- 1Department of Pharmaceutical Chemistry, Faculty of Medicine, University of Banja Luka, Banja Luka, Bosnia and Herzegovina
- 2Department of Pharmaceutical Chemistry, Faculty of Pharmacy, University of Belgrade, Belgrade, Serbia
Rational drug design implies usage of molecular modeling techniques such as pharmacophore modeling, molecular dynamics, virtual screening, and molecular docking to explain the activity of biomolecules, define molecular determinants for interaction with the drug target, and design more efficient drug candidates. Kinases play an essential role in cell function and therefore are extensively studied targets in drug design and discovery. Kinase inhibitors are clinically very important and widely used antineoplastic drugs. In this review, computational methods used in rational drug design of kinase inhibitors are discussed and compared, considering some representative case studies.
Kinases As Targets For Developing Anticancer Drugs
Kinases belong to a large family of enzymes that catalyze transfer of high energy phosphate group from adenosine triphosphate (ATP) to substrates, such as proteins (the protein-tyrosine kinases, the serine-threonine specific kinases), lipids (phosphatidylinositol kinases, sphingosine kinases), carbohydrates, and nucleic acids (Duong-Ly and Peterson, 2013). Phosphorylation of the substrate modulates its activity and/or interaction with other molecules leading to different physiological responses. It is estimated that 50% of all proteins are constantly undergoing reversible phosphorylation and dephosphorylation, which emphasizes the role of protein kinases in almost all aspects of cell function, including proliferation, cell growth, apoptosis, and signal transduction (Graves and Krebs, 1999; Manning et al., 2002).
Dysregulated, overexpressed, or mutated protein kinases are found in many diseases, including cancer, and over the past two decades they became extensively examined targets for the development of new antineoplastic drugs (Blume-Jensen and Hunter, 2001; Cohen, 2002). There are 53 kinase inhibitors (KIs) currently approved by the FDA (FDA, 2019), while over 200 potential inhibitors are in different phases of clinical trials worldwide (Carles et al., 2018). Majority of the approved drugs are orally active and effective against various malignancies (Table 1; Roskoski, 2019a,b).
Structures of the selected KIs commonly used for treatment of cancer are shown in Figure 1. These drugs target different protein kinases that are frequently upregulated in cancer cells. The epidermal growth factor receptor (EGFR) is a member of the ErbB family of tyrosine kinase receptors that is overexpressed or mutated in non-small cell lung cancer and represents the primary target for drugs such erlotinib and gefitinib (Bethune et al., 2010). Lapatinib and neratinib bind to intracellular domain of the human epidermal growth factor receptor 2 (HER2/neu), another member of the ErbB tyrosine kinases, which elevated levels are found in approximately 20–30% of breast cancers (Collins et al., 2019). Imatinib possesses activity against non-receptor breakpoint cluster region (Bcr)-Abelson leukemia virus (Abl) tyrosine kinase that is formed as a result of a chromosome rearrangement and has been implicated in the pathogenesis of nearly all cases of chronic myeloid leukemia (CML) and acute lymphoblastic leukemia with the Philadelphia chromosome (Iqbal and Iqbal, 2014). Although imatinib is a relatively specific Bcr-Abl inhibitor, it also inhibits the CD117 tyrosine kinase associated with gastrointestinal stromal tumors and has consequently been approved for this indication (Buchdunger et al., 2000). The vascular endothelial growth factor family of receptors (VEGFR) contains a tyrosine kinase domain which activation can lead to induction of signaling pathways that regulate cell proliferation, survival, and promotion of tumor angiogenesis (Morabito et al., 2006). Agents that target VEGFR, including lenvatinib, sorafenib and vandetanib, are frequently used for treatment of thyroid cancers. Vemurafenib, dabrafenib, and encorafenib target BRAF, a serine/threonine protein kinase which mutation is expressed at about 50–60% of cutaneous melanomas where it leads to continuous activation of mitogen-activated protein kinase (MAPK) pathway and uncontrolled proliferation of cancer cells (Yu et al., 2019).
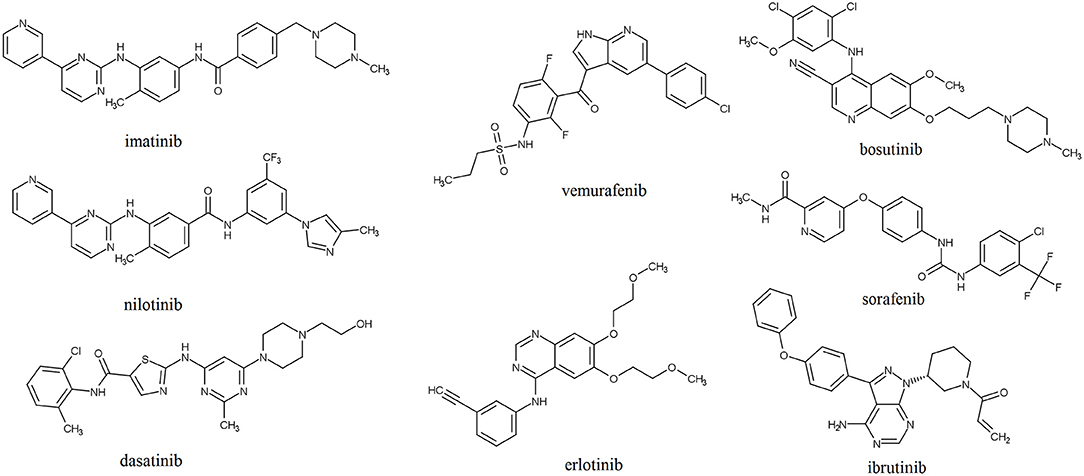
Figure 1. Structures of selected protein kinase inhibitors that have been approved for clinical use.
Structure of Protein Kinases
The human genome encodes at least 518 protein kinases (Manning et al., 2002). Out of them, 478 share highly conserved catalytic domains. The remaining 40 do not share the sequence similarity, but their folding is similar to the folding of “typical” PKs (Caballero and Alzate-Morales, 2012). In 1991, Knighton solved the X-ray structure of cyclic AMP-dependent PK and described its structure for the first time. This description can apply to all currently known protein kinases. The characteristic architecture of the catalytic domain of PK consists of a small, amino-terminal N-lobe and a large α-helical carboxy-terminal C-lobe which are connected with a small hinge region (Figure 2; Knighton et al., 1991). The N-terminal lobe is dominated by five β-strands (β1–β5) and one conserved α-helix (helix C) that occurs in active (αC-in) or inactive (αC-out) orientations. The C-lobe consists of eight α-helices and four short conserved β-strands (β6–β9) which include residues that participate in the phosphorylation of protein substrates. The small and large lobes form a catalytic cleft where ATP binds (Knighton et al., 1991; Roskoski, 2019a). The hydrophobic residues of the cleft form a binding pocket for ATP. The charged residues in the active site bind and position the γ-phosphate of ATP and divalent cation and take part in the catalysis (Knight et al., 2007).
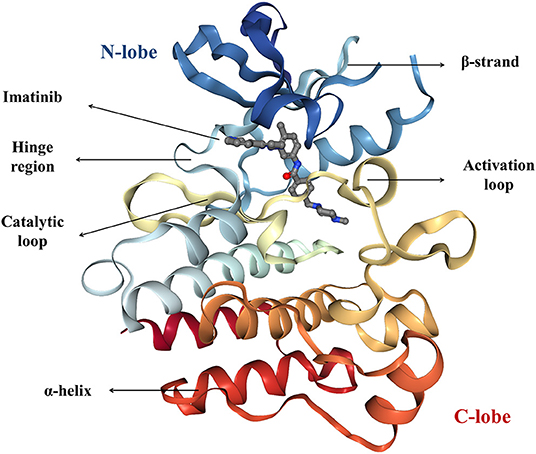
Figure 2. The crystal structure of imatinib-bound form of the Abl kinase (PDB accession code: 2HYY), colored as rainbow from N-lobe (blue) to C-lobe (red). Imatinib is represented as ball and stick.
Conserved residues play crucial roles in positioning ATP, stabilizing the active-conformation and in the catalytic mechanism, and they are mostly found in and around the active site but also in other parts of the protein kinase domain (Knight et al., 2007). Almost all protein kinases possess a conserved K/E/D/D (Lys/Glu/Asp/Asp) signature that is important for the catalysis. Lysine and glutamic acid residues belong to the N-lobe, and the two aspartic acid residues are found in the C-lobe. Lysine residue binds to the α- and β-phosphates of ATP. Formation of the salt bridge between the carboxylate group of aspartic acid and the amino group of lysine stabilizes its interactions with the α- and β-phosphates, and it is required for kinase activation (Roskoski, 2015, 2019a,b).
The N-lobe contains a conserved flexible glycine-rich GxGxxG motif (also called P-loop) between β1 and β2 that folds over the nucleotide and places the γ-phosphate of ATP during the catalysis (Taylor and Kornev, 2011). As mentioned above, lysine from the β3-strand forms a salt bridge with the conserved glutamate near the center of the protein-kinase αC-helix which is necessary for the formation of the active enzyme, and this structure corresponds to the “αC-in” conformation (Roskoski, 2015, 2019a,b).
The C-lobe is important for both the protein-substrate binding as well as nucleotide binding (Roskoski, 2015). The C-lobe contains a mobile activation loop of 20–30 residues which can take open or closed conformation. The activation loop begins with the DFG motif (Asp-Phe-Gly) and extends up to an APE motif (Ala-Pro-Glu) (Modi and Dunbrack, 2019). In the active conformation a divalent metal ion, Mg2+ (or sometimes Mn2+), interacts with a highly conserved aspartic acid residue from the DFG motif. It coordinates with the α and γ phosphates of ATP and facilitates the phosphorylation and coordinates the ATP binding (Adams, 2001). At the other end, glutamic acid from APE motif is fixed by the formation of a salt bridge with arginine from the C-lobe (Roskoski, 2015, 2019b). In addition to these, another motif on the C-lobe is highly conserved suggesting it plays an important role in the catalysis—HRD (rarely YRD) motif. The aspartate residue of this motif is required for the orientation of the hydroxyl group of the substrate peptide at the P-site and the transfer of the phosphoryl group. Arginine residue interacts with the phosphorylated activation segment thereby contributing to its correct orientation. Histidine (or in rare cases tyrosine) is considered to be involved in the maintenance of the conserved rigid organization of the catalytic core (La Sala et al., 2016).
The main differences between tyrosine kinases and serine/threonine kinases are found in the protein-substrate binding site. In serine/threonine kinases, the phosphorylatable serine or threonine of the protein substrate interacts with backbone residues near the end of the activation segment. Basic residues of the protein-substrate N-terminal interact with surface acidic residues of the C-lobe. Additionally, peptide substrate is fixed by serine in the glycine rich loop and lysine in the catalytic loop and also to threonine in the P+1 loop. These three residues are highly conserved in the majority of protein-serine/threonine kinases, and they are positioning the target hydroxyl group of a substrate in the catalytic cleft (near the γ-phosphate of ATP) where the phosphotransfer reaction happens (P-site). Since both serine and threonine hydroxyls are linked to the β-carbon, they have similar mechanisms of the catalysis. On the other hand, in tyrosine kinases, after DFG motif, there is a very stable region that contains the three tyrosine phosphorylation sites. The protein substrate chain positions in a manner that one of the tyrosines is oriented with its hydroxyl group lying in phosphorylation site P-site. The following tyrosine residue lies in the P+1 site. Proline residue interacts with the tyrosyl residue of the protein-substrate and is responsible for positioning the P-site tyrosine in the phosphotransfer site. The tyrosine ring is also positioned by Arg (Hubbard et al., 1994; Taylor et al., 1995; Roskoski, 2015).
Moreover, many protein kinases are regulated by different mechanisms such as dimerization, binding of allosteric effectors, or other modifications important for subcellular localization that can modulate their activity. Binding of an allosteric modulator leads to conformational changes that mostly involve structural reorganization of the activation loop, making it a primary end point of allosteric regulation. Effectors or regulatory subunits bind outside the catalytic site, causing the changes in loop conformation through conformational changes of other substructural elements. In most of the cases, regulators bind the αC helix at different locations, allowing control of catalysis from distal regions (Shi et al., 2006). Nevertheless, the αC helix is not the only allosteric binding site, in fact, they are very diverse (Figure 3; Ohren et al., 2004; Vanderpool et al., 2009; Jahnke et al., 2010; Martin et al., 2012; Park et al., 2015; Rettenmaier et al., 2015; Ung et al., 2018). Therefore, understanding the diversity of allosteric regulatory sites among the kinase superfamily gives a unique opportunity for the creation of novel selective allosteric kinase antagonists (Lamba and Ghosh, 2012).
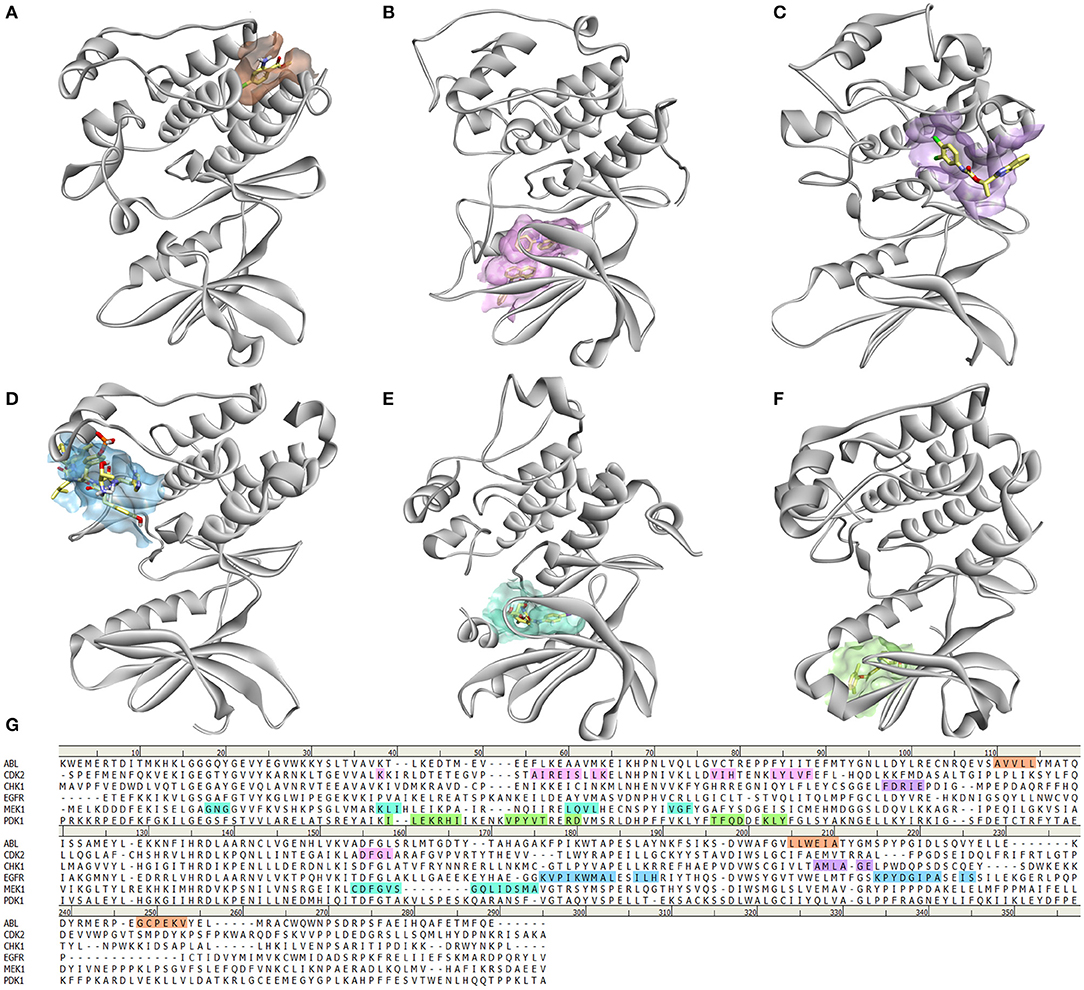
Figure 3. Diversity of allosteric binding pockets in different protein kinases. (A) ABL kinase in complex with fragment FRAG1 (PDB: 3MS9) (Jahnke et al., 2010); (B) CDK2 in complex with 2 molecules of 8-anilino-1-naphthalene sulfonic acid (PBD: 4EZ7) (Martin et al., 2012); (C) CHK1 bound to allosteric inhibitor (1S)-1-(1H-benzimidazol-2-yl)ethyl (3,4-dichlorophenyl)carbamate (PDB: 3JVR) (Vanderpool et al., 2009); (D) EGFR in complex with Mig6 protein (PDB: 4R3P) (Park et al., 2015); (E) PDK1 with PIF pocket inhibitor RF4 (Rettenmaier et al., 2015); (F) MEK1 in complex with 5-bromo-N-(2,3-dihydroxyprpoxy)-3,4-difluoro-2-[(2fluoro-4-iodophenyl)amino]benzamide (PDB: 1S9J) (Ohren et al., 2004); (G) Sequence alignment of these kinases showing which amino acids are involved in the binding of allosteric modulators.
Active/Inactive States
Basically, protein kinases reside in one active state and multiple inactive states (Figure 4). In active kinase conformation, activation loop forms a cleft that binds the substrate. When the substrate peptide binds, it interacts with the HRD motif (His-Arg-Asp). Asp from the DFG motif binds a magnesium ion that interacts directly with an oxygen atom of the β phosphate of ATP. This is followed by formation of a salt bridge between the Glu from the C-helix with a Lys residue in the β3 strand. When the salt bridge is formed, the lysine side chain forms hydrogen bonds with oxygen atoms of α and β phosphates of ATP. The Glycine-rich Loop of the N-lobe stabilizes the phosphates of the bound ATP molecule during catalysis (Taylor and Kornev, 2011; Modi and Dunbrack, 2019). In an inactive conformation, usually the activation loop is blocking the substrate binding, and DFG motif is incompatible with the binding ATP and magnesium ion required for catalysis. Many attempts have been made in order to achieve classification for these conformations and to study interaction of inhibitors in different states (Mobitz, 2015; Ung et al., 2018; Modi and Dunbrack, 2019), and they are all based on the position of highly conserved DFG motif.
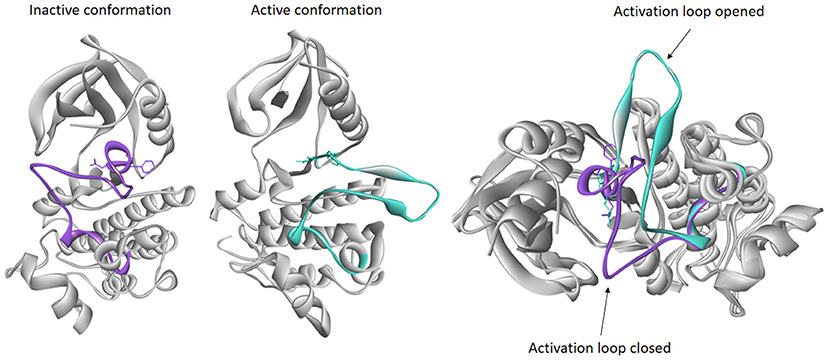
Figure 4. Examples of inactive and active conformations of epidermal growth factor receptor (EGFR) kinase (PDB accession codes: 4HJO and 2GS6, respectively), and their superimposition. The activation loop of inactive conformation (purple) is closed therefore blocking the substrate to enter catalytic loop, while activation loop in active conformation (turquoise) is opened allowing the ATP to bind.
