Improving Source Apportionment of Urban Aerosol Using Multi-Isotopic Fingerprints (MIF) and Positive Matrix Factorization (PMF): Cross-Validation and New Insights
- 1Department of Atmospheric Sciences, Institute of Astronomy, Geophysics and Atmospheric Sciences, University of São Paulo, São Paulo, Brazil
- 2Centro de Pesquisas Geocronológicas, Instituto de Geociências, Universidade de São Paulo, São Paulo, Brazil
Urban air pollution is a matter of concern due to its health hazards and the continuous population growth exposed to it at different urban areas worldwide. Nowadays, more than 55% of the world population live in urban areas. One of the main challenges to guide pollution control policies is related to pollutant source assessment. In this line, U.S. Environmental Protection Agency's Positive Matrix Factorization (EPA-PMF) has been extensively employed worldwide as a reference model for quantification of source contributions. However, EPA-PMF presents issues associated to source identification and discrimination due to the collinearities among the source tracers. Multi-Isotopic Fingerprints (MIF) have demonstrated good resolution for source discrimination, since urban sources are characterized by specific isotopic signatures. Source quantification based on total aerosol mass is the main limitation of MIF. This study reports strategies for PMF and MIF combination to improve source identification/discrimination and its quantification in urban areas. We have three main findings: (1) cross-validation of PMF source identification based on Pb and Zn isotopic fingerprints, (2) source apportionment in the MIF model for total PM mass, and (3) new insights into potential Zn isotopic signatures of biomass burning and secondary aerosol. We support future studies on the improvement of isotopic fingerprints database of sources based on diverse elements or compounds to boost advances of MIF model applications in atmospheric sciences.
Introduction
Air pollution is a major environmental threat to public health and climate change, which results in millions of premature deaths each year and affects the radiative balance and cloud formation process in the atmosphere (World Health Organization, 2017). Large amounts of particulate matter (PM) emitted globally come from urban areas mainly contributed by the transport sector, industrial processes, and domestic fuel burning (Karagulian et al., 2015). Recent findings have evidenced direct association between concentrations of fine particles (PM2.5) and mortality (Anenberg et al., 2019). Knowledge of the source origin and contribution to human exposure are essential to targeting effective strategies for air quality management (Thunis et al., 2019).
Multivariate receptor modeling (e.g., PCA, PMF2, ME-2, and EPA-PMF) has been extensively employed to assess source apportionment investigations in environmental science (Reff et al., 2007). Positive Matrix Factorization (PMF) was developed by Paatero and Tapper (1993, 1994), and most recently, a freely available graphical interface was developed by the U.S. Environmental Protection Agency (EPA-PMF). The PMF model decomposes a matrix of samples in two matrices (factor contributions and profiles) in order to quantify the contributions of specific pollutant sources (Paatero et al., 2005; Brown et al., 2015). Despite important improvements of PMF in relation to PCA models, some limitations of these models, like source identification, are implied in receptor multivariate analysis based on elemental concentrations (Figure 1).
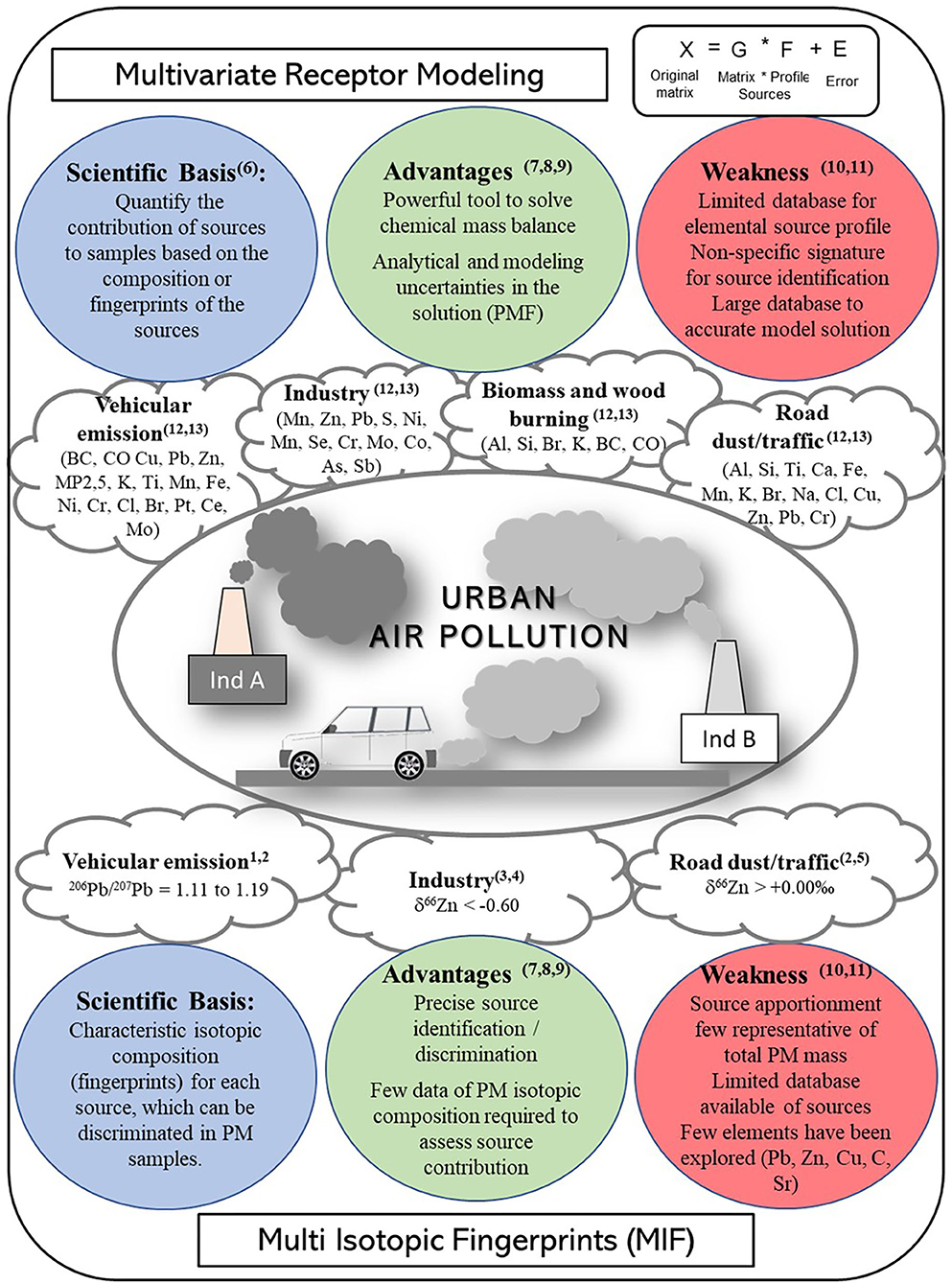
Figure 1. Scientific basis to source identification/discrimination and apportionment in urban aerosol using MIF and PMF models. Advantages and disadvantages of both models. Brief review of main species profiles and isotopic signatures used to source identification. (1) Gioia et al. (2017), (2) Souto-Oliveira et al. (2018, 2019), (3) Mattielli et al. (2009), (4) Yin et al. (2015), (5) Dong et al. (2017), (6) Norris et al. (2014), (7) Brown et al. (2015), (8) Paatero et al. (2014), (9) Reff et al. (2007), (10) Belis et al. (2013), (11) Hopke (2016), (12) Calvo et al. (2013), (13) Supplementary Table 1.
Isotope fingerprints show great potential to source tracing in the environment and has recently been used for many purposes (Aebischer et al., 2015; Araújo et al., 2016, 2018, 2019; Gelly et al., 2019; Nazarpour et al., 2019). The main advantage in the combination of stable (C, O, Zn, Cu, and Fe) and radiogenic (Pb, Sr, and Nd) isotopes is found on the improvement of the source discrimination resolution. Heavy radiogenic isotopes being produced by radioactive decay should be unaffected by mass fractionation in comparison to stable isotopes (Blum and Erel, 2003; Vallero, 2014). Therefore, radiogenic isotopes present specific signatures for each source and have been applied as powerful fingerprints (Komárek et al., 2008). On the other hand, isotopic fractionation of stable isotopes, caused by physical–chemical reactions, must be characterized in order to trace pollutant dispersion in the environment (Wiederhold, 2015).
