- 1Department of Behavioural Science and Health, University College London, London, United Kingdom
- 2Policy and Implementation Research, Cancer Research UK, London, United Kingdom
- 3Department of Clinical, Educational, and Health Psychology, University College London, London, United Kingdom
COVID-19 pandemic restrictions impacted dietary habits during the initial months of the pandemic, but long-term effects are unclear. In this longitudinal study, self-selected UK adults (n = 1,733, 71.1% female, 95.7% white ethnicity) completed three online surveys (May–June, August–September, and November–December 2020, with a retrospective pre-pandemic component in the baseline survey), self-reporting sociodemographics, lifestyle, and behaviours, including high fat, salt, and sugar (HFSS) snacks, HFSS meals, and fruit and vegetable (FV) intake. Data were analysed using generalised estimating equations. Monthly HFSS snacks portion intake increased from pre-pandemic levels (48.3) in May–June (57.6, p < 0.001), decreased in August–September (43.7, p < 0.001), before increasing back to pre-pandemic levels in November–December (49.2, p < 0.001). A total of 48.5% self-reported increased [25.9 (95% confidence interval: 24.1, 27.8)] and 47.7% self-reported decreased [24.1 (22.4, 26.0)] monthly HFSS snacks portion intakes in November–December compared with pre-pandemic levels. Monthly HFSS meals portion intake decreased from pre-pandemic levels (7.1) in May–June (5.9, p < 0.001), was maintained in August–September (5.9, p = 0.897), and then increased again in November–December (6.6, p < 0.001) to intakes that remained lower than pre-pandemic levels (p = 0.007). A total of 35.2% self-reported increased [4.8 (4.3, 5.3)] and 44.5% self-reported decreased [5.1 (4.6, 5.6)] monthly HFSS meals portion intakes in November–December compared with pre-pandemic levels. The proportion meeting FV intake recommendations was stable from pre-pandemic through to August–September (70%), but decreased in November–December 2020 (67%, p = 0.034). Increased monthly HFSS snacks intake was associated with female gender, lower quality of life, and – in a time - varying manner – older age and higher HFSS meals intake. Increased monthly HFSS meals intake was associated with female gender, living with adults only, and higher HFSS snacks intake. Reduced FV intake was associated with higher body mass index (BMI) and lower physical activity. These results suggest large interindividual variability in dietary change during the first year of the pandemic, with important public health implications in individuals experiencing persistent increases in unhealthy diet choices, associated with BMI, gender, quality of life, living conditions, physical activity, and other dietary behaviours.
Introduction
2020 has seen widespread disruption to the lives of individuals across the globe due to the COVID-19 pandemic. In the UK, pandemic restrictions were imposed from late March 2020 onwards. Since then, varying levels of restrictions have impacted how individuals and societies live their lives (1). Restrictions were slowly eased from June until September, before progressively becoming stricter, with full lockdown conditions in December 2020. Diet is a major factor influencing bodyweight, blood pressure, metabolic health, and the risk of non-communicable disease. As such, diet is one of the largest contributors to the burden of disease [as measured using disability-adjusted life years (DALYs)] globally and in the UK (2, 3). The widespread disruption to people's lifestyles from the COVID-19 pandemic may result in significant shifts in health behaviours, including diet.
Factors that can impact energy intake and dietary behaviour including food accessibility, changes in work life, home life, stress, and other health behaviours including sleep, physical activity, smoking, and alcohol consumption have been affected by COVID-19 restrictions (4–7). Closures of restaurants and fast food outlets, increased usage of food delivery services, changes in the affordability and availability of foods alongside disruptions to the home and working environment, as well as changes in employment status may all impact diet behaviour during the pandemic (8–13). Indeed, initial reports find that a significant proportion of adults altered their food choices and dietary habits at the start of the pandemic compared to pre-pandemic food choices and habits (9, 14–17). Despite no overall change in diet quality, there has been large interindividual variability (1, 5, 14, 17–19). A scoping review of 23 studies (17 cross-sectional) from the initial months of the pandemic demonstrate that individuals are making favourable and unfavourable changes to their diet, including changes in snacking, high fat, salt, or sugar (HFSS) food intake, and fruit and vegetable (FV) intake (14). Significant proportions of people have increased their overall food intake and are snacking more (5, 7, 14, 19, 20), but equally people have also decreased their overall food intake and are snacking less (1, 9, 17, 19).
These changes in diet behaviours during the pandemic are associated with several factors including age, gender, body mass index (BMI), physical activity, and experiencing a larger psychological impact and larger shifts in lifestyle as a result of lockdown restrictions (4, 14, 21–27). Given the relationship between a sub-optimal diet and relative risk of cardiovascular disease, cancer, and all-cause mortality, there could be serious long-term public health consequences if the initial unfavourable changes in dietary behaviours during the pandemic are maintained (28, 29). Understanding the dietary changes that have occurred, and the key predictors associated with these changes is important to identify at-risk groups of unhealthful dietary change, to inform future interventions, develop targeted approaches, and guide efficient resource allocation. Given the impact of culture on diet and the specific impacts of lockdown restrictions across the globe, it is important to assess the longitudinal impact, including in the UK. However, studies to date assessing the influence of COVID-19 lockdown restrictions on dietary behaviours in UK adults have largely been cross-sectional and undertaken during the initial months of lockdown (7, 14, 19). Some cross-sectional analyses show dietary changes during August–October 2020 compared to the pre-pandemic period (1, 30, 31), and changes in consumer habits during November–December 2020 (10). Herle et al. identified multiple eating trajectories of UK adults during the initial months of the pandemic, including individuals with an initial increase in eating at the start of the pandemic, followed by a gradual return to pre-pandemic food intake by May 2020 (21). One longitudinal UK study analysing food purchases found increased calorie intake across 2020 (16), but longitudinal analyses are scarce (16, 21, 32). Dietary changes compared to pre-pandemic levels and key predictors of any change during the first year of the pandemic to December 2020 in UK adults are largely unknown.
The HEalth BEhaviours during the COVID-19 pandemic (HEBECO) study is a longitudinal UK cohort assessing the impact of the COVID-19 pandemic on health behaviours, and their influences. The objective of this study was to address the following research questions (RQs):
RQ1. What was the average (i) HFSS snacks, (ii) HFSS meals, and (iii) FV intake in UK adults before, at the beginning of, and at 3- and 6-month follow-ups during the COVID-19 pandemic?
RQ2. To what extent are sociodemographic, COVID-19-related, and behavioural factors associated with a change in (i) HFSS snacks, (ii) HFSS meals, or (iii) a reduction in FV intake across 6 months of follow-up during the COVID-19 pandemic compared with pre-pandemic intakes in UK adults?
Materials and Methods
Study Design
The study design has been previously reported (33). Briefly, this study is a longitudinal analysis of data from an online study of adults, the HEBECO study.1 The study was approved by the Ethics Committee at the UCL Division of Psychology and Language Sciences (CEHP/2020/759). Participants were self-selected and gave consent prior to data collection. The full recruitment strategy is available online (see footnote 1). Participants were recruited through multiple online channels including paid and unpaid advertisements across social media (Facebook, Google, and Reddit) and mailing lists of UK universities, charities, local government, and networks within Cancer Research UK and Public Health England. Data were captured and managed by the REDCap electronic data system at UCL (34, 35). The surveys used in this analysis cover a period of 8 months since the beginning of the pandemic (May–December 2020), as well as a retrospective survey at baseline of the pre-pandemic period. Baseline data were collected between 5 May and 14 June 2020 (inclusive). The 3-months follow-up survey corresponds to the periods of eased pandemic restrictions in the UK during August–September 2020, and the 6-months follow-up survey corresponds to the tighter restrictions in the UK during November–December 2020. The re-introduction of tighter restrictions at the end of 2020 varied across the UK. Tiered restrictions were introduced after a UK-wide lockdown during November and December, with some regions remaining under strict lockdown, and others with more relaxed restrictions. The study protocol and statistical analysis plan were pre-registered on the Open Science Framework prior to analysis.2 Deviations from the pre-registered protocol are described in the Supplementary Materials. The main study protocol3 provides further detail on the survey.
Study Sample
The analysis uses data from UK adults (18+) who completed baseline data collection and provided data of interest at the 6-months follow-up survey as a minimum, for the outcome variables defined below.
Measures
Full details of outcome and predictor measures can be found in the Supplementary Materials, and have been previously defined (33).
Outcomes
Participants were asked at baseline, “Before COVID-19, how often did you usually eat or drink...” for nine food items: eight HFSS food items [(i) ready meals, (ii) fast food, (iii) takeaways, (iv) sugary or sweetened drinks, (v) sweets or chocolate, (vi) cakes and biscuits, (vii) desserts, and (viii) savoury snacks], and one item for fruit and vegetable intake. For each food item, respondents could answer on a 7-point scale: “A few times per day,” “Once a day,” “A few times per week,” “Once a week,” “A few times per month,” “Once a month,” “Less often/never,” and “Not sure.” Participants were also asked at baseline “Since COVID-19, how often did you usually eat or drink...,” and then at 3- and 6-month follow-ups, “In the past month, how often did you usually eat or drink...” for the same nine food items. The HEBECO study food item questions are based on previous research study survey questions, and are derived from Public Health England's sugar reduction programme definitions as policy-relevant measures (36–38).
