Genome-Wide SNP Discovery and Mapping QTLs for Seed Iron and Zinc Concentrations in Chickpea (Cicer arietinum L.)
- 1Department of Genetics and Plant Breeding, University of Agricultural Sciences - Raichur (UAS-R), Raichur, India
- 2Center of Excellence in Genomics and Systems Biology, International Crops Research Institute for the Semi-Arid Tropics (ICRISAT), Hyderabad, India
- 3Zonal Agricultural Research Station, University of Agricultural Sciences - Raichur, Kalaburagi, India
Biofortification through plant breeding is a cost-effective and sustainable approach towards addressing micronutrient malnutrition prevailing across the globe. Screening cultivars for micronutrient content and identification of quantitative trait loci (QTLs)/genes and markers help in the development of biofortified varieties in chickpea (Cicer arietinum L.). With the aim of identifying the genomic regions controlling seed Fe and Zn concentrations, the F2:3 population derived from a cross between MNK-1 and Annigeri 1 was genotyped using genotyping by sequencing approach and evaluated for Fe and Zn concentration. An intraspecific genetic linkage map comprising 839 single nucleotide polymorphisms (SNPs) spanning a total distance of 1,088.04 cM with an average marker density of 1.30 cM was constructed. By integrating the linkage map data with the phenotypic data of the F2:3 population, a total of 11 QTLs were detected for seed Fe concentration on CaLG03, CaLG04, and CaLG05, with phenotypic variation explained ranging from 7.2% (CaqFe3.4) to 13.4% (CaqFe4.2). For seed Zn concentration, eight QTLs were identified on CaLG04, CaLG05, and CaLG08. The QTLs individually explained phenotypic variations ranging between 5.7% (CaqZn8.1) and 13.7% (CaqZn4.3). Three QTLs for seed Fe and Zn concentrations (CaqFe4.4, CaqFe4.5, and CaqZn4.1) were colocated in the “QTL-hotspot” region on CaLG04 that harbors several drought tolerance-related QTLs. We identified genes in the QTL regions that encode iron–sulfur metabolism and zinc-dependent alcohol dehydrogenase activity on CaLG03, iron ion binding oxidoreductase on CaLG04, and zinc-induced facilitator-like protein and ZIP zinc/iron transport family protein on CaLG05. These genomic regions and the associated markers can be used in marker-assisted selection to increase seed Fe and Zn concentrations in agronomically superior chickpea varieties.
Introduction
Micronutrients are vital for healthy growth and development of the human body, and their adequate intake through diet is a prerequisite for various metabolic functions. However, a majority of the world's population face difficulties in meeting the recommended daily allowances (RDA). This has led to micronutrient malnutrition (also known as “hidden hunger”) that affects one in three people worldwide (1). Iron (Fe) and zinc (Zn) are important micronutrients that play a major role in the normal growth and development of humans by acting as cofactors for several proteins including hemoglobin, cytochrome, and transcription factors (2). Fe and Zn deficiency causes anemia, tissue hypoxia, dwarfism, lowered disease immunity, stunting, poor cognitive development, and orificial and acral dermatitis (3, 4). It is estimated that by 2050, around 1.4 billion women and children would be Fe deficient and 175 million zinc deficient (5). This risk will be high in Southeast Asia, Africa, and the Middle East. Micronutrient malnutrition has received increased global attention in recent decades, with efforts to address it by various strategies such as mineral supplementation and dietary diversification. Biofortification of staple crops through plant breeding is a highly cost-effective and long-term strategy to enhance micronutrient density in crop plants (6). Efforts in this direction have involved understanding the genetic architecture of Fe and Zn concentrations in seeds of cereals crops (7–10). More recently, the focus has been on pulses that serve as secondary staples, mainly because of their increased protein content (11). Among legumes, common bean (Phaseolus vulgaris), lentil (Lens culinaris), Pea (Pisum sativum), and mung bean (Vigna radiata) have been targeted for micronutrient studies (6).
Chickpea, a highly nutritious diploid (2n = 2x = 16) legume crop plant having a genome size of 738 Mbp (12) ranks second after dry beans in terms of global production among pulses, with over 14.77 million tons produced in 2017 (FAOSTAT, accessed on 17 February 2020). Chickpea is considered an excellent whole food and a source of carbohydrate (52–71%), protein (18–24%), fiber (10–23%), fat (4–10%), minerals and vitamins, dietary fiber, folate, β-carotene, and some important fatty acids (13, 14). Genomic research during the last decade enabled the decoding of the genome sequence (12) and sequencing of several germplasm lines (15–17) and provided insights into the genetics of complex abiotic and biotic stresses (18). Besides simplifying these complex traits, molecular breeding lines with enhanced drought tolerance and disease resistance were developed and released for commercial cultivation (19–21). Nevertheless, very few genomic studies have attempted to understand the variability for nutritionally important traits. The variability study (22) for physicochemical, nutritional and cooking quality traits among 30 chickpea germplasm lines indicated that higher 100-seed weight reduced cooking time. Using principal component analysis, variation for seed and flour characteristics among 79 chickpea germplasm accessions used in European breeding programs revealed higher protein and fiber in desi and higher fat content in kabuli types (23). Recently, studies on genotype and environment effects on seed quality traits demonstrated that environment plays an important role in variation in amylose and amylopectin content (24). None of these studies utilized genomics tools to understand the genetics of these traits.
Marker trait associations for protein content have been reported using simple sequence repeat markers (25). In the case of chickpea, there are very few studies on Fe and Zn accumulation in seeds. Genetic variations in the concentration of Fe (36.2–86.4 mg kg−1) and Zn (18.6–62.2 mg kg−1) were reported in 94 diverse accessions of chickpea (26). Fe and Zn in seeds appear to be a complex trait governed by a number of major genes/quantitative trait loci (QTLs), and the concentration of these minerals is highly influenced by edaphic and environmental factors (2). Recently, besides the variation in seed mineral concentration among cultivars and breeding lines (27, 28), markers linked with seed Fe and Zn content were reported in a select set of germplasm collections (2, 26). The identification of new sources of Fe and Zn concentrations, their genetic dissection, and using them to diversify the breeding population is a continuous process in developing high-yielding chickpea varieties with enhanced nutrition content. Cost-effective and high-throughput discovery and genotyping of single nucleotide polymorphism (SNP) markers using genotyping-by-sequencing (GBS) approach (29) have been widely used in many crop species including chickpea to assess diversity, in genome-wide association studies, in constructing high-density genetic linkage maps, and QTL identification (2, 30–33).