Isotopes of only one element can present some limitations to distinguish among many different sources in complex systems (Cheng and Hu, 2010). This drawback has been outlined by a combination of isotopes of two or more elements (Figure 1), named here as the Multi-Isotopic Fingerprints (MIF) approach, which has improved source identification and discrimination of PM in urban areas and other sites (Widory et al., 2004, 2010; Cloquet et al., 2006; Dolgopolova et al., 2006; Guéguen et al., 2012; Sherman et al., 2015; Ochoa González et al., 2016; Dong et al., 2017; Souto-Oliveira et al., 2018, 2019; Schleicher et al., 2020). However, source contributions are quantified for those specific elements only, being few representatives of total PM mass, considering low elemental mass of Pb, Cu, and Zn (<1%) compared to total PM mass.
Taking into account the current gaps of multivariate receptor modeling and MIF (Figure 1), our study has two main goals. The first goal is to assess isotopic fingerprints as an alternative to validate source identification in the EPA-PMF model. The second goal is to improve the estimation of source contribution in the MIF model based on total PM mass. In order to examine strategies to achieve these aims, we performed EPA-PMF analysis, assessing uncertainties with bootstrap and displacement parameters and employing the elemental concentrations and Pb and Zn isotopic signatures measured in PM2.5 samples in São Paulo City. The Metropolitan Area of São Paulo (MASP) is the biggest urban area in South America and is one of the top 10 megacities in the world. Air pollution at MASP has been well-described by several previous studies (Andrade et al., 2017 and Supplementary Table 1) and has some similarities when compared to other megacities and unique characteristics such as the high contribution of bio-fuels (gasohol and bio-diesel) in the transport sector (Nogueira et al., 2015).
Methodology
Elemental and Black Carbon Concentrations
The PM2.5 was sampled in winter of 2013 on the rooftop (~12 m) of the Astronomy, Geophysics, and Atmospheric Sciences Institute at University of São Paulo campus (USP, 23.56°S, 46.73°W), which is located in the west side of the urbanized area of São Paulo City. The sampling site is situated in the western side of the city and is located approximately 1 km northeast and 4 km north of the two main roads with intense traffic (Marginal Tietê and Marginal Pinheiros, respectively). Each sample was collected during 12-h intervals in polycarbonate membranes (Whatman®) under constant air flow (16 L min−1). Other details of sampling procedures are described in Souto-Oliveira et al. (2018). The PM2.5 mass concentration, deposited in polycarbonate filters (47 mm diameter), was determined gravimetrically using a microbalance (Mettler-Toledo, Columbus, OH, USA) with 1 μg of readability, which presents <1% of aerosol mass.
Elemental concentrations were determined by an Energy Dispersive X-ray Fluorescence Spectrometer (EDX 700; Shimadzu Corporation, Analytical Instruments Division, Tokyo, Japan). The spectrometer operates at 5–50 kV and 1–1,000 μA, using a low-power Rh-target tube, and the elemental characteristic X-ray radiation emitted from the aerosol sample is detected with a Si(Li) detector. The spectra obtained were processed and quantified with the Win-QXAS program, available from the International Atomic Energy Agency (https://www-legacy.iaea.org/OurWork/ST/NA/NAAL/pci/ins/xrf/pciXRFdown.php).
The BC analysis was performed by optical reflectance with a smoke stain reflectometer (model 43D; Diffusion Systems Ltd, London, UK) and detailed previously by Souto-Oliveira et al. (2016) and Hetem and Andrade (2016). The concentrations of CO and SO2 regulated gases, measured by the Environmental Agency of São Paulo State, were used to support source attribution to PMF factors [details on the equipment and data treatment are described at Environmental Agency of São Paulo (CETESB), 2020].
PMF and Multi-Isotopic System Analysis
We used a correlation matrix plot (CM) with Pearson's correlation and a principal component analysis (PCA) in order to analyze the relationships within the dataset. The non-significant correlations (p < 0.01) were excluded. The PCA was calculated using the varimax rotation. The number of components was limited to five due to the eigenvalue of the sixth being <1. Both analyses were implemented in R language using the psych (Revelle, 2020) and corrplot (Wei and Simko, 2017) packages.
The source apportionment analysis was performed using the PMF-EPA model (version 5.0) provided by EPA. Several runs with different numbers of factors, species, and categorization were fulfilled in order to obtain the best adjustment and physical validity of the model. The goodness of fit was evaluated by minimization of Q (true, robust, and expected) and a scaled residual between −2 and +2, following the recommendations of Reff et al. (2007). In addition, uncertainties estimation of PMF solutions was assessed via bootstrapping (Brown et al., 2015). All tests to model optimization are described in the Supplementary Information.
The source apportionment results estimated via MIF based on Pb and Zn isotopic signatures and concentrations were accounted for during PMF runs for improvements of MIF source attribution estimations as well, as discussed in section Results and Discussion. All the Pb and Zn isotopic signatures and MIF model results were previously reported by Souto-Oliveira et al. (2018). The Pb and Zn isotopic compositions are denoted as 206Pb/207Pb ratios and δ66ZnJMC values. The JMC (Lyon, batch 3-0749L) is known as the international standard and has been extensively used as reference to report Zn isotopic compositions.
Results and Discussion
Correlations and Multivariate (PCA and PMF) Analysis
CM and multivariate (PCA and PMF) statistical analysis were employed to assess the database (Supplementary Table 2) regarding the investigation of elemental profiles for each factor and support for source attribution. Another new methodology involved examining strategies and relevance of including Pb and Zn isotopic data on PCA and PMF analysis, considering that any studies have already explored this approach, to the best of the authors' knowledge. The raw dataset (Supplementary Table 2) was assessed with CM (Supplementary Figure 1) in order to examine main correlations between species and support PCA and PMF species profiles. Strong correlations (R > 0.7) were found (Supplementary Figure 1) among crustal elements (Al, Si, Fe, Ti, Ca, and K), whereas PM2.5 was correlated with BC. Two other groups with strong correlations, among species, were Pb, Cu, Zn, and Cl and P, S, and Mn. The Pb and Zn isotopic compositions and Cr did not show any positive correlation with other species, in line with PCA and PMF, which presented better adjustments after removal of these species (Supplementary Tables 3, 4).
PCA showed best adjustments with four factors, taking into account best-explained variance (Supplementary Table 3). In the PMF analysis, change in the number of factors showed a slight decrease in Q/Qexpec when changing from three to four factors and a great increase when changing from four to five factors. However, two important sources (road dust and biomass burning) remain combined in the same factor (Supplementary Figure 2), when only four factors were considered. The solution with five factors was chosen (Figure 2) in order to split these sources. In addition, all factors were mapped more than 85%, in the solution with five factors, which means that bootstrap uncertainties can be interpreted and the number of factors is appropriated (Supplementary Table 4). Bootstrap is an important parameter to detect random errors and, to a lesser extent, effects of rotational ambiguity (Norris et al., 2014). In this line, displacement consists of uncertainties estimation related to data uncertainties (data noise) and rotational ambiguity, which is focused on the lower %dQ and no factor changes (swaps) (Brown et al., 2015). Despite the main changes in factor or species during assessment of PMF settings, %dQ remains <0.1% and any swaps occurred (Supplementary Table 4), ensuring the absence of rotational ambiguity in our PMF results.
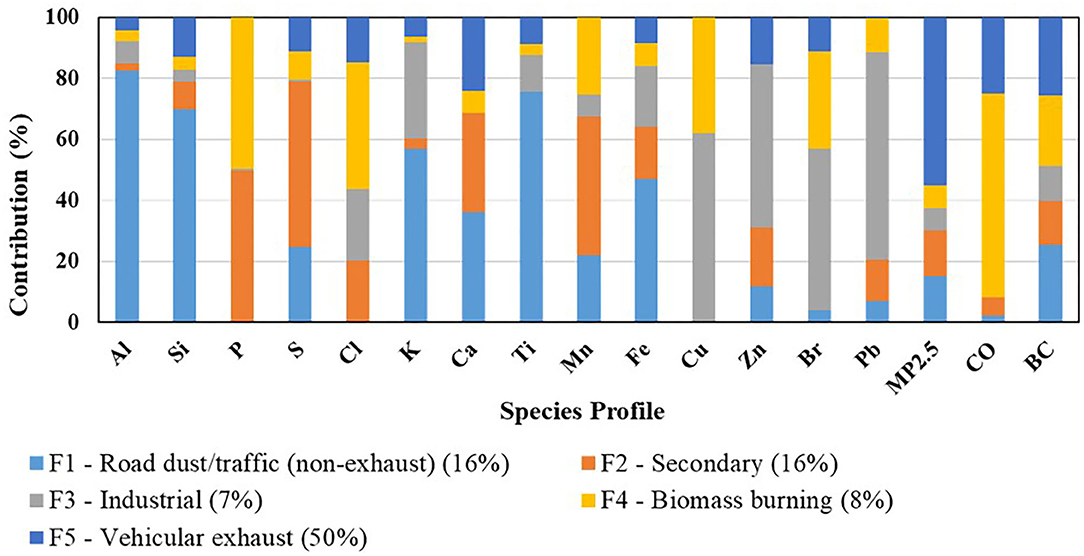
Figure 2. EPA-PMF species profiles, obtained with species concentrations (elemental, CO, and BC) measured in PM2.5 from São Paulo City, during winter 2013. The percentual source contributions (shown in parentheses), were calculated by average of factor contribution in each sample. This percentual was calculated using contributions of each factor, in concentration units, against total PM mass. These estimations were performed using base run output of EPA-PMF analysis.
