- Vidarium – Nutrition, Health and Wellness Research Center, Grupo Empresarial Nutresa, Medellín, Colombia
Next-generation sequencing technologies have found a widespread use in the study of host–microbe interactions due to the increase in their throughput and their ever-decreasing costs. The analysis of human-associated microbial communities using a marker gene, particularly the 16S rRNA, has been greatly benefited from these technologies – the human gut microbiome research being a remarkable example of such analysis that has greatly expanded our understanding of microbe-mediated human health and disease, metabolism, and food absorption. 16S studies go through a series of in vitro and in silico steps that can greatly influence their outcomes. However, the lack of a standardized workflow has led to uncertainties regarding the transparency and reproducibility of gut microbiome studies. We, here, discuss the most common challenges in the archetypical 16S rRNA workflow, including the extraction of total DNA, its use as template in PCR with primers that amplify specific hypervariable regions of the gene, amplicon sequencing, the denoising and removal of low-quality reads, the detection and removal of chimeric sequences, the clustering of high-quality sequences into operational taxonomic units, and their taxonomic classification. We recommend the essential technical information that should be conveyed in publications for reproducibility of results and encourage non-experts to include procedures and available tools that mitigate most of the problems encountered in microbiome analysis.
Introduction
The gut microbiome, our “second genome,” is the most intimate connection we have with the environment. During the last decade, the study of the gut microbiome has revolutionized our understanding of human health and disease, metabolism, and food absorption. This research field has gone beyond being a mere object of study and is now recognized as an object of intervention (1) that may eventually assist in personalized diagnostic assessment, risk stratification, disease prevention, treatment decision-making, and patients’ follow-up (2).
The gut microbiome is the target of therapies for gastrointestinal diseases, such as infection by Clostridium difficile or inflammatory bowel disease, metabolic conditions, such as obesity and diabetes, and non-gastrointestinal pathologies, like allergy and autism (3–5). Dietary manipulation through supplementation with pre- and probiotics, and the modulation of the microbial community with antibiotics or fecal matter transplants have been studied (6, 7) and successfully applied (8). In vitro models that simulate the gastrointestinal tract and that allow the fine tuning of physicochemical conditions have been developed to test the effect of different substances on particular bacterial species or even the whole microbial community (9, 10).
However, understanding how the gut microbiome contributes to the pathogenesis of complex disorders or to nutrient absorption will critically depend upon the accuracy with which we characterize this microbial community. Next-generation sequencing (NGS) technologies (11–13) are currently of wide use to this end because of their capacity to measure non-cultivable organisms, relatively low cost, and high throughput. NGS platforms have allowed measuring microbial diversity with an ever-increasing throughput and read length (14, 15) and at a constantly decreasing cost (16), which has granted the possibility for a new wave of researchers to get involved in projects of considerable size and complexity, to carry sophisticated quantitative evaluations and to study low-abundance microorganisms. The outstanding increase in the number of publications in recent years (2,319 papers published in 2015; source: Scopus) is a proof of this. It raises, nonetheless, questions about how aware all these researchers are about pitfalls in microbiome analyses.
One of the most used ways to examine the gut microbiome is to use a marker gene or barcode to identify microorganisms and reconstruct their phylogenetic relationships; the 16S rRNA gene is the most used for that purpose, although others have been proposed and used (17–19). As shown in Figure 1, most 16S studies follow a common workflow (20): total DNA is extracted from a sample (e.g., feces in the case of the gut microbiome) and used as template in PCR with primers that amplify specific regions of the 16S rRNA gene; the PCR products are sequenced using any technology (formerly Sanger but more recently NGS platforms, such as Roche 454, Illumina, Ion Torrent, PacBio) and raw sequences are processed using bioinformatic pipelines that include the denoising and removal of low-quality reads, the detection and removal of chimeric sequences, the clustering of the curated sequences into operational taxonomic units (OTUs), and their taxonomic classification. The output data can then be used to perform ecological and statistical tests (e.g., α and β diversity analyses). A careless execution of any single procedure in the workflow and the cumulative effect of the inherent bias of each step, which can be reduced but not totally eradicated as we shall see, can result in a biased representation of the microbial community under study or erroneous estimations of the changes induced by interventions.
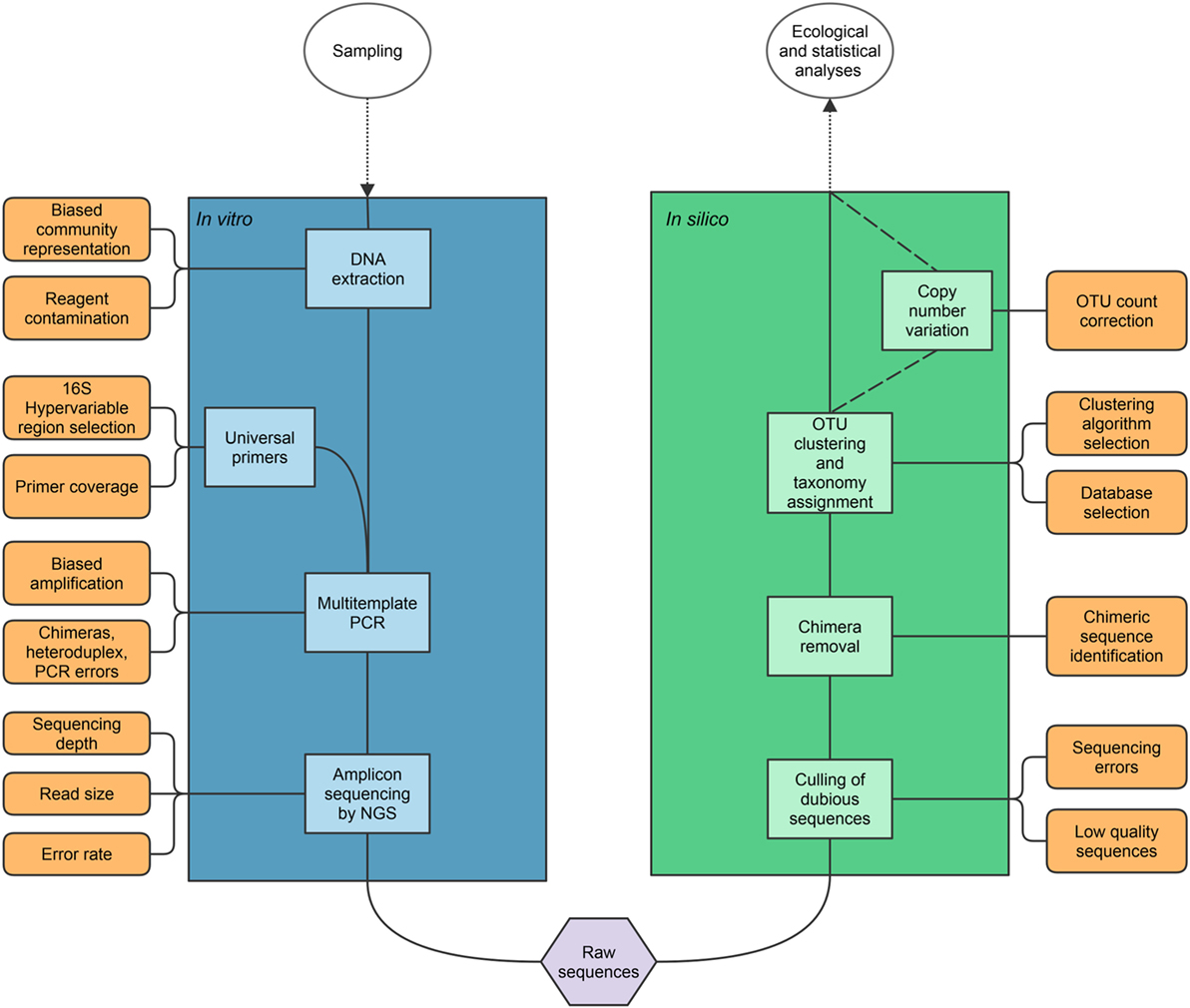
Figure 1. Schematic view of the archetypical workflow in 16S rRNA studies, and some of the problems associated with each step. Dotted lines link the workflow with steps beyond the scope of the review, and dashed lines represent non-standard steps.
The unification of analysis procedures and the implementation of standardized workflows in order to minimize the variation introduced to the results have been recurrent topics on symposia (21), editorials (22), and opinion papers (23, 24). We, here, go over each step in the workflow of an archetypical 16S study, from DNA extraction to the generation and classification of OTUs, briefly explain their principles, draw attention to their potential biases and propose some solutions to (reasonably) mitigate them, including available software tools. In addition, we highlight instances where direct comparisons between studies are discouraged and recommend the essential information that should be included when describing a microbiome study for reproducibility of results.
While some of the issues discussed here have been separately reviewed elsewhere [benefits and problems of barcode sequencing (36), primer selection (37), DNA extraction and PCR biases (38), sequence curation (39), taxonomic classification (40)], they have frequently been overlooked in publications of original datasets. We wish to encourage newcomer scientists to implement rigorous analyses so that they get confident results that better represent the microbial communities under scrutiny. Upstream and downstream procedures, namely, experimental design and sample collection, calculation of diversity indices, rarefaction curves, hypothesis testing, and other ecological and statistical analyses are of the uttermost importance; however, they vary between different kinds of studies and are beyond the scope of this paper. They have been reviewed elsewhere (41–45).
