- 1The Key Laboratory of Image Processing and Intelligent Control, Ministry of Education, The School of Artificial Intelligence and Automation, Huazhong University of Science and Technology, Wuhan, China
- 2Concordia Institute for Information Systems Engineering, Concordia University, Montreal, QC, Canada
- 3Institute of Advanced Technology, Nanjing University of Posts and Telecommunications, Nanjing, China
Editorial on the Research Topic
Distributed learning, optimization, and control methods for future power grids
The development of renewable energy sources makes an important contribution to low-carbon sustainable development. However, renewable energy such as wind and photovoltaic energy are highly random and intermittent, which brings great challenges to the operation and control of power networks. The traditional method is to build a large number of peak-shaving power stations and arrange a large number of energy storage devices to stabilize the fluctuations of renewable energy, and the cost may be prohibitive to be sustainable. In the future power network, advanced sensors, actuators, and communication equipment will be deployed on various systems such as generators, substations, transformers, distributed energy resources, air conditioners, and electric vehicles. A challengeable issue arises on how to manage these hundreds of millions of active endpoints. Recently, distributed learning, optimization, and control methods for networked systems have received growing attention. Distributed learning, optimization, and control methods for managing hundreds of millions of active endpoints in the future power network are expected to enable a stable and economic operation of the power network with a high proportion of new energy, as illustrated in Figure 1.
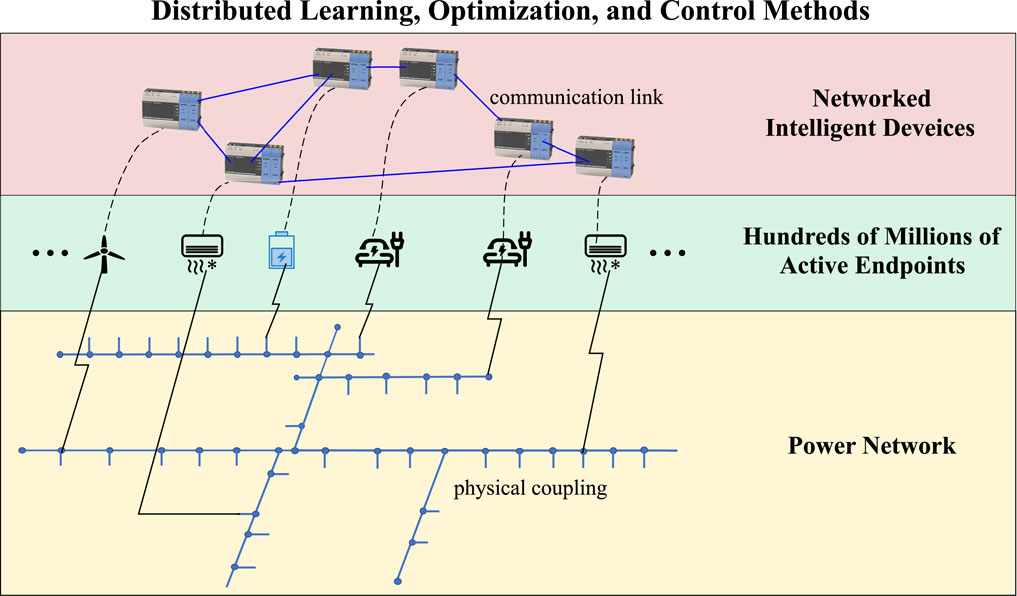
FIGURE 1. Simplified diagram of distributed learning, optimization, and control methods for future power grids.
This issue contains nine research articles focusing on distributed control and optimization for power systems and smart power electronics, multi-agent reinforcement learning in power systems, advanced energy management and economic dispatch, etc.
The paper by Ma et al. proposes a multi-time-scale control method based on a deep Q network-deep deterministic policy gradient (DQN-DDPG) algorithm to maintain optimal voltage in distribution networks. It optimizes voltage regulation for longer times using the DQN algorithm and shorter times using the DDPG algorithm. Then, based on the Markov decision process transformation, the design strategy of the DQN-DDPG algorithm is proposed to extend the energy storage capacity with respect to the stated objectives and constraints, taking into account the state of charge of the energy storage. A simulation platform backs up the proposed strategy, showing its effectiveness compared to a particle swarm optimization algorithm.
The stable operation of microgrid clusters is the focus and difficulty of current research. Shi et al. propose a ring-based multi-agent microgrid cluster energy management strategy, which realizes the centerless coordinated autonomous operation of microgrid clusters with high stability. They also offer switchable control strategies for the microgrid cluster’s varying control objectives, allowing for seamless grid connectivity changes. Simulation results prove that the proposed method enhances the stability, autonomy, and efficiency of energy utilization in microgrid clusters.