The most recent classification was published by Modi and Dunbrack. They have divided kinase structures into three clusters based on the spatial position of the DFG-Phe side chain into DFG-in, DFG-out, and DFG-inter (intermediate) conformations. Each of these three clusters was further divided based on the dihedral angles required to place the Phe side chain, resulting in total of eight clusters: six for DFG-in and one cluster each for the DFG-out and DFG-inter groups. DFG-in represents the DFG motif orientations where DFG-Phe is packed against or under the C-helix. It contains many conformations, among all the typical DFG-in active conformation belongs to this group. DFG-out represents the structures where DFG-Phe is moved into the ATP binding pocket. DFG-inter represents the conformations in which the DFG-Phe side chain is out of the C-helix pocket but has not moved completely to a DFG-out conformation. Usually in this conformation DFG-Phe is pointing upward toward the β-sheets while dividing the active site into two halves (Modi and Dunbrack, 2019). This classification offers insight into active and inactive kinase conformations which are of great importance in structure-based design of kinase inhibitors.
Types of Kinase Inhibitors
Many reviewers have categorized KIs based on their binding modes into three classes, labeled as types I, II, and III kinase inhibitors (Roskoski, 2016; Bhullar et al., 2018). Type I inhibitors, such as gefitinib, bind to the active DFG-in conformation of a kinase in the phosphorylated ATP catalytic site, and they usually contain a heterocycle that mimic the purine ring of ATP. Considering that the ATP active site is highly conserved among different protein kinases, these ATP-competitive inhibitors display low selectivity profile which may lead to off-target side effects. While the physiological relevance of many off-target effects is still unclear, it was demonstrated that the lack of selectivity is connected with preclinical and clinical cardiotoxicity of kinase inhibitors (Force and Kolaja, 2011; Yang and Papoian, 2012). Possible mechanism behind the KI induced cardiotoxicity lies in binding of these drugs to colony-stimulating factor 1 receptor (CSF1R) (Hasinoff, 2010). Type II inhibitors, such as imatinib, bind to the inactive (DFG-out) conformation of a kinase in the unphosphorylated ATP catalytic site (Dar and Shokat, 2011). Zuccotto introduced type I½ inhibitors as compounds that bind to active ATP catalytic site as type I inhibitors but elongate into the back cavity of the ATP site giving rise to interactions specific for type II pharmacophore. These inhibitors represented by dasatinib, lapatinib, and vemurafenib, display higher selectivity profile as compared to Type I KIs (Zuccotto et al., 2010). Types III and IV are allosteric inhibitors that bind outside the ATP-binding site. Type III includes trametinib that binds to the allosteric site close to the ATP pocket, whereas Type IV inhibitors bind to a pocket distant from the ATP-binding site. Bivalent inhibitor spanning two regions of the protein kinase is termed as Type V (Wong et al., 2017), while KIs that form an irreversible covalent bond with the catalytic site represent Type VI inhibitors (afatinib and ibrutinib) (Roskoski, 2016).
Developing and Overcoming Resistance to Kinase Inhibitors
Despite the significant advances achieved by the use of protein kinase inhibitors, drug resistance remains one of the greatest challenges toward successful cancer treatment. Various mechanisms can underpin the development of resistance to KIs, including alterations in protein kinases, aberration of downstream pathways, or bypass mechanism that activates parallel signaling pathways (Holohan et al., 2013). Mutations of Bcr-Abl kinase domain were found in over 90% of patients with CML who relapsed after an initial response to imatinib. These mutations include different amino acid substitutions at the active site residues or changes in the kinase flexibility that impair its ability to adopt the inactive conformation required for optimal imatinib binding (Shah et al., 2002). Dasatinib is a novel Abl kinase inhibitor that can bind to both the active (mutated) and inactive (normal) conformations of Bcr-Abl, and its activity has been demonstrated in all imatinib-resistant CML patients, with the exception of those with the T315I mutation that prevents formation of critical hydrogen bond (Shah et al., 2004; Burgess et al., 2005). Docking of dasatinib to three Bcr-Abl conformations (active, inactive, and intermediate inactive) showed that dasatinib binds preferentially to an active conformation, and that binding affinity significantly decreases when the kinase adopts inactive conformation (Laurini et al., 2013). Drug combinations targeting different upstream and downstream components within a single pathway, or targeting parallel kinase pathways, have been proved in clinical trials as an efficient method to overcame or delay therapeutic resistance. For instance, treatment with dabrafenib, a selective BRAF inhibitor, and trametinib, a selective MAPK kinase inhibitor, significantly improved progression-free survival of melanoma patients (Flaherty et al., 2012).
In silico Methods Used In Drug Design
Since the approval of imatinib in 2001, protein kinases have received significant attention from academic and pharmaceutical companies, reflected in a large number of publications, solved crystal structures, and identified small molecule inhibitors for about one-fifth of the human kinome (Wu et al., 2015b). Considerable progress in this field is much owed to the use of computational methods that were able to provide valuable information on structural characteristic of both the kinase and the ligand that are important for favorable interaction and desired inhibitory activity (Agafonov et al., 2015). To design inhibitors for protein kinases it is necessary to understand the structure and dynamics of these enzymes, substrate recognition, and reaction of phosphorylation, product release as well as differences between active and inactive conformations.
There are two main approaches within the framework of computer-aided drug design (CADD): structure-based drug design (SBDD), and ligand-based drug design (LBDD). SBDD is based on structural information gathered from biological targets and includes in silico methods such as molecular docking, structure-based virtual screening (SBVS), and molecular dynamics (MD). In contrast, in the absence of information on targets, LBDD relies on the knowledge of ligands that interact with a specific target, and these methods include ligand-based virtual screening (LBVS), similarity searching, quantitative structure-activity relationship (QSAR) modeling, and pharmacophore generation (Ferreira et al., 2015). Over the last years, a large number of studies have reported successful use of CADD in design and discovery of new drugs (Lu et al., 2018b). In this study we provide the comprehensive review of computational tools that led to discovery, design and optimization of KIs as anticancer drugs.
Ligand-Based Methods in Drug Design
QSAR modeling involves the formation of a mathematical relationship between experimentally determined biological activity and quantitatively defined chemical characteristics that describe the analyzed molecule (descriptors) within a set of structurally similar compounds. The QSAR concept originated in the 1860s, when Crum-Brown and Fraser proposed the idea that the physiological action of a compound in a particular biological system is a function of its chemical constituent, while the modern era of QSAR modeling is associated with the work of Hansch et al. in the early 1960s (Hansch et al., 1962). The aim of the QSAR modeling is to utilize the information on structure and activity obtained from a relatively small series of data to ensure that the best lead compounds enter further studies, minimizing the time and the expense of drug development process (Cherkasov et al., 2014).
Classical 2D-QSAR models correlate physicochemical parameters, such as electronic, hydrophobic or steric characteristics of compounds, to biological activity, while the more advanced 3D-QSAR modeling adds quantum chemical parameters. One of the first approaches used in deriving 3D-QSAR models was CoMFA (comparative molecular field analysis). With this analysis, molecules were described with electrostatic and steric fields, which were correlated to biological activity by means of partial least squares regression (PLS) (Cramer et al., 1988). In addition to the steric and electrostatic descriptors, another approach used in deriving 3D-QSAR models was Comparative Molecular Similarity Index Analysis (CoMSIA). CoMSIA approach additionally uses three novel fields comparing to CoMFA, describing the ligand's hydrophobic properties, the presence of the hydrogen bond donors (HBD), and the presence of hydrogen bond acceptors (HBA) (Klebe et al., 1994). The main limitation of the CoMFA/CoMSIA methods is that they are largely dependent on the alignment of 3D-molecular structures which is often a slow process prone to subjectivity. Recently, modern QSAR programs that use new generation of 3D-descriptors, so-called grid-independent (GRIND) descriptors, have been developed and used for multivariate analyses and 3D-QSAR modeling (Pastor et al., 2000; Duran et al., 2009; Smajić et al., 2015; Gagic et al., 2016b).
Recent cases of reported QSAR studies aimed at providing useful information to guide the discovery of new potent KIs are listed in Table 2. Some of them will be discussed in this chapter.
Koneru et al. have used QSAR combined with molecular dynamics to redesign second-generation Src kinase inhibitor RL-45 in order to withstand the gatekeeper residue mutation and enhance binding affinity. They integrated fragment-based drug discovery (FBDD) technique with QSAR and molecular dynamics to assess novel Src kinase inhibitors. Newly designed compounds were assumed to be able to mitigate mutation-related Src kinase resistance and to bind more efficiently to the kinase active site and were proposed for further synthesis (Koneru et al., 2019). Wang et al. applied QSAR studies on a series of 2-acylamino-3-aminothienopyridine analogs in order to design new IKK-β inhibitors (Wang et al., 2019a). Obtained information on physicochemical, structural, electrostatic, and steric properties revealed that bulky aryl substituents at position C3 on the piperidine ring have favorable effect on activity, which led to the design of an in-house library. Compounds with best predicted activities were further subjected to docking studies. Based on these results two new compounds B01 and B02 were identified as potential IKK-β inhibitors, with predicted pIC50 activities of 7.18 and 7.17, and binding affinities of 41.6 and 40.1 kcal/mol, respectively.
Comparative 2D- and 3D-QSAR studies, followed by molecular docking were conducted on a series of quinazoline derivatives acting as EGFR inhibitors (Noolvi and Patel, 2013). According to the 2D-QSAR multiple linear regression (MLR) model, anticancer activity of quinazoline derivatives was influenced by lipophilicity and number of hydrogen bond donors. Presence of short chain ethers such as methoxy-, ethoxy- at C-6 and C-7 positions of quinazoline was found favorable for the activity, while N-containing groups should not be directly attached to the quinazoline ring. 3D-QSAR kNN-MFA (k-nearest neighbor molecular field analysis) revealed that the presence of electronegative groups on the anilino moiety site, electropositive groups at position C7, and a bulky aromatic substituent at C4 increases the EGFR kinase inhibitory activity.
Virtual screening (VS) refers to a group of in silico methods widely used in drug discovery to search large-scale compound databases in order to select a more manageable number of candidates with the highest probability of displaying the desired biological activity (Gagic et al., 2016a; Oluic et al., 2017; Vucicevic et al., 2017; Banegas-Luna et al., 2018). This method has been very popular among pharmaceutical companies since it enables developing drugs in time and cost-effective manner and increases the chance of selected candidates to reach clinical studies. Considering the constant improvement of computational power, it is expected that in the near future VS will be a reasonable alternative to high throughput screening (HTS) (Kumar et al., 2015). There are generally two approaches to screen molecular libraries: LBVS that will be discussed in this section and SBVS.
LBVS is often applied when there are known active compounds, but the target of action is not known, or the crystallographic structure of the protein is not available. These active compounds are then used as ligands to screen molecular libraries based on the similar property principle, which states that structurally similar compounds should possess similar biochemical properties (Nikolic et al., 2015; Bajorath, 2017). For each compound from the virtual library, the similarity with the known active is calculated. Many different strategies for measuring similarity have been developed, including Cosine coefficient, Euclidean distance, Soergel distance, and Tanimoto coefficient (Bajusz et al., 2015). Compounds are ranked based on the similarity score and those at the top are selected as virtual hit molecules for further optimization and synthesis. Modern VS protocols include additional filtering steps in order to exclude compounds that e.g., have low similarity score, do not fall within the Lipinski's rule of five, are not feasible for synthesis or are not available for purchase (Neves et al., 2018).
Besides similarity searches, pharmacophore search is one of the most commonly used LBVS techniques. Given a list of known actives, pharmacophore model can be derived to define the minimum structural requirements that molecule must possess in order to exhibit good activity profile (Vittorio et al., 2019). It is then possible to search large databases, such as PubChem (Kim et al., 2019), ChEMBL (Mendez et al., 2019), and DrugBank (Wishart et al., 2018), for identification of lead compounds that fit to the pharmacophore structure (Bacilieri and Moro, 2006). Several studies that describe the use of LBVS methodology in discovery of potential kinase inhibitors have been listed in Table 3. Pharmacophore-based VS model was employed to search for new tumor progression locus-2 (Tpl2) inhibitors (Teli and Rajanikant, 2012). Tpl2 is a serine/threonine kinase in the MAPK signaling pathway that regulates cell proliferation, survival, and death and participates in many processes of tumor development (Lee et al., 2015). For this purpose, Asinex database was screened using PHASE 3.0 module of the Schrodinger molecular modeling software which resulted in six potential Tpl2 kinase inhibitors. A 3D QSAR pharmacophore model was developed from the structures of known inhibitors of MAPK1 (ERK2) and used for virtual screening of ZINC database (Irwin et al., 2012) that contains over 750 million compounds, DrugBank with 13,443 drugs (Wishart et al., 2018), NCI (https://cactus.nci.nih.gov/ncidb2.2/) with 250,250 structures, Maybridge (https://www.maybridge.com) with over 53,000 compounds and Chembank database (Seiler et al., 2008). Top screened compounds were then subjected to molecular docking that identified new scaffolds with high potency and selectivity against ERK2 (Larif et al., 2014).
It can be concluded that VS strategies, especially Pharmacophore-based VS and combined use of VS and molecular docking, can be a reliable tool for future discovery of new KIs and have a potential to replace a HTS that is costly and time consuming process.
Case Studies
Application of quantitative structure-activity relationship in structure elucidation of Lyn kinase inhibitors
The generalized linear model (GLM) and the artificial neural network (ANN) QSAR models were combined with structural analysis in order to define pharmacophore of Lyn kinase inhibitors (Naboulsi et al., 2018). Lyn kinase is a member of the Src family of tyrosine kinases that was found to be correlated with chemotherapeutic resistance of cancer cells in patients with CML (Chakraborty et al., 2013; Aira et al., 2018). Derived pharmacophore for the inhibition of Lyn kinase suggested the presence of planar heterocyclic ring that contains HBD and HBA, a spacer that allows free bond rotation and central hydrophobic area that is linked to the aromatic ring substituted with lipophilic groups. These structural futures can be found in nilotinib and dasatinib that are approved for treatment of CML (Figure 1). Pyrimidine moiety of nilotinib has the role of the hydrogen bonding region; the attached amino group serves as a spacer that is linked to hydrophobic benzyl group connected with another aromatic ring that is substituted with lipophilic trifluoromethyl group and methylimidazole. Aminopyrimidine moiety is also present in dasatinib that is indicated in CML patients that developed resistance to nilotinib (Okabe et al., 2011). Dasatinib, instead of central hydrophobic benzene ring, contains thiazole connected to an aromatic ring with lipophilic substituents. Results of these QSAR studies can be of great help in future design and lead to optimization of new, more potent Lyn kinase inhibitors for treatment of patients with imatinib and nilotinib-resistant CML.
Quantum mechanical based quantitative structure-activity relationship of N-phenylquinazolin-4-amine derivatives as epidermal growth factor receptor inhibitors
Recently, Simeon at al. applied several 2D- and 3D-QSAR methodologies on a series of EGFR inhibitors, derivatives of N-phenylquinazolin-4-amine (Simeon et al., 2019). 2D QSAR models were created using physico-chemical descriptors, e-state indices and molecular fingerprints, while 3D-QSAR models were developed using CoMFA, CoMSIA, and quantum mechanical (QM) methods. Based on the calculated statistical parameters, the QM-QSAR model displayed better predictive power compared to the other models. Development of this model started with docking of N-phenylquinazolin-4-amine analogs to the EGFR active site and calculation of pairwise interaction energies between each inhibitor and amino acid residues using quantum mechanics. Distances that hold information about the position of the quinazoline ring and the aniline pharmacophores within the active site of the EGFR were extracted and used as descriptors for the QM-QSAR model. Combined 2D- physico-chemical and QM-QSAR model showed even better predictivity and provided more precise information about structural characteristics that are important for EGFR inhibitory activity. Based on the results of this study, it can be concluded that a combination of classical and more advanced quantum mechanical QSAR methodologies represents a good concept for future design of new EGFR inhibitors.
Discovery of potential FGFR1 inhibitors using pharmacophore-based virtual screening
Pharmacophore-based VS protocol was developed in Maestro 9.0 software package (https://www.schrodinger.com/) and used to screen SPECS database (http://www.specs.net) for potential FGFR1 inhibitors (Zhou et al., 2015). Database was previously filtered to extract only compounds with drug-like properties that comply with the Lipinski's rule of 5. Activities of top ranked compounds were predicted with constructed atom-based 3D-QSAR model, and those with highest activities were purchased for experimental enzyme assay. Nineteen hits exhibited moderate inhibitory activity with more than 50% FGFR1 inhibition at 50 μM concentration and IC50 values of most active compounds were 7.9 and 55.5 μM. It should be mentioned that the identified compounds had low structural similarity with previously reported FGFR1 inhibitors and offered novel chemical scaffolds for future optimization of FGFR1 inhibitors.
Structure based methods in drug design
Recent progresses in the field of X-ray crystallography, Nuclear Magnetic Resonance (NMR) techniques, and cryo-electron microscopy (CEM) caused a significant increase in the number of known 3D structures of proteins (Sun et al., 2011). With known 3D structures of proteins, docking became a method of choice in drug design.
Molecular docking predicts the most probable orientation of one molecule toward another (Lengauer and Rarey, 1996). It can be performed between a small molecule and a target protein (ligand-protein docking) or between two proteins (protein-protein docking). In ligand-protein docking, which will be discussed here, the samples of conformations of small molecules–ligands are placed into the binding sites of protein, where scoring functions are used to calculate which of these conformations best fits the target protein binding site (Sousa et al., 2006; Warren et al., 2006). Overall, docking protocols include search algorithm and a scoring function. Initially, the search algorithm is used to orient small molecules in the target binding site (Taylor et al., 2002). Sampling of conformational space has to be carried out with acceptable accuracy to determine the conformation that best fits the binding site, but fast enough to evaluate a large number of docked ligands. With today's computer power it would be impossible to explore all the degrees of freedom for ligand and protein complex. Therefore, there are different ways to overcome this problem. Search algorithms can be systematic and stochastic and deterministic (Novič et al., 2016). Systematic search algorithms sample the search space at predefined intervals while stochastic make random changes until a user-defined termination criterion is met, and because of that outcome can vary (Morris and Lim-Wilby, 2008). Search algorithm is then followed by scoring function that estimates the affinity of ligand through the assessment of interactions between ligands and potential targets (Kitchen et al., 2004). Scoring functions can be physics-based, empirical, knowledge-based, and machine learning-based (Liu and Wang, 2015; Li et al., 2019). The physic-based scoring function computes the free energy of binding by summing up the van der Waals and electrostatic interactions between the protein–ligand (enthalpy), and adding the torsion entropy of ligand as well as the solvation/desolvation effect described by explicit and implicit solvent models (Huang et al., 2006; Liu and Wang, 2015). Empirical scoring function estimates the binding affinity of a complex by accumulating significant energetic factors for protein–ligand binding (hydrogen bonds, hydrophobic effects, steric clashes, etc.). It uses a training set with known binding affinities of protein–ligand complex and optimizes the weights of the energetic factors by the means of regression analysis (Eldridge et al., 1997; Liu and Wang, 2015). The knowledge-based scoring functions also uses structural information of large set of known protein–ligand complexes and converts it into distance-dependent Helmholtz free interaction energies (Muegge and Martin, 1999; Li et al., 2019). Machine-learning based scoring functions for docking are getting more interests nowadays. These methods combine QSAR analysis and protein–ligand interaction evaluation. They combine QSAR analysis and protein–ligand interaction evaluation. The training set of protein–ligand complexes with known structures and binding affinities is required for a model calculation. Structural interaction fingerprints between a protein and a ligand are coded with certain descriptors (electrostatic interactions, hydrogen bonds, or aromatic stacking, surface or shape properties, molecular weight, number of rotatable single bonds, etc.). Then, different machine-learning algorithms are employed for variable selection (Deng et al., 2004; Zhang et al., 2006).