To estimate monthly portion intake frequency, responses for all food item questions were converted into monthly portion frequencies, based on previous research (33). Assuming a minimum of 4 weeks per calendar month, an answer of “A few times per day” was scaled up to 56 portions per month (i.e., 2 daily portions × 7 days × 4 weeks), “Once a day” was scaled up to 28 portions per month, “Few times per week” as 12 portions per month, “Once a week” as 4 portions per month, “Few times per month” as 2 portions per month, “Once a month” as 1 portion per month, and “Less often/never” as 0.5 portions per month.
Using the above monthly portion intake frequencies, (iv) sugary or sweetened drinks, (v) sweets or chocolate, (vi) cakes and biscuits, (vii) desserts, and (viii) savoury snacks monthly frequencies were summed to produce a “HFSS snacks intake” monthly portion frequency. (i) Ready meals, (ii) fast food, and (iii) takeaways monthly frequencies were summed to produce a “HFSS meals intake” monthly portion frequency. The change scores “Change in self-reported HFSS snacks intake” and “Change in self-reported HFSS meals intake” used as outcomes in RQ2 were computed from pre-pandemic HFSS snacks and HFSS meals intakes retrospectively reported at baseline, which were deducted from HFSS snacks and HFSS meals intakes reported at the time of the baseline survey, and at 3- and 6-month follow-up surveys.
FV intake was converted into a binary outcome variable, grouped into “Consuming a few portions per day of fruit and vegetables” (responses of “A few times per day”) vs. “Less than a few portions per day” (all other responses besides “A few times per day”). This cut-off was used to reflect health recommendations for several daily portions of fruit and vegetables (39). The binary change score used as the outcome in RQ2 was computed as a categorical reduction (“Reduced intake” vs. “All other”) in FV intake at the time of the baseline survey, and at 3- and 6-month follow-up surveys, compared to pre-pandemic levels retrospectively reported at baseline.
HFSS snacks intake was the primary outcome of interest, given that a systematic review identified large changes in snacking during the initial months of the pandemic (19), which in turn have been associated with self-reported weight change during the pandemic (5, 40, 41).
Explanatory Variables
Time-Invariant
Explanatory variables recorded at baseline included gender (female vs. all other), age (continuous), ethnicity (white vs. all other), occupation and work from home (categorical: unemployed (which includes retired persons and full-time parents/carers), employed and working from home, employed and not working from home), living arrangements (living alone, living with children (with or without adults), living with adults only), and a socioeconomic score. The socioeconomic score (categorical score from 0–3) was based on household income, housing status, and level of education; participants scored 0 if they had an income <£50,000, lived in unowned housing and had no higher education, or scored 1, 2, or 3 if participants met 1, 2, or all 3 criteria of having an income of ≥£50,000, owning their housing/having a mortgage, or having higher education. The current cohort had a higher income on average than the average UK income. As such, £50,000 was used as the cut-off point.
Sensitivity analyses also included an unhealthy eating through boredom, stress, or comfort variable. This measure was not included in the main RQ2 analysis as not all participants were shown the question to reduce participant burden. At baseline, participants were asked to what extent they agreed with the following statements: “I eat unhealthy food out of boredom,” “I eat unhealthy food because I'm stressed,” “I eat unhealthy food because it's comforting” on a 0–100 scale, where 0 = completely disagree; 50 = neutral; and 100 = completely agree. A continuous mean score (0–100) was computed for eating for comfort, stress, and from boredom. A higher score indicated eating unhealthy food out of boredom, stress, or comfort.
Time-Variant
Explanatory variables reported at baseline, 3-, and 6-month follow-up surveys included BMI (continuous: self-reported weight in kilograms divided by self-reported height in metres squared), isolation status (total/some isolation vs. general/no isolation), quality of life, an average continuous rating from 1-5 of quality of living, wellbeing, social and family relationships (1 = poor, 5 = excellent), and health behaviours as detailed below.
Physical activity was a continuous measure of metabolic equivalent (MET)-hours per week. At each time point, participants self-reported the number of days they performed strengthening physical activity (SPA) per week, and the number of days and average duration of a session of moderate or vigorous physical activity (MVPA) per week. The number of days performing SPA per week was multiplied by an average session duration of 45 minutes and multiplied by 4 to convert to MET-minutes per week, to reflect the nature of SPA as moderate- to high-intensity bouts, interspersed with rest periods (42). The 45-min length reflects the American College of Sports Medicine recommendations for the typical number of exercises, reps, sets, and duration of rest periods for a resistance training session (43, 44). MVPA number of days per week was multiplied by the self-reported average session length, and then multiplied by 6 to convert to MET-minutes per week, as an average of moderate and vigorous physical activity (45). Scores were then summed for SPA and MVPA and divided by 60 to produce a MET-h per week score. An upper limit of 4 standard deviations above the mean MET-h per week was applied, as some individuals self-reported activity levels not physically possible. This upper limit corresponded to ~8 h of moderate physical activity per day, which is several standard deviations above the physical activity levels reported from large observational studies (46).
Alcohol consumption was based on government low-risk drinking recommendations (≤14 weekly alcohol units vs. >14 weekly alcohol units) (47), and smoking status was based on the self-reported use of tobacco or cigarettes (yes vs. no).
HFSS snacks intake, HFSS meals intake, and FV intake were also used as continuous explanatory variables (but excluded in analyses of the same kind, e.g., HFSS snacks intake was excluded from “Change in self-reported HFSS snacks intake” analyses).
Statistical Analysis
Statistical analysis was conducted in SPSS Statistics version 27 (IBM). Significance was defined as p < 0.05.
RQ1
We described baseline participant characteristics in RQ1 [weighted participant characteristics, based on census data from the Office for National Statistics for age, gender, country of living, ethnicity, education, and income are presented in the Supplementary Materials (48)].
We reported the unweighted means with 95% confidence intervals (95% CI) for HFSS snacks and HFSS meals monthly portion intake and the proportion consuming a few portions of FV per day at each timepoint. We also reported the percentage of the sample increasing or decreasing HFSS snacks or HFSS meals intake, or categorically increasing or decreasing FV intake from the reference timepoint (pre-pandemic levels reported at baseline, levels during May–June 2020 reported at baseline, or at 3-months follow-up). Lastly, we reported the mean change in portion frequency (with 95% CI) in those increasing or decreasing HFSS snacks or HFSS meals intake between timepoints.
Given that more distantly spaced participant measures over time are expected to be less closely correlated (49), an unadjusted, unweighted generalised estimating equation (GEE) using the AR(1) covariance structure was used to assess changes in self-reported HFSS snacks and HFSS meals monthly portion intake over time, using pairwise time comparisons between timepoints, adjusted for with sequential Šidák correction.
RQ2
GEE models were used to determine the association between the explanatory variables and (i) changes in HFSS snacks intake and (ii) changes in HFSS meals intake across the follow-up period. The GEE models for a change in HFSS snacks and HFSS meals monthly portion intake used the identity link function for a linear scale response, as the change scores were normally distributed continuous outcome variables. The GEE models for FV used a binary logistic model and logit link function for the binary outcome variable (reduced FV intake vs. all other).
Univariate GEE models were computed to determine the association between each explanatory variable and changes in HFSS snacks intake, changes in HFSS meals intake, and a reduction in FV intake. Each explanatory variable model was adjusted for a main effect of time and for an “explanatory variable*time” interaction. Fully adjusted GEE models containing all explanatory variables were then computed.
All significant explanatory variable*time interactions were then added to the fully adjusted GEE model containing all explanatory variables, to assess temporal differences in the association of explanatory variables with continuous changes in HFSS snacks and HFSS meals monthly portion intake, or a reduction in FV intake over time. The time variable was categorical, as the trajectory of change in dietary intakes was not expected to be linear (50). Explanatory variable*time interactions were retained in the full GEE model if they improved goodness of fit [Quasi-likelihood under Independence Model Criterion (QIC) QIC > 2] over the full GEE model without interactions, and the interaction itself remained significant (p < 0.05).
Independent variables were retained after checking for collinearity using Pearson correlations, with all correlations r < 0.4.
For binary outcomes using the logit link function, linearity of logit assumptions were checked, as detailed in the Supplementary Materials.
