- 1PCBA Department of Civil Engineering, University of the Western Plains Ezequiel Zamora, San Carlos, Venezuela
- 2Satellite Image Analysis and Processing Laboratory, Institute of Atmospheric Sciences, Federal University of Alagoas, Maceio, Brazil
- 3Sisters of Mercy of the Americas, Climate & Sustainability, Fairfax, VA, United States
- 4Atmospheric Science Research Laboratory, Department of Physics, SRM Institute of Science and Technology, Kattankulathur, India
- 5Department of Physics, Tribhuvan University, Kathmandu, Nepal
- 6National Semi-Arid Institute, Ministry of Science, Technology, Innovations and Communications, Campina Grande, Brazil
The Amazon River Basin (ARB) plays an essential role in global climate regulation. Recent studies have revealed signs of increasing drought conditions in different parts of the basin. Although human activities have degraded large areas, little work has been done to assess whether prolonged drought may exacerbate land degradation. Among different methods for monitoring land degradation, the Sustainable Development Goal (SDG) indicator 15.3.1 adopted by the United Nations Convention to Combat Desertification (UNCCD) allows a comprehensive assessment of the impacts of land degradation due to its multi-factor nature and scalability. The aims of this study are twofold: 1) to assess the status of land degradation using the SDG indicator 15.3.1 from 2001 to 2020 in the Amazon basin; and 2) to explore the relationship between the detectability of land degradation using the UNCCD approach and long-term drought severity. The Standardized Precipitation Index (SPI), Standardized Precipitation-Evapotranspiration Index (SPEI), and self-calibrating Palmer Drought Severity Index (scPDSI) were used as drought indices. The results revealed 757,704 km2 (12.67% of the basin) as degraded land, which was reflected by a downward trend in land productivity dynamics followed by the combined downward trend in land productivity, Soil Organic Carbon (SOC) degradation, and land cover degradation. The largest land degradation hotspot was identified along the southwestern boundary of the Amazon River Basin. Furthermore, there was strong evidence that the detection of land degradation through SDG indicator 15.3.1 is sensitive to long-term drought, particularly when applied to rainfed croplands.
1 Introduction
The Amazon River Basin (ARB) is a hotspot of biodiversity and ecosystem function in South America (Ritter et al., 2019). It plays an essential role in the hydrological and carbon cycles at the regional and global scales through precipitation recycling and acting as a carbon sink (Marengo, 2005). These cycles are dependent on the spatiotemporal patterns of precipitation (Haghtalab et al., 2020). The moisture transport from the Atlantic Ocean partially controls the temporal variability of rainfall (Sorí et al., 2017). The precipitation is mainly modulated by sea surface temperature (SST) anomalies over the tropical Atlantic region and the El Niño-Southern Oscillation (ENSO) phenomenon (Satyamurty et al., 2013). Some of these mechanisms and their interactions may lead to drought events in different parts of the Amazon basin (Marengo et al., 2013). On the other hand, the feedback between the climate and the tropical rainforest influences precipitation at the local and regional scales (Bagley et al., 2014) and thus must be considered as part of any drought assessments on the vegetation.
Drought originates from a deficiency of precipitation over a specific region. The temporal persistence can affect different sectors as this anomaly propagates through the hydrological cycle, so the scientific literature recognizes four major types: meteorological, hydrological, agricultural, and socioeconomic drought (Mishra and Singh, 2010). Drought has various key features (i.e., intensity, spatial extent, timing, and duration), which can be derived from numerical indices based on observed measurements, remote sensing, or modeled data. Nowadays, the increased availability of satellite missions dedicated to Earth observation has allowed the development of satellite-based indices to monitor drought conditions in large regions with limited access to observational data (Ahmadalipour et al., 2017), such as the Amazon basin. Unlike ground-based drought indices, these indices provide comprehensive spatial coverage, long time series, and data completeness. Their attributes have motivated the progressive adaptation of ground-based drought indices to satellite data to overcome their point-in-space nature (Zargar et al., 2011). The Standardized Precipitation Index (SPI, McKee et al., 1993), Standardized Precipitation Evapotranspiration Index (SPEI, Vicente-Serrano et al., 2010), and self-calibrating Palmer Drought Severity Index (scPDSI, Wells et al., 2004) represent examples of drought indices that take advantage of satellite data.
The SPI has been widely used to monitor meteorological droughts due to its multi-scalar nature and capacity to identify different drought types. It has also been endorsed by the World Meteorological Organization (WMO) in preference to others of a similar character (Hayes et al., 2011). The SPI considers the cumulative precipitation at different time scales, is fitted with a probability distribution, and is then transformed to the normal distribution while considering a baseline period (Zargar et al., 2011). There is a wide variety of satellite-based gridded precipitation products. However, the Climate Hazards Group InfraRed Precipitation with Stations (CHIRPS) offers significant advantages because it combines satellite and rain gauge information (Funk et al., 2015). CHIRPS also has data availability on a longer temporal scale (1981–present), high spatial resolution (0.05°), low latency (15°days), and different temporal resolutions (e.g., daily, pentadal, dekadal, monthly, and annual). Moreover, it has been assessed through comparisons to gauge measurements in the Amazon basin, exhibiting an acceptable performance on a monthly time scale (da Motta Paca et al., 2020; Haghtalab et al., 2020). Therefore, the CHIRPS-based SPI is ideal for meteorological drought monitoring throughout the Amazon basin (Oliveira-Júnior et al., 2021).
The calculation of the SPEI involves the same procedure as the SPI, except that it considers precipitation (P) minus potential evapotranspiration (PET) rather than precipitation alone. For this reason, the SPEI is better suited for evaluating meteorological drought impacts under global climate change (Zargar et al., 2011).
Unlike the SPI and SPEI, the scPDSI is more appropriate for identifying agricultural droughts. It has been developed based on a two-layer soil moisture balance in the rooting zone, whose inputs are the soil water capacity, precipitation, and PET. The processing steps include a self-calibrating phase where a set of empirical constants are replaced with dynamically calculated values considering the characteristics of the local climate (van der Schrier et al., 2013). The positive values of the scPDSI, SPI, and SPEI represent wetter than average conditions, while negative values denote drier than average conditions.
There are currently various free global SPEI, SPI, and scPDSI products available, including the Global SPEI Database (Vicente-Serrano et al., 2010) and global scPDSI (van der Schrier et al., 2013). The key benefit of the SPI, SPEI, and scPDSI derived from satellite data is that these are comparable across space and time because they use a similar drought category classification (Zargar et al., 2011). Despite having so many drought indices, there is no universally accepted index to assess drought. This paradox is mainly due to the complexity of the drought phenomenon and the attributes of each drought index (Mishra and Singh, 2010). As mentioned earlier, the SPEI and SPI show better performance than the scPDSI in detecting meteorological drought. However, the scPDSI is a more reliable indicator of drought-induced decline in plant photosynthetic activity (Keyantash and Dracup, 2002; van der Schrier et al., 2013). This does not imply that one is better than another but rather that each one has a specific scope of application. Obviously, a comprehensive drought assessment must integrate several drought indices to capture underlying processes that are not apparent due to complex water-vegetation-atmosphere interactions (Hao and Singh, 2015). For these reasons, the SPI, SPEI, and scPDSI were adopted for drought characterization in this study.
Drought varies significantly in duration, spatial extent, and intensity in the Amazon basin (Paredes-Trejo et al., 2021). For instance, the Amazon basin suffered two unprecedented droughts in 2005 and 2010. Both events caused massive forestry losses through wildfires (Panisset et al., 2018). Recent studies have suggested that the high deforestation rate observed from satellite images in recent years could have exacerbated their impacts (Bullock et al., 2020). However, it is still unclear whether a concomitant effect between the drought and human activities may be leading to accelerated land degradation in the Amazon basin.
Land degradation is a serious environmental problem at present. The United Nations Convention to Combat Desertification (UNCCD) defines this term as the reduction of the biological or economic productivity of the land because of a combination of pressures, including climate variations and human activities (Diallo, 2008). Several satellite-based approaches have been applied to analyzing land degradation in the Amazon basin (Lu et al., 2007). Most of them assess the long-term land degradation, exploiting the strong relationship between the in-situ Net Primary Productivity (NPP) measurements and a reflectance-based vegetation index, such as the Normalized Difference Vegetation Index (NDVI) (Li et al., 2021; Rotllan-Puig et al., 2021). The challenge of this type of method is to discriminate between the biogenic (i.e., insect attacks), climate (i.e., drought), and anthropogenic (i.e., deforestation) disturbances (Easdale et al., 2018). In the Amazon basin, it is well known that extreme droughts significantly decreased NDVI values in grasslands and pastures (Barbosa et al., 2015).
The determination of transitions from one land cover and land use (LCLU) class to another for two specified dates using satellite-based land cover maps has been used to evaluate land degradation in the Amazon basin (Souza et al., 2020). Among the state-of-the-art LCLU maps for this basin, those developed by the MapBiomas network using Google Earth Engine have the highest accuracy (Souza et al., 2020). Nevertheless, this approach is not entirely suitable for degradation detection. The adopted classification system and uncertainties in the LCLU map may miss relevant transitions for detecting land degradation (Neves et al., 2020).
The UNCCD adopted a novel approach for land degradation assessment through satellite-based data in 2021 called the Sustainable Development Goal (SDG) indicator 15.3.1. This indicator expresses the proportion of land degraded over the total land area for a baseline and subsequent reporting periods. The calculation of the SDG indicator 15.3.1 is based on three sub-indicators: 1) trend in land cover, which identifies where degradation occurs through land cover change; 2) trend in land productivity, which estimates the change in long-term land productivity derived from the NDVI-based annual productivity; and 3) trend in soil organic carbon (SOC) stocks, which estimates the change in the SOC. Three metrics allow us to measure land productivity: 1) trend, which measures the trajectory of productivity change; 2) state, which compares the current productivity level against historical registers of productivity; and 3) performance, which indicates the level of local productivity compared with other areas with exhibiting similar land productivity potential in the targeted region. The three sub-indicators are integrated using a one-out-all-out (1OAO) method. The premise is that land degradation occurs when any sub-indicator shows degradation (Sims et al., 2019).