DNA Extraction
The first step, once the samples are collected, is the extraction of total DNA, which will then be used as template for PCR amplification of the marker gene. After the DNA is extracted and purified, the workflow for most 16S studies becomes roughly the same. Fecal samples are composed of microorganisms that differ in characteristics, such as size and cell wall composition, and that are present in different proportions. This can make the purification of a DNA sample that accurately represents the original community (i.e., that keeps all species and their abundances at the same relative proportions) a challenge, as different sample handling and DNA extraction protocols can yield samples with different bacterial ratios. It has been shown, for instance, that frozen fecal samples yield a higher amount of DNA from Gram-positive than from Gram-negative bacteria, probably due to the effect that the freeze–thaw cycle can have over the Gram-positive cell wall (46).
Differences in gut microbial community patterns can also arise due to the principles of the genetic material extraction protocols, causing the over or underrepresentation of the same microbial group in DNA extracted from subsamples of the same source (47). Some DNA extraction kits use bead-containing lysing matrices and vigorous shaking steps that contribute to the disruption of the cell wall, whereas others rely on chemical lysis (48). Several studies have consistently demonstrated that protocols that involve a bead-beating step yield higher quantities of bacterial DNA, and, most importantly, these samples tend to be a more comprehensive representation of the microbial community, regardless of the source material and analysis method (49–51). The differences between subsamples extracted with different kits can even be statistically significant, which is why it has been suggested that data from studies using different extraction methods should not be compared (52). Opportunely, studies are increasingly using similar DNA extraction protocols. For instance, the PowerSoil® DNA isolation kit (MoBio) has become popular because it performs well in a wide variety of samples, including human feces. Although using the same extraction protocol does not guarantee accurate representation of the microbial community under study, it allows comparison among studies.
Another issue with DNA extraction is that, due to the non-specificity of marker gene and metagenomic assays, they are highly sensitive to contamination with foreign microbial DNA. The presence of bacterial DNA from sources other than the original sample can alter the outcome of the analysis in a way that it no longer mirrors the original community it is supposed to reflect. Contamination sources may include the PCR reagents (53, 54), ultra pure water (55, 56), and, even, the DNA extraction reagents (57, 58). The genetic material extracted from samples with low biomass is more prone to being drowned by contaminant DNA (59, 60), and the contamination profile varies between laboratories, extraction kits, and batches from the same kit (60). Procedures to reduce the effect of contamination include the maximization of starting biomass from which DNA is extracted, the randomization of the order in which samples are to be processed, the collection, processing and sequencing of technical controls of the reagents to be used (storage media, DNA extraction kits, and PCR kits), the recording of the kit lots as additional metadata, and the quantification of negative-control sequences (60).
Today, there is no standard procedure on how to deal with sequences showing up in technical controls. One suggestion would be to compare the abundance in real samples and controls: if an OTU has similar relative abundance in samples and controls, it is likely a contaminant; otherwise, it probably is not. This approach has the drawback that the threshold in which the abundance of an OTU is considered a contaminant is subjective (61). Another method involves the removal of OTUs whose abundance is negatively correlated with amplicon concentration, as it is assumed that the signal from contaminant sequences in low biomass samples is less likely to be drowned by the signal of real data (61). In any case, it is necessary to be aware of taxa that are present in negative controls, taxa statistically associated with a particular batch of reagents, and taxa biologically unexpected in the treated samples.
Multi-Template PCR
In marker gene studies, total DNA is used as template for the PCR amplification of the barcode region. As in single-template PCR, the efficiency of multi-template PCR is influenced by the GC content of the target region (62), the DNA concentration (63), and the thermocycling conditions (64). However, because of the multiorganismal origin of the gut microbiome, a series of particular difficulties and artifacts, such as primer mismatches, gene copy number variation (CNV), chimeras, heteroduplex, and skewed template-to-product ratios, are encountered and can distort the diversity measures. Primer selection, CNV normalization, and chimeric sequence removal are discussed below; for a detailed discussion of reagents and PCR conditions in multi-template assays, see Ref. (65).
16S rRNA Gene Hypervariable Regions
Due to its ubiquity in prokaryotes, low horizontal gene transfer, and ability to differentiate closely related organisms, the 16S rRNA gene has been used for decades in the study of diversity and ecology of microorganisms (66–68). However, most NGS platforms are not capable of covering the full length of the gene (ca. 1,500 bp) (68). This is why short regions within the gene (e.g., hypervariable V1–V9 regions) have been prioritized with the advent of these newer technologies (69). Hypervariable regions are supposed to act as proxies of the complete gene. Actually, there is correlation between the phylogenies generated using different hypervariable regions or combinations thereof and the phylogenies generated with the whole gene (69), but the strength of these correlations varies among regions (70) because their different evolutionary rates limit their capacity to serve as surrogates of full-length sequences (71, 72). Because of these disparities, the OTU count of different 16S regions can be inconsistent (70, 73), which, in turn, makes studies using different hypervariable regions incomparable (71). Currently, there is no consensus of which region best reflects the gut microbial community (69, 74, 75). While read length increases in newer NGS technologies, one empirical way to overcome comparability between studies would be to sequence the same hypervariable region. This is, indeed, what is seen in many gut microbiome studies today: since the Illumina MiSeq platform gives one of the bests value for money of all NGS, most microbiome researchers are moving to sequence the V4 region since its size (ca. 250 bp) fits well the read size of this platform at its current version.
Primer Selection
In order to amplify the selected 16S hypervariable region, a set of broad-range primers (so-called “universal primers”) must be used. These primers are usually designed to hybridize with the conserved regions flanking the sequence of interest. Universal primers work under the assumption that the flanking regions are conserved among a wide range of microbial groups, which allows the correct annealing and amplification of the desired PCR product (76). The rationale behind this approach is as good as possible but it still has problems, as mutations also occur within the flanking regions. The use of primers with a suboptimal coverage rate can lead to selective amplification of the template DNA, that is, the sub-representation or selection against a given microbial group (77). Thus, the relative content of sequences may be modified, resulting in a deviation from the true gut-community composition (77–79).
In short, studies evaluating biases introduced by primer selection have demonstrated that there is no such thing as a truly “universal primer,” since there is no single pair of primers that can be used to amplify all prokaryotic or even bacterial groups. Genome evolution being what it is, the practical way to overcome this limitation and compare results among studies is to use similar pairs of primers and allow for degenerate sites in them. This is the preferred approach in some recent studies that make extensive use of modified 515F (5′ GTGYCAGCMGCCGCGGTAA 3′) and 806R (5′ GGACTACNVGGGTWTCTAAT 3′) primers that amplify the V4 region (80–82).
Amplicon Sequencing by NGS
Next-generation sequencing technologies refer to various strategies that rely on a combination of template preparation, sequencing and imaging, and genome alignment and assembly methods (83). The major advance offered by NGS is the ability to produce an enormous volume of data cheaply and fast. The transition from Sanger to NGS has opened new horizons in the gut-microbiome field by making it possible to collect millions of sequences, spanning hundreds of samples (80). A good example of this is the Human Microbiome Project, which used NGS to characterize the diversity of bacteria, archaea, and viruses that inhabit various areas of the human body in several hundreds healthy individuals (84). In the last decade, the throughput of NGS technologies has dramatically increased, and the operation cost has reduced, which, in turn, has boosted its use in microbial studies. However, the major drawback of all NGS technologies is that they raise concerns regarding the quality of data.
When sequencing genomes, multiple reads are used to construct a consensus and the error rate, defined as the number of errors per total base call (25), is, thus, reduced since each nucleotide in the original sequence is called several times by different reads. Such approach cannot be used when sequencing marker gene amplicons, such as the 16S rRNA, because each individual read is considered an identifier of an independent organism (e.g., a bacterium), and it is not possible to assemble the amplicon sequences (34); hence, the reduction of the error rate by other means becomes imperative.
One strategy to determine how many errors are introduced at each NGS run consists of sequencing a synthetic mixture of genomic DNA (mock community), comprising several known bacterial species, along with the samples. Reads are compared with a reference database of the marker gene, and errors are identified in pairwise alignments of each experimentally generated sequence relative to the closest reference sequence (25, 32, 34). Sequencing mock communities to assess the error rate of each individual amplicon sequencing run should become a standard step in microbial community analysis (see http://www.hmpdacc.org/HMMC/) (25, 29).
Currently, Roche 454 GS-FLX, Illumina MiSeq, Ion Torrent PGM, and PacBio SMRT are the most used platforms for the study of the gut microbiome (35, 85–87). However, each technology performs differently in the trade-off between read length, sequence throughput, and error rate (Table 1). As mentioned above, since hypervariable regions correlate differently with the whole 16S rRNA gene (88, 89), it is arguably better to sequence shorter reads at greater depths and with lower error rates (e.g., Illumina, Ion Torrent) than longer reads with higher error rates (e.g., PacBio) (34). The former allows the detection of low-abundance microorganisms (90, 91) and the avoidance of unnecessary greater computing times due to the description of non-existent organisms caused by artifactual sequences. Although increased read length usually improves classification, platforms such as PacBio are currently limited by their high sequencing error and low yield of sequencing data relative to the other platforms (34).