This study reports on high-throughput genome-wide marker discovery and genotyping using GBS approach and QTLs for Fe and Zn concentrations in chickpea, using an F2:3 intraspecific mapping population developed from MNK-1 × Annigeri 1.
Materials and Methods
Mapping Population and DNA Extraction
A mapping population consisting of 188 F2 plants was developed from a cross between MNK-1 and Annigeri 1. MNK-1 is a kabuli type, extra-large-seeded chickpea variety having Fe and Zn concentrations of 72.88 and 30.98 mg kg−1, respectively. Annigeri 1 is a desi-type, medium seed size variety having Fe and Zn concentrations of 98.67 and 37.07 mg kg−1, respectively. Fresh, young leaves were collected from parental lines, and each F2 individual and high-quality genomic DNA was extracted using high throughput NucleoSpin® 96 Plant II Kit (Macherey-Nagel, Düren, Germany) following the manufacturer's protocol. The DNA was quantified using a spectrophotometer (Shimadzu UV160A, Japan) and used to prepare the GBS library for SNP discovery. Individual F2 plants were selfed, and seeds harvested were advanced to develop an F2:3 population for phenotyping seed Fe and Zn contents.
Genotyping-by-Sequencing and SNP Calling
Genotyping by sequencing was carried out for SNP calling between the parental genotypes and genotyping the 188 F2 progenies following the protocol described by Elshire et al. (29). GBS libraries were prepared by restriction digestion of DNA of each of the F2s as well as the parents with ApeKI endonuclease (recognition site: G/CWCG), followed by ligation with uniquely barcoded adapters using T4 DNA ligase. An equal proportion of barcoded adapters ligated DNA fragments from each sample were mixed to construct the GBS libraries, which were amplified and purified in order to remove excess adapters, followed by sequencing on the HiSeq 2500 platform (Illumina Inc., San Diego, CA, USA). The sequence reads from fastq files were used for SNP identification using GBSv2 (34) pipeline implemented in TASSEL v5 (35). In short, the sequencing reads were first searched for barcode information and reads with no Ns. The barcode containing reads was then demultiplexed according to the barcode sequence and trimmed. The remaining good quality and distinct reads (tags) were retrieved in the form of fastq and aligned against the draft genome sequence of chickpea (36) using Bowtie2 (37). The alignment file was then parsed through the remaining GBSv2 pipeline for SNP calling and genotyping. The SNPs identified were then filtered in order to retain data with contrasting alleles in parental genotypes and those having <30% missing data for further analysis.
Phenotyping for Seed Fe and Zn
During the crop season of 2019, F2:3 progenies (plant:progeny rows) were planted following augmented design at the Zonal Agricultural Research Station, Kalaburagi, Karnataka (latitude, 17.36 and longitude, 76.82). Each experimental block consisted of a single 2-m row plot with 30 cm spacing between rows and 10 cm interplant spacing. The experimental plot was divided into six blocks to reduce heterogeneity, and each occupied 27 lines plus 4 checks (MNK-1, Annigeri 1, KAK-2, and BGD-103) replicated twice in each block. Standard cultivation practices were followed to ensure healthy crop growth.
Mature seeds of each parental line and mapping individual (five to six representative plants from each F2:3 progeny line) were collected separately during harvesting stage. Care was taken to avoid the contamination of seeds with dust and inert particles during harvest and while sample preparation. Five grams of dry seed sample (with 5.6% moisture content) from each accession was ground into fine powder, and 0.5 g of fine ground powder was put in a reaction vessel to which was added 10 ml of di-acid mixture (nitric acid and hydrochloric acid in a 9:1 ratio). The vessel was sealed and loaded into the chamber and microwave of the Microwave Digestion System (Anton Paar Company, USA), which was set to run for 40 min at 180°C. After the vessels cooled down to <50°C, they were removed from the chamber and moved to an exhaust clean hood to vent excess pressure slowly. The digested samples from the vessel were then placed in a clean container and diluted with distilled water to make up a volume of 50 ml in a conical flask. They were then analyzed for Fe and Zn concentrations. An atomic absorption spectrophotometer (Analytik Jena AG, contrAA 700, Germany) was used to estimate Fe and Zn concentrations based on the absorption of light at a wavelength of 370 and 388 nm, respectively. Both Fe and Zn concentrations were estimated and expressed as mg kg−1 (ppm) seed.
Statistical Analysis
Variance analysis was done using PROC MIXED of SAS v9.4 (38), considering blocks and checks as fixed and F2:3 progeny lines as random. Repeated checks were used to estimate error mean square. Best linear unbiased predictors (BLUPs) and best linear unbiased estimates (BLUEs) were estimated for random and fixed factors, respectively. Genetic variability parameters including mean, range, frequency distribution, phenotypic coefficient of variance (PCV), genotypic coefficient of variance (GCV), broad sense heritability [h2(bs)], and genetic advance per mean (GAM) were computed using SAS.
Genetic Linkage Map Construction
The allele calls for all genotypes were compiled and used for genetic linkage analysis by employing JoinMap V4.1 program (39). In total, 185 F2s were used to construct the genetic map after discarding three individuals with insufficient data. Chi square goodness-of-fit test was performed (p < 0.05) to test the segregation distortion for each marker. Highly distorted and unlinked markers were excluded from the analysis. Regression mapping algorithm with maximum recombination frequency of 0.3 at minimum logarithm of odds (LODs) of 3 and jump threshold of 5 were used to group the markers into eight linkage groups (LGs). Ripple command was used after adding each marker locus to confirm marker order. Map distance was estimated using Kosambi's mapping function (40). The final high-resolution linkage map was generated using LinkageMapView package in R software (41).
