- 1Department of Geography, University of British Columbia, Vancouver, BC, Canada
- 2Department of Biology, University of Puerto Rico, San Juan, Puerto Rico
- 3School of Earth and Environmental Sciences, University of Queensland, Brisbane, QLD, Australia
- 4Instituto de Ecologia Regional, Universidad Nacional de Tucuman – CONICET, San Miguel de Tucumán, Argentina
- 5Department of Ecology, Evolution, and Environmental Biology, Columbia University, New York, NY, United States
Carbon sequestration through tropical reforestation and natural regeneration could make an important contribution to climate change mitigation, given that forest cover in many tropical regions increased during the early part of the 21st century. The size of this carbon sink will depend on the degree to which second-growth forests are permanent and protected from re-clearing. Yet few studies have assessed permanence of reforestation, especially not at a large spatial scale. Here, we analyzed a 14-year time series (2001–2014) of remotely sensed land-cover data, covering all tropical Latin America and the Caribbean, to quantify the extent of second-growth forest permanence. Our results show that in many cases, reforestation in Latin America and the Caribbean during the early 21st century reversed by 2014, limiting carbon sequestration. In fact, reversals of reforestation, in which some or all gains in forest cover in the early 2000s were subsequently lost, were ten times more common than sustained increases in forest cover. Had reversals of reforestation been avoided, forests could have sequestered 0.58 Pg C, over four times more carbon than we estimate was sequestered after accounting for impermanence (0.14 Pg), representing a loss of 75% of carbon sequestration potential. Differences in the prevalence of reforestation reversals across countries suggest an important role for socio-economic, political, and ecological context, with political transitions and instability increasing the likelihood of reversals. These findings suggest that national commitments to reforestation may fall short of their carbon sequestration goals without provisions to ensure long-term permanence of new forests.
Introduction
Limiting climate warming to two degrees Celsius or less will almost certainly require negative emissions – the removal of CO2 from the atmosphere through deliberate actions by humans (van Vuuren et al., 2013; Fuss et al., 2014). Reforestation through natural regeneration is an attractive option for achieving negative emissions, as it is relatively inexpensive, bears low environmental risks, and can have additional benefits, such as biodiversity conservation (Kindermann et al., 2008; van Vuuren et al., 2013; Lewis et al., 2019; Strassburg et al., 2019) and livelihood opportunities (Locatelli et al., 2015). The potential for natural forest regeneration to contribute to climate change mitigation is especially high in tropical regions, where second-growth forests gain 90% of the biomass of old-growth forest after an average of 66 years of regrowth (Chazdon et al., 2016; Poorter et al., 2016). Forest restoration and natural regeneration accordingly play a large role in global climate change mitigation and sustainable development commitments.
While deforestation continues in some tropical regions, forest cover has increased in others (Rudel et al., 2005; Sloan, 2008; Asner et al., 2009; Redo et al., 2012; Aide et al., 2013). Globally, tree cover has increased by over 2.24 million km2 since 1984 (Song et al., 2018). Although many tropical regions are still experiencing net deforestation, between 2000 and 2010, woody vegetation cover increased on over 360,000 km2 in Latin America and the Caribbean (Aide et al., 2013). These changes give cause for optimism about the contribution of tropical second-growth forests to climate change mitigation.
Though these reforestation trends are encouraging, the extent to which short-term (5–10 year) increases in forest cover persist over longer time scales, a prerequisite for carbon sequestration, remains uncertain. Increases in forest cover occur when socioeconomic and biophysical conditions are favorable (Chazdon et al., 2020). For example, industrialization, rural-to-urban migration, and agricultural intensification can lead to cessation of cultivation and forest regeneration on marginal agricultural lands (Rudel et al., 2000; DeFries and Pandey, 2010; Aide et al., 2013). Factors such as globalization, policy changes, and armed conflict also play important roles in driving forest cover change (Rudel et al., 2000; Hecht et al., 2006; Grau and Aide, 2008; Meyfroidt and Lambin, 2011; Sánchez-Cuervo and Aide, 2013). However, changing socioeconomic or biophysical conditions can inhibit or even reverse increases in forest cover (Suding et al., 2004; Aide et al., 2019).
Recent research has suggested that regenerating and restored forests have a high probability of being cleared. If second-growth forests lack permanence, their contributions to negative emissions will be limited (Schwartz et al., 2017b). For example, a study in the Peruvian Amazon found that re-growing forests had a high probability of being cleared within 5 years (Schwartz et al., 2017b), while in the Brazilian Amazon, 50% of all secondary forests were recleared within 8 years (Nunes et al., 2020). In Costa Rica, 50% of second growth forests were cleared within 20 years of regrowth (Algeet-Abarquero et al., 2015; Reid et al., 2018). However, the degree to which these findings extend to broader geographic scales has not been tested.
Impermanence of reforestation trends might have been overlooked in earlier studies of forest cover change because of the analytical methods used. These analyses typically detect trends by comparing snapshots of forest cover at two dates or by fitting linear models to time-series data, allowing for only three possibilities – deforestation, reforestation, or no change (Asner et al., 2009; Redo et al., 2012; Aide et al., 2013; Nanni et al., 2019). Restricting possible trajectories of land cover change to this narrow set of options may mask non-linear dynamics occurring at time scales shorter than study durations.
Here, we consider a broader set of possible trajectories of forest cover change to assess permanence of reforestation in Latin American and the Caribbean (LAC) between 2001 and 2014. To do so, we use a land cover time series to identify reforestation reversals – areas where forest cover initially increased but later decreased during the study period. We compare the prevalence of reforestation reversals relative to areas where reforestation was sustained or where other trends predominated. We also use these time series to estimate carbon sequestration due to reforestation from 2001 to 2014 and compare the magnitude of observed sequestration with a scenario in which no reforestation reversal occurred.
Materials and Methods
Remote Sensing of Forest Cover
We used annual land cover maps for Latin America and the Caribbean (LAC) for the period 2001–2014 (detailed descriptions of the dataset can be found in Clark et al., 2012; Aide et al., 2013; Graesser et al., 2015; Nanni et al., 2019). The annual maps were created by classifying 250 m Moderate Resolution Imaging Spectroradiometer (MODIS) satellite data using Random Forest land-cover classification models defined for each biome. The models included the following land cover categories: woody vegetation (including natural tree and shrub cover), tree plantations, cropland, pastureland, and other (i.e., bare soil, ice, snow, rock, sand dunes, built-up structures, and water).