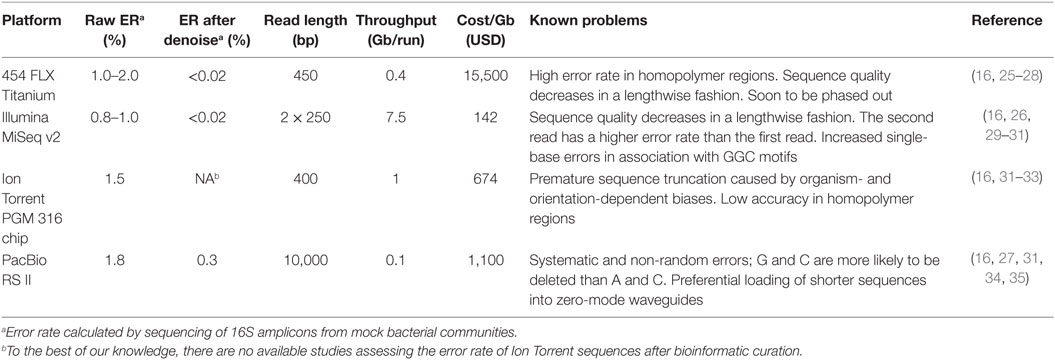
Table 1. Specifications of the most commonly used sequencing platforms in microbial community characterization studies.
Culling of Dubious Sequences
Up to this point, procedures in the archetypical workflow described in Figure 1 take place in vitro. Hereafter, treatment of raw DNA sequences occurs in silico. To reduce sequencing error rates, it has become mandatory to apply stringent sequence curation and denoising algorithms. Inadequate cleaning of reads can have many negative effects including limited ability to identify chimeras and inflation of α and β diversity metrics (92). Low-quality sequences, artifacts, and contamination can compromise the downstream analyses and, thus, must be removed from the dataset.
The first step is the removal of reads with ambiguous base calls (N) in the barcode or in the marker gene amplicon, as it is not possible to determine the true nucleotide sequence (93). On the other hand, mismatches in the primers and barcodes are usually allowed up to a certain number; the removal of sequences with less than three mismatches has little effect on the reduction of the error rate (93). Emulsion-PCR-based platforms (e.g., 454, Ion Torrent) are known for producing homopolymer-associated indel errors (33); these artifacts have been shown to account for a large proportion of errors in benchmark studies using mock communities and to be associated with low-quality scores (92). Therefore, reads with homopolymers longer than eight nucleotides should be culled (25).
In addition, in most sequencing platforms (e.g., 454, Illumina, Ion Torrent), quality scores reduce in a lengthwise fashion, and it is possible to identify breakpoints where the quality criteria are not met. Sequences can be trimmed to those breakpoints to reduce the overall error rate. Two trimming approaches have been widely used: a “hard cutoff” method trims the sequences at the first nucleotide with a quality score below a given threshold (94); this minimizes the error rate but also reduces the average sequence length. Another method, called “sliding window,” calculates the average quality score within a sequence window (or substring) and trims when the average quality score within that window drops below a threshold; the latter method has the advantage that reduces the overall error rate without reducing the average sequence length (25). Reads with anomalous lengths (well above or below the expected value for a given technology) are also removed, as they likely represent PCR or sequencing errors, or become not informative as a result of the quality trimming (93).
The use of a pre-clustering algorithm has also been shown to reduce the number of sequences that are the result of sequencing errors and to predict with higher accuracy the number of expected OTUs in template preparations of known taxonomic composition (95). It assumes that rare sequences are more likely to derive from abundant sequences and can, therefore, be merged if they are within a specified similarity threshold. This threshold must always be lower than the value used for OTU clustering, usually 1% (25).
Also, contaminant sequences must be removed from the dataset. Due to the nature of the 16S rRNA gene, mitochondria, chloroplast (96), and other eukaryotic sequences are likely to be amplified and should be identified and discarded, along with sequences unclassified at the domain level; according to the scope of the study and the primers used, bacterial or archaeal sequences would also be needed to get removed.
Chimera Removal
Sequences composed of two or more parents are named chimeras. Chimeras are a serious concern in studies of the gut microbiome because they can lead to the description of non-existent organisms and inflate diversity metrics. This kind of artifact arises from errors during PCR, and several factors influence its appearance, such as DNA damage (97), the amplification of highly similar sequences (98), a high number of cycles, and short elongation times (99). This suggests that prematurely terminated amplicons that anneal to a homologous template to prime the next PCR cycle are likely to be the major cause of chimera formation.
The detection of chimeras in libraries of 16S amplicons is particularly challenging, as sequences are short and highly similar. There are multiple algorithms designed to detect and remove chimeric sequences (100–107), which follow the same basic principle: substrings or fragments of the query sequence are compared to a set of reference sequences in order to establish if the said substrings match different references. Once a chimera is identified, it is removed from the dataset. Some algorithms use allegedly chimera-free 16S sequence databases as reference, including Chimera Slayer (105) and DECIPHER (108). Others [e.g., Perseus (106), UCHIME (107)] use a database-free approach that assumes that the most abundant sequences from the query dataset are unlikely to be chimeric and can, therefore, be used as reference. Database dependency influences the ability of different algorithms to identify and remove chimeras (109). Database-independent algorithms have the advantage of being able to detect them even if the studied community is poorly described (25). In contrast, database-dependent algorithms rely on reference collections that only contain gene sequences from cultured bacteria and are not expected to perform as well on samples that contain sequences from yet uncultured organisms (24), something very common in studies of the gut microbiome. Thus, the use of algorithms that do not rely on databases should be preferred in order to minimize the inflation of diversity caused by chimeras, especially when dealing with poorly characterized gut microbial communities.
OTU Clustering and Taxonomy Assignment
Sequence Grouping
In order to describe and compare gut microbiomes or shifts in the gut microbiome following intervention, diversity metrics should be estimated (e.g., Chao-1, UniFrac), which requires information about the composition and abundance of organisms in said communities. Currently, two approaches are used to characterize microbial communities: taxonomic-dependent (also called phylotype analysis) and OTU-based methods (110).
The taxonomic-dependent methods rely on reference databases of full-length 16S rRNA gene sequences from cultured microorganisms (i.e., with a known taxonomy). Some popular reference databases are Greengenes (111), SILVA (112), and RDP (113). Query sequences are compared against the reference database and assigned to the organism of the best-matched reference (114). While this approach is computationally fast and allows the straightforward taxonomic labeling of a query sequence, indicating its relationship to previously characterized microorganisms, it is hindered by the lack of well-annotated or incomplete databases (115). This is exacerbated when working with genes other than the 16S rRNA or with sequences from hard-to-culture or yet uncultured organisms, as is usually the case of colonic microbes, making them inherently limited (116).
On the other hand, OTU-based methods do not rely on reference databases; they calculate a distance matrix among all query sequences and group them based on their similarity at a given threshold. Since grouping does not require previous taxonomic information, these OTU-based methods perform very well with poorly characterized microorganisms. OTU-based methods are not without faults, however. They are usually computationally exigent and prone to overestimation due to low-quality sequences, contamination, chimeras, etc. (117).
In turn, most OTU-clustering algorithms fall into two broad categories, hierarchical clustering (HC) and greedy heuristic clustering (GHC). HC and GHC differ in the methods for comparison of sequences and clustering into OTUs, their computational requirements, and the accuracy of the result. HC methods start by generating distance matrices that measure the distance between each pair of sequences in the dataset, either by multiple [e.g., Mothur (118)] or pairwise [e.g., ESPRIT (119)] sequence alignments, and then apply standard HC (single, complete or average linkage clustering) to group OTUs at a given threshold (usually, 97%). While debated (120), the use of multiple sequence alignment is preferred over pairwise alignments because it preserves positional homology across all sequences (121). The incorporation of the secondary structure of the 16S rRNA molecule into the alignment provides additional biological information that strengthens the confidence that positional homology is being conserved (122, 123). HC methods are computationally complex; however, several approaches have been devised to reduce their complexity and computer memory requirements (116, 119, 121), and software such as Mothur (from version 1.27.0) performs well with reasonable computer capacities.
Yet, computational requirements of HC algorithms can be a real headache in the analysis of many fecal samples; GHC algorithms have been developed to this end. They process input sequences one at a time, hence, avoiding the comparison of all pairs of sequences and the construction of a distance matrix (115). In GHC, the query sequence is compared against a set of seed sequences (or centroids) that are representative of existing clusters; if the similarity of the query and the seed sequences is above a given threshold (usually, 97%), the query sequence is assigned to the existing cluster, otherwise it becomes the seed of a new cluster or it is discarded. The seed sequences can be obtained either by generating them de novo [e.g., CD-HIT (124) or UCLUST (125)] or from a database of predefined centroids [e.g., UCLUST as implemented by QIIME (126, 127)]; the latter approach has the same limitations of other database-dependent methods, as discussed above. Furthermore, the centroid databases are constructed by clustering full-length sequences at a defined threshold; when used to cluster partial sequences, problems may arise. Some taxa may have identical sequences within a specific 16S sub-region, yet, they can be below the predefined threshold when the full-length sequence is considered; the opposite would also be true.
