction by radiation of chromosome aberrations inTradescantia. J Genet. (1942) 44:216–45. doi: 10.1007/bf02982830
45. Gatenby RA, Maini PK. Mathematical oncology: cancer summed up. Nature. (2003) 421:321. doi: 10.1038/421321a
46. Araujo R, McElwain D. A history of the study of solid tumour growth: the contribution of mathematical modelling. B Math Biol. (2003) 66:1039. doi: 10.1016/j.bulm.2003.11.002
47. Lowengrub J, Frieboes H, Jin F, Chuang Y-L, Li X, Macklin P, et al. Non-linear modelling of cancer: bridging the gap between cells and tumours. Nonlinearity. (2009) 23:R1–91. doi: 10.1088/0951-7715/23/1/r01
48. Gerlee P. The model muddle: in search of tumor growth laws. Cancer Res. (2013) 73:2407–11. doi: 10.1158/0008-5472.can-12-4355
49. Benzekry S, Lamont C, Beheshti A, Tracz A, Ebos JM, Hlatky L, et al. Classical mathematical models for description and prediction of experimental tumor growth. PLoS Comput Biol. (2014) 10:e1003800. doi: 10.1371/journal.pcbi.1003800
50. Nagy JD. The ecology and evolutionary biology of cancer: a review of mathematical models of necrosis and tumor cell diversity. Math Biosci Eng Mbe. (2005) 2:381–418. doi: 10.3934/mbe.2005.2.381
51. Merlo L, Pepper JW, Reid BJ, Maley CC. Cancer as an evolutionary and ecological process. Nat Rev Cancer. (2006) 6:924–35. doi: 10.1038/nrc2013
52. Cunningham JJ, Gatenby RA, Brown JS. Evolutionary dynamics in cancer therapy. Mol Pharmaceut. (2011) 8:2094–100. doi: 10.1021/mp2002279
53. Maley CC, Aktipis A, Graham TA, Sottoriva A, Boddy AM, Janiszewska M, et al. Classifying the evolutionary and ecological features of neoplasms. Nat Rev Cancer. (2017) 17:605–19. doi: 10.1038/nrc.2017.69
54. Kim PS, Lee PP, Levy D. Modeling regulation mechanisms in the immune system. J Theor Biol. (2006) 246:33–69. doi: 10.1016/j.jtbi.2006.12.012
55. Hillen T, Enderling H, Hahnfeldt P. The tumor growth paradox and immune system-mediated selection for cancer stem cells. Bull Math Biol. (2013) 75:161–84. doi: 10.1007/s11538-012-9798-x
56. Wilkie KP, Hahnfeldt P. Tumor–immune dynamics regulated in the microenvironment inform the transient nature of immune-induced tumor dormancy. Cancer Res. (2013) 73:3534–44. doi: 10.1158/0008-5472.can-12-4590
57. Kareva I, Berezovskaya F. Cancer immunoediting: a process driven by metabolic competition as a predator–prey–shared resource type model. J Theor Biol. (2015) 380:463–72. doi: 10.1016/j.jtbi.2015.06.007
58. Yagawa Y, Robertson-Tessi M, Zhou SL, Anderson AR, Mulé JJ, Mailloux AW. Systematic screening of chemokines to identify candidates to model and create ectopic lymph node structures for cancer immunotherapy. Sci Rep. (2017) 7:15996. doi: 10.1038/s41598-017-15924-2
59. Walker R, Poleszczuk J, Pilon-Thomas S, Kim S, Anderson A, Czerniecki BJ, et al. Immune interconnectivity of anatomically distant tumors as a potential mediator of systemic responses to local therapy. Sci Rep. (2018) 8:9474. doi: 10.1038/s41598-018-27718-1
60. McGuire MF, Enderling H, Wallace DI, Batra J, Jordan M, Kumar S, et al. Formalizing an Integrative, multidisciplinary cancer therapy discovery workflow. Cancer Res. (2013) 73:6111–7. doi: 10.1158/0008-5472.can-13-0310
61. Powathil GG, Adamson DJ, Chaplain MA. Towards predicting the response of a solid tumour to chemotherapy and radiotherapy treatments: clinical insights from a computational model. PLoS Comput Biol. (2013) 9:e1003120. doi: 10.1371/journal.pcbi.1003120
