Ferroptosis-related differentially expressed genes serve as new biomarkers in ischemic stroke and identification of therapeutic drugs
- 1School of Pharmacy, Minzu University of China, Beijing, China
- 2Key Laboratory of Ethnomedicine, Minzu University of China, Ministry of Education, Beijing, China
Background: Iron is an essential nutrient element, and iron metabolism is related to many diseases. Ferroptosis is an iron-dependent form of regulated cell death associated with ischemic stroke (IS). Hence, this study intended to discover and validate the possible ferroptosis-related genes involved in IS.
Materials and methods: GSE16561, GSE37587, and GSE58294 were retrieved from the GEO database. Using R software, we identified ferroptosis-related differentially expressed genes (DEGs) in IS. Protein-protein interactions (PPIs) and enrichment analyses were conducted. The ROC curve was plotted to explore the diagnostic significance of those identified genes. The consistent clustering method was used to classify the IS samples. The level of immune cell infiltration of different subtypes was evaluated by ssGSEA and CIBERSORT algorithm. Validation was conducted in the test sets GSE37587 and GSE58294.
Results: Twenty-one ferroptosis-related DEGs were detected in IS vs. the normal controls. Enrichment analysis shows that the 21 DEGs are involved in monocarboxylic acid metabolism, iron ion response, and ferroptosis. Moreover, their expression levels were pertinent to the age and gender of IS patients. The ROC analysis demonstrated remarkable diagnostic values of LAMP2, TSC22D3, SLC38A1, and RPL8 for IS. Transcription factors and targeting miRNAs of the 21 DEGs were determined. Vandetanib, FERRIC CITRATE, etc., were confirmed as potential therapeutic drugs for IS. Using 11 hub genes, IS patients were categorized into C1 and C2 subtypes. The two subtypes significantly differed between immune cell infiltration, checkpoints, and HLA genes. The 272 DEGs were identified from two subtypes and their biological functions were explored. Verification was performed in the GSE37587 and GSE58294 datasets.
Conclusion: Our findings indicate that ferroptosis plays a critical role in the diversity and complexity of the IS immune microenvironment.
Introduction
There are genetic and environmental risk factors that interact to cause ischemic stroke (IS). Society and families are burdened by IS because it is the leading cause of disability (1). IS patients must continue taking medication for a long period after stroke onset, bringing about huge financial, mental, and time-wise burdens. IS risk factors include hypertension, diabetes, hyperlipidemia, and smoking. However, the molecular mechanism remains undetermined. Studies showed that early IS diagnosis can positively impact therapeutic outcomes and prognoses (2). Therefore, a better understanding of IS and identifying new biomarkers and therapeutic targets are urgently needed.
Iron is the most abundant trace element in the human body and is also considered indispensable for IS development (3). Ferroptosis is a unique type of programmed cell death distinguished by excessive iron buildup and lipid peroxidation (4). A recent study suggested that ferroptosis played an essential role in tumorigenesis and cancer progression (5). Additionally, ferroptosis is highly involved in many other diseases, such as IS and heart diseases (6). Moreover, research also proved that ferroptosis-related gene signatures could be used as a biomarker to diagnose, predict, and treat multiple diseases (7, 8). Nevertheless, the function of ferroptosis-related genes in IS is yet unclear.
In addition, stroke is often followed by post-stroke infection due to systemic immunosuppression, which has a worse outcome (9). It has been shown that immunomodulatory approaches, such as T-cell transfer and activators of natural killer T cells (NKTs), can reduce post-stroke immunosuppression (10). Immunomodulatory approaches can effectively manage stroke and its complications by targeting multiple elements of the immune system (11). Several well-known drugs, like azithromycin and metformin, can change the innate immune response. Both of these drugs are known to protect the brain after a stroke (12). So, it is important to figure out how immunosuppression works in stroke so that these drugs can be used to treat people. However, the immune mechanisms implicated in IS and IS-associated systemic immunosuppression are still poorly understood.