As with other steps in the workflow discussed here, there is a trade-off between complexity and accuracy. Different clustering methods can yield different results from identical datasets; their performance varies according to the complexity and the abundance ratio of the sequences in the dataset and the selected similarity threshold (117). Benchmark studies have consistently shown that methods such as complete linkage (HC), average linkage (HC), and CD-HIT (GHC) are robust to changing OTU thresholds and produce consistent clusters. On the other hand, single linkage (HC) produces OTUs that are not homogeneous and together with UCLUST (GHC) and UPARSE (GHC) have been shown to be very sensitive to threshold definitions and to have reproducibility issues, thus, in our opinion, their use should be less encouraged (115, 128, 129).
Taxonomic Assignment
In order to establish the biological significance of any intervention on the gut microbiome, it is usually desired to give a taxonomic classification to the previously detected OTUs. Several methods for the taxonomic assignment of 16S rRNA gene sequences are available and are based on different principles, such as k-mer count [SINA (130), RDP Bayesian classifier (88)], multiple sequence alignment [NAST (131)], BLAST [TUIT (132)], and machine learning algorithms [16S classifier (133)], among others. Although new algorithms continue to be developed, the RDP Bayesian classifier remains the most widely used tool for taxonomic assignment of 16S sequences; it provides taxonomic assignments from domain to genus, with confidence estimates for each assignment. The misclassification rate of short sequences varies approximately from 16 to 20% according to the dataset used to train the algorithm and the 16S rRNA gene region (114). As with others database-dependent methods, flaws in the databases will unavoidably lead to flaws in classification; fortunately, the approach used to label OTUs can reduce the error.
Regardless of the algorithm, OTUs can be classified either by assigning them the taxonomy of a representative sequence (127) or by classifying every sequence in the OTU and assigning the taxonomy by majority consensus (116). The former method can yield a less robust classification; if an OTU is composed of related sequences but with divergent taxonomies, the classification of a single sequence can lead to an erroneous classification of the entire OTU. Therefore, we recommended using majority-consensus taxonomy to the cost of a less detailed classification (genus, species).
Copy Number Variation
A problem that arises when studying the gut microbiome is the difference in the number of copies of the 16S rRNA gene among species, which can range from a single copy up to 15 (134). This variation can lead to erroneous abundance assessment; at equal number of cells, taxa with few copies of the 16S rRNA gene have lower amplicon counts than taxa with more copies of the gene. Therefore, CNV can result in over or underestimation of microbial abundance. CNV has not deserved full attention; yet, it is of utmost importance since it can result in a biased description of the microbial community. Indeed, it has been suggested that bacterial diversity could be overestimated by a factor of 3 due to 16S CNV (135).
In microorganisms with known 16S rRNA gene copy number, CNV could be corrected by weighting read counts by the inverse of its gene copy number. However, the problem is more difficult to deal with in cases where the gene copy number is unknown. A possible solution in these cases is to use the value of a closely related organism (136). Another possibility is to place 16S reads on a phylogenetic tree and calculate gene copy number using phylogenetically independent contrasts (137, 138). While these methods have been shown to improve the measures of diversity and abundance of microbial communities, they rely on databases of 16S and sequenced genomes, which, as with phylotype-based clustering, lack information of uncultured and poorly cultured organisms. In cases of poorly studied deep evolutionary lineages (say, rare phyla), CNV correction is definitely an unsolved issue.
Although CNV can move away estimates of diversity from reality, it must be noted that researchers usually want to compare these estimates between treatments (e.g., obese vs. lean, vaginal delivery vs. C-section, probiotic vs. placebo). In other words, we look for relative changes in the abundance of OTUs A, B, and C; even if they would be badly estimated due to the assumption that they only have one 16S rRNA gene each, what is important is to see how populations change under different tested conditions. The take-home message from CNV is that we should emphasize more comparisons of the same OTU among samples than comparisons among OTUs within samples.
Essential Information That Should Be Included when Describing a Microbiome Study
In order to guarantee reproducibility of results, we encourage researchers and journals to explicitly include and require the following technical information in microbiome publications: (I) DNA extraction method, including the type of extraction kit if one was used and modifications to the standard protocol proposed by the manufacturer; (II) description of how DNA contamination was controlled for (e.g., DNA extraction of negative controls); (III) 16S rRNA hypervariable region targeted including the nucleotide sequences of the primers used; (IV) sequencing technology employed; (V) description of sequencing error-rate assessment (e.g., was a mock community sequenced in parallel with the samples?); and (VI) description of in silico analyses (culling of dubious sequences, removal of chimeras, OTU clustering and taxonomy assignment, copy number variation correction), including the code or command lines with parameters used if appropriate.
Conclusion
The study of the gut microbiome is revolutionizing medicine and science by allowing understanding how microbes are intimately involved in many physiological processes. The gut microbiome is shifting from an appealing object of study to a precision medicine target. NGS have enabled the possibility to gather the most impressive amount of microbiome data at costs and speeds that were unthinkable a decade ago. However, these technologies have introduced new challenges in data analysis that researchers must take care of. We have, here, discussed some of these challenges and suggested ways to control them using available tools (see Table 2 for our recommendations to reduce the impact of these pitfalls). Our hope is that, while a minimum information standard that unifies the procedures of microbiome studies is established, researchers implement rigorous analyses so that their results better represent the microbial communities under scrutiny. Only by making as stringent as possible analyses and by guaranteeing the transparency and reproducibility of microbiome analyses (139) we will give the field its first dose of “healthy skepticism” (140).
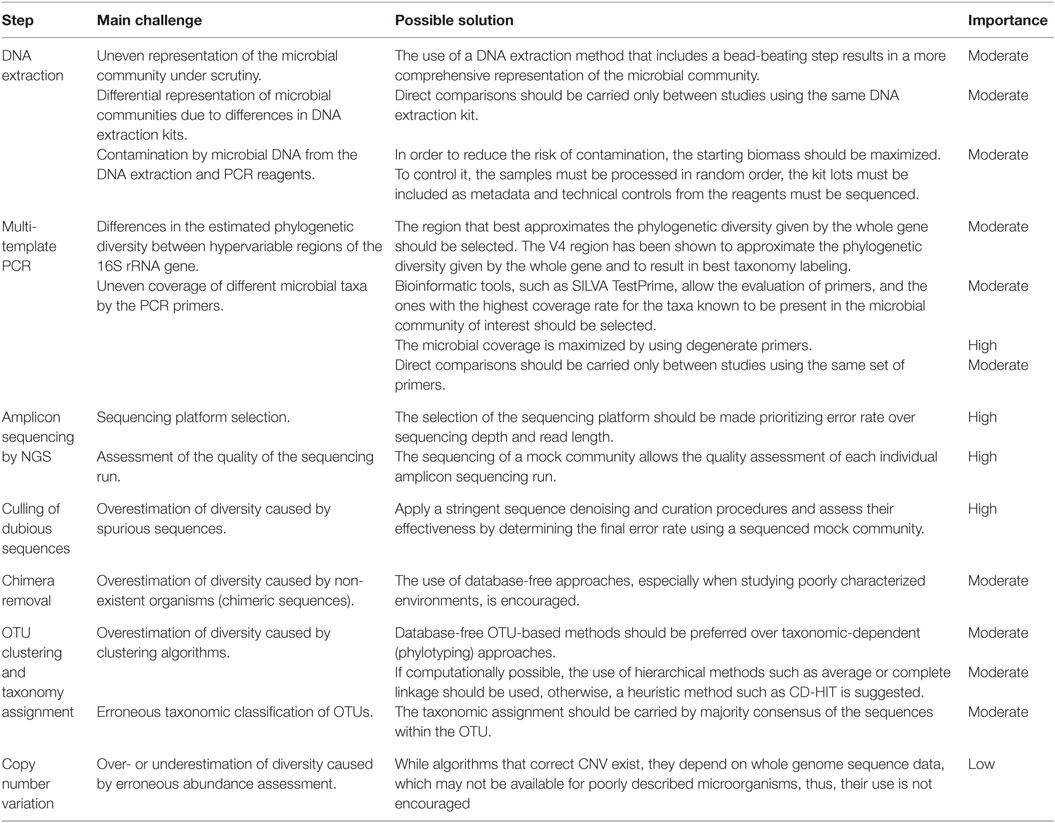
Table 2. Recommendations to reduce the impact of biases introduced in the different steps of the analysis of microbial communities using the 16S rRNA gene.
Author Contributions
JC-Z and JE devised, wrote, and made corrections to the manuscript.
Conflict of Interest Statement
The authors declare that the research was conducted in the absence of any commercial or financial relationships that could be construed as a potential conflict of interest.
