- 1Laboratory of Tree-Ring Research and School of Geography, Development and Environment, University of Arizona, Tucson, AZ, United States
- 2School of Human Evolution and Social Change and Center for Social Dynamics and Complexity, Arizona State University, Tempe, AZ, United States
When droughts and floods struck ancient agrarian societies, complex networks of exchange and interaction channeled resources into affected settlements and migrant flows away from them. Did these networks evolve in part to connect populations living in differing climate regimes? Here, I examine this relationship with a long-term archaeological case study in the pre-Hispanic North American Southwest, analyzing 4.3 million artifacts from a 250-year period at nearly 500 archaeological sites. I use these artifacts to estimate how the flow of social information changed over time, and to measure how the intensity of social interaction between sites varied as a function of distance and several regional drought patterns. Social interaction decayed with distance, but ties between sites in differing oceanic and continental climate regimes were often stronger than expected by distance alone. Accounting for these different regional drivers of local climate variability will be crucial for understanding the social impacts of droughts and floods in the past and present.
1 Introduction
Exchange networks are part of the broad toolkit of social and physical infrastructure humans use to manage environmental risk in social-ecological systems (Anderies, 2015). The environment can structure these exchange networks by influencing the costs and benefits of social interaction. Recent theoretical and empirical work highlights how spatial, social, and environmental factors interact with networks of exchange and interaction (Fafchamps and Gubert, 2007; Bloch et al., 2008; Nolin, 2010; Verdery et al., 2012; Freeman et al., 2014; Koster and Leckie, 2014; Hao et al., 2015; Schnegg, 2015). Distance is a key factor in such systems, making it difficult to monitor conditions in potential migration destinations (Anderies and Hegmon, 2011) and know the resources and reputation of potential interaction partners (Fafchamps and Gubert, 2007), as well as increasing the metabolic costs of transport (Drennan, 1984). For agricultural societies in water-limited environments, hydroclimate variability–changes in the balance of precipitation and evapotranspiration–may be another key factor. The benefits of interacting with others in different drought regimes can outweigh the costs of traveling longer distances. As a consequence, we might expect a greater “investment of social energy in the maintenance of social ties” between populations experiencing poorly or negatively correlated climate variability (Rautman, 1993). Norms and institutions that maintain ties between different climate regimes are likely to evolve (Durante, 2009). This process is difficult to measure in the present day due to the mismatch between the generational time scale on which cultural evolution occurs and the limited time horizons available to contemporary social sciences. Instead, we can turn to the archaeological record.
Archaeology focuses on the material correlates of human behavior and is unique in addressing how social and physical infrastructure modulate human interactions with the environment over long time spans. Not only do archaeologists catalog the remains of field systems, road networks, canals, and other components of hard infrastructure directly, but also the ceramics, raw materials, and luxury goods that are the material correlates of past networks of exchange and interaction. A powerful idea in archaeology is that, because of the interaction between societies and their biophysical environments, the spatial and temporal patterns of environmental variability can be used to predict “ideal” cultural responses and compared to archaeological observations (Halstead and O’Shea, 1989). Yet in practice it is often difficult to find archaeological data fit for purpose due to the incomplete nature of the archaeological record and the paucity of detailed paleoclimate data at the scales most relevant to human populations.
The North American Southwest is an exception. The climate of this region has been intensively studied by paleoclimatologists and climate modelers (Cook et al., 1999; Sheppard et al., 2002; McCabe et al., 2004; Herweijer et al., 2007; Cook et al., 2011; Bocinsky and Kohler, 2014; Coats et al., 2015; Routson et al., 2016; Ault et al., 2018). Its aridity aids archaeological site preservation and recovery, and nearly two centuries of survey and excavation have yielded extensive, high quality settlement pattern data (Hill et al., 2004). Detailed inventories of material culture at hundreds of archaeological sites provide an unparalleled view of the structure and dynamics of past social networks. This archaeological record attests to extensive exchange networks of durable goods such as ceramics and obsidian (Malville, 2001; Taliaferro et al., 2010; Mills et al., 2013a), and there is evidence for the long-distance transport of limited quantities of maize to the large regional center at Chaco Canyon (Benson et al., 2009; Benson, 2010). The populations of the Southwest also underwent massive social transformation, migration, and population decline in the late 13th century contemporaneous with one of the worst droughts in the last 1,000 years (Hill et al., 2004). Past work has suggested a relationship between the intensity of social interaction and patterns of drought variability, but has been limited by small sample sizes or sparse climate data (Rautman, 1993; Johnson, 1990; Cordell et al., 2007). The question is returning to the fore with the advent of high resolution climate observations and reconstructions, facilitating more detailed accounting of the spatial patterns of drought in the North American Southwest (Strawhacker et al., 2017; Strawhacker et al., 2020), and more detailed archaeological datasets (Borck et al., 2015). Simulations suggest that the precise nature of environmental variability is critical for exchange dynamics (Freeman et al., 2014). With these advances in our ability to map droughts in space and time comes the need to more precisely define what patterns of climate variability are actually important.
Here, I draw on hydroclimate data from the past and present to isolate specific reoccurring climate patterns, or modes of variability, in the American Southwest. I then compare these patterns to prehistoric social networks, inferred from a dataset of 4.3 million ceramic artifacts from nearly 500 archaeological sites, to examine the relationship between hydroclimate variability, distance, and social interaction over a 250-year span.
2 Methods
2.1 Archaeological Interaction Networks
I analyzed data from nearly 500 archaeological sites in the Southwest Social Networks (SWSN) database, a compendium of material-culture data from well-dated sites west of the Continental Divide in Arizona and New Mexico (Mills et al., 2013a; Mills et al., 2013b; Peeples and Haas, 2013; Borck et al., 2015; Hill et al., 2015; Mills et al., 2015). Version 1.0 of the SWSN database cataloged nearly 4.3 million ceramic artifacts and nearly 5,000 obsidian artifacts, providing quantitative estimates of the topology of the region-wide social network during five 50-year time steps spanning the period 1200–1450 CE (Mills et al., 2013a; Mills et al., 2015). Raw site-level ceramic counts were allocated to each time step according to an apportioning procedure that combined the occupation span of each site and the production span of each ware type with a parametric assumption of the wares’ changing popularity through time (Mills et al., 2013a; Peeples and Haas, 2013).