Each approach mentioned above has strengths, weaknesses, and limitations, but the UNCCD approach attempts to provide a robust procedure for monitoring land degradation (Prince, 2019). Since that studies assessing land degradation through SDG indicator 15.3.1 across the Amazon basin are currently lacking, the key contributions of this paper were to assess the land degradation using that indicator and the relationship between the detectability of land degradation and long-term drought severity in the entire basin. The premise is that the delayed response of vegetation to drought could be misunderstood by the SDG indicator 15.3.1 as a degradation process. In this context, the drought severity derived from SPI, SPEI, or scPDSI is taken as a proxy for long-term drought conditions.
2 Data and methods
2.1 Study area
The study was conducted in the Amazon River Basin (ARB), located between 5.3°N and 20.1°S and 49.2–79.5°W (Figure 1). At Óbidos, a city near the basin’s outlet, the mainstream drains 4,670,000 km2 with an annual mean flow of 163,000 m3/s (Nobre et al., 2009). The elevation ranges from near 0 m above sea level (a.s.l.) in the eastern lowlands to more than 3,000 m a.s.l. In the higher Andes, with an estimated 24 million inhabitants (Tritsch and Le Tourneau, 2016). The forest is the predominant land cover, covering about 83% of its entire surface (Ometto et al., 2016). The spatiotemporal precipitation variability is modulated by the moisture transport from the Atlantic Ocean, which is controlled by SST anomalies over the tropical Atlantic region and the ENSO phenomenon (Marengo, 2005). Mean annual precipitation ranges from around 1,500 to >2,500 mm. The rainy season is mainly concentrated from March to May in the northern portion of the Amazon basin, while the dry season co-occurs in the southern portion; this pattern is reversed during the northern region’s dry season (i.e., December to February) (Marengo, 2004). While precipitation varies seasonally and spatially in different parts of the Amazon basin, the temperature regime is more stable; the annual average air temperature generally remains between 24°C and 26°C (Kayano et al., 2017). The Köeppen-Geiger climate zones Af, Am, and BWh are dominant in about 93% of the basin (Beck et al., 2018).
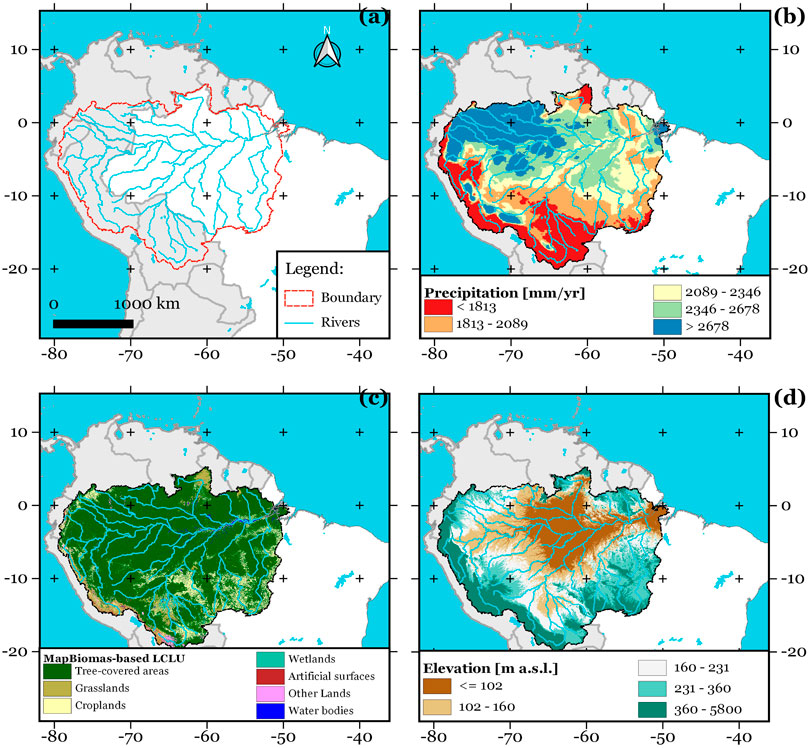
FIGURE 1. The study area. Map of (A) the hydrographic network; (B) mean annual precipitation from the CHIRPS dataset for the period 2000–2018; (C) LCLU from MapBiomas at 2020 aggregated to the UNCCD classes (Sims et al., 2019); (D) terrain elevation according to the Shuttle Radar Topographic Mission (Jarvis et al., 2008).
2.2 Datasets
2.2.1 CHIRPS-based standardized precipitation index
The CHIRPS dataset is considered a reliable dataset for drought studies in the Amazon basin, as confirmed in previous studies (da Motta Paca et al., 2020; Haghtalab et al., 2020; Oliveira-Júnior et al., 2021). Therefore, the SPI derived from the gridded CHIRPS precipitation product (available at https://bit.ly/3hoyFWn; version 2; spatial resolution: 0.05°) at a 12-month time scale (hereafter referred to as SPI12) was used to assess drought conditions in time over the Amazon basin. In this context, the SPI12 for December 2001 considers the total P from January 2001 to December 2001. The rationale behind this time scale is to focus attention on long-term drought events to ensure consistency with the UNCCD approach used to assess land degradation and drought (Sims et al., 2019; Barker et al., 2021). A reference period of 1981–2010 was used to calculate the SPI12. The SPI12 was calculated using the R package SPEI (version 1.7) developed by Beguería et al. (2014); further details can be found in McKee et al. (1993).
2.2.2 Standardized precipitation evapotranspiration index
In order to assess long-term atmospheric dryness in the Amazon basin, the SPEI at 0.5° spatial resolution and a 12-month time scale (hereafter referred to as SPEI12) was extracted from the global gridded SPEI database (available at https://bit.ly/3C5r0WH; version 2.6). This database is maintained by the Spanish National Research Council (CSIC), covering January 1901 to December 2018 at several time scales. The CSIC uses P and PET from the Climate Research Unit Time Series (CRU TS) dataset (version 4.03) developed by the University of East Anglia to calculate SPEI12. PET is derived from the Food and Agriculture Organization of the United Nations-56 (FAO-56) Penman-Monteith approach (Beguería et al., 2014). The CSIC-based SPEI12 has been used in some climate studies in the Amazon basin, showing a low percentage of uncertainty compared to ground-based benchmark sites (Awange et al., 2016). Other dataset details are provided in Vicente-Serrano et al. (2010).
2.2.3 Self-calibrating palmer drought severity index
The impact of drought conditions on the root-zone soil moisture was assessed through the global scPDSI dataset (available at https://bit.ly/3C7Uh32; version 4.05; spatial resolution: 0.5°). Similar to SPEI, P and PET from the CRU TS dataset (version 4.05) are used to calculate scPDSI. Nevertheless, different from the SPEI and SPI, this drought index is based on a monthly time scale. The scPDSI has proved to be a reliable index to assess the soil moisture stress suffered by plants during the growing season in the Amazon basin (Lewis et al., 2011; Jiménez-Muñoz et al., 2016), which was the primary motivation for choosing the scPDSI. Further technical details about the scPDSI are presented by Wells et al. (2004).
2.2.4 Land cover and land use maps
In order to assess the land cover change in the Amazon basin, the MapBiomas LCLU dataset (available at https://bit.ly/3hL2Ib8; version 3; hereafter referred to as MapBiomas) at 30 m spatial resolution was used. These maps are derived from satellite images from the Landsat program. For the first level, they provide six LCLU classes (i.e., forest, non-forest formation, farming, non-vegetated area, water, and not observed) compatible with the Food and Agriculture Organization (FAO)’s Land Cover Classification System (LCCS) hierarchical structure (Souza et al., 2020). Its high overall accuracy in the Brazilian Amazon (about 95%) verified in recent studies (Anderson et al., 2018) has been the primary motivation for using it in this study. More details about the uncertainty of the product can be found in Neves et al. (2020).
2.2.5 Normalized difference vegetation index
The land productivity change in the Amazon basin was assessed through the 16-day MOD13Q1 NDVI product (available at https://lpdaac.usgs.gov; collection 6; hereafter referred to as NDVI) at 250 m spatial resolution derived from the Moderate Resolution Imaging Spectrometer (MODIS) sensor aboard the Terra platform. The NDVI has been the most widely used spectral index for vegetation monitoring in the Amazon basin because it correlates well with several biophysical vegetation parameters such as green biomass and NPP (Atkinson et al., 2011; Barbosa et al., 2015). Furthermore, the UNCCD recommends the NDVI as the standard vegetation index for assessing land productivity. As such, this study chose the NDVI as an empirical proxy for NPP. More technical details of the MODIS-based NDVI are outlined in Friedl et al. (2010).
2.2.6 Soil organic carbon stock
The long-term change of SOC stocks in the Amazon basin was inferred using the global SoilGrids dataset at 250 m spatial resolution (available at https://soilgrids.org/; version 2.0). The SoilGrids provides SOC stock estimates for 0–30 cm with pixel-scale spatial uncertainty (Hengl et al., 2017), making it a suitable alternative in regions without a SOC database like the Amazon basin (Sims et al., 2019).
2.3 Methodology
2.3.1 Land degradation
The geographic extent of degradation is based on the UNCCD approach for measuring land degradation (Sims et al., 2019). The study period encompassed 2001 to 2020, which was chosen for two reasons. First, the MOD13Q1 product provides NDVI time series from 2001 onwards. Second, it allows attention to be focused on long-term changes in NPP induced by drought.