Molecular docking can be employed in many parts of drug discovery process, such as structure–activity studies, lead optimization, structure based virtual screening, binding modes defining, chemical mechanism studies, etc. (Nikolic et al., 2013; Bautista-Aguilera et al., 2014; Oluic et al., 2017; Albert et al., 2019). Most popular docking programs are DOCK (Kuntz et al., 1982), Autodock (Morris et al., 2009), AD Vina (Trott and Olson, 2010), GOLD (Verdonk et al., 2003), GLIDE from Schrödinger suite (Halgren et al., 2004), and they mostly differ in search algorithms and scoring functions they use. It is always recommendable to explore several different docking programs and then decide on the best one for the specific protein-ligand complexes.
For the last decade, molecular docking has been widely used in design of protein kinase inhibitors (Table 4). Tsou et al. designed 4-(phenylaminomethylene) isoquinoline-1, 3(2H, 4H)-dione derivatives, an original class of potent inhibitors that selectively inhibit CDK4 over CDK2 and CDK1 activities. They used SAR and docking to identify interactions between the ligands and residues of the protein's ATP binding pocket and to find interactions with amino acids unique to CDK4 (His82, Val83, and Asp84) and to optimize compounds with improved activity and selectivity toward CDK4 (Tsou et al., 2008). Gopalsamy et al. identified a compound as B-Raf inhibitor from high throughput screening (HTS) and used docking into the crystal structure of B-Raf-Sorafenib complex (1UWH) (Wan et al., 2004) to identify important protein–ligand interactions (two hydrogen bonds with Glu500 and Asp593, and hydrophobic interactions with Ile462, Trp530, Phe582, Ile 512, His 573, and Ile 571) and to optimize the scaffold to obtain compound with improved potency (Gopalsamy et al., 2009). In 2018, Amr et al. synthetized a series of macrocyclic pyrido-pentapeptide candidates, and identified their activity in vitro on several kinases. Following docking study of the best compound into VEGFR-2, EGFR, PDGFR, provided information of the binding mode and important protein-ligand interactions which can be further used as a guideline for future design (Amr et al., 2018). In their efforts to design 2-phenazinamine derivatives as Bcr-Abl tyrosine kinase inhibitors, Kale and Sonwane combined molecular docking studies with G-QSAR (Group-Based QSAR). Their in silico studies predicted better activity for the thiazolidones and benzenesulfonyl derivatives of phenazinamines than doxorubicin. However, in vitro cytotoxic activity was good, though still less than of doxorubicin (Kale and Sonwane, 2018).
Molecular dynamics (MD) is a simulation technique for studying time dependent evolution of molecular system. Relying on principles of classical mechanics, in MD simulations, positions, and velocities of atoms are computed by classical (Newtonian) laws of motion (Klepeis et al., 2009). The forces acting on these atoms are computed using potential energy functions known as force fields. All common force fields express potential energy through bonded terms (covalent bond-stretching, angle-bending, torsion potential, improper torsions) and non-bonded terms (Lenard Jones repulsion and dispersion and Coulomb electrostatics) (Vanommeslaeghe et al., 2014). Several force fields were found to provide quite accurate representations of the structure and dynamics of a number of small globular proteins on the sub-microsecond timescale (Beauchamp et al., 2012). Most commonly used force fields today are CHARMM (Yin and MacKerell, 1998), AMBER (Weiner et al., 1984; Cornell et al., 1995), GROMOS (Oostenbrink et al., 2004), OPLS (Jorgensen et al., 1996), and COMPASS (Sun, 1998) force fields since they include various chemical groups present in macromolecules and drug-like entities.
Recent algorithmic advances and increase in computational power have enabled simulation studies of protein systems on biophysically-relevant timescales. Combined with modern improvements in the quality of force field parameters, protein structure prediction and modeling has advanced impressively (Beauchamp et al., 2012; Raval et al., 2012; Piana et al., 2014). Providing structural and dynamical insight into the studied molecular system difficult to obtain experimentally, as well as thermodynamics and kinetic understanding of the system, MD simulations are usually referred to as “computational microscopes” (Dror et al., 2012). In this review, we discuss the usefulness of MD and MD-based methods in the discovery of kinase inhibitors through different case studies presented below.
Structure-based virtual screening (SBVS) is based on the knowledge of the 3D structure of the target protein, obtained by X-ray crystallography, NMR, cryo-EM or homology modeling (Lionta et al., 2014). Nowadays, the SBVS methods are enabled thanks to a large number of 3D structural information deposited in the PDB. As described above, by using the 3D structural information of the protein target, we are now able to investigate the basic molecular interactions involved in ligand-protein binding and understand experimental results up to atomic levels. In SBVS, large libraries of commercially available drug-like compounds that are computationally screened against proteins of known structure and those that are predicted to bind well can be experimentally tested (Benod et al., 2013; Vucicevic et al., 2016; Oluic et al., 2017).
Case Studies
Structure-based design of imidazo [4,5-b]pyridin-2-one-based p38 mitogen-activated protein kinase inhibitors
Using structure-based drug design, Kaieda et al. have identified a series of potent p38 mitogen-activated protein kinase inhibitors. First they identified the lead compound with moderate inhibitory activity toward p38 MAP kinase by means of high-throughput screening. The lead compound was then crystalized with the MAP kinase. The X-ray crystallographic results showed that carbonyl group of the compound forms two hydrogen bonds with the backbone amide of Met109 and Gly110 of the enzyme (Figure 5A). The hinge backbone conformation of their crystal structure was different from that typically seen in protein kinases. Namely, usually the backbone amide and carbonyl group of Met109 are directed toward the ATP binding site and accessible for creation of hydrogen bonding with ligand. In the obtained crystal structure a flip of the peptide bond between Met109 and Gly110 was noticed which led to a switching of the hydrogen-bond acceptor and donor distribution around the peptide plane, instead. It was assumed that this flip could be responsible for the high kinase selectivity. After switching the scaffold of the carbonylpiperidine group while maintaining this binding mode, a series of synthetized imidazo[4,5-b]pyridin-2-one derivatives were identified as potent inhibitors of the p38 MAP kinase (Figure 5B; Kaieda et al., 2019).
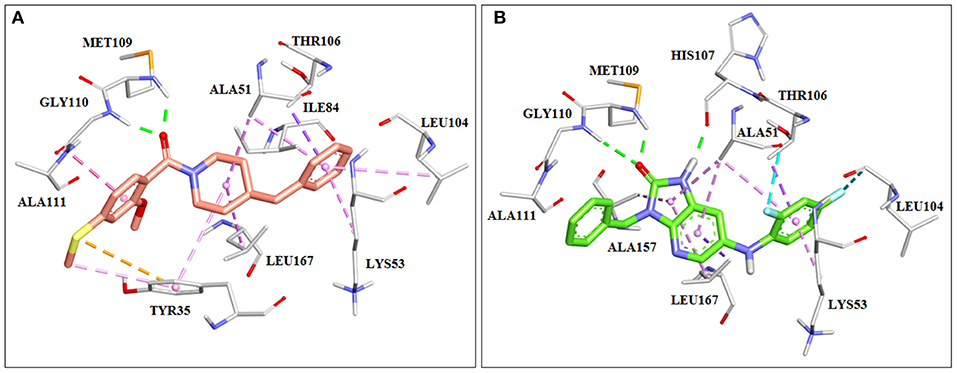
Figure 5. Crystal structures of p38 mitrogen-activated protein (MAP) kinase with imidazo[4,5-b]pyridin-2-one derivatives. (A) Lead compound found by HTS (PDB: 6M95). (B) Potent p38 MAP kinase inhibitor designed using structure-based drug design (SBDD) approach (PDB: 6M9L).
Discovery of novel Pim-1 kinase inhibitors by support vector machine, pharmacophore modeling and molecular docking
In 2011 Ren et al. reported the discovery of novel potent Pim-1 inhibitors by combining ligand- and structure-based filtering methods. In order to find new molecules, a pipeline was created that consisted of support vector machine-based VS (SVM-based VS), pharmacophore-based VS (PB-VS), and docking-based VS (DB-VS) and screened approximately 20 million molecules. Protocol was evaluated by using the library which contained 203 known Pim-1 inhibitors and around 117,000 generated decoys. For validation of the performance of VS, the percentage of predicted compounds in known inhibitors, percentage of known inhibitors in predicted compounds, as well as enrichment factor were calculated. The combined protocol showed much better performance than solely SB-VS, PB-VS, and DB-VS. Finally, 47 compounds were selected for further in vitro Pim-1 kinase inhibitory assay for an inhibitor concentration of 10 μM, and 15 compounds showed nanomolar level or low micromolar inhibition potency against Pim-1. In conclusion, new scaffolds with the potential for the future chemical development were found (Ren et al., 2011).
Discovery of pazopanib, vascular endothelial growth factor family of receptor inhibitor
In 2008 Harris et al. published a paper explaining their discovery of pazopanib. That was a good example of usage of homology modeling and SBDD in the discovery of a drug that is today on the market. Since the crystal structure of VEGFR2 was not available at that time, a homology model of the VEGFR2 enzyme based on FGFR crystal structures was created to predict the binding mode of dimethoxyquinazoline analogs. It was noticed that the pyrimidine and the quinazoline bound similarly in the ATP binding site, making the hydrogen bonds with the Cys919 of the backbone (Figure 6). Crystallization of these compounds with VEGFR2 confirmed in silico results (PDB: 1Y6A, 1Y6B). Finally a series of new analogs was designed, synthetized, and tested in vitro, which led to the discovery of pazopanib (Harris et al., 2005, 2008).
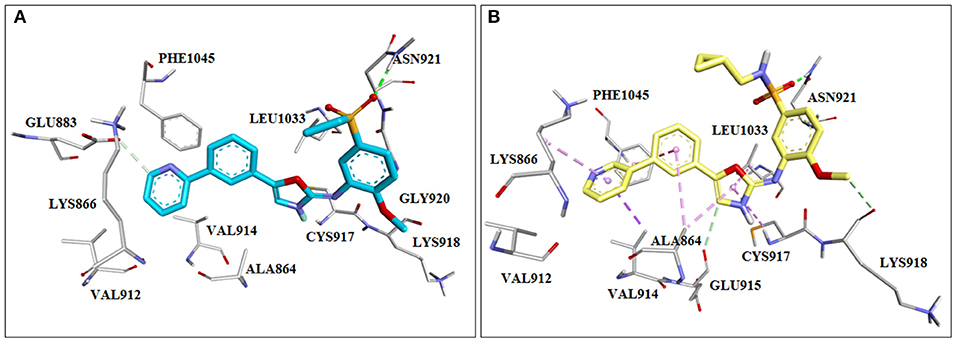
Figure 6. Crystal structures of initial screening hits for inhibitors of the vascular endothelial growth factor (VEGF) that lead to the discovery of pazopanib. (A) PDB: 1Y6A, (B) PDB: 1Y6B.
Rational discovery of dual-indication multitarget phosphodiesterase/ kinase inhibitor
One of the latest studies published this year by Lim et al. combined molecular docking with other bioinformatics tools, with the goal of finding multi-target-multi-indication drugs (Lim et al., 2019). They have used structural and chemical genomics data and combined tools from bioinformatics, chemoinformatics, protein-ligand docking, and machine learning to create a novel structural systems pharmacology platform−3D-REMAP. It used four networks as input: 1. protein–ligand association, 2. off-target, 3. ligand–ligand similarity, and 4. protein–protein similarity. The protein–ligand associations were obtained from ChEMBL, DrugBank, and from other publications about kinome assays (Christmann-Franck et al., 2016; Drewry et al., 2017; Klaeger et al., 2017; Merget et al., 2017) and protein structure-based off-target prediction from binding pocket similarity search and protein–ligand docking. Ligand–ligand similarity was calculated in MadFast software from ChemAxon, and protein–protein similarity was run through BLAST. Moreover, to validate and show advantages of their platform, they searched for marketed drugs that could be dual-indication agents. In their study, they focused on drugs that could reduce the cardiotoxicity of anti-cancer therapy. They predicted that levosimendan, a phosphodiesterase (PDE) inhibitor which is used for heart failure, also inhibits serine/threonine-protein kinase RIO kinase 1 (RIOK1) and several other kinases [Ca2+/calmodulin-dependent protein kinase II (CAMK2), FMS-like tyrosine kinase 3 (FLT3), RIOK3, etc.]. To validate their results they tested anti-cancer activity of levosimendan for more than 200 cancer cell lines. Their experimental results showed that levosimendan is active against several cancers, particularly lymphoma, through the inhibition of RIOK1 and its RNA processing pathway (Lim et al., 2019). Since this study is brand new, the time will tell whether levosimendan will be a candidate for clinical research.
Fragment-based drug design of kinase inhibitors
Discovery of kinase inhibitors is a highly competitive process wherein teams of experienced researchers, both from academia and industry, use all the previous knowledge and new ideas to provide more effective therapies for patients. Depending on the available methodologies, one research group may start their drug discovery project with a high-throughput screening (HTS) campaign and search for the bioactive (HIT) compounds against the studied kinase. Selected HIT molecules usually possess drug-like properties and should be further optimized with the aid of lead optimization techniques. Contrary to drug-like molecules, fragments have a smaller number of heavy atoms (HA) and they should comply with Rule of Three (RO3), in which molecular weight is <300 Da, number of hydrogen bond donors and acceptors should be ≤ 3 and clogP is ≤ 3 (Congreve et al., 2003).
Fragment molecules tend to show high micromolar to millimolar affinities for a certain biological target. The advantages of using fragments in drug design studies of novel kinase inhibitors are numerous:
- Fragments displaying affinity to the examined biological target can overcome the entropy barrier and their binding is related to the favorable enthalpy contribution (Murray and Verdonk, 2002);
- Comparing to drug-sized molecules, pharmacokinetic and physicochemical properties of fragments could be more efficiently optimized (Leach and Hann, 2011);
- Drug-sized molecules may suffer from a potential loss of complementarity with the studied targets, whereas the fragments seldom possess functional groups that establish ligand–protein intermolecular clashes (Hann et al., 2001);
- Given all the above, FBDD projects can lead to increased HIT rates and discovery of novel fragments interesting from different points of view (binding affinity, synthetic accessibility, intellectual property).
Historically, first FBDD projects were applied by a technique named “SAR by NMR” (structure-activity relationship by nuclear magnetic resonance) (Shuker et al., 1996). In this paper, authors successfully developed a potent compound with nanomolar affinity to FK506 binding protein (FKBP) by merging two building blocks. Except for NMR, protein-fragment interacting patterns are characterized by other biophysical methods such as X-ray crystallography, surface plasmon resonance (SRC), high concentration screening (HCS) assays, isothermal titration calorimetry (ITC), fluorescence correlation spectroscopy and many more (Sun et al., 2011). The choice of a particular method depends on the previous experience in FBDD projects and also the size of fragment libraries.
Until now, fragment-based drug discovery (FBDD) method resulted in FDA approval of three kinase inhibitors—vemurafenib (Bollag et al., 2012), venetoclax (Deeks, 2016), and erdafitinib (Markham, 2019). These excellent textbook examples of FBDD are developed by different biophysical methods; nevertheless, the present review focuses on various in silico techniques frequently used in fragment identification and optimization.
In recent years, experimental screening procedures may be replaced by computational methods to reduce the costs and time for early stages of FBDD project (Alves Avelar et al., 2019; Ruzic et al., 2019). It appears that in silico studies may support kinase drug discovery at almost every stage of fragment-based drug design projects. Various ligand-based virtual screening (Giordanetto et al., 2011), structure-based (Warner et al., 2006; Zhao et al., 2012), and quantum mechanical (Machrouhi et al., 2010) techniques have been proved as successful in novel fragment identification. Before running any virtual screening protocol, computational chemists must pay attention to the valid preparation of fragment library database. The fragment library databases should obey the aforementioned Rule of 3 (RO3); additionally, their chemical properties are filtered through certain software which removes possible toxicophores and pan-assay interference compounds (PAINS) (Baell and Walters, 2014). Nowadays, computational chemists may use kinase fragment libraries which may assist faster identification of novel hinge binding motifs. Moreover, fragments that target distal pockets from the ATP binding pocket could be scanned by allosteric kinase library, such as Enamine Allosteric Kinase Library (https://enamine.net).
Case Studies
Identification of PI3K p110β selective fragment
Intracellular lipid kinases that transfer a phosphate group from ATP to certain cell membrane's phospholipids (Phosphoinositide-4,5-biphosphate, PIP2) belong to the family of phosphoinositide 3-kinases (PI3K). These enzymes regulate important cellular events and present interesting drug targets in anticancer drug discovery. Giordanetto et al. (2011) successfully identified fragments that showed selective p110β inhibition. At the time this study was performed, the crystal structure of p110β isoform was not available. Consequently, the homology model was built in MODELLER (Webb and Sali, 2016) by using the crystal structure of p110γ isoform. In this study, authors used AstraZeneca's virtual fragment database and subjected 183,330 fragments to a molecular docking study in GLIDE software (Schrödinger, New York). The poses and orientation of the fragments in the ATP binding pocket were inspected, as well as hydrogen bonding interactions with amino acid residues in the hinge region, affinity and selectivity pocket. The authors reported five chemical classes of fragments (Figure 7A) based on the different heterocyclic rings interacting with the hinge region in p110β and their in vitro enzymatic profiles against four human PI3K isoforms (p110α, p110β, p110γ, and p110δ). Overall, the hit rate achieved from this screening was 8.57%, indicating good performance of the molecular docking-based search for novel and chemically interesting fragments as PI3K hinge binders. The authors continued this study with the morpholine derivative, compound (1) (Figure 7B), which showed moderate potency against p110β (IC50 = 34 μM), but its inhibition of the other p110 isoforms was not determined at the tested concentrations.
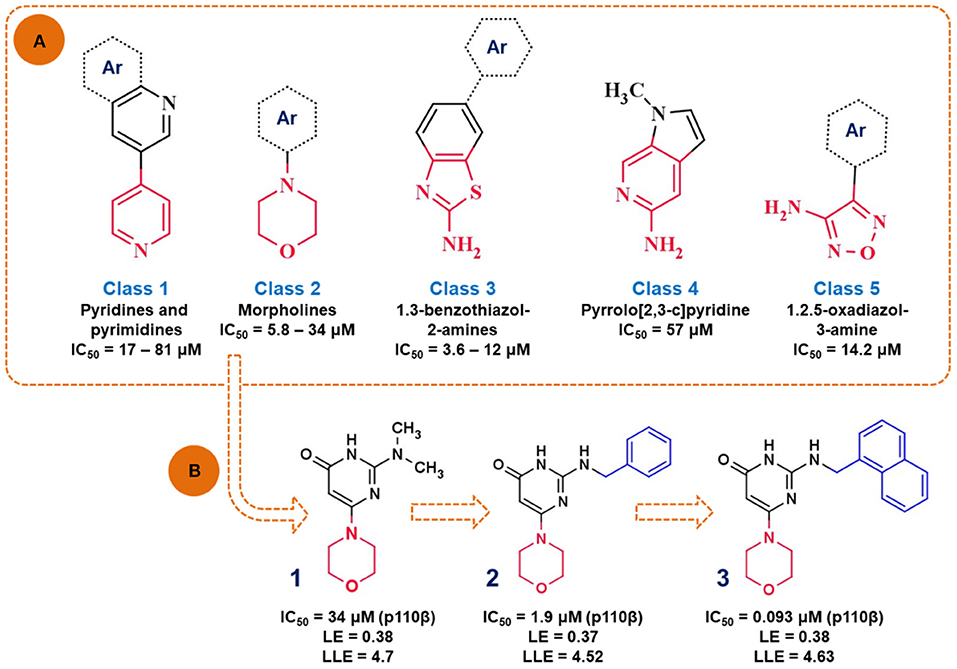
Figure 7. (A) Presentation of the identified scaffolds by in vitro biochemical screening as PI3K inhibitors (hinge interacting moieties are labeled in red), Ar—presents carbocyclic or heterocyclic aromatic rings; (B) the scheme of lead optimization of the selected morpholine derivatives.