Sensitivity Analyses
The supplementary analyses used complete cases only (those participants completing all dietary measures at all three surveys: baseline, 3-, and 6-month follow-ups). Further analyses were then conducted for the primary outcome of HFSS snacks intake using two binary logistic GEE models with logit link function for an “increase” in HFSS snacks intake vs. “all other,” and a “decrease” in HFSS snacks intake vs. “all other.” A change was defined as an increase or decrease in intake by 10% or more from pre-pandemic intakes retrospectively self-reported at baseline, based on a cut-off used in a previous large study of dietary intakes during the pandemic (4).
Analyses were repeated for the sub-sample of participants self-reporting eating behaviour measures. Univariate GEE models were computed for changes in HFSS snacks intake, changes in HFSS meals intake, and a reduction in FV intake outcomes using the unhealthy eating through boredom, stress, or comfort variable. Unhealthy eating through boredom, stress, or comfort was also added to the fully adjusted GEE models (with and without significant time interactions from the univariate models).
Multiple observations in the literature have indicated that gender, smoking, and physical activity are likely related to changes in snacking behaviour during the pandemic (4, 7). Bayes Factor analyses were pre-registered online in the event of non-significant findings for gender, smoking, and physical activity with change in HFSS snacks intake in the main analysis.4 Snacking Bayes factors prior mean differences were obtained from differences in change in daily energy intake from before COVID-19 to since COVID-19 between predictors and converted into snacking portions per month (further details can be found in the Supplementary Materials). Alternative hypotheses were modelled using a half-normal distribution with a peak at zero, given that smaller effect sizes nearer to null are more likely than larger effect sizes (51, 52). The standard deviation (SD) was set to 5.32 for gender, 7.35 for physical activity, and 11.17 for smoking for a change in HFSS snack portions per month (4). Bayes factors were calculated using an online calculator: http://bayesfactor.info/.
Results
Out of a total of 2,992 UK adult participants recruited into the HEBECO baseline survey, 1,733 (weighted = 1,532) participants met the inclusion criteria for analyses. The unweighted baseline characteristics are shown in Table 1 (for weighted characteristics, see Supplementary Table 1). Included participants were more likely to be female, older, of white ethnicity, have a higher BMI, be unemployed (which includes retired persons and full-time parents/carers), have a higher socioeconomic score, live with adults only, be in total or some isolation, have a higher quality of life score, consume fewer HFSS meals, consume more FV, and be less likely to smoke.
What Were the Intakes of HFSS Snacks, Meals, and FV in UK Adults Before, at the Beginning of, and at 3- and 6-Month Follow-Ups During the COVID-19 Pandemic?
HFSS snacks monthly portion intake increased from before the pandemic to the start of the pandemic (by May–June 2020) (48.3 to 57.6 per month, p < 0.001; Figure 1). At 3-months follow-up (by August–September 2020), HFSS snacks monthly portion intake significantly decreased (to 43.7 per month, p < 0.001). At 6-months follow-up (by November–December 2020), HFSS snacks monthly portion intake significantly increased (to 49.2 per month, p < 0.001) to intakes that were not significantly different to retrospectively reported intakes before the pandemic (p = 0.297).
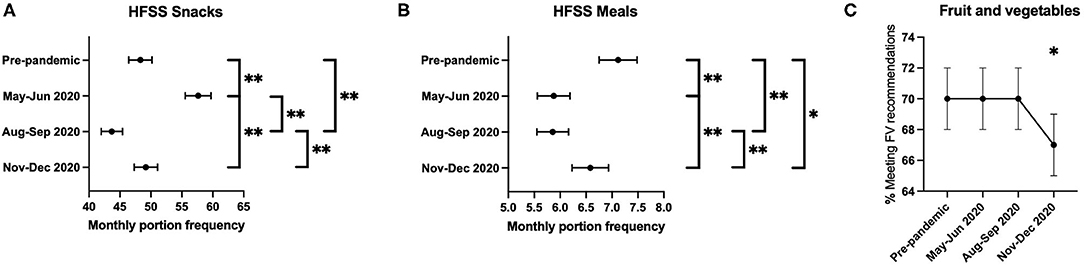
Figure 1. (A–C) Means, 95% confidence intervals, and pairwise comparisons between pre-pandemic, baseline (May–June 2020), 3-month (August–September 2020), and 6-month (November–December 2020) follow-up surveys. *Denotes pairwise comparisons between categories were significant at the 0.05 level. **Denotes pairwise comparisons comparisons between categories were significant at the 0.005 level.
HFSS meals monthly portion intake decreased from before the pandemic to the start of the pandemic (by May–June 2020) (7.1 to 5.9 per month, p < 0.001; Figure 1). At 3-months follow-up (by August–September 2020), HFSS meals monthly portion intake was maintained (5.9 per month, p = 0.897). At 6-months follow-up (by November–December 2020), HFSS meals monthly portion intake significantly increased (5.9–6.6 per month, p < 0.001) to intakes that were significantly lower than retrospectively reported intakes before the pandemic (7.1 to 6.6 per month, p = 0.007).
For FV intake, 70% [95% CI: 68, 72] were consuming a few portions per day before the pandemic, at the start of the pandemic [95% CI: 68, 72] (May–June 2020), and at 3-months follow-up [95% CI: 68, 72] (August–September 2020), but this significantly decreased to 67% [95% CI: 65, 69] (p = 0.034) at 6-months follow-up (November–December 2020) (Figure 1).
From the pre-pandemic period to 6-months follow-up (November–December 2020), 48.5% of individuals self-reported an increase in HFSS snacks intake, by an average of 25.9 [95% CI: 24.1, 27.8] portions per month. A similar proportion (47.7%) reported a decrease in HFSS snacks intake, by an average of 24.1 [95% CI: 22.4, 25.9] portions per month (Table 2). From pre-pandemic to 6-months follow-up, 35.2% of individuals self-reported an increase in HFSS meals intake, by an average of 4.8 [95% CI: 4.3, 5.3] portions per month. A total of 44.5% self-reported a decrease in HFSS meals intake, by an average of 5.1 [95% CI: 4.6, 5.6] portions per month. For FV intake, 11.4% were no longer meeting daily FV intake recommendations, and 8.4% were now meeting daily FV intake recommendations at 6-months follow-up compared with pre-pandemic levels.
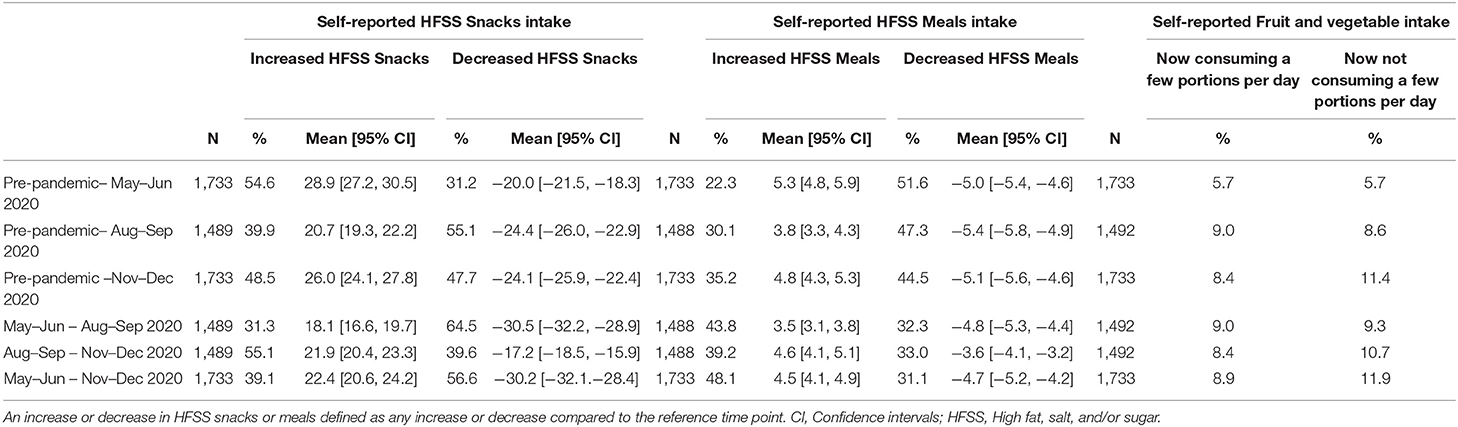
Table 2. Unweighted proportions increasing or decreasing HFSS snacks, HFSS meals, and fruit and vegetables intake, and mean changes with 95% confidence intervals in HFSS snacks and HFSS meals intake.
The changes in portion frequency consumption across the pandemic for each food item is shown in Supplementary Figure 1.
Which Explanatory Variables Were Associated With Changes in Monthly HFSS Snacks and Meals Intakes, or a Reduction in FV Intake in UK Adults Across 6 Months of Follow-Up During the COVID-19 Pandemic?