QTL Analysis
The genotyping data of the intraspecific linkage map and phenotypic data for iron and zinc content from the F2:3 population were used for QTL analysis using QTL Cartographer V2.5 software (42). Composite interval mapping (CIM) method was used by selecting Model 6 with the default window size of 10 cM, control marker number 5, and using backward stepwise regression. For each trait, the LOD score threshold was determined by performing 1,000 permutations at significance level of p ≤ 0.05. The amount of phenotypic variation explained was determined using the coefficient of determination (R2) value and expressed as phenotypic variation explained (PVE) percentage.
Identification of Genes in QTL Regions
The QTL region's coordinates were compared to the reference genome's gff file using bedtools (43) to identify the genes lying in the QTL region and functionally categorized using UniProt KB database (http://www.uniprot.org/).
Results
Genetic Variability for Seed Fe and Zn Concentrations
The parental lines of the mapping population had wide variation for seed Fe and Zn concentrations. Parent MNK-1 had seed Fe and Zn concentrations of 72.88 and 30.98 mg kg−1, respectively, while parental line Annigeri 1 had seed Fe and Zn concentrations of 98.67 and 37.07 mg kg−1, respectively. The F2:3 population showed wide variability for both the traits (Table 1, Figure 1). Seed Fe concentration ranged from 51.60 to 118.74 mg kg−1 with a mean of 82.15 mg kg−1. Seed Zn concentration ranged from 17.89 to 45.60 mg kg−1 with a mean of 36.10 mg kg−1. Analysis of variance (ANOVA) results indicated highly significant genotypic effects (Table 2). A high GCV (21.89%) and PCV (22.17%) for seed Fe concentration and moderate GCV (14.07%) and PCV (15.11%) for seed Zn concentration were observed among the F2:3 progenies. Very high broad sense heritability values of 97.50 and 86.68% were observed for seed Fe and Zn concentrations, respectively.

Table 1. Phenotypic variation for seed iron and zinc concentrations in F2:3 generation of the cross MNK-1 × Annigeri 1.
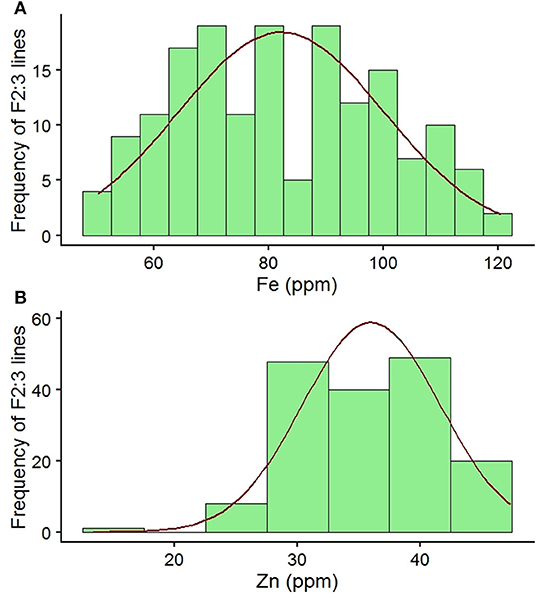
Figure 1. (A, B) Frequency distribution of seed Fe and Zn concentrations among F2:3 individuals of an intraspecific mapping population (MNK-1 × Annigeri 1) along with checks and parental lines.
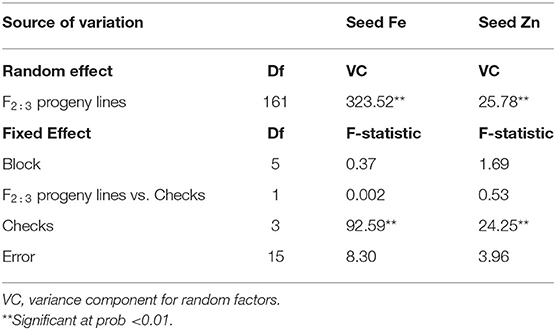
Table 2. Variance component for F2:3 progeny lines and F-statistic for block, checks, and check vs. progeny lines for seed Fe and Zn concentrations ANOVA for augmented design in F2:3 generation of the cross MNK-1 × Annigeri 1.
Identification of SNPs Using GBS Approach and Construction of Intraspecific Linkage Map
Genotype by sequencing of the parental genotypes generated ~211.46 Mb for MNK-1 and ~144.44 Mb for Annigeri1. For 188F2s, ~46.6 Gb was generated with average of ~257.76 Mb per sample. A total of 31,311 SNPs were identified, of which 7,204 (23%) were polymorphic between MNK-1 and Annigeri 1, and the remaining 17,034 (54.40%) were monomorphic. A set of 7,204 polymorphic SNPs was used for linkage map analysis. As a result, a total of 839 SNPs were mapped on the eight chickpea chromosomes postfiltration of SNPs' set (Supplementary Table 1). A total of 7,204 SNPs from GBS data were used to generate the intraspecific linkage map. Of these, 839 SNPs were grouped into eight linkage groups (CaLG), whereas the remaining 6,365 SNPs were unlinked to any of the linkage groups. Marker density per linkage group varied from 24 (CaLG05) to 318 (CaLG04), with an overall average of 104.8 markers per linkage group (Table 3, Figure 2). The final linkage map had a total length of 1,088.04 cM, with CaLG05 (164.17 cM) being the largest and CaLG08 (116.98 cM) being the smallest. The average intermarker distance in the linkage map was 1.30 cM, with CaLG04 being the densest (0.49 cM) and CaLG05 being the least dense (6.84 cM). The final linkage map is shown in Figure 2.