CM (Supplementary Figure 1), PCA (Supplementary Table 3), and PMF (Supplementary Table 4) results agreed on the lack of correlation among Pb and Zn isotope composition. To address this issue, we can consider that multivariate tools are based on linear correlations among species and variance of these species in the samples. On the other hand, MIF is not based on the variance, but instead on specific isotopic signatures of each source, such that discrete ranges of isotopic scales identify/discriminate between different sources. An additional explanation is based on non-linear correlation observed in the Pb and Zn elemental concentration vs. isotopic composition (206Pb/207Pb ratios and δ66Zn values) distributions (Souto-Oliveira et al., 2018). Therefore, the distinct scientific basis of PCA/PMF and MIF models could explain those insignificant correlations. An alternative approach to fix this limitation is further discussed in Section MIF Improvement and PMF-MIF Combined Source Apportionment.
Source attribution of PMF factors (Figure 2) was supported by previous works, which reported species profiles and source apportionment in São Paulo City (Supplementary Table 1). These previous studies provide important background to upcoming source apportionment assessments based on receptor modeling. In addition, PMF-species profiles (Figure 2) are in accordance with CM-species groups (Supplementary Figure 1), ensuring robustness of species profiles obtained in our analysis.
Factor 1 presented higher contributions of crustal elements (Al, Si, Ti, Fe, Ca, and K), linked to road dust, and lower contributions of BC, Zn, Pb, S, and Mn species, associated with road traffic. This species profile is in line with CM (Supplementary Figure 1) and PCA (Supplementary Table 3) analysis as well as studies performed in other cities and current road tunnels characterization carried in the São Paulo City (Amato et al., 2011; Gunawardana et al., 2012; Hetem and Andrade, 2016; Jithin and Srimuruganandam, 2020; Nory et al., 2021). It is important to mention that urban road dust is associated with road traffic, which combines contributions of vehicular non-exhaust emissions (tire wear and brakes abrasion) and road pavement/furniture (Thorpe and Harrison, 2008). This factor, named here as road dust/traffic, accounted for 16% of the PM2.5.
Factor 2 presented higher contributions of S and P, in line with CM (Supplementary Figure 1), and minor contributions of BC, Mn, Fe, Zn, and Pb (Figure 2). An important PM2.5 source related to S and P, in sulfate form, is the particle formation process, which generates secondary aerosol in the atmosphere. Many inorganic (sulfate and nitrate) and organic compounds (primary and secondary) as well as physical–chemical conditions (temperature, solar radiation, and oxidants) are involved in this process (Holmes, 2007; Rönkkö and Timonen, 2019). In urban areas, a pool of anthropogenic activities (fossil fuel combustion, biomass, wood and waste burning, cooking, and industrial emissions) generate inorganic and volatile organic compounds, found to be important raw materials for secondary aerosol formation (Gordon et al., 2014; Kanellopoulos et al., 2021). Therefore, secondary aerosol combines contributions of different sources but presents specific formation process and characteristic species profile. Previous findings have showed higher concentrations of S and P in fossil fuels (oil, gasoline, and diesel) and minor concentrations of metals (Fe, Zn, Mn) (Jones et al., 2014; ACEA, 2018). The association of S and P with oil burning (Supplementary Table 1) and an empirical S vs. P correlation, linked to fuels, have been reported in São Paulo City (Brito et al., 2013; Marien, 2018).
Industries are present inside and outside MASP with two main industrial areas (Cubatão and Maua). Factor 3 presented species profile related to Pb, Cu, Zn, and Br elements (Figure 2), aligned with CM (Supplementary Figure 1), and showed a contribution of 7%, in line with earlier estimations (Supplementary Table 1). Cubatão is in the coastal region, about 48 km southeast of São Paulo City (sampling site), and ranked as one of the biggest Latin American industrial areas. Interestingly, chloride, a tracer of sea salt and industrial activities, had also contributed to factor 3. Aerosol transportation from the Cubatão industrial area to São Paulo City has been well-documented using Pb isotopes and will be further discussed in Section Validation of PMF Source Identification by Isotopic Fingerprints (Gioia et al., 2017; Souto-Oliveira et al., 2018).
Factor 4 accounted for 8% of PM2.5 mass and presented strong association with CO, Cl, and P with minor contributions of Br and BC. Some works have demonstrated the linkage of those species with biomass burning (Bond et al., 2013; Calvo et al., 2013; Tian et al., 2016; Andreae, 2019). Recent studies have characterized and estimated contributions of biomass and wood burning to air pollution in São Paulo, coming from pizzerias (in the City), rural activities (São Paulo State), and forest burning in the north region of Brazil (Amazonas and Pantanal) (Pereira et al., 2016; Andrade et al., 2017; Ribeiro et al., 2018; Lima et al., 2020). Also, biomass burning transport to São Paulo City was previously demonstrated (Souto-Oliveira et al., 2016; Vara-Vela et al., 2018). PM2.5, CO, and BC species have been related to vehicular exhaust [Andrade et al., 2015; Environmental Agency of São Paulo (CETESB), 2020]. These species were retained in factor 5 (Figure 2) and grouped in CM (Supplementary Figure 1). Factor 5 accounted for 50% of PM2.5 mass, in accordance with intense vehicular traffic, regularly found in the São Paulo City, as well as previous source apportionment estimation carried out since 1994 (Supplementary Table 1). Linkage of this factor with vehicular exhaust was confirmed by Zn isotopes signatures, as discussed in Section Validation of PMF Source Identification by Isotopic Fingerprints.
Validation of PMF Source Identification by Isotopic Fingerprints
The Zn isotope signatures vs. PMF factor regression was employed to examine isotopic fingerprint applicability as an alternative to cross-validate the PMF source attribution. PMF factors related to road dust/traffic (non-exhaust) showed significant (p < 0.05) positive correlation (R = 0.63) with δ66ZnJMC values (Figure 3A). This observation is in line with heavier Zn isotopes (δ66ZnJMC > 0.00‰) found in the road dust and vehicular non-exhaust samples (Dong et al., 2017; Souto-Oliveira et al., 2018; Nory et al., 2021). PMF factors attributed to vehicular exhaust showed negative correlation with δ66ZnJMC values (Figure 3B), in accordance with preliminary lighter Zn isotopes signatures (δ66ZnJMC > −0.60 and <0.00‰) characterized in the vehicular exhaust from São Paulo City (Gioia et al., 2008).
