- 1Department of Twin Research and Genetic Epidemiology, King's College London, London, United Kingdom
- 2Division of Endocrinology and Metabolism, First Department of Internal Medicine, Diabetes Center, Medical School, AHEPA University Hospital, Thessaloniki, Greece
- 3Department of Cellular and Molecular Nutrition, School of Nutritional Sciences and Dietetics, Tehran University of Medical Sciences, Tehran, Iran
- 4Department of Hypertension, Medical University of Lodz, Łódz, Poland
- 5Polish Mother's Memorial Hospital Research Institute (PMMHRI), Łódz, Poland
- 6Cardiovascular Research Centre, University of Zielona Gora, Zielona Gora, Poland
Background/Aim: Several observational studies evaluated the links between serum monounsaturated fatty acids (MUFAs) and cardiovascular events with controversial results. In the present study, Mendelian randomization (MR) analysis was applied to obtain unconfounded estimates of the causal associations of genetically determined serum MUFAs with coronary heart disease (CHD), myocardial infarction (MI), cardioembolic stroke (CS), and ischemic stroke (IS).
Methods: Four MUFAs were studied (i.e., 10-heptadecenoate, myristoleic, oleic, and palmitoleic acid). Data from the largest genome-wide association studies on MUFAs, CHD, MI, and stroke were analyzed. Inverse variance weighted method (IVW), weighted median (WM)-based method, MR-Egger, as well as MR-pleiotropy residual sum and outlier were applied. To rule out the impact of single-nucleotide polymorphism (SNP), the leave-one-out method was also performed.
Results: Genetically higher-serum 10-heptadecenoate levels did not affect the risk of CHD (IVW = Beta: −0.304, p = 0.185), MI (IVW = Beta: −0.505, p = 0.066), CS (IVW = Beta: −0.056, p = 0.945), and IS (IVW = Beta: −0.121, p = 0.767). Similarly, no significant associations were observed for myristoleic acid (CHD: IVW = Beta: 0.008; MI: IVW = Beta: 0.041; CS: IVW = Beta: 0.881; IS: IVW = Beta: 0.162), oleic acid (CHD: IVW = Beta: −0.2417; MI: IVW = Beta: −0.119; CS: IVW = Beta: 1.059; IS: IVW = Beta: 0.008491), and palmitoleic acid (CHD: IVW = Beta: −0.06957; MI: IVW = Beta: −0.01255; CS: IVW = Beta: 1.042; IS: IVW = Beta: −0.1862). A low likelihood of heterogeneity and pleiotropy was reported, and the observed associations were not driven by single SNPs.
Conclusions: In the present MR analysis, serum MUFA levels were not associated with the risk of CHD, MI, CS, and IS. Further research, evaluating more MUFAs, is required to elucidate the links between MUFAs and CVD to contribute to health policy decisions in reducing CVD risk.
Introduction
Monounsaturated fatty acids (MUFAs) are a subgroup of fatty acids containing a single double bond, with two forms of configuration: cis and trans (1). Major sources of exogenous MUFAs include vegetable oils, high-fat fruits (such as olives and avocado), red meat, milk products, and nuts (2). MUFAs are also endogenously synthesized in the liver and the adipose tissue by microsomal stearoyl-CoA desaturase-1 (SCD1) from their saturated fatty acid–acetyl-coenzyme A precursors (3).
It is widely known that fatty acid metabolism and composition can be altered during diseases, resulting in beneficial (4, 5) or adverse events (6, 7). In this context, cardiovascular (CV) health may be affected by MUFAs through effects on various markers associated with CHD (8), including serum lipid and lipoprotein profile (9), vascular function markers (10), and postprandial vascular function (11). Indeed some sources of MUFA, such as plant oils, may decrease the risk of CV disease (CVD) in the general population (12). For example, supplemented palmitoleate beneficially influenced the lipid profile [i.e., decreased triglycerides and low-density lipoprotein cholesterol (LDL-C) and increased high-density lipoprotein cholesterol (HDL-C)] as well as reduced the high-sensitivity C-reactive protein (CRP) levels of adults with dyslipidemia (13). Furthermore, circulating palmitoleate levels positively correlated with insulin sensitivity (14).
A significant association between dietary MUFAs and CV risk has been suggested, but the underlying mechanisms are not fully understood yet. A possible explanation may be that endogenously synthesized MUFAs are the major substrate for hepatic triacylglycerol (TAG) synthesis (15), a CVD risk factor (16). Furthermore, serum MUFAs are associated with inflammation and particularly with serum CRP levels (17, 18). Consequently, serum MUFAs can lead to CVD via promoting the inflammation process (15).
The activity of SCD1 is enhanced in various cardiometabolic diseases, including diabetes, obesity, and atherosclerosis (19). The increased activity of SCD1 was reported to promote endogenous MUFA synthesis in patients with chronic kidney disease (CKD) (15). In these patients, the serum MUFA levels were positively related to TAG, CRP, and CKD progression and negatively related to HDL-C and LDL-C (15). Furthermore, MUFAs were a strong predictor of CVD incidence in CKD patients (15).