The first step (i.e., land productivity) was to compute the annual integrals of NDVI time series during each year’s growing season at the pixel level. They are then used to calculate three measures of change: trajectory, state, and performance for each pixel. The trajectory is calculated by fitting a linear regression to the annually integrated values of NDVI. The trend slope and the statistical significance of said slope are determined through the Thiel-Sen median (Hu et al., 2020) and the Mann-Kendall non-parametric test (Yue et al., 2002). Positive trend slopes at a level of 95% confidence indicate a trend of increasing productivity (i.e., improvement), and significant negative values indicate decreasing productivity (i.e., degradation). In contrast, non-significant trends indicate a stable condition. The state is calculated by defining the periods 2001–2017 and 2018–2020 as the baseline and comparison. The mean and standard deviation are computed for both periods, and then a t-test for the mean difference is applied to compare the 2018–2020 productivity level to the 2001–2017 measurements. This metric compares land productivity in recent years to historical observations in a given area (Sims et al., 2019). Positive differences at a level of 95% confidence indicate a state of increasing productivity (i.e., improvement), significant negative differences indicate decreasing productivity (i.e., degradation), while non-significant differences indicate a stable condition. The performance is calculated by comparing the mean of the annually summed NDVI against the 90th percentile for other pixels with the same Land Cover/Ecosystem Functional Unit (LCEU) throughout the study area for the 2001–2020 period. When the ratio is less than 0.5, that pixel is considered degraded; otherwise, it is not considered degraded. The trajectory, state, and performance metrics are combined to determine degradation at the land productivity sub-indicator level, as indicated in Supplementary Figure S1. The trajectory, state, and performance metrics outcomes are shown as categorical maps (i.e., degraded/stable/improvement) at a 250 m pixel resolution.
The second step (i.e., land cover change) was to assess changes in MapBiomas maps for 2001 (i.e., baseline year) and 2020 (i.e., target year). Both maps were reclassified to the seven UNCCD land cover classes (i.e., tree-covered, grassland, cropland, wetland, artificial, other land, and water body). Supplementary Figure S2 shows how the MapBiomas legend was harmonized with UNCCD classes. Once the MapBiomas maps were reclassified, an analysis of LCLU change was performed using the transition matrix shown in Supplementary Figure S3 to identify the key degradation processes.
The third step (i.e., SOC stock) was to assess changes in SOC over the 2001–2020 period. A combined land cover/SOC approach is applied based on carbon conversion coefficients proposed by the 2006 IPCC Guidelines for National Greenhouse Gas Inventories (IPCC, 2006). This methodology relates the SOC stock from the SoilGrids dataset to environmental and management factors, with different methods and defaults for mineral and organic soils. Pixels with a SOC change ≤−10% are considered to be degraded; if SOC change ≥10%, it is identified as an improvement; while outside that range is stable (Sims et al., 2019). The outcomes of the SOC stock and land cover change sub-indicators are categorical maps at 250 m pixel resolution with three classes: degraded, stable, and improvement. For more information on the rationale behind this approach, see Sims et al. (2019).
The fourth step was to integrate the three sub-indicators described above to generate the SDG indicator 15.3.1, following the 1OAO rule. If one sub-indicator shows degradation in a pixel, it is considered degraded (see Supplementary Figure S4). This method generates a categorical map (i.e., degradation/stable/improvement; hereafter referred to as land degradation map) with a spatial resolution of 250 m. All methods were implemented through Trends. Earth (available at https://bit.ly/3JGmb94; version 1.99.8) under the QGIS software. Supplementary Figures S5, S6 summarize the process used for each sub-indicator.
The contribution of the sub-indicators to the land degradation map was explored through cross-tabulations applied to a stack of raster layers. Cross-tabulations were implemented using the R package raster (version 3.5.15; Hijmans and Van Etten, 2019) to count the number of combined sub-indicators at the pixel level.
2.3.2 Characterization of drought
The SPI12 and SPEI12 time series for each December from 2001 to 2020 were adopted to characterize the meteorological drought. The underlying assumption behind this defined time scale and month is that an annual aggregation can capture long-term extreme drought conditions (Paredes-Trejo et al., 2021). This time scale has also been used in previous studies to evaluate the impact of drought on different types of vegetation (Panisset et al., 2018), changes in land productivity (Faiz et al., 2020), and SOC stocks (Metcalfe et al., 2010; Liu et al., 2014). The annual mean scPDSI from 2001 to 2020 characterized the agricultural drought.
For this study, a dry spell starts when SPI12 (SPEI12 or scPDSI) ≤−1.00 for at least two consecutive calendar months, and it ends when SPI12 (SPEI12 or scPDSI) >−1.00 at a given pixel following the onset of a drought. Four drought intensity classes were adopted to measure the change in the extent of drought conditions over time. Classes include mild drought (−1 to 0), moderate drought (−1.5 to −1), severe drought (−2 to −1.5), and extreme drought (≤−2) (McKee et al., 1993; Barker et al., 2021). The total area exposed to drought clustered by drought class and month was derived from counts of the number of pixels.
The long-term drought severity per pixel was also assessed. The drought severity was computed by summating the negative SPI12 (SPEI12 or scPDSI) values during the time window under study, the result from which was converted to an absolute value. The following step was to resample the three drought severity maps at 250 m using the bilinear interpolation method to match their spatial resolution with the land degradation map. Finally, to ensure the comparability and consistency between the drought severity maps, their values were scaled from 0 to 1 at the pixel level, where 0 and 1 represent the lowest and highest drought severity values, respectively. Supplementary Figure S7 shows the process used for drought characterization.
2.3.3 Comparison between the drought severity and land degradation
The higher the value of drought severity, the more significant the potential impacts on the land productivity and SOC stock. Based on this premise, the drought severity maps based on SPI12, SPEI12, and scPDSI were compared to the trajectory, state, performance, and land degradation maps. This choice was based on their dependence on the annualized NDVI. Since these maps have a large number of pixels (about 9.6 × 107), a stratified sampling with three strata (i.e., degradation, stable, and improvement) was applied. The randomly selected pixels were set to 5,000 pixels for each stratum (hereafter referred to as benchmark sites) to guarantee that the sample is representative and balanced (Levine and Wilks, 2000). This procedure was implemented using the sampling R package version 2.90 (Tillé et al., 2016).
The non-parametric Kruskal-Wallis test was conducted to explore if degraded/not degraded pixels show significant differences in drought severity. The statistical significance threshold to identify robust evidence that the detection of degraded pixels is affected by drought was 5% (i.e., p-value ≤0.05). The non-parametric Dunn’s test with adjusted p-values via the Bonferroni method (Ma, 2019) further refined the comparison. These statistical tests were chosen because they do not require the assumption of normality in the stratified data (Boschetti et al., 2006). Both non-parametric tests were implemented with the R package stats (version 4.1.2) developed by R Core Team. The statistical tests and their simplified procedure are outlined in Table 1.
3 Results
3.1 Land degradation in the Amazon basin
The trajectory of productivity change between 2001 and 2020 showed 358,507 km2 (about 6% of the basin; Figure 2A) under land degradation in the Amazon basin. The most significant areas of land degradation occurred in the Brazilian states Pará, Mato Grosso, and Rondônia (up to 202,000 km2), and Santa Cruz in Bolivia (36,190 km2). In addition, when the productivity level of the last 3 years was compared with the previous historical observations of the productivity of the land for each pixel (i.e., the state metric), 500,583 km2 was identified as degraded land (about 8% of the Amazon basin; Figure 2B). In this case, the extent of degraded land dominated three Brazilian states: Pará, Mato Grosso, and Amazonas (221,855 km2). On the other hand, around 0.66% of the basin (39,086 km2) experienced a declining local productivity trend compared to other regions with similar productivity potential (i.e., the performance metric). This situation was particularly relevant in the Roraima state in Brazil and the La Paz and Potosí states in Bolivia (Figure 2C). However, to have a complete picture of land degradation processes in the basin (see Supplementary Figure S8), these metrics must be integrated through the land productivity land sub-indicator (Prince, 2019).
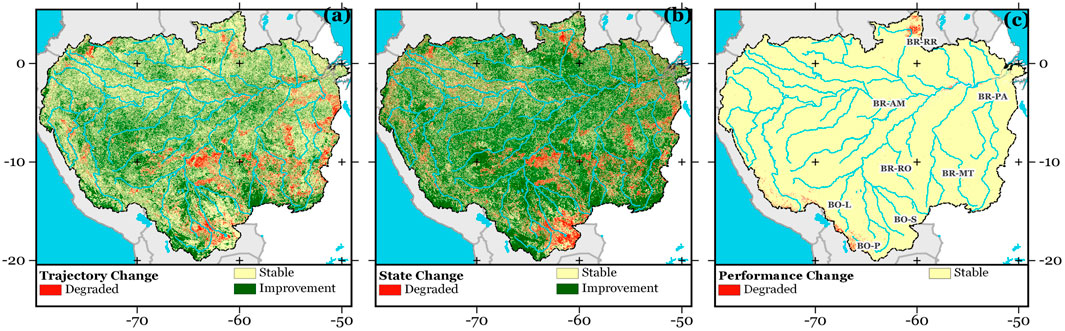
FIGURE 2. Map of the metrics (A) trajectory; (B) state; and (C) performance in the Amazon basin for the 2001–2020 period. The location of the Brazilian states is shown as BR-RR, Roraima; BR-AM, Amazonas; BR-PA, Pará; BR-MT, Mato Grosso; and BR-RO, Rondônia. The location of the Bolivian states is shown as BO-L, La Paz; BO-S, Santa Cruz; and BO-P, Potosí.
The land productivity sub-indicator revealed 353,694 km2 of land (about 6% of the basin; Figure 3A), where the productivity levels declined between 2001 and 2020. In addition, there were 262,779 km2 and 10,342 km2 with signs of moderate degradation and potentially stressed (4.63% of the basin), respectively. According to this sub-indicator, the most extensive degraded land coverage occurred in Pará (76,567 km2), Mato Grosso (74,136 km2), and Rondônia (50,619 km2), followed by Santa Cruz with 36,100 km2 in Bolivia. A detailed review of this indicator revealed that those areas with declining land productivity show a degraded state (43% of the affected area), a degraded trajectory (20% of the affected area), or both land conditions (36% of the affected area).