In the unadjusted GEE models (Supplementary Table 2), female gender, higher baseline BMI, total or some isolation, a lower quality of life score, a higher HFSS meals intake, and lower physical activity levels were significantly associated with an increase in self-reported HFSS snacks intake across 6 months of follow-up during the pandemic. In the fully adjusted GEE model (Table 3), female gender [B = 6.568 (95% CI: 3.653, 9.483)] and a lower quality of life score [B = −2.882 (95% CI: −4.387, −1.377)] were associated with an increase in monthly HFSS snacks intake across the pandemic. HFSS meals intake was also significantly associated with a change in monthly HFSS snacks intake across the pandemic, but this was time-varying. Similarly, age showed significant time interactions and improved model fit.
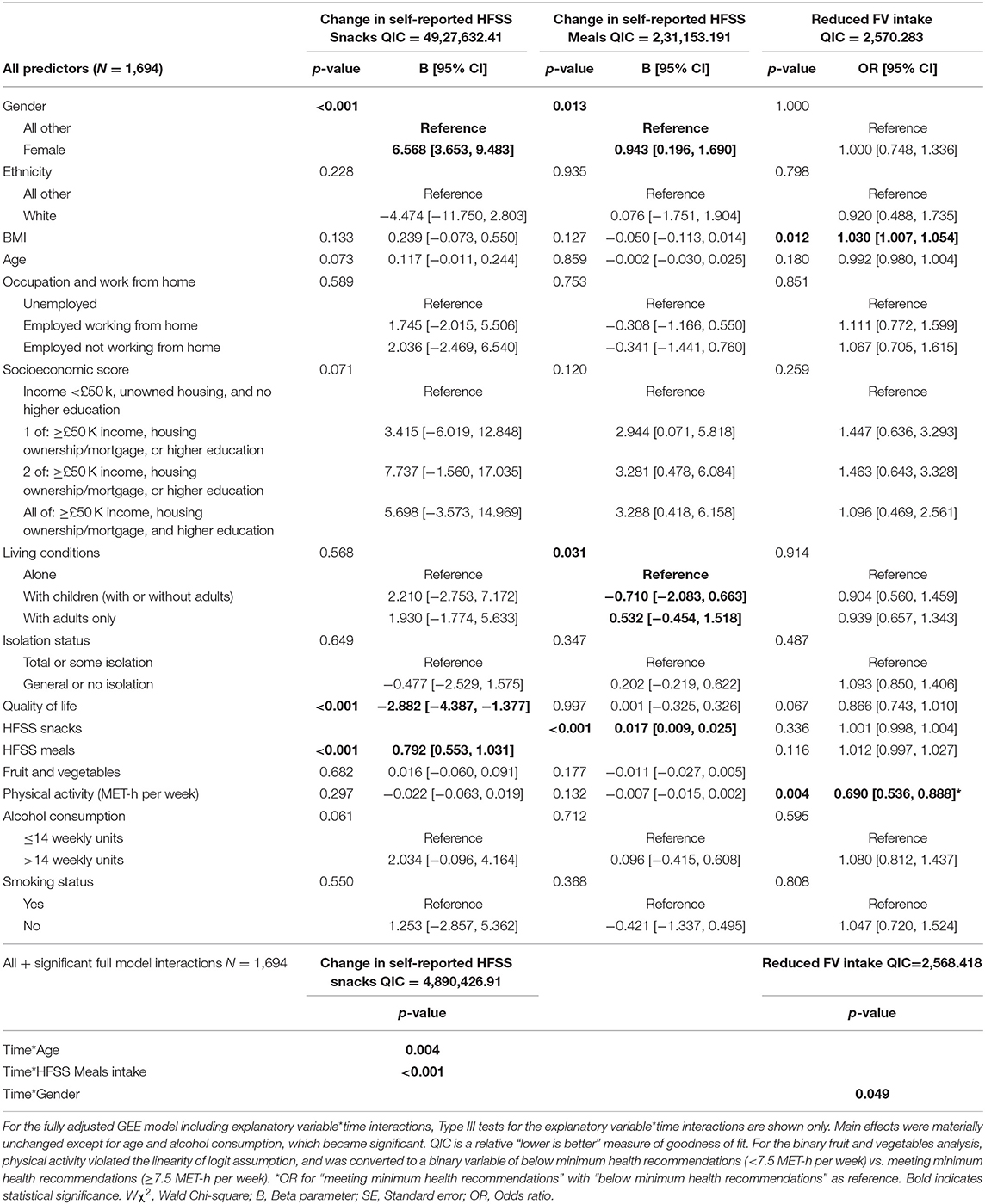
Table 3. Fully adjusted GEE model containing all predictor variables and the fully adjusted GEE model including significant explanatory variable*time interactions.
Figure 2 exhibits the time-varying associations of age and HFSS meals intake with a change in HFSS snacks intake across the pandemic. All ages increased HFSS snacks intake at the start of the pandemic, but younger ages tended to decrease HFSS snacks intake during the latter months of 2020 (November–December 2020), whereas older ages tended to maintain or increase intakes from pre-pandemic levels. Higher HFSS meals intakes were associated with larger increases in HFSS snacks intake at the start of the pandemic (May–June), with higher and lower HFSS meals intakes decreasing HFSS snacks intake at 3-months follow-up (August–September). Individuals with a higher HFSS meals intake then returned to, or increased HFSS snacks intake above pre-pandemic levels at 6-months follow-up, whereas those with lower HFSS meals intakes maintained the reduced HFSS snacks intake.
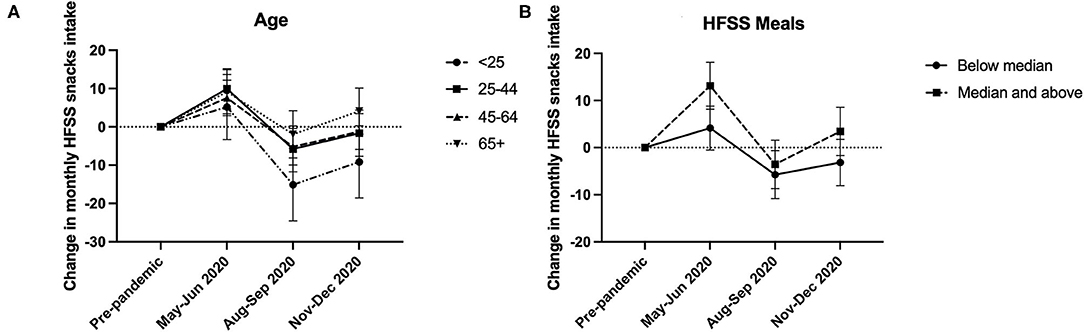
Figure 2. Graphical illustrations of the time-varying associations of (A) age and (B) HFSS meals intake with change in monthly HFSS snacks intake at baseline (May–June 2020), 3-month (August–September 2020), and 6-month follow-ups (November–December 2020) compared with pre-pandemic intakes.
In the unadjusted GEE models of HFSS meals intake (Supplementary Table 2), female gender and a higher HFSS snacks intake were associated with an increase in HFSS meals across the pandemic. In the fully adjusted GEE model (Table 3), female gender [B = 0.943 (95% CI: 0.196, 1.690)], living with adults only [B = 0.532 (95% CI: −0.454, 1.518)], and higher intakes of HFSS snacks [B = 0.017 (95% CI: 0.009, 0.025)] were associated with an increase in HFSS meals intake across the pandemic. There were no significant explanatory variable*time interactions.
In the unadjusted GEE models of FV intake (Supplementary Table 2), higher BMI, lower socioeconomic score, general or no isolation (compared with total or some isolation), lower quality of life, higher HFSS meals intake, and lower physical activity levels were associated with a reduction in FV intake. In the fully adjusted GEE model (Table 3), reduced FV intake across the pandemic was associated with a higher BMI [odds ratio (OR) = 1.030 (95% CI: 1.007, 1.054)] and lower physical activity levels [OR = 0.690 (95% CI: 0.536, 0.888)]. A gender interaction with time while significant, did not improve model fit and was not retained.
Sensitivity Analyses
Complete case analyses of RQ1 and RQ2 demonstrated materially unchanged differences in HFSS snacks and HFSS meals intakes across timepoints and in adjusted analyses compared with the main analysis (Supplementary Tables 3–5). For a change in FV intake, however, the gender interaction with time improved model fit and remained significant when added to the fully adjusted model, as shown in Supplementary Figure 2.
Unadjusted analyses of binary outcomes for HFSS snacks used in the sensitivity analysis are presented in Supplementary Table 6. In the full binary logistic GEE models for an increase or decrease in HFSS snacks intakes vs. all other, female gender, a higher BMI, a lower quality of life score, and a higher HFSS meals intake were more likely to increase HFSS snacks intake across the pandemic (Supplementary Table 7). Non-female gender, younger age, higher quality of life, lower HFSS meals intake, and low-risk alcohol consumption were associated with decreased HFSS snacks intake across the pandemic. Age and alcohol consumption interactions had a time-varying impact on increased HFSS snacks intake vs. all other (Supplementary Figure 3). There were no significant explanatory variable*time interactions for a decrease in HFSS snacks vs. all other (Supplementary Table 6).