I aggregated the point-based SWSN data into 10 km grid cells (Figure 1A) so that the network estimates were less sensitive to local settlement dispersal or aggregation as reflected in the assemblages at individual sites (Paliou and Bevan, 2016). The choice of 10 km grid cells reflects a day’s round-trip travel, bounding the area for farming and raw material collection around a site, so the procedure effectively smooths over the approximate area of each site’s resource catchment (Varien, 1999; Hill et al., 2015). Then I calculated a modified version of the Jensen-Shannon divergence (Masucci et al., 2011) between the empirical frequency distributions of 15 decorated ceramic wares at each of the grid cells as
where
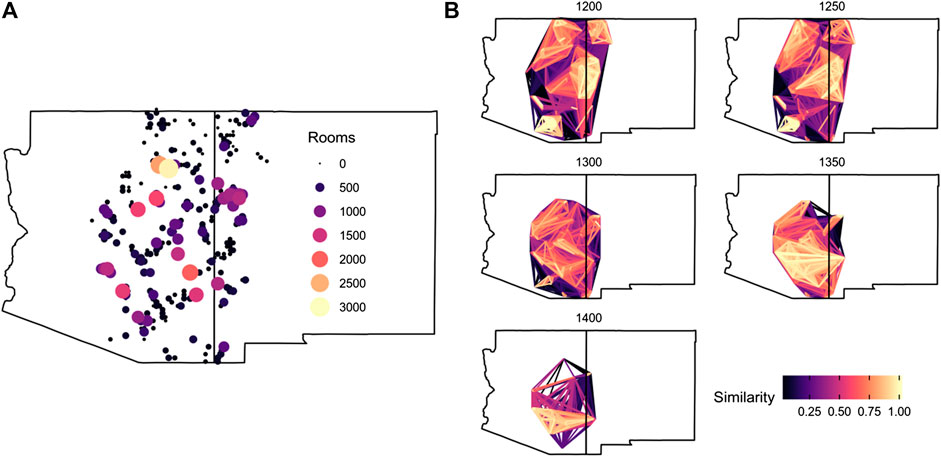
FIGURE 1. The Southwest Social Networks dataset, version 1.0 (Mills et al., 2013a). (A) Site locations for all time periods in the dataset, aggregated into 10 km patches to reduce biases from local settlement aggregation and dispersal. Site sizes are estimated from room counts. (B) Social networks reconstructed from ceramic assemblage similarity between each pair of sites. A similarity coefficient of one means that the sites share the same decorated ceramic wares in the exact same proportions, and a coefficient of zero means there is no overlap in the ceramic assemblages. The similarity network can be interpreted as the degree of social interaction and cultural transmission between sites, whether via migration, trade, or copying. Networks are shown over successive 50-year time spans, starting at 1200 CE.
The resulting cultural similarity network (Figure 1B) is similar to that resulting from other similarity measures such as the Brainerd-Robinson index (Mills et al., 2013a) save for different behavior in the tails of the distribution, but the Jensen-Shannon index provides a more natural interpretation as a measure of information flow. By focusing on a general measure of information flow, aggregate patterns of social interaction can be inferred regardless of the precise mechanisms of that interaction (e.g., trade, migration, shared history or raw materials). The index can thus be loosely interpreted as a probability of interaction between two sites, with identical patterns of ceramic discard at two sites indicating a higher probability of interaction than between sites that share no ware types in common.
2.2 Hydroclimate Variability
To estimate large-scale patterns of interannual drought and flood variability, I analyzed a 122-year record of the Standardized Precipitation-Evapotranspiration Index (SPEI) from across the US states of Arizona, New Mexico, Colorado, Utah, and California (Abatzoglou et al., 2017). The large spatial domain sampled variability across the western US climate zone, ensuring that estimated spatio-temporal climate patterns were not sensitive to the exact location and dimension of the archaeological study area. SPEI is the normalized deviation from the average climatic water balance for a given month on varying time scales (Vicente-Serrano et al., 2010). I focused on the 12-month SPEI calculated in the August of each year, which captures the water balance over the year leading up to each summer growing season. This index was calculated from 4 km temperature and precipitation grids interpolated from weather-station observations using the topographically-sensitive PRISM algorithm (Daly et al., 1997).
Weather can vary for many reasons across space and time, so it is important to separate climatic signal from random noise. Principal Components Analysis (PCA) of spatiotemporal data is a common tool for extracting “modes of variability” in the climate sciences (Lorenz, 1956; Hannachi et al., 2007), but its use for this purpose is rare in archaeology (though see (Weiss, 1982; Cordell et al., 2007)). PCA of a dataset’s space-time covariance matrix decomposes it into sets of orthogonal time series (principal components) and spatial patterns (eigenvectors), arranged by their contributions to the total observed variance (eigenvalues). The resulting modes of variability are an efficient means of representing a complex spatiotemporal field by a limited set of patterns.
After multiplying each grid cell by the cosine of its latitude to weight for differences cell area, I performed PCA on the stack of 122 annual SPEI maps via singular value decomposition. Then, I selected the leading modes of variability using both a scree test and North’s rule of thumb, which is a method to detect degenerate patterns caused by temporal autocorrelation in the observed data (North et al., 1982). I rotated the leading PC modes using a varimax rotation in order to relax the spatial orthogonality constraints of the PCA analysis and reveal coherent, physically meaningful patterns (Richman, 1986). The resulting eigenvectors were then multiplied by the square root of the corresponding eigenvalues to yield correlation coefficients and were mapped in space. I refer to these resulting spatial patterns as empirical orthogonal functions (EOFs), and their associated time series as principal components (PCs). The PC amplitude time series were then compared to the observational record, and the signs of the eigenvalues and vectors were reversed to match the historical record (so that a positive time series value corresponded to a positive SPEI and vice versa). To determine whether these patterns were robust over time, the observed EOFs were compared to the EOFs of a SPEI reconstruction over the past millennium (Steiger et al., 2018) (see SI for details).
2.3 Least-Cost Networks
Distance ultimately constrains social interaction, as the further one travels to interact with a partner the greater will be the cost in time, energy, and other resources. In order to control for the effect of distance on social interaction, I calculated the least-cost network between all sites in the SWSN network. The topography of the study area was represented using a 90 m SRTM DEM, resampled to 250 m to reduce computation time and smooth fine-scale topographic noise. A cost matrix was calculated containing, for each DEM cell, the amount of time in seconds it would take a foot traveler to move to each of the 16 neighboring cells. Time costs were calculated using a version of Tobler’s hiking function, which estimates walking speed from terrain slope. The function was modified to make it isotropic (i.e., averaging the uphill and downhill walking speeds) and adding an extra penalty to very steep slopes consistent with human cognitive biases (Pingel, 2010). This cost matrix (time) was then inverted to represent conductance (speed), facilitating a sparse matrix representation and estimation of least cost paths using efficient graph theoretic algorithms (van Etten, 2017). The resulting transition matrix was used to calculate all pairwise isotropic least cost paths between the centroids of each pair of 10 km grid cells containing archaeological materials.