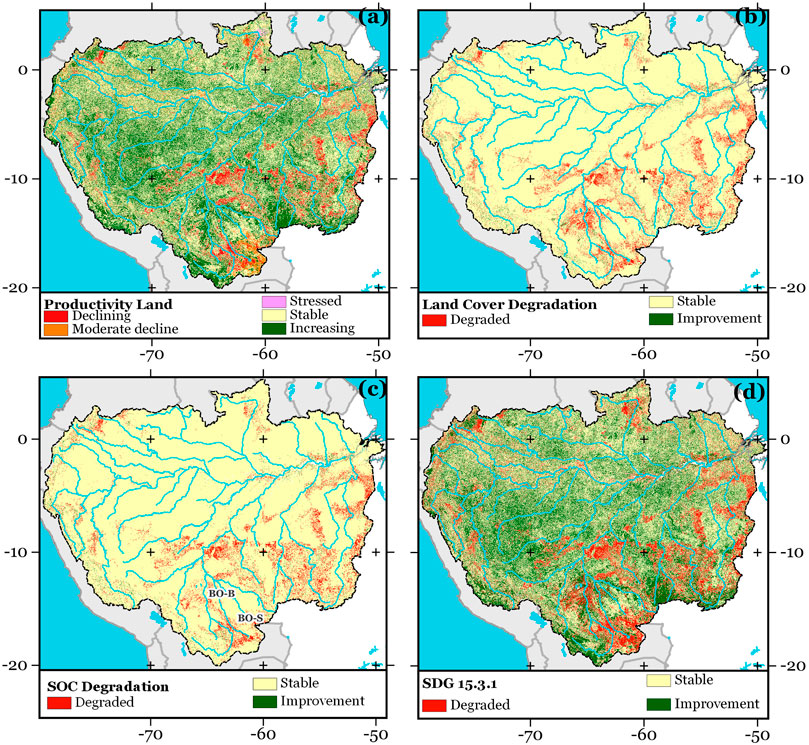
FIGURE 3. Map of the sub-indicators (A) land productivity; (B) land cover degradation; and (C) SOC degradation; together with (D) the SDG indicator 15.3.1 for the period 2001–2020. The location of the Bolivian states is shown as BO-B, El Beni; and BO-S, Santa Cruz.
The land cover degradation sub-indicator showed 318,484 km2 (5.32% of the basin; Figure 3B) as degraded land for 2001–2020. Most of them are localized in Mato Grosso, Pará, and Rondônia (Brazil; up to 114,000 km2) and El Beni and Santa Cruz (up to 42,852 km2) in Bolivia (see Supplementary Figure S9). The main driver of land degradation identified through this sub-indicator was the transition from forest to cropland (260,356 km2; 4.36% of the basin), followed by wetlands to grasslands (19,142 km2; 0.32% of the basin).
The SOC degradation sub-indicator applied to the entire Amazon basin identified 292,348 km2 of degraded land (Figure 3C; 4.89% of the basin) for 2001–2020. In this case, Mato Grosso (51,470 km2), Pará (40,905 km2), Rondônia (23,019 km2) in Brazil, and Santa Cruz (818,943 km2) in Bolivia have shown the largest degradation hotspots (see Supplementary Figure S9).
An inspection of Figure 3D reveals the highest land degradation in the states Pará (138,033 km2), Mato Grosso (129,647 km2), Amazonas (75,317 km2), and Rondônia (66,458 km2). This indicator identified 757,704 km2 (12.67% of the basin), where land degradation was driven primarily by a declining trend of land productivity (57.46% of the degraded land) or a combined declining trend of land productivity, SOC degradation, and land cover degradation (25.02% of the degraded land) (see Supplementary Figure S10). These findings prove that multiple factors are behind the drivers of land degradation in those lands identified as degraded. Therefore, it is not adequate to categorize land as degraded using only one sub-indicator (e.g., land cover change) in the Amazon basin.
Despite the advantages of SDG indicator 15.3.1 over its sub-indicators for capturing the most relevant facets of land degradation (Sims et al., 2019), the effects of seasonal and inter-annual climate variability on NDVI should be taken into account during its interpretation, particularly in cases of multi-annual extreme events (Marengo et al., 2008b; Barbosa et al., 2015). For this reason, the long-term drought condition in the Amazon basin from 2001 to 2020 was analyzed.
3.2 Drought severity in the Amazon basin
The most remarkable geographical extent of drought conditions occurred in 2015/2016, when the area exposed to drought was greater than 70% of the basin. Supplementary Figures S11–S13 also show that large regions have been affected by recurrent droughts between 2001 and 2020. This result is consistent with recent studies where the intensification of drought conditions has been attributed to large-scale climate variability drivers acting over the basin, such as ENSO (Lima et al., 2014; Jiménez-Muñoz et al., 2016; Paredes-Trejo et al., 2021). Figure 4 shows the proportion of land under drought for each drought intensity class to the total land in the Amazon basin. The percentage of land under drought reflected by the SPI12, SPEI12, and scPDSI for each year has been relatively similar. Nevertheless, it should be noted that the scPDSI tended to offer higher values for the extreme drought class than that derived from SPI12 and SPEI12.
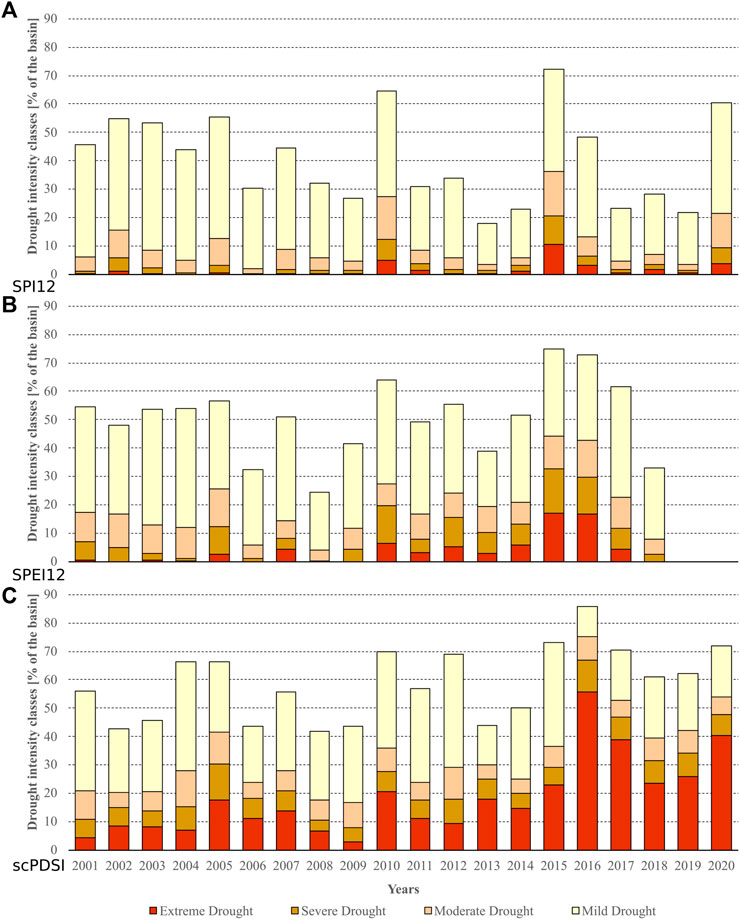
FIGURE 4. Percentage of the area under drought conditions for each drought intensity class according to the values of (A) SPI12; (B) SPEI; and (C) scPDSI in December over the Amazon basin during 2001–2020. The calculation of the area under drought conditions is based on the number of pixels within the drought intensity classes.
While drought intensity classes provide a robust way to compare the land exposed to drought in time (Figure 4), the drought severity maps identify regions where this hazard has been more serious and may become an important driver of land degradation. The spatial patterns of drought severity derived from SPI12, SPEI12, and scPDSI are shown in Figure 5. Areas showing high drought severity for the SPEI12 tended to coincide with areas with high drought severity for the scPDSI (Figures 5B,C). According to both maps, the most relevant drought hotspot covers most of Mato Grosso and southwestern Pará in Brazil. Furthermore, the three drought severity maps identified most of upper Caquetá in Colombia as a drought hotspot. Regardless of the drought index, drought hotspots are characterized by the dominance of croplands, followed by forests and grasslands (see Figure 1C).
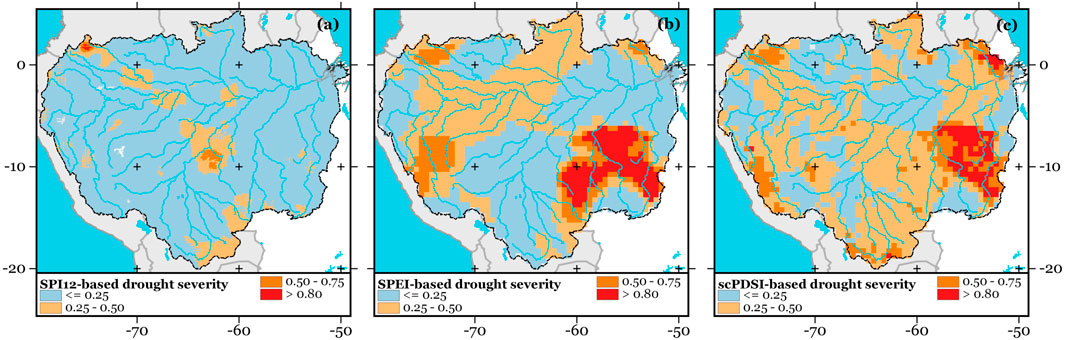
FIGURE 5. Map of the drought severity derived from (A) SPI12; (B) SPEI12; and (C) scPDSI in the Amazon basin for the 2001–2020 period. For comparison, the values of drought severity were scaled from 0 (minimum drought severity) to 1 (maximum drought severity) at the pixel level.