Overall, there is conflicting and limited evidence in relation to the links between serum MUFAs and CVD. The aim of the present study was to evaluate the causal associations of genetically determined serum MUFAs with coronary heart disease (CHD), myocardial infarction (MI), cardioembolic stroke (CS), and ischemic stroke (IS) by applying Mendelian randomization (MR) analysis on data from the largest genome-wide association studies (GWAS) on MUFAs.
Methods
Study Design
A two-sample MR study design was used, in which summary statistics from different GWAS were analyzed for the exposures (serum MUFAs) and outcomes (CHD, MI, CS, and IS), to estimate the effects of exposure on outcome (20). Essentially, we applied methods to estimate the unbiased effect of genetic predictors of serum MUFAs to extensively genotyped case–control studies of CHD, MI, CS, and IS.
Genetic Predictors of Exposures
We retrieved summary data for the associations between single-nucleotide polymorphisms (SNPs) and four circulating MUFAs [i.e., 10-heptadecenoate (17:1), myristoleic acid (14:1; tetradecenoic), oleic acid (18:1; octadecenoic), and palmitoleic acid (16:1; hexadecenoic)] from the GWAS (including 7,824 adult samples of European ancestry) (Supplementary Table 1). Genotyping, quality control, and imputation procedures are described elsewhere (21). If a SNP was unavailable for the outcome GWAS summary statistics, we identified proxy SNPs with a minimum linkage disequilibrium (LD) r2 = 0.8. To minimize bias in effect estimates induced by the correlation between SNPs, we restricted our genetic instrument to independent SNPs not in LD (p = 0.0001). We refer to a set of SNPs that proxy serum MUFAs as “genetic instruments.”
Genetic Predictors of Outcomes
Genetic associations with CHD were obtained from the largest publicly available extensively genotyped CHD case (n ≤ 76,014)–control (n ≤ 264,785) study based on a meta-analysis, with the use of double genomic control correction, of the CARDIoGRAMplusC4D 1000 Genomes case(n = 60,801)–control (n = 123,504) study, the UK Biobank SOFT CAD study (cases n = 10,801, controls n = 137,371), and two small case (n = 4,120)–control (n = 3,910) studies from Germany and Greece (22). The CARDIoGRAMplusC4D 1000 Genomes participants are largely of European descent (77%) with detailed phenotyping of CHD, MI, or both based on medical records, clinical diagnosis, and procedures that indicate CHD (22, 23).
Genetic associations with different stroke types were obtained from the largest available extensively genotyped dataset, i.e., the METASTROKE, a collaboration of the International Stroke Genetics Consortium, including GWAS data on 34,217 ischemic cases and 404,630 controls of European ancestry from across 15 countries (24). The majority of the cases involved brain imaging confirmation. Additional phenotype descriptions and details of individual studies, including data collection and genetic data quality control procedures, are reported elsewhere (24).
Statistics
We combined the effect of instruments using inverse variance weighted (IVW) method. To address the potential effect of pleiotropic variants on the final effect estimate, we performed sensitivity analysis, including weighted median (WM) and MR-Egger. The sensitivity analysis was conducted using the leave-one-out method to identify instruments that might drive the MR results. The WM estimate provides correct estimates as long as SNPs accounting for ≥50% of the weight are valid instruments. Inverse variance is used to weight the variants and bootstrapping is applied to estimate the confidence intervals (CIs) (20). MR-Egger is able to make estimates even under the assumption that all SNPs are invalid instruments, as long as the assumption of instrument strength independent of direct effect (InSIDE) is satisfied (20). However, the InSIDE assumption cannot be easily verified. Average directional pleiotropy across genetic variants was assessed from the p-value of the intercept term from MR-Egger (20). Furthermore, causal estimates in MR-Egger are less precise than those obtained by using IVW MR (25). The analysis using MR-Egger has a lower false-positive rate but a higher false-negative rate than IVW, i.e., it has a lower statistical power (26).
Heterogeneity between individual genetic variant estimates was assessed by the use of the Q′ heterogeneity statistic (27). The Q′ statistic uses modified second-order weights that are a derivation of a Taylor series expansion, taking into account the uncertainty in both the numerator and the denominator of the instrumental variable ratio (27). Linkage disequilibrium (LD) clumping (0.0001) was applied on all SNPs with p-value less than GWAS level.