Unadjusted analyses of unhealthy eating through boredom, stress, or comfort are also presented in Supplementary Table 8. In fully adjusted GEE models including unhealthy eating through boredom, stress, or comfort, a higher score was significantly associated with an increase in HFSS snacks intake (Supplementary Table 9). Unhealthy eating through boredom, stress, or comfort had a time-varying impact (Supplementary Figure 4).
Because of the null findings reported here for smoking status on a change in HFSS snacks intake, Bayes factors were calculated. The Bayes factor suggests the data provided evidence for no effect of smoking status (BF = 0.32) on change in HFSS snacks intake.
Discussion
In this study of a sample of UK adults, initial average trends in HFSS snacking and meal consumption are not maintained across the pandemic. There is substantial interindividual variability, with some individuals experiencing long-term, unhealthy dietary behaviour changes. Combined, our results suggest that gender, BMI, living conditions, quality of life, other dietary behaviours, and physical activity are associated with adverse self-reported dietary behaviour changes.
In Context of COVID-19 Research
Reviews from the initial months of the pandemic indicate an overall trend for increased food consumption (7, 53), with increased FV consumption in some individuals, but also increased HFSS foods consumption in others (6, 54). In particular, increased snacking has been identified during the start of the pandemic (19, 54–56), with a greater proportion of individuals increasing than decreasing HFSS snacks intake (19, 54), as with our results.
Dietary changes can have both protective and harmful impacts on health and well-being. Understanding the contexts associated with dietary changes is central to developing targeted interventions. Female gender, higher BMI, lower quality of life, reduced physical activity, and experiencing a greater negative impact from the pandemic have been previously recognised as predictors of unhealthy dietary changes during the pandemic (55, 57, 58). Our study builds upon the current literature, showing these factors are important long-term predictors of adverse dietary change during the pandemic.
Pandemic-related dietary changes may have occurred from shop closures, or changes in food availability, accessibility, or shopping habits (31, 55). Increased HFSS snacking at the start of the pandemic may have resulted from convenience, or greater inconvenience of accessing fresh foods (55, 59). Given their high availability, affordability, and long shelf-life, individuals may have prioritised HFSS snacks over fresh foods from the uncertainty over food supply during the pandemic (55, 59–61).
In our study, a lower quality of life or unhealthy eating through stress, boredom, or comfort was associated with unhealthy changes in HFSS foods intake during the first year of the pandemic. Individuals tend to consume more palatable and less healthy foods during stressful life periods (62). A greater decline in mental health or increased stress, boredom, or anxiety from COVID-19 has been associated with increased ultra-processed, HFSS foods intake, decreased FV intake, and using snacking as a coping mechanism (9, 14, 32, 55, 56, 63–65). The increased HFSS snacking at the start of the pandemic may reflect such maladaptive coping mechanisms (63, 66). For some individuals, the increase may have been maintained through strengthening of a cue-trigger-reward feedback cycle and habit formation (67).
At the start of the UK lockdown in 2020, being female (vs. not) was associated with greater dietary changes, namely to consistently eat less, and to eat more (21). There may be psycho-social, cultural, and environmental reasons for this possible difference. For example, it is more likely for females to use any form of coping strategy than males (68). UK females also experienced greater disruption to sleep at the start of the pandemic (69), which can alter dietary behaviour (70). The association between female gender and self-reported adverse dietary behaviour changes in this study may therefore reflect maladaptive coping strategies of female participants, with a reduction in healthy foods consumption, and increases in unhealthy foods consumption. The initially high levels of COVID-19-related stress experienced by women at the start of the pandemic declined, which may suggest women then formed unhealthy dietary habits (71, 72).
We found that living with adults was associated with increased HFSS meals intake. Higher numbers of adults were shown to consume more meals per day during the second COVID-19 wave in the UK (October 2020) compared with pre-pandemic, with increases in both ready meals and homemade meals (30). More shared mealtimes during lockdown may have altered eating behaviours (73), with existing or changing HFSS meal habits of some adults in the household potentially influencing the dietary habits of others (73).
Policy Implications
The World Health Organisation recommends limiting HFSS foods to reduce the risk of weight gain, cardiovascular disease, and high blood pressure (55, 74). In the UK, average free sugar and saturated fat consumption exceeds recommendations, and average FV intakes are below recommendations (31, 75). This study suggests the pandemic is associated with long-term adverse changes in dietary behaviours, which could amplify the existing sub-optimal dietary patterns of UK adults. A poor diet is the largest behavioural risk factor for DALYs lost (3) and second only to smoking for years of life lost (76), indicating a strong need for policy action to help individuals make healthy dietary choices. The pandemic has impacted people differently, therefore strategies need to consider and prioritise those who might be vulnerable to sustained unhealthy dietary changes (e.g., women, older individuals, or the physically inactive), and those who face greater barriers to healthy change.
The new UK government obesity campaign needs to not only ensure that HFSS foods are less accessible (e.g., placing lower limits on cost or limiting advertising), but also ensure that healthier options such as fruit and vegetables are more accessible (i.e., cheaper or more readily available) (77, 78). COVID-19 restrictions have altered the work-life balance for many individuals. Strategies need to consider the social impact from changes in household dynamics in the COVID-19 era on dietary behaviours and how to incorporate healthy eating into social norms and identities (79). Given the impact of COVID-19 on face-to-face communication and increased telecommunication, remotely accessible resources should be made available for successful behavioural change and habit formation, promoting autonomy and satisfaction from healthful dietary changes (67).
Strengths and Limitations
There are several strengths of this study. This is one of the first studies in UK adults examining changes in dietary behaviours and predictors of dietary change across the first year of the COVID-19 pandemic, from May to December 2020 compared with pre-pandemic. The longitudinal nature builds upon the largely cross-sectional current literature, providing a greater understanding of the long-term impacts of the pandemic on dietary behaviour. The analysis included a range of variables that reflect the wide-ranging impact of the pandemic, with time-varying measures to reflect the changing conditions of the pandemic over time. A range of health behaviours were also considered that are important for dietary behaviour. The use of GEE models for the longitudinal analysis provided several advantages over common analytical methods, including the ability to handle repeated measures, model different data distributions, and use time-varying predictors. Complete case analyses and sensitivity analyses with binary cut-offs demonstrating largely similar associations indicate the robustness of the associations identified in this study.
However, there are several limitations which may have introduced bias. First, the study sample was self-selected and largely female, older, and well-educated. Second, there were differences in various characteristics between included and excluded participants. Included participants were more likely to be female, of white ethnicity, and have a higher socioeconomic score, which may limit the generalisability of results. It cannot be ruled out that the associations between female gender and adverse self-reported dietary changes are an artefact of sample selection. Third, causality cannot be concluded from the observational study design. Fourth, measures of interest were self-reported; however epidemiological studies during COVID-19 have been largely self-reported, and dietary assessments in general are routinely self-reported (80). The survey did not include a complete dietary analysis of all food groups or energy intake. Individuals generally tend to underestimate energy intake, and more so in individuals living with being overweight or obesity (80, 81). However, this study focused on key food groups and their frequency of consumption, including HFSS foods and fruit and vegetables. Furthermore, self-reported dietary data still hold important value to inform health policy (82). Assessing fruit and vegetable intake using separate questions may have provided further insights into self-reported dietary changes. Using dietary change scores as the outcome variables enabled participants to act as their own controls which helped to minimise within-subject measurement error. Participants were also not explicitly asked if their diet had changed, nor told that dietary change was an outcome of interest, reducing the risk of expectation bias. The use of several diet recalls at each survey would help to strengthen the study findings. Fifth, participants were asked about their behaviours in the past week or month, which may have introduced a recall bias. Sixth, the survey focused on HFSS snacking specifically, rather than any snacking. However, most studies to date have considered HFSS snacks (19). Seventh, the unhealthy eating through boredom, stress, or comfort variable was based on a visual analogue scale using relevant questions to assess influences on eating. However, use of a validated eating behaviour measure may have aided generalisability of results. Eighth, our results are presented for the UK as a whole. There were varying restrictions across the UK during the November–December follow-up study, which may have differently impacted individuals. However, isolation status was not significantly associated with dietary changes. The window for completing the 6-months follow-up survey combined with the variation in imposing stricter restrictions during December within the same region means that participants may have completed the survey under different levels of restrictions.
Conclusion
While HFSS snacks intake fluctuated across the first year of the pandemic, it returned to pre-pandemic levels by the end of 2020. In contrast, HFSS meals also fluctuated but remained below pre-pandemic levels by November–December 2020. FV intake, while initially stable, decreased by the end of the year compared with pre-pandemic levels. These changes at population level do however, mask large interindividual changes in dietary behaviours, driven by differences in anthropometric (BMI), sociodemographic (gender), lifestyle (quality of life and living conditions), and behavioural (other dietary choices and physical activity levels) factors.