Some differences in the spatial distribution of drought severity are observed when the drought severity maps are compared (Figure 5). This discrepancy is mainly due to the fact that SPEI12 and scPDSI are better suited than SPI12 to identify the influence of soil water stress and atmospheric dryness on land productivity dynamics (Vicente-Serrano et al., 2015). Unlike the SPEI12 and scPDSI, the SPI12 only considers annualized precipitation. Consequently, the SPI12 cannot discriminate a greater increase in atmospheric evaporative demand due to a dryer and warmer atmosphere. In this sense, the absence of high drought severities over a given region in the map based on SPI12 does not necessarily represent an improvement in the productivity of the vegetation.
Several studies have demonstrated that the NDVI is sensitive to drought conditions in the Amazon basin (Anderson et al., 2007; Marengo et al., 2008a; Zhao et al., 2017), in addition to being strongly influenced by local factors, including the dominant land cover type (Barbosa et al., 2015). However, how the drought severity affects the detection of land degradation under the SDG indicator 15.3.1 approach is unknown. The next section further explores the concomitant effect of both physical processes using drought severity maps as benchmarks.
3.3 Influence of long-term drought on the detection of land degradation
Figure 6 shows the values of scPDSI-based drought severity of the trajectory, state, and performance metrics along with the SDG indicator 15.3.1 grouped by the land condition at the benchmark sites. For a balanced comparison, 5,000 sites correspond to degraded land, 5,000 sites to stable land, and the remaining 5,000 sites to improvement according to SDG indicator 15.3.1 (see Supplementary Figure S14). In all cases, sites categorized as degraded exhibited more drought severity than that observed for other land conditions (significant at 95% level). The same response was observed when the drought severity based on SPI12 was used as a reference (see Supplementary Figure S15). This fact shows that the detection of land degradation through the SDG indicator 15.3.1 depended partly on the severity of drought (Figure 4).
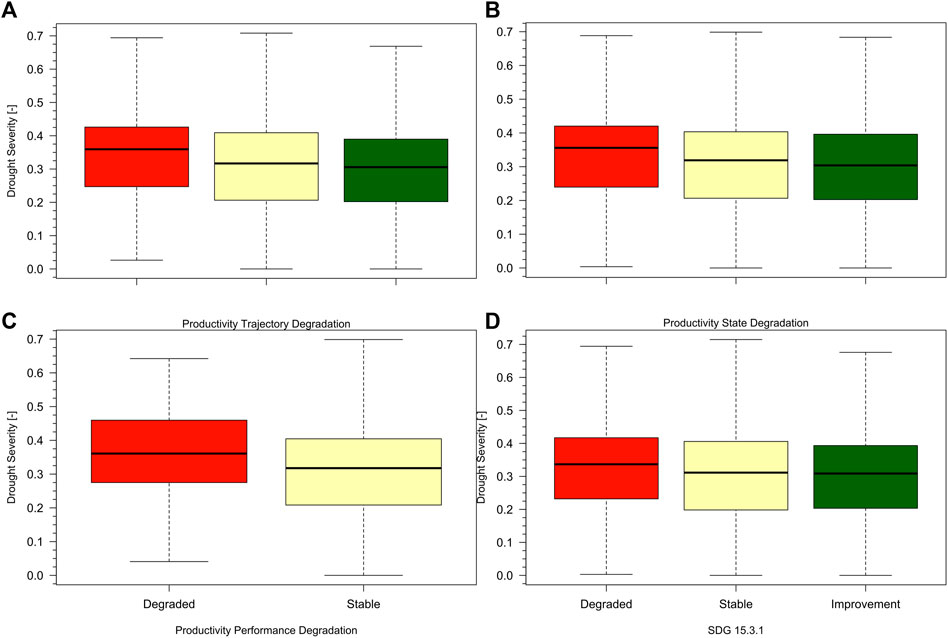
FIGURE 6. Box plots showing the scPDSI-based average drought severity for the (A) productivity trajectory degradation; (B) productivity state degradation; (C) productivity performance degradation; and (D) the SDG indicator 15.3.1 at benchmark sites randomly distributed in the Amazon basin for the period 2001–2020. For all panels, the differences in average drought severity between the degraded, stable, and improvement categories were statistically significant at the 95% level. The thick line represents the median, while the other horizontal lines of the box represent the maximum, upper quartile, lower quartile, and minimum. For clarity, the outliers were omitted.
When the SPEI12-based drought severity map was examined, a differential response in drought severity (significant at the 95% level) was only observed across state and performance metrics (see Supplementary Figure S16). These findings confirm a weak spatial coherence between the drought severity based on SPEI12 and land condition estimated via the SDG indicator 15.3.1. Even when drought conditions are present, SPEI12 cannot capture long-term changes in NPP through annualized NDVI at the pixel level (i.e., trajectory). In this context, the scPDSI is better suited to assess land degradation processes triggered by drought conditions in the Amazon basin.
4 Discussion
In this study, the physical processes behind land degradation and its relationship with drought variability were analyzed to better understand how the detection of degraded land through the SDG indicator 15.3.1 adopted by the UNCCD can be affected by long-term droughts in the Amazon basin.
4.1 Long-term land degradation in the Amazon basin
The land degradation processes mostly coincided with a downward trend in land productivity without a negative signal in other sub-indicators, followed in relative importance by the combined downward trend in land productivity, SOC degradation, and land cover degradation (Figure 7). The SDG indicator 15.3.1 revealed several land degradation hotspots in the Amazon basin between 2001 and 2020, most of which were located in the Brazilian states of Pará, Mato Grosso, Amazonas, and Rondônia. This result ties well with previous studies wherein such land degradation hotspots were identified (Anderson et al., 2018; Arima et al., 2021; Marengo et al., 2022).
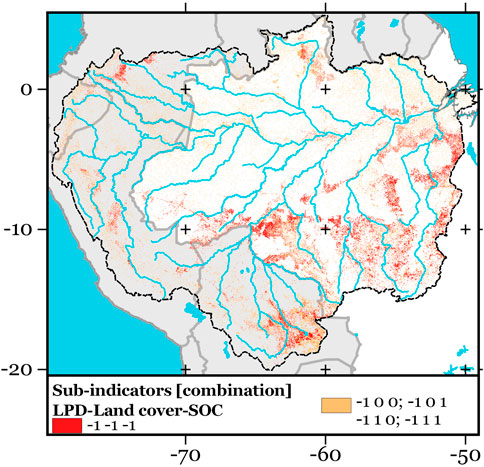
FIGURE 7. Spatial pattern of dominant combined sub-indicators of SDG indicator 15.3.1 in the Amazon basin for 2001–2020. Where “−1” depicts Degraded, “0” is Stable, and “1” is Improvement for each sub-indicator. All sub-indicators combinations are listed in Supplementary Figure S10.
The negative trend in productivity of land without degraded land in terms of SOC and land cover was dominant in those regions that did not exhibit negative LCLU transitions (Supplementary Figure S3). The states where this condition was confirmed were Roraima and Rondônia in Brazil, Santa Cruz in Bolivia, and Caquetá in Colombia. These locations are characterized by large rainfed crops (e.g., rice, soybeans, corn, cotton, and sugarcane) and pastures established before 2001 and remaining until 2020, both of which are susceptible to rainfall variability (Zhang et al., 2021). Consequently, the land degradation captured by the SDG indicator 15.3.1 in these regions may have been driven by regional climate variability, including indirect impacts such as widespread fire-induced tree mortality (Machado-Silva et al., 2020). These findings are consistent with studies based on MODIS NDVI time-series data, where phenological changes in rainfed crops and pastures are exacerbated by climate variability. (Hilker et al., 2014; Morton et al., 2016).
The combined negative change in all three sub-indicators was observed over about 3.13% of the Amazon basin (187,312 km2). In Figure 7, it can be seen that most of these degraded lands surround croplands and pastures that only showed degradation in land productivity. In this context, it may correspond to the expansion of the agricultural Frontier between 2001 and 2020. Usually, this would lead to short-term declines in rainfed crop yields and progressive land degradation (Sims et al., 2019). This assumption is supported by recent studies highlighting the impacts of large monocultures and fragmented landscapes on biodiversity in the southwestern Amazon basin (de Almeida et al., 2020; Marengo et al., 2022).
In some cases, the land degradation processes directly related to the LCLU transition were evident (Supplementary Figure S17). Among them, deforestation is ranked first (i.e., from forest to farmland; 81.79% of the Amazon basin), followed by wetland drainage (i.e., from wetland to grassland/cropland/artificial/other land; 8.98% of the Amazon basin) and vegetation loss (i.e., from forest to grassland/other land; or from grassland/cropland to other land; 5.05% of the Amazon basin). The same land degradation processes were recognized by Ometto et al. (2016), Santos et al. (2020), and Souza et al. (2020). However, a limitation of the land cover degradation sub-indicator is that, as a consequence of the uncertainties inherent in the type of satellite data, sensors, time window, and spatial resolution, among other factors, the estimated degraded area was slightly different from those reported in recent literature (Neves et al., 2020). Furthermore, it is important to highlight that under the traditional land degradation approach, based on LCLU transitions, the coupling of land productivity change and land degradation without the active presence of other degradation drivers often goes undetected. If the driver of land degradation triggers a long-term NDVI change followed by its slow recovery on a multi-year time scale, such as long-term droughts, it is particularly likely to go undetected (Asner and Alencar, 2010; Panisset et al., 2018).
A less obvious factor influencing the SDG indicator 15.3.1 is SOC degradation. Unlike land productivity, SOC degradation showed a remarkable coupling to land cover degradation (Supplementary Figure S10), reflected in a high spatial coherence between them (Figures 3B,C). This result agrees with a few observational studies that pointed out a decline in SOC stock triggered by the conversion of tropical forests to cattle pastures and agriculture in the Amazon basin (Cerri et al., 2007).