62. Werner B, Scott JG, Sottoriva A, Anderson A, Traulsen A, Altrock PM. The cancer stem cell fraction in hierarchically organized tumors can be estimated using mathematical modeling and patient-specific treatment trajectories. Cancer Res. (2016) 76:1705–13. doi: 10.1158/0008-5472.can-15-2069
63. Forouzannia F, Enderling H, Kohandel M. Mathematical modeling of the effects of tumor heterogeneity on the efficiency of radiation treatment schedule. Bull Math Biol. (2018) 80:283–93. doi: 10.1007/s11538-017-0371-5
64. Alfonso J, Parsai S, Joshi N, Godley A, Shah C, Koyfman SA, et al. Temporally feathered intensity-modulated radiation therapy: a planning technique to reduce normal tissue toxicity. Med Phys. (2018) 45:3466–74. doi: 10.1002/mp.12988
65. Ribba B, Boetsch C, Nayak TK, Grimm H, Charo JM, Evers S, et al. Prediction of the optimal dosing regimen using a mathematical model of tumour uptake for immunocytokine-based cancer immunotherapy. Clin Cancer Res. (2018) 24:3325–33. doi: 10.1158/1078-0432.ccr-17-2953
66. Moore H, Strauss L, Ledzewicz U. Optimization of combination therapy for chronic myeloid leukemia with dosing constraints. J Math Biol. (2018) 77:1533–61. doi: 10.1007/s00285-018-1262-6
67. Meier-Schellersheim M, Fraser ID, Klauschen F. Multiscale modeling for biologists. Wiley Interdiscip Rev Syst Biol Med. (2009) 1:4–14. doi: 10.1002/wsbm.33
68. Zhang J, Cunningham JJ, Brown JS, Gatenby RA. Integrating evolutionary dynamics into treatment of metastatic castrate-resistant prostate cancer. Nat Commun. (2017) 8:1816. doi: 10.1038/s41467-017-01968-5
69. Kaznatcheev A, Peacock J, Basanta D, Marusyk A, Scott JG. Fibroblasts and alectinib switch the evolutionary games played by non-small cell lung cancer. Nat Ecol Evol. (2019) 3:450–6. doi: 10.1038/s41559-018-0768-z
70. Gatenby RA. A change of strategy in the war on cancer. Nature. (2009) 459:459508a. doi: 10.1038/459508a
71. Gillies RJ, Verduzco D, Gatenby RA. Evolutionary dynamics of carcinogenesis and why targeted therapy does not work. Nat Rev Cancer. (2012) 12:487–93. doi: 10.1038/nrc3298
72. Silva AS, Kam Y, Khin ZP, Minton SE, Gillies RJ, Gatenby RA. Evolutionary approaches to prolong progression-free survival in breast cancer. Cancer Res. (2012) 72:6362–70. doi: 10.1158/0008-5472.CAN-12-2235
73. Enriquez-Navas PM, Wojtkowiak JW, Gatenby RA. Application of evolutionary principles to cancer therapy. Cancer Res. (2015) 75:4675–80. doi: 10.1158/0008-5472.CAN-15-1337
74. Enriquez-Navas PM, Kam Y, Das T, Hassan S, Silva A, Foroutan P, et al. Exploiting evolutionary principles to prolong tumor control in preclinical models of breast cancer. Sci Transl Med. (2016) 8:327ra24. doi: 10.1126/scitranslmed.aad7842
75. Gatenby RA, Brown J. Mutations, evolution and the central role of a self-defined fitness function in the initiation and progression of cancer. Biochim Biophys Acta Rev Cancer. (2017) 1867:162–6. doi: 10.1016/j.bbcan.2017.03.005
76. Gallaher J, Enriquez-Navas PM, Luddy KA, Gatenby RA, Anderson AR. Spatial heterogeneity and evolutionary dynamics modulate time to recurrence in continuous and adaptive cancer therapies. Cancer Res. (2018) 78:2127–39. doi: 10.1158/0008-5472.can-17-2649
77. Wang K, Mitra S, Foster TH. A comprehensive mathematical model of microscopic dose deposition in photodynamic therapy. Med Phys. (2006) 34:282–93. doi: 10.1118/1.2401041
78. Valentine R, Wood K, Brown C, Ibbotson S, Moseley H. Monte carlo simulations for optimal light delivery in photodynamic therapy of non-melanoma skin cancer. Phys Med Biol. (2012) 57:6327–45. doi: 10.1088/0031-9155/57/20/6327