2.4 Spatial Interaction Models
Spatial interaction models are used across the social and natural sciences (Wilson, 1971; Fotheringham and O’Kelly, 1989; Sen and Smith, 1995; Bavaud, 2008; Murphy et al., 2010; Head and Mayer, 2015). In a regression context, a spatial interaction model estimates the pairwise flow–of resources, migrants, or information–among entities as a multiplicative function of predictors influencing the production and attraction of flows as well as measures of their mutual separation or other generalized costs of moving. Archaeologists have used statistical spatial interaction models sparingly (Tobler and Wineburg, 1971; Hodder, 1974; Johnson, 1990) because of the rarity of archaeological data on social interaction strength, although the method is common in simulation studies where data quality is less of a restriction (Bevan and Wilson, 2013; Evans et al., 2011; Davies et al., 2014; Paliou and Bevan, 2016). The conceptual justification for the use of spatial interaction models on archaeological networks is similar to that used in molecular ecology (Murphy et al., 2010), with information flows among a spatially-structured metapopulation measured by the divergence of those populations (Mesoudi, 2018).
Data of this type have three features that make traditional statistical spatial interaction modeling difficult. These are: 1) the data are bounded between zero and one; 2) the measures are pairwise symmetric; 3) we have no exact functional expectations for the specific terms in the spatial interaction model because empirical work on this scale and type is rare. To address these issues, I used a generalized additive model (Wood, 2006), a semiparametric extension to generalized linear models useful for more complex spatial interaction models (Lebacher et al., 2018).
Specifically, I fit models of the form
where the logit function maps the data from
3 Results
3.1 Six Drought Patterns Explain 83% of Observed Drought Variability in the American Southwest
I used PCA to decompose the 122-year gridded observational record of western US summer moisture availability into a reduced set of spatio-temporal patterns. The leading six principal component time series together explain 83% of the variance in the observational record. The PCs represent time series that are maximally representative of the entire data set (Figure 2). I rotated the six PCs before mapping, in order to capture more physically meaningful patterns and minimize statistical artifacts. PCs beyond the leading six were not retained for rotation and mapping, as they represent spatially and temporally incoherent variability and spurious correlations introduced by sampling error in the observational record. The same PC time series are also present in the coarse-resolution SPEI reconstruction, where they explain 96% of the reconstructed variance over the last millennium (Supplementary Figures S5, S12, S13).
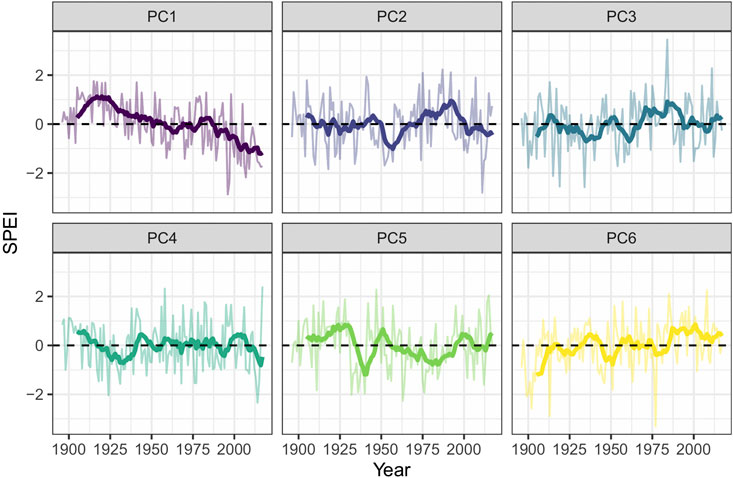
FIGURE 2. Time series associated with the leading six PCs for the observational period, after varimax rotation. The y-axis corresponds to the 12-month Standardized Precipitation-Evapotranspiration Index (SPEI), the normalized deviation from the average climatic water balance for a given month on 12-month time scale. SPEI values can be interpreted as z-scores in a normal distribution (i.e., a value of 1 is one standard deviation wetter than average for that location, −1 is one standard deviation drier). 10-year moving averages superimposed over raw annual values.
3.2 Different Drought Patterns Are Associated With Different Zones of Oceanic or Continental Influence
To reveal the latent spatial structures associated with the temporal modes of variability, I mapped the spatial patterns associated with each of the leading six PCs (Figure 3). The results are robust, recurring patterns of spatially-coherent variability, and can be interpreted as the degree to which the 122-year record at each grid cell correlates with the associated rotated PC time series. These spatial patterns are known as the (rotated) empirical orthogonal functions (EOFs). The patterns are consistent across observations and reconstructions (Supplementary Figure S9) and regardless of the exact SPEI time scale used to calculate them, which supports their overall robustness. The spatial and temporal patterns associated with the leading six PCs allows us to trace the sources of each mode of variability back to the global climate system.
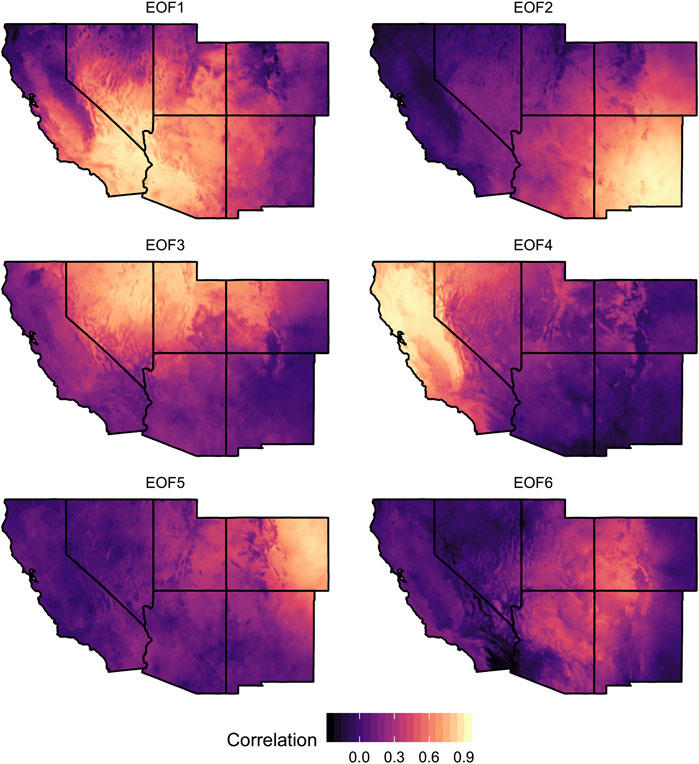
FIGURE 3. Leading six rotated empirical orthogonal functions (EOFs) associated with the respective PC time series in Figure 2, derived from gridded PRISM climate data (Daly et al., 2008; Abatzoglou et al., 2017). These regions represent different oceanic and atmospheric influences; people living in the same EOF will often experience dry and wet years at the same time as one another.