Sensitivity Analysis
MR-Egger and MR pleiotropy residual sum and outlier (MR-PRESSO) tests were used for sensitivity analysis (27). MR-Egger and MR-PRESSO may provide correct estimates as long as the instrument strength independent of direct effect assumption is satisfied (26). MR-Egger can be imprecise, particularly if the associations for SNPs on exposure are similar or the number of genetic instruments is low (27). A non-null MR-Egger intercept suggests that the IVW estimate is invalid. MR-Egger does not explicitly identify outliers. MR-PRESSO detects and, if necessary, corrects for potentially pleiotropic outliers (27). The MR-PRESSO framework detects effect estimates that are outliers and removes them from the analysis by regressing the variant–outcome associations on variant–exposure associations. A global heterogeneity test is then implemented to compare the observed distance between residual sums of squares of all variants to the regression line with the distance expected under the null hypothesis of no pleiotropy (28).
MR-robust adjusted profile score (RAPS) was also applied. This method can correct for pleiotropy using robust adjusted profile scores. We consider, as results, causal estimates that agreed in direction and magnitude across MR methods, passed nominal significance in IVW MR, and did not show evidence of bias from horizontal pleiotropy using heterogeneity tests. All analyses were performed using the R software (version 3.4.2 R Core Team, 2017).
Ethics
The present analysis uses published or publicly available summary data. No original data were collected for this study. Ethical approval for each of the studies included in the present analysis can be found in the original publications (including informed consent from each participant). The study conforms to the ethical guidelines of the 1975 Declaration of Helsinki.
Results
The instrument associations for serum MUFA levels are shown in the Supplementary Table 1. The instruments have F-statistics higher than threshold, making significant bias from the use of weak instruments unlikely (29). The results, expressed as Beta-coefficient for serum MUFAs per one standard deviation (SD) increase in outcomes, are presented in Tables 1–4.
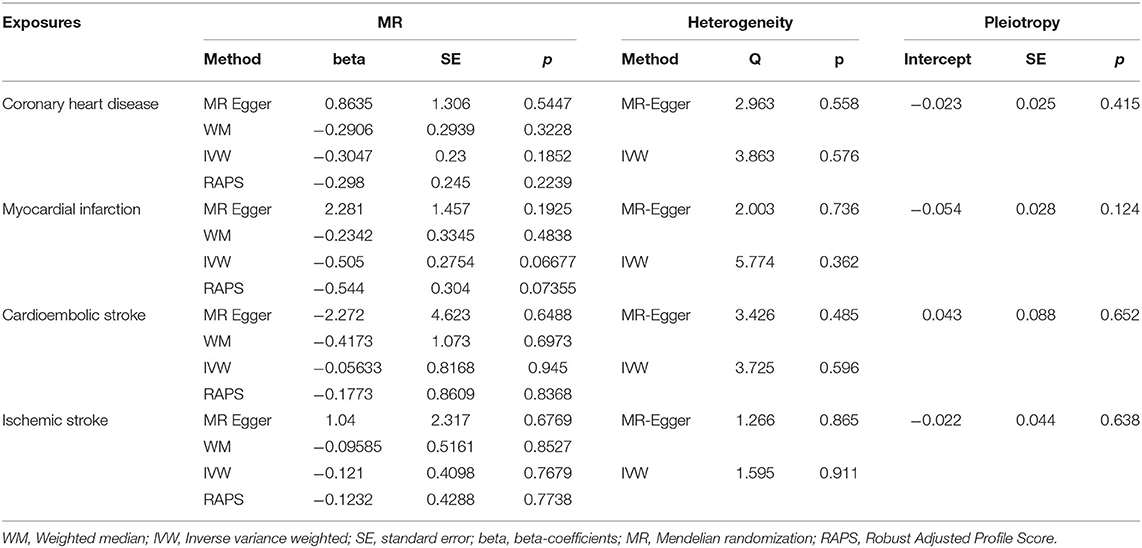
Table 1. Results of the Mendelian Randomization (MR) analysis for 10-heptadecenoate (17:1) and cardiovascular events.
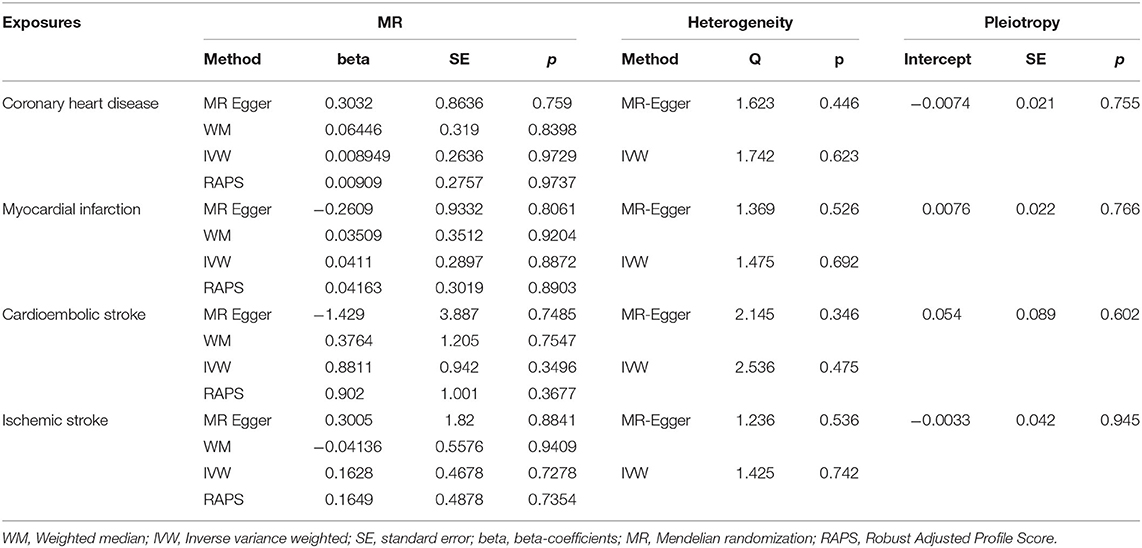
Table 2. Results of the Mendelian Randomization (MR) analysis for Myristoleic acid (14:1) and cardiovascular events.