79. Lopez N, Mulet R, Rodríguez R. Tumor reactive ringlet oxygen approach for Monte Carlo modeling of photodynamic therapy dosimetry. J Photochem Photobiol B Biol. (2016) 160:383–91. doi: 10.1016/j.jphotobiol.2016.04.014
80. Kareliotis G, Liossi S, Makropoulou M. Assessment of singlet oxygen dosimetry concepts in photodynamic therapy through computational modeling. Photodiagn Photodyn. (2018) 21:224–33. doi: 10.1016/j.pdpdt.2017.12.016
81. Yuan J, Mahama-Relue P, Fournier R, Hampton J. Predictions of mathematical models of tissue oxygenation and generation of singlet oxygen during photodynamic therapy. Radiat Res. (1997) 148:386–94. doi: 10.2307/3579524
82. Penjweini R, Kim MM, Finlay JC, Zhu TC. Investigating the impact of oxygen concentration and blood flow variation on photodynamic therapy. Proc Spie Int Soc Opt Eng. (2016) 9694:96940L. doi: 10.1117/12.2211120
83. Gkigkitzis I. Theoretical aspects and modelling of cellular decision making, cell killing and information-processing in photodynamic therapy of cancer. BMC Med Genom. (2013) 6:S3. doi: 10.1186/1755-8794-6-s3-s3
84. López-Marín N, Mulet R. in vivo modelling of apoptosis induced by photodynamic therapy. J Theor Biol. (2018) 436:8–17. doi: 10.1016/j.jtbi.2017.09.028
85. López-Marína N, Mulet R, Rodríguez R. Photodynamic therapy: toward a systemic computational model. J Photochem Photobiol B Biol. (2018) 189:201–13. doi: 10.1016/j.jphotobiol.2018.10.020
86. Svarc A, Jurin M, Borović S, Zorc H. A mathematical model for the effect of red light penetration depth on tumor growth. Acta Med Croatica. (1996) 50:119–24.
87. Leder K, Pitter K, LaPlant Q, Hambardzumyan D, Ross BD, Chan TA, et al. Mathematical modeling of PDGF-driven glioblastoma reveals optimized radiation dosing schedules. Cell. (2014) 156:603–16. doi: 10.1016/j.cell.2013.12.029
88. Walker R, Enderling H. From concept to clinic: mathematically informed immunotherapy. Curr Prob Cancer. (2016) 40:68–83. doi: 10.1016/j.currproblcancer.2015.10.004
89. Poleszczuk JT, Luddy KA, Prokopiou S, Robertson-Tessi M, Moros EG, Fishman M, et al. Abscopal benefits of localized radiotherapy depend on activated t-cell trafficking and distribution between metastatic lesions. Cancer Res. (2016) 76:1009–18. doi: 10.1158/0008-5472.can-15-1423
90. Scott JG, Fletcher AG, Anderson AR, Maini PK. Spatial metrics of tumour vascular organisation predict radiation efficacy in a computational model. PLoS Comput Biol. (2016) 12:e1004712. doi: 10.1371/journal.pcbi.1004712
91. Geng C, Paganetti H, Grassberger C. Prediction of treatment response for combined chemo- and radiation therapy for non-small cell lung cancer patients using a bio-mathematical model. Sci Rep. (2017) 7:13542. doi: 10.1038/s41598-017-13646-z
92. Benzekry S, Lamont C, Barbolosi D, Hlatky L, Hahnfeldt P. Mathematical modeling of tumor–tumor distant interactions supports a systemic control of tumor growth. Cancer Res. (2017) 77:5183–93. doi: 10.1158/0008-5472.can-17-0564
93. Lewin TD, Maini PK, Moros EG, Enderling H, Byrne HM. The evolution of tumour composition during fractionated radiotherapy: implications for outcome. B Math Biol. (2018) 80:1207–35. doi: 10.1007/s11538-018-0391-9
94. Formenti SC, Demaria S. Radiation Therapy to convert the tumor into an in situ vaccine. Int J Radiat Oncol Biol Phys. (2012) 84:879–80. doi: 10.1016/j.ijrobp.2012.06.020
95. Formenti SC, Demaria S. Combining radiotherapy and cancer immunotherapy: a paradigm shift. J Nat Cancer Inst. (2013) 105:256–65. doi: 10.1093/jnci/djs629