Training data for each classifier were collected by overlaying a grid of MODIS pixels (250 × 250 m) onto multi-temporal high-resolution imagery in Google Earth and registering the land cover class and date. More than 60,000 MODIS pixels from labeled polygons were used to create the classification models in this study. For training data, woody vegetation pixels were defined as pixels with >80% cover of trees or shrubs, based on visual interpretation of high-resolution Google Earth imagery. Plantations were distinguished from woody vegetation by obvious rows and homogenous color and height. Crop and pasture were frequently confused and these two classes were combined. Because of the relatively large size of MODIS pixels, many pixels are mixed. In training data, the use of mixed pixels was limited: only pixels with > 80% cover were included as training data. In the classification, the class that received the most votes in the Random Forest was assigned. Validation pixels were assigned a class based on the majority land cover class present in that pixel from visual inspection. More details on in the collection and interpretation of the training and validation data can be found in Clark and Aide (2011).
These data were associated with the pixel statistics to create a Random Forest classification model for each mapping zone, defined by ecoregions and biomes (Olson et al., 2001). To train a zone-specific Random Forest model, land cover samples within the mapping zone of interest and the samples’ Google Earth high-resolution image acquisition date were paired with MODIS time series variables. Then, all samples within the biome (for all years) were aggregated and used to fit a model for the biome. The zone-specific Random Forest models were then applied annually to produce 14 land cover maps for each mapping region.
Previous assessments of this dataset have indicated that at the pixel level, overall accuracy of the classification is 80% (Clark et al., 2012) and that the classification distinguishes woody vegetation from other land cover types with 87% accuracy (Nanni et al., 2019), with accuracy as high as 93% in the tropical humid forest biome (Aide et al., 2013). A comparison of woody vegetation change in our dataset and annual land cover maps from the Brazilian government’s PRODES program1 yielded an R2 of 0.88 (Clark et al., 2012). For this study, to further assess the agreement between our dataset estimate of pixel-level changes and that of other global forest cover datasets, we compared pixel-level changes in our dataset to the Hansen et al. Global Forest Cover dataset (Hansen et al., 2013). We found that pixel-level changes in our dataset showed 79% overall agreement with the Global Forest Cover dataset (Supplementary Table S1, see Supplementary Material for more details).
For further analysis, we summed land cover classes within 40 km wide hexagonal grid cells (∼1200 km2). This hexagon size is approximately equal to the average municipality in LAC (Graesser et al., 2015). By summing land cover within these hexagons, we capture dynamics on relatively fine scales, while limiting per pixel geo-registration and misclassification errors. For all analyses, we considered only hexagons that overlapped at least 80% with tropical forest biomes (Tropical and Subtropical Moist Broadleaf Forest, Tropical and Subtropical Dry Broadleaf Forest, and Tropical and Subtropical Coniferous Forest biomes) according to (Olson et al., 2001).
Identification of Trends in Forest Cover
To describe trends in forest cover in individual hexagons, we used a shape selection algorithm designed for time series of Landsat data (Moisen et al., 2016). The algorithm uses non-parametric statistical methods to fit several candidate shapes to a time series of data. These candidate shapes include flat, increasing (hereafter reforestation), decreasing (hereafter deforestation), vee (hereafter deforestation reversal), and inverse vee (hereafter reforestation reversal, Figure 1). For each time series the algorithm fits all five candidate shapes to the data. It then uses the Bayesian information criterion (BIC) to select the shape with the best fit for each cell’s time series.
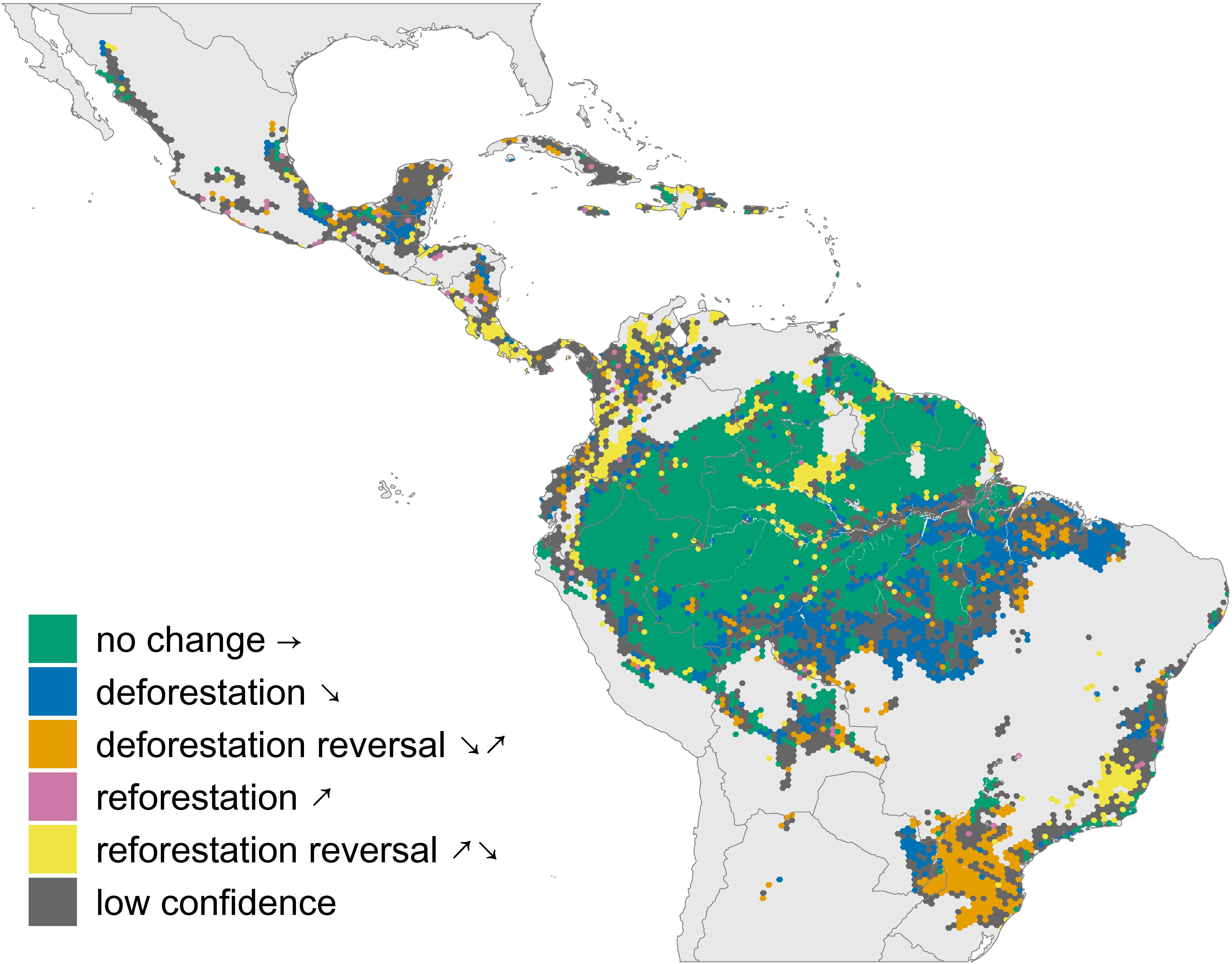
Figure 1. Trajectories of forest change in tropical Latin America and the Caribbean. Light gray areas indicate biomes other than tropical forest. Dark gray areas indicate forested regions where noisy trajectories prevented classification. Arrows in the legend illustrate the shape of the forest cover trajectory change over time.