The origins of each drought pattern can be determined by examining the EOF maps, along with the correlations of the PCs to global sea surface temperatures and the timing of extreme dry and wet years. EOF1 reflects southwesterly flow from the tropical Pacific, bringing moisture across the low desert zones of California and Arizona. The pattern attenuates with elevation and as distance from the ocean increases. PC1 shows a broad drying trend to the present day, possibly related to increased evaporative demand due to recent warming, although the spatial pattern in the associated EOF is not itself anthropogenic. EOF2 similarly represents southeasterly flow from the Gulf of Mexico, centered on eastern New Mexico. As with EOF1, the pattern attenuates with increasing elevation and distance from the ocean due to orography and continentality, respectively. It represents cyclonic storms coming from the Gulf of Mexico, in turn influenced by variability in Atlantic sea surface temperatures. PC2 shows a major dry period centered on the Texas/New Mexico drought of 1956. EOF3 represents northerly flow associated with polar continental cold fronts, and its associated PC shows a wet peak in the 1983 Salt Lake City floods. EOF4 represents the influence of westerly flow off the Pacific Ocean and the orographic effect of the Sierra Nevada mountains intercepting this flow, and is associated with events such as the 1924 drought in California. EOF5 is centered over the great plains and attenuates across the Rocky Mountains, and was most strongly expressed during the Dust Bowl of the 1920s. EOF6 is centered on the Colorado Plateau, likely reflecting local circulation of hot continental air masses.
3.3 The Intensity of Social Interaction Decays Nonlinearly With Distance
I calculated the cost of moving between each pair of archaeological sites as the shortest amount of time it would take a foot traveler to move between them. I then used a nonlinear regression model to estimate the functional relationship between distance and interaction. The null model for the statistical network analysis was that distance alone explained the intensity of social interaction as measured by the similarity in the decorated ceramic assemblages at each pair of sites. This null model was sufficient to explain 37.8% of the variance in the ceramic similarity data. The empirical distance deterrence function estimated on all time periods using a penalized regression spline predicts a falloff in interaction at distances of more than 100 h (Figure 4). As expected, the resulting distance-based network predicts many strong interactions at close distances, and the residuals of the model show long-distance transitive ties.
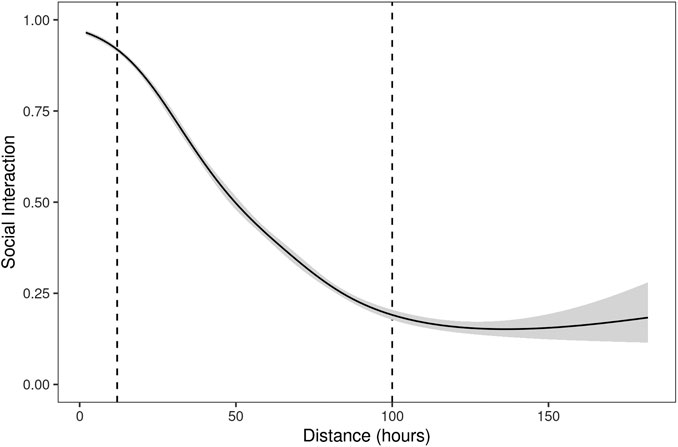
FIGURE 4. Empirical distance deterrence function estimated with a generalized additive model, describing how the intensity of social interaction, defined as the information flow between two settlements and measured by the similarity in their decorated ceramic assemblages, decreases as a function of distance. Shaded area indicates the 95% confidence interval for the smooth function. Dashed lines indicate key thresholds in the function at 10 and 100 hours.
3.4 Hydroclimate Variability Explains a Moderate But Clear Proportion of the Intensity of Social Interaction
A model predicting information flow using distance and climatic dissimilarity, measured as the absolute difference between the EOF loadings of a pair of sites, explains 42.5% of the variance in the ceramic similarity data. The increase over the distance-only null model is moderate but statistically significant, and the EOF model is superior in all measures of parsimony and goodness-of-fit. This difference changes over time, and refitting each model on data from each time step individually reveals that the improvement in the explanatory power of the EOF model over the distance-only null is most pronounced at and after 1300 CE (see SI). The improvement in explanatory power over the null model is quite small in the 1200 and 1250 CE time steps. This pattern suggests that ties shaped in part by the EOF patterns are more common during and after the period of regional relocation around 1300 CE.
The smooth functions estimated in the EOF model are all close to piecewise linear on the scale of the linear predictor, but the intensity of these functional relationships varies smoothly over time and across EOFs (Figure 5). Increasing distance along a particular EOF sometimes increases the intensity of social interaction, as was expected ahead of time, but some EOFs (3, 6) appear to slightly reduce social interaction at larger differences. The smoothness penalty also selects some EOFs out of the model entirely by estimating functions close to a horizontal line, and almost all the functions are flat when the climate differences are less than 0.2. Surprisingly, the fluctuations in the effect size of a particular EOF have no clear association with the sign of the associated PC amplitude time series reconstructed for each period (Supplementary Figure S14). This suggests that additional dynamic processes, such as cultural memory or institutional growth and decay, are in effect on time scales longer than a single generation. These processes may explain why different sets of EOF patterns appear to influence social interaction before and after the period of drought and interregional migration ca. 1275–1300 CE.
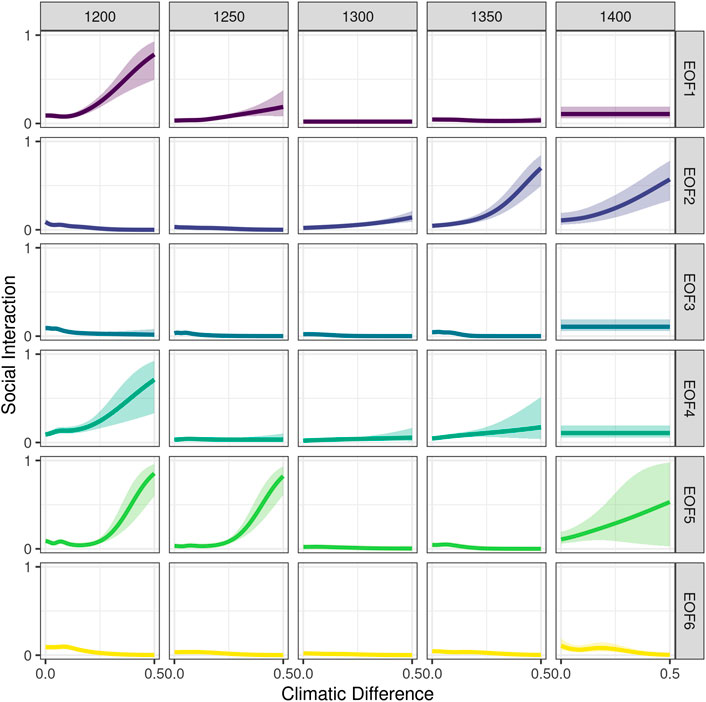
FIGURE 5. Estimated smooth functions describing how the intensity of social interaction increases or decreases with increasing distance along each of six spatial drought patterns from Figure 3, compared over five time steps. As above, information flow is inferred from the similarity of the decorated ceramic assemblages at each pair of sites. Climatic difference is defined as the absolute difference between the EOF loadings of each pair of sites. Shaded regions correspond to the 95% confidence intervals for the smooth functions.