4.2 Influence of the drought variability on land degradation in the Amazon basin
From the brief discussion above, it can be argued that drought has an obvious implication on land degradation. At first, by comparing Figures 2, 5C, 6, one can see that these metrics may reasonably capture the influence of drought variability on NPP through annualized NDVI. The Amazon basin was exposed to prolonged and severe droughts between 2001 and 2020 (Figure 4), which is directly in line with previous findings (Lima and AghaKouchak, 2017; Sorí et al., 2017; Anderson et al., 2018; Paredes-Trejo et al., 2021). As mentioned, prolonged rainfall deficits may have partially contributed to the downward trend in land productivity without a negative signal in other sub-indicators (Figure 7). This singularity, in turn, would allow the propagation of the drought signal to the land productivity sub-indicator, favouring the classification of those pixels exposed to drought as degraded land, as suggested in Supplementary Figure S8.
The results also revealed one interesting feature regarding the concomitant action between the long-term dryness and LCLU on the SDG indicator 15.3.1. Among the benchmark sites, the artificial lands tended to be classified as degraded (90% of them), followed by croplands (86% of them) and other lands (40.33% of them). In contrast, although the tree-covered lands were exposed to high drought severity (0.309), these showed a relatively low incidence of degradation (19.34% of them). This result implies that the forested regions exhibit greater resilience than other types of LCLU to multi-annual droughts in terms of change in annualized NDVI (see Supplementary Figure S18). Previous research suggests that during prolonged droughts, the tropical forest phenology is mainly driven by soil water availability and adaptation strategy to avoid excessive loss of water used by plants (e.g., the stomatal closure) (Betts et al., 2004; Fisher et al., 2006). These mechanisms can help to explain their high resilience to drought.
Overall, our results provide strong evidence that a multi-year drought can favour the identification of false negatives, where land productivity may be declining due to climate conditions rather than anthropogenic or biological factors. However, while long-term drought alone does not cause land degradation, it may exacerbate the susceptibility of land to human-induced changes. In this context, the rainfed croplands are most likely to be classified as degraded by the UNCCD approach (Anderson et al., 2018; Bullock et al., 2020).
It is important to emphasize that the land degradation processes identified here are heavily dependent on scale, spatial resolution, and uncertainties inherent to each dataset used in this study. Naturally, these restrictions could have masked some degradation land processes that are only evident at a finer scale (e.g., invasion of woody plants in grasslands). Regardless of those technical barriers, the operational implementation of the UNCCD approach to land degradation monitoring in the Amazon basin should consider the effect of long-term drought on the metrics behind the land productivity sub-indicator to minimize the misidentification of degraded land.
5 Conclusion
The Amazon basin has experienced recent extreme droughts. Three satellite-based drought indices and the SDG indicator 15.3.1 adopted by the UNCCD were used to assess land degradation and better understand how drought variability affects the detection of land degradation processes in the Amazon basin. The newest version of the CHIRPS-based SPI and the SPEI and scPDSI derived from the CRU TS dataset were used as drought indices. The SDG indicator 15.3.1 was calculated using the procedures described in the second version of the Good Practice Guidance for SDG Indicator 15.3.1 (Sims et al., 2019). The annual LCLU maps from the MapBiomas project at 30 m spatial resolution and the 16-day MOD13Q1 NDVI and SoilGrids dataset at 250 m spatial resolution were used as inputs. The following conclusions can be drawn from this study:
• The UNCCD approach estimated 757,704 km2 (12.67% of the basin) as degraded land for 2001–2020, mainly reflected by a significant decline in land productivity dynamics, followed by the combined downward trend in land productivity, SOC degradation, and land cover degradation.
• The most significant land degradation hotspots were localized in the southern, southwestern, and eastern portions of the Amazon basin, where large monocultures and pastures dominate.
• The drought severity measured using the scPDSI showed greater spatial consistency with SDG indicator 15.3.1 than that with the SPI and SPEI.
• The results provide strong evidence that the detection of degraded land via the SDG indicator 15.3.1 is sensitive to long-term drought conditions. Under severe drought conditions, the indicator tended to assign a higher percentage of degraded land than under non-drought conditions, particularly on rainfed cropland.
Currently, several climate correction methods have been incorporated in the latest version of the UNCDD approach to minimize the effect of climate variability on land productivity dynamics (Sims et al., 2019). They are the rainfall use efficiency (Le Houérou, 1984), residual trends (Evans and Geerken, 2004), and Relative RUE (Evans and Geerken, 2004). However, this study did not explore their abilities to improve the detection of land degradation under long-term drought conditions, so it remains an open topic that will be assessed in future works.Data availability statement
The original contributions presented in the study are included in the article/Supplementary Material, further inquiries can be directed to the corresponding author. The source data used in Figures 3, 5 are available as GeoTIFF files through Zenodo at https://zenodo.org/record/6499616.
Author contributions
FP-T, HB, and JG conceived and designed the experiments; TK performed the experiments; MK and CdO analyzed the data and contributed to the analysis tools; FP-T, HB, and JG wrote the paper, but all authors discussed the results and enhanced the final draft of the manuscript. All authors have read and agreed to the published version of the manuscript.
Funding
This work was supported by the Coordenação de Aperfeiçoamento de Pessoal de Nível Superior (CAPES 12/2020), Brazil, through Epidemias—Telemedicina e Análise de Dados Médicos, under the Grant/Award Number (23038.013745/2020-69 to HB).
Acknowledgments
The authors thank Editor and two reviewers for their insightful comments and valuable suggestions.
Conflict of interest
The authors declare that the research was conducted in the absence of any commercial or financial relationships that could be construed as a potential conflict of interest.
Publisher’s note
All claims expressed in this article are solely those of the authors and do not necessarily represent those of their affiliated organizations, or those of the publisher, the editors, and the reviewers. Any product that may be evaluated in this article, or claim that may be made by its manufacturer, is not guaranteed or endorsed by the publisher.
Supplementary material
The Supplementary Material for this article can be found online at: https://www.frontiersin.org/articles/10.3389/feart.2022.939908/full#supplementary-material
References
Ahmadalipour, A., Moradkhani, H., Yan, H., and Zarekarizi, M. (2017). “Remote sensing of drought: Vegetation, soil moisture, and data assimilation,” in Remote sensing of hydrological extremes (Springer), 121–149. doi:10.1007/978-3-319-43744-6_7
Anderson, L. O., Malhi, Y., Aragao, L. E. O. C., and Saatchi, S. (2007). “Spatial patterns of the canopy stress during 2005 drought in Amazonia,” in 2007 IEEE International Geoscience and Remote Sensing Symposium (IEEE), 2294–2297. doi:10.1109/IGARSS.2007.4423299
Anderson, L. O., Ribeiro Neto, G., Cunha, A. P., Fonseca, M. G., Mendes de Moura, Y., Dalagnol, R., et al. (2018). Vulnerability of Amazonian forests to repeated droughts. Phil. Trans. R. Soc. B 373, 20170411. doi:10.1098/rstb.2017.0411
Arima, E., Barreto, P., Taheripour, F., and Aguiar, A. (2021). Dynamic Amazonia: The EU–mercosur trade agreement and deforestation. Land 10, 1243. doi:10.3390/land10111243
Asner, G. P., and Alencar, A. (2010). Drought impacts on the Amazon forest: the remote sensing perspective. New Phytol. 187, 569–578. doi:10.1111/j.1469-8137.2010.03310.x
Atkinson, P. M., Dash, J., and Jeganathan, C. (2011). Amazon vegetation greenness as measured by satellite sensors over the last decade. Geophys. Res. Lett. 38, L19105. doi:10.1029/2011GL049118
Awange, J. L., Mpelasoka, F., and Goncalves, R. M. (2016). When every drop counts: Analysis of Droughts in Brazil for the 1901-2013 period. Sci. Total Environ. 566–567, 1472–1488. doi:10.1016/j.scitotenv.2016.06.031
Bagley, J. E., Desai, A. R., Harding, K. J., Snyder, P. K., and Foley, J. A. (2014). Drought and deforestation: Has land cover change influenced recent precipitation extremes in the Amazon? J. Clim. 27, 345–361. doi:10.1175/JCLI-D-12-00369.1
Barbosa, H. A., Lakshmi Kumar, T. V., and Silva, L. R. M. (2015). Recent trends in vegetation dynamics in the South America and their relationship to rainfall. Nat. Hazards 77, 883–899. doi:10.1007/s11069-015-1635-8
Barker, L. J., Rickards, N. J., Sarkar, S., Hannaford, J., King-Okumu, C., and Rees, G. (2021). Good practice guidance for national reporting on UNCCD strategic objective 3: To mitigate, adapt to, and manage the effects of drought in order to enhance resilience of vulnerable populations and ecosystems. Bonn, Germany: United Nations Convention to Combat Desertification (UNCCD).