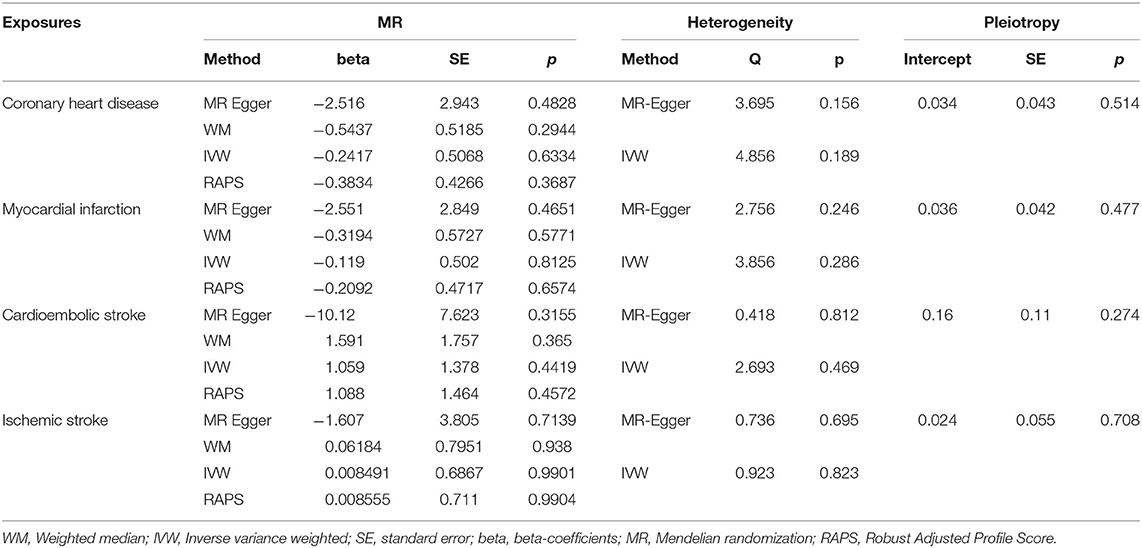
Table 3. Results of the Mendelian Randomization (MR) analysis for Oleic acid (18:1) and cardiovascular events.
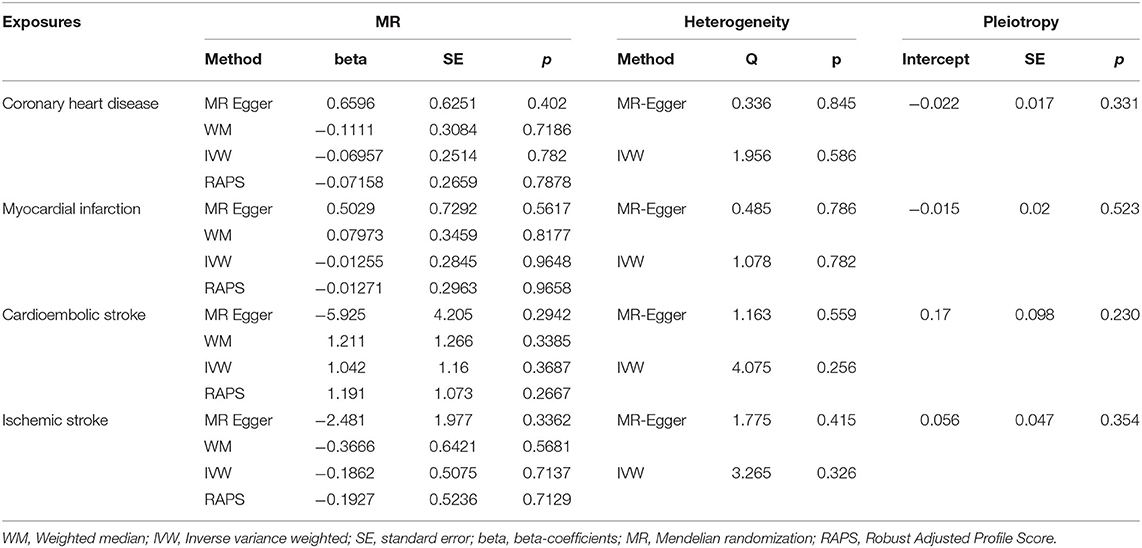
Table 4. Results of the Mendelian Randomization (MR) analysis for Palmitoleic acid (16:1) and cardiovascular events.