We applied this algorithm to the 14-year time series of woody vegetation cover (tree and shrub cover, not including plantations) for each hexagon. To reduce the influence of outliers and inter-annual noise in the time series, we developed a bootstrapping procedure to determine the best-fit shape for each cell. For each time series, we removed three observations at random and used the shape selection algorithm to determine the best-fit shape for the subset of the time series. We repeated this procedure 300 times and identified the shape that was identified as the best fit in the largest number of iterations. We restricted subsequent analyses to hexagons whose shapes were selected with high confidence, which we defined as being selected in ≥90% of iterations. Hexagons in which the majority shape was selected in less than 90% of iterations were designated as “low confidence” and excluded from subsequent analyses (30.3% of hexagons). Furthermore, because the shape selection algorithm will assign a shape to a time-series regardless of the magnitude of change, we classified trajectories where the total change in forest cover was less than 1% of the grid cell (∼20 km2) as “flat” (28% of hexagons). This was done to avoid including locations with only very slight changes in our analysis so as not to inflate the frequency of change.
To understand the trajectories in more detail, for each grid cell, we calculated the total magnitude of reforestation in each grid cell as the difference between the initial and maximum forest cover. We calculated the net change during the study period as forest cover in 2014 minus forest cover in 2001. To investigate the geographic patterns of reforestation reversals, we compared the relative frequency of trajectory shapes across the seven LAC countries with over 100 hexagons that fall within forest biomes (Supplementary Table S2).
Carbon Potential Calculation
We quantified the implications of reversals of reforestation for carbon sequestration with a bookkeeping approach in which we estimated carbon accumulation in each hexagon given the observed trajectory and a region-specific rate of carbon uptake in young second-growth forests. We compared two quantities: (1) the carbon potential, i.e., the amount of carbon that would have been sequestered by new forests had no re-clearing occurred and (2) carbon sequestration observed in new forests when accounting for reversals of reforestation. We considered only reforestation relative to forest cover in 2001 and clearing of these new forests. We ignored deforestation relative to 2001, as our goal was not to quantify emissions due to deforestation, but rather, foregone carbon sequestration potential due to reversals of reforestation.
To quantify carbon potential and observed carbon sequestration, we analyzed the time series of yearly observations of woody vegetation cover for each grid cell (w0, w1, w2, …, w13), in a process analogous to a stage structured population model. For each year (t), starting in 2002, change in forest cover (r) is calculated as:
where w is woody vegetation cover (in hectares) and w0 is initial forest cover in 2001, such that we are only considering reforestation and reversal of reforestation, and do not account for deforestation of forests established prior to 2001. When rt is positive, the corresponding number of hectares are added to the youngest stage class. In subsequent time steps, those forests will advance to the next stage class, i.e., age 1 year. Negative rt is analogous to death, and is subtracted from the existing “population,” i.e., previous years’ reforestation, removing the youngest forests first until the corresponding number of hectares have been removed from the total or there are no remaining forests. At the end of each time step, forests in the “population” advance to the next stage, i.e., age 1 year.
After the final time step, we calculated carbon stored in second-growth forest. To do so, we first calculate a regional rate of carbon sequestration in each hexagon as the zonal average of total aboveground biomass and its uncertainty after 20 years of secondary forest growth, using the geographic data from Poorter et al. (2016). We approximated annual rates of biomass accumulation as 1/20th of this quantity, which assumes linear accumulation of carbon during the first 20 years of succession. We used this value to calculate total AGB stored in second-growth forests in each hexagon, given the age class structure in 2014 derived from the yearly woody vegetation cover observations as described above. To estimate stored carbon, AGB values were divided by two, under the assumption that carbon makes up 50% of biomass (Chave et al., 2009).
We repeated this procedure twice. First, we conducted the calculations exactly as described above. This calculation yielded estimates of “observed carbon.” Second, we conducted the same procedure, but calculated rt as follows:
Under this set of rules, no deforestation occurs, and all new forests added to the “population” continue to age until the end of the study period. The amount of carbon stored in second-growth forests in this scenario is the “carbon potential.” This procedure was done for all cells that experienced a net increase in forest cover relative to 2001 at some point during the study period, including all trajectory shapes.
Results
Trajectories of Forest Cover Change
Most classified hexagons (59.7%) experienced no change in forest cover during the study period. Where forest cover changed, deforestation was the most common trajectory, observed in 18.8% of classified hexagons. Deforestation reversals occurred in 8.7% of hexagons. Reversals of reforestation were nearly ten times as common as sustained increases in forest cover, occurring in 11.7% of classified hexagons (Figures 1, 2). Only 1.1% of hexagons experienced sustained reforestation.