In the following study, authors aimed to improve the affinity of the compound (1) by substituting the dimethylamino group with a more voluminous 2-(benzylamino) moiety (Giordanetto et al., 2012). The novel compound (2) showed improved potency (IC50 = 1.9 μM) and efficiency (LE = 0.37 and LLE = 4.52) toward p110β. The rationale for this chemical modification relies on the observation that the bulkier substituents might target amino acid residues M804 and W812 in the proximal selectivity pocket. Finally, compound (3) was synthesized by introducing the naphthyl group, which in turn attributed to the nanomolar potency (IC50 = 0.093 μM) and improved p110β selectivity profile of compound (3).
Identification of mitogen-activated protein kinase-interacting kinase 1 inhibitors
Mitogen-activated protein (MAP) kinase interacting kinases 1 and 2 (MNK1 and MNK2) carry out phosphorylation reaction of eukaryotic translation initiation factor 4E (eIF4E) on serine 209 (Wendel et al., 2007). This translation factor is involved in different cellular pathways, such as Ras/Raf/MEK/ERK and PI3-kinase/protein kinase B (Akt) signaling pathways (Proud, 2015). The overexpression of phosphorylated eIF4E leads to several malignant diseases, such as lymphomas, breast cancer, and glioblastoma (Astanehe et al., 2012). The significance of MNK1/2 enzymes in malignant transformation of the cell has led to high demand for drug design of MNK1/2 inhibitors.
One remarkable study was performed in 2010, where Oyarzabal et al. identified a highly potent and efficient fragment entirely by in silico modeling. In this comprehensive study, authors combined different virtual screening techniques to identify pharmacological tools for MNK1 inhibition. Initially, the Centro Nacional de Investigaciones Oncológicas (CNIO) database was filtered according to the molecular weight (<300 Da) and calculated solubility values (threshold −4 mol/L). By performing this prefiltering procedure, the authors extracted 42,168 fragment-like compounds for virtual screenings (Oyarzabal et al., 2010).
Availability of the crystal structure of MNK2 complexed with staurosporine (PDB: 2HW7) enabled creating minimal substructure, required for crucial interactions with MNK2 (Figure 8). The GOLD software (Jones et al., 1997) used in this study was able to reproduce the binding mode of staurosporine in MNK2. The virtual substructure was docked in the crystal structure of MNK1 (PDB: 2HW6) to similarly elucidate crucial amino acid interactions in the ATP binding pocket. MNK1 pharmacophore prepared in this way was used for pharmacophore fitting study, as a molecular docking alternative and 92 compounds were extracted according to their goodness of fit with the pre-defined substructure.
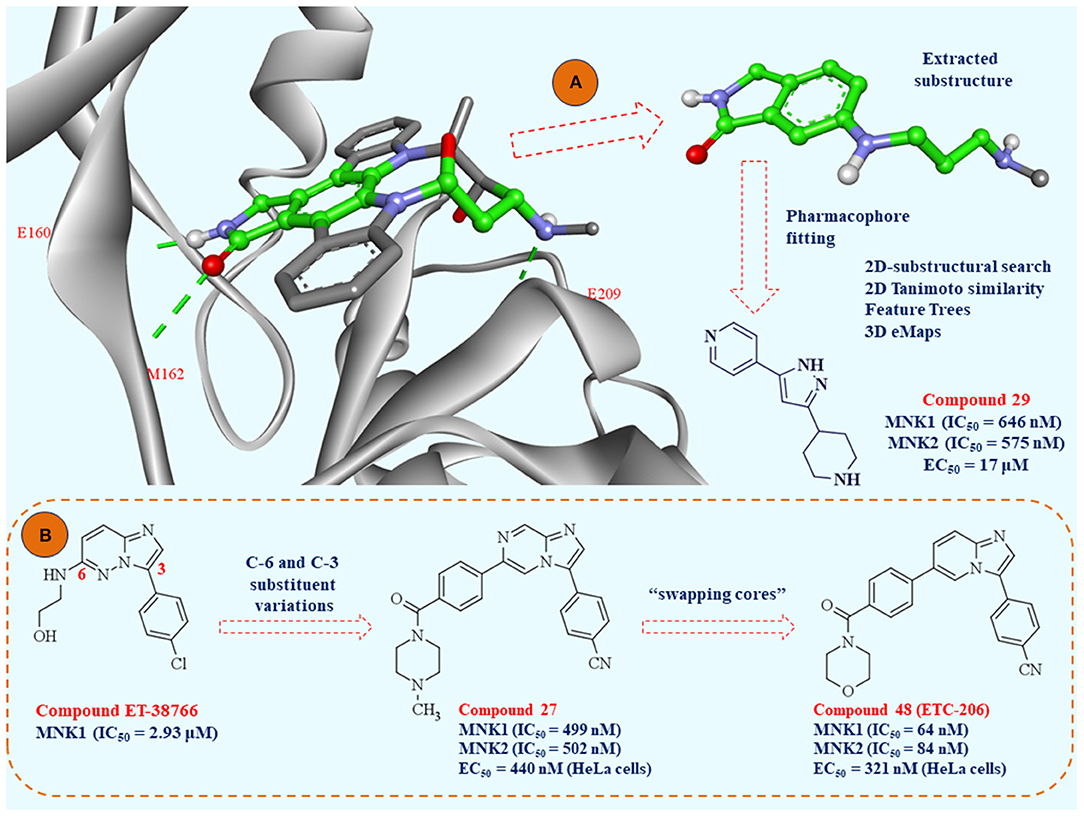
Figure 8. (A) Crystal structure of MNK-2 complexed with staurosporine (PDB: 2HW7); the atoms of the substructure used in the study of pharmacophore fitting screening are labeled in green; (B) the lead optimization strategies starting from fragment ET-38766 to clinical candidate ETC-206.
Structure-based virtual screening protocols were combined with ligand-based virtual screenings of CNIO database and external virtual database of compounds collected by the authors who performed this study. These strategies involved 2D-substructural searches, 2D Tanimoto structural similarity, Feature Trees similarity, and three-dimensional shape and electrostatic similarities based on two reported MNK1 inhibitors. Finally, the authors selected 1,236 compounds for biochemical MNK1 assay and 26 of them were active. The hit ratio of this screening was 2.10% and 10 different scaffolds were represented. Interestingly, one compound (Figure 8, compound 29) demonstrated nanomolar MNK1 (IC50 = 646 nM) and MNK2 (IC50 = 575 nM) inhibition. Additionally, at the cellular level, compound 29 showed an antiproliferative effect against acute myeloid leukemia cell line (MV4:11, EC50 = 17 μM) with dose-dependent decrease in phosphorylation on serine 209 in eIF4E. In conclusion, this study identified 26 hit molecules as MNK1 inhibitors, with 19 of them as fragments with high ligand efficiency values. Among the 26 identified hits, there were 10 diverse chemotypes represented for further drug design studies.
Researchers from A-STAR were particularly interested in imidazopyridazine scaffold (chemotype III in the study of Oyarzabal et al.) as a starting fragment for lead optimization of MNK1/2 inhibitors (Yang et al., 2018). Extensive SAR study of imidazopyridazine derivatives was based on in silico conclusions defined in their previous computational study (Kannan et al., 2017). Concisely, researchers in this study aimed to modify the heterocyclic core in positions 3 and 6, with later modification of the imidazopyridazine scaffold (swapping cores strategy). All the derivatives synthesized in this study were firstly examined by molecular docking studies in Glide 2017-3 software (www.schrodinger.com). By detailed computational analysis of the important amino acid residues in the ATP pocket of MNK1/2 kinases, researchers performed initial lead optimization of the fragment (compound ET-38766) to compound 27 (Figure 8B). Novel compound 27 bears imidazopyrazine scaffold, with improved potency against MNK-1 and MNK-2, cell permeability and improved pharmacokinetic properties. After finding optimal substituents in positions 3 and 6, the final step of lead optimization was focused on detailed DFT study to select the final heterocyclic core of MNK1/2 inhibitors. Initially, it was unclear from molecular dynamics (MD) simulations whether the imidazopyrazine N-7 contributes favorably to the binding affinity of MNK inhibitors. To examine this, the authors performed DFT study and demonstrated that N-7 is mostly solvent exposed, thus the final selected heterocyclic core was imidazopyridine. The most promising compound 48 (Figure 8B) later designated as ETC-206, was presented as superior compared to other derivatives in the study. This compound was investigated for the synergism with dasatinib in vivo and currently is in phase I clinical trial for the blast crisis chronic myeloid leukemia (BC-CML).
Computational approaches in rational discovery of allosteric kinase inhibitors
Although targeting of highly conserved ATP-binding site by Type I and Type II inhibitors provides limited selectivity, inhibiting multiple kinases with a single small-molecule inhibitor was proven to be a useful strategy for therapeutic intervention. However, development of highly selective small-molecule kinase inhibitors remains a pressing concern where targeting of allosteric sites emerged as a promising approach (Wu et al., 2015a). Some of the advantages of targeting allosteric sites include increased selectivity and low toxicity of such inhibitors due to low evolutional conservation of allosteric sites compared to orthosteric (ATP-binding) sites (Fang et al., 2013). Additionally, overcoming of point mutation-associated drug resistance, especially for mutations in the ATP-binding site reported for almost all of ATP-competitive inhibitors, could be another advantage of developing allosteric kinase inhibitors (Gibbons et al., 2012).
While exploitation of allosteric sites represents a very promising strategy, it remains challenging from the aspect of rational drug discovery. Some of the major obstacles include identification of allosteric binding sites, which are usually hidden in less populated higher energy conformations of the proteins. Those conformations are poorly accessible to current experimental methods of structural biology (Lu et al., 2018a). Additionally, allosteric effectors are susceptible to “mode switching,” where minor chemical modification of ligand induces critical change in activity (Wenthur et al., 2014). Although known CADD workflows for discovery of drugs directed to orthosteric binding sites are being used in allosteric inhibitors discovery (Rastelli et al., 2014; Schoepfer et al., 2018), they provide limited utility rising the need for development of more spatialized tools and workflows (Greener and Sternberg, 2018).
Identification of allosteric pockets is a crucial first step in rational discovery of allosteric inhibitors. As will discussed below, a plethora of computationally inexpensive methodologies have been developed for this purpose and many of them are even implemented as web servers. While these methodologies provide fast and inexpensive highway in the discovery of druggable allosteric pockets, proper understanding of the allosteric mechanism is impossible without considering underlying conformational landscape and free-energy profiles where more computationally demanding molecular dynamics based approaches have a predominant role. In this review, we discuss few examples of computational methodologies used for direct discovery of novel allosteric sites and/or allosteric kinase inhibitors. For detailed description of recent breakthroughs in computational methodologies used for allosteric inhibitors discovery in general, the interested reader is referred to the recent reviews (Wagner et al., 2016; Lu et al., 2019).
Automatic Computational Tools/Web Servers to Investigate Allostery
Structure-based computational tools AlloSite and recently advanced descendant AllositePro (http://mdl.shsmu.edu.cn/AST/) are intended for fast detection of allosteric site in input PDB structures. Initial detection of allosteric sites is based on Fpocket, a fast open source protein pocket detection software package based on Voronoi tessellation (Le Guilloux et al., 2009). While Allosite uses a machine-learning model to re-rank detected pockets in terms of their allosteric character, AllositePro additionally implements normal-mode analysis (NMA) perturbation with elastic network models to account for protein flexibility. NMA is a technique developed for investigation of the vibrational motion of a harmonic oscillating system in the immediate vicinity of its equilibrium. Under assumption that the potential energy landscape in the vicinity of a minimized atomic structure is approximately harmonic, NMA eliminates the need to integrate the equations of motion and makes NMA much less computationally demanding compared to MD (Bahar and Rader, 2005). Zhang et al. demonstrated utility of AllositePro in identification of novel allosteric site on CDK2 kinase. Existence of novel site was validated in mutagenic analysis (Song et al., 2017). Recently, the same group developed AlloFinder, integrated allosterome mapping, and virtual screening workflow implemented as web server (http://mdl.shsmu.edu.cn/ALF/). AlloFinder relies on AllositePro algorithm for detection of allosteric sites, Allolike filter for pre-filtering of ligand library to enrich allosteric-like compounds (Wang et al., 2012), AutoDock Vina algorithm for docking (Trott and Olson, 2010), and Alloscore empirical scoring function for scoring allosteric modulator-protein complexes (Li et al., 2016). In the final step, alosterome mapping is used to detect highly similar allosteric sites among known human allosteric sites and to rule out selective ligands. This approach was retrospectively validated on several kinase targets (Huang et al., 2018).
CavityPlus (http://www.pkumdl.cn:8000/cavityplus/index.php) is another web server for detection of potential allosteric sites that works on similar principle (Xu et al., 2018). CavityPlus is aimed to detect potential binding sites on the surface of a given protein and rank them based on ligandability and druggability scores. This server integrates several functionalities: CAVITY for detection and scoring of potential binding sites (Yuan et al., 2013); CavPharmer for generation of receptor-based pharmacophores (Chen et al., 2014); CorrSite for prediction of allostery based on NMA motion correlation analysis between allosteric and orthosteric sites (Ma et al., 2016); CivCys for detection of binding sites for covalent inhibitors (Zhang et al., 2017). Functionalities of CavityPlus were successfully used for identification of allosteric binding site on Polo-like kinase 1 (Plk1). Subsequent molecular-docking-based virtual screening on allosteric site resulted in identification of few potent Plk1 inhibitors (Yun et al., 2016).
Another successful implementation of web server based tools for allosteric drug discovery is Kinase Atlas (https://kinase-atlas.bu.edu/) (Yueh et al., 2019). Kinase Atlas is systematic collection of mostly unexplored allosteric sites (binding hot spots) calculated for 4,910 PDB structures of 376 distinct kinases. The hot spots are identified by FTMap. This method places molecular probes (small organic molecules) on a dense grid around the protein and finds favorable positions using an empirical energy function and CHARMM potential. After clustering of obtained positions for each probe, regions that bind several probe clusters are marked as hot spots (Kozakov et al., 2015). Authors of the study identified novel allosteric site on CDK2 and screened library of 1,280 molecules using disulphide-based fragment screening. Two potent and novel allosteric inhibitors were described.
Molecular Dynamics-Based Approaches to Investigate Allostery
Molecular dynamics-based approaches in rational discovery of allosteric kinase inhibitors have potential to provide exclusive insight in atomic-level dynamical mechanism of allostery, to explore conformational landscape and capture kinase conformational states inaccessible to current experimental methodologies. Therefore, molecular-dynamics-based approaches, even though being computationally intensive, could detect previously unknown conformations and hidden allosteric binding pockets (Guo and Zhou, 2016; Lu et al., 2018a).
Combination of conventional MD simulations with other standard SBDD approaches resulted in identification of novel allosteric sites and discovery of novel allosteric ligands in several cases. For example, Perez et al. identified novel inhibitory allosteric site and inhibitors of p38α by using MD simulations starting from the X-ray structure of binary complex of p38α and its interacting partner MAPK-activated protein kinase 2 (MK2). MD simulations permitted definition of pharmacophoric features of small peptide inhibitors derived from sequence of MK2. Subsequent virtual screening study resulted in first small molecule allosteric inhibitor for identified binding site (Gomez-Gutierrez et al., 2016). Cournia et al. verified existence of allosteric site on human PI3Kα previously described in murine PI3Kα using combination of FTMap, MD, and in vitro assays. Intriguingly, MD simulations revealed different binding mode of studied allosteric inhibitor in murine, WT, and mutant forms of PI3Kα and consequent differences in propagation of allosteric signal to orthosteric ATP-binding site (Gkeka et al., 2015).
Computational costs of insufficient conformational sampling often limit application of conventional MD simulations in investigating allostery phenomena. Currently, there is a large gap between the time scale which can be reached in MD simulations and that observed in experiments. Several strategies for enhancing the sampling of MD simulations have been proposed (Aci-Seche et al., 2016; Yang et al., 2019). Two recently reported studies demonstrating full power of enhanced sampling methods (Markov-state modeling based adaptive sampling and parallel tempering in the well-tempered ensemble) are discussed below with special reference to atomic-level description of allosteric communication and discovery of cryptic allosteric pockets.
Pande et al. investigated activation pathway of c-Src kinase using massively distributed MD simulations (550 μs) on Folding@HOME (Shirts and Pande, 2000) Markov-state modeling (MSM) and adaptive sampling algorithms in order to provide description of factors underlying thermodynamics and kinetics of c-Src activation and to identify key structural intermediates (Shukla et al., 2014). Briefly, MSM models represent kinetical description of a system's underlying free-energy landscape, useful for characterization of probability of dynamical transitions between conformational states identified in many independent MD simulations and for extrapolation of long time system's behavior (Sengupta and Strodel, 2018). In this study intermediate conformational state which could be stabilized to block the c-Src activation pathway, was described through MSM analysis for the first time. Further analysis on identified c-Src conformational state revealed the existence of allosteric pocket and surprisingly high structural similarity to known complex of CDK2 bound to allosteric inhibitor—ANS (Betzi et al., 2011). Further simulations confirmed binding of ANS to the novel allosteric site of c-Src and blockage of activation process by stabilization of intermediate states. Additionally, the long-range residues coupling analysis identified myristate-binding pocket as another potential target for development of allosteric modulators of c-Src. Taken together, results of this study highlighted large-scale MD coupled with MSM modeling as an indispensable tool for identification of novel conformational states, potential allosteric pockets, and study of mechanisms of allostery in kinases.
In another example, authors explored the possibility of bidirectional communication between allosteric so-called PIF-pocket and ATP-binding site in PDK1 protein kinase using a combination of experimental techniques and enhanced-sampling simulations [parallel tempering simulations in the well-tempered ensemble (PT-WTE)] (Schulze et al., 2016). Results of PT-WTE MD revealed bidirectional mechanisms of communication between the ATP-binding site and allosteric site. Interestingly, this study for the first time demonstrated how different ligands which bind to the ATP-binding site differently modulate responses of allosteric site in interaction with a partner protein (e.g., enhance or inhibit interaction). Providing computer platform for rational design of allosteric modulators, the authors of this study opened an exciting avenue for future discovery of novel class of kinase inhibitors with less on-target side effects and more specific modulation of signaling pathways.
Case Study
Rational design of clinical candidate Asciminib—allosteric Bcr-Abl1 inhibitor
Asciminib belongs to a class of drugs designed to inhibit Bcr-Abl by binding to an allosteric pocket known as myristate-binding pocket. Rational development of Asciminib started with fragment-based screening using NMR assay (Schoepfer et al., 2018). Although determined NMR-based dissociation constants (Kd) for fragment hits were satisfactory, none of the fragments were active in biochemical and cellular assays. Subsequent X-ray studies revealed inability of fragment hits to induce assembled inactive state by bending of helix I, previously reported as conformational change important or autoinhibition of Abl by myristoilation (Nagar et al., 2003). Following this finding, the authors established another screening assay, the NMR-based conformational assay, which monitors the conformational state of C-terminal helix I (Jahnke et al., 2010). NMR-based conformational assay was used to investigate identified fragments and series of known allosteric modulators—derivatives of GNF-2 (Adrian et al., 2006; Figure 9). Results of the study revealed that compounds which bind to myristoyl pocket and do not induce helix I bending were actually functional activators of Abl1 (by interfering with autoinhibition mechanism of Abl1). Critical bending of helix I was found to be induced by the presence of CF3O– group from GNF-2. Based on these findings, CADD techniques (molecular docking, similarity and pharmacophore searches) were used to design compound X in respect to X-ray structure with bent helix I conformation. Subsequent introduction of CF3O– group finally led to the first active allosteric inhibitor. Molecular modeling techniques were used in combination with X-ray crystallography in order to optimize potency and drug-like properties of the compound. Although only standard CADD techniques were reported in the discovery of Asciminib, recent application of molecular dynamics-based approaches demonstrated utility of such techniques in examination of mechanisms of resistance and effects of dual targeting of ATP-binding and allosteric site providing rationale for development of novel drugs (El Rashedy et al., 2018; Meng et al., 2018; Zhan et al., 2019).