Acknowledgments
We thank Colciencias for funding the Young Researcher internship of JC-Z and CABBIO-Colciencias for sponsoring the attendance of JC-Z to the CABBIO course organized by the Environmental Microbiology Lab of “Centro Nacional Patagónico” in Puerto Madryn, Chubut, Argentina. We also thank Roberto Jiménez-Cardona for insightful comments on the manuscript.
References
1. Brüssow H. Human microbiota: “the philosophers have only interpreted the world in various ways. The point, however, is to change it”. Microb Biotechnol (2015) 8(1):11–2. doi: 10.1111/1751-7915.12259
2. Zmora N, Zeevi D, Korem T, Segal E, Elinav E. Taking it personally: personalized utilization of the human microbiome in health and disease. Cell Host Microbe (2016) 19(1):12–20. doi:10.1016/j.chom.2015.12.016
3. Foxx-Orenstein AE, Chey WD. Manipulation of the gut microbiota as a novel treatment strategy for gastrointestinal disorders. Am J Gastroenterol Suppl (2012) 1(1):41–6. doi:10.1038/ajgsup.2012.8
4. He C, Shan Y, Song W. Targeting gut microbiota as a possible therapy for diabetes. Nutr Res (2015) 35(5):361–7. doi:10.1016/j.nutres.2015.03.002
5. Butel M-J. Probiotics, gut microbiota and health. Médecine Mal Infect. (2014) 44(1):1–8. doi:10.1016/j.medmal.2013.10.002
6. Cammarota G, Ianiro G, Bibbò S, Gasbarrini A. Gut microbiota modulation: probiotics, antibiotics or fecal microbiota transplantation? Intern Emerg Med (2014) 9(4):365–73. doi:10.1007/s11739-014-1069-4
7. Walsh CJ, Guinane CM, O’Toole PW, Cotter PD. Beneficial modulation of the gut microbiota. FEBS Lett (2014) 588(22):4120–30. doi:10.1016/j.febslet.2014.03.035
8. van Nood E, Vrieze A, Nieuwdorp M, Fuentes S, Zoetendal EG, de Vos WM, et al. Duodenal infusion of donor feces for recurrent Clostridium difficile. N Engl J Med (2013) 368(5):407–15. doi:10.1056/NEJMoa1205037
9. Alander M, De Smet I, Nollet L, Verstraete W, von Wright A, Mattila-Sandholm T. The effect of probiotic strains on the microbiota of the simulator of the human intestinal microbial ecosystem (SHIME). Int J Food Microbiol (1999) 46(1):71–9. doi:10.1016/S0168-1605(98)00182-2
10. Chung WSF, Walker AW, Louis P, Parkhill J, Vermeiren J, Bosscher D, et al. Modulation of the human gut microbiota by dietary fibres occurs at the species level. BMC Biol (2016) 14(1):3. doi:10.1186/s12915-015-0224-3
11. Kovacs A, Ben-Jacob N, Tayem H, Halperin E, Iraqi FA, Gophna U. Genotype is a stronger determinant than sex of the mouse gut microbiota. Microb Ecol (2011) 61(2):423–8. doi:10.1007/s00248-010-9787-2
12. Tang K, Liu K, Jiao N, Zhang Y, Chen C-TA. Functional metagenomic investigations of microbial communities in a shallow-sea hydrothermal system. PLoS One (2013) 8(8):e72958. doi:10.1371/journal.pone.0072958
13. Afshinnekoo E, Meydan C, Chowdhury S, Jaroudi D, Boyer C, Bernstein N, et al. Geospatial resolution of human and bacterial diversity with city-scale metagenomics. Cell Syst (2015) 1(1):72–87. doi:10.1016/j.cels.2015.01.001
14. Liu L, Li Y, Li S, Hu N, He Y, Pong R, et al. Comparison of next-generation sequencing systems. J Biomed Biotechnol (2012) 2012:251364. doi:10.1155/2012/251364
15. Frey KG, Herrera-Galeano JE, Redden CL, Luu TV, Servetas SL, Mateczun AJ, et al. Comparison of three next-generation sequencing platforms for metagenomic sequencing and identification of pathogens in blood. BMC Genomics (2014) 15(1):96. doi:10.1186/1471-2164-15-96
16. Glenn TC. Field guide to next-generation DNA sequencers. Mol Ecol Resour (2011) 11(5):759–69. doi:10.1111/j.1755-0998.2011.03024.x
17. Case RJ, Boucher Y, Dahllöf I, Holmström C, Doolittle WF, Kjelleberg S. Use of 16S rRNA and rpoB genes as molecular markers for microbial ecology studies. Appl Environ Microbiol (2007) 73(1):278–88. doi:10.1128/AEM.01177-06
18. Wu D, Jospin G, Eisen JA. Systematic identification of gene families for use as markers for phylogenetic and phylogeny-driven ecological studies of bacteria and archaea and their major subgroups. PLoS One (2013) 8(10):e77033. doi:10.1371/journal.pone.0077033
19. Gaby JC, Buckley DH. A comprehensive aligned nifH gene database: a multipurpose tool for studies of nitrogen-fixing bacteria. Database (Oxford) (2014) 2014(0):bau001. doi:10.1093/database/bau001
20. Barriuso J, Valverde JR, Mellado RP. Estimation of bacterial diversity using next generation sequencing of 16S rDNA: a comparison of different workflows. BMC Bioinformatics (2011) 12(1):473. doi:10.1186/1471-2105-12-473
21. Ravel J, Blaser MJ, Braun J, Brown E, Bushman FD, Chang EB, et al. Human microbiome science: vision for the future, Bethesda, MD, July 24 to 26, 2013. Microbiome (2014) 2(1):16. doi:10.1186/2049-2618-2-16
22. Frick J-S, Haller D. Intestinal microbiota: from sequencing to function. Int J Med Microbiol (2016). doi:10.1016/j.ijmm.2016.02.007
23. Pekkala S, Munukka E, Rintala A, Huovinen P. The microbiome studies in metabolic diseases have advanced but are poorly standardized and lack a mechanistic perspective. J Diabetes Metab (2015) 6:480. doi:10.4172/2155-6156.1000480
24. Avershina E, Rudi K. Confusion about the species richness of human gut microbiota. Benef Microbes (2015) 6(5):657–9. doi:10.3920/BM2015.0007
25. Schloss PD, Gevers D, Westcott SL. Reducing the effects of PCR amplification and sequencing artifacts on 16S rRNA-based studies. PLoS One (2011) 6(12):e27310. doi:10.1371/journal.pone.0027310
26. Luo C, Tsementzi D, Kyrpides N, Read T, Konstantinidis KT. Direct comparisons of Illumina vs. Roche 454 sequencing technologies on the same microbial community DNA sample. PLoS One (2012) 7(2):e30087. doi:10.1371/journal.pone.0030087
27. Mosher JJ, Bernberg EL, Shevchenko O, Kan J, Kaplan LA. Efficacy of a 3rd generation high-throughput sequencing platform for analyses of 16S rRNA genes from environmental samples. J Microbiol Methods (2013) 95(2):175–81. doi:10.1016/j.mimet.2013.08.009
28. Nederbragt AJ. On the middle ground between open source and commercial software – the case of the Newbler program. Genome Biol (2014) 15(4):113. doi:10.1186/gb4173
29. Kozich JJ, Westcott SL, Baxter NT, Highlander SK, Schloss PD. Development of a dual-index sequencing strategy and curation pipeline for analyzing amplicon sequence data on the MiSeq Illumina sequencing platform. Appl Environ Microbiol (2013) 79(17):5112–20. doi:10.1128/AEM.01043-13
30. Schröder J, Bailey J, Conway T, Zobel J. Reference-free validation of short read data. PLoS One (2010) 5(9):e12681. doi:10.1371/journal.pone.0012681
31. Quail MA, Smith M, Coupland P, Otto TD, Harris SR, Connor TR, et al. A tale of three next generation sequencing platforms: comparison of Ion Torrent, Pacific Biosciences and Illumina MiSeq sequencers. BMC Genomics (2012) 13(1):341. doi:10.1186/1471-2164-13-341