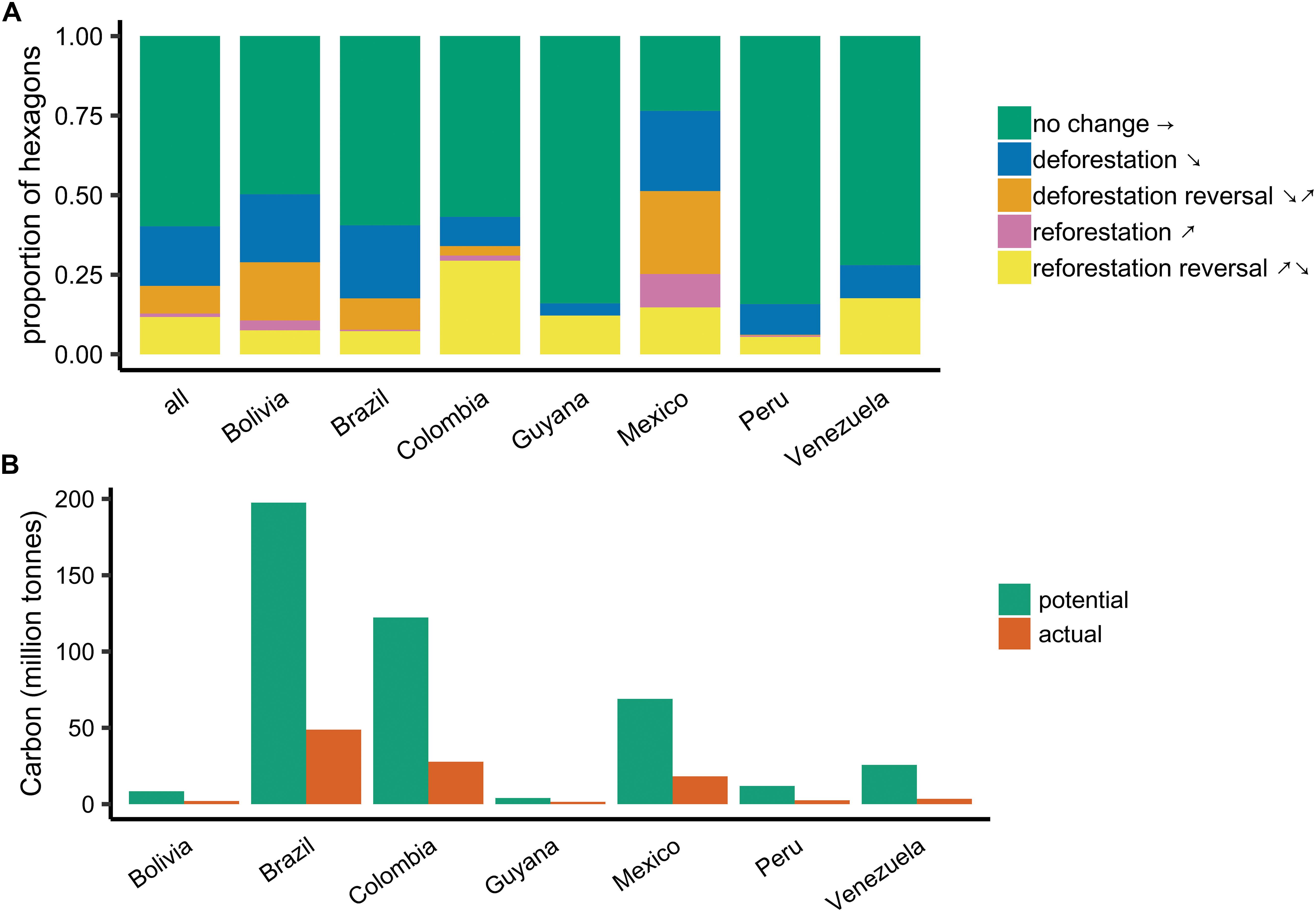
Figure 2. (A) Relative proportions of hexagon forest cover change trajectories across countries and biomes. Seven countries with the largest amount of tropical forest area are shown; see Supplementary Table S1 for data on additional countries. Hexagons that could not be assigned a trajectory with high confidence are omitted. (B) Comparison of carbon sequestration potential without reforestation reversal and estimated actual carbon uptake in seven LAC countries with most tropical forest area.
In regions where reforestation reversals occurred, forest cover increased by a total of 5,983,781 hectares (across hexagons mean = 9.0%, SD = 9.2%) before it began to decline. By 2014, only 31% (1,832,394 hectares) of these new forests remained. Although most of the reforestation reversal regions still experienced a net increase in forest cover between 2001 and 2014 (mean = 1.8%, sd = 5.8%; Supplementary Figure S2), these gains were significantly smaller than in regions where reforestation was sustained (mean = 9.7%, SD = 9.5%, Supplementary Figure S2, t = 6.9, p < 0.0001). Where deforestation reversals occurred, 61.8% of hexagons underwent net reforestation relative to 2001. Across deforestation reversal hexagons, forest cover increased by a net total of 1,945,119 hectares relative to forest cover in 2001 (across hexagons mean = 2.2%, SD = 7.6%).
We found significant differences in the distribution of forest cover change trajectories across countries (Figure 2A, χ2 = 673.99, p < 0.0001). Reforestation reversals were relatively rare in Bolivia (7.5% of hexagons) and Brazil (7.3%), where deforestation and deforestation reversals were more common, and in Peru (5.5%), where forest cover is still high in remote regions. Colombia and Venezuela had the highest rates of reforestation reversals, at 29.4 and 17.6% of hexagons, respectively.
Effects of Reforestation Reversals on Carbon Sequestration
Without reclearing, forests that established between 2001 and 2014 would have sequestered 0.58 Pg C (95% c.i.: [0.09, 1.15]; Figure 3A). Only 0.14 Pg C (95% ci: [0.02, 0.28]) was captured in new forests by the end of the study period (Figure 3B). This gap represents a loss of 76% of the carbon potential of reforestation in Latin America and the Caribbean between 2001 and 2014 (Figure 3C). The magnitude of lost carbon potential differed across countries, ranging from 27% (Guyana) to 87% (Venezuela; Figure 2B).