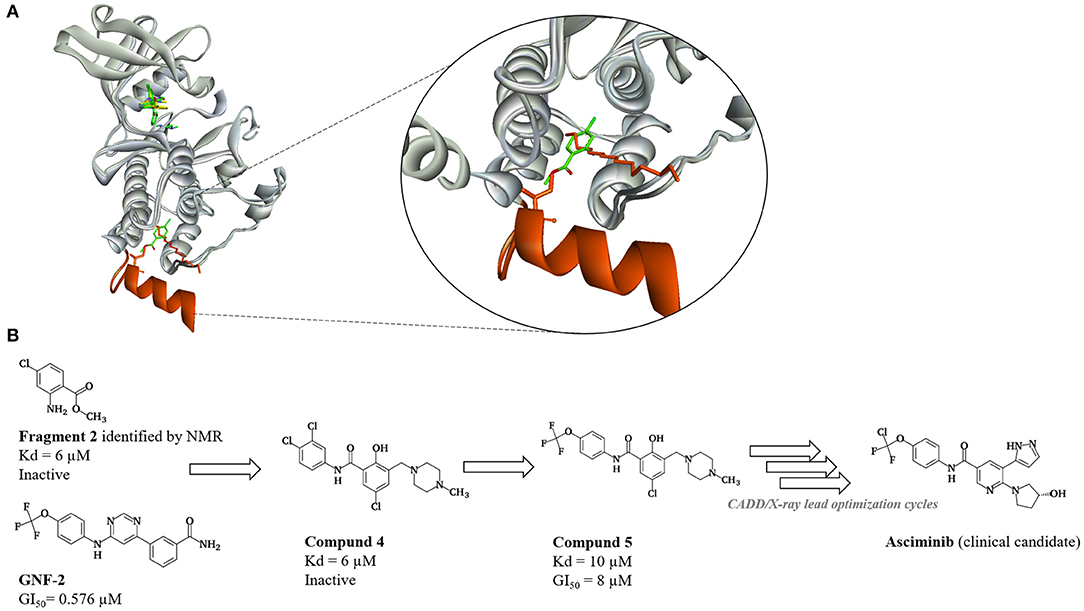
Figure 9. Discovery of Asciminib (Schoepfer et al., 2018). (A) Representation of initial hypothesis regarding bending of helix I (orange). Assembled inactive state of ABL1 kinase in complex with myristic acid (orange sticks) (PDB: 1OPL, SH3, and SH2 domains omitted for clarity) is superimposed on ABL1 in complex with fragment 2 (green sticks) (PDB: 3MS9). Steric clash between isoleucin I521 (orange sticks) on helix I and fragment 2 prevents full bending of helix I and formation of assembled inactive state of ABL1. Helix I is not visible in the PDB: 3MS9. (B) Medicinal chemistry progression from fragment 2 to fragment derived hit 4, first active hit–compound 5 and finally clinical candidate—Asciminib.
Machine learning methods to predict kinase-compound interactions
Nowadays, we are seeing the widespread use of machine learning in many areas, including pharmaceutical industry, especially in drug design. Popular computational methods initially used in pharmaceutical research were quantitative structure activity relationships (QSAR) and quantitative structure property relationship (QSPR), which were adequate for small datasets. However, with the rapid growth of databases (thanks to methods such as high-throughput in vitro screening and X-ray crystallography), it became inevitable to develop different in silico tools that can manage bigger data (Ekins, 2016). Today, many different machine learning methods such as support vector machines (SVM), k-Nearest Neighbors, Artificial Neural Networks (ANN), Deep Learning (DL), etc. are used in pharmaceutical research and they can be applied in various processes of drug design from virtual screening to de novo drug design (Buchwald et al., 2011; Drewry et al., 2017; Konze et al., 2019; Kuthuru et al., 2019; Lee et al., 2019; Zhavoronkov et al., 2019).
Many different machine learning models were created for the prediction of drug–target interactions (DTI), and many DTI methods have been applied to the protein kinases family (Kuthuru et al., 2019). Unlike LB and SB methods, DTI prediction uses the information from both protein and ligand and these methods can be similarity based or descriptor-based. One of the first similarity-based methods for identification of drug–target interactions was introduced by Yamanishi et al. in 2008. It used the known drug structure, protein sequence and drug–target interaction network to determine unknown ligand–target interactions. The main hypothesis is that two compounds that have high structure similarity might probably interact with similar target proteins, and vice versa two proteins with high sequence similarity might probably interact with similar drugs (Yamanishi et al., 2008). On the other hand, descriptor-based models use feature vectors from known drug structures and protein sequences, as inputs for machine learning methods, such SVM, AAN, DL, etc. In 2011, Buchwald et al. used SVM to prepare the model for prediction of protein kinases–ligand interactions. They used a set of binding data obtained from 113 different protein kinases and 20 inhibitors obtained through ATP site-dependent binding competition assays. They focused on vector features that describe the structure of molecules that are connected with certain chemical environment–protein active site sequence and created a SVM model with good predictivity (Buchwald et al., 2011).
Recently, the use of ANN, especially deep learning methods saw a significant increase in the process of drug design (Ekins, 2016; Merk et al., 2018; Putin et al., 2018; Konze et al., 2019). Deep generative models are utilizing neural networks to generate new objects (drugs) with desired properties (for example activity, Ki, IC50). These methods should be able to produce chemically correct structures without the need for including fragment libraries and/or rules for their combination (Merk et al., 2018). The ability to produce novel chemical structures with certain properties makes deep generative models suitable for the discovery of novel possible therapeutics (Zhavoronkov et al., 2019). In 2018, Merk et al. applied generative models to come up with novel bioactive, synthesizable drugs. They trained the model with more than 500,000 SMILES of bioactive compounds with their activity properties extracted from the ChEMBL (KD, Ki, IC/EC50 values <1 μM). Additionally, the model was fine-tuned to enable the de novo generation of target-specific ligands on retinoid X receptors (RXR) and/or peroxisome proliferator-activated receptors (PPAR). Finally, none of the generated compounds was identical to compounds from the training sets, and they were residing within the RXR/PPAR region of the fine-tuning set (Merk et al., 2018).
Case Studies
Predictive proteochemometric models for kinases derived from 3D protein field-based descriptors
Subramanian et al. described the development of proteochemometric models for 1,572 inhibitors and 95 kinases obtained from Kinase SARfari (https://chembl.gitbook.io/chembl-interface-documentation/legacy-resources#kinase-sarfari) and CHEMBL database, using 3D structure of proteins and active and inactive ligands. Proteins were described with molecular interaction fields derived from Schrödinger's WaterMaps, while different 1D, 2D, and 3D descriptors were used to describe the ligands. Separate training sets were created for the ligands and targets. Different methods were used for preparation of the proteochemometric models: support vector machines (SVM) and random forests (RF). The ligand prediction model was trained on the ligand training set and was used for ligand prediction model and target training set for target predicting model. In the end, they validated all the models using internal and external validation. This approach allows creation of not only predictive proteochemometrics model for protein kinases, but also preparation of visually interpretable models. This allows interpretation of kinase–ligand interactions, which can be used, for example, for optimization of ligand in order to achieve optimal activity and/or selectivity. Having visually interpretable models is the advantage compared to classical DTI methods that use only 2D information (Subramanian et al., 2013, 2016).
Deep learning model for identification of potent discoidin domain receptor 1 kinase inhibitors
Recently, Zhavoronkov et al. created a deep generative model for de novo small-molecule design—GENTRL (GENerative Tensorial Reinforcement Learning). Besides the effectiveness of a compound against a given biological target, GENTRL also takes into account its dissimilarity from other molecules in the literature and patent space, as well as its synthetic feasibility. For the proof-of-concept GENTRL was used to design potential Discoidin domain receptor 1 (DDR1) kinase inhibitors. Data was collected from different data sets: ZINC data set, known DDR1 kinase inhibitors data set, common kinase inhibitors, molecules with activity on non-kinase targets, patent data, and used to train the model. The model was generated by combining reinforcement learning with a reward, variational inference, and tensor decompositions. Finally the randomly elected six compounds that have not been previously published or patented were designed, synthesized, and experimentally tested. The whole process lasted only 46 days, which suggests that the application of drug design methods such as this will reduce the time and cost of drug discovery process (Zhavoronkov et al., 2019).
Concluding Remarks
In silico approaches are viable, usually cheaper and faster alternative to experimental drug discovery techniques. This review summarizes the most important computational tools that have led to the discovery of kinase inhibitors, many of which are in clinical use today as promising anticancer drugs. Computational approaches, such as QSAR modeling, ligand-based and structure-based virtual screening, molecular docking, molecular dynamics, quantum mechanics, fragment-based drug design, and machine learning methods, provide unique insight in the conformational landscape of kinases, structural requirements for inhibitory activity, binding modes and atomistic mechanisms of allostery, which represent indispensable information for rational de novo design. One of the main advantages of computational approaches is the possibility of introduction of new groups on the known scaffolds and in silico prediction of activities and binding affinities. Known scaffolds of the approved KIs include pyrimidine (imatinib, dasatinib, nilotinib), quinazoline (erlotinib, gefitinib, afatinib, vandetanib), pyridine (sorafenib), pirrolopyridine (vemurafenib), pyrazolopyridine (ibrutinib) etc. In silico modification of these scaffolds resulted in the design of many kinase inhibitors with enhanced predicted activities and binding affinities which can serve as lead compounds for further synthesis and preclinical testing. New chemical scaffolds that possess kinase inhibitory activity (imidazopyridazine, imidazopyridine, isoquinoline, phenazinamine, etc.) have also been proposed by computational approach and represent a good starting point for discovery of new kinase inhibitors. Due to increases in computational power, algorithmic improvements and increased accuracy, in silico approaches are yet expected to radically shape the era of kinase inhibitor discovery. Of note is to emphasize that not all drug discovery projects could be initiated and guided only with computational studies. The computational chemist must be aware of the structural biology of the studied targets, their dynamical changes influenced upon fragment/ligand binding. Whenever possible, it is advised to start CADD studies with experimental data and continue in silico optimization with combined modeling approaches, as much as possible. This review highlights the recent advances in discovery of kinase inhibitors by in silico approaches and can be useful for future design and synthesis of new kinase inhibitors as anticancer drugs.
Author Contributions
ZG wrote the introduction and LB methods. TD wrote the structure of protein kinases, machine learning methods, and SB methods. DR wrote FB methods. ND wrote modeling of allosteric kinase inhibitors. KN contributed to the conception of the manuscript, collection of the data, drafting and revising. All authors have contributed to the bibliographical research and interpretation of the work, to its critical revision, and approved the final version of the manuscript.
Conflict of Interest
The authors declare that the research was conducted in the absence of any commercial or financial relationships that could be construed as a potential conflict of interest.
Acknowledgments
The authors kindly acknowledge national project number 172033 supported by the Ministry of Education, Science and Technological development of the Republic of Serbia.
References
Aci-Seche, S., Ziada, S., Braka, A., Arora, R., and Bonnet, P. (2016). Advanced molecular dynamics simulation methods for kinase drug discovery. Future Med. Chem. 8, 545–566. doi: 10.4155/fmc.16.9
Adams, J. A. (2001). Kinetic and catalytic mechanisms of protein kinases. Chem. Rev. 101, 2271–2290. doi: 10.1021/cr000230w
Adrian, F. J., Ding, Q., Sim, T., Velentza, A., Sloan, C., Liu, Y., et al. (2006). Allosteric inhibitors of Bcr-abl-dependent cell proliferation. Nat. Chem. Biol. 2, 95–102. doi: 10.1038/nchembio760
Agafonov, R. V., Wilson, C., and Kern, D. (2015). Evolution and intelligent design in drug development. Front. Mol. Biosci. 2:27. doi: 10.3389/fmolb.2015.00027
Aira, L. E., Villa, E., Colosetti, P., Gamas, P., Signetti, L., Obba, S., et al. (2018). The oncogenic tyrosine kinase Lyn impairs the pro-apoptotic function of Bim. Oncogene 37, 2122–2136. doi: 10.1038/s41388-017-0112-0
Albert, L., Penalver, A., Djokovic, N., Werel, L., Hoffarth, M., Ruzic, D., et al. (2019). Modulating protein-protein interactions with visible-light-responsive peptide backbone photoswitches. Chembiochem 20, 1417–1429. doi: 10.1002/cbic.201800737
Alves Avelar, L. A., Ruzic, D., Djokovic, N., Kurz, T., and Nikolic, K. (2019). Structure-based design of selective histone deacetylase 6 zinc binding groups. J. Biomol. Struct. Dyn. 1–12. doi: 10.1080/07391102.2019.1652687. [Epub ahead of print].
Amr, A. E. E., Abo-Ghalia, M. H., Moustafa, G. O., Al-Omar, M. A., Nossier, E. S., and Elsayed, E. A. (2018). Design, synthesis and docking studies of novel macrocyclic pentapeptides as anticancer multi-targeted kinase inhibitors. Molecules 23:2416. doi: 10.3390/molecules23102416
Astanehe, A., Finkbeiner, M. R., Krzywinski, M., Fotovati, A., Dhillon, J., Berquin, I. M., et al. (2012). MKNK1 is a YB-1 target gene responsible for imparting trastuzumab resistance and can be blocked by RSK inhibition. Oncogene 31, 4434–4446. doi: 10.1038/onc.2011.617
Bacilieri, M., and Moro, S. (2006). Ligand-based drug design methodologies in drug discovery process: an overview. Curr. Drug Discov. Technol. 3, 155–165. doi: 10.2174/157016306780136781
Baell, J., and Walters, M. A. (2014). Chemistry: chemical con artists foil drug discovery. Nature 513, 481–483. doi: 10.1038/513481a
Bahar, I., and Rader, A. J. (2005). Coarse-grained normal mode analysis in structural biology. Curr. Opin. Struct. Biol. 15, 586–592. doi: 10.1016/j.sbi.2005.08.007
Bajorath, J. (2017). Molecular similarity concepts for informatics applications. Methods Mol. Biol. 1526, 231–245. doi: 10.1007/978-1-4939-6613-4_13
Bajusz, D., Racz, A., and Heberger, K. (2015). Why is Tanimoto index an appropriate choice for fingerprint-based similarity calculations? J. Cheminform. 7:20. doi: 10.1186/s13321-015-0069-3
Banegas-Luna, A. J., Ceron-Carrasco, J. P., and Perez-Sanchez, H. (2018). A review of ligand-based virtual screening web tools and screening algorithms in large molecular databases in the age of big data. Future Med. Chem. 10, 2641–2658. doi: 10.4155/fmc-2018-0076
Bautista-Aguilera, O. M., Esteban, G., Bolea, I., Nikolic, K., Agbaba, D., Moraleda, I., et al. (2014). Design, synthesis, pharmacological evaluation, QSAR analysis, molecular modeling and ADMET of novel donepezil-indolyl hybrids as multipotent cholinesterase/monoamine oxidase inhibitors for the potential treatment of Alzheimer's disease. Eur. J. Med. Chem. 75, 82–95. doi: 10.1016/j.ejmech.2013.12.028
Beauchamp, K. A., Lin, Y. S., Das, R., and Pande, V. S. (2012). Are protein force fields getting better? A systematic benchmark on 524 diverse NMR measurements. J. Chem. Theory Comput. 8, 1409–1414. doi: 10.1021/ct2007814
Benod, C., Carlsson, J., Uthayaruban, R., Hwang, P., Irwin, J. J., Doak, A. K., et al. (2013). Structure-based discovery of antagonists of nuclear receptor LRH-1. J. Biol. Chem. 288, 19830–19844. doi: 10.1074/jbc.M112.411686
Bethune, G., Bethune, D., Ridgway, N., and Xu, Z. (2010). Epidermal growth factor receptor (EGFR) in lung cancer: an overview and update. J. Thorac. Dis. 2, 48–51.
Betzi, S., Alam, R., Martin, M., Lubbers, D. J., Han, H., Jakkaraj, S. R., et al. (2011). Discovery of a potential allosteric ligand binding site in CDK2. ACS Chem. Biol. 6, 492–501. doi: 10.1021/cb100410m
Bhullar, K. S., Lagaron, N. O., McGowan, E. M., Parmar, I., Jha, A., Hubbard, B. P., et al. (2018). Kinase-targeted cancer therapies: progress, challenges and future directions. Mol. Cancer 17:48. doi: 10.1186/s12943-018-0804-2
Blume-Jensen, P., and Hunter, T. (2001). Oncogenic kinase signalling. Nature 411, 355–365. doi: 10.1038/35077225
Bollag, G., Tsai, J., Zhang, J., Zhang, C., Ibrahim, P., Nolop, K., et al. (2012). Vemurafenib: the first drug approved for BRAF-mutant cancer. Nat. Rev. Drug Discov. 11, 873–886. doi: 10.1038/nrd3847
Buchdunger, E., Cioffi, C. L., Law, N., Stover, D., Ohno-Jones, S., Druker, B. J., et al. (2000). Abl protein-tyrosine kinase inhibitor STI571 inhibits in vitro signal transduction mediated by c-kit and platelet-derived growth factor receptors. J. Pharmacol. Exp. Ther. 295, 139–145.