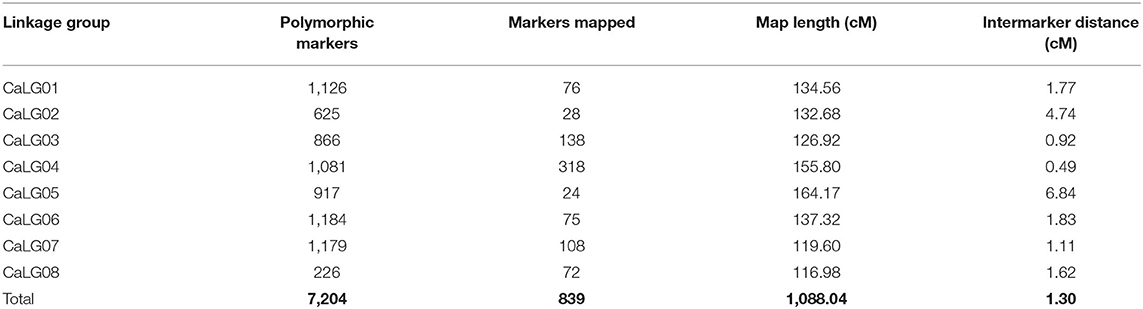
Table 3. Features of an intraspecific genetic map based on the F2 population of the cross MNK-1 × Annigeri 1.
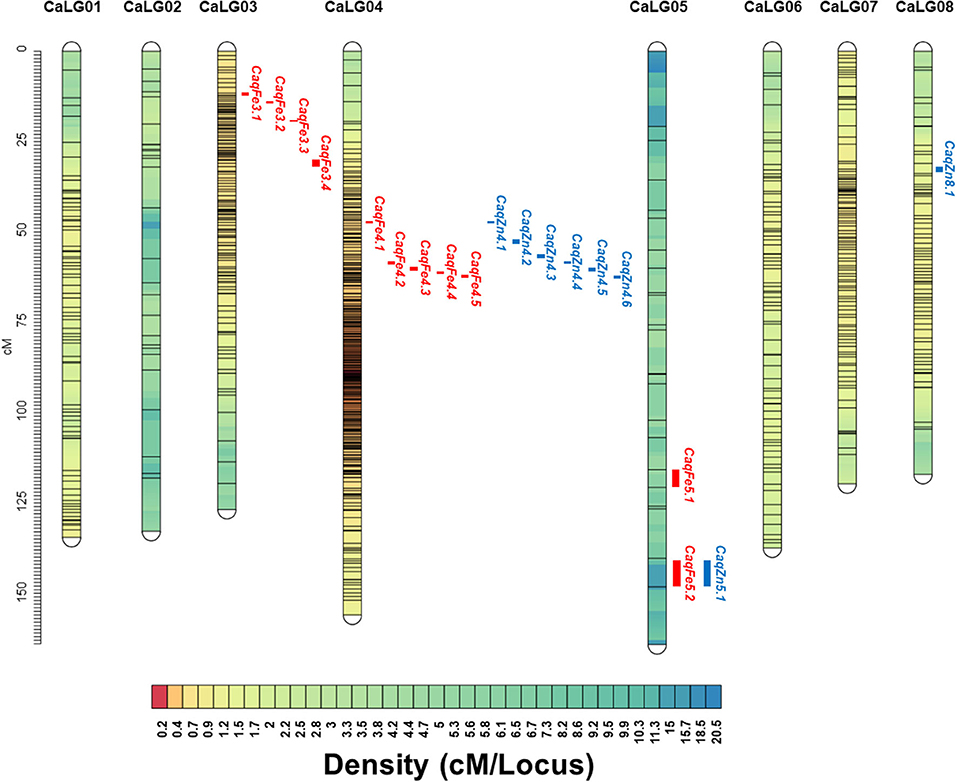
Figure 2. Intraspecific genetic linkage map of chickpea constructed using the F2 population derived from the cross MNK-1 × Annigeri 1. The linkage map with eight linkage groups (CaLG01–CaLG08) shows the quantitative trait loci (QTLs) for seed Fe and Zn concentrations; 11 QTLs for seed Fe and 8 QTLs for seed Zn concentration are shown on the left side of the corresponding linkage group.
QTL Mapping for Seed Fe and Zn Concentrations
Genotyping data of mapped 839 SNPs was integrated with the phenotypic data of the Fe and Zn concentrations and analyzed using composite interval mapping (CIM) method. For seed Fe concentration, a total of 11 QTLs were mapped on CaLG03, CaLG04, and CaLG05 with LOD thresholds ranging from 3.0 (CaqFe3.4 and CaqFe5.2) to 4.8 (CaqFe4.2) (Table 4, Figure 2). PVE by individual QTLs ranged from 7.2% (CaqFe3.4) to 13.4% (CaqFe4.2). At all the five loci on CaLG04 (CaqFe4.1, CaqFe4.2, CaqFe4.3, CaqFe4.4, and CaqFe4.5), alleles from parent MNK-1 favored seed Fe concentration. For seed Zn concentration, eight QTLs were detected on CaLG04, CaLG05, and CaLG08 (Table 4, Figure 2). QTLs CaqZn5.1 and CaqZn8.1 had the lowest LOD threshold of 3.0, whereas QTL CaqZn4.3 had the highest LOD score of 6.3. The PVE of these QTLs ranged between 5.7% (CaqZn8.1) and 13.7% (CaqZn4.3). At all the loci except CaqZn5.1, the alleles from parent MNK-1 favored seed Zn concentration.
Colocalization of Seed Fe and Zn Concentration QTLs and Genes in the QTL Regions
Based on the physical position of the SNP markers linked with the seed Fe and Zn QTLs, we found that three QTLs (CaqFe4.4, CaqFe4.5, and CaqZn4.1) are colocalized in the “QTL-hotspot” region that harbors several drought tolerance related QTLs on CaLG04, as reported earlier (Table 5). We identified a total of 7,496 genes in the QTL regions reported in the study (Supplementary Table 2). Among these, we identified three genes—Ca_20872, Ca_00798, and Ca_01283—in the CaqFe3.1 QTL region that encode for iron–sulfur assembly protein IscA-like 1, iron–sulfur cluster assembly protein IscA, and Zn-dependent alcohol dehydrogenase family class III protein, respectively. Further, gene Ca_04513 that encodes for iron ion binding oxidoreductase was identified in the CaqFe4.4 QTL region. Interestingly, we identified gene Ca_01633 that encodes for ZIP zinc/iron transport family protein in the QTL region of CaqZn5.1.