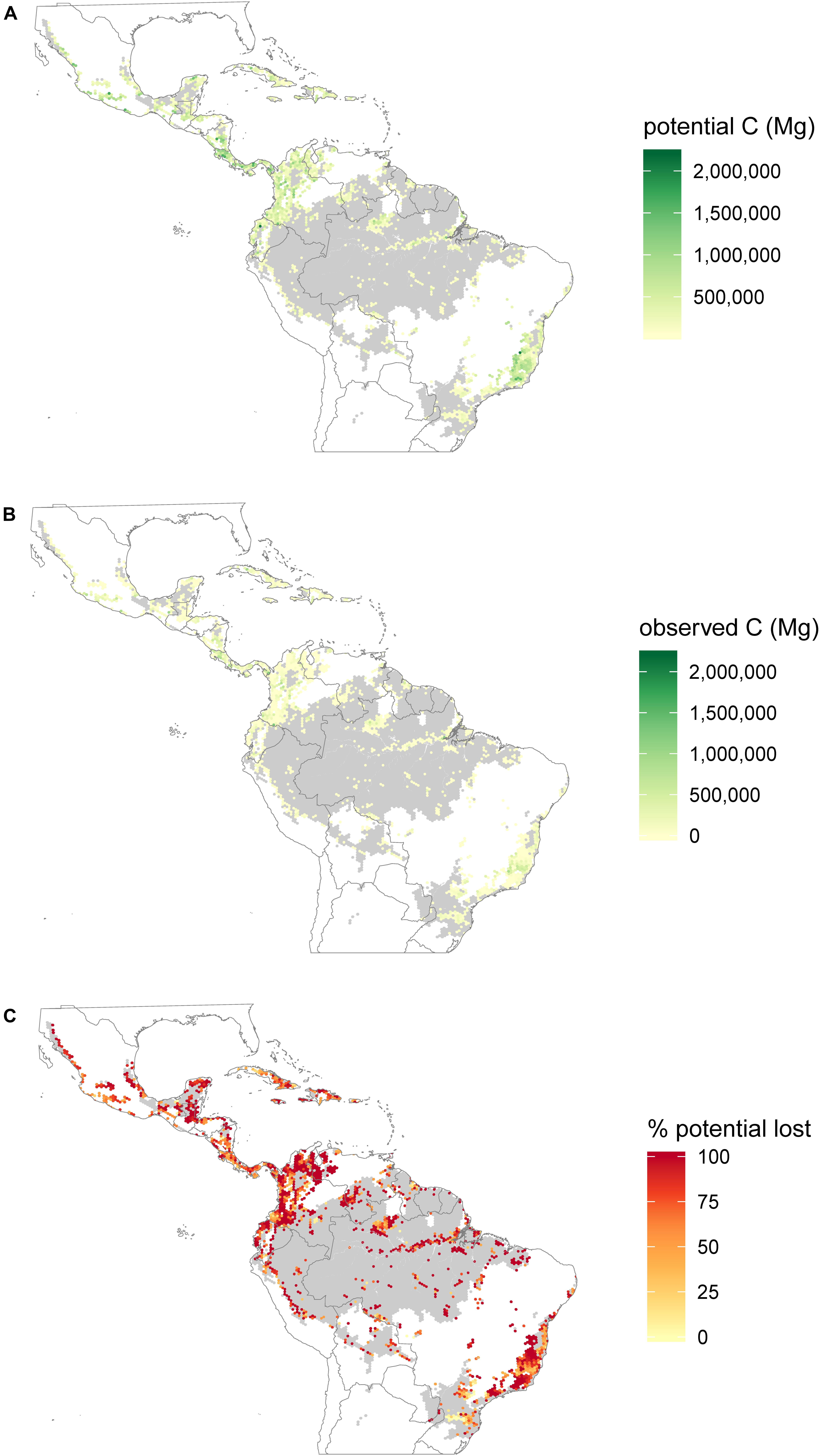
Figure 3. (A) Potential carbon sequestration in new forests, had no re-clearing of reforestation occurred, (B) observed carbon sequestration in new forests, when accounting for re-clearing, and (C) percent of carbon potential foregone due to reversal of reforestation.
Discussion
Our results show that reforestation trends in Latin America and the Caribbean in the early 2000s reversed in many regions, undoing some or all the previous forest cover gains. These reversals severely limit carbon sequestration: we estimate that in their absence, tropical second-growth forests could have sequestered over four times more carbon between 2001 and 2014. This result further calls into question the recent claims that “global tree restoration [is] our most effective climate solution to date” (Bastin et al., 2019). Reversals of reforestation also limit second-growth forests’ contribution to biodiversity conservation, as tropical second-growth forests require a median time of 50 years to recover species richness similar to that of old growth forests (Rozendaal et al., 2019). Without permanence, the contribution of reforestation to climate mitigation and biodiversity conservation will be severely curtailed, though clearing of second-growth forests may ultimately serve to buffer primary forest loss (Wang et al., 2020).
Detection of reforestation reversals in this study was possible only because our methods allowed for flexible trajectories and because we analyzed a multi-date time series of land cover data. Because most reforestation reversals still resulted in net gains in forest cover from 2001 to 2014, a methodological approach to identify binary deforestation/reforestation trends in forest cover would have led to most of these trajectories being classified as reforestation (e.g., Nanni et al., 2019). While this is strictly correct, our method reveals a more complex and dynamic reality, where short-term increases in forest cover do not necessarily indicate persistent trends. Reforestation and deforestation, which occurred in 19.9% of hexagons, were no more common than the more dynamic categories of reforestation reversals or deforestation reversals (20.4%). Together with recent findings of cyclical “reforestation treadmills”’ in planted areas in tropical regions (Sloan et al., 2019), our results contribute to a growing body of evidence that suggests that fitting linear trends or calculating net changes across two dates can mask important dynamics at shorter time scales. Detailed land cover time series and non-linear methods are essential for better understanding land cover dynamics. Furthermore, our conceptual models must move beyond classical conceptions of forest transition theory where forest cover increases are assumed to be sustained (Mather, 1992).
Several methodological limitations add uncertainty to our results. First, we used land cover data derived from MODIS imagery (6.25 hectare pixels). This relatively large pixel size may result in omission of some second-growth forests from our dataset, as many second-growth forest patches are quite small (less than one hectare; Schwartz et al., 2017a). These small patches comprising mixed pixels with other land cover types might not be detected by the classification, especially during early stages of growth when canopies are open and short. Overlooking these young forests and small patches might bias our estimates of carbon storage of second growth forest. However, our estimates of carbon potential lost are likely conservative since smaller and younger patches are more prone to reclearing (Schwartz et al., 2017b; Reid et al., 2018).
Second, our analysis was not able to classify all land-cover time series as one of the candidate shapes. Specifically, about 30% of hexagons were assigned the “low confidence” trajectory. This class likely reflects noisiness in the underlying classification as it was common in regions where, according to a previous study with this dataset, our random forest classifier showed lower rates of internal agreement (Clark et al., 2012). This class could also occur in places that experienced frequent, cyclical disturbances during the study period, though it is not possible to distinguish between these scenarios with this dataset. Local-scale analyses of forest permanence and future improvements to land cover data products will help resolve some of these uncertainties.
Some reforestation is ephemeral by nature. Specifically, activities such as timber harvesting and shifting cultivation involve cyclical reforestation and deforestation dynamics (Rudel et al., 2016). We expect these activities would lead to cycles on local scales – e.g., on individual fields or within forest stands. Rather than reflecting these inherently cyclical activities, our results reflect landscape- to regional-scale trends since we aggregated land cover pixels to 1200 km2 hexagons. This aggregation may mask important local-scale dynamics such as simultaneous deforestation and reforestation, which would result in no net change in aggregated forest cover. Though beyond the scope of this study, further research into the prevalence of these dynamics and the drivers of reforestation and clearing at the pixel scale (e.g., Schwartz et al., 2017b; Nunes et al., 2020) could help better understand regional trends in forest cover and improve estimates of carbon sequestration through reforestation.