4 Discussion and Conclusion
The six spatial patterns of hydroclimate variability isolated here are consistent with the general mechanistic understanding of hydroclimate variability in the American Southwest. These patterns represent different zones of moisture transport, reflecting the influence of topography and marine or continental moisture sources (Liu et al., 2010; Hu et al., 2011). These spatial and temporal drought patterns, and their hypothesized drivers in the global climate system, are largely consistent with those from other studies using varied observational data and time windows (Comrie and Glenn, 1999; Cook et al., 1999; McCabe and Dettinger, 1999; McCabe et al., 2004; Ryu et al., 2010; Seager and Hoerling, 2014; Herrmann et al., 2016). These patterns from the observational period also appear in drought reconstructions spanning the past millennium, emphasizing the fact that these are robust, time invariant spatial modes.
Objective measures of hydroclimate variability, as opposed to point-to-point sample correlations, help isolate the most important drivers of that variability. Droughts and pluvials associated with tropical Pacific and Atlantic influences seem to have been most important for structuring social interaction, with ties connecting these regions greater than expected by chance and distance alone. Tropical Pacific sea surface temperatures are known to be the primary driver of variability in Southwest, with additional influences from moisture sources in the North Pacific and Atlantic (McCabe et al., 2004). A disruption in these patterns is thought to be one reason why droughts in this period led to such social transformation, as the networks of social infrastructure that had developed over previous centuries were unable to adapt fast enough to unusual conditions (Cordell et al., 2007).
In spite of the robustness of these spatial patterns, there remains considerable diversity in the functional responses of human social networks to these drought patterns. One possible explanation is that large-scale climate regimes influence the formation of ethnolinguistic groups. Quotidian interaction may have been biased toward groups of shared ethnolinguistic affiliation, as kinship and ethnicity both influence social exchanges (Nolin, 2010). At larger, regional scales, goods and information might also flow on sociopolitical hierarchies (Crumley, 1979). Populations in the late pre-Hispanic Southwest were also out of equilibrium (Hill et al., 2004). Strong social networks take time to form and effort to maintain and monitor. Free-riders who avoid that effort can damage this critical social infrastructure when it is most needed (Kohler and West, 1996). A simulation approach could better capture these processes and more clearly resolve social responses to interannual climate variability. Dynamic, as opposed to statistical, spatial interaction models can explicitly trace the coevolution of social and physical infrastructure networks (Bevan and Wilson, 2013). Simulations can also explore the biases that static archaeological data introduce in representing dynamic social processes (Crema et al., 2014, 2016).
The residuals of the statistical network models retain unexplained structure. These structures appear to represent cultural clusters, a common feature in social networks that is not accounted for by either distance or drought variability. One source of this error may be irrigation-dependent groups who relied on streamflow driven by remote precipitation and evapotranspiration and may thus have had more complex, indirect dependencies on the large-scale climate patterns isolated here (Nelson et al., 2010). At finer scales, the model residuals also display evidence of transitivity and triad closure, with more closed triangle structures than would be expected by chance. Although this feature is common in human social networks, it is also to be expected because the measure of cultural similarity is a metric subject to the triangle inequality. Statistical methods specifically designed for such structures will be useful in future work (Stillman et al., 2017). In addition, the archaeological data are not spatially extensive enough to sample the full range of hydroclimate variability. Given the relative spatial scales of the environmental and cultural data, there is a risk that many different correlated climate patterns will be indistinguishable at the scale of the cultural data. Correlations between competing hypotheses are a source of error in model selection using information criteria (Shirk et al., 2018). In spite of these concerns, these results highlight two key points: the need to use objective and physically meaningful measures of hydroclimate to assess the social impacts of climate variability in the past and the need to capture social dynamics out of equilibrium with the biophysical environment.
These results refine our understanding of the geography of human adaptation to climate and climate change, and emphasize the role of social interaction in increasing the robustness of human populations to environmental variability. Much of the world’s food is still grown on small farms, and these farmers rely on complex spatial networks of formal and informal arrangements in much the same way as their predecessors have for thousands of years. Prehistoric exchange infrastructure evolved in part in response to robust, time-invariant spatial climate patterns. But social adaptions to one mode of variability are fragile to changes in the nature of that variability (Janssen et al., 2007). Large-scale patterns of hydroclimate variability act as a dynamic selective environment in which societies evolve new norms and institutions for regulating social interaction. Tracing the flows of information and energy within these complex social-ecological systems is essential for understanding their long-term behavior, and leveraging our archaeological understanding of why such systems succeed or fail will be critical to anticipating the impact of impending climate changes on farming communities in the developing world.
Data Availability Statement
All original contributions presented in the study are included in the article or Supplementary Material in a reproducible R Markdown document. A preprocessed version of the SWSN v1.0 data, aggregated to 10 km grid cells to mask individual site locations, is available in the Supplementary Material with links to additional input climate and topographic raster data. Further inquiries can be directed to the corresponding author.
Author Contributions
The author confirms being the sole contributor of this work and has approved it for publication.
Funding
Data and methods from the Southwest Social Networks project were supported by the National Science Foundation (NSF) Human and Social Dynamics Program Awards 0827007 (University of Arizona) and 0827011 (Archaeology Southwest), the NSF Archaeology Program Awards 1355374 (University of Arizona) and 1355381 (Archaeology Southwest), and the NSF Resource Implementation for Data Intensive Research (RIDIR) program Awards 1738258 (University of Arizona), 1738245 (Arizona State University), 1738181 (University of Colorado Boulder), and 1738062 (Archaeology Southwest).
Conflict of Interest
The author declares that the research was conducted in the absence of any commercial or financial relationships that could be construed as a potential conflict of interest.
Acknowledgments
Matt Peeples, Michael Barton, and Marty Anderies made extensive comments on earlier versions of this manuscript, and Archaeology Southwest and the Southwest Social Networks project kindly provided access to the SWSN v1.0 data. The contents of this manuscript have previously appeared in the author's doctoral dissertation (Gauthier, 2019).
Supplementary Material
The Supplementary Material for this article can be found online at: https://www.frontiersin.org/articles/10.3389/feart.2020.620856/full#supplementary-material.