In this study, by comparing IS and normal samples in the GSE16561 dataset, differentially expressed genes (DEGs) were identified, which were then intersected with ferroptosis-related genes in the FerrDb database. We performed an enrichment analysis, as well as an investigation of expression levels and clinical significance. Unsupervised cluster analysis was performed on patients based on hub gene expression, and the characteristics of the immune microenvironment among different subtypes were analyzed. Validation was carried out in the GSE37587 and GSE58294 datasets (Figure 1).
Materials and methods
Data source
Data chips, microarrays, and gene expression data from GEO1 are available for research and analysis (13). Datasets were included and excluded according to the following criteria: (i) genomic wide expression mRNA microarray data had to be included, (ii) IS samples were required to be included, and (iii) specimen numbers must be greater than 30.
Three gene expression datasets, GSE16561 (14) and GSE37587 (15), derived from the GEO (GPL6883, Illumina HumanRef-8 v3.0 expression bead chip, array, Homo sapiens) were obtained with corresponding clinical data. GSE58294 (16) derived from the GEO (GPL570, Affymetrix Human Genome U133 Plus 2.0 Array, Homo sapiens) were obtained with corresponding clinical data. Data of the datasets were extracted from the total RNA of whole blood. Altogether, 39 IS and 24 normal whole blood samples were obtained from the GSE16561 cohort, 68 IS whole blood samples were obtained from the GSE37587 cohort, and 69 IS and 23 normal whole blood samples were obtained from the GSE16561 cohort.
A total of 388 ferroptosis-related genes were found in the FerrDb database2 (17; Supplementary Table 1) after removing duplicates. These genes include drivers, suppressors, and markers.
Quality control
GSE16561
The raw expression profile GSE16561_RAW.tar was downloaded from the GEO database. Probes were annotated to their respective gene symbols via the GPL6883 platform file. Mean expression levels were used to compute gene symbols from several probes. Quantile normalization and log2 transformations were applied to raw data. Two abnormal IS samples (3100193_Stroke and 3100137_Stroke) were excluded based on principal component analysis (PCA). The following analysis included 37 IS and 24 normal samples. The GSE58294 dataset was also preprocessed according to the above process.
GSE37587
The raw expression profile GSE37587_non-normalized.txt.gz was downloaded from the GEO database. Probes were annotated to their corresponding gene symbols using the GPL6883 annotation file. Mean expression levels were used to compute gene symbols from several probes. Following the log2-transformation and quantile normalization, the raw expression files of the GSE16561 and the GSE37587 were combined. The batch correction was performed with the ComBat algorithm of the ‘‘sva’’ R package.3 The final dataset comprised 68 IS samples from GSE37587 and 24 normal samples from GSE16561. According to PCA, 3 abnormal IS samples were deleted (GSM922927, GSM922908, and GSM922905), thus 65 IS samples and 24 normal samples were included in the subsequent analysis. At the same time, a total of 107 IS samples from the two data sets were used for cluster analysis.
Identification of ferroptosis-related differentially expressed genes and functional analysis
Limma4 (18) was used to identify DEGs between IS and normal samples in the GSE16561 (adjustment p < 0.05 and | log2FC| > 0.5). Heat maps were generated using the R package ‘‘pheatmap,’’5 exhibiting the top 20 genes with the most significant upregulation or downregulation, respectively. Twenty-one ferroptosis-related DEGs were obtained with a Venn diagram using the R package ‘‘Venn,’’6 and their expression correlation was calculated with the R package ‘‘corrplot’’7 and visualized using the ‘‘circlize’’ package8 (19). The ability of the 21 ferroptosis-related DEGs to distinguish between IS and normal samples was determined with PCA. Metascape (20) was used for functional analysis. Cut-off value: P < 0.05.
Construct a diagnostic model of 21 ischemic stroke-associated ferroptosis genes
A diagnostic model was constructed by the least absolute shrinkage and selection operator (Lasso) analysis to analyze and identify the redundancy factors. Finally, receiver operating feature (ROC) scores were used to evaluate the diagnostic performance of the model.
Bioinformatics analysis of 21 ferroptosis-related differentially expressed genes
In this study, Wilcoxon rank sum tests were used to examine the association between ferroptosis-related DEGs and age and gender of IS patients. Via the Enrichr platform9 (21), the transcription factors, upstream miRNAs, and small-molecular drugs of the 21 ferroptosis-related DEGs were predicted using the TRRUST, miRTarBase, and DSigDB databases, respectively. The ROC curve was generated using the ‘‘pROC’’ package10 (22) and visualized with the ‘‘ggplot2’’ package11 (23).