Variation in the prevalence of reforestation reversals across countries highlights the strong influence of regional and local context on land cover dynamics. For example, the high rates of reforestation reversals observed in Colombia and Venezuela likely reflect complex political, social, and economic realities. Much of the reforestation reversal in Colombia occurred in the Andes. There, during the early part of the study period, woody vegetation cover increased, associated with rural-to-urban migration and land abandonment driven by violence and conflict (Sánchez-Cuervo et al., 2012). But, as the peace process progressed (2007–2014) much of this reforestation reversed. More recently, rates of deforestation have accelerated, particularly in the lowlands. These trends suggest that the post-conflict peace process, including rural development incentives and the government’s difficulties establishing a presence in remote regions, has initiated new land-use dynamics (Hettler et al., 2017; Armenteras et al., 2019; Clerici et al., 2019).
In Venezuela, deforestation has been associated with large dam projects (Sy et al., 2015), oil exploration (Richards and VanWey, 2015; de Cárdenas García, 2017) and gold mining in the Orinoco basin. Reforestation reversals in Venezuela could be linked to food shortages, though how recent political and economic challenges have influenced land-use change in Venezuela requires further research. Conventional forest transition models assume a relatively constant transition toward reforestation as countries develop (Mather, 1992; Mather and Needle, 1998) but dramatic political changes may substantially alter this pattern, in particular if considering finer temporal scales. The cases of Colombia and Venezuela illustrate that political instability (or transitions from instability toward stability) may have profound effects in land cover trajectories. More generally, the socioeconomic conditions that favor reforestation may prove to be ephemeral in the face of economic crises, political unrest, and other shifts.
Many LAC nations have committed to reforestation and forest restoration under the Bonn challenge, a global effort to restore 150 million hectares of degraded land by 20202. Colombia has pledged 1 million hectares forest restoration (0.09 Pg C sequestration), while Brazil has pledged 12 million hectares (1.14 Pg). Within this context, our findings represent substantial foregone carbon sequestration, larger than Colombia’s and nearly half Brazil’s Bonn challenge commitments. The Bonn Challenge commitments involve establishment of new forests either through restoration or natural regeneration, but notably do not include strong provisions to ensure permanence. Our results suggest that newly established forests in LAC are often ephemeral and at risk of clearing, especially during periods of political transition and/or instability. For example, one of the first acts of the newly elected Brazilian government in 2019 was to limit enforcement of the Brazilian forest code, which would remove protection on up to 15 million hectares of forest (Freitas et al., 2018).
How to best improve permanence of tropical second-growth forests is not clear, especially given land-use tradeoffs and growing demand for agricultural land (Lambin and Meyfroidt, 2011) and the complex tradeoffs and relationships between second-growth and primary forest clearing (e.g., Wang et al., 2020). To date, limited research has focused on policies and practices to enhance permanence of second-growth forests (but see Chazdon et al., 2020). Further research into the drivers of reforestation reversals and permanence could help guide development of policies and management practices to better protect second-growth forests. Permanence can and should be incorporated into restoration planning from the earliest stages. For example, a recent study identifying restoration opportunities in tropical forest landscapes included a variable to represent the chances that restored forests persist over time (Brancalion et al., 2019). Explicit plans to promote permanence of restored or naturally regenerated forests will be necessary to achieve the Bonn Challenge goals, and for tropical second-growth forests to make a substantial contribution to climate change mitigation.
Data Availability Statement
The data analyzed in this study are available at doi: 10.6084/m9.figshare.12502022.v1.
Author Contributions
NS, TA, HG, and MU contributed to the conception and design of the study. JG analyzed the remote sensing imagery and developed the database. NS performed the statistical analysis and wrote the first draft of the manuscript. NS, TA, and HG wrote sections of the manuscript. All authors contributed to the manuscript revision, read and approved the submitted version.
Funding
This project was partially funded by a grant from the Dynamics of Coupled Natural and Human Systems program of the U.S. National Science Foundation (# 0709598) to TA. NS acknowledges support from NSF PRFB 1711366.
Conflict of Interest
The authors declare that the research was conducted in the absence of any commercial or financial relationships that could be construed as a potential conflict of interest.
The handling Editor declared a past co-authorship with several of the authors NS and MU.
Supplementary Material
The Supplementary Material for this article can be found online at: https://www.frontiersin.org/articles/10.3389/ffgc.2020.00085/full#supplementary-material
Footnotes
References
Aide, T. M., Clark, M. L., Grau, H. R., López-Carr, D., Levy, M. A., Redo, D., et al. (2013). Deforestation and reforestation of Latin America and the Caribbean (2001-2010). Biotropica 45, 262–271. doi: 10.1111/j.1744-7429.2012.00908.x
Aide, T. M., Grau, H. R., Graesser, J., Andrade-Nuñez, M. J., Aráoz, E., Barros, A. P., et al. (2019). Woody vegetation dynamics in the tropical and subtropical Andes from 2001 to 2014: satellite image interpretation and expert validation. Glob. Chang. Biol. 25, 2112–2126. doi: 10.1111/gcb.14618
Algeet-Abarquero, N., Sanchez-Azofeifa, A., Bonatti, J., and Marchamalo, M. (2015). Land cover dynamics on Osa Region, Costa Rica: secondary forest is here to stay. Reg. Env. Chang. 15, 1461–1472. doi: 10.1007/s10113-014-0714-9
Armenteras, D., Schneider, L., and Dávalos, L. M. (2019). Fires in protected areas reveal unforeseen costs of Colombian peace. Nat. Ecol. Evol. 3, 20–23.
Asner, G. P., Rudel, T. K., Aide, T. M., Defries, R., and Emerson, R. (2009). A contemporary assessment of change in humid tropical forests. Conserv. Biol. 23, 1386–1395. doi: 10.1111/j.1523-1739.2009.01333.x
Bastin, J.-F., Finegold, Y., Garcia, C., Mollicone, D., Rezende, M., Routh, D., et al. (2019). The global tree restoration potential. Science 365, 76–79. doi: 10.1126/science.aax0848
Brancalion, P. H. S., Niamir, A., Broadbent, E., Crouzeilles, R., Barros, F. S. M., Almeyda Zambrano, A. M., et al. (2019). Global restoration opportunities in tropical rainforest landscapes. Sci. Adv. 5:eaav3223.