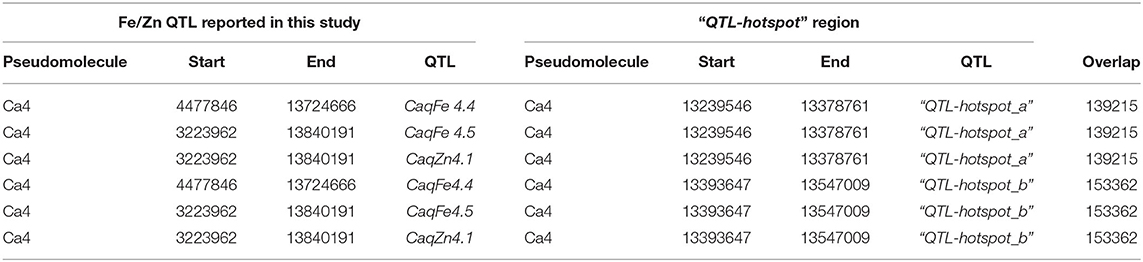
Table 5. Colocalization of Fe and Zn Quantitative trait loci (QTLs) in the “QTL-hotspot” region reported by Varshney et al. (44).
Discussion
Growing hunger and high levels of different forms of malnutrition are major challenges to achieving food and nutritional security. Effective interventions like biofortification aimed at guaranteeing access to nutritious foods are needed to address micronutrient malnutrition or hidden hunger. In several pulse crops, biofortification through plant breeding has gained momentum in the past decade (6). In chickpea, knowledge of genetic variation underlying the concentration of important seed minerals such as Fe and Zn and their association with yield-related traits is vital for accelerating breeding for biofortified varieties. In the present study, an F2:3 population of chickpea was used to identify QTLs for seed Fe and Zn concentrations.
The parental lines MNK-1 and Annigeri 1 had seed Fe concentrations of 72.88 and 98.67 mg kg−1 and seed Zn concentrations of 30.98 and 37.07 mg kg−1, respectively. The seed Fe concentration values of the cultivars are comparatively higher than the range of 49–56 mg kg−1 in chickpea cultivars reported by Vandemark et al. (28). With respect to seed Zn concentration, the parental values are comparable (35–43 mg kg−1) to Vandemark et al. (28) but higher than the range of 21–28 mg kg−1 as reported by Ray et al. (27) for chickpea cultivars. Jayalaxmi et al. (45) reported that Fe concentration varied from 26 to 146 mg kg−1 and Zn concentration from 35 to 77 mg kg−1 in 56 chickpea varieties. Analysis of variance (ANOVA) results inferred a highly significant difference among F2:3 individuals for both seed Fe and Zn concentrations (Table 2). The seed Fe and Zn concentrations were in the range of 51.60–118.74 and 17.89–45.60 mg kg−1, respectively (Table 1). It is evident from the distribution graph that F2:3 individuals recorded values beyond the checks for both seed Fe and Zn concentrations (Figure 1). In addition, a very high broad sense heritability was observed among the F2:3 individuals for both seed Fe [h2(bs) = 97.50] and Zn [h2(bs) = 86.68] concentrations. This indicates the feasibility of early generation selection that may result in improving these traits. Given that there are reports of significant environment effects on the concentration of minerals (26, 27) and this study was conducted only at a single location, better genotype × environment can be realized by further evaluating the advanced generation population across different locations or years.
Identification of genetic variants like SNPs is a key step in several genetic studies, including linkage mapping and QTL identification. Among the different genetic variants, SNP markers have become the markers of choice due to their high abundance and cost effectiveness. GBS provides increased efficiency in SNP discovery and genotyping by enabling high multiplexing of samples and simple library preparation procedures (29). This method involves only a targeted sequencing approach and does not require the complete genome sequencing of all individuals of the mapping population and parental genotypes. Due to its high-throughput efficiency, GBS has been used in recent studies in SNP identification and QTL mapping for important traits in chickpea (33, 46, 47). This study employed the GBS approach to identify SNPs in an intraspecific F2 mapping population derived from two popular cultivars of chickpea. As a result, 7,204 novel SNPs were identified and used for genetic linkage analysis. The SNPs identified in this study were higher compared to other GBS studies in chickpea (33, 46) but lower than those reported by Deokar et al. (47). Of the 7,204 SNPs used, 839 were mapped on to eight linkage groups covering a total map distance of 1,088.04 cM and average marker density of 1.30 cM. The map density is comparable with that of Jaganathan et al. (33) and less dense compared to that of Hiremath et al. (48) and Deokar et al. (47, 49). Of these 839 SNPs, a majority (37%) were mapped on CaLG04. Earlier, Jaganathan et al. (33) made similar observations that may be attributed to the presence of high repeat-rich regions in Ca4 pseudomolecule of chickpea as reported by Varshney et al. (36).
The intraspecific linkage map was utilized to identify QTLs linked to seed Fe and Zn concentrations. A total of 11 QTLs for seed Fe concentration were detected on CaLG03, CaLG04, and CaLG05, which explained phenotypic variance in the range of 7.2–13.4%. For seed Zn concentration, eight QTLs were identified on CaLG03, CaLG05, and CaLG08. The phenotypic variance explained by these QTLs ranged between 5.7 and 13.7%. Previous studies have reported QTLs for seed Fe on chromosomes 1, 4, and 6 (26) and on chromosomes 1, 3, and 4 (2) and for seed Zn on chromosomes 1, 4, and 7 (26) and on chromosomes 2 and 3 (2). In all these studies, the distribution of QTLs on multiple chromosomes clearly indicates the quantitative nature and complexity of seed Fe and Zn concentration traits.