Buchwald, F., Richter, L., and Kramer, S. (2011). Predicting a small molecule-kinase interaction map: a machine learning approach. J. Cheminform. 3:22. doi: 10.1186/1758-2946-3-22
Burgess, M. R., Skaggs, B. J., Shah, N. P., Lee, F. Y., and Sawyers, C. L. (2005). Comparative analysis of two clinically active BCR-ABL kinase inhibitors reveals the role of conformation-specific binding in resistance. Proc. Natl. Acad. Sci. U.S.A. 102, 3395–3400. doi: 10.1073/pnas.0409770102
Caballero, J., and Alzate-Morales, J. H. (2012). Molecular dynamics of protein kinase-inhibitor complexes: a valid structural information. Curr. Pharm. Des. 18, 2946–2963. doi: 10.2174/138161212800672705
Carles, F., Bourg, S., Meyer, C., and Bonnet, P. (2018). PKIDB: a curated, annotated and updated database of protein kinase inhibitors in clinical trials. Molecules 23:908. doi: 10.3390/molecules23040908
Chakraborty, S., Lin, Y. H., Leng, X., Miranda, R. N., Medeiros, L. J., Shpall, E., et al. (2013). Activation of Jak2 in patients with blast crisis chronic myelogenous leukemia: inhibition of Jak2 inactivates Lyn kinase. Blood Cancer J. 3:e142. doi: 10.1038/bcj.2013.41
Chen, J., Ma, X., Yuan, Y., Pei, J., and Lai, L. (2014). Protein-protein interface analysis and hot spots identification for chemical ligand design. Curr. Pharm. Des. 20, 1192–1200. doi: 10.2174/13816128113199990065
Cherkasov, A., Muratov, E. N., Fourches, D., Varnek, A., Baskin, I. I., Cronin, M., et al. (2014). QSAR modeling: where have you been? Where are you going to? J. Med. Chem. 57, 4977–5010. doi: 10.1021/jm4004285
Christmann-Franck, S., van Westen, G. J., Papadatos, G., Beltran Escudie, F., Roberts, A., Overington, J. P., et al. (2016). Unprecedently large-scale kinase inhibitor set enabling the accurate prediction of compound-kinase activities: a way toward selective promiscuity by design? J. Chem. Inf. Model. 56, 1654–1675. doi: 10.1021/acs.jcim.6b00122
Cohen, P. (2002). Protein kinases — the major drug targets of the twenty-first century? Nat. Rev. Drug Discov. 1, 309–315. doi: 10.1038/nrd773
Collins, D. M., Conlon, N. T., Kannan, S., Verma, C. S., Eli, L. D., Lalani, A. S., et al. (2019). Preclinical characteristics of the irreversible pan-HER kinase inhibitor neratinib compared with lapatinib: implications for the treatment of HER2-positive and HER2-mutated breast cancer. Cancers 11:737. doi: 10.3390/cancers11060737
Congreve, M., Carr, R., Murray, C., and Jhoti, H. (2003). A ‘Rule of Three' for fragment-based lead discovery? Drug Discov. Today 8, 876–877. doi: 10.1016/S1359-6446(03)02831-9
Cornell, W. D., Cieplak, P., Bayly, C. I., Gould, I. R., Merz, K. M., Ferguson, D. M., et al. (1995). A second generation force field for the simulation of proteins, nucleic acids, and organic molecules. J. Am. Chem. Soc. 117, 5179–5197. doi: 10.1021/ja00124a002
Cramer, R. D., Patterson, D. E., and Bunce, J. D. (1988). Comparative molecular field analysis (CoMFA). 1. Effect of shape on binding of steroids to carrier proteins. J. Am. Chem. Soc. 110, 5959–5967. doi: 10.1021/ja00226a005
Dar, A. C., and Shokat, K. M. (2011). The evolution of protein kinase inhibitors from antagonists to agonists of cellular signaling. Annu. Rev. Biochem. 80, 769–795. doi: 10.1146/annurev-biochem-090308-173656
de Angelo, R. M., Almeida, M. O., de Paula, H., and Honorio, K. M. (2018). Studies on the dual activity of EGFR and HER-2 inhibitors using structure-based drug design techniques. Int. J. Mol. Sci. 19:3728. doi: 10.3390/ijms19123728
Debnath, S., Kanakaraju, M., Islam, M., Yeeravalli, R., Sen, D., and Das, A. (2019). In silico design, synthesis and activity of potential drug-like chrysin scaffold-derived selective EGFR inhibitors as anticancer agents. Comput. Biol. Chem. 83:107156. doi: 10.1016/j.compbiolchem.2019.107156
Deeks, E. D. (2016). Venetoclax: first global approval. Drugs 76, 979–987. doi: 10.1007/s40265-016-0596-x
Deng, W., Breneman, C., and Embrechts, M. J. (2004). Predicting protein-ligand binding affinities using novel geometrical descriptors and machine-learning methods. J. Chem. Inf. Comput. Sci. 44, 699–703. doi: 10.1021/ci034246+
Drewry, D. H., Wells, C. I., Andrews, D. M., Angell, R., Al-Ali, H., Axtman, A. D., et al. (2017). Progress towards a public chemogenomic set for protein kinases and a call for contributions. PLoS ONE 12:e0181585. doi: 10.1371/journal.pone.0181585
Dror, R. O., Dirks, R. M., Grossman, J. P., Xu, H., and Shaw, D. E. (2012). Biomolecular simulation: a computational microscope for molecular biology. Annu. Rev. Biophys. 41, 429–452. doi: 10.1146/annurev-biophys-042910-155245
Duong-Ly, K. C., and Peterson, J. R. (2013). The human kinome and kinase inhibition. Curr. Protoc. Pharmacol. 60, 2.9.1–2.9.14. doi: 10.1002/0471141755.ph0209s60
Duran, A., Zamora, I., and Pastor, M. (2009). Suitability of GRIND-based principal properties for the description of molecular similarity and ligand-based virtual screening. J. Chem. Inf. Model. 49, 2129–2138. doi: 10.1021/ci900228x
Ekins, S. (2016). The next era: deep learning in pharmaceutical research. Pharm. Res. 33, 2594–2603. doi: 10.1007/s11095-016-2029-7
El Rashedy, A. A., Olotu, F. A., and Soliman, M. E. S. (2018). Dual drug targeting of mutant Bcr-Abl induces inactive conformation: new strategy for the treatment of chronic myeloid leukemia and overcoming monotherapy resistance. Chem. Biodivers. 15:e1700533. doi: 10.1002/cbdv.201700533
Eldridge, M. D., Murray, C. W., Auton, T. R., Paolini, G. V., and Mee, R. P. (1997). Empirical scoring functions: I. The development of a fast empirical scoring function to estimate the binding affinity of ligands in receptor complexes. J. Comput. Aided Mol. Des. 11, 425–445. doi: 10.1023/A:1007996124545
Fang, Z., Grutter, C., and Rauh, D. (2013). Strategies for the selective regulation of kinases with allosteric modulators: exploiting exclusive structural features. ACS Chem. Biol. 8, 58–70. doi: 10.1021/cb300663j
FDA (2019). Novel Drug Approvals for 2019. Available online at: https://www.fda.gov/drugs/new-drugs-fda-cders-new-molecular-entities-and-new-therapeutic-biological-products/novel-drug-approvals-2019
Ferreira, L. G., Dos Santos, R. N., Oliva, G., and Andricopulo, A. D. (2015). Molecular docking and structure-based drug design strategies. Molecules 20, 13384–13421. doi: 10.3390/molecules200713384
Fischer, T., Najjar, A., Totzke, F., Schachtele, C., Sippl, W., Ritter, C., et al. (2018). Discovery of novel dual inhibitors of receptor tyrosine kinases EGFR and PDGFR-beta related to anticancer drug resistance. J. Enzyme Inhib. Med. Chem. 33, 1–8. doi: 10.1080/14756366.2017.1370583
Flaherty, K. T., Infante, J. R., Daud, A., Gonzalez, R., Kefford, R. F., Sosman, J., et al. (2012). Combined BRAF and MEK inhibition in melanoma with BRAF V600 mutations. N. Engl. J. Med. 367, 1694–1703. doi: 10.1056/NEJMoa1210093
Force, T., and Kolaja, K. L. (2011). Cardiotoxicity of kinase inhibitors: the prediction and translation of preclinical models to clinical outcomes. Nat. Rev. Drug Discov. 10, 111–126. doi: 10.1038/nrd3252
Gagic, Z., Ivkovic, B., Srdic-Rajic, T., Vucicevic, J., Nikolic, K., and Agbaba, D. (2016a). Synthesis of the vitamin E amino acid esters with an enhanced anticancer activity and in silico screening for new antineoplastic drugs. Eur. J. Pharm. Sci. 88, 59–69. doi: 10.1016/j.ejps.2016.04.008
Gagic, Z., Nikolic, K., Ivkovic, B., Filipic, S., and Agbaba, D. (2016b). QSAR studies and design of new analogs of vitamin E with enhanced antiproliferative activity on MCF-7 breast cancer cells. J. Taiwan Inst. Chem. Eng. 59, 33–44. doi: 10.1016/j.jtice.2015.07.019
Gao, Y., Wang, H., Wang, J., and Cheng, M. (2019). In silico studies on p21-activated kinase 4 inhibitors: comprehensive application of 3D-QSAR analysis, molecular docking, molecular dynamics simulations, and MM-GBSA calculation. J. Biomol. Struct. Dyn. 1–15. doi: 10.1080/07391102.2019.1673823. [Epub ahead of print].
Gibbons, D. L., Pricl, S., Kantarjian, H., Cortes, J., and Quintas-Cardama, A. (2012). The rise and fall of gatekeeper mutations? The BCR-ABL1 T315I paradigm. Cancer 118, 293–299. doi: 10.1002/cncr.26225
Giordanetto, F., Kull, B., and Dellsén, A. (2011). Discovery of novel class 1 phosphatidylinositide 3-kinases (PI3K) fragment inhibitors through structure-based virtual screening. Bioorg. Med. Chem. Lett. 21, 829–835. doi: 10.1016/j.bmcl.2010.11.087
Giordanetto, F., Wallberg, A., Cassel, J., Ghosal, S., Kossenjans, M., Yuan, Z. Q., et al. (2012). Discovery of 4-morpholino-pyrimidin-6-one and 4-morpholino-pyrimidin-2-one-containing Phosphoinositide 3-kinase (PI3K) p110beta isoform inhibitors through structure-based fragment optimisation. Bioorg. Med. Chem. Lett. 22, 6665–6670. doi: 10.1016/j.bmcl.2012.08.101
Gkeka, P., Papafotika, A., Christoforidis, S., and Cournia, Z. (2015). Exploring a non-ATP pocket for potential allosteric modulation of PI3Kalpha. J. Phys. Chem. B 119, 1002–1016. doi: 10.1021/jp506423e
Gomez-Gutierrez, P., Campos, P. M., Vega, M., and Perez, J. J. (2016). Identification of a Novel Inhibitory Allosteric Site in p38alpha. PLoS ONE 11:e0167379. doi: 10.1371/journal.pone.0167379
Gopalsamy, A., Ciszewski, G., Hu, Y., Lee, F., Feldberg, L., Frommer, E., et al. (2009). Identification of pyrazolo[1,5-a]pyrimidine-3-carboxylates as B-Raf kinase inhibitors. Bioorg. Med. Chem. Lett. 19, 2735–2738. doi: 10.1016/j.bmcl.2009.03.129
Graves, J. D., and Krebs, E. G. (1999). Protein phosphorylation and signal transduction. Pharmacol. Ther. 82, 111–121. doi: 10.1016/S0163-7258(98)00056-4
Greener, J. G., and Sternberg, M. J. (2018). Structure-based prediction of protein allostery. Curr. Opin. Struct. Biol. 50, 1–8. doi: 10.1016/j.sbi.2017.10.002
Guo, J., and Zhou, H. X. (2016). Protein allostery and conformational dynamics. Chem. Rev. 116, 6503–6515. doi: 10.1021/acs.chemrev.5b00590
Halder, A. K., Giri, A. K., and Cordeiro, M. (2019). Multi-target chemometric modelling, fragment analysis and virtual screening with ERK inhibitors as potential anticancer agents. Molecules 24:E3909. doi: 10.3390/molecules24213909
Halgren, T. A., Murphy, R. B., Friesner, R. A., Beard, H. S., Frye, L. L., Pollard, W. T., et al. (2004). Glide: a new approach for rapid, accurate docking and scoring. 2. Enrichment factors in database screening. J. Med. Chem. 47, 1750–1759. doi: 10.1021/jm030644s
Hann, M. M., Leach, A. R., and Harper, G. (2001). Molecular complexity and its impact on the probability of finding leads for drug discovery. J. Chem. Inf. Comput. Sci. 41, 856–864. doi: 10.1021/ci000403i
Hansch, C., Maloney, P. P., Fujita, T., and Muir, R. M. (1962). Correlation of biological activity of phenoxyacetic acids with Hammett substituent constants and partition coefficients. Nature 194, 178–180. doi: 10.1038/194178b0
Harris, P. A., Boloor, A., Cheung, M., Kumar, R., Crosby, R. M., Davis-Ward, R. G., et al. (2008). Discovery of 5-[[4-[(2,3-dimethyl-2H-indazol-6-yl)methylamino]-2-pyrimidinyl]amino]-2-methyl-b enzenesulfonamide (Pazopanib), a novel and potent vascular endothelial growth factor receptor inhibitor. J. Med. Chem. 51, 4632–4640. doi: 10.1021/jm800566m
Harris, P. A., Cheung, M., Hunter, R. N. III., Brown, M. L., Veal, J. M., Nolte, R. T., et al. (2005). Discovery and evaluation of 2-anilino-5-aryloxazoles as a novel class of VEGFR2 kinase inhibitors. J. Med. Chem. 48, 1610–1619. doi: 10.1021/jm049538w
Hasinoff, B. B. (2010). The cardiotoxicity and myocyte damage caused by small molecule anticancer tyrosine kinase inhibitors is correlated with lack of target specificity. Toxicol. Appl. Pharmacol. 244, 190–195. doi: 10.1016/j.taap.2009.12.032
Hazhazi, H., Melkemi, N., Salah, T., and Bouachrine, M. (2019). DFT-based reactivity and combined QSAR, molecular docking of 1,2,4,5-Tetrazine derivatives as inhibitors of Pim-1 kinase. Heliyon 5:e02451. doi: 10.1016/j.heliyon.2019.e02451
Holohan, C., Van Schaeybroeck, S., Longley, D. B., and Johnston, P. G. (2013). Cancer drug resistance: an evolving paradigm. Nat. Rev. Cancer 13, 714–726. doi: 10.1038/nrc3599
Hu, X., Wang, D., Tong, Y., Tong, L., Wang, X., Zhu, L., et al. (2017). Design, synthesis, and evaluation of ribose-modified anilinopyrimidine derivatives as EGFR tyrosine kinase inhibitors. Front. Chem. 5:101. doi: 10.3389/fchem.2017.00101
Huang, M., Song, K., Liu, X., Lu, S., Shen, Q., Wang, R., et al. (2018). AlloFinder: a strategy for allosteric modulator discovery and allosterome analyses. Nucleic Acids Res. 46, W451–W458. doi: 10.1093/nar/gky374
Huang, N., Kalyanaraman, C., Irwin, J. J., and Jacobson, M. P. (2006). Physics-based scoring of protein-ligand complexes: enrichment of known inhibitors in large-scale virtual screening. J. Chem. Inf. Model. 46, 243–253. doi: 10.1021/ci0502855
Hubbard, S. R., Wei, L., Ellis, L., and Hendrickson, W. A. (1994). Crystal structure of the tyrosine kinase domain of the human insulin receptor. Nature 372, 746–754. doi: 10.1038/372746a0
Hussain, A., and Verma, C. K. (2019). Ligand- and structure-based pharmacophore modeling, docking study reveals 2-[[4-[6-(isopropylamino) pyrimidin-4-yl]-1H-pyrrolo[2,3-b] pyridin-6-yl] amino] ethanol as a potential anticancer agent of CDK9/cyclin T1 kinase. J. Cancer Res. Ther. 15, 1131–1140. doi: 10.4103/jcrt.JCRT_47_18
Iqbal, N., and Iqbal, N. (2014). Imatinib: a breakthrough of targeted therapy in cancer. Chemother. Res. Pract. 2014:357027. doi: 10.1155/2014/357027
Irwin, J. J., Sterling, T., Mysinger, M. M., Bolstad, E. S., and Coleman, R. G. (2012). ZINC: a free tool to discover chemistry for biology. J. Chem. Inf. Model. 52, 1757–1768. doi: 10.1021/ci3001277
Jahnke, W., Grotzfeld, R. M., Pelle, X., Strauss, A., Fendrich, G., Cowan-Jacob, S. W., et al. (2010). Binding or bending: distinction of allosteric Abl kinase agonists from antagonists by an NMR-based conformational assay. J. Am. Chem. Soc. 132, 7043–7048. doi: 10.1021/ja101837n
James, N., Shanthi, V., and Ramanathan, K. (2019). Density functional theory and molecular simulation studies for prioritizing anaplastic lymphoma kinase inhibitors. Appl. Biochem. Biotechnol. doi: 10.1007/s12010-019-03156-1. [Epub ahead of print].
Jones, G., Willett, P., Glen, R. C., Leach, A. R., and Taylor, R. (1997). Development and validation of a genetic algorithm for flexible docking. J. Mol. Biol. 267, 727–748. doi: 10.1006/jmbi.1996.0897
Jorgensen, W. L., Maxwell, D. S., and Tirado-Rives, J. (1996). Development and testing of the OPLS all-atom force field on conformational energetics and properties of organic liquids. J. Am. Chem. Soc. 118, 11225–11236. doi: 10.1021/ja9621760
Kaieda, A., Takahashi, M., Fukuda, H., Okamoto, R., Morimoto, S., Gotoh, M., et al. (2019). Structure-based design, synthesis, and biological evaluation of imidazo[4,5-b]pyridin-2-one-based p38 MAP kinase inhibitors: part 1. ChemMedChem 14, 1022–1030. doi: 10.1002/cmdc.201900129
Kale, M. A., and Sonwane, G. M. (2018). Molecular docking, G-QSAR studies, synthesis and anticancer screening of some new 2-phenazinamines as Bcr-Abl tyrosine kinase inhibitors. Curr. Drug Discov. Technol. doi: 10.2174/1570163815666180913122542. [Epub ahead of print].