Chave, J., Coomes, D., Jansen, S., Lewis, S. L., Swenson, N. G., and Zanne, A. E. (2009). Towards a worldwide wood economics spectrum. Ecol. Lett. 12, 351–366. doi: 10.1111/j.1461-0248.2009.01285.x
Chazdon, R., Lindenmayer, D., Guariguata, M. R., Crouzeilles, R., Rey Benayas, J. M., and Lazos Chavero, E. (2020). Fostering natural forest regeneration on former agricultural land through economic and policy interventions. Environ. Res. Lett. 15:043002. doi: 10.1088/1748-9326/ab79e6
Chazdon, R. L., Broadbent, E. N., Rozendaal, D. M. A., Bongers, F., Zambrano, A. M. A., Aide, T. M., et al. (2016). Carbon sequestration potential of second-growth forest regeneration in the Latin American tropics. Sci. Adv. 2:e1501639. doi: 10.1126/sciadv.1501639
Clark, M. L., and Aide, T. M. (2011). Virtual interpretation of Earth Web-Interface Tool (VIEW-IT) for collecting land-use/land-cover reference data. Remote Sens. 3, 601–620. doi: 10.3390/rs3030601
Clark, M. L., Aide, T. M., and Riner, G. (2012). Land change for all municipalities in Latin America and the Caribbean assessed from 250-m MODIS imagery (2001-2010). Remote Sens. Environ. 126, 84–103. doi: 10.1016/j.rse.2012.08.013
Clerici, N., Salazar, C., Pardo-Díaz, C., Jiggins, C. D., Richardson, J. E., and Linares, M. (2019). Peace in Colombia is a critical moment for Neotropical connectivity and conservation: save the northern Andes-Amazon biodiversity bridge. Conserv. Lett. 12:e12594. doi: 10.1111/conl.12594
de Cárdenas García, J. (2017). The new integrated oil service contracts in a Venezuela in dire straits. J. Energy Nat. Resour. Law 35, 417–431. doi: 10.1080/02646811.2017.1371412
DeFries, R., and Pandey, D. (2010). Urbanization, the energy ladder and forest transitions in India’s emerging economy. Land use policy 27, 130–138. doi: 10.1016/j.landusepol.2009.07.003
Freitas, F. L. M., Sparovek, G., Berndes, G., Persson, U. M., Englund, O., Barretto, A., et al. (2018). Potential increase of legal deforestation in Brazilian Amazon after Forest Act revision. Nat. Sustain. 1, 665–670.
Fuss, S., Canadell, J. G., Peters, G. P., Tavoni, M., Andrew, R. M., Ciais, P., et al. (2014). Betting on negative emissions. Nat. Clim. Chang. 4, 850–853. doi: 10.1038/nclimate2392
Graesser, J., Aide, T. M., Grau, H. R., and Ramankutty, N. (2015). Cropland/pastureland dynamics and the slowdown of deforestation in Latin America. Environ. Res. Lett. 10:034017. doi: 10.1088/1748-9326/10/3/034017
Grau, H. R., and Aide, M. (2008). Globalization and land-use transitions in Latin America. Ecol. Soc. 13:16.
Hansen, M. C. C., Potapov, P. V., Moore, R., Hancher, M., Turubanova, S. A. A., Tyukavina, A., et al. (2013). High-resolution global maps of 21st-Century forest cover change. Science 342, 850–853. doi: 10.1126/science.1244693
Hecht, S. B., Kandel, S., Gomes, I., Cuellar, N., and Rosa, H. (2006). Globalization, forest resurgence, and environmental politics in El Salvador. World Dev. 34, 308–323. doi: 10.1016/j.worlddev.2005.09.005
Hettler, B., Thieme, A., and Finer, M. (2017). Deforestation Patterns in the Colombian Amazon. Available online at: https://maaproject.org/maap-deforestation-patterns-colombian-amazon/ (accessed June 13, 2020).
Kindermann, G., Obersteiner, M., Sohngen, B., Sathaye, J., Andrasko, K., Rametsteiner, E., et al. (2008). Global cost estimates of reducing carbon emissions through avoided deforestation. Proc. Natl. Acad. Sci. U.S.A. 105, 10302–10307. doi: 10.1073/pnas.0710616105
Lambin, E. F., and Meyfroidt, P. (2011). Global land use change, economic globalization, and the looming land scarcity. Proc. Natl. Acad. Sci. U.S.A. 108, 3465–3472. doi: 10.1073/pnas.1100480108
Lewis, S. L., Wheeler, C. E., Mitchard, E. T. A., and Koch, A. (2019). Regenerate natural forests to store carbon. Nature 568, 25–28.