Data Availability Statement
The raw data supporting the conclusions of this article will be made available by the authors, without undue reservation.
Ethics Statement
The studies involving human participants were reviewed and approved by UCL Division of Psychology and Language 1220 Sciences (CEHP/2020/759). Participants provided their informed consent to participate in this study.
Author Contributions
SD, JM, JN, EB, DK, AH, and LS: conceptualisation and methodology. EB: statistical support. SD and JM: formal analysis. SD: first manuscript draft. EB, LS, DK, and AH: writing—review and editing. AH and LS: supervision. All authors approved the final manuscript.
Funding
This project was partially funded by an ongoing Cancer Research UK Programme Grant to UCL Tobacco and Alcohol Research Group (C1417/A22962) and by SPECTRUM, a UK Prevention Research Partnership Consortium (MR/S037519/1). SD and JM are funded by an MRC grant (MR/N013867/1).
Conflict of Interest
The authors declare that the research was conducted in the absence of any commercial or financial relationships that could be construed as a potential conflict of interest.
The reviewer LS declared a shared affiliation with the authors to the handling Editor at time of review.
Publisher's Note
All claims expressed in this article are solely those of the authors and do not necessarily represent those of their affiliated organizations, or those of the publisher, the editors and the reviewers. Any product that may be evaluated in this article, or claim that may be made by its manufacturer, is not guaranteed or endorsed by the publisher.
Supplementary Material
The Supplementary Material for this article can be found online at: https://www.frontiersin.org/articles/10.3389/fnut.2021.788043/full#supplementary-material
Abbreviations
BF, Bayes factor; BMI, body mass index; CI, confidence interval; DALYs, disability-adjusted life years; FV, fruit and vegetables; GEE, generalized estimating equation; HEBECO, HEalth BEhaviours during the COVID-19 pandemic; HFSS, high fat, salt, and/or sugar; MET, metabolic equivalent; MVPA, moderate to vigorous physical activity; OR, odds ratio; SD, standard deviation; SPA, strengthening physical activity; QIC, Quasi-likelihood under Independence Model Criterion; WHO, World Health Organisation.
Footnotes
References
1. Mazidii M, Leeming E, Merino J, Nguyen L, Selvachandran S, Maher T, et al. Impact of COVID-19 on health behaviours and body weight: a prospective observational study in a cohort of 1.1 million UK and US individuals. Res Squ [Preprint]. (2021). doi: 10.21203/rs.3.rs-179013/v1
2. Stanaway JD, Afshin A, Gakidou E, Lim SS, Abate D, Abate KH, et al. Global, regional, and national comparative risk assessment of 84 behavioural, environmental and occupational, and metabolic risks or clusters of risks for 195 countries and territories, 1990–2017: a systematic analysis for the Global Burden of Disease Study 2017. Lancet. (2018) 392:1923–94. doi: 10.1016/S0140-6736(18)32225-6
3. Newton JN, Briggs ADM, Murray CJL, Dicker D, Foreman KJ, Wang H, et al. Changes in health in England, with analysis by English regions and areas of deprivation, 1990-2013: a systematic analysis for the Global Burden of Disease Study (2013). Lancet Lond Engl. (2015) 386:2257–74. doi: 10.1016/S0140-6736(15)00195-6
4. Deschasaux-Tanguy M, Druesne-Pecollo N, Esseddik Y, de Edelenyi FS, Allès B, Andreeva VA, et al. Diet and physical activity during the coronavirus disease 2019 (COVID-19) lockdown (March–May 2020): results from the French NutriNet-Santé cohort study. Am J Clin Nutr. (2021). 113:924–38. doi: 10.1093/ajcn/nqaa336
5. Khan MA, Menon P, Govender R, Samra A, Nauman J, Ostlundh L, et al. Systematic review of the effects of pandemic confinements on body weight and their determinants. Br J Nutr. (2021) 2021:1–74. doi: 10.1017/S0007114521000921
6. Doraiswamy S, Cheema S, Al Mulla A, Mamtani R. COVID-19 lockdown and lifestyles: a narrative review. F1000Research. (2021) 10:363. doi: 10.12688/f1000research.52535.1
7. Chew HSJ, Lopez V. Global impact of COVID-19 on weight and weight-related behaviors in the adult population: a scoping review. Int J Environ Res Public Health. (2021) 18:1876:1–1876:27. doi: 10.3390/ijerph18041876
8. Wielgoszewska B, Maddock J, Green MJ, Gessa GD, Parsons S, Griffith GJ, et al. The UK coronavirus job retention scheme and changes in diet, physical activity and sleep during the COVID-19 pandemic: evidence from eight longitudinal studies. MedRxiv [Preprint]. (2021) 2021:2021.06.08.21258531. doi: 10.1101/2021.06.08.21258531
9. Robinson E, Boyland E, Chisholm A, Harrold J, Maloney NG, Marty L, et al. Obesity, eating behavior and physical activity during COVID-19 lockdown: a study of UK adults. Appetite. (2021) 156:104853. doi: 10.1016/j.appet.2020.104853
10. Ogundijo DA, Tas AA, Onarinde BA. Exploring the impact of COVID-19 pandemic on eating and purchasing behaviours of people living in England. Nutrients. (2021) 13:1499. doi: 10.3390/nu13051499
11. Albalawi A, Hambly C, Speakman JR. The impact of the novel coronavirus movement restrictions in the United Kingdom on food outlet usage and body mass index. Obes Sci Pract. (2021) 7:302–6. doi: 10.1002/osp4.477
12. Kubo Y, Ishimaru T, Hino A, Nagata M, Ikegami K, Tateishi S, et al. A cross-sectional study of the association between frequency of telecommuting and unhealthy dietary habits among Japanese workers during the COVID-19 pandemic. MedRxiv [Preprint]. (2021) 2021:2021.06.28.21259558. doi: 10.1101/2021.06.28.21259558
13. Salon D, Conway MW, Silva DC da, Chauhan RS, Derrible S, Mohammadian A (Kouros), et al. The potential stickiness of pandemic-induced behavior changes in the United States. Proc Natl Acad Sci USA. (2021) 118:e2106499118. doi: 10.1073/pnas.2106499118
14. Bennett G, Young E, Butler I, Coe S. The impact of lockdown during the COVID-19 outbreak on dietary habits in various population groups: a scoping review. Front Nutr. (2021) 8:626432. doi: 10.3389/fnut.2021.626432
15. Wilson JJ, McMullan I, Blackburn NE, Klempel N, Yakkundi A, Armstrong NC, et al. Changes in dietary fat intake and associations with mental health in a UK public sample during the COVID-19 pandemic. J Public Health Oxf Engl. (2021) 2021:fdab009. doi: 10.1093/pubmed/fdab009
16. Stroud R, Smith K, O'Connell M. The dietary impact of the COVID-19 pandemic. IFS. (2021). doi: 10.1920/wp.ifs.2021.1821 Available online at: https://ifs.org.uk/publications/15503
17. Buckland NJ, Swinnerton LF, Ng K, Price M, Wilkinson LL, Myers A, et al. Susceptibility to increased high energy dense sweet and savoury food intake in response to the COVID-19 lockdown: the role of craving control and acceptance coping strategies. Appetite. (2021) 158:105017. doi: 10.1016/j.appet.2020.105017
18. Food Standards Agency. The COVID-19 Consumer Research. Food Standards Agency (2021). Available online at: https://www.food.gov.uk/research/research-projects/the-covid-19-consumer-research (accessed on March 24, 2021).
19. Bakaloudi DR, Jeyakumar DT, Jayawardena R, Chourdakis M. The impact of COVID-19 lockdown on snacking habits, fast-food and alcohol consumption: a systematic review of the evidence. Clin Nutr Edinb Scotl. (2021). doi: 10.1016/j.clnu.2021.04.020. [Epub ahead of print].