32. Salipante SJ, Kawashima T, Rosenthal C, Hoogestraat DR, Cummings LA, Sengupta DJ, et al. Performance comparison of Illumina and Ion Torrent next-generation sequencing platforms for 16S rRNA-based bacterial community profiling. Appl Environ Microbiol (2014) 80(24):7583–91. doi:10.1128/AEM.02206-14
33. Loman NJ, Misra RV, Dallman TJ, Constantinidou C, Gharbia SE, Wain J, et al. Performance comparison of benchtop high-throughput sequencing platforms. Nat Biotechnol (2012) 30(5):434–9. doi:10.1038/nbt.2198
34. Schloss PD, Jenior ML, Koumpouras CC, Westcott SL, Highlander SK. Sequencing 16S rRNA gene fragments using the PacBio SMRT DNA sequencing system. PeerJ (2016) 4:e1869. doi:10.7717/peerj.1869
35. Fichot EB, Norman RS. Microbial phylogenetic profiling with the Pacific Biosciences sequencing platform. Microbiome (2013) 1(1):10. doi:10.1186/2049-2618-1-10
36. Zhou J, He Z, Yang Y, Deng Y, Tringe SG, Alvarez-Cohen L. High-throughput metagenomic technologies for complex microbial community analysis: open and closed formats. MBio (2015) 6(1):e02288–14. doi:10.1128/mBio.02288-14
37. Tremblay J, Singh K, Fern A, Kirton ES, He S, Woyke T, et al. Primer and platform effects on 16S rRNA tag sequencing. Front Microbiol (2015) 6:771. doi:10.3389/fmicb.2015.00771
38. Hazen TC, Rocha AM, Techtmann SM. Advances in monitoring environmental microbes. Curr Opin Biotechnol (2013) 24(3):526–33. doi:10.1016/j.copbio.2012.10.020
39. Preheim SP, Perrotta AR, Friedman J, Smilie C, Brito I, Smith MB, et al. Computational methods for high-throughput comparative analyses of natural microbial communities. Methods Enzymol (2013) 531:353–70. doi:10.1016/B978-0-12-407863-5.00018-6
40. Jovel J, Patterson J, Wang W, Hotte N, O’Keefe S, Mitchel T, et al. Characterization of the gut microbiome using 16S or shotgun metagenomics. Front Microbiol (2016) 7:459. doi:10.3389/fmicb.2016.00459
41. Schloss PD. Evaluating different approaches that test whether microbial communities have the same structure. ISME J (2008) 2(3):265–75. doi:10.1038/ismej.2008.5
42. Prosser JI. Replicate or lie. Environ Microbiol (2010) 12(7):1806–10. doi:10.1111/j.1462-2920.2010.02201.x
43. Lennon JT. Replication, lies and lesser-known truths regarding experimental design in environmental microbiology. Environ Microbiol (2011) 13(6):1383–6. doi:10.1111/j.1462-2920.2011.02445.x
44. Knight R, Jansson J, Field D, Fierer N, Desai N, Fuhrman JA, et al. Unlocking the potential of metagenomics through replicated experimental design. Nat Biotechnol (2012) 30(6):513–20. doi:10.1038/nbt.2235
45. McMurdie PJ, Holmes S. Waste not, want not: why rarefying microbiome data is inadmissible. PLoS Comput Biol (2014) 10(4):e1003531. doi:10.1371/journal.pcbi.1003531
46. Bahl MI, Bergström A, Licht TR. Freezing fecal samples prior to DNA extraction affects the firmicutes to bacteroidetes ratio determined by downstream quantitative PCR analysis. FEMS Microbiol Lett (2012) 329(2):193–7. doi:10.1111/j.1574-6968.2012.02523.x
47. Guo F, Zhang T. Biases during DNA extraction of activated sludge samples revealed by high throughput sequencing. Appl Microbiol Biotechnol (2013) 97(10):4607–16. doi:10.1007/s00253-012-4244-4
48. Ariefdjohan MW, Savaiano DA, Nakatsu CH. Comparison of DNA extraction kits for PCR-DGGE analysis of human intestinal microbial communities from fecal specimens. Nutr J (2010) 9:23. doi:10.1186/1475-2891-9-23
49. Feinstein LM, Sul WJ, Blackwood CB. Assessment of bias associated with incomplete extraction of microbial DNA from soil. Appl Environ Microbiol (2009) 75(16):5428–33. doi:10.1128/AEM.00120-09
50. Smith B, Li N, Andersen AS, Slotved HC, Krogfelt KA. Optimising bacterial DNA extraction from faecal samples: comparison of three methods. Open Microbiol J (2011) 5:14–7. doi:10.2174/1874285801105010014
51. Wesolowska-Andersen A, Bahl MI, Carvalho V, Kristiansen K, Sicheritz-Pontén T, Gupta R, et al. Choice of bacterial DNA extraction method from fecal material influences community structure as evaluated by metagenomic analysis. Microbiome (2014) 2(1):19. doi:10.1186/2049-2618-2-19
52. Henderson G, Cox F, Kittelmann S, Miri VH, Zethof M, Noel SJ, et al. Effect of DNA extraction methods and sampling techniques on the apparent structure of cow and sheep rumen microbial communities. PLoS One (2013) 8(9):e74787. doi:10.1371/journal.pone.0074787
53. Rand KH, Houck H. Taq polymerase contains bacterial DNA of unknown origin. Mol Cell Probes (1990) 4(6):445–50. doi:10.1016/0890-8508(90)90003-I
54. Shen H, Rogelj S, Kieft TL. Sensitive, real-time PCR detects low-levels of contamination by Legionella pneumophila in commercial reagents. Mol Cell Probes (2006) 20(3–4):147–53. doi:10.1016/j.mcp.2005.09.007
55. Kulakov LA, McAlister MB, Ogden KL, Larkin MJ, O’Hanlon JF. Analysis of bacteria contaminating ultrapure water in industrial systems. Appl Environ Microbiol (2002) 68(4):1548–55. doi:10.1128/AEM.68.4.1548-1555.2002
56. McAlister MB, Kulakov LA, O’Hanlon JF, Larkin MJ, Ogden KL. Survival and nutritional requirements of three bacteria isolated from ultrapure water. J Ind Microbiol Biotechnol (2002) 29(2):75–82. doi:10.1038/sj.jim.7000273
57. Tanner MA, Goebel BM, Dojka MA, Pace NR. Specific ribosomal DNA sequences from diverse environmental settings correlate with experimental contaminants. Appl Envir Microbiol (1998) 64(8):3110–3.
58. Corless CE, Guiver M, Borrow R, Edwards-Jones V, Kaczmarski EB, Fox AJ. Contamination and sensitivity issues with a real-time universal 16S rRNA PCR. J Clin Microbiol (2000) 38(5):1747–52.
59. Grahn N, Olofsson M, Ellnebo-Svedlund K, Monstein H-J, Jonasson J. Identification of mixed bacterial DNA contamination in broad-range PCR amplification of 16S rDNA V1 and V3 variable regions by pyrosequencing of cloned amplicons. FEMS Microbiol Lett (2003) 219(1):87–91. doi:10.1016/S0378-1097(02)01190-4
60. Salter SJ, Cox MJ, Turek EM, Calus ST, Cookson WO, Moffatt MF, et al. Reagent and laboratory contamination can critically impact sequence-based microbiome analyses. BMC Biol (2014) 12(1):87. doi:10.1186/s12915-014-0087-z
61. Jervis-Bardy J, Leong LEX, Marri S, Smith RJ, Choo JM, Smith-Vaughan HC, et al. Deriving accurate microbiota profiles from human samples with low bacterial content through post-sequencing processing of Illumina MiSeq data. Microbiome (2015) 3(1):19. doi:10.1186/s40168-015-0083-8
62. Pinto AJ, Raskin L. PCR biases distort bacterial and archaeal community structure in pyrosequencing datasets. PLoS One (2012) 7(8):e43093. doi:10.1371/journal.pone.0043093
63. Polz MF, Cavanaugh CM. Bias in template-to-product ratios in multitemplate PCR. Appl Environ Microbiol (1998) 64(10):3724–30.