96. Heuvers ME, Aerts JG, Cornelissen R, Groen H, Hoogsteden HC, Hegmans JP. Patient-tailored modulation of the immune system may revolutionize future lung cancer treatment. BMC Cancer. (2012) 12:580. doi: 10.1186/1471-2407-12-580
97. Vignali DA, Collison LW, Workman CJ. How regulatory T cells work. Nat Rev Immunol. (2008) 8:nri2343. doi: 10.1038/nri2343
98. Reits EA, Hodge JW, Herberts CA, Groothuis TA, Chakraborty M, K., Wansley E, et al. Radiation modulates the peptide repertoire, enhances MHC class I expression, and induces successful antitumor immunotherapy. J Exp Med. (2006) 203:1259–71. doi: 10.1084/jem.20052494
99. Ciernik IF, Romero P, Berzofsky JA, Carbone DP. Ionizing radiation enhances immunogenicity of cells expressing a tumor-specific T-cell epitope. Int J Radiat Oncol Biol Phys. (1999) 45:735–41. doi: 10.1016/s0360-3016(99)00226-6
100. Schnarr K, Dayes I, Sathya J, Boreham D. Individual radiosensitivity and its relevance to health physics. Dose Resp. (2007) 5:333–48. doi: 10.2203/dose-response.07-022.schnarr
101. Teng F, Mu D, Meng X, Kong L, Zhu H, Liu S, et al. Tumor infiltrating lymphocytes (TILs) before and after neoadjuvant chemoradiotherapy and its clinical utility for rectal cancer. Am J Cancer Res. (2015) 5:2064–74.
102. Poleszczuk J, Luddy K, Chen L, Lee JK, Harrison LB, Czerniecki BJ, et al. Neoadjuvant radiotherapy of early-stage breast cancer and long-term disease-free survival. Breast Cancer Res. (2017) 19:75. doi: 10.1186/s13058-017-0870-1
103. Enderling H, Hlatky L, Hahnfeldt P. Immunoediting: evidence of the multifaceted role of the immune system in self-metastatic tumor growth. Theor Biol Med Model. (2012) 9:31. doi: 10.1186/1742-4682-9-31
104. Poleszczuk J, Enderling H. The optimal radiation dose to induce robust systemic anti-tumor immunity. Int J Mol Sci. (2018) 19:3377. doi: 10.3390/ijms19113377
105. Serre R, Benzekry S, Padovani L, Meille C, André N, Ciccolini J, et al. Mathematical modeling of cancer immunotherapy and its synergy with radiotherapy. Cancer Res. (2016) 76:4931–40. doi: 10.1158/0008-5472.can-15-3567
Keywords: photomedicine, mathematical oncology, photodynamic therapy, immunotherapy, computational modeling, combination therapy, cancer
Citation: Spring BQ, Lang RT, Kercher EM, Rizvi I, Wenham RM, Conejo-Garcia JR, Hasan T, Gatenby RA and Enderling H (2019) Illuminating the Numbers: Integrating Mathematical Models to Optimize Photomedicine Dosimetry and Combination Therapies. Front. Phys. 7:46. doi: 10.3389/fphy.2019.00046
Received: 12 December 2018; Accepted: 11 March 2019;
Published: 02 April 2019.
Edited by:
Zhen Cheng, Stanford University, United StatesReviewed by:
Shuanglong Liu, University of Southern California, United StatesWazir Muhammad, Yale University, United States
Copyright © 2019 Spring, Lang, Kercher, Rizvi, Wenham, Conejo-Garcia, Hasan, Gatenby and Enderling. This is an open-access article distributed under the terms of the Creative Commons Attribution License (CC BY). The use, distribution or reproduction in other forums is permitted, provided the original author(s) and the copyright owner(s) are credited and that the original publication in this journal is cited, in accordance with accepted academic practice. No use, distribution or reproduction is permitted which does not comply with these terms.
*Correspondence: Bryan Q. Spring, b.spring@northeastern.edu
Heiko Enderling, heiko.enderling@moffitt.org