20. Yang G, Lin X, Fang A, Zhu H. Eating habits and lifestyles during the initial stage of the COVID-19 lockdown in china: a cross-sectional study. Nutrients. (2021) 13:970. doi: 10.3390/nu13030970
21. Herle M, Smith AD, Bu F, Steptoe A, Fancourt D. Trajectories of eating behavior during COVID-19 lockdown: longitudinal analyses of 22,374 adults. Clin Nutr Espen. (2021) 42:158–65. doi: 10.1016/j.clnesp.2021.01.046
22. Al-Musharaf S. Prevalence and predictors of emotional eating among healthy young saudi women during the COVID-19 pandemic. Nutrients. (2020) 12:2923. doi: 10.3390/nu12102923
23. Bonaccio M, Costanzo S, Ruggiero E, Persichillo M, Esposito S, Olivieri M, et al. Changes in ultra-processed food consumption during the first Italian lockdown following the COVID-19 pandemic and major correlates: results from two population-based cohorts. Public Health Nutr. (2021) 24:3905–15. doi: 10.1017/S1368980021000999
24. Cecchetto C, Aiello M, Gentili C, Ionta S, Osimo SA. Increased emotional eating during COVID-19 associated with lockdown, psychological and social distress. Appetite. (2021) 160:105122. doi: 10.1016/j.appet.2021.105122
25. Di Renzo L, Gualtieri P, Cinelli G, Bigioni G, Soldati L, Attinà A, et al. Psychological aspects and eating habits during COVID-19 home confinement: results of EHLC-COVID-19 Italian online survey. Nutrients. (2020) 12:2152. doi: 10.3390/nu12072152
26. Kaufman-Shriqui V, Navarro DA, Raz O, Boaz M. Multinational dietary changes and anxiety during the coronavirus pandemic-findings from Israel. Isr J Health Policy Res. (2021) 10:28. doi: 10.1186/s13584-021-00461-1
27. Ruggiero E, Mignogna C, Costanzo S, Persichillo M, Castelnuovo AD, Esposito S, et al. Changes in the consumption of foods characterising the Mediterranean dietary pattern and major correlates during the COVID-19 confinement in Italy: results from two cohort studies. Int J Food Sci Nutr. (2021) 72:1105–17. doi: 10.1080/09637486.2021.1895726
28. Schwingshackl L, Schwedhelm C, Hoffmann G, Lampousi A-M, Knüppel S, Iqbal K, et al. Food groups and risk of all-cause mortality: a systematic review and meta-analysis of prospective studies. Am J Clin Nutr. (2017) 105:1462–73. doi: 10.3945/ajcn.117.153148
29. Aune D, Giovannucci E, Boffetta P, Fadnes LT, Keum N, Norat T, et al. Fruit and vegetable intake and the risk of cardiovascular disease, total cancer and all-cause mortality—a systematic review and dose-response meta-analysis of prospective studies. Int J Epidemiol. (2017) 46:1029–56. doi: 10.1093/ije/dyw319
30. Skotnicka M, Karwowska K, Kłobukowski F, Wasilewska E, Małgorzewicz S. Dietary habits before and during the COVID-19 epidemic in selected european countries. Nutrients. (2021) 13:1690. doi: 10.3390/nu13051690
31. Public Health England. National Diet and Nutrition Survey: Diet and Physical Activity—A Follow-up Study During COVID-19. (2021). Available online at: https://www.gov.uk/government/statistics/ndns-diet-and-physical-activity-a-follow-up-study-during-covid-19 (accessed September 23, 2021).
32. Villadsen A, Patalay P, Bann D. Mental health in relation to changes in sleep, exercise, alcohol and diet during the COVID-19 pandemic: examination of four UK cohort studies. MedRxiv [Preprint]. (2021) 2021:2021.03.26.21254424. doi: 10.1101/2021.03.26.21254424
33. Dicken SJ, Mitchell JJ, Newberry Le Vay J, Beard E, Kale D, Herbec A, et al. Impact of COVID-19 pandemic on weight and BMI among UK adults: a longitudinal analysis of data from the HEBECO study. Nutrients. (2021) 13:2911. doi: 10.3390/nu13092911
34. Harris PA, Taylor R, Thielke R, Payne J, Gonzalez N, Conde JG. Research electronic data capture (REDCap)–a metadata-driven methodology and workflow process for providing translational research informatics support. J Biomed Inform. (2009) 42:377–81. doi: 10.1016/j.jbi.2008.08.010
35. Harris PA, Taylor R, Minor BL, Elliott V, Fernandez M, O'Neal L, et al. The REDCap consortium: building an international community of software platform partners. J Biomed Inform. (2019) 95:103208. doi: 10.1016/j.jbi.2019.103208
36. Critchlow N, Bauld L, Thomas C, Hooper L, Vohra J. Awareness of marketing for high fat, salt or sugar foods, and the association with higher weekly consumption among adolescents: a rejoinder to the UK government's consultations on marketing regulation. Public Health Nutr. (2020) 23:2637–46. doi: 10.1017/S1368980020000075
37. Thomas C, Hooper L, Petty R, Thomas F, Rosenberg G, Vohra J. 10 Years On: New Evidence on TV Marketing and Junk Food Eating Amongst 11–19 Year Olds 10 Years After Broadcast Regulations. (2018). Available online at: https://www.basw.co.uk/resources/10-years-new-evidence-tv-marketing-and-junk-food-eating-amongst-11-19-year-olds-10-years (accessed April 8, 2021).
38. Newman A, Newberry Le Vay J, Critchlow N, Froguel A, Clark M, Vohra J. The HFSS beat goes on: awareness of marketing for high fat, salt and sugar foods and the association with consumption in the 2017 and 2019. Youth Obesity Policy Surveys. (2020). Available online at: hfss_beat_goes_on_december_2020_-_full_report/the_hfss_beat_goes_on_december_2020_-_full_report.pdf (accessed March 18, 2021).
39. NHS. Eat Well. (2019). Available online at: https://www.nhs.uk/live-well/eat-well/ (accessed April 15, 2019).
40. Bhutani S, vanDellen MR, Cooper JA. Longitudinal weight gain and related risk behaviors during the COVID-19 pandemic in adults in the US. Nutrients. (2021) 13:671. doi: 10.3390/nu13020671
41. Kriaucioniene V, Bagdonaviciene L, Rodríguez-Pérez C, Petkeviciene J. Associations between changes in health behaviours and body weight during the COVID-19 quarantine in Lithuania: the Lithuanian COVIDiet study. Nutrients. (2020) 12:3119. doi: 10.3390/nu12103119
42. Sigal RJ, Kenny GP, Wasserman DH, Castaneda-Sceppa C. Physical activity/exercise and type 2 diabetes. Diabetes Care. (2004) 27:2518–39. doi: 10.2337/diacare.27.10.2518
43. American College of Sports Medicine. American College of Sports Medicine position stand. Progression models in resistance training for healthy adults. Med Sci Sports Exerc. (2009). 41:687–708. doi: 10.1249/MSS.0b013e3181915670
44. Garber CE, Blissmer B, Deschenes MR, Franklin BA, Lamonte MJ, Lee I-M, et al. American college of sports medicine position stand. Quantity and quality of exercise for developing and maintaining cardiorespiratory, musculoskeletal, and neuromotor fitness in apparently healthy adults: guidance for prescribing exercise. Med Sci Sports Exerc. (2011) 43:1334–59. doi: 10.1249/MSS.0b013e318213fefb
45. IPAQ. Guidelines for Data Processing and Analysis of the International Physical Activity Questionnaire (IPAQ)—Short Form. (2004). Available online at: https://www.physio-pedia.com/images/c/c7/Quidelines_for_interpreting_the_IPAQ.pdf (accessed June 12, 2021).
46. Kaminsky LA, Montoye AHK. Physical activity and health: what is the best dose? J Am Heart Assoc. (2014) 3:e001430. doi: 10.1161/JAHA.114.001430
47. GOV.UK. Alcohol Consumption: Advice on Low Risk Drinking. (2016). Available online at: https://www.gov.uk/government/publications/alcohol-consumption-advice-on-low-risk-drinking (accessed May 27, 2021).
48. Office for National Statistics. Population Estimates for the UK, England and Wales, Scotland and Northern Ireland. (2019). Available online at: https://www.ons.gov.uk/peoplepopulationandcommunity/populationandmigration/populationestimates/bulletins/annualmidyearpopulationestimates/mid2018 (accessed April 7, 2021).