The paper by Xiao et al. proposes a joint sensor secure rate and energy efficiency optimization algorithm to enhance Wireless Sensor Network (WSN) performance in intelligent management of a photovoltaic power system, obtaining the optimal topology and transmission power design. The proposed algorithm comprehensively considers the factors those affect the security and efficiency of WSN. Moreover, Block Coordinate Descent technology is utilized to minimize the energy consumption of WSN and maximize the secure rate of sensor networks, thus ensuring the security and reliability of monitoring. Finally, the sensor association, modeled as a 0–1 multi-knapsack optimization problem, is compared using different methods.
With the increase of devices in power grids, a critical challenge emerges on how to collect information from massive devices, as well as how to manage these devices. Zhao et al. explore device scheduling, where a portion of mobile devices are selected at each time slot to collect more valuable sensing data. However, the device scheduling task is difficult due to the lack of a priori knowledge and the large amount of data. Therefore, the device scheduling problem is reformulated as a multi-armed bandit program, which is solved by a device scheduling algorithm based on upper confidence bound policy and virtual queue theory. Simulation results verify the effectiveness of the proposed algorithm, in terms of performance regret and convergence rate.
The trading of virtual power plants (VPPs) should not only consider the economy but also its degree of low carbon. The paper by Chu et al. construct a unified bidding strategy for multi-VPPs that considers carbon–electricity integration trading. After each VPP determines its internal trading strategy, multi-game trading strategy between multiple VPPs is designed to achieve unified trading. Simulations verify that this approach can enhance the efficiency and trading income of VPPs, promoting new energy consumption.
The paper by Han proposes a fault-tolerant control scheme using a wavelet analysis and consensus algorithm to tackle voltage and frequency regulation issues in smart grids impacted by faults. Faults are identified through a wavelet analysis, followed by a distributed fault estimator to capture attack signals. The simulation of a smart grid with four distributed generations in MATLAB/Simulink illustrates the method’s effectiveness in achieving voltage and frequency regulation objectives.
The paper by Shen et al. proposes a magnetic induction positioning and communication system for underwater use, including an energy-efficient distributed control algorithm for base stations. This technology uses a network of base stations and an Autonomous Underwater Vehicle with three-axis source coils for precise location and communication. The algorithm controls base stations to meet operational needs while minimizing energy use, achieving stable, long-term function.
The paper by Yang et al. proposes a Lyapunov-based power sharing control scheme and a fixed-time-based distributed optimization algorithm to achieve optimal power sharing of sources in a DC microgrid. The controller uses a ratio consensus protocol to achieve proportional power sharing by modifying the microgrid’s voltage profile. The optimizer, integrating a finite-time weighted consensus algorithm with an iterative algebraic operation, calculates optimal power dispatch to minimize generation costs. Both units function in a wholly distributed manner. Stability and convergence analyses of the control scheme and optimization algorithm are provided.
The paper by Qi et al. proposes a novel visual-admittance-based model predictive control scheme to cope with the problem of vision/force control and several constraints of a nuclear collaborative robotic visual servoing system. The scheme considers the desired image features and force commands in the image feature space, and then uses the desired force commands as constraints for model predictive control, thus eliminating overshooting in interactive force control in most cases. Simulation results with a two-degree-of-freedom robot manipulator confirm this method’s effectiveness.
Author contributions
Z-WL: Writing–original draft. X-KL: Writing–review and editing. JY: Writing–review and editing. CD: Writing–review and editing.
Conflict of interest
The authors declare that the research was conducted in the absence of any commercial or financial relationships that could be construed as a potential conflict of interest.
Publisher’s note
All claims expressed in this article are solely those of the authors and do not necessarily represent those of their affiliated organizations, or those of the publisher, the editors and the reviewers. Any product that may be evaluated in this article, or claim that may be made by its manufacturer, is not guaranteed or endorsed by the publisher.
Keywords: distributed learning, distributed optimization, distributed control, data analytics, microgrids, demand response
Citation: Liu Z-W, Liu X-K, Yan J and Deng C (2023) Editorial: Distributed learning, optimization, and control methods for future power grids. Front. Energy Res. 11:1337268. doi: 10.3389/fenrg.2023.1337268
Received: 12 November 2023; Accepted: 20 November 2023;
Published: 04 December 2023.
Edited and reviewed by:
ZhaoYang Dong, Nanyang Technological University, SingaporeCopyright © 2023 Liu, Liu, Yan and Deng. This is an open-access article distributed under the terms of the Creative Commons Attribution License (CC BY). The use, distribution or reproduction in other forums is permitted, provided the original author(s) and the copyright owner(s) are credited and that the original publication in this journal is cited, in accordance with accepted academic practice. No use, distribution or reproduction is permitted which does not comply with these terms.
*Correspondence: Zhi-Wei Liu, endsaXVAaHVzdC5lZHUuY24=