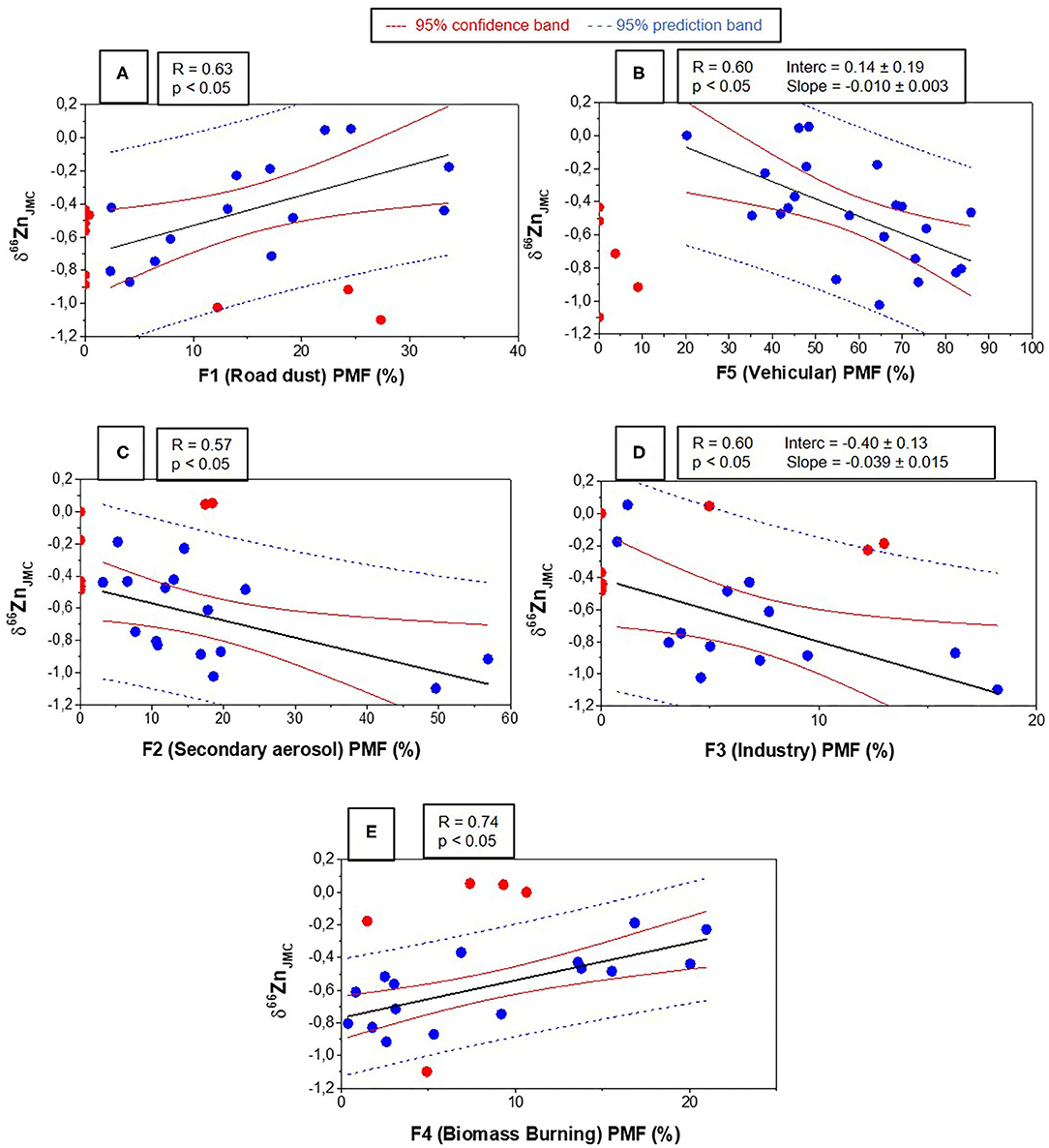
Figure 3. PMF-factors vs. d66Zn values regressions, performed to examine correlations between PMF-factors and Zn isotopic signatures. These regressions confirmed PMF source attributions, based on Zn isotopic fingerprints related to each source. As example, heavier Zn isotopes found in road dust (A) and light Zn signatures on vehicular (B) and lighter one on industrial emissions (D). In addition, these regressions suggest new Zn isotopic signatures trends based on PMF-factors, like secondary aerosol (C) and biomass burning (E). Data outside of prediction bands and with zero percentage were classified as outliers (in red).
Factor 3 percentual contributions, related to industrial source, presented negative correlation with δ66ZnJMC values (Figure 3C), as expected, considering that industrial emissions have been reported by lower δ66ZnJMC values, enriched with lighter Zn isotopes, against raw materials (ore) and residual phase (Mattielli et al., 2009; Yin et al., 2015). In the São Paulo City, industrial emission was linked to δ66ZnJMC < −0.60‰ (Souto-Oliveira et al., 2018). Despite lighter Zn signatures, reported to vehicular exhaust and industrial sources, those can be split into different δ66ZnJMC ranges (Figure 1). Interestingly, PMF vs. δ66ZnJMC linear regression fits are aligned with these ranges, considering intercept (0.14 ± 0.19 and −0.40 ± 0.13) and slopes (−0.010 ± 0.003 and −0.039 ± 0.015) estimated for vehicular exhaust and industrial emissions, respectively.
The Zn isotopic signatures of vehicular exhaust and industrial emissions must be extensively assessed in future works, considering different vehicle combustion features, such as fuel compositions and motor types, and industrial processes. However, here we can assume the occurrence of Zn isotopic fractionation during vehicular combustion, taking into account previous works that reported isotopic fractionation during the industrial combustion process, described below, as well as the negative linear trend found here between δ66ZnJMC and PMF vehicular exhaust contributions (Mattielli et al., 2009; Borrok et al., 2010).
To the best of the authors' knowledge, until now, the Zn isotopic signatures of biomass burning and secondary aerosol were not characterized. However, here we have observed positive correlations between δ66Zn values and PMF factors, associated with biomass burning, suggesting PM emissions enriched in heavier Zn isotopes in this source (Figure 3E). Aligned with this evidence, industrial combustion of coal and tire-derived fuels has evidenced that the PM produced (solid phase) are enriched by heavier Zn isotopes, with higher δ66Zn values, while gas phase is enriched with lighter isotopes, showing lower δ66Zn values (Borrok et al., 2010; Ochoa Gonzalez and Weiss, 2015). These works support the positive correlation between biomass burning and δ66Zn values found here.
In relation to secondary aerosol, negative correlation was observed between PMF factor contributions and δ66Zn values (Figure 3D). It is important to consider that secondary aerosol is formed by inorganic and organic compounds found in vapor/liquid phase in the atmosphere via photochemistry reactions (Holmes, 2007). Considering that lighter Zn isotopes are enriched in vapor phase during the combustion process (Borrok et al., 2010), we suppose that vapor phase with lower δ66ZnJMC values contributes to secondary aerosol formation. However, it is imperative experimental investigations to confirm these evidences, considering the large heterogeneity of biomass burning and complexity of secondary aerosol composition.
MIF Improvement and PMF–MIF Combined Source Apportionment
One important limitation of the MIF model is related to quantification of source contribution, considering that these estimations are based on few elements. Therefore, source contributions calculated by the MIF model may be under- or overestimated in relation to total PM mass. In order to fix this limitation, MIF vs. PMF linear regression (Figure 4) was employed to recalculate the original MIF results, denoted as MIF-orig (Souto-Oliveira et al., 2018). Vehicular source contributions accounted for by MIF and PMF (F1-road dust/traffic and F5-vehicular traffic exhaust) showed a significant (p < 0.05) correlation (R = 0.64) (Figure 4A). Also, industrial emissions of both models showed a positive correlation (Figure 4B). These results endorse the strategy of validating source attribution of PMF factors by MIF.