Kannan, S., Poulsen, A., Yang, H. Y., Ho, M., Ang, S. H., Eldwin, T. S. W., et al. (2017). Correction to probing the binding mechanism of Mnk inhibitors by docking and molecular dynamics simulations. Biochemistry 56, 5964–5965. doi: 10.1021/acs.biochem.7b00996
Khodair, A. I., Alsafi, M. A., and Nafie, M. S. (2019). Synthesis, molecular modeling and anti-cancer evaluation of a series of quinazoline derivatives. Carbohydr. Res. 486:107832. doi: 10.1016/j.carres.2019.107832
Kim, S., Chen, J., Cheng, T., Gindulyte, A., He, J., He, S., et al. (2019). PubChem 2019 update: improved access to chemical data. Nucleic Acids Res. 47, D1102–D1109. doi: 10.1093/nar/gky1033
Kitchen, D. B., Decornez, H., Furr, J. R., and Bajorath, J. (2004). Docking and scoring in virtual screening for drug discovery: methods and applications. Nat. Rev. Drug Discov. 3, 935–949. doi: 10.1038/nrd1549
Klaeger, S., Heinzlmeir, S., Wilhelm, M., Polzer, H., Vick, B., Koenig, P. A., et al. (2017). The target landscape of clinical kinase drugs. Science 358:eaan4368. doi: 10.1126/science.aan4368
Klebe, G., Abraham, U., and Mietzner, T. (1994). Molecular similarity indices in a comparative analysis (CoMSIA) of drug molecules to correlate and predict their biological activity. J. Med. Chem. 37, 4130–4146. doi: 10.1021/jm00050a010
Klepeis, J. L., Lindorff-Larsen, K., Dror, R. O., and Shaw, D. E. (2009). Long-timescale molecular dynamics simulations of protein structure and function. Curr. Opin. Struct. Biol. 19, 120–127. doi: 10.1016/j.sbi.2009.03.004
Knight, J. D., Qian, B., Baker, D., and Kothary, R. (2007). Conservation, variability and the modeling of active protein kinases. PLoS ONE 2:e982. doi: 10.1371/journal.pone.0000982
Knighton, D. R., Zheng, J. H., Ten Eyck, L. F., Ashford, V. A., Xuong, N. H., Taylor, S. S., et al. (1991). Crystal structure of the catalytic subunit of cyclic adenosine monophosphate-dependent protein kinase. Science 253, 407–414. doi: 10.1126/science.1862342
Koneru, J. K., Sinha, S., and Mondal, J. (2019). In Silico reoptimization of binding affinity and drug-resistance circumvention ability in kinase inhibitors: a case study with RL-45 and Src kinase. J. Phys. Chem. B 123, 6664–6672. doi: 10.1021/acs.jpcb.9b02883
Konze, K. D., Bos, P. H., Dahlgren, M. K., Leswing, K., Tubert-Brohman, I., Bortolato, A., et al. (2019). Reaction-based enumeration, active learning, and free energy calculations to rapidly explore synthetically tractable chemical space and optimize potency of cyclin-dependent kinase 2 inhibitors. J. Chem. Inf. Model. 59, 3782–3793. doi: 10.1021/acs.jcim.9b00367
Kozakov, D., Grove, L. E., Hall, D. R., Bohnuud, T., Mottarella, S. E., Luo, L., et al. (2015). The FTMap family of web servers for determining and characterizing ligand-binding hot spots of proteins. Nat. Protoc. 10, 733–755. doi: 10.1038/nprot.2015.043
Kumar, V., Krishna, S., and Siddiqi, M. I. (2015). Virtual screening strategies: recent advances in the identification and design of anti-cancer agents. Methods 71, 64–70. doi: 10.1016/j.ymeth.2014.08.010
Kuntz, I. D., Blaney, J. M., Oatley, S. J., Langridge, R., and Ferrin, T. E. (1982). A geometric approach to macromolecule-ligand interactions. J. Mol. Biol. 161, 269–288. doi: 10.1016/0022-2836(82)90153-X
Kuthuru, S., Szafran, A. T., Stossi, F., Mancini, M. A., and Rao, A. (2019). Leveraging image-derived phenotypic measurements for drug-target interaction predictions. Cancer Inform. 18:1176935119856595. doi: 10.1177/1176935119856595
La Sala, G., Riccardi, L., Gaspari, R., Cavalli, A., Hantschel, O., and De Vivo, M. (2016). HRD motif as the central hub of the signaling network for activation loop autophosphorylation in Abl kinase. J. Chem. Theory Comput. 12, 5563–5574. doi: 10.1021/acs.jctc.6b00600
Lamba, V., and Ghosh, I. (2012). New directions in targeting protein kinases: focusing upon true allosteric and bivalent inhibitors. Curr. Pharm. Des. 18, 2936–2945. doi: 10.2174/138161212800672813
Larif, S., Salem, C. B., Hmouda, H., and Bouraoui, K. (2014). In silico screening and study of novel ERK2 inhibitors using 3D QSAR, docking and molecular dynamics. J. Mol. Graph. Model. 53, 1–12. doi: 10.1016/j.jmgm.2014.07.001
Laurini, E., Posocco, P., Fermeglia, M., Gibbons, D. L., Quintas-Cardama, A., and Pricl, S. (2013). Through the open door: preferential binding of dasatinib to the active form of BCR-ABL unveiled by in silico experiments. Mol. Oncol. 7, 968–975. doi: 10.1016/j.molonc.2013.06.001
Le Guilloux, V., Schmidtke, P., and Tuffery, P. (2009). Fpocket: an open source platform for ligand pocket detection. BMC Bioinformatics 10:168. doi: 10.1186/1471-2105-10-168
Leach, A. R., and Hann, M. M. (2011). Molecular complexity and fragment-based drug discovery: ten years on. Curr. Opin. Chem. Biol. 15, 489–496. doi: 10.1016/j.cbpa.2011.05.008
Lee, H. W., Choi, H. Y., Joo, K. M., and Nam, D. H. (2015). Tumor progression locus 2 (Tpl2) kinase as a novel therapeutic target for cancer: double-sided effects of Tpl2 on cancer. Int. J. Mol. Sci. 16, 4471–4491. doi: 10.3390/ijms16034471
Lee, I., Keum, J., and Nam, H. (2019). DeepConv-DTI: prediction of drug-target interactions via deep learning with convolution on protein sequences. PLoS Comput. Biol. 15:e1007129. doi: 10.1371/journal.pcbi.1007129
Lengauer, T., and Rarey, M. (1996). Computational methods for biomolecular docking. Curr. Opin. Struct. Biol. 6, 402–406. doi: 10.1016/S0959-440X(96)80061-3
Li, J., Fu, A., and Zhang, L. (2019). An overview of scoring functions used for protein-ligand interactions in molecular docking. Interdiscip. Sci. 11, 320–328. doi: 10.1007/s12539-019-00327-w
Li, S., Shen, Q., Su, M., Liu, X., Lu, S., Chen, Z., et al. (2016). Alloscore: a method for predicting allosteric ligand-protein interactions. Bioinformatics 32, 1574–1576. doi: 10.1093/bioinformatics/btw036
Lim, H., He, D., Qiu, Y., Krawczuk, P., Sun, X., and Xie, L. (2019). Rational discovery of dual-indication multi-target PDE/Kinase inhibitor for precision anti-cancer therapy using structural systems pharmacology. PLoS Comput. Biol. 15:e1006619. doi: 10.1371/journal.pcbi.1006619
Lionta, E., Spyrou, G., Vassilatis, D. K., and Cournia, Z. (2014). Structure-based virtual screening for drug discovery: principles, applications and recent advances. Curr. Top. Med. Chem. 14, 1923–1938. doi: 10.2174/1568026614666140929124445
Liu, J., and Wang, R. (2015). Classification of current scoring functions. J. Chem. Inf. Model. 55, 475–482. doi: 10.1021/ci500731a
Liu, J., Wen, Y., Gao, L., Gao, L., He, F., Zhou, J., et al. (2020). Design, synthesis and biological evaluation of novel 1H-1,2,4-triazole, benzothiazole and indazole-based derivatives as potent FGFR1 inhibitors viafragment-based virtual screening. J. Enzyme Inhib. Med. Chem. 35, 72–84. doi: 10.1080/14756366.2019.1673745
Lu, S., He, X., Ni, D., and Zhang, J. (2019). Allosteric modulator discovery: from serendipity to structure-based design. J. Med. Chem. 62, 6405–6421. doi: 10.1021/acs.jmedchem.8b01749
Lu, S., Ji, M., Ni, D., and Zhang, J. (2018a). Discovery of hidden allosteric sites as novel targets for allosteric drug design. Drug Discov. Today 23, 359–365. doi: 10.1016/j.drudis.2017.10.001
Lu, W., Zhang, R., Jiang, H., Zhang, H., and Luo, C. (2018b). Computer-aided drug design in epigenetics. Front. Chem. 6:57. doi: 10.3389/fchem.2018.00057
Ma, X., Meng, H., and Lai, L. (2016). Motions of allosteric and orthosteric ligand-binding sites in proteins are highly correlated. J. Chem. Inf. Model. 56, 1725–1733. doi: 10.1021/acs.jcim.6b00039
Machrouhi, F., Ouhamou, N., Laderoute, K., Calaoagan, J., Bukhtiyarova, M., Ehrlich, P. J., et al. (2010). The rational design of a novel potent analogue of the 5'-AMP-activated protein kinase inhibitor compound C with improved selectivity and cellular activity. Bioorg. Med. Chem. Lett. 20, 6394–6399. doi: 10.1016/j.bmcl.2010.09.088
Manning, G., Whyte, D. B., Martinez, R., Hunter, T., and Sudarsanam, S. (2002). The protein kinase complement of the human genome. Science 298, 1912–1934. doi: 10.1126/science.1075762
Markham, A. (2019). Erdafitinib: first global approval. Drugs 79, 1017–1021. doi: 10.1007/s40265-019-01142-9
Martin, M. P., Alam, R., Betzi, S., Ingles, D. J., Zhu, J. Y., and Schonbrunn, E. (2012). A novel approach to the discovery of small-molecule ligands of CDK2. Chembiochem 13, 2128–2136. doi: 10.1002/cbic.201200316
Melge, A. R., Kumar, L. G., Pavithran, K., Nair, S. V., Manzoor, K., and Gopi Mohan, C. (2019). Predictive models for designing potent tyrosine kinase inhibitors in chronic myeloid leukemia for understanding its molecular mechanism of resistance by molecular docking and dynamics simulations. J. Biomol. Struct. Dyn. 37, 4747–4766. doi: 10.1080/07391102.2018.1559765
Mendez, D., Gaulton, A., Bento, A. P., Chambers, J., De Veij, M., Felix, E., et al. (2019). ChEMBL: towards direct deposition of bioassay data. Nucleic Acids Res. 47, D930–D940. doi: 10.1093/nar/gky1075
Meng, Y., Gao, C., Clawson, D. K., Atwell, S., Russell, M., Vieth, M., et al. (2018). Predicting the conformational variability of Abl tyrosine kinase using molecular dynamics simulations and markov state models. J. Chem. Theory Comput. 14, 2721–2732. doi: 10.1021/acs.jctc.7b01170
Merget, B., Turk, S., Eid, S., Rippmann, F., and Fulle, S. (2017). Profiling prediction of kinase inhibitors: toward the virtual assay. J. Med. Chem. 60, 474–485. doi: 10.1021/acs.jmedchem.6b01611
Merk, D., Friedrich, L., Grisoni, F., and Schneider, G. (2018). De novo design of bioactive small molecules by artificial intelligence. Mol. Inform. 37. doi: 10.1002/minf.201700153
Mobitz, H. (2015). The ABC of protein kinase conformations. Biochim. Biophys. Acta 1854(10 Pt B), 1555–1566. doi: 10.1016/j.bbapap.2015.03.009
Modi, V., and Dunbrack, R. L. Jr. (2019). Defining a new nomenclature for the structures of active and inactive kinases. Proc. Natl. Acad. Sci. U.S.A. 116, 6818–6827. doi: 10.1073/pnas.1814279116
Mohamed, T. K., Batran, R. Z., Elseginy, S. A., Ali, M. M., and Mahmoud, A. E. (2019). Synthesis, anticancer effect and molecular modeling of new thiazolylpyrazolyl coumarin derivatives targeting VEGFR-2 kinase and inducing cell cycle arrest and apoptosis. Bioorg. Chem. 85, 253–273. doi: 10.1016/j.bioorg.2018.12.040
Mohareb, R. M., Samir, E. M., and Halim, P. A. (2019). Synthesis, and anti-proliferative, Pim-1 kinase inhibitors and molecular docking of thiophenes derived from estrone. Bioorg. Chem. 83, 402–413. doi: 10.1016/j.bioorg.2018.10.067
Morabito, A., De Maio, E., Di Maio, M., Normanno, N., and Perrone, F. (2006). Tyrosine kinase inhibitors of vascular endothelial growth factor receptors in clinical trials: current status and future directions. Oncologist 11, 753–764. doi: 10.1634/theoncologist.11-7-753
Morris, G. M., Huey, R., Lindstrom, W., Sanner, M. F., Belew, R. K., Goodsell, D. S., et al. (2009). AutoDock4 and AutoDockTools4: automated docking with selective receptor flexibility. J. Comput. Chem. 30, 2785–2791. doi: 10.1002/jcc.21256
Morris, G. M., and Lim-Wilby, M. (2008). Molecular docking. Methods Mol. Biol. 443, 365–382. doi: 10.1007/978-1-59745-177-2_19
Muegge, I., and Martin, Y. C. (1999). A general and fast scoring function for protein-ligand interactions: a simplified potential approach. J. Med. Chem. 42, 791–804. doi: 10.1021/jm980536j
Murray, C. W., and Verdonk, M. L. (2002). The consequences of translational and rotational entropy lost by small molecules on binding to proteins. J. Comput. Aided Mol. Des. 16, 741–753. doi: 10.1023/A:1022446720849
Naboulsi, I., Aboulmouhajir, A., Kouisni, L., Bekkaoui, F., and Yasri, A. (2018). Combining a QSAR approach and structural analysis to derive an SAR map of Lyn kinase inhibition. Molecules 23:3271. doi: 10.3390/molecules23123271
Nagar, B., Hantschel, O., Young, M. A., Scheffzek, K., Veach, D., Bornmann, W., et al. (2003). Structural basis for the autoinhibition of c-Abl tyrosine kinase. Cell 112, 859–871. doi: 10.1016/S0092-8674(03)00194-6
Najjar, A., Platzer, C., Luft, A., Assmann, C. A., Elghazawy, N. H., Erdmann, F., et al. (2019). Computer-aided design, synthesis and biological characterization of novel inhibitors for PKMYT1. Eur. J. Med. Chem. 161, 479–492. doi: 10.1016/j.ejmech.2018.10.050
Neves, B. J., Braga, R. C., Melo-Filho, C. C., Moreira-Filho, J. T., Muratov, E. N., and Andrade, C. H. (2018). QSAR-based virtual screening: advances and applications in drug discovery. Front. Pharmacol. 9:1275. doi: 10.3389/fphar.2018.01275
Nikolic, K., Agbaba, D., and Stark, H. (2015). Pharmacophore modeling, drug design and virtual screening on multi-targeting procognitive agents approaching histaminergic pathways. J. Taiwan Inst. Chem. Eng. 46, 15–29. doi: 10.1016/j.jtice.2014.09.017
Nikolic, K., Veljkovic, N., Gemovic, B., Srdic-Rajic, T., and Agbaba, D. (2013). Imidazoline-1 receptor ligands as apoptotic agents: pharmacophore modeling and virtual docking study. Comb. Chem. High Throughput Screen 16, 298–319. doi: 10.2174/1386207311316040004
Noolvi, M. N., and Patel, H. M. (2013). A comparative QSAR analysis and molecular docking studies of quinazoline derivatives as tyrosine kinase (EGFR) inhibitors: a rational approach to anticancer drug design. J. Saudi Chem. Soc. 17, 361–379. doi: 10.1016/j.jscs.2011.04.017
Novič, M., Tibaut, T., Anderluh, M., Borišek, J., and Tomašič, T. (2016). “The comparison of docking search algorithms and scoring functions,” in Methods and Algorithms for Molecular Docking-Based Drug Design and Discovery, ed S. Dastmalchi, M. Hamzeh-Mivehroud, B. Sokouti. (Hershey, PA: IGI Global, 99–127.
Ohren, J. F., Chen, H., Pavlovsky, A., Whitehead, C., Zhang, E., Kuffa, P., et al. (2004). Structures of human MAP kinase kinase 1 (MEK1) and MEK2 describe novel noncompetitive kinase inhibition. Nat. Struct. Mol. Biol. 11, 1192–1197. doi: 10.1038/nsmb859
Okabe, S., Tauchi, T., Tanaka, Y., and Ohyashiki, K. (2011). Dasatinib preferentially induces apoptosis by inhibiting Lyn kinase in nilotinib-resistant chronic myeloid leukemia cell line. J. Hematol. Oncol. 4:32. doi: 10.1186/1756-8722-4-32
Oluic, J., Nikolic, K., Vucicevic, J., Gagic, Z., Filipic, S., and Agbaba, D. (2017). 3D-QSAR, virtual screening, docking and design of dual PI3K/mTOR inhibitors with enhanced antiproliferative activity. Comb. Chem. High Throughput Screen 20, 292–303. doi: 10.2174/1386207320666170427143858
Oostenbrink, C., Villa, A., Mark, A. E., and van Gunsteren, W. F. (2004). A biomolecular force field based on the free enthalpy of hydration and solvation: the GROMOS force-field parameter sets 53A5 and 53A6. J. Comput. Chem. 25, 1656–1676. doi: 10.1002/jcc.20090
Oyarzabal, J., Zarich, N., Albarran, M. I., Palacios, I., Urbano-Cuadrado, M., Mateos, G., et al. (2010). Discovery of mitogen-activated protein kinase-interacting kinase 1 inhibitors by a comprehensive fragment-oriented virtual screening approach. J. Med. Chem. 53, 6618–6628. doi: 10.1021/jm1005513
Park, E., Kim, N., Ficarro, S. B., Zhang, Y., Lee, B. I., Cho, A., et al. (2015). Structure and mechanism of activity-based inhibition of the EGF receptor by Mig6. Nat. Struct. Mol. Biol. 22, 703–711. doi: 10.1038/nsmb.3074
Pastor, M., Cruciani, G., McLay, I., Pickett, S., and Clementi, S. (2000). GRid-INdependent descriptors (GRIND): a novel class of alignment-independent three-dimensional molecular descriptors. J. Med. Chem. 43, 3233–3243. doi: 10.1021/jm000941m
Piana, S., Klepeis, J. L., and Shaw, D. E. (2014). Assessing the accuracy of physical models used in protein-folding simulations: quantitative evidence from long molecular dynamics simulations. Curr. Opin. Struct. Biol. 24, 98–105. doi: 10.1016/j.sbi.2013.12.006
Platzer, C., Najjar, A., Rohe, A., Erdmann, F., Sippl, W., and Schmidt, M. (2018). Identification of PKMYT1 inhibitors by screening the GSK published protein kinase inhibitor set I and II. Bioorg. Med. Chem. 26, 4014–4024. doi: 10.1016/j.bmc.2018.06.027
Proud, C. G. (2015). Mnks, eIF4E phosphorylation and cancer. Biochim. Biophys. Acta 1849, 766–773. doi: 10.1016/j.bbagrm.2014.10.003
Putin, E., Asadulaev, A., Ivanenkov, Y., Aladinskiy, V., Sanchez-Lengeling, B., Aspuru-Guzik, A., et al. (2018). Reinforced adversarial neural computer for de novo molecular design. J. Chem. Inf. Model. 58, 1194–1204. doi: 10.1021/acs.jcim.7b00690
Rastelli, G., Anighoro, A., Chripkova, M., Carrassa, L., and Broggini, M. (2014). Structure-based discovery of the first allosteric inhibitors of cyclin-dependent kinase 2. Cell Cycle 13, 2296–2305. doi: 10.4161/cc.29295
Raval, A., Piana, S., Eastwood, M. P., Dror, R. O., and Shaw, D. E. (2012). Refinement of protein structure homology models via long, all-atom molecular dynamics simulations. Proteins 80, 2071–2079. doi: 10.1002/prot.24098
Ren, J. X., Li, L. L., Zheng, R. L., Xie, H. Z., Cao, Z. X., Feng, S., et al. (2011). Discovery of novel Pim-1 kinase inhibitors by a hierarchical multistage virtual screening approach based on SVM model, pharmacophore, and molecular docking. J. Chem. Inf. Model. 51, 1364–1375. doi: 10.1021/ci100464b
Rettenmaier, T. J., Fan, H., Karpiak, J., Doak, A., Sali, A., Shoichet, B. K., et al. (2015). Small-molecule allosteric modulators of the protein kinase PDK1 from structure-based docking. J. Med. Chem. 58, 8285–8291. doi: 10.1021/acs.jmedchem.5b01216
Roskoski, R. Jr. (2015). A historical overview of protein kinases and their targeted small molecule inhibitors. Pharmacol. Res. 100, 1–23. doi: 10.1016/j.phrs.2015.07.010
Roskoski, R. Jr. (2016). Classification of small molecule protein kinase inhibitors based upon the structures of their drug-enzyme complexes. Pharmacol. Res. 103, 26–48. doi: 10.1016/j.phrs.2015.10.021