Genetically higher-serum 10-heptadecenoate levels had no significant effect on the risk of CHD (IVW = Beta: −0.304, p = 0.185, Table 1), MI (IVW = Beta: −0.505, p = 0.066, Table 1), CS (IVW = Beta: −0.056, p = 0.945, Table 1), and IS (IVW = Beta: −0.121, p = 0.767, Table 1). Similar results were obtained for myristoleic acid (for CHD: IVW = Beta: 0.008, p = 0.972; for MI: IVW = Beta: 0.041, p = 0.887; for CS: IVW = Beta: 0.881, p = 0.349; for IS: IVW = Beta: 0.162, p = 0.727, Table 2), oleic acid (for CHD: IVW = Beta: −0.2417, p = 0.6334; for MI: IVW = Beta: −0.119, p = 0.8125; for CS: IVW = Beta: 1.059, p = 0.4419; for IS: IVW = Beta: 0.008491, p = 0.9901, Table 3), and palmitoleic acid (for CHD: IVW = Beta: −0.06957, p = 0.782; for MI: IVW = Beta: −0.01255, p = 0.9648; for CS: IVW = Beta: 1.042, p = 0.3687; for IS: IVW = Beta: −0.1862, p = 0.7137, Table 4).
Heterogeneity results and pleiotropy bias are also shown in Tables 1–4. Estimation based on both MR Egger and IVW was higher than 0.05, indicating low heterogeneity (all IVW p > 0.256, all MR Egger p > 0.156). Furthermore, MR-PRESSO analysis did not indicate any outliers for all estimates. The horizontal pleiotropy test, with very negligible Egger regression intercept, also showed a low likelihood of pleiotropy for all of our estimations (all p > 0.124). The results of the MR-RAPS were identical with the IVW estimates, highlighting again a low chance of pleiotropy. The leave-one-out method demonstrated that the associations were not driven by single SNPs.
Discussion
The present MR analysis showed that genetically higher-serum MUFA (10-heptadecenoate, myristoleic, oleic, and palmitoleic acids) levels did not affect the risk of CHD, MI, CS, and IS. These associations were not driven by single SNPs, and there was a low chance of heterogeneity and pleiotropy.
Heptadecanoic acid was inversely associated with the risk for MI in 385 women in a population-based cohort (OR = 0.74, 95% CI: 0.58–0.95); in a multivariate analysis, however, this link became non-significant (30). Furthermore, among 2,907 US adults followed up for 22 years, no significant associations were observed between heptadecanoic acid and CVD, CHD, or stroke (31). Similarly, heptadecanoic acid level was not related to the risk of total stroke, IS, or hemorrhagic stroke in the Health Professionals Follow-Up Study (51,529 men) and the Nurses' Health Study (121,700 women) (32). However, a previous meta-analysis (13 prospective studies; 7,680 CVD cases) showed a marginal inverse correlation between heptadecanoic acid and CVD events (relative risk = 0.82, 95% CI: 0.68–0.99) (33). Data on the associations of myristoleic acid with CVD are missing.
Plasma oleic acid was a predictor for CVD events (HR = 1.41; p = 0.008) among 6,568 participants of the Multi-Ethnic Study of Atherosclerosis (34). Furthermore, the multivariable-adjusted OR for IS related to a one-SD increment in serum oleic acid was 1.20 (95% CI: 1.01–1.43) in a nested prospective case–control study involving participants from the Women's Health Initiative Observational Study (35). In contrast, oleic acid was inversely associated with acute MI (OR = 0.42; p = 0.03) in a case–control study (n = 297) (36). Furthermore, there is evidence that oleic acid may act protectively against stroke (37). In this context, higher plasma oleic acid was related to 73% lower stroke incidence (95% CI: 10–92%; p = 0.03) among 1,245 participants from the Three-City Study followed up for a median of 5.25 years (38).