In this study, several colocated QTLs for seed Fe and Zn concentrations were identified on CaLG04 and CaLG05. For instance, QTLs CaqFe4.1-CaqZn4.1, CaqFe4.2-CaqZn4.2, CaqFe4.3-CaqZn4.1, and CaqFe4.5-CaqZn4.1 were colocalized on CaLG04 in the same marker interval SCA4_11398699-SCA4_11244334, SCA4_23943928-SCA4_13840227, SCA4_13787448-SCA4_13845581, and SCA4_13840191-SCA4_3223962, respectively (Table 4, Figure 2). On CaLG05, QTLs CaqFe5.1-CaqZn5.1 were colocalized within the SNP marker interval of SCA5_37174786-SCA5_35312501. Previous studies have reported QTL colocalization for micronutrient concentration in chickpea on chromosomes 4, 5, and 7 (2) and also in rice (10). Hence, these genomic regions on chromosome 4 may contain conserved QTLs for seed Fe and Zn concentrations across diverse genetic backgrounds. Pleiotropy or tight linkage of genes controlling multiple traits may be the reason for the colocalization of QTLs (50). These regions with colocated QTLs will be useful for simultaneous improvement using marker-assisted selection (10).
Interestingly, QTLs for seed Fe and Zn were also mapping in the “QTL-hotspot region” reported earlier (44). This genomic region was successfully introgressed into different elite genetic backgrounds, and molecular breeding lines with enhanced climate resilience have been reported (36). The molecular breeding lines may also be rich in seed Fe and Zn, as the QTLs are in the genomic region that was introgressed. By comparing the QTL region's coordinates to the kabuli reference genome of chickpea (12), the identified genes were found to be involved in Fe- and Zn-related activities. The F2:3 population exhibited wide variation for seed Fe and Zn concentrations. A high heritability was obtained for both the traits studied.
This study was successful in using the GBS approach to develop a linkage map for an intraspecific population in chickpea. The map consisted of 839 SNPs spanning 1,088.04 cM. Genomic regions for seed Fe and Zn concentrations were mapped on multiple linkage groups, and the linked SNPs were reported. These QTLs are good candidates for further validation, functional characterization, and gene cloning to clarify the role of these genes in Fe and Zn homeostasis in chickpea. The SNPs linked to seed Fe and Zn concentrations have potential for use as informative molecular tags in marker-assisted breeding to improve seed Fe and Zn concentrations in chickpea varieties.
Data Availability Statement
All datasets generated for this study are included in the article/Supplementary Material.
Author Contributions
IS and MT conceived the idea. SS conducted the experiments, analyzed the data, and prepared the manuscript along with BM. AV and AR performed statistical analysis. VV and PB did the GBS analysis. RL, DM, LC, SY, and KJ contributed to the germplasm characterization and mapping population development and phenotyping. AC and RKV contributed consumables and to the generation of genotyping data. All authors contributed to the article and approved the submitted version.
Conflict of Interest
The authors declare that the research was conducted in the absence of any commercial or financial relationships that could be construed as a potential conflict of interest.
Acknowledgments
This work has been carried out as part of the CGIAR Research Program on GLDC. ICRISAT is a member of the CGIAR System Organization.
Supplementary Material
The Supplementary Material for this article can be found online at: https://www.frontiersin.org/articles/10.3389/fnut.2020.559120/full#supplementary-material
References
1. Ritchie H, Reay DS, Higgins P. Quantifying, projecting, and addressing India's hidden hunger. Front Sustain Food Syst. (2018) 2:11. doi: 10.3389/fsufs.2018.00011
2. Upadhyaya HD, Bajaj D, Narnoliya L, Das S, Kumar V, Gowda CLL, et al. Genome-wide scans for delineation of candidate genes regulating seed-protein content in chickpea. Front Plant Sci. (2016) 7:302. doi: 10.3389/fpls.2016.00302
3. Tulchinsky TH. Micronutrient deficiency conditions: global health issues. Public Health Rev. (2010) 32:243. doi: 10.1007/BF03391600
4. Bailey RL, West KP, Black RE. The epidemiology of global micronutrient deficiencies. Ann Nutr Metab. (2015) 66:2233. doi: 10.1159/000371618
5. Smith MR, Myers SS. Impact of anthropogenic CO2 emissions on global human nutrition. Nat Clim Chang. (2018) 8:834–9. doi: 10.1038/s41558-018-0253-3
6. Jha AB, Warkentin TD. Biofortification of pulse crops: status and future perspectives. Plant J. (2020) 9:73. doi: 10.3390/plants9010073
7. Tiwari VK, Rawat N, Chhuneja P, Neelam K, Aggarwal R, Randhawa GS. Mapping of quantitative trait Loci for grain iron and zinc concentration in diploid A genome wheat. J Hered. (2009) 100:771–6. doi: 10.1093/jhered/esp030
8. Anuradha K, Agarwal S, Venkateswara RY, Rao KV, Viraktamath BC, Sarla N. Mapping QTLs and candidate genes for iron and zinc concentrations in unpolished rice of Madhukar × Swarna RILs. Gene. (2012) 508:233–40. doi: 10.1016/j.gene.2012.07.054
9. Jin T, Zhou J, Chen J, Zhu L, Zhao Y, Huang Y. The genetic architecture of zinc and iron content in maize grains as revealed by QTL mapping and meta-analysis. Breed Sci. (2013) 63:317–24. doi: 10.1270/jsbbs.63.317
10. Calayugan MIC, Formantes AK, Amparado A, Descalsota-Empleo GI, Nha CT, Inabangan-Asilo MA, et al. Genetic analysis of agronomic traits and grain iron and zinc concentrations in a doubled haploid population of rice (Oryza sativa L.). Sci Rep. (2020) 10:2283. doi: 10.1038/s41598-020-59184-z
11. Iqbal A, Khalil IA, Ateeq N, Khan MS. Nutritional quality of important food legumes. Food Chem. (2006) 97:331–5. doi: 10.1016/j.foodchem.2005.05.011
12. Varshney RK, Song C, Saxena RK, Azam S, Yu S, Sharpe AG, et al. Draft genome sequence of chickpea (Cicer arietinum L.). provides a resource for trait improvement. Nat Biotechnol. (2013) 31:240–6. doi: 10.1038/nbt.2491