64. Ishii K, Fukui M. Optimization of annealing temperature to reduce bias caused by a primer mismatch in multitemplate PCR. Appl Environ Microbiol (2001) 67(8):3753–5. doi:10.1128/AEM.67.8.3753-3755.2001
65. Kalle E, Gulevich A, Rensing C. External and semi-internal controls for PCR amplification of homologous sequences in mixed templates. J Microbiol Methods (2013) 95(2):285–94. doi:10.1016/j.mimet.2013.09.014
66. Woese CR, Fox GE. Phylogenetic structure of the prokaryotic domain: the primary kingdoms. Proc Natl Acad Sci U S A. (1977) 74(11):5088–90. doi:10.1073/pnas.74.11.5088
67. Head IM, Saunders JR, Pickup RW. Microbial evolution, diversity, and ecology: a decade of ribosomal RNA analysis of uncultivated microorganisms. Microb Ecol (1998) 35(1):1–21. doi:10.1007/s002489900056
68. Tringe SG, Hugenholtz P. A renaissance for the pioneering 16S rRNA gene. Curr Opin Microbiol (2008) 11(5):442–6. doi:10.1016/j.mib.2008.09.011
69. Youssef N, Sheik CS, Krumholz LR, Najar FZ, Roe BA, Elshahed MS. Comparison of species richness estimates obtained using nearly complete fragments and simulated pyrosequencing-generated fragments in 16S rRNA gene-based environmental surveys. Appl Environ Microbiol (2009) 75(16):5227–36. doi:10.1128/AEM.00592-09
70. Hamp TJ, Jones WJ, Fodor AA. Effects of experimental choices and analysis noise on surveys of the “rare biosphere”. Appl Environ Microbiol (2009) 75(10):3263–70. doi:10.1128/AEM.01931-08
71. Schloss PD. The effects of alignment quality, distance calculation method, sequence filtering, and region on the analysis of 16S rRNA gene-based studies. PLoS Comput Biol (2010) 6(7):e1000844. doi:10.1371/journal.pcbi.1000844
72. Yarza P, Yilmaz P, Pruesse E, Glöckner FO, Ludwig W, Schleifer K-H, et al. Uniting the classification of cultured and uncultured bacteria and archaea using 16S rRNA gene sequences. Nat Rev Microbiol (2014) 12(9):635–45. doi:10.1038/nrmicro3330
73. Cai L, Ye L, Tong AHY, Lok S, Zhang T. Biased diversity metrics revealed by bacterial 16S pyrotags derived from different primer sets. PLoS One (2013) 8(1):e53649. doi:10.1371/journal.pone.0053649
74. Chakravorty S, Helb D, Burday M, Connell N, Alland D. A detailed analysis of 16S ribosomal RNA gene segments for the diagnosis of pathogenic bacteria. J Microbiol Methods (2007) 69(2):330–9. doi:10.1016/j.mimet.2007.02.005
75. Kumar PS, Brooker MR, Dowd SE, Camerlengo T. Target region selection is a critical determinant of community fingerprints generated by 16S pyrosequencing. PLoS One (2011) 6(6):e20956. doi:10.1371/journal.pone.0020956
76. Wang Y, Qian P.-Y. Conserved regions in 16S ribosome RNA sequences and primer design for studies of environmental microbes. In: Encyclopedia of Metagenomics. Boston, MA: Springer US (2015). p. 106–10. doi:10.1007/978-1-4899-7478-5_772
77. Klindworth A, Pruesse E, Schweer T, Peplies J, Quast C, Horn M, et al. Evaluation of general 16S ribosomal RNA gene PCR primers for classical and next-generation sequencing-based diversity studies. Nucleic Acids Res (2013) 41(1):e1. doi:10.1093/nar/gks808
78. Mao D-P, Zhou Q, Chen C-Y, Quan Z-X. Coverage evaluation of universal bacterial primers using the metagenomic datasets. BMC Microbiol (2012) 12(1):66. doi:10.1186/1471-2180-12-66
79. Wu J-H, Hong P-Y, Liu W-T. Quantitative effects of position and type of single mismatch on single base primer extension. J Microbiol Methods (2009) 77(3):267–75. doi:10.1016/j.mimet.2009.03.001
80. Caporaso JG, Lauber CL, Walters WA, Berg-Lyons D, Lozupone CA, Turnbaugh PJ, et al. Global patterns of 16S rRNA diversity at a depth of millions of sequences per sample. Proc Natl Acad Sci U S A. (2011) 108(Suppl):4516–22. doi:10.1073/pnas.1000080107
81. Yatsunenko T, Rey FE, Manary MJ, Trehan I, Dominguez-Bello MG, Contreras M, et al. Human gut microbiome viewed across age and geography. Nature (2012) 486(7402):222–7. doi:10.1038/nature11053
82. Fierer N, Leff JW, Adams BJ, Nielsen UN, Bates ST, Lauber CL, et al. Cross-biome metagenomic analyses of soil microbial communities and their functional attributes. Proc Natl Acad Sci U S A. (2012) 109(52):21390–5. doi:10.1073/pnas.1215210110
83. Metzker ML. Sequencing technologies – the next generation. Nat Rev Genet (2010) 11(1):31–46. doi:10.1038/nrg2626
84. The Human Microbiome Project Consortium. A framework for human microbiome research. Nature (2012) 486(7402):215–21. doi:10.1038/nature11209
85. Turnbaugh PJ, Hamady M, Yatsunenko T, Cantarel BL, Duncan A, Ley RE, et al. A core gut microbiome in obese and lean twins. Nature (2009) 457(7228):480–4. doi:10.1038/nature07540
86. Candon S, Perez-Arroyo A, Marquet C, Valette F, Foray A-P, Pelletier B, et al. Antibiotics in early life alter the gut microbiome and increase disease incidence in a spontaneous mouse model of autoimmune insulin-dependent diabetes. PLoS One (2015) 10(5):e0125448. doi:10.1371/journal.pone.0125448
87. Cobaugh KL, Schaeffer SM, DeBruyn JM. Functional and structural succession of soil microbial communities below decomposing human cadavers. PLoS One (2015) 10(6):e0130201. doi:10.1371/journal.pone.0130201
88. Wang Q, Garrity GM, Tiedje JM, Cole JR. Naive Bayesian classifier for rapid assignment of rRNA sequences into the new bacterial taxonomy. Appl Environ Microbiol (2007) 73(16):5261–7. doi:10.1128/AEM.00062-07
89. Liu Z, DeSantis TZ, Andersen GL, Knight R. Accurate taxonomy assignments from 16S rRNA sequences produced by highly parallel pyrosequencers. Nucleic Acids Res (2008) 36(18):e120. doi:10.1093/nar/gkn491
90. Lundin D, Severin I, Logue JB, Ostman O, Andersson AF, Lindström ES. Which sequencing depth is sufficient to describe patterns in bacterial α- and β-diversity? Environ Microbiol Rep (2012) 4(3):367–72. doi:10.1111/j.1758-2229.2012.00345.x
91. Pedrós-Alió C. The rare bacterial biosphere. Ann Rev Mar Sci (2012) 4:449–66. doi:10.1146/annurev-marine-120710-100948
92. Kunin V, Engelbrektson A, Ochman H, Hugenholtz P. Wrinkles in the rare biosphere: pyrosequencing errors can lead to artificial inflation of diversity estimates. Environ Microbiol (2010) 12(1):118–23. doi:10.1111/j.1462-2920.2009.02051.x
93. Huse SM, Huber JA, Morrison HG, Sogin ML, Welch DM. Accuracy and quality of massively parallel DNA pyrosequencing. Genome Biol (2007) 8(7):R143. doi:10.1186/gb-2007-8-7-r143
94. Caporaso JG, Lauber CL, Walters WA, Berg-Lyons D, Huntley J, Fierer N, et al. Ultra-high-throughput microbial community analysis on the Illumina HiSeq and MiSeq platforms. ISME J (2012) 6(8):1621–4. doi:10.1038/ismej.2012.8
95. Huse SM, Welch DM, Morrison HG, Sogin ML. Ironing out the wrinkles in the rare biosphere through improved OTU clustering. Environ Microbiol (2010) 12(7):1889–98. doi:10.1111/j.1462-2920.2010.02193.x
96. Hanshew AS, Mason CJ, Raffa KF, Currie CR. Minimization of chloroplast contamination in 16S rRNA gene pyrosequencing of insect herbivore bacterial communities. J Microbiol Methods (2013) 95(2):149–55. doi:10.1016/j.mimet.2013.08.007
97. Pääbo S, Irwin DM, Wilson AC. DNA damage promotes jumping between templates during enzymatic amplification. J Biol Chem (1990) 265(8):4718–21.
98. Wang G, Wang Y. Frequency of formation of chimeric molecules as a consequence of PCR coamplification of 16S rRNA genes from mixed bacterial genomes. Appl Envir Microbiol (1997) 63(12):4645–50.
99. Wang GC, Wang Y. The frequency of chimeric molecules as a consequence of PCR co-amplification of 16S rRNA genes from different bacterial species. Microbiology (1996) 142(Pt 5):1107–14. doi:10.1099/13500872-142-5-1107
100. Huber T, Faulkner G, Hugenholtz P. Bellerophon: a program to detect chimeric sequences in multiple sequence alignments. Bioinformatics (2004) 20(14):2317–9. doi:10.1093/bioinformatics/bth226
101. Ashelford KE, Chuzhanova NA, Fry JC, Jones AJ, Weightman AJ. At least 1 in 20 16S rRNA sequence records currently held in public repositories is estimated to contain substantial anomalies. Appl Environ Microbiol (2005) 71(12):7724–36. doi:10.1128/AEM.71.12.7724-7736.2005
102. Gonzalez JM, Zimmermann J, Saiz-Jimenez C. Evaluating putative chimeric sequences from PCR-amplified products. Bioinformatics (2005) 21(3):333–7. doi:10.1093/bioinformatics/bti008
103. Ashelford KE, Chuzhanova NA, Fry JC, Jones AJ, Weightman AJ. New screening software shows that most recent large 16S rRNA gene clone libraries contain chimeras. Appl Environ Microbiol (2006) 72(9):5734–41. doi:10.1128/AEM.00556-06
104. Gontcharova V, Youn E, Wolcott RD, Hollister EB, Gentry TJ, Dowd SE. Black Box Chimera Check (B2C2): a windows-based software for batch depletion of chimeras from bacterial 16S rRNA gene datasets. Open Microbiol J (2010) 4:47–52. doi:10.2174/1874285801004010047
105. Haas BJ, Gevers D, Earl AM, Feldgarden M, Ward DV, Giannoukos G, et al. Chimeric 16S rRNA sequence formation and detection in Sanger and 454-pyrosequenced PCR amplicons. Genome Res (2011) 21(3):494–504. doi:10.1101/gr.112730.110
106. Quince C, Lanzen A, Davenport RJ, Turnbaugh PJ. Removing noise from pyrosequenced amplicons. BMC Bioinformatics (2011) 12(1):38. doi:10.1186/1471-2105-12-38
107. Edgar RC, Haas BJ, Clemente JC, Quince C, Knight R. UCHIME improves sensitivity and speed of chimera detection. Bioinformatics (2011) 27(16):2194–200. doi:10.1093/bioinformatics/btr381
108. Wright ES, Yilmaz LS, Noguera DR. DECIPHER, a search-based approach to chimera identification for 16S rRNA sequences. Appl Environ Microbiol (2012) 78(3):717–25. doi:10.1128/AEM.06516-11
109. Pible O, Armengaud J. Improving the quality of genome, protein sequence, and taxonomy databases: a prerequisite for microbiome meta-omics 2.0. Proteomics (2015) 15(20):3418–23. doi:10.1002/pmic.201500104
110. Chang Q, Luan Y, Chen T, Fuhrman JA, Sun F. Computational methods for the analysis of tag sequences in metagenomics studies. Front Biosci (Schol Ed) (2012) 4:1333–43.