49. Ballinger GA. using generalized estimating equations for longitudinal data analysis. Organ Res Methods. (2004) 7:127–50. doi: 10.1177/1094428104263672
50. Azuero A, Pisu M, McNees P, Burkhardt J, Benz R, Meneses K. An application of longitudinal analysis with skewed outcomes. Nurs Res. (2010) 59:301–7. doi: 10.1097/NNR.0b013e3181e507f1
51. Lakens D, McLatchie N, Isager PM, Scheel AM, Dienes Z. Improving Inferences about Null Effects with Bayes Factors and Equivalence Tests. J Gerontol B Psychol Sci Soc Sci. (2020) 75:45–57. doi: 10.1093/geronb/gby065
52. Dienes Z. Using Bayes to get the most out of non-significant results. Front Psychol. (2014) 5:781. doi: 10.3389/fpsyg.2014.00781
53. Neira C, Godinho R, Rincón F, Mardones R, Pedroso J. Consequences of the COVID-19 syndemic for nutritional health: a systematic review. Nutrients. (2021) 13:1168. doi: 10.3390/nu13041168
54. Zupo R, Castellana F, Sardone R, Sila A, Giagulli VA, Triggiani V, et al. Preliminary trajectories in dietary behaviors during the COVID-19 pandemic: a public health call to action to face obesity. Int J Environ Res Public Health. (2020) 17:7073. doi: 10.3390/ijerph17197073
55. Zhang X, Chen B, Jia P, Han J. Locked on salt? Excessive consumption of high-sodium foods during COVID-19 presents an underappreciated public health risk: a review. Environ Chem Lett. (2021) 1:1–13. doi: 10.2139/ssrn.3804495
56. Zeigler Z. COVID-19 self-quarantine and weight gain risk factors in adults. Curr Obes Rep. (2021) 10:423–433. doi: 10.1007/s13679-021-00449-7
57. Salazar-Fernández C, Palet D, Haeger PA, Román Mella F. The perceived impact of COVID-19 on comfort food consumption over time: the mediational role of emotional distress. Nutrients. (2021) 13:1910. doi: 10.3390/nu13061910
58. Rolland B, Haesebaert F, Zante E, Benyamina A, Haesebaert J, Franck N. Global changes and factors of increase in caloric/salty food intake, screen use, and substance use during the early COVID-19 containment phase in the general population in france: survey study. JMIR Public Health Surveill. (2020) 6:e19630. doi: 10.2196/19630
59. Jafri A, Mathe N, Aglago EK, Konyole SO, Ouedraogo M, Audain K, et al. Food availability, accessibility and dietary practices during the COVID-19 pandemic: a multi-country survey. Public Health Nutr. (2021) 24:1798–805. doi: 10.1017/S1368980021000987
60. Monteiro C, Cannon G, Lawrence M, Louzada ML, Machado P. Ultra-processed foods, Diet Quality, and Health Using the NOVA Classification System. (2019). Available online at: http://www.fao.org/3/ca5644en/ca5644en.pdf (accessed March 3, 2020).
61. Janssen M, Chang BPI, Hristov H, Pravst I, Profeta A, Millard J. Changes in Food consumption during the COVID-19 pandemic: analysis of consumer survey data from the first lockdown period in Denmark, Germany, and Slovenia. Front Nutr. (2021) 8:635859. doi: 10.3389/fnut.2021.635859
62. Devonport TJ, Nicholls W, Fullerton C. A systematic review of the association between emotions and eating behaviour in normal and overweight adult populations. J Health Psychol. (2019) 24:3–24. doi: 10.1177/1359105317697813
63. Coulthard H, Sharps M, Cunliffe L, van den Tol A. Eating in the lockdown during the Covid 19 pandemic; self-reported changes in eating behaviour, and associations with BMI, eating style, coping and health anxiety. Appetite. (2021) 161:105082. doi: 10.1016/j.appet.2020.105082
64. Sadler JR, Thapaliya G, Jansen E, Aghababian AH, Smith KR, Carnell S. COVID-19 stress and food intake: protective and risk factors for stress-related palatable food intake in U.S. Adults. Nutrients. (2021) 13:901. doi: 10.3390/nu13030901
65. McAtamney K, Mantzios M, Egan H, Wallis DJ. Emotional eating during COVID-19 in the United Kingdom: exploring the roles of alexithymia and emotion dysregulation. Appetite. (2021) 161:105120. doi: 10.1016/j.appet.2021.105120
66. Theobald C, White A. British nutrition foundation healthy eating week 2020–insights into the effect of COVID-19 on eating activity habits of adults children in the UK. Nutr Bull. (2021). 46:238–45. doi: 10.1111/nbu.12500
67. Brewer JA, Ruf A, Beccia AL, Essien GI, Finn LM, Lutterveld R van, et al. Can mindfulness address maladaptive eating behaviors? Why traditional diet plans fail and how new mechanistic insights may lead to novel interventions. Front Psychol. (2018) 9:1418. doi: 10.3389/fpsyg.2018.01418
68. Fluharty M, Fancourt D. How Have People Been Coping during the COVID-19 Pandemic? Patterns and Predictors of Coping Strategies amongst 26,016 UK Adults. BMC Psychol. (2021) 9:107–9. doi: 10.31234/osf.io/nx7y5
69. Bann D, Villadsen A, Maddock J, Hughes A, Ploubidis GB, Silverwood R, et al. Changes in the behavioural determinants of health during the COVID-19 pandemic: gender, socioeconomic and ethnic inequalities in five British cohort studies. J Epidemiol Community Health. (2021) 75:1136–42. doi: 10.1101/2020.07.29.20164244
70. Greer SM, Goldstein AN, Walker MP. The impact of sleep deprivation on food desire in the human brain. Nat Commun. (2013) 4:2259. doi: 10.1038/ncomms3259
71. Aknin L, Neve J-ED, Dunn E, Fancourt D, Goldberg E, Helliwell J, et al. Mental health during the first year of the COVID-19 pandemic: a review and recommendations for moving forward. Perspect Psychol Sci. (in press). doi: 10.31234/osf.io/zw93g
72. Saunders R, Buckman JEJ, Fonagy P, Fancourt D. Understanding different trajectories of mental health across the general population during the COVID-19 pandemic. Psychol Med. (2021) 2021:1–9. doi: 10.1017/S0033291721000957
73. Robinson E, Blissett J, Higgs S. Social influences on eating: implications for nutritional interventions. Nutr Res Rev. (2013) 26:166–76. doi: 10.1017/S0954422413000127
74. World Health Organisation. Healthy Diet. (2018). Available online at: https://www.who.int/publications/m/item/healthy-diet-factsheet394 (accessed February 25, 2021).
75. GOV.UK. National Diet and Nutrition Survey Rolling programme Years 9 to 11 (2016/2017 to 2018/2019). (2020). Available online at: https://assets.publishing.service.gov.uk/government/uploads/system/uploads/attachment_data/file/943114/NDNS_UK_Y9-11_report.pdf (accessed February 26, 2021).
76. Steel N, Ford JA, Newton JN, Davis ACJ, Vos T, Naghavi M, et al. Changes in health in the countries of the UK and 150 English Local authority areas 1990–2016: a systematic analysis for the global burden of disease study 2016. Lancet Lond Engl. (2018) 392:1647–61. doi: 10.1016/S0140-6736(18)32207-4
77. GOV.UK. Tackling Obesity: Empowering Adults and Children to Live Healthier Lives. (2020). Available online at: https://www.gov.uk/government/publications/tackling-obesity-government-strategy/tackling-obesity-empowering-adults-and-children-to-live-healthier-lives (accessed June 28, 2021).
78. GOV.UK. New Obesity Strategy Unveiled as Country Urged to Lose Weight to Beat Coronavirus (COVID-19) and Protect the NHS. (2020). Available online at: https://www.gov.uk/government/news/new-obesity-strategy-unveiled-as-country-urged-to-lose-weight-to-beat-coronavirus-covid-19-and-protect-the-nhs (accessed June 28, 2021).
79. Conklin AI, Forouhi NG, Surtees P, Khaw K-T, Wareham NJ, Monsivais P. Social relationships and healthful dietary behaviour: evidence from over-50s in the EPIC cohort, UK. Soc Sci Med. (2014) 100:167–75. doi: 10.1016/j.socscimed.2013.08.018
80. McClung HL, Ptomey LT, Shook RP, Aggarwal A, Gorczyca AM, Sazonov ES, et al. Dietary intake and physical activity assessment: current tools, techniques, and technologies for use in adult populations. Am J Prev Med. (2018) 55:e93–104. doi: 10.1016/j.amepre.2018.06.011
81. Hill RJ, Davies PS. The validity of self-reported energy intake as determined using the doubly labelled water technique. Br J Nutr. (2001) 85:415–30. doi: 10.1079/BJN2000281
Keywords: COVID-19, dietary behaviours, HFSS, snacking, BMI, health behaviours, weight management, diet
Citation: Dicken SJ, Mitchell JJ, Newberry Le Vay J, Beard E, Kale D, Herbec A and Shahab L (2022) Impact of the COVID-19 Pandemic on Diet Behaviour Among UK Adults: A Longitudinal Analysis of the HEBECO Study. Front. Nutr. 8:788043. doi: 10.3389/fnut.2021.788043
Received: 01 October 2021; Accepted: 22 November 2021;
Published: 13 January 2022.
Edited by:
Rino Bellocco, University of Milano-Bicocca, ItalyReviewed by:
Nicola Joy Buckland, The University of Sheffield, United KingdomLucy Serpell, University College London, United Kingdom
Copyright © 2022 Dicken, Mitchell, Newberry Le Vay, Beard, Kale, Herbec and Shahab. This is an open-access article distributed under the terms of the Creative Commons Attribution License (CC BY). The use, distribution or reproduction in other forums is permitted, provided the original author(s) and the copyright owner(s) are credited and that the original publication in this journal is cited, in accordance with accepted academic practice. No use, distribution or reproduction is permitted which does not comply with these terms.
*Correspondence: Samuel J. Dicken, c2FtdWVsLmRpY2tlbi4yMEB1Y2wuYWMudWs=
†These authors share senior authorship