Palmitelaidic acid was related to a lower risk of CVD after adjusting for age, gender, and race in a cross-sectional study using data from the National Health and Nutrition Examination Survey 1999–2000 and 2009–2010 (39). Similarly, palmitic acid was inversely associated with acute MI (OR = 0.58; p = 0.03) in a case–control study (n = 297) (36). In contrast, a high content of palmitoleic acid was suggested as a marker of increased CHD risk (40). In this context, in a prospective nested case–control study of 12,840 participants from the Circulatory Risk in Communities Study, the multivariate OR of coronary artery disease for the highest vs. the lowest quartiles of palmitoleic acid was 3.2 (95% CI: 1.7–6.1) (41). Furthermore, positive linear associations were observed between palmitoleic acid and IS [for plasma cholesterol ester fraction: HR = 1.86 (95% CI: 1.20–2.87); p = 0.003; for phospholipid fraction: HR = 1.52 (95% CI: 0.99–2.34); p = 0.005] in 3,870 participants from the Minneapolis field center of the Atherosclerosis Risk in Communities study (35). Similarly, a previous meta-analysis (13 prospective studies; n = 7,680 CVD cases) found no association between trans-palmitoleic acid, CHD, and stroke (33). Trans-palmitoleic acid levels did not correlate with the risk of total stroke, IS, or hemorrhagic stroke in two large cohort studies [i.e., the Health Professionals Follow-Up Study (51,529 men) and the Nurses' Health Study (121,700 women)] (32). Finally, in another meta-analysis including 32 observational studies of dietary fatty acids (512,420 participants) and 27 randomized clinical trials of fatty acid supplementation (105,085 participants), no associations were observed between fatty acids and coronary disease (42).
In conclusion, the present MR analysis showed that serum MUFA levels did not correlate with the risk of CHD, MI, CS, and IS. Further studies, investigating also other MUFAs, are needed to clarify the links between MUFAs, CHD, MI, CS, and IS. This research is clinically important for health policy decisions in reducing CVD risk.
Data Availability Statement
All datasets presented in this study are included in the article/Supplementary Material.
Author Contributions
All authors contributed to the design, interpretation, and writing of the paper.
Conflict of Interest
NK has given talks, attended conferences, and participated in trials sponsored by Astra Zeneca, Bausch Health, Boehringer Ingelheim, Elpen, Menarini, Mylan, NovoNordisk, Sanofi, and Servier.
The remaining authors declare that the research was conducted in the absence of any commercial or financial relationships that could be construed as a potential conflict of interest.
Supplementary Material
The Supplementary Material for this article can be found online at: https://www.frontiersin.org/articles/10.3389/fnut.2020.00123/full#supplementary-material
References
1. Kris-Etherton PM. Monounsaturated fatty acids and risk of cardiovascular disease. Circulation. (1999) 100:1253–8. doi: 10.1161/01.CIR.100.11.1253
2. Siriwardhana N, Kalupahana NS, Cekanova M, LeMieux M, Greer B, Moustaid-Moussa N. Modulation of adipose tissue inflammation by bioactive food compounds. J Nutr Biochem. (2013) 24:613–23. doi: 10.1016/j.jnutbio.2012.12.013
3. Legrand-Poels S, Esser N, L'homme L, Scheen A, Paquot N, Piette J. Free fatty acids as modulators of the NLRP3 inflammasome in obesity/type 2 diabetes. Biochem Pharmacol. (2014) 92:131–41. doi: 10.1016/j.bcp.2014.08.013
4. Barberger-Gateau P, Samieri C, Cunnane SC. Long-chain omega3 polyunsaturated fatty acids and cognition in older people: interaction with APOE genotype. OCL. (2016) 23:D111. doi: 10.1051/ocl/2015022
5. Hennessy AA, Ross RP, Devery R, Stanton C. The health promoting properties of the conjugated isomers of α-linolenic acid. Lipids. (2011) 46:105–19. doi: 10.1007/s11745-010-3501-5
6. Huang X, Lindholm B, Stenvinkel P, Carrero JJ. Dietary fat modification in patients with chronic kidney disease: n-3 fatty acids and beyond. J Nephrol. (2013) 26:960–74. doi: 10.5301/jn.5000284
7. Turolo S, Edefonti A, Syren ML, Marangoni F, Morello W, Agostoni C, et al. Fatty acids in nephrotic syndrome and chronic kidney disease. J Renal Nutr. (2018) 28:145–55. doi: 10.1053/j.jrn.2017.08.005
8. Elizondo A, Araya J, Rodrigo R, Poniachik J, Csendes A, Maluenda F, et al. Polyunsaturated fatty acid pattern in liver and erythrocyte phospholipids from obese patients. Obesity. (2007) 15:24–31. doi: 10.1038/oby.2007.518