Beck, H. E., Zimmermann, N. E., McVicar, T. R., Vergopolan, N., Berg, A., Wood, E. F., et al. (2018). Present and future Köppen-Geiger climate classification maps at 1-km resolution. Sci. Data 5, 180214. doi:10.1038/sdata.2018.214
Beguería, S., Vicente-Serrano, S. M., Reig, F., and Latorre, B. (2014). Standardized precipitation evapotranspiration index (SPEI) revisited: parameter fitting, evapotranspiration models, tools, datasets and drought monitoring. Int. J. Climatol. 34, 3001–3023. doi:10.1002/joc.3887
Betts, R. A., Cox, P. M., Collins, M., Harris, P. P., Huntingford, C., Jones, C. D., et al. (2004). The role of ecosystem-atmosphere interactions in simulated Amazonian precipitation decrease and forest dieback under global climate warming. Theor. Appl. Climatol. 78, 157–175. doi:10.1007/s00704-004-0050-y
Boschetti, L., Kunzle, A., Brivio, P., and Mussio, L. (2006). “Non parametric statistical tests for the analysis of multiple-sensor time series of remotely sensed data,” in 2006 IEEE International Symposium on Geoscience and Remote Sensing (IEEE), 200–203. doi:10.1109/IGARSS.2006.56
Bullock, E. L., Woodcock, C. E., Souza, C., and Olofsson, P. (2020). Satellite-based estimates reveal widespread forest degradation in the Amazon. Glob. Chang. Biol. 26, 2956–2969. doi:10.1111/gcb.15029
Cerri, C. E. P., Easter, M., Paustian, K., Killian, K., Coleman, K., Bernoux, M., et al. (2007). Predicted soil organic carbon stocks and changes in the Brazilian Amazon between 2000 and 2030. Agric. Ecosyst. Environ. 122, 58–72. doi:10.1016/j.agee.2007.01.008
da Motta Paca, V. H., Espinoza-Dávalos, G., Moreira, D., and Comair, G. (2020). Variability of trends in precipitation across the Amazon River basin determined from the CHIRPS precipitation product and from station records. Water 12, 1244. doi:10.3390/w12051244
de Almeida, A. S., Vieira, I. C. G., and Ferraz, S. F. B. (2020). Long-term assessment of oil palm expansion and landscape change in the eastern Brazilian Amazon. Land use Policy 90, 104321. doi:10.1016/j.landusepol.2019.104321
Diallo, H. A. (2008). “United Nations convention to Combat desertification (UNCCD),” in The future of drylands (Dordrecht: Springer Netherlands), 13–16. doi:10.1007/978-1-4020-6970-3_4
Easdale, M. H., Bruzzone, O., Mapfumo, P., and Tittonell, P. (2018). Phases or regimes? Revisiting NDVI trends as proxies for land degradation. Land Degrad. Dev. 29, 433–445. doi:10.1002/ldr.2871
Evans, J., and Geerken, R. (2004). Discrimination between climate and human-induced dryland degradation. J. Arid. Environ. 57, 535–554. doi:10.1016/S0140-1963(03)00121-6
Faiz, M. A., Liu, D., Fu, Q., Naz, F., Hristova, N., Li, T., et al. (2020). Assessment of dryness conditions according to transitional ecosystem patterns in an extremely cold region of China. J. Clean. Prod. 255, 120348. doi:10.1016/j.jclepro.2020.120348
Fisher, R. A., Williams, M., Do Vale, R. L., Da Costa, A. L., and Meir, P. (2006). Evidence from Amazonian forests is consistent with isohydric control of leaf water potential. Plant, Cell Environ. 29, 151–165. doi:10.1111/j.1365-3040.2005.01407.x
Friedl, M. A., Sulla-Menashe, D., Tan, B., Schneider, A., Ramankutty, N., Sibley, A., et al. (2010). Sulla-MenasheMODIS Collection 5 global land cover: Algorithm refinements and characterization of new datasets. Remote Sens. Environ. 114, 168–182. doi:10.1016/j.rse.2009.08.016
Funk, C., Peterson, P., Landsfeld, M., Pedreros, D., Verdin, J., Shukla, S., et al. (2015). The climate hazards infrared precipitation with stations—a new environmental record for monitoring extremes. Sci. Data 2, 150066. doi:10.1038/sdata.2015.66
Haghtalab, N., Moore, N., Heerspink, B. P., and Hyndman, D. W. (2020). Evaluating spatial patterns in precipitation trends across the Amazon basin driven by land cover and global scale forcings. Theor. Appl. Climatol. 140, 411–427. doi:10.1007/s00704-019-03085-3
Hao, Z., and Singh, V. P. (2015). Drought characterization from a multivariate perspective: A review. J. Hydrol. X. 527, 668–678. doi:10.1016/j.jhydrol.2015.05.031
Hayes, M., Svoboda, M., Wall, N., and Widhalm, M. (2011). The lincoln declaration on drought indices: Universal meteorological drought index recommended. Bull. Am. Meteorol. Soc. 92, 485–488. doi:10.1175/2010BAMS3103.1
Hengl, T., Mendes de Jesus, J., Heuvelink, G. B. M., Ruiperez Gonzalez, M., Kilibarda, M., Blagotić, A., et al. (2017). SoilGrids250m: Global gridded soil information based on machine learning. PLoS One 12, e0169748. doi:10.1371/journal.pone.0169748
Hijmans, R. J., and Van Etten, J. (2019). raster: Geographic data analysis and modeling. R package version 3.0-7.
Hilker, T., Lyapustin, A. I., Tucker, C. J., Hall, F. G., Myneni, R. B., Wang, Y., et al. (2014). Vegetation dynamics and rainfall sensitivity of the Amazon. Proc. Natl. Acad. Sci. U. S. A. 111, 16041–16046. doi:10.1073/pnas.1404870111
Hu, Z., Liu, S., Zhong, G., Lin, H., and Zhou, Z. (2020). Modified Mann-Kendall trend test for hydrological time series under the scaling hypothesis and its application. Hydrological Sci. J. 65, 2419–2438. doi:10.1080/02626667.2020.1810253
IPCC (2006). 2006 IPCC guidelines for national greenhouse gas inventories. Editors H. Eggleston, L. Buendia, K. Miwa, T. Ngara, and K. Tanabe (Kanagawa: Intergovernmental Panel on Climate Change). Available at: http://www.ipcc-nggip.iges.or.jp/public/2006gl/index.htm.
Jarvis, A., Reuter, H. I., Nelson, A., and Guevara, E. (2008). Hole-filled SRTM for the globe version 4. available from CGIAR-CSI SRTM 90m Database (http//srtm. csi. cgiar. org) (Accessed February 15, 2022).
Jiménez-Muñoz, J. C., Mattar, C., Barichivich, J., Santamaría-Artigas, A., Takahashi, K., Malhi, Y., et al. (2016). Record-breaking warming and extreme drought in the Amazon rainforest during the course of El Niño 2015–2016. Sci. Rep. 6, 33130. doi:10.1038/srep33130
Kayano, M. T., Andreoli, R. V., de Souza, R. A. F., and Garcia, S. R. (2017). Spatiotemporal variability modes of surface air temperature in South America during the 1951-2010 period: ENSO and non-ENSO components. Int. J. Climatol. 37, 1–13. doi:10.1002/joc.4972
Keyantash, J., and Dracup, J. A. (2002). The quantification of drought: An evaluation of drought indices. Bull. Am. Meteorol. Soc. 83, 1167–1180. doi:10.1175/1520-0477-83.8.1167
Le Houérou, H. N. (1984). Rain use efficiency: a unifying concept in arid-land ecology. J. Arid. Environ. 7, 213–247. doi:10.1016/S0140-1963(18)31362-4
Levine, R. A., and Wilks, D. S. (2000). Statistical methods in the atmospheric sciences. J. Am. Stat. Assoc. 95, 344. doi:10.2307/2669579
Lewis, S. L., Brando, P. M., Phillips, O. L., van der Heijden, G. M. F., and Nepstad, D. (2011). The 2010 Amazon drought. Science 331, 554. doi:10.1126/science.1200807
Li, H., Yang, X., and Zhang, K. (2021). Understanding global land degradation processes interacted with complex biophysics and socioeconomics from the perspective of the Normalized Difference Vegetation Index (1982–2015). Glob. Planet. Change 198, 103431. doi:10.1016/j.gloplacha.2021.103431
Lima, C. H. R., and AghaKouchak, A. (2017). Droughts in Amazonia: Spatiotemporal variability, teleconnections, and seasonal predictions. Water Resour. Res. 53, 10824–10840. doi:10.1002/2016WR020086
Lima, L. S., Coe, M. T., Soares Filho, B. S., Cuadra, S. V., Dias, L. C. P., Costa, M. H., et al. (2014). Feedbacks between deforestation, climate, and hydrology in the southwestern Amazon: implications for the provision of ecosystem services. Landsc. Ecol. 29, 261–274. doi:10.1007/s10980-013-9962-1
Liu, Y., Zhou, Y., Ju, W., Wang, S., Wu, X., He, M., et al. (2014). Impacts of droughts on carbon sequestration by China’s terrestrial ecosystems from 2000 to 2011. Biogeosciences 11, 2583–2599. doi:10.5194/bg-11-2583-2014
Lu, D., Batistella, M., Mausel, P., and Moran, E. (2007). Mapping and monitoring land degradation risks in the Western Brazilian Amazon using multitemporal Landsat TM/ETM+ images. Land Degrad. Dev. 18, 41–54. doi:10.1002/ldr.762
Ma, Y. Z. (2019). “Statistical analysis of geoscience data,” in Quantitative geosciences: Data analytics, geostatistics, reservoir characterization and modeling (Cham: Springer International Publishing), 49–75. doi:10.1007/978-3-030-17860-4_3
Machado-Silva, F., Libonati, R., Melo de Lima, T. F., Bittencourt Peixoto, R., de Almeida França, J. R., de Avelar Figueiredo Mafra Magalhães, M., et al. (2020). Drought and fires influence the respiratory diseases hospitalizations in the Amazon. Ecol. Indic. 109, 105817. doi:10.1016/j.ecolind.2019.105817
Marengo, J. A., Nobre, C., Tomasella, J., Cardoso, M. ., and Oyama, M. (2008a). Hydro-climatic and ecological behaviour of the drought of Amazonia in 2005. Phil. Trans. R. Soc. B 363, 1773–1778. doi:10.1098/rstb.2007.0015
Marengo, J. A., Nobre, C. A., Tomasella, J., Oyama, M. D., Sampaio de Oliveira, G., de Oliveira, R., et al. (2008b). The drought of Amazonia in 2005. J. Clim. 21, 495–516. doi:10.1175/2007JCLI1600.1
Marengo, J. A., Alves, L. M., Soares, W. R., Rodriguez, D. A., Camargo, H., Riveros, M. P., et al. (2013). Two contrasting severe seasonal extremes in tropical South America in 2012: Flood in Amazonia and drought in northeast Brazil. J. Clim. 26, 9137–9154. doi:10.1175/JCLI-D-12-00642.1
Marengo, J. A., Jimenez, J. C., Espinoza, J.-C., Cunha, A. P., and Aragão, L. E. O. (2022). Increased climate pressure on the agricultural frontier in the Eastern Amazonia–Cerrado transition zone. Sci. Rep. 12, 457. doi:10.1038/s41598-021-04241-4
Marengo, J. A. (2004). Interdecadal variability and trends of rainfall across the Amazon basin. Theor. Appl. Climatol. 78, 79–96. doi:10.1007/s00704-004-0045-8
Marengo, J. A. (2005). Characteristics and spatio-temporal variability of the Amazon River basin water budget. Clim. Dyn. 24, 11–22. doi:10.1007/s00382-004-0461-6
McKee, T. B., Doesken, N. J., and Kleist, J. (1993). “The relationship of drought frequency and duration to time scales,” in Proceedings of the 8th Conference on Applied Climatology, 17 - 22 January 1993 (Anaheim, California: American Meteorological Society), 179–183. Available at: https://www.jstor.org/stable/26230365.