Roskoski, R. Jr. (2019a). Cyclin-dependent protein serine/threonine kinase inhibitors as anticancer drugs. Pharmacol. Res. 139, 471–488. doi: 10.1016/j.phrs.2018.11.035
Roskoski, R. Jr. (2019b). Properties of FDA-approved small molecule protein kinase inhibitors. Pharmacol. Res. 144, 19–50. doi: 10.1016/j.phrs.2019.03.006
Ruzic, D., Petkovic, M., Agbaba, D., Ganesan, A., and Nikolic, K. (2019). Combined ligand and fragment-based drug design of selective histone deacetylase - 6 inhibitors. Mol. Inform. 38:e1800083. doi: 10.1002/minf.201800083
Schoepfer, J., Jahnke, W., Berellini, G., Buonamici, S., Cotesta, S., Cowan-Jacob, S. W., et al. (2018). Discovery of Asciminib (ABL001), an allosteric inhibitor of the tyrosine kinase activity of BCR-ABL1. J. Med. Chem. 61, 8120–8135. doi: 10.1021/acs.jmedchem.8b01040
Schulze, J. O., Saladino, G., Busschots, K., Neimanis, S., Suss, E., Odadzic, D., et al. (2016). Bidirectional allosteric communication between the ATP-binding site and the regulatory PIF pocket in PDK1 protein kinase. Cell Chem. Biol. 23, 1193–1205. doi: 10.1016/j.chembiol.2016.06.017
Seiler, K. P., George, G. A., Happ, M. P., Bodycombe, N. E., Carrinski, H. A., Norton, S., et al. (2008). ChemBank: a small-molecule screening and cheminformatics resource database. Nucleic Acids Res. 36, D351–D359. doi: 10.1093/nar/gkm843
Sengupta, U., and Strodel, B. (2018). Markov models for the elucidation of allosteric regulation. Philos. Trans. R. Soc. Lond. B. Biol. Sci. 373:20170178. doi: 10.1098/rstb.2017.0178
Shah, N. P., Nicoll, J. M., Nagar, B., Gorre, M. E., Paquette, R. L., Kuriyan, J., et al. (2002). Multiple BCR-ABL kinase domain mutations confer polyclonal resistance to the tyrosine kinase inhibitor imatinib (STI571) in chronic phase and blast crisis chronic myeloid leukemia. Cancer Cell 2, 117–125. doi: 10.1016/S1535-6108(02)00096-X
Shah, N. P., Tran, C., Lee, F. Y., Chen, P., Norris, D., and Sawyers, C. L. (2004). Overriding imatinib resistance with a novel ABL kinase inhibitor. Science 305, 399–401. doi: 10.1126/science.1099480
Shi, Z., Resing, K. A., and Ahn, N. G. (2006). Networks for the allosteric control of protein kinases. Curr. Opin. Struct. Biol. 16, 686–692. doi: 10.1016/j.sbi.2006.10.011
Shiri, F., Pirhadi, S., and Ghasemi, J. B. (2016). Alignment independent 3D-QSAR, quantum calculations and molecular docking of Mer specific tyrosine kinase inhibitors as anticancer drugs. Saudi Pharm. J. 24, 197–212. doi: 10.1016/j.jsps.2015.03.012
Shirts, M., and Pande, V. S. (2000). COMPUTING: screen savers of the world unite! Science 290, 1903–1904. doi: 10.1126/science.290.5498.1903
Shuker, S. B., Hajduk, P. J., Meadows, R. P., and Fesik, S. W. (1996). Discovering high-affinity ligands for proteins: SAR by NMR. Science 274, 1531–1534. doi: 10.1126/science.274.5292.1531
Shukla, D., Meng, Y., Roux, B., and Pande, V. S. (2014). Activation pathway of Src kinase reveals intermediate states as targets for drug design. Nat. Commun. 5:3397. doi: 10.1038/ncomms4397
Simeon, S., Jongkon, N., Chotpatiwetchkul, W., and Gleeson, M. P. (2019). Insights into the EGFR SAR of N-phenylquinazolin-4-amine-derivatives using quantum mechanical pairwise-interaction energies. J. Comput. Aided Mol. Des. 33, 745–757. doi: 10.1007/s10822-019-00221-z
Smajić, M., Nikoli,ć, K., Vujić, Z., Ahmetović, L., and Kuntić, V. (2015). 3D-QSAR studies and pharmacophore identification of AT1 receptor antagonists. Med. Chem. Res. 25, 51–61. doi: 10.1007/s00044-015-1470-1
Sobhy, M. K., Mowafy, S., Lasheen, D. S., Farag, N. A., and Abouzid, K. A. M. (2019). 3D-QSAR pharmacophore modelling, virtual screening and docking studies for lead discovery of a novel scaffold for VEGFR 2 inhibitors: design, synthesis and biological evaluation. Bioorg. Chem. 89:102988. doi: 10.1016/j.bioorg.2019.102988
Song, K., Liu, X., Huang, W., Lu, S., Shen, Q., Zhang, L., et al. (2017). Improved method for the identification and validation of allosteric sites. J. Chem. Inf. Model. 57, 2358–2363. doi: 10.1021/acs.jcim.7b00014
Sousa, S. F., Fernandes, P. A., and Ramos, M. J. (2006). Protein-ligand docking: current status and future challenges. Proteins 65, 15–26. doi: 10.1002/prot.21082
Subramanian, V., Prusis, P., Pietila, L. O., Xhaard, H., and Wohlfahrt, G. (2013). Visually interpretable models of kinase selectivity related features derived from field-based proteochemometrics. J. Chem. Inf. Model. 53, 3021–3030. doi: 10.1021/ci400369z
Subramanian, V., Prusis, P., Xhaard, H., and Wohlfahrt, G. (2016). Predictive proteochemometric models for kinases derived from 3D protein field-based descriptors. Medchemcomm 7, 1007–1015. doi: 10.1039/C5MD00556F
Sudha, A., Srinivasan, P., and Rameshthangam, P. (2015). Exploration of potential EGFR inhibitors: a combination of pharmacophore-based virtual screening, atom-based 3D-QSAR and molecular docking analysis. J. Recept. Signal Transduct. Res. 35, 137–148. doi: 10.3109/10799893.2014.942461
Sun, C., Petros, A. M., and Hajduk, P. J. (2011). Fragment-based lead discovery: challenges and opportunities. J. Comput. Aided Mol. Des. 25, 607–610. doi: 10.1007/s10822-011-9451-z
Sun, H. (1998). COMPASS: An ab initio force-field optimized for condensed-phase applicationsoverview with details on alkane and benzene compounds. J. Phys. Chem. B 102, 7338–7364. doi: 10.1021/jp980939v
Taylor, R. D., Jewsbury, P. J., and Essex, J. W. (2002). A review of protein-small molecule docking methods. J. Comput. Aided Mol. Des. 16, 151–166. doi: 10.1023/A:1020155510718
Taylor, S. S., and Kornev, A. P. (2011). Protein kinases: evolution of dynamic regulatory proteins. Trends Biochem. Sci. 36, 65–77. doi: 10.1016/j.tibs.2010.09.006
Taylor, S. S., Radzio-Andzelm, E., and Hunter, T. (1995). How do protein kinases discriminate between serine/threonine and tyrosine? Structural insights from the insulin receptor protein-tyrosine kinase. FASEB J. 9, 1255–1266. doi: 10.1096/fasebj.9.13.7557015
Teli, M. K., and Rajanikant, G. K. (2012). Pharmacophore generation and atom-based 3D-QSAR of novel quinoline-3-carbonitrile derivatives as Tpl2 kinase inhibitors. J. Enzyme Inhib. Med. Chem. 27, 558–570. doi: 10.3109/14756366.2011.603128
Trott, O., and Olson, A. J. (2010). AutoDock Vina: improving the speed and accuracy of docking with a new scoring function, efficient optimization, and multithreading. J. Comput. Chem. 31, 455–461. doi: 10.1002/jcc.21334
Tsou, H. R., Otteng, M., Tran, T., Floyd, M. B. Jr., Reich, M., Birnberg, G., et al. (2008). 4-(Phenylaminomethylene)isoquinoline-1,3(2H,4H)-diones as potent and selective inhibitors of the cyclin-dependent kinase 4 (CDK4). J. Med. Chem. 51, 3507–3525. doi: 10.1021/jm800072z
Ung, P. M., Rahman, R., and Schlessinger, A. (2018). Redefining the protein kinase conformational space with machine learning. Cell Chem. Biol. 25, 916–924.e912. doi: 10.1016/j.chembiol.2018.05.002
Vanderpool, D., Johnson, T. O., Ping, C., Bergqvist, S., Alton, G., Phonephaly, S., et al. (2009). Characterization of the CHK1 allosteric inhibitor binding site. Biochemistry 48, 9823–9830. doi: 10.1021/bi900258v
Vanommeslaeghe, K., Guvench, O., and MacKerell, A. D. Jr. (2014). Molecular mechanics. Curr. Pharm. Des. 20, 3281–3292. doi: 10.2174/13816128113199990600
Verdonk, M. L., Cole, J. C., Hartshorn, M. J., Murray, C. W., and Taylor, R. D. (2003). Improved protein-ligand docking using GOLD. Proteins 52, 609–623. doi: 10.1002/prot.10465
Vittorio, S., Seidel, T., Germano, M. P., Gitto, R., Ielo, L., Garon, A., et al. (2019). A combination of pharmacophore and docking-based virtual screening to discover new tyrosinase inhibitors. Mol. Inform. doi: 10.1002/minf.201900054. [Epub ahead of print].
Vucicevic, J., Nikolic, K., and Mitchell, J. B. O. (2017). Rational drug design of antineoplastic agents using 3D-QSAR, cheminformatic, and virtual screening approaches. Curr. Med. Chem. 26, 3874–3889. doi: 10.2174/0929867324666170712115411
Vucicevic, J., Srdic-Rajic, T., Pieroni, M., Laurila, J. M., Perovic, V., Tassini, S., et al. (2016). A combined ligand- and structure-based approach for the identification of rilmenidine-derived compounds which synergize the antitumor effects of doxorubicin. Bioorg. Med. Chem. 24, 3174–3183. doi: 10.1016/j.bmc.2016.05.043
Wagner, J. R., Lee, C. T., Durrant, J. D., Malmstrom, R. D., Feher, V. A., and Amaro, R. E. (2016). Emerging computational methods for the rational discovery of allosteric drugs. Chem. Rev. 116, 6370–6390. doi: 10.1021/acs.chemrev.5b00631
Wan, P. T. C., Garnett, M. J., Roe, S. M., Lee, S., Niculescu-Duvaz, D., Good, V. M., et al. (2004). Mechanism of activation of the RAF-ERK signaling pathway by oncogenic mutations of B-RAF. Cell 116, 855–867. doi: 10.1016/S0092-8674(04)00215-6
Wang, J. L., Li, L., Hu, M. B., Wu, B., Fan, W. X., Peng, W., et al. (2019a). In silico drug design of inhibitor of nuclear factor kappa B kinase subunit beta inhibitors from 2-acylamino-3-aminothienopyridines based on quantitative structure-activity relationships and molecular docking. Comput. Biol. Chem. 78, 297–305. doi: 10.1016/j.compbiolchem.2018.12.021
Wang, Q., Zheng, M., Huang, Z., Liu, X., Zhou, H., Chen, Y., et al. (2012). Toward understanding the molecular basis for chemical allosteric modulator design. J. Mol. Graph. Model. 38, 324–333. doi: 10.1016/j.jmgm.2012.07.006
Wang, T., Peng, T., Wen, X., Wang, G., Sun, Y., Liu, S., et al. (2019b). Design, synthesis and preliminary biological evaluation of benzylsulfone coumarin derivatives as anti-cancer agents. Molecules 24:4034. doi: 10.3390/molecules24224034
Wang, Y., Tang, C., Yao, S., Lai, H., Li, R., Xu, J., et al. (2019c). Discovery of a novel protein kinase C activator from Croton tiglium for inhibition of non-small cell lung cancer. Phytomedicine 65:153100. doi: 10.1016/j.phymed.2019.153100
Warner, S. L., Bashyam, S., Vankayalapati, H., Bearss, D. J., Han, H., Mahadevan, D., et al. (2006). Identification of a lead small-molecule inhibitor of the Aurora kinases using a structure-assisted, fragment-based approach. Mol. Cancer Ther. 5, 1764–1773. doi: 10.1158/1535-7163.MCT-05-0524
Warren, G. L., Andrews, C. W., Capelli, A. M., Clarke, B., LaLonde, J., Lambert, M. H., et al. (2006). A critical assessment of docking programs and scoring functions. J. Med. Chem. 49, 5912–5931. doi: 10.1021/jm050362n
Webb, B., and Sali, A. (2016). Comparative Protein Structure Modeling Using MODELLER. Curr. Protoc. Bioinformatics 54, 5.6.1–5.6.37. doi: 10.1002/cpbi.3
Weiner, S. J., Kollman, P. A., Case, D. A., Singh, U. C., Ghio, C., Alagona, G., et al. (1984). A new force field for molecular mechanical simulation of nucleic acids and proteins. J. Am. Chem. Soc. 106, 765–784. doi: 10.1021/ja00315a051
Wendel, H. G., Silva, R. L., Malina, A., Mills, J. R., Zhu, H., Ueda, T., et al. (2007). Dissecting eIF4E action in tumorigenesis. Genes Dev. 21, 3232–3237. doi: 10.1101/gad.1604407
Wenthur, C. J., Gentry, P. R., Mathews, T. P., and Lindsley, C. W. (2014). Drugs for allosteric sites on receptors. Annu. Rev. Pharmacol. Toxicol. 54, 165–184. doi: 10.1146/annurev-pharmtox-010611-134525
Wishart, D. S., Feunang, Y. D., Guo, A. C., Lo, E. J., Marcu, A., Grant, J. R., et al. (2018). DrugBank 5.0: a major update to the DrugBank database for 2018. Nucleic Acids Res. 46, D1074–D1082. doi: 10.1093/nar/gkx1037
Wong, M. L., Murphy, J., Harrington, E., Gower, C. M., Jain, R. K., Schirle, M., et al. (2017). Examining the influence of specificity ligands and ATP-competitive ligands on the overall effectiveness of bivalent kinase inhibitors. Proteome Sci. 15:17. doi: 10.1186/s12953-017-0125-1
Wu, P., Clausen, M. H., and Nielsen, T. E. (2015a). Allosteric small-molecule kinase inhibitors. Pharmacol. Ther. 156, 59–68. doi: 10.1016/j.pharmthera.2015.10.002
Wu, P., Nielsen, T. E., and Clausen, M. H. (2015b). FDA-approved small-molecule kinase inhibitors. Trends Pharmacol. Sci. 36, 422–439. doi: 10.1016/j.tips.2015.04.005
Xu, Y., Wang, S., Hu, Q., Gao, S., Ma, X., Zhang, W., et al. (2018). CavityPlus: a web server for protein cavity detection with pharmacophore modelling, allosteric site identification and covalent ligand binding ability prediction. Nucleic Acids Res. 46, W374–W379. doi: 10.1093/nar/gky380
Yamanishi, Y., Araki, M., Gutteridge, A., Honda, W., and Kanehisa, M. (2008). Prediction of drug-target interaction networks from the integration of chemical and genomic spaces. Bioinformatics 24, i232–i240. doi: 10.1093/bioinformatics/btn162
Yang, B., and Papoian, T. (2012). Tyrosine kinase inhibitor (TKI)-induced cardiotoxicity: approaches to narrow the gaps between preclinical safety evaluation and clinical outcome. J. Appl. Toxicol. 32, 945–951. doi: 10.1002/jat.2813
Yang, H., Chennamaneni, L. R., Ho, M. W. T., Ang, S. H., Tan, E. S. W., Jeyaraj, D. A., et al. (2018). Optimization of selective mitogen-activated protein kinase interacting kinases 1 and 2 inhibitors for the treatment of blast crisis leukemia. J. Med. Chem. 61, 4348–4369. doi: 10.1021/acs.jmedchem.7b01714
Yang, Y. I., Shao, Q., Zhang, J., Yang, L., and Gao, Y. Q. (2019). Enhanced sampling in molecular dynamics. J. Chem. Phys. 151:070902. doi: 10.1063/1.5109531
Yin, D., and MacKerell, A. D. (1998). Combinedab initio/empirical approach for optimization of Lennard-Jones parameters. J. Comp. Chem. 19, 334–348. doi: 10.1002/(SICI)1096-987X(199802)19:3<334::AID-JCC7>3.0.CO;2-U
Yu, C., Liu, X., Yang, J., Zhang, M., Jin, H., Ma, X., et al. (2019). Combination of immunotherapy with targeted therapy: theory and practice in metastatic melanoma. Front. Immunol. 10:990. doi: 10.3389/fimmu.2019.00990
Yuan, Y., Pei, J., and Lai, L. (2013). Binding site detection and druggability prediction of protein targets for structure-based drug design. Curr. Pharm. Des. 19, 2326–2333. doi: 10.2174/1381612811319120019
Yueh, C., Rettenmaier, J., Xia, B., Hall, D. R., Alekseenko, A., Porter, K. A., et al. (2019). Kinase Atlas: druggability analysis of potential allosteric sites in kinases. J. Med. Chem. 62, 6512–6524. doi: 10.1021/acs.jmedchem.9b00089
Yun, T., Qin, T., Liu, Y., and Lai, L. (2016). Discovery of non-ATP-competitive inhibitors of polo-like kinase 1. ChemMedChem 11, 713–717. doi: 10.1002/cmdc.201600051
Zhan, J. Y., Ma, J., and Zheng, Q. C. (2019). Molecular dynamics investigation on the Asciminib resistance mechanism of I502L and V468F mutations in BCR-ABL. J. Mol. Graph. Model. 89, 242–249. doi: 10.1016/j.jmgm.2019.03.018
Zhang, G., and Ren, Y. (2018). Molecular modeling and design studies of purine derivatives as novel CDK2 inhibitors. Molecules 23:2924. doi: 10.3390/molecules23112924
Zhang, S., Golbraikh, A., and Tropsha, A. (2006). Development of quantitative structure-binding affinity relationship models based on novel geometrical chemical descriptors of the protein-ligand interfaces. J. Med. Chem. 49, 2713–2724. doi: 10.1021/jm050260x
Zhang, W., Pei, J., and Lai, L. (2017). Statistical analysis and prediction of covalent ligand targeted cysteine residues. J. Chem. Inf. Model. 57, 1453–1460. doi: 10.1021/acs.jcim.7b00163
Zhao, B., Zhao, C., Hu, X., Xu, S., Lan, Z., Guo, Y., et al. (2019a). Design, synthesis and 3D-QSAR analysis of novel thiopyranopyrimidine derivatives as potential antitumor agents inhibiting A549 and Hela cancer cells. Eur. J. Med. Chem. 185:111809. doi: 10.1016/j.ejmech.2019.111809
Zhao, H., Dong, J., Lafleur, K., Nevado, C., and Caflisch, A. (2012). Discovery of a novel chemotype of tyrosine kinase inhibitors by fragment-based docking and molecular dynamics. ACS Med. Chem. Lett. 3, 834–838. doi: 10.1021/ml3001984
Zhao, Y., Liu, F., He, G., Li, K., Zhu, C., Yu, W., et al. (2019b). Discovery of arylamide-5-anilinoquinazoline-8-nitro derivatives as VEGFR-2 kinase inhibitors: synthesis, in vitro biological evaluation and molecular docking. Bioorg. Med. Chem. Lett. 29:126711. doi: 10.1016/j.bmcl.2019.126711
Zhavoronkov, A., Ivanenkov, Y. A., Aliper, A., Veselov, M. S., Aladinskiy, V. A., Aladinskaya, A. V., et al. (2019). Deep learning enables rapid identification of potent DDR1 kinase inhibitors. Nat. Biotechnol. 37, 1038–1040. doi: 10.1038/s41587-019-0224-x
Zhou, N., Xu, Y., Liu, X., Wang, Y., Peng, J., Luo, X., et al. (2015). Combinatorial pharmacophore-based 3D-QSAR analysis and virtual screening of FGFR1 inhibitors. Int. J. Mol. Sci. 16, 13407–13426. doi: 10.3390/ijms160613407
Keywords: kinase inhibitors, rational drug design, molecular modeling, drug discovery, pharmacophore
Citation: Gagic Z, Ruzic D, Djokovic N, Djikic T and Nikolic K (2020) In silico Methods for Design of Kinase Inhibitors as Anticancer Drugs. Front. Chem. 7:873. doi: 10.3389/fchem.2019.00873
Received: 28 September 2019; Accepted: 04 December 2019;
Published: 08 January 2020.
Edited by:
Simone Brogi, University of Pisa, ItalyReviewed by:
Elif Ozkirimli, Bogaziçi University, TurkeyAnna Maria Almerico, University of Palermo, Italy
Mahmoud A. Al-Sha'er, Zarqa Private University, Jordan
Chandrabose Selvaraj, Central European Institute of Technology, Brno University of Technology, Czechia
Copyright © 2020 Gagic, Ruzic, Djokovic, Djikic and Nikolic. This is an open-access article distributed under the terms of the Creative Commons Attribution License (CC BY). The use, distribution or reproduction in other forums is permitted, provided the original author(s) and the copyright owner(s) are credited and that the original publication in this journal is cited, in accordance with accepted academic practice. No use, distribution or reproduction is permitted which does not comply with these terms.
*Correspondence: Katarina Nikolic, a25pa29saWNAcGhhcm1hY3kuYmcuYWMucnM=