9. Mika A, Sledzinski T. Alterations of specific lipid groups in serum of obese humans: a review. Obes Rev. (2017) 18:247–72. doi: 10.1111/obr.12475
10. Sun K, Tordjman J, Clément K, Scherer PE. Fibrosis and adipose tissue dysfunction. Cell Metab. (2013) 18:470–7. doi: 10.1016/j.cmet.2013.06.016
11. Yetukuri L, Katajamaa M, Medina-Gomez G, Seppänen-Laakso T, Vidal-Puig A, Orešič M. Bioinformatics strategies for lipidomics analysis: characterization of obesity related hepatic steatosis. BMC Syst Biol. (2007) 1:12. doi: 10.1186/1752-0509-1-12
12. Hammad S, Pu S, Jones PJ. Current evidence supporting the link between dietary fatty acids and cardiovascular disease. Lipids. (2016) 51:507–17. doi: 10.1007/s11745-015-4113-x
13. Bernstein AM, Roizen MF, Martinez L. Purified palmitoleic acid for the reduction of high-sensitivity C-reactive protein and serum lipids: a double-blinded, randomized, placebo controlled study. J Clin Lipidol. (2014) 8:612–7. doi: 10.1016/j.jacl.2014.08.001
14. Stefan N, Kantartzis K, Celebi N, Staiger H, Machann J, Schick F, et al. Circulating palmitoleate strongly and independently predicts insulin sensitivity in humans. Diabetes Care. (2010) 33:405–7. doi: 10.2337/dc09-0544
15. Mika A, Sikorska-Wiśniewska M, Małgorzewicz S, Stepnowski P, Alicja D, Tomasz S, et al. Potential contribution of monounsaturated fatty acids to cardiovascular risk in chronic kidney disease. Pol Arch Intern Med. (2018) 128:755–63. doi: 10.20452/pamw.4376
16. Jacobson TA, Ito MK, Maki KC, Orringer CE, Bays HE, Jones PH, et al. National lipid association recommendations for patient-centered management of dyslipidemia: part 1–executive summary. J Clin Lipidol. (2014) 8:473–88. doi: 10.1016/j.jacl.2014.07.007
17. Qatanani M, Lazar MA. Mechanisms of obesity-associated insulin resistance: many choices on the menu. Genes Dev. (2007) 21:1443–55. doi: 10.1101/gad.1550907
18. Kaska L, Mika A, Stepnowski P, Proczko M, Ratnicki-Sklucki K, Sledzinski T, et al. The relationship between specific fatty acids of serum lipids and serum high sensitivity C-reactive protein levels in morbidly obese women. Cell Physiol Biochem. (2014) 34:1101–8. doi: 10.1159/000366324
19. Liu J, Cinar R, Xiong K, Godlewski G, Jourdan T, Lin Y, et al. Monounsaturated fatty acids generated via stearoyl CoA desaturase-1 are endogenous inhibitors of fatty acid amide hydrolase. Proc Natl Acad Sci USA. (2013) 110:18832–7. doi: 10.1073/pnas.1309469110
20. Bowden J, Davey Smith G, Haycock PC, Burgess S. Consistent estimation in mendelian randomization with some invalid instruments using a weighted median estimator. Genet Epidemiol. (2016) 40:304–14. doi: 10.1002/gepi.21965
21. Shin SY, Fauman EB, Petersen AK, Krumsiek J, Santos R, Huang J, et al. An atlas of genetic influences on human blood metabolites. Nat Genet. (2014) 46:543–50. doi: 10.1038/ng.2982
22. Nelson CP, Goel A, Butterworth AS, Kanoni S, Webb TR, Marouli E, et al. Association analyses based on false discovery rate implicate new loci for coronary artery disease. Nat Genet. (2017) 49:1385–91. doi: 10.1038/ng.3913
23. Nikpay M, Goel A, Won HH, Hall LM, Willenborg C, Kanoni S, et al. A comprehensive 1,000 genomes-based genome-wide association meta-analysis of coronary artery disease. Nat Genet. (2015) 47:1121–30. doi: 10.1038/ng.3396
24. Malik R, Chauhan G, Traylor M, Sargurupremraj M, Okada Y, Mishra A, et al. Multiancestry genome-wide association study of 520,000 subjects identifies 32 loci associated with stroke and stroke subtypes. Nat Genet. (2018) 50:524–37. doi: 10.1038/s41588-018-0058-3
25. Bowden J, Davey Smith G, Burgess S. Mendelian randomization with invalid instruments: effect estimation and bias detection through egger regression. Int J Epidemiol. (2015) 44:512–25. doi: 10.1093/ije/dyv080
26. Burgess S, Bowden J, Fall T, Ingelsson E, Thompson SG. Sensitivity analyses for robust causal inference from mendelian randomization analyses with multiple genetic variants. Epidemiol. (2017) 28:30–42. doi: 10.1097/EDE.0000000000000559
27. Bowden J, Del Greco MF, Minelli C, Davey Smith G, Sheehan N, Thompson J. A framework for the investigation of pleiotropy in two-sample summary data mendelian randomization. Stat Med. (2017) 36:1783–802. doi: 10.1002/sim.7221
28. Verbanck M, Chen CY, Neale B, Do R. Detection of widespread horizontal pleiotropy in causal relationships inferred from Mendelian randomization between complex traits and diseases. Nat Genet. (2018) 50:693–8. doi: 10.1038/s41588-018-0099-7