Based on the STRING database (24), PPI networks were constructed, in which the interactions with a score higher than 0.4 were considered statistically significant. The hub genes were selected with the plug-in CytoNCA (25) of the Cytoscape software V3.7.1 (26) and subjected to functional enrichment analysis using GeneMANIA12 (27). FDR < 0.05 was used as a cutoff point.
Consensus clustering analysis
ConsensusClusterPlus (28) was used for cluster analysis. We used an agglomerative km clustering algorithm with one Pearson correlation distance and resampled 80% of the samples 10 times. Empirical cumulative distribution function plots were used to determine the optimal number of clusters.
Immune cell infiltration analysis
Single sample gene set enrichment analysis (ssGSEA) was used to analyze the infiltration levels of immune cells based on 29 immune-related markers’ expression profiles. Also, CIBERSORT (29) was used to further analyze immune cell infiltration levels. Wilcoxon rank-sum tests were used to determine differences in immune cell proportions. The statistical significance threshold was set at p < 0.05.
Gene set variation analysis
The GSVA (30) approach was used to examine important pathways and molecular processes by obtaining the h.all.v7.4.symbols.gmt and c2.cp.kegg.v7.4.symbols.gmt subsets from the Molecular Signatures Database (31). The minimum gene set was set to 5 and the maximum gene set was set to 5,000, and the enrichment scores were calculated for each sample in each gene set. The final enrichment score matrix was obtained. The differences in GSVA scores between subtypes for each gene set were compared using the limma package. FDR < 0.05 was used as a cutoff point.
Identification of differentially expressed genes between different subtypes
Differentially expressed genes were screened for subtypes in the integrated dataset using the R package limma with | Fold Change| > 1.5 and FDR < 0.05. The differential genes were shown by volcano plot and heat map.
Functional enrichment analysis
Biological functions were analyzed using the ClusterProfiler package (32), which includes GO and KEGG. Use the Benjamini–Hochberg method to adjust the p-value for multiple tests. P < 0.05 was used as a cutoff point.
Statistical analysis
Statistical analysis was performed using R 4.1.0. Wilcoxon or Student’s t-test compared the two groups. Pearson’s or Spearman’s test determined the variables’ correlation. A Chi-square test was performed to compare two categorized variable groups. The statistical significance threshold was set at p < 0.05.
Results
Data preprocessing
GSE16561
The box plot of the raw data demonstrated that gene expression levels were unevenly distributed across different samples (Figure 2A), which was processed via quantile normalization (Figure 2B). According to the 2D and 3D PCA plots (Figures 2C,D), two abnormal IS samples in the normal controls were deleted. Further validation distinguished between the groups and illustrated good clustering of samples within the same group (Figures 2E,F).