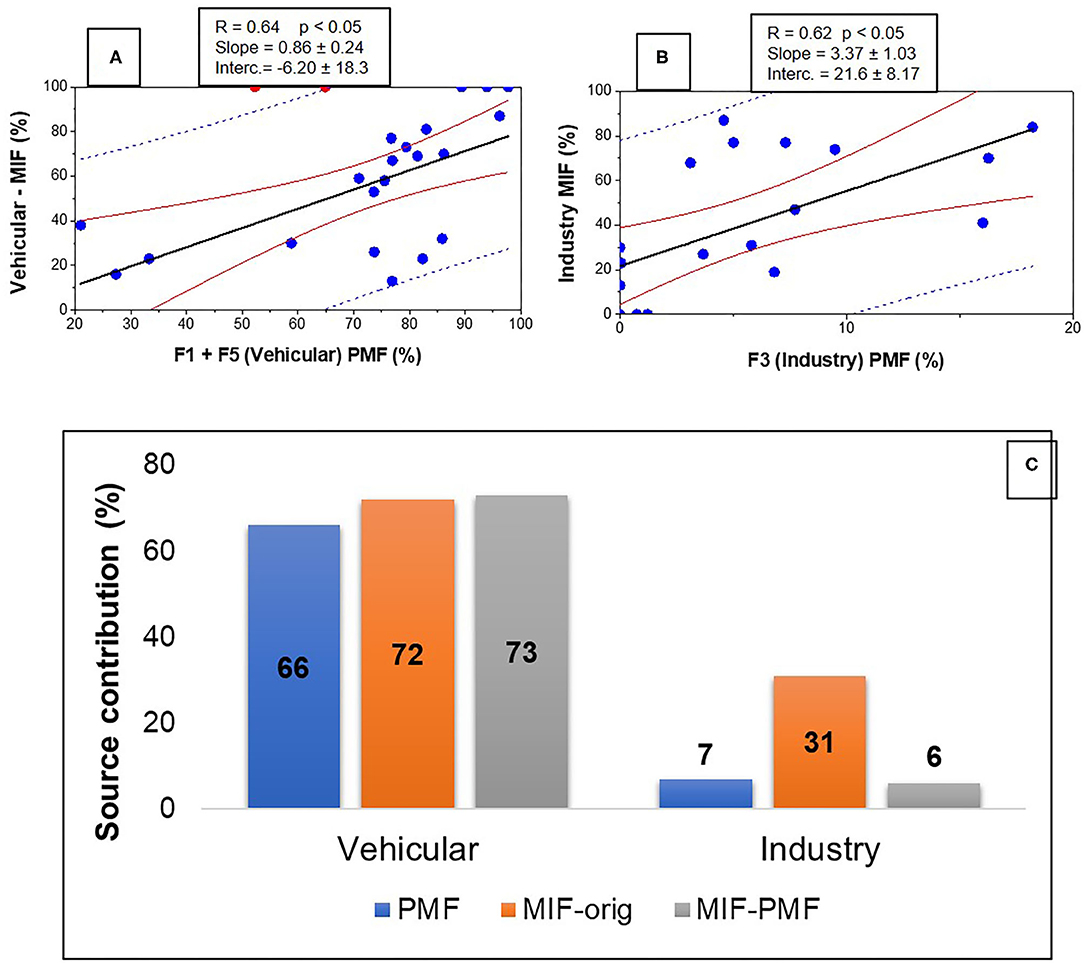
Figure 4. PMF vs. MIF percentual contributions regressions for vehicular (A) and industrial (B) sources, estimated in PM2.5 from São Paulo City. These regressions were employed to recalculate previous MIF (Orig) results (Souto-Oliveira et al., 2018) in order to report MIF-contributions in relation to total PM mass. The new results were denoted as MIF-PMF (C), based on combination of both models.
The comparison of source contribution percentages obtained by PMF, MIF-orig, and those recalculated, denoted as MIF-PMF, is illustrated in Figure 4C. The vehicular contribution was very similar between PMF (72%), MIF-orig (66%), and MIF-PMF (75%). Industrial contribution modeled by MIF-orig (36%) was four times overestimated in comparison to PMF (7%). However, it must be remembered that MIF-orig is an estimation of industrial contribution to concentrations of Pb and Zn, which are mainly associated to that source. On the other hand, industrial contribution (6%) estimated by MIF-PMF was remarkably similar to PMF results, endorsing the validity of MIF-PMF correction strategy to report contribution for total PM mass.
The combination of PMF and MIF showed relevant improvements to source identification and discrimination in both models (Figure 5). Non-exhaust and exhaust vehicular emission, retained in PMF factors 1 and 5, respectively, showed the main (66%) contribution to PM2.5 in São Paulo City. Vehicular exhaust was characterized by less radiogenic Pb isotopes and lighter Zn isotopes combined with BC, CO, and PM2.5 species (Gioia et al., 2008, 2017; Souto-Oliveira et al., 2018). Road dust and road traffic/non-exhaust were characterized by heavier Zn isotopic signatures and crustal (Al, Si, Ti, Fe, and Ca) and BC, Zn, and Pb species profiles, respectively (Dong et al., 2017; Souto-Oliveira et al., 2018; Nory et al., 2021). Biomass burning showed a positive correlation with δ66Zn values (Figure 3E), suggesting that this source is enriched with heavier Zn isotopes, which is supported by coal and tire-fuel combustion studies (Borrok et al., 2010; Ochoa Gonzalez and Weiss, 2015).
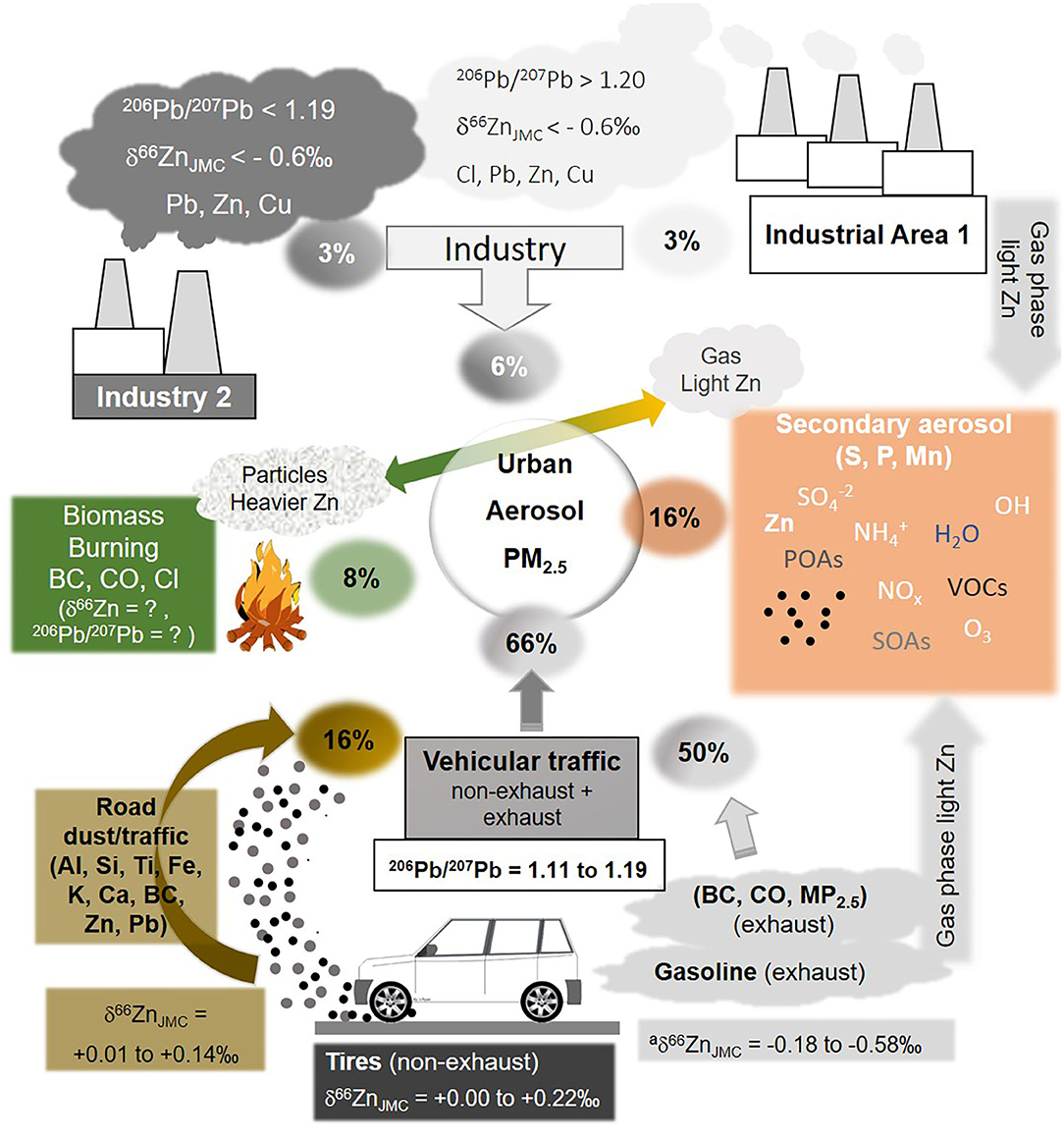
Figure 5. Schematic source identification and apportionment using Multi-Isotopic Fingerprints (MIF) and species profiles obtained by EPA-PMF model for PM2.5 from São Paulo City. Species profiles from EPA-PMF could be cross-validated by isotopic fingerprints. Industrial source contributions were differentiated using Pb and Zn isotopic signatures. Positive linear regression between biomass burning vs. Zn isotopes suggested that particles enriched with heavier Zn signatures. Negative linear regression between secondary aerosol vs. Zn isotopic signatures suggested particles enriched with lighter Zn.