References
Abatzoglou, J. T., McEvoy, D. J., and Redmond, K. T. (2017). The west wide drought tracker: drought monitoring at fine spatial scales. Bull. Am. Meteorol. Soc. 98 (9), 1815–1820. doi:10.1175/bams-d-16-0193.1
Anderies, J. M., and Hegmon, M. (2011). Robustness and resilience across scales: migration and resource degradation in the prehistoric US southwest. Ecol. Soc. 16 (2), 22. doi:10.5751/es-03825-160222
Anderies, J. M. (2015). Understanding the dynamics of sustainable social-ecological systems: human behavior, institutions, and regulatory feedback networks. Bull. Math. Biol. 77 (2), 259–280. doi:10.1007/s11538-014-0030-z
Ault, T. R., George, S. S., Smerdon, J. E., Coats, S., Mankin, J. S., Carrillo, C. M., et al. (2018). A robust null hypothesis for the potential causes of megadrought in Western North America. J. Clim. 31 (1), 3–24. doi:10.1175/jcli-d-17-0154.1
Bavaud, F. (2008). The endogenous analysis of flows, with applications to migrations, social mobility and opinion shifts. J. Math. Sociol. 32 (4), 239–266. doi:10.1080/00222500802352493
Benson, L. V., Stein, J. R., and Taylor, H. E. (2009). Possible sources of archaeological maize found in Chaco Canyon and aztec ruin, New Mexico. J. Archaeol. Sci. 36 (2), 387–407. doi:10.1016/j.jas.2008.09.023
Benson, L. V. (2010). Who provided maize to Chaco Canyon after the mid-12th-century drought?. J. Archaeol. Sci. 37 (3), 621–629. doi:10.1016/j.jas.2009.10.027
Bevan, A., and Wilson, A. (2013). Models of settlement hierarchy based on partial evidence. J. Archaeol. Sci. 40 (5), 2415–2427. doi:10.1016/j.jas.2012.12.025
Bloch, F., Genicot, G., and Ray, D. (2008). Informal insurance in social networks. J. Econ. Theor. 143 (1), 36–58. doi:10.1016/j.jet.2008.01.008
Bocinsky, R. K., and Kohler, T. A. (2014). A 2,000-year reconstruction of the rain-fed maize agricultural niche in the US Southwest. Nat. Commun. 5, 5618. doi:10.1038/ncomms6618
Borck, L., Mills, B. J., Peeples, M. A., and Clark, J. J. (2015). Are social networks survival networks? An example from the late pre-hispanic US southwest. J. Archaeol. Method Theor. 22 (1), 33–57. doi:10.31235/osf.io/ubvxy
Clarke, R. T., Rothery, P., and Raybould, A. F. (2002). Confidence limits for regression relationships between distance matrices : estimating gene flow with distance. J. Agric. Biol. Environ. Stat. 7 (3), 361–372. doi:10.1198/108571102320
Coats, S., Smerdon, J. E., Cook, B. I., and Seager, R. (2015). Are simulated megadroughts in the North American southwest forced?. J. Clim. 28 (1), 124–142. doi:10.1175/jcli-d-14-00071.1
Comrie, A. C., and Glenn, E. C. (1999). Principal components-based regionalization of precipitation regimes across the southwest United States and northern Mexico, with an application to monsoon precipitation variability. Clim. Res. 10 (3), 201–215. doi:10.3354/cr010201
Cook, E. R., Meko, D. M., Stahle, D. W., and Cleaveland, M. K. (1999). Drought reconstructions for the continental United States. J. Clim. 12 (4), 1145–1163. doi:10.1175/1520-0442(1999)012
Cook, B. I., Cook, E. R., Anchukaitis, K. J., Seager, R., and Miller, R. L. (2011). Forced and unforced variability of twentieth century North American droughts and pluvials. Clim. Dynam. 37 (5), 1097–1110. doi:10.1007/s00382-010-0897-9
Cordell, L. S., Van West, C. R., Dean, J. S., and Muenchrath, D. A. (2007). Mesa Verde settlement history and relocation: climate change, social networks, and Ancenstral Pueblo migration. KIVA 72 (4), 379–405. doi:10.1179/kiv.2007.72.4.001
Crema, E. R., Kerig, T., and Shennan, S. (2014). Culture, space, and metapopulation: a simulation-based study for evaluating signals of blending and branching. J. Archaeol. Sci. 43, 289–298. doi:10.1016/j.jas.2014.01.002
Crema, E. R., Kandler, A., and Shennan, S. (2016). Revealing patterns of cultural transmission from frequency data: equilibrium and non-equilibrium assumptions. Sci. Rep. 6, 39122. doi:10.1038/srep39122
Crumley, C. L. (1979). Three Locational Models: An Epistemological Assessment for Anthropology and Archaeology. Adv. Archaeol. Meth. Theory 2, 141–173.
Daly, C., Halbleib, M., Smith, J. I., Gibson, W. P., Doggett, M. K., Taylor, G. H., et al. (2008). Physiographically sensitive mapping of climatological temperature and precipitation across the conterminous United States. Int. J. Climatol. 28, 2031–2064. doi:10.1002/joc.1688
Daly, C., Taylor, G., and Gibson, W. (1997). The prism approach to mapping precipitation and temperature. AMS Conf. Appl. Climatol. 1, 1–4
Davies, T., Fry, H., Wilson, A., Palmisano, A., Altaweel, M., and Radner, K. (2014). Application of an entropy maximizing and dynamics model for understanding settlement structure: the Khabur Triangle in the Middle Bronze and Iron Ages. J. Archaeol. Sci. 43 (1), 141–154. doi:10.1016/j.jas.2013.12.014
Drennan, R. D. (1984). Long-distance transport costs in pre-Hispanic Mesoamerica. Am. Anthropol. 86 (1), 105–112. doi:10.1525/aa.1984.86.1.02a00100
Durante, R. (2009). Risk, cooperation and the economic origins of social trust: an empirical investigation., 1–45. Available at: http://papers.ssrn.com/abstract=1576774
Evans, T., Rivers, R., and Knappett, C. (2011). Interactions in space for archaeological models. Adv. Complex Syst. 15, 1150009. doi:10.1142/s021952591100327x
Fafchamps, M., and Gubert, F. (2007). The formation of risk sharing networks. J. Dev. Econ. 83 (2), 326–350. doi:10.1016/j.jdeveco.2006.05.005
Fotheringham, S. A., and O’Kelly, M. E. (1989). Spatial interaction models: formulations and applications. Dordrecht: Kluwer Academic Publishers.
Freeman, J., Anderies, J. M., Torvinen, A., and Nelson, B. A. (2014). Crop specialization, exchange and robustness in a semi-arid environment. Hum. Ecol. 42 (2), 297–310. doi:10.1007/s10745-013-9638-0
Gauthier, N. E. (2019). Archaeological approaches to population growth and social interaction in semiarid environments: pattern, process, and feedbacks. PhD thesis. Tempe: Arizona State University.