111. DeSantis TZ, Hugenholtz P, Larsen N, Rojas M, Brodie EL, Keller K, et al. Greengenes, a chimera-checked 16S rRNA gene database and workbench compatible with ARB. Appl Environ Microbiol (2006) 72(7):5069–72. doi:10.1128/AEM.03006-05
112. Quast C, Pruesse E, Yilmaz P, Gerken J, Schweer T, Yarza P, et al. The SILVA ribosomal RNA gene database project: improved data processing and web-based tools. Nucleic Acids Res (2013) 41(Database issue):D590–6. doi:10.1093/nar/gks1219
113. Cole JR, Wang Q, Fish JA, Chai B, McGarrell DM, Sun Y, et al. Ribosomal database project: data and tools for high throughput rRNA analysis. Nucleic Acids Res (2014) 42(Database issue):D633–42. doi:10.1093/nar/gkt1244
114. Vinje H, Liland KH, Almøy T, Snipen L. Comparing K-mer based methods for improved classification of 16S sequences. BMC Bioinformatics (2015) 16(1):205. doi:10.1186/s12859-015-0647-4
115. Sun Y, Cai Y, Huse SM, Knight R, Farmerie WG, Wang X, et al. A large-scale benchmark study of existing algorithms for taxonomy-independent microbial community analysis. Brief Bioinform (2012) 13(1):107–21. doi:10.1093/bib/bbr009
116. Schloss PD, Westcott SL. Assessing and improving methods used in operational taxonomic unit-based approaches for 16S rRNA gene sequence analysis. Appl Environ Microbiol (2011) 77(10):3219–26. doi:10.1128/AEM.02810-10
117. Chen W, Zhang CK, Cheng Y, Zhang S, Zhao H. A comparison of methods for clustering 16S rRNA sequences into OTUs. PLoS One (2013) 8(8):e70837. doi:10.1371/journal.pone.0070837
118. Schloss PD, Westcott SL, Ryabin T, Hall JR, Hartmann M, Hollister EB, et al. Introducing mothur: open-source, platform-independent, community-supported software for describing and comparing microbial communities. Appl Environ Microbiol (2009) 75(23):7537–41. doi:10.1128/AEM.01541-09
119. Sun Y, Cai Y, Liu L, Yu F, Farrell ML, McKendree W, et al. ESPRIT: estimating species richness using large collections of 16S rRNA pyrosequences. Nucleic Acids Res (2009) 37(10):e76. doi:10.1093/nar/gkp285
120. Wang X, Cai Y, Sun Y, Knight R, Mai V. Secondary structure information does not improve OTU assignment for partial 16s rRNA sequences. ISME J (2012) 6(7):1277–80. doi:10.1038/ismej.2011.187
121. Schloss PD. A high-throughput DNA sequence aligner for microbial ecology studies. PLoS One (2009) 4(12):e8230. doi:10.1371/journal.pone.0008230
122. Keller A, Förster F, Müller T, Dandekar T, Schultz J, Wolf M. Including RNA secondary structures improves accuracy and robustness in reconstruction of phylogenetic trees. Biol Direct (2010) 5:4. doi:10.1186/1745-6150-5-4
123. Schloss PD. Secondary structure improves OTU assignments of 16S rRNA gene sequences. ISME J (2013) 7(3):457–60. doi:10.1038/ismej.2012.102
124. Li W, Godzik A. Cd-hit: a fast program for clustering and comparing large sets of protein or nucleotide sequences. Bioinformatics (2006) 22(13):1658–9. doi:10.1093/bioinformatics/btl158
125. Edgar RC. Search and clustering orders of magnitude faster than BLAST. Bioinformatics (2010) 26(19):2460–1. doi:10.1093/bioinformatics/btq461
126. Caporaso JG, Kuczynski J, Stombaugh J, Bittinger K, Bushman FD, Costello EK, et al. QIIME allows analysis of high-throughput community sequencing data. Nat Methods (2010) 7(5):335–6. doi:10.1038/nmeth.f.303
127. Kuczynski J, Stombaugh J, Walters WA, González A, Caporaso JG, Knight R. Using QIIME to analyze 16S rRNA gene sequences from microbial communities. Curr Protoc Bioinformatics (2011) Chapter 10:Unit10.7. doi:10.1002/0471250953.bi1007s36
128. Schmidt TSB, Matias Rodrigues JF, von Mering C. Ecological consistency of SSU rRNA-based operational taxonomic units at a global scale. PLoS Comput Biol (2014) 10(4):e1003594. doi:10.1371/journal.pcbi.1003594
129. Schmidt TSB, Matias Rodrigues JF, von Mering C. Limits to robustness and reproducibility in the demarcation of operational taxonomic units. Environ Microbiol (2015) 17(5):1689–706. doi:10.1111/1462-2920.12610
130. Pruesse E, Peplies J, Glöckner FO. SINA: accurate high-throughput multiple sequence alignment of ribosomal RNA genes. Bioinformatics (2012) 28(14):1823–9. doi:10.1093/bioinformatics/bts252
131. DeSantis TZ, Hugenholtz P, Keller K, Brodie EL, Larsen N, Piceno YM, et al. NAST: a multiple sequence alignment server for comparative analysis of 16S rRNA genes. Nucleic Acids Res (2006) 34(Web Server issue):W394–9. doi:10.1093/nar/gkl244
132. Tuzhikov A, Panchin A, Shestopalov VI. TUIT, a BLAST-based tool for taxonomic classification of nucleotide sequences. Biotechniques (2014) 56(2):78–84. doi:10.2144/000114135
133. Chaudhary N, Sharma AK, Agarwal P, Gupta A, Sharma VK. 16S classifier: a tool for fast and accurate taxonomic classification of 16S rRNA hypervariable regions in metagenomic datasets. PLoS One. Public Library of Science (2015) 10(2):e0116106. doi:10.1371/journal.pone.0116106
134. Klappenbach JA, Dunbar JM, Schmidt TM. rRNA operon copy number reflects ecological strategies of bacteria. Appl Environ Microbiol (2000) 66(4):1328–33. doi:10.1128/AEM.66.4.1328-1333.2000
135. Acinas SG, Marcelino LA, Klepac-Ceraj V, Polz MF. Divergence and redundancy of 16S rRNA sequences in genomes with multiple rrn operons. J Bacteriol (2004) 186(9):2629–35. doi:10.1128/JB.186.9.2629-2635.2004
136. Větrovský T, Baldrian P. The variability of the 16S rRNA gene in bacterial genomes and its consequences for bacterial community analyses. PLoS One (2013) 8(2):e57923. doi:10.1371/journal.pone.0057923
137. Kembel SW, Wu M, Eisen JA, Green JL. Incorporating 16S gene copy number information improves estimates of microbial diversity and abundance. PLoS Comput Biol (2012) 8(10):e1002743. doi:10.1371/journal.pcbi.1002743
138. Angly FE, Dennis PG, Skarshewski A, Vanwonterghem I, Hugenholtz P, Tyson GW. CopyRighter: a rapid tool for improving the accuracy of microbial community profiles through lineage-specific gene copy number correction. Microbiome. (2014) 2:11. doi:10.1186/2049-2618-2-11
139. Ravel J, Wommack KE. All hail reproducibility in microbiome research. Microbiome (2014) 2(1):8. doi:10.1186/2049-2618-2-8
Keywords: gut microbiome, 16S rRNA, next-generation sequencing, personalized medicine, personalized nutrition
Citation: de la Cuesta-Zuluaga J and Escobar JS (2016) Considerations For Optimizing Microbiome Analysis Using a Marker Gene. Front. Nutr. 3:26. doi: 10.3389/fnut.2016.00026
Received: 25 May 2016; Accepted: 26 July 2016;
Published: 08 August 2016
Edited by:
Fernando Lizcano, Universidad de La Sabana, ColombiaCopyright: © 2016 de la Cuesta-Zuluaga and Escobar. This is an open-access article distributed under the terms of the Creative Commons Attribution License (CC BY). The use, distribution or reproduction in other forums is permitted, provided the original author(s) or licensor are credited and that the original publication in this journal is cited, in accordance with accepted academic practice. No use, distribution or reproduction is permitted which does not comply with these terms.
*Correspondence: Juan S. Escobar, anNlc2NvYmFyQHNlcnZpY2lvc251dHJlc2EuY29t