13. Yadav RDS, Srivastava JP. DUS characteristics of chickpea varieties. Seed Tech News. (2002) 32:29–30.
14. Jukanti AK, Gaur PM, Gowda CLL, Chibbar RN. Nutritional quality and health benefits of chickpea (Cicer arietinum L.): a review. Br J Nutr. (2012) 108:S11–26. doi: 10.1017/S0007114512000797
15. Thudi M, Khan AW, Kumar V, Gaur PM, Katta K, Garg V, et al. Whole genome re-sequencing reveals genome wide variations among parental lines of mapping populations in chickpea (Cicer arietinum L.). BMC Plant Biol. (2016) 16:10. doi: 10.1186/s12870-015-0690-3
16. Thudi M, Chitikineni A, Liu X, He W, Roorkiwal M, Yang W, et al. Recent breeding programs enhanced genetic diversity in both desi and kabuli varieties of chickpea (Cicer arietinum L.). Sci Rep. (2016) 6:38636. doi: 10.1038/srep38636
17. Varshney RK, Thudi M, Roorkiwal M, He W, Upadhyaya HD, Yang W, et al. Resequencing of 429 chickpea accessions from 45 countries provides insights into genome diversity, domestication and agronomic traits. Nat Genet. (2019) 51:857–64. doi: 10.1038/s41588-019-0401-3
18. Roorkiwal M, Bharadwaj C, Barmukh R, Dixit GP, Thudi M, Gaur PM, et al. Integrating genomics for chickpea improvement: achievements and opportunities. Theor Appl Genet. (2020) 133:1703–20. doi: 10.1007/s00122-020-03584-2
19. Varshney RK, Mohan SM, Gaur PM, Chamarthi SK, Singh VK, Srinivasan S, et al. Marker-assisted backcrossing to introgress resistance to Fusarium wilt (FW) race 1 and Ascochyta blight (AB) in C 214, an elite cultivar of chickpea. Plant Genome. (2014) 7:1–11. doi: 10.3835/plantgenome2013.10.0035
20. Pratap A, Chaturvedi SK, Tomar R, Rajan N, Malviya N, Thudi M, et al. Marker-assisted introgression of resistance to fusarium wilt race 2 in Pusa 256, an elite cultivar of desi chickpea. Mol Genet Genomics. (2017) 292:1237–45. doi: 10.1007/s00438-017-1343-z
21. Mannur DM, Babbar A, Thudi M, Sabbavarapu MM, Roorkiwal M, Sharanabasappa BY, et al. Super Annigeri 1 and improved JG 74: two Fusarium wilt-resistant introgression lines developed using marker-assisted backcrossing approach in chickpea (Cicer arietinum L.). Mol Plant Breed. (2019) 39:2. doi: 10.1007/s11032-018-0908-9
22. Saxena AK, Sharma S, Sandhu JS, Gupta SK, Kaur J. Variability and correlation studies for nutritional and cooking quality traits in chickpea (Cicer arietinum L.). Indian J Genet. (2013) 73:51–6. doi: 10.5958/j.0019-5200.73.1.007
23. Serrano C, Carbas B, Castanho A, Soares A, Patto MCV, Brites C. Characterization of nutritional quality traits of a chickpea (Cicer arietinum L.). germplasm collection exploited in chickpea breeding in Europe. Crop Pasture Sci. (2017) 68:1031–40. doi: 10.1071/CP17129
24. Cobos MJ, Izquierdo I, Sanz MA, Tomás A, Gil J, Flores F, et al. Genotype and environment effects on sensory, nutritional, and physical traits in chickpea (Cicer arietinum L.). Span J Agric Res. (2016) 14:e0709. doi: 10.5424/sjar/2016144-8719
25. Jadhav AA, Rayate SJ, Mhase LB, Thudi M, Chitikineni A, Harer PN, et al. Marker-trait association study for protein content in chickpea (Cicer arietinum L.). J Genet. (2015) 94:279–86. doi: 10.1007/s12041-015-0529-6
26. Diapari M, Sindhu A, Warkentin TD, Bett K, Tar'an B. Genetic diversity and association mapping of iron and zinc concentrations in chickpea (Cicer arietinum L.). Genome Res. (2014) 57:459–68. doi: 10.1139/gen-2014-0108
27. Ray H, Bett KE, Tar'an B, Vandenberg A, Thavarajah D, Warkentin T. Mineral micronutrient content of cultivars of field pea, chickpea, common bean, and lentil grown in Saskatchewan, Canada. Crop Sci. (2014) 54:1698–708. doi: 10.2135/cropsci2013.08.0568
28. Vandemark GJ, Grusak MA, McGee RJ. Mineral concentrations of chickpea and lentil cultivars and breeding lines grown in the U.S. Pacific Northwest. Crop J. (2018) 6:253–62. doi: 10.1016/j.cj.2017.12.003
29. Elshire RJ, Glaubitz JC, Sun Q, Poland JA, Kawamoto K, Buckler ES, et al. A robust, simple genotyping-by-sequencing (GBS) approach for high diversity species. PLoS ONE. (2011) 6:e19379. doi: 10.1371/journal.pone.0019379
30. Poland JA, Brown PJ, Sorrells ME, Jannink JL. Development of high-density genetic maps for barley and wheat using a novel two-enzyme genotyping-by-sequencing approach. PLoS ONE. (2012) 7:e32253. doi: 10.1371/journal.pone.0032253
31. Huang YF, Poland JA, Wight CP, Jackson EW, Tinker NA. Using genotyping-by-sequencing (GBS) for genomic discovery in cultivated oat. PLoS ONE. (2014) 9:e102448. doi: 10.1371/journal.pone.0102448
32. Bajaj D, Das S, Badoni S, Kumar V, Singh M, Bansal KC. Genome-wide high-throughput SNP discovery and genotyping for understanding natural (functional) allelic diversity and domestication patterns in wild chickpea. Sci Rep. (2015) 5:12468. doi: 10.1038/srep12468
33. Jaganathan D, Thudi M, Kale S, Azam S, Roorkiwal M, Gaur P, et al. Genotyping-by-sequencing based intra-specific genetic map refines a “QTL-hotspot” region for drought tolerance in chickpea. Mol Gen Genomics. (2015) 290:559–71. doi: 10.1007/s00438-014-0932-3
34. Glaubitz JC, Casstevens TM, Lu F, Harriman J, Elshire RJ, Sun Q, et al. TASSEL-GBS: a high capacity genotyping by sequencing analysis pipeline. PLoS ONE. (2014) 9:e90346. doi: 10.1371/journal.pone.0090346
35. Bradbury PJ, Zhang Z, Kroon DE, Casstevens TE, Ramdoss Y, Buckler ES. TASSEL: software for association mapping of complex traits in diverse samples, J Bioinform. (2007) 23:2633–5. doi: 10.1093/bioinformatics/btm308
36. Varshney RK, Gaur PM, Chamarthi SK, Krishnamurthy L, Tripathi S, Kashiwagi J, et al. Fast-Track introgression of “QTL-hotspot” for root traits and other drought tolerance traits in JG 11, an elite and leading variety of chickpea. Plant Genome. (2013) 6:1–9. doi: 10.3835/plantgenome2013.07.0022
37. Langmead B, Salzberg S. Fast gapped-read alignment with Bowtie 2. Nat Methods. (2012) 9:357–9. doi: 10.1038/nmeth.1923
39. Van Ooijen W, Jansen J. JoinMap 4.1 Software for the Calculation of Genetic Linkage Maps in Experimental Populations. Cambridge: Kyazma ® (2013).