Halstead, P., and O’Shea, J. (1989). “Bad year economics: cultural responses to risk and uncertainty.” in: Introduction: cultural responses to risk and uncertainty. Editors P. Halstead and J. O’Shea. Cambridge: Cambridge University Press.
Hannachi, A., Jolliffe, I. T., and Stephenson, D. B. (2007). Empirical orthogonal functions and related techniques in atmospheric science: a review. Int. J. Climatol. 27, 1119–1152. doi:10.1002/joc.1499
Hao, Y., Armbruster, D., Cronk, L., and Athena Aktipis, C. (2015). Food sharing networks in lowland Nicaragua An application of the social relations model to count data. Evol. Hum. Behav. 36, 265–273. doi:10.1016/j.evolhumbehav.2014.12.003
Herrmann, S. M., Didan, K., Barreto-Munoz, A., and Crimmins, M. A. (2016). Divergent responses of vegetation cover in Southwestern US ecosystems to dry and wet years at different elevations. Environ. Res. Lett. 11 (12), 124005. doi:10.1088/1748-9326/11/12/124005
Herweijer, C., Seager, R., Cook, E. R., and Emile-Geay, J. (2007). North American droughts of the last millennium from a gridded network of tree-ring data. J. Clim. 20, 1353–1376. doi:10.1175/JCLI4042.1
Hill, J. B., Clark, J. J., Doelle, W. H., and Lyons, P. D. (2004). Prehistoric demography in the southwest: migration, coalescence, and hohokam population decline. Am. Antiq. 69, 689–716. doi:10.2307/4128444
Hill, J. B., Peeples, M. A., Huntley, D. L., and Carmack, H. J. (2015). Spatializing social network analysis in the late precontact U.S. southwest. Adv. Archaeol. Pract. 3, 63–77. doi:10.7183/2326-3768.3.1.63
Hodder, I. (1974). Regression analysis of some trade and marketing patterns. World Archaeol. 6 (2), 172–189. doi:10.1080/00438243.1974.9979600
Hu, Q., Feng, S., and Oglesby, R. J. (2011). Variations in North American summer precipitation driven by the Atlantic multidecadal oscillation. J. J. Clim. 24 (21), 5555–5570. doi:10.1175/2011JCLI4060.1
Janssen, M. A., Anderies, J. M., and Ostrom, E. (2007). Robustness of social-ecological systems to spatial and temporal variability. Soc. Nat. Res. 20, 307–322. doi:10.1080/08941920601161320
Johnson, J. R. (1990). Chumash social geography: a quantitative analysis. Proceedings of the Society of California Archaeology. 3, 149–173.
Kohler, T. A., and West, C. R. (1996). “The calculus of self-interest in the development of cooperation: sociopolitical development and risk among the Northern Anasazi,” in: Evolving complexity and environmental risk in the Prehistoric Southwest. Editors J. Tainter and B. B. Tainter. Reading, MA: Addison-Wesley.
Koster, J. M., and Leckie, G. (2014). Food sharing networks in lowland Nicaragua An application of the social relations model to count data. Social Networks. 38, 100–110. doi:10.1016/j.socnet.2014.02.002
Liu, Z., Bowen, G. J, and Welker, J. M. (2010). Atmospheric circulation is reflected in precipitation isotope gradients over the conterminous United States. J. Geophys. Res. Atmos. 115, 1–14. doi:10.1029/2010JD014175
Lebacher, M., Thurner, P. W., and Kauermann, G. (2018). International arms trade: a dynamic separable network model with heterogeneity components. arXiv preprint.
Lorenz, E. N. (1956). Empirical orthogonal functions and statistical weather prediction. Cambridge, MA: Statistical Forecast Project Report 1 Department of Meteorology MIT 49.
Malville, N. J. (2001). Long-distance transport of bulk goods in the pre-hispanic American southwest. J. Anthropol. Archaeol. 20 (2), 230–243. doi:10.1006/jaar.2000.0373
Masucci, A. P., Kalampokis, A., Eguíluz, V. M., and Hernández-García, E. (2011). Wikipedia information flow analysis reveals the scale-free architecture of the semantic space. PLoS One 6 (2), e17333. doi:10.1371/journal.pone.0017333
McCabe, G. J., Palecki, M. A., and Betancourt, J. L. (2004). Pacific and Atlantic Ocean influences on multidecadal drought frequency in the United States. Proc. Natl. Acad. Sci. U.S.A. 101 (12), 4136–4141. doi:10.1073/pnas.0306738101
McCabe, G. J., and Dettinger, M. D. (1999). Decadal variations in the strength of ENSO teleconnections with precipitation in the western United States. Int. J. Climatol. 19 (13), 1399–1410.
Mesoudi, A. (2018). Migration, acculturation, and the maintenance of between-group cultural variation. PLoS One 13 (10), 1–23. doi:10.1371/journal.pone.0205573
Mills, B. J., Clark, J. J., Peeples, M. A., Haas, W. R., Roberts, J. M., Hill, J. B., et al. (2013a). Transformation of social networks in the late pre-Hispanic US Southwest. Proc. Natl. Acad. Sci. U.S.A. 110 (15), 5785–5790. doi:10.1073/pnas.1219966110
Mills, B. J., Roberts, J. M., Clark, J. J., Haas, W. R., Huntley, D., Peeples, M. A., et al. (2013b). “The dynamics of social networks in the late prehispanic US southwest,” in Network analysis in archaeology: new approaches to regional interaction. (Oxford : Oxford University Press), Chap. 8, 181–202.
Mills, B. J., Peeples, M. A., Haas, W. R., Borck, L., Clark, J. J., and Roberts, J. M. (2015). Multiscalar perspectives on social networks in the late prehispanic southwest. Am. Antiq. 80 (1), 3–24. doi:10.7183/0002-7316.79.4.3
Murphy, M. A., Dezzani, R., Pilliod, D. S., and Storfer, A. (2010). Landscape genetics of high mountain frog metapopulations. Mol. Ecol. 19 (17), 3634–3649. doi:10.1111/j.1365-294X.2010.04723.x
Nelson, M. C., Kintigh, K., Abbott, D. R., and Anderies, J. M. (2010). The cross-scale interplay between social and biophysical context and the vulnerability of irrigation-dependent societies: archaeology’s longterm perspective. Ecol. Soc. 15 (3). doi:10.5751/es-03389-150331
Nolin, D. A. (2010). Food-Sharing networks in lamalera, Indonesia: reciprocity, kinship, and distance. Hum. Nat. 21 (3), 243–268. doi:10.1007/s12110-010-9091-3
North, G. R., Bell, T. L., and Cahalan, R. F. (1982). Sampling errors in the estimation of empirical orthogonal funtions. Mon. Weather Rev. 110 (7), 699–706.