Industrial emissions were earlier characterized by lighter Zn isotopes (δ66ZnJMC < −0.60‰) and Pb isotopes, which could differentiate between the Cubatão industrial area (206Pb/207Pb > 1.20) and other industries (206Pb/207Pb <1.19) (Mattielli et al., 2009; Yin et al., 2015; Gioia et al., 2017; Souto-Oliveira et al., 2018). Here, Zn isotopic signatures showed negative correlation with PMF factor 3, associated with industrial emissions (Figure 3D), confirming source attribution of this PMF factor. In line with this, the MIF industry showed a significant and positive correlation with PMF factor contributions, which reinforces source attribution of PMF factor analysis. This source accounted for 6% of PM2.5 mass, and the contribution of Cubatão (3%) and other industries (3%) could be estimated based on the MIF model (Figure 5). It is important to mention that the MIF model assimilates source discrimination resolution features of Pb and Zn isotopes (Souto-Oliveira et al., 2018) and therefore can differentiate between industrial emissions of specific areas (Cubatão and other industries), whereas in PMF, these sources were observed in the same factor. Secondary aerosol source accounted for 16% of PM2.5 and was associated with S, P, and Mn species here and in earlier works (Supplementary Table 1). The PMF factor contributions of this source showed negative correlation with Zn isotopes, indicating that lighter Zn could be attributed to secondary aerosol and gas phase of anthropogenic sources.
Conclusion
The PMF and MIF solutions were combined to provide a step forward to assess source identification and apportionment of urban aerosol. Here, three main advances could be reached: (1) cross-validation of source identification based on two different tools (PMF elemental profiles and MIF isotopic fingerprints), (2) improvement of source contribution in the MIF model to total PM2.5 mass, and (3) new insights into the investigation of Zn signatures in atmospheric processes and pollutant sources.
Cross-validation of PMF source identification by isotopic fingerprints seems to pose a significant way to improve the accuracy of source apportionment investigation. However, it is very important to advance the isotopic fingerprints and species profile characterization of the main sources. This approach is essential to develop a large source database in order to support robust MIF and PMF identification/discrimination. The strategy to recalculate the MIF model results to PM mass, based on linear regression with PMF solutions, is a valid method to be explored in future studies. On the other hand, the MIF model must be improved by inclusion of additional elements (Fe, S, Cu, C, and O) and also by elemental ratios, which could be a great alternative to fix over- or underestimations in relation to PM mass. The potential enrichment of heavier Zn isotopes in biomass burning particles and lighter Zn isotopes in secondary aerosol particles, suggested here, must be further investigated in future works in order to improve knowledge of isotopic fractionation in the source dispersion and atmospheric processes.
Data Availability Statement
The original contributions presented in the study are included in the article/Supplementary Material, further inquiries can be directed to the corresponding author/s.
Author Contributions
CS-O manuscript write, figures creation, MIF, and PMF analysis. LK CM/PCA analysis and manuscript reviewer. MA and MB manuscript reviewer and funds acquisition. All authors contributed to the article and approved the submitted version.
Funding
We thank Brazilian research funding agencies for making this work possible: the Fundação de Amparo à Pesquisa do Estado de São Paulo (FAPESP) for financial support of the METROCLIMA project (process 2016/18438-0) and CE Souto-Oliveira post-doc scholarship (grant no. 2019/16885-8), Coordenação de Aperfeiçoamento de Pessoal de Nível Superior (CAPES), and Conselho Nacional de Desenvolvimento Científico e Tecnológico (CNPq, grant no. 167722/2018-2) by LK PhD scholarship.
Conflict of Interest
The authors declare that the research was conducted in the absence of any commercial or financial relationships that could be construed as a potential conflict of interest.
Acknowledgments
We also thank the reviewers for valuable suggestions that greatly improved this work.
Supplementary Material
The Supplementary Material for this article can be found online at: https://www.frontiersin.org/articles/10.3389/fenvs.2021.623915/full#supplementary-material
References
ACEA (2018). Worldwide fuel charter | gasoline and diesel fuel sixth edition. Available: https://www.acea.be/publications/article/worldwide-fuel-charter-2019-gasoline-and-diesel-fuel (accessed October 22, 2020).
Aebischer, S., Cloquet, C., Carignan, J., Maurice, C., and Pienitz, R. (2015). Disruption of the geochemical metal cycle during mining: multiple isotope studies of lake sediments from Schefferville, subarctic Québec. Chem. Geol. 412, 167–178. doi: 10.1016/j.chemgeo.2015.07.028
Amato, F., Pandolfi, M., Moreno, T., Furger, M., Pey, J., Alastuey, A., et al. (2011). Sources and variability of inhalable road dust particles in three European cities. Atmos. Environ. 45, 6777–6787. doi: 10.1016/j.atmosenv.2011.06.003
Andrade, M. F., Kumar, P., Freitas, E. D., Ynoue, R., Martins, J., Martins, L. D., et al. (2017). Air quality in the megacity of São Paulo: evolution over the last 30 years and future perspectives. Atmos Environ. 159, 66–82. doi: 10.1016/j.atmosenv.2017.03.051
Andrade, M. F., Ynoue, R. Y., Freitas, E. D., Todesco, E., Vara-Vela, A. L., Ibarra, S., et al. (2015). Air quality forecasting system for Southeastern Brazil. Front. Environ. Sci. 3, 1–14. doi: 10.3389/fenvs.2015.00009
Andreae, M. O. (2019). Emission of trace gases and aerosols from biomass burning – an updated assessment. Atmos. Chem. Phys. Discuss. 19, 1–27. doi: 10.5194/acp-2019-303
Anenberg, S. C., Achakulwisut, P., Brauer, M., Moran, D., Apte, J. S., and Henze, D. K. (2019). Particulate matter-attributable mortality and relationships with carbon dioxide in 250 urban areas worldwide. Sci. Rep. 9:11552. doi: 10.1038/s41598-019-48057-9
Araújo, D. F., Boaventura, G. R., Machado, W., Viers, J., Weiss, D., Patchineelam, S. R., et al. (2016). Tracing of anthropogenic zinc sources in coastal environments using stable isotope composition. Chem. Geol. 449, 226–235. doi: 10.1016/j.chemgeo.2016.12.004
Araújo, D. F., Machado, W., Weiss, D., Mulholland, D. S., Garnier, J., Souto-Oliveira, C. E., et al. (2018). Applied Geochemistry Zinc isotopes as tracers of anthropogenic sources and biogeochemical processes in contaminated mangroves. Appl. Geochem. 95, 25–32. doi: 10.1016/j.apgeochem.2018.05.008
Araújo, D. F., Ponzevera, E., Briant, N., Bruzac, S., Sireau, T., and Brach-Papa, C. (2019). Copper, zinc and lead isotope signatures of sediments from a mediterranean coastal bay impacted by naval activities and urban sources. Appl. Geochem. 111:104440. doi: 10.1016/j.apgeochem.2019.104440
Belis, C. A., Karagulian, F., Larsen, B. R., and Hopke, P. K. (2013). Critical review and meta-analysis of ambient particulate matter source apportionment using receptor models in Europe. Atmos. Environ. 69, 94–108. doi: 10.1016/j.atmosenv.2012.11.009
Blum, J. D., and Erel, Y. (2003). Radiogenic isotopes in weathering and hydrology in treatise on geochemistry. Elsevier Sci. 5, 365–392. doi: 10.1016/B0-08-043751-6/05082-9
Bond, T. C., Doherty, S. J., Fahey, D. W., Forster, P. M., Berntsen, T., Deangelo, B. J., et al. (2013). Bounding the role of black carbon in the climate system. a scientific assessment. JGR Atmos. 118, 5380–5552. doi: 10.1002/jgrd.50171
Borrok, D. M., Gieré, R., Ren, M., and Landa, E. R. (2010). Zinc isotopic composition of particulate matter generated during the combustion of coal and coal + tire-derived fuels. Environ. Sci. Technol. 44, 9219–9224. doi: 10.1021/es102439g
Brito, J., Rizzo, L. V., Herckes, P., Vasconcellos, P. C., Caumo, S. E. S., Fornaro, A., et al. (2013). Physical–chemical characterisation of the particulate matter inside two road tunnels in the São Paulo Metropolitan Area. Atmos. Chem. Phys. 13, 12199–12213. doi: 10.5194/acp-13-12199-2013
Brown, S. G., Eberly, S., Paatero, P., and Norris, G. A. (2015). Methods for estimating uncertainty in PMF solutions: Examples with ambient air and water quality data and guidance on reporting PMF results. Sci. Total Environ. 518–519, 626–635. doi: 10.1016/j.scitotenv.2015.01.022
Calvo, A. I., Alves, C., Castro, A., Pont, V., Vicente, A. M., and Fraile, R. (2013). Research on aerosol sources and chemical composition: past, current and emerging issues. Atmos. Res. 120–121, 1–28. doi: 10.1016/j.atmosres.2012.09.021
Cheng, H., and Hu, Y. (2010). Lead (Pb) isotopic fingerprinting and its applications in lead pollution studies in China: a review. Environ. Pollut. 158, 1134–46. doi: 10.1016/j.envpol.2009.12.028
Cloquet, C., Carignan, J., and Libourel, G. (2006). Isotopic composition of Zn and Pb atmospheric depositions in an urban/Periurban area of northeastern France. Environ. Sci. Technol. 40, 6594–6600. doi: 10.1021/es0609654
Dolgopolova, A., Weiss, D. J., Seltmann, R., Kober, B., Mason, T. F. D., Coles, B., et al. (2006). Use of isotope ratios to assess sources of Pb and Zn dispersed in the environment during mining and ore processing within the Orlovka–Spokoinoe mining site (Russia). Appl. Geochem. 21, 563–579. doi: 10.1016/j.apgeochem.2005.12.014
Dong, S., Ochoa, R., Harrison, R. M., Green, D., North, R., Fowler, G., et al. (2017). Isotopic signatures suggest important contributions from recycled gasoline, road dust and non-exhaust traffic sources for copper, zinc and lead in PM 10 in London, United Kingdom. Atmos. Environ. 165, 88–98. doi: 10.1016/j.atmosenv.2017.06.020
Environmental Agency of São Paulo (CETESB) (2020). Air Quality System Database. Available online at: https://qualar.cetesb.sp.gov.br/qualar/home.do (accessed June 15, 2020).