29. Palmer TM, Lawlor DA, Harbord RM, Sheehan NA, Tobias JH, Timpson NJ, et al. Using multiple genetic variants as instrumental variables for modifiable risk factors. Stat Methods Med Res. (2012) 21:223–42. doi: 10.1177/0962280210394459
30. Warensjö E, Jansson J-H, Cederholm T, Boman K, Eliasson M, Hallmans G, et al. Biomarkers of milk fat and the risk of myocardial infarction in men and women: a prospective, matched case-control study. Am J Clin Nutr. (2010) 92:194–202. doi: 10.3945/ajcn.2009.29054
31. de Oliveira Otto MC, Lemaitre RN, Song X, King IB, Siscovick DS, Mozaffarian D. Serial measures of circulating biomarkers of dairy fat and total and cause-specific mortality in older adults: the cardiovascular health study. Am J Clin Nutr. (2018) 108:476–84. doi: 10.1093/ajcn/nqy117
32. Yakoob MY, Shi P, Hu FB, Campos H, Rexrode KM, Orav EJ, et al. Circulating biomarkers of dairy fat and risk of incident stroke in US men and women in 2 large prospective cohorts. Am J Clin Nutr. (2014) 100:1437–47. doi: 10.3945/ajcn.114.083097
33. Liang J, Zhou Q, Kwame Amakye W, Su Y, Zhang Z. Biomarkers of dairy fat intake and risk of cardiovascular disease: a systematic review and meta analysis of prospective studies. Crit Rev Food Sci Nutr. (2018) 58:1122–30. doi: 10.1080/10408398.2016.1242114
34. Steffen BT, Duprez D, Szklo M, Guan W, Tsai MY. Circulating oleic acid levels are related to greater risks of cardiovascular events and all-cause mortality: the multi-ethnic study of atherosclerosis. J Clin Lipidol. (2018) 12:1404–12. doi: 10.1016/j.jacl.2018.08.004
35. Yamagishi K, Folsom AR, Steffen LM, Investigators AS. Plasma fatty acid composition and incident ischemic stroke in middle-aged adults: the atherosclerosis risk in communities (ARIC) study. Cerebrovasc Dis. (2013) 36:38–46. doi: 10.1159/000351205
36. Lopes C, Aro A, Azevedo A, Ramos E, Barros H. Intake and adipose tissue composition of fatty acids and risk of myocardial infarction in a male portuguese community sample. J Am Dietetic Assoc. (2007) 107:276–86. doi: 10.1016/j.jada.2006.11.008
37. Ricci S, Celani MG, Righetti E, Caruso A, De Medio G, Trovarelli G, et al. Fatty acid dietary intake and the risk of ischaemic stroke: a multicentre case-control study. J Neurol. (1997) 244:360–4. doi: 10.1007/s004150050102
38. Samieri C, Féart C, Proust-Lima C, Peuchant E, Tzourio C, Stapf C, et al. Olive oil consumption, plasma oleic acid, and stroke incidence: the three-city study. Neurology. (2011) 77:418–25. doi: 10.1212/WNL.0b013e318220abeb
39. Zhang Q, Yang Y, Hu M, Li H, Zhong Q, Huang F. Relationship between plasma trans-fatty acid isomer concentrations and self-reported cardiovascular disease risk in US adults. Int J Food Sci Nutr. (2018) 69:976–84. doi: 10.1080/09637486.2018.1428538
40. Messner T, Sihm H, Vessby B. Fatty acid composition in serum among males 4-16 years after myocardial infarction. Int J Circumpolar Health. (1998) 57:22–31.
41. Chei C-L, Yamagishi K, Kitamura A, Kiyama M, Sankai T, Okada T, et al. Serum fatty acid and risk of coronary artery disease—circulatory risk in communities study (CIRCS). Circ J. (2018) 82:3013–20. doi: 10.1253/circj.CJ-18-0240
Keywords: mendelian randomization, serum monounsaturated fatty acids, coronary heart disease, myocardial infarction, cardioembolic stroke, ischemic stroke
Citation: Mazidi M, Katsiki N, Shekoohi N and Banach M (2020) Monounsaturated Fatty Acid Levels May Not Affect Cardiovascular Events: Results From a Mendelian Randomization Analysis. Front. Nutr. 7:123. doi: 10.3389/fnut.2020.00123
Received: 12 May 2020; Accepted: 30 June 2020;
Published: 02 September 2020.
Edited by:
Daniele Vergara, University of Salento, ItalyReviewed by:
Guy Rousseau, Université de Montréal, CanadaMeghit Boumediene Khaled, University of Sidi-Bel-Abbès, Algeria
Copyright © 2020 Mazidi, Katsiki, Shekoohi and Banach. This is an open-access article distributed under the terms of the Creative Commons Attribution License (CC BY). The use, distribution or reproduction in other forums is permitted, provided the original author(s) and the copyright owner(s) are credited and that the original publication in this journal is cited, in accordance with accepted academic practice. No use, distribution or reproduction is permitted which does not comply with these terms.
*Correspondence: Mohsen Mazidi, bW9oc2VuLm1hemlkaUBrY2wuYWMudWs=