Paliou, E., and Bevan, A. (2016). Evolving settlement patterns, spatial interaction and the socio-political organisation of late Prepalatial south-central Crete. J. Anthropol. Archaeol. 42, 184–197. doi:10.1016/j.jaa.2016.04.006
Peeples, M. A., and Haas, W. R. (2013). Brokerage and social capital in the prehispanic U.S. Southwest. Am. Anthropol. 115 (2), 232–247. doi:10.1111/aman.12006
Pingel, T. J. (2010). Modeling slope as a contributor to route selection in mountainous areas. Cartogr. Geogr. Inf. Sci. 37 (2), 137–148. doi:10.1559/152304010791232163
Rautman, A. E. (1993). Resource variability, risk, and the structure of social networks: an example from the prehistoric southwest. Am. Antiq. 58 (3), 403–424. doi:10.2307/282104
Richman, M. B. (1986). Review article: rotation of principal components. J. Climatol. 6, 293–335. doi:10.1002/joc.3370060305
Routson, C. C., Woodhouse, C. A., Overpeck, J. T., Betancourt, J. L., and McKay, N. P. (2016). Teleconnected ocean forcing of Western North American droughts and pluvials during the last millennium. Quat. Sci. Rev. 146, 238–250. doi:10.1016/j.quascirev.2016.06.017
Ryu, J. H., Svoboda, M. D., Lenters, J. D., Tadesse, T., and Knutson, C. L. (2010). Potential extents for ENSO-driven hydrologic drought forecasts in the United States. Climatic Change 101 (3), 575–597. doi:10.1007/s10584-009-9705-0
Schnegg, M. (2015). Reciprocity on demand : sharing and exchanging food in northwestern Namibia. Hum. Nat. 26 (3), 313–330. doi:10.1007/s12110-015-9236-5
Seager, R., and Hoerling, M. (2014). Atmosphere and ocean origins of North American droughts. J. Clim. 27 (12), 4581–4606. doi:10.1175/jcli-d-13-00329.1
Sen, A., and Smith, T. E. (1995). Gravity models of spatial interaction behavior. Oxford: Springer-Verlag.
Sheppard, P. R., Comrie, A. C., Packin, G. D., Angersbach, K., and Hughes, M. K. (2002). The climate of the US southwest. Clim. Res. 21, 219–238. doi:10.3354/cr021219
Shirk, A. J., Landguth, E. L., and Cushman, S. A. (2018). A comparison of regression methods for model selection in individual-based landscape genetic analysis. Mol. Ecol. Resour. 18 (1), 55–67. doi:10.1111/1755-0998.12709
Steiger, N. J., Smerdon, J. E., Cook, E. R., and Cook, B. I. (2018). A reconstruction of global hydroclimate and dynamical variables over the Common Era. Sci. Data 5, 1–15. doi:10.1038/sdata.2018.86
Stillman, P. E., Wilson, J. D., Denny, M. J., Desmarais, B. A., Bhamidi, S., Cranmer, S. J., et al. (2017). Statistical modeling of the default mode brain network reveals a segregated highway structure. Sci. Rep. 7 (1), 11694–11714. doi:10.1038/s41598-017-09896-6
Strawhacker, C., Snitker, G., Peeples, M. A., Kinzig, A. P., Kintigh, K. W., Bocinsky, K., et al. (2020). A landscape perspective on climate-driven risks to food security: exploring the relationship between climate and social transformation in the prehispanic U.S. Southwest. Am. Antiq. 85 (3), 427–451. doi:10.1017/aaq.2020.35
Strawhacker, C., Snitker, G., Spielmann, K. A., Wasiolek, M., Sandor, J., Kinzig, A., et al. (2017). “Risk landscapes and domesticated landscapes: food Security in the Salinas province,” in Landscapes of social transformation in the salinas province and the eastern pueblo world. Editor K. A. Spielmann. (Tucson: University of Arizona Press), 103–118.
Taliaferro, M. S., Schriever, B. A., and Shackley, M. S. (2010). Obsidian procurement, least cost path analysis, and social interaction in the Mimbres area of southwestern New Mexico. J. Archaeol. Sci. 37 (3), 536–548. doi:10.1016/j.jas.2009.10.018
Tobler, W., and Wineburg, S. (1971). A cappadocian speculation. Nature 231 (5297), 39–41. doi:10.1038/231039a0
van Etten, J. (2017). R package gdistance: distances and routes on geographical grids. J. Stat. Software 76 (13), 1–21. doi:10.18637/jss.v076.i13
Varien, M. (1999). Sedentism and mobility in a social landscape: mesa Verde and beyond. Tucson: University of Arizona Press.
Verdery, A. M., Entwisle, B., Faust, K., and Rindfuss, R. R. (2012). Social and spatial networks: kinship distance and dwelling unit proximity in rural Thailand. Soc. Networks 34 (1), 112–127. doi:10.1016/j.socnet.2011.04.003
Vicente-Serrano, S. M., Beguería, S., López-Moreno, J. I., Angulo, M., and El Kenawy, A. (2010). A new global 0.5 gridded dataset (19012006) of a multiscalar drought index: comparison with current drought index datasets based on the palmer drought severity index. J. Hydrometeorol. 11 (4), 1033–1043. doi:10.1175/2010jhm1224.1
Weiss, B. (1982). The decline of Late Bronze Age civilization as a possible response to climatic change. Climatic Change 4 (2), 173–198.
Wilson, A. (2008). Boltzmann, Lotka and Volterra and spatial structural evolution: an integrated methodology for some dynamical systems. J. R. Soc. Interface. 5 (25), 865–871. doi:10.1098/rsif.2007.1288
Wilson, A. (1971). Family of spatial interaction models, and associated developments. Environ. Plann. 3 (1), 1–32. doi:10.1068/a030001
Keywords: drought patterns, archaeological networks, spatial interaction model, empirical orthogonal functions, climate risk management
Citation: Gauthier N (2021) Hydroclimate Variability Influenced Social Interaction in the Prehistoric American Southwest. Front. Earth Sci. 8:620856. doi: 10.3389/feart.2020.620856
Received: 27 October 2020; Accepted: 17 December 2020;
Published: 29 January 2021.
Edited by:
Jade D’Alpoim Guedes, University of California, San Diego, United StatesReviewed by:
Grant Snitker, University of Georgia, United StatesLi Wu, Anhui Normal University, China
Copyright © 2021 Gauthier. This is an open-access article distributed under the terms of the Creative Commons Attribution License (CC BY). The use, distribution or reproduction in other forums is permitted, provided the original author(s) and the copyright owner(s) are credited and that the original publication in this journal is cited, in accordance with accepted academic practice. No use, distribution or reproduction is permitted which does not comply with these terms.
*Correspondence: Nicolas Gauthier, bmdhdXRoaWVyQGFyaXpvbmEuZWR1