Gelly, R., Fekiacova, Z., Guihou, A., Doelsch, E., Deschamps, P., and Keller, C. (2019). Lead, zinc, and copper redistributions in soils along a deposition gradient from emissions of a Pb-Ag smelter decommissioned 100 years ago. Sci. Total Environ. 665, 502–512. doi: 10.1016/j.scitotenv.2019.02.092
Gioia, S., Weiss, D., Coles, B., Arnold, T., and Babinski, M. (2008). Accurate and precise zinc isotope ratio measurements in urban aerosols. Anal. Chem. 80, 9776–9780. doi: 10.1007/s00216-007-1635-y
Gioia, S. M. C. L., Babinski, M., Weiss, D. J., Spiro, B., Kerr, A. A. F. S., Veríssimo, T. G., et al. (2017). An isotopic study of atmospheric lead in a megacity after phasing out of leaded gasoline. Atmos. Environ. 149, 70–83. doi: 10.1016/j.atmosenv.2016.10.049
Gordon, T. D., Presto, A. A., Nguyen, N. T., Robertson, W. H., Na, K., Sahay, K. N., et al. (2014). Secondary organic aerosol production from diesel vehicle exhaust: impact of aftertreatment, fuel chemistry and driving cycle. Atmos. Chem. Phys. 14, 4643–4659. doi: 10.5194/acp-14-4643-2014
Guéguen, F., Stille, P., Lahd Geagea, M., Perrone, T., and Chabaux, F. (2012). Atmospheric pollution in an urban environment by tree bark biomonitoring - part II: Sr, Nd and Pb isotopic tracing. Chemosphere 86, 641–647. doi: 10.1016/j.chemosphere.2011.11.008
Gunawardana, C., Goonetilleke, A., Egodawatta, P., Dawes, L., and Kokot, S. (2012). Source characterisation of road dust based on chemical and mineralogical composition. Chemosphere 87, 163–170. doi: 10.1016/j.chemosphere.2011.12.012
Hetem, I. G., and Andrade, M. F. (2016). Characterization of fine particulate matter emitted from the resuspension of road and pavement dust in the Metropolitan Area of São Paulo, Brazil. Atmosphere 7:31. doi: 10.3390/atmos7030031
Holmes, N. S. (2007). A review of particle formation events and growth in the atmosphere in the various environments and discussion of mechanistic implications. Atmos. Environ. 41, 2183–2201. doi: 10.1016/j.atmosenv.2006.10.058
Hopke, P. K. (2016). Review of receptor modeling methods for source apportionment. J. Air Waste. Manag. Assoc. 66, 237–259. doi: 10.1080/10962247.2016.1140693
Jithin, J., and Srimuruganandam, B. (2020). Investigation of road dust characteristics and its associated health risks from an urban environment. Environ. Geochem. Health 42, 2819–2840. doi: 10.1007/s10653-020-00521-6
Jones, F., Bankiewicz, D., and Hupa, M. (2014). Occurrence and sources of zinc in fuels. Fuel 117, 763–775. doi: 10.1016/j.fuel.2013.10.005
Kanellopoulos, P. G., Verouti, E., Chrysochou, E., Koukoulakis, K., and Bakeas, E. (2021). Primary and secondary organic aerosol in an urban / industrial site: sources, health implications and the role of plastic enriched waste burning. J. Environ. Sci. 99, 222–238. doi: 10.1016/j.jes.2020.06.012
Karagulian, F., Belis, C. A., Dora, C. F. C., Prüss-Ustün, A. M., Bonjour, S., Adair-Rohani, H., et al. (2015). Contributions to cities' ambient particulate matter (PM): a systematic review of local source contributions at global level. Atmos. Environ. 120, 475–483. doi: 10.1016/j.atmosenv.2015.08.087
Komárek, M., Ettler, V., Chrastný, V., and Mihaljevic, M. (2008). Lead isotopes in environmental sciences: a review. Environ. Int. 34, 562–77. doi: 10.1016/j.envint.2007.10.005
Lima, F. D. M., Pérez-Martínez, P. J., Andrade, M. F., Kumar, P., and Miranda, R. M. (2020). Characterization of particles emitted by pizzerias burning wood and briquettes: a case study at São Paulo, Brazil. Environ. Sci. Pollut. Res. 27, 35875–35888. doi: 10.1007/s11356-019-07508-6
Marien, Y. (2018). Quantificação das fontes locais de MP2.5 na cidade de São Paulo para o período de 2012 a 2014 (Master dissertetion), Universidade de São Paulo, São Paulo. Available online at: https://www.iag.usp.br/pos/meteorologia/portugues/dissertacoestestes/quantifica%C3%A7%C3%A3o-das-fontes-locais-de-mp25-na-cidadfe-de-s%C3%A3o (accessed April 07, 2021).
Mattielli, N., Petit, J. C. J., Deboudt, K., Flament, P., Perdrix, E., Taillez, A., et al. (2009). Zn isotope study of atmospheric emissions and dry depositions within a 5 km radius of a Pb–Zn refinery. Atmos. Environ. 43, 1265–1272. doi: 10.1016/j.atmosenv.2008.11.030
Nazarpour, A., Watts, M. J., Madhani, A., and Elahi, S. (2019). Source, Spatial distribution and pollution assessment of Pb, Zn, Cu, and Pb, isotopes in urban soils of Ahvaz City, a semi-arid metropolis in southwest Iran. Sci. Rep. 9:5349. doi: 10.1038/s41598-019-41787-w
Nogueira, T., Cordeiro, D. S., Muñoz, A. A., Fornaro, A., Miguel, A., and Andrade, M. F. (2015). “Bioethanol and biodiesel as vehicular fuels in Brazil — assessment of atmospheric impacts from the long period of biofuels use,” in Biofuels - Status and Perspective, ed Krzysztof Biernat (IntechOpen), 378–412. doi: 10.5772/60944
Norris, G., Duvall, R., Brown, S., and Bai, S. (2014). EPA Positive Matrix Factorization (PMF) 5.0 Fundamentals and User Guide. Washington, DC: Environmental Protection Agency, Office of Research and Development. Available online at: www.epa.gov (accessed October 27, 2020).