Locatelli, B., Catterall, C. P., Imbach, P., Kumar, C., Lasco, R., Marín-Spiotta, E., et al. (2015). Tropical reforestation and climate change: beyond carbon. Restor. Ecol. 23, 337–343. doi: 10.1111/rec.12209
Mather, A. S., and Needle, C. (1998). The forest transition: a theoretical basis. Area 30, 117–124. doi: 10.1111/j.1475-4762.1998.tb00055.x
Meyfroidt, P., and Lambin, E. F. (2011). Global forest transition: prospects for an end to deforestation. Annu. Rev. Environ. Resour. 36, 343–371. doi: 10.1146/annurev-environ-090710-143732
Moisen, G. G., Meyer, M. C., Schroeder, T. A., Liao, X., Schleeweis, K. G., Freeman, E. A., et al. (2016). Shape selection in Landsat time series: a tool for monitoring forest dynamics. Glob. Chang. Biol. 22, 3518–3528. doi: 10.1111/gcb.13358
Nanni, A. S., Sloan, S., Aide, T. M., Graesser, J., Edwards, D., and Grau, H. R. (2019). The neotropical reforestation hotspots: a biophysical and socioeconomic typology of contemporary forest expansion. Glob. Environ. Chang. 54, 148–159. doi: 10.1016/j.gloenvcha.2018.12.001
Nunes, S., Oliveira, L., Siqueira, J., Morton, D. C., and Souza, C. M. (2020). Unmasking secondary vegetation dynamics in the Brazilian Amazon. Environ. Res. Lett. 15:034057. doi: 10.1088/1748-9326/ab76db
Olson, D. M., Dinerstein, E., Wikramanayake, E. D., Burgess, N. D., Powell, G. V. N., Underwood, E. C., et al. (2001). Terrestrial ecoregions of the World: a new map of life on Earth. Bioscience 51:933. doi: 10.1641/0006-3568(2001)051
Poorter, L., Bongers, F., Aide, T. M., Almeyda Zambrano, A. M., Balvanera, P., Becknell, J. M., et al. (2016). Biomass resilience of Neotropical secondary forests. Nature 530, 211–214. doi: 10.1038/nature16512
Redo, D. J., Grau, H. R., Aide, T. M., and Clark, M. L. (2012). Asymmetric forest transition driven by the interaction of socioeconomic development and environmental heterogeneity in Central America. Proc. Natl. Acad. Sci. U.S.A. 109, 8839–8844. doi: 10.1073/pnas.1201664109
Reid, J. L., Fagan, M. E., Lucas, J., Slaughter, J., and Zahawi, R. A. (2018). The ephemerality of secondary forests in southern Costa Rica. Conserv. Lett. 12:e12607. doi: 10.1111/conl.12607
Richards, P., and VanWey, L. (2015). Where deforestation leads to urbanization: how resource extraction is leading to urban growth in the Brazilian Amazon. Ann. Assoc. Am. Geogr. 105, 806–823. doi: 10.1080/00045608.2015.1052337
Rozendaal, D. M. A., Bongers, F., Aide, T. M., Alvarez-Dávila, E., Ascarrunz, N., Balvanera, P., et al. (2019). Biodiversity recovery of Neotropical secondary forests. Sci. Adv. 5:eaau3114. doi: 10.1126/sciadv.aau3114
Rudel, T. K., Coomes, O. T., Moran, E., Achard, F., Angelsen, A., Xu, J., et al. (2005). Forest transitions: towards a global understanding of land use change. Glob. Environ. Chang. 15, 23–31. doi: 10.1016/j.gloenvcha.2004.11.001
Rudel, T. K., Perez-Lugo, M., and Zichal, H. (2000). When fields revert to forest: development and spontaneous reforestation in post-war Puerto Rico. Prof. Geogr. 52, 386–397. doi: 10.1111/0033-0124.00233
Rudel, T. K., Sloan, S., Chazdon, R., and Grau, R. (2016). The drivers of tree cover expansion: global, temperate, and tropical zone analyses. Land Use Policy 58, 502–513. doi: 10.1016/j.landusepol.2016.08.024
Sánchez-Cuervo, A. M., and Aide, T. M. (2013). Consequences of the armed conflict, forced human displacement, and land abandonment on forest cover change in colombia: a multi-scaled analysis. Ecosystems 16, 1052–1070.
Sánchez-Cuervo, A. M., Aide, T. M., Clark, M. L., and Etter, A. (2012). Land cover change in Colombia: surprising forest recovery trends between 2001 and 2010. PLoS One. 7:e43943. doi: 10.1371/journal.pone.0043943
Schwartz, N. B., Uriarte, M., DeFries, R., Bedka, K. M., Fernandes, K., Gutierrez-Velez, V., et al. (2017a). Fragmentation increases wind disturbance impacts on forest structure and carbon stocks in a western Amazonian landscape. Ecol. Lett. 27, 1901–1915. doi: 10.1002/eap.1576
Schwartz, N. B., Uriarte, M., Defries, R., Gutierrez-velez, V. H., and Pinedo-, M. A. (2017b). Land-use dynamics influence estimates of carbon sequestration potential in tropical second-growth Land-use dynamics influence estimates of carbon sequestration potential in tropical second-growth forest. Environ. Res. Lett. 12:074023. doi: 10.1088/1748-9326/aa708b
Sloan, S. (2008). Reforestation amidst deforestation: simultaneity and succession. Glob. Environ. Chang. 18, 425–441. doi: 10.1016/j.gloenvcha.2008.04.009
Sloan, S., Meyfroidt, P., Rudel, T. K., Bongers, F., and Chazdon, R. (2019). The forest transformation: planted tree cover and regional dynamics of tree gains and losses. Glob. Environ. Chang. 59:101988.
Song, X. P., Hansen, M. C., Stehman, S. V., Potapov, P. V., Tyukavina, A., Vermote, E. F., et al. (2018). Global land change from 1982 to 2016. Nature 560, 639–643. doi: 10.1038/s41586-018-0411-9
Strassburg, B. B. N., Beyer, H. L., Crouzeilles, R., Iribarrem, A., Barros, F., de Siqueira, M. F., et al. (2019). Strategic approaches to restoring ecosystems can triple conservation gains and halve costs. Nat. Ecol. Evol. 3, 62–70. doi: 10.1038/s41559-018-0743-8
Suding, K. N., Gross, K. L., and Houseman, G. R. (2004). Alternative states and positive feedbacks in restoration ecology. Trends Ecol. Evol. 19, 46–53. doi: 10.1016/j.tree.2003.10.005
Sy, V. D., Herold, M., Achard, F., Beuchle, R., Clevers, J. G. P. W., Lindquist, E., et al. (2015). Land use patterns and related carbon losses following deforestation in South America. Environ. Res. Lett. 10:124004. doi: 10.1088/1748-9326/10/12/124004
van Vuuren, D. P., Deetman, S., van Vliet, J., van den Berg, M., van Ruijven, B. J., and Koelbl, B. (2013). The role of negative CO2 emissions for reaching 2 °C—insights from integrated assessment modelling. Clim. Change 118, 15–27. doi: 10.1007/s10584-012-0680-5
Keywords: deforestation, reforestation, second growth forests, forest persistence, carbon sequestration and storage, tropical forest, land-use change
Citation: Schwartz NB, Aide TM, Graesser J, Grau HR and Uriarte M (2020) Reversals of Reforestation Across Latin America Limit Climate Mitigation Potential of Tropical Forests. Front. For. Glob. Change 3:85. doi: 10.3389/ffgc.2020.00085
Received: 01 May 2020; Accepted: 18 June 2020;
Published: 14 July 2020.
Edited by:
Catarina C. Jakovac, Wageningen University and Research, NetherlandsReviewed by:
Robin L. Chazdon, University of the Sunshine Coast, AustraliaPaula Meli, University of La Frontera, Chile
Copyright © 2020 Schwartz, Aide, Graesser, Grau and Uriarte. This is an open-access article distributed under the terms of the Creative Commons Attribution License (CC BY). The use, distribution or reproduction in other forums is permitted, provided the original author(s) and the copyright owner(s) are credited and that the original publication in this journal is cited, in accordance with accepted academic practice. No use, distribution or reproduction is permitted which does not comply with these terms.
*Correspondence: Naomi B. Schwartz, bmFvbWkuc2Nod2FydHpAdWJjLmNh