40. Kosambi DD. The estimation of map distances from recombination values. Ann Hum Genet. (1943) 12:172–5. doi: 10.1111/j.1469-1809.1943.tb02321.x
41. Lisa AO, Robert WR, Steven GB, Cory RB. Linkage map view-rendering high-resolution linkage and QTL maps. J Bioinform. (2018) 34:306–7. doi: 10.1093/bioinformatics/btx576
42. Wang S, Basten CJ, Zeng ZB. Windows QTL Cartographer 2.5. Department of Statistics, North Carolina State University, Raleigh, NC (2012).
43. Quinlan AR, Hall IM. BEDTools: a flexible suite of utilities for comparing genomic features. J Bioinform. (2010) 26:841–2. doi: 10.1093/bioinformatics/btq033
44. Varshney RK, Thudi M, Nayak SN, Gaur PM, Kashiwagi J, Krishnamurthy L, et al. Genetic dissection of drought tolerance in chickpea (Cicer arietinum L.). Theor Appl Genet. (2014) 127:445–62. doi: 10.1007/s00122-013-2230-6
45. Jayalakshmi V, Reddy AT, Nagamadhur KV. Genetic diversity and variability for protein and micro nutrients in advance breeding lines and chickpea varieties grown in Andhra Pradesh. Legum Res. (2018) 42:768–72. doi: 10.18805/LR-3933
46. Verma S, Gupta S, Bandhiwal N, Kumar T, Bharadwaj C, Bhatia S, et al. High-density linkage map construction and mapping of seed trait QTLs in chickpea (Cicer arietinum L.). using Genotyping-by-Sequencing (GBS). Sci Rep. (2015) 5:17512. doi: 10.1038/srep17512
47. Deokar A, Sagi M, Tar'an B. Genome-wide SNP discovery for development of high-density genetic map and QTL mapping of ascochyta blight resistance in chickpea (Cicer arietinum L.). Theor Appl Genet. (2019) 132:1861. doi: 10.1007/s00122-019-03322-3
48. Hiremath PJ, Kumar A, Penmetsa RV, Farmer A, Schlueter JA, Chamarthi SK, et al. Large-scale development of cost-effective SNP marker assays for diversity assessment and genetic mapping in chickpea and comparative mapping in legumes. Plant Biotechnol J. (2012) 10:716–32. doi: 10.1111/j.1467-7652.2012.00710.x
49. Deokar AA, Ramsay L, Sharpe AG, Diapari M, Sindhu A, Bett K, et al. Genome wide SNP identification in chickpea for use in development of a high density genetic map and improvement of chickpea reference genome assembly. BMC Genomics. (2014) 15:708. doi: 10.1186/1471-2164-15-708
Keywords: chickpea, seed, iron, zinc, SNP, QTL
Citation: Sab S, Lokesha R, Mannur DM, Somasekhar, Jadhav K, Mallikarjuna BP, C L, Yeri S, Valluri V, Bajaj P, Chitikineni A, Vemula A, Rathore A, Varshney RK, Shankergoud I and Thudi M (2020) Genome-Wide SNP Discovery and Mapping QTLs for Seed Iron and Zinc Concentrations in Chickpea (Cicer arietinum L.). Front. Nutr. 7:559120. doi: 10.3389/fnut.2020.559120
Received: 05 May 2020; Accepted: 21 August 2020;
Published: 15 October 2020.
Edited by:
Ajay Kumar, North Dakota State University, United StatesReviewed by:
Anupam Singh, Shriram Bioseed Genetics, IndiaDebasis Chattopadhyay, National Institute of Plant Genome Research (NIPGR), India
Copyright © 2020 Sab, Lokesha, Mannur, Somasekhar, Jadhav, Mallikarjuna, C, Yeri, Valluri, Bajaj, Chitikineni, Vemula, Rathore, Varshney, Shankergoud and Thudi. This is an open-access article distributed under the terms of the Creative Commons Attribution License (CC BY). The use, distribution or reproduction in other forums is permitted, provided the original author(s) and the copyright owner(s) are credited and that the original publication in this journal is cited, in accordance with accepted academic practice. No use, distribution or reproduction is permitted which does not comply with these terms.
*Correspondence: Mahendar Thudi, t.mahendar@cgiar.org; I. Shankergoud, ishankergoud@rediffmail.com