Nory, R. M., Graciano Figueiredo, A. M., Souto-Oliveira, C. E., and Babinski, M. (2021). Urban contamination sources in tunnel dusts from São Paulo city: elemental and isotopic characterization. Atmos. Environ. 118188. doi: 10.1016/j.atmosenv.2021.118188
Ochoa González, R., Strekopytov, S., Amato, F., Querol, X., Reche, C., and Weiss, D. J. (2016). New insights from zinc and copper isotopic compositions of atmospheric particulate matter from two major European cities. Environ. Sci. Technol. 50, 9816–9824. doi: 10.1021/acs.est.6b00863
Ochoa Gonzalez, R., and Weiss, D. (2015). Zinc isotope variability in three coal-fired power plants: a predictive model for determining isotopic fractionation during combustion. Environ. Sci. Technol. 49, 12560–12567. doi: 10.1021/acs.est.5b02402
Paatero, P., Eberly, S., Brown, S. G., and Norris, G. A. (2014). Methods for estimating uncertainty in factor analytic solutions. Atmos. Meas. Tech. 7, 781–797. doi: 10.5194/amt-7-781-2014
Paatero, P., Hopke, P. K., Begum, B. A., and Biswas, S. K. (2005). A graphical diagnostic method for assessing the rotation in factor analytical models of atmospheric pollution. Atmos. Environ. 39, 193–201. doi: 10.1016/j.atmosenv.2004.08.018
Paatero, P., and Tapper, U. (1993). Analysis of different modes of factor analysis as least squares fit problems. Chem. Intell. Lab. Syst. 18, 183–194. doi: 10.1016/0169-7439(93)80055-M
Paatero, P., and Tapper, U. (1994). Positive matrix factorization: a non-negative factor model with optimal utilization of error estimates of data values. Environmetrics 5, 111–126. doi: 10.1002/env.3170050203
Pereira, G., Siqueira, R., Rosário, N. E., Longo, K. L., Freitas, S. R., and Cardozo, F. S. (2016). Assessment of fire emission inventories during the South American Biomass Burning Analysis (SAMBBA) experiment. Atmos. Chem. Phys. 16, 6961–6975. doi: 10.5194/acp-16-6961-2016
Reff, A., Eberly, S. I., and Bhave, P. V. (2007). Receptor modeling of ambient particulate matter data using positive matrix factorization: review of existing methods receptor modeling of ambient particulate matter data using positive matrix factorization. J. Air Waste. Manag. 57, 146–154. doi: 10.1080/10473289.2007.10465319
Revelle, W. (2020). psych: Procedures for Psychological, Psychometric, and Personality Research. Northwestern University, Evanston, Illinois. R package version 2.0.9. Available online at: https://CRAN.R-project.org/package=psych (accessed October 27, 2020).
Ribeiro, I. O., Andreoli, R. V., Kayano, M. T., Sousa, T. R., Medeiros, A. S., Godoi, R. H. M., et al. (2018). Biomass burning and carbon monoxide patterns in Brazil during the extreme drought years of 2005, 2010, and 2015. Environ. Pollut. 243, 1008–1014. doi: 10.1016/j.envpol.2018.09.022
Rönkkö, T., and Timonen, H. (2019). Overview of sources and characteristics of nanoparticles in urban traffic-influenced areas. J. Alzheimer's Dis. 72, 15–28. doi: 10.3233/JAD-190170
Schleicher, N. J., Dong, S., Packman, H., Little, S. H., Gonzalez, R. O., Najorka, J., et al. (2020). A global assessment of copper, zinc, and lead isotopes in mineral dust sources and aerosols. Front. Earth Sci. 8:167. doi: 10.3389/feart.2020.00167
Sherman, L. S., Blum, J. D., Dvonch, J. T., Gratz, L. E., and Landis, M. S. (2015). Science of the total environment the use of Pb, Sr, and Hg isotopes in Great Lakes precipitation as a tool for pollution source attribution. Sci. Total Environ. 502, 362–374. doi: 10.1016/j.scitotenv.2014.09.034
Souto-Oliveira, C. E., Andrade, M. F., Kumar, P., Lopes FJ da, S., Babinski, M., and Landulfo, E. (2016). Effect of local and remote sources and new particle formation events on the activation properties of cloud condensation nuclei in the Brazilian megacity of São Paulo. Atmos. Chem. Phys. 16, 14635–14656. doi: 10.5194/acp-16-14635-2016
Souto-Oliveira, C. E., Babinski, M., Araújo, D. F., and Andrade, M. F. (2018). Multi-isotopic fingerprints (Pb, Zn, Cu) applied for urban aerosol source apportionment and discrimination. Sci. Total Environ. 626, 1350–1366. doi: 10.1016/j.scitotenv.2018.01.192
Souto-Oliveira, C. E., Babinski, M., Araújo, D. F., Weiss, D. J., and Ruiz, I. R. (2019). Multi-isotope approach of Pb, Cu and Zn in urban aerosols and anthropogenic sources improves tracing of the atmospheric pollutant sources in megacities. Atmos. Environ. 198, 427–437. doi: 10.1016/j.atmosenv.2018.11.007
Thorpe, A., and Harrison, R. M. (2008). Sources and properties of non-exhaust particulate matter from road traffic: a review. Sci. Total Environ. 400, 270–282. doi: 10.1016/j.scitotenv.2008.06.007
Thunis, P., Clappier, A., Tarrason, L., Cuvelier, C., Monteiro, A., Pisoni, E., et al. (2019). Source apportionment to support air quality planning: strengths and weaknesses of existing approaches. Environ. Int. 130:104825. doi: 10.1016/j.envint.2019.05.019
Tian, Y. Z., Shi, G. L., Huang-Fu, Y. Q., Song, D. L., Liu, J. Y., Zhou, L. D., et al. (2016). Seasonal and regional variations of source contributions for PM10 and PM2.5 in urban environment. Sci. Total Environ. 557–558, 697–704. doi: 10.1016/j.scitotenv.2016.03.107
Vallero, D. (2014). Inherent Properties of Air Pollutants in Fundamentals of Air Pollution (Fifth Edition). ScienceDirect, Academic Press. 139–195. doi: 10.1016/B978-0-12-401733-7.00006-2
Vara-Vela, A., de Fátima Andrade, M., Zhang, Y., Kumar, P., Ynoue, R. Y., Souto-Oliveira, C. E., et al. (2018). Modeling of atmospheric aerosol properties in the São Paulo metropolitan area: impact of biomass burning. J. Geophys. Res. Atmos. 123, 9935–9956. doi: 10.1029/2018JD028768
Wei, T., and Simko, V. (2017). R package “corrplot”: Visualization of a Correlation Matrix (Version 0.84). Available online at: https://github.com/taiyun/corrplot (accessed October 27, 2020).
Widory, D., Liu, X., and Dong, S. (2010). Isotopes as tracers of sources of lead and strontium in aerosols (TSP & PM2.5) in Beijing. Atmos. Environ. 44, 3679–3687. doi: 10.1016/j.atmosenv.2010.06.036
Widory, D., Roy, S., Le Moullec, Y., Goupil, G., Cocherie, A., and Guerrot, C. (2004). The origin of atmospheric particles in Paris: a view through carbon and lead isotopes. Atmos. Environ. 38, 953–961. doi: 10.1016/j.atmosenv.2003.11.001
Wiederhold, J. G. (2015). Metal stable isotope signatures as tracers in environmental geochemistry. Environ. Sci. Technol. 49, 2606–2624. doi: 10.1021/es504683e
World Health Organization (2017). (WHO) website. Available online at: http://www.who.int/phe/health_topics/outdoorair/en/ (accessed January 10, 2018).
Keywords: air polluition, megacities, vehicular emission, industrial emission, secondary aerosol, biomass burning, road dust, pollutant sources
Citation: Souto-Oliveira CE, Kamigauti LY, Andrade MdF and Babinski M (2021) Improving Source Apportionment of Urban Aerosol Using Multi-Isotopic Fingerprints (MIF) and Positive Matrix Factorization (PMF): Cross-Validation and New Insights. Front. Environ. Sci. 9:623915. doi: 10.3389/fenvs.2021.623915
Received: 02 November 2020; Accepted: 23 February 2021;
Published: 16 April 2021.
Edited by:
Nina Jasmin Schleicher, Imperial College London, United KingdomReviewed by:
Nadine Mattielli, Université libre de Bruxelles, BelgiumDarius Ceburnis, National University of Ireland Galway, Ireland
Copyright © 2021 Souto-Oliveira, Kamigauti, Andrade and Babinski. This is an open-access article distributed under the terms of the Creative Commons Attribution License (CC BY). The use, distribution or reproduction in other forums is permitted, provided the original author(s) and the copyright owner(s) are credited and that the original publication in this journal is cited, in accordance with accepted academic practice. No use, distribution or reproduction is permitted which does not comply with these terms.
*Correspondence: Carlos Eduardo Souto-Oliveira, carlos.edu.oliveira@usp.br