Metcalfe, D. B., Meir, P., Aragão, L. E. O. C., Lobo-do-Vale, R., Galbraith, D., Fisher, R. A., et al. (2010). Shifts in plant respiration and carbon use efficiency at a large-scale drought experiment in the eastern Amazon. New Phytol. 187, 608–621. doi:10.1111/j.1469-8137.2010.03319.x
Mishra, A. K., and Singh, V. P. (2010). A review of drought concepts. J. Hydrol. X. 391, 202–216. doi:10.1016/j.jhydrol.2010.07.012
Morton, D. C., Noojipady, P., Macedo, M. M., Gibbs, H., Victoria, D. C., Bolfe, E. L., et al. (2016). Reevaluating suitability estimates based on dynamics of cropland expansion in the Brazilian Amazon. Glob. Environ. Change 37, 92–101. doi:10.1016/j.gloenvcha.2016.02.001
Neves, A. K., Körting, T. S., Fonseca, L. M. G., and Escada, M. I. S. (2020). Assessment of TerraClass and MapBiomas data on legend and map agreement for the Brazilian Amazon biome. Acta Amaz. 50, 170–182. doi:10.1590/1809-4392201900981
Nobre, C. A., Marengo, J. A., and Artaxo, P. (2009). “Understanding the climate of Amazonia: Progress from LBA,” in Amazonia and global change. Editors M. Keller, M. Bustamante, J. Gash, and P. Silva Dias, 145–147. Geophys. Mon. Ser. doi:10.1029/2009GM000903
Oliveira-Júnior, J. F., Silva Junior, C. A., Teodoro, P. E., Rossi, F. S., Blanco, C. J. C., Lima, M., et al. (2021). Confronting CHIRPS dataset and in situ stations in the detection of wet and drought conditions in the Brazilian Midwest. Int. J. Climatol. 41, joc.7080. doi:10.1002/joc.7080
Ometto, J. P., Sousa-Neto, E. R., and Tejada, G. (2016). “Land use, land cover and land use change in the Brazilian Amazon (1960–2013),” in Interactions between biosphere, atmosphere and human land use in the Amazon basin, 369–383. Ecological Studies. doi:10.1007/978-3-662-49902-3_15
Panisset, J. S., Libonati, R., Gouveia, C. M. P., Machado-Silva, F., França, D. A., França, J. R. A., et al. (2018). Contrasting patterns of the extreme drought episodes of 2005, 2010 and 2015 in the Amazon Basin. Int. J. Climatol. 38, 1096–1104. doi:10.1002/joc.5224
Paredes-Trejo, F., Barbosa, H. A., Giovannettone, J., Lakshmi Kumar, T. V., Thakur, M. K., de Oliveira Buriti, C., et al. (2021). Long-term spatiotemporal variation of droughts in the Amazon River basin. Water 13, 351. doi:10.3390/w13030351
Prince, S. D. (2019). Challenges for remote sensing of the Sustainable Development Goal SDG 15.3.1 productivity indicator. Remote Sens. Environ. 234, 111428. doi:10.1016/j.rse.2019.111428
Ritter, C. D., Zizka, A., Barnes, C., Nilsson, R. H., Roger, F., Antonelli, A., et al. (2019). Locality or habitat? Exploring predictors of biodiversity in Amazonia. Ecography 42, 321–333. doi:10.1111/ecog.03833
Rotllan-Puig, X., Ivits, E., and Cherlet, M. (2021). LPDynR: A new tool to calculate the land productivity dynamics indicator. Ecol. Indic. 133, 108386. doi:10.1016/j.ecolind.2021.108386
Santos, D. C., Souza-Filho, P. W. M., Rocha Nascimento, W., Cardoso, G. F., and Santos, J. F. (2020). Land cover change, landscape degradation, and restoration along a railway line in the Amazon biome, Brazil. Land Degrad. Dev. 31, 2033–2046. doi:10.1002/ldr.3514
Satyamurty, P., da Costa, C. P. W., and Manzi, A. O. (2013). Moisture source for the Amazon basin: a study of contrasting years. Theor. Appl. Climatol. 111, 195–209. doi:10.1007/s00704-012-0637-7
Sims, N. C., England, J. R., Newnham, G. J., Alexander, S., Green, C., Minelli, S., et al. (2019). Developing good practice guidance for estimating land degradation in the context of the United Nations Sustainable Development Goals. Environ. Sci. Policy 92, 349–355. doi:10.1016/j.envsci.2018.10.014
Sorí, R., Marengo, J., Nieto, R., Drumond, A., and Gimeno, L. (2017). “Drought and wet episodes in Amazonia: the role of atmospheric moisture transport,” in Proceedings of First International Electronic Conference on the Hydrological Cycle (Basel, Switzerland: MDPI), 4846. doi:10.3390/CHyCle-2017-04846
Souza, C. M., Shimbo, J. Z., Rosa, M. R., Parente, L. L., Alencar, A. A., Rudorff, B. F. T., et al. (2020). Reconstructing three decades of land use and land cover changes in Brazilian biomes with Landsat archive and earth engine. Remote Sens. (Basel). 12, 2735. doi:10.3390/rs12172735
Tillé, Y., Matei, A., Matei, M. A., and Imports, M. (2016). Package ‘sampling’. Surv. Sampl. Kasutatud. 23, 2017.
Tritsch, I., and Le Tourneau, F.-M. (2016). Population densities and deforestation in the Brazilian Amazon: New insights on the current human settlement patterns. Appl. Geogr. 76, 163–172. doi:10.1016/j.apgeog.2016.09.022
van der Schrier, G., Barichivich, J., Briffa, K. R., and Jones, P. D. (2013). A scPDSI-based global data set of dry and wet spells for 1901-2009. J. Geophys. Res. Atmos. 118, 4025–4048. doi:10.1002/jgrd.50355
Vicente-Serrano, S. M., Beguería, S., and López-Moreno, J. I. (2010). A multiscalar drought index sensitive to global warming: The standardized precipitation evapotranspiration index. J. Clim. 23, 1696–1718. doi:10.1175/2009JCLI2909.1
Vicente-Serrano, S., Cabello, D., Tomás-Burguera, M., Martín-Hernández, N., Beguería, S., Azorin-Molina, C., et al. (2015). Drought variability and land degradation in semiarid regions: Assessment using remote sensing data and drought indices (1982–2011). Remote Sens. (Basel). 7, 4391–4423. doi:10.3390/rs70404391
Wells, N., Goddard, S., and Hayes, M. J. (2004). A self-calibrating palmer drought severity index. J. Clim. 17, 2335–2351. doi:10.1175/1520-0442(2004)017<2335:ASPDSI>2.0.CO;2
Yue, S., Pilon, P., and Cavadias, G. (2002). Power of the Mann–Kendall and Spearman’s rho tests for detecting monotonic trends in hydrological series. J. Hydrol. X. 259, 254–271. doi:10.1016/S0022-1694(01)00594-7
Zargar, A., Sadiq, R., Naser, B., and Khan, F. I. (2011). A review of drought indices. Environ. Rev. 19, 333–349. doi:10.1139/a11-013
Zhang, M., Abrahao, G., Cohn, A., Campolo, J., and Thompson, S. (2021). A MODIS-based scalable remote sensing method to estimate sowing and harvest dates of soybean crops in Mato Grosso, Brazil. Heliyon 7, e07436. doi:10.1016/j.heliyon.2021.e07436
Keywords: Amazonia, drought, Amazon River basin, land degradation, land-use change, standardized precipitation index, spatiotemporal droughts
Citation: Paredes-Trejo F, Barbosa H, Giovannettone J, Kumar TVL, Kumar Thakur M and de Oliveira Buriti C (2022) Drought variability and land degradation in the Amazon River basin. Front. Earth Sci. 10:939908. doi: 10.3389/feart.2022.939908
Received: 09 May 2022; Accepted: 08 July 2022;
Published: 11 August 2022.
Edited by:
Jose A. Marengo, Centro Nacional de Monitoramento e Alertas de Desastres Naturais (CEMADEN), BrazilReviewed by:
Ahmed Kenawy, Mansoura University, EgyptReet Kamal Tiwari, Indian Institute of Technology Ropar, India
Copyright © 2022 Paredes-Trejo, Barbosa, Giovannettone, Kumar, Kumar Thakur and de Oliveira Buriti. This is an open-access article distributed under the terms of the Creative Commons Attribution License (CC BY). The use, distribution or reproduction in other forums is permitted, provided the original author(s) and the copyright owner(s) are credited and that the original publication in this journal is cited, in accordance with accepted academic practice. No use, distribution or reproduction is permitted which does not comply with these terms.
*Correspondence: Franklin Paredes-Trejo, ZnBhcmVkZXNAdW5lbGxlei5lZHUudmU=