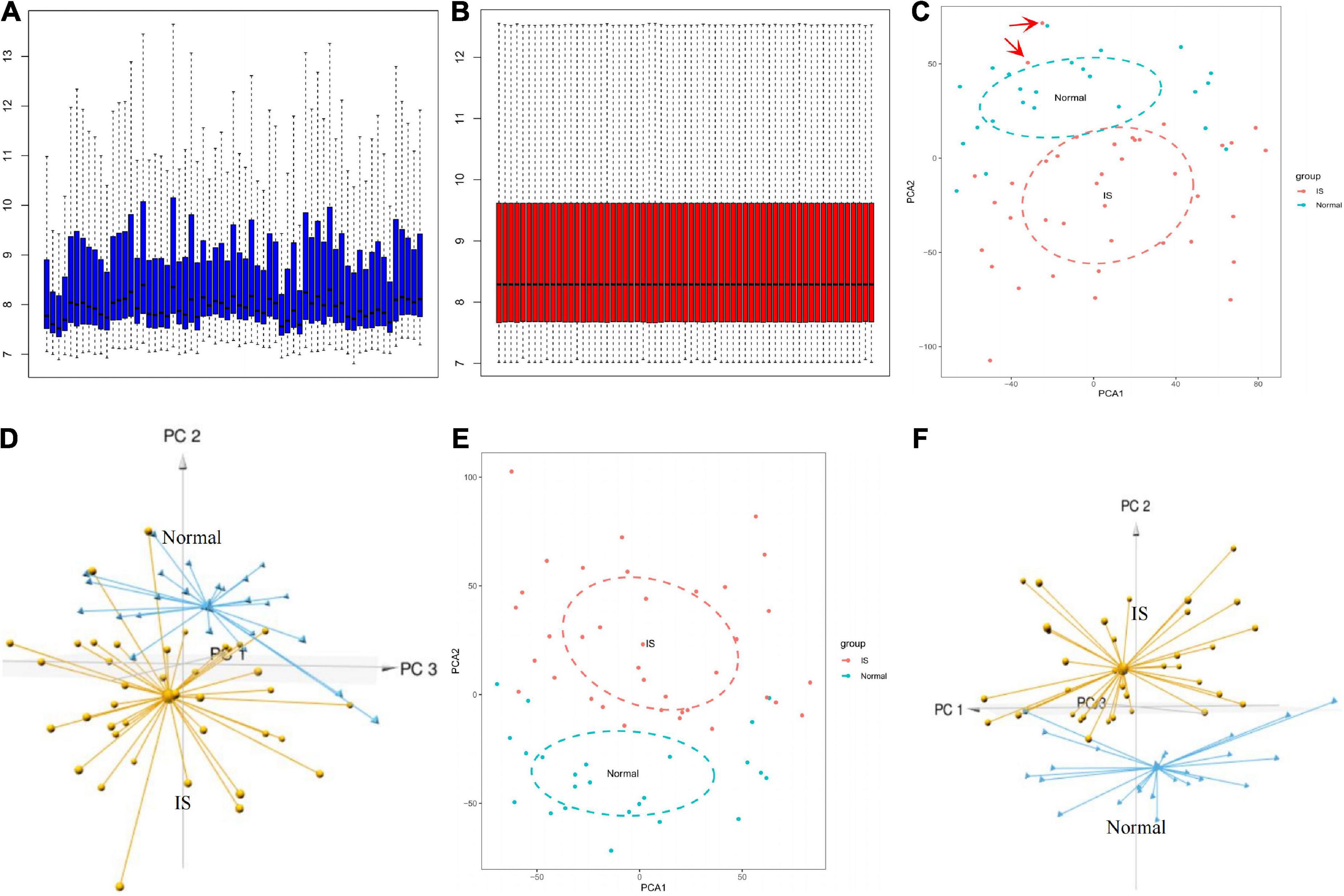
Figure 2. GSE16561 data set preprocessing. Box plot showing the gene expression level between different samples before (A) and after (B) normalization. 2D and 3D PCA plots demonstrated the distribution of samples before (C,D) and after (E,F) pretreatment. PCA, principal components analysis.
GSE37587 test set
Normalized gene expression data exhibited a uniform distribution in the samples (Supplementary Figure 1A). Three abnormal IS samples were excluded from the normal control based on the 2D and 3D PCA plots (Supplementary Figures 1B,C). PCA was repeated and demonstrated excellent discrimination between the groups and good clustering of samples within the same group (Supplementary Figures 1D,E).
Ferroptosis-related differentially expressed genes differentiate ischemic stroke patients from normal controls
According to | log2FC | > 0.5 and adjustment p < 0.05, 584 DEGs were obtained from the GSE16561 dataset, including 319 genes up-regulated and 265 genes down-regulated in IS samples (Figure 3A; Supplementary Table 2). The top 20 genes with the most significant upregulation or downregulation were selected to plot the Heat map (Figure 3B). To investigate the association between IS and ferroptosis, 21 intersecting genes were obtained between 584 DEGs and 388 ferroptosis-related genes (Figure 3C). Correlation analysis suggested correlations between the expression of those genes in the GSE16561 dataset (Figure 3D). Prominently, the 21 genes fully differentiated IS cases from normal controls in the GSE16561 dataset as analyzed by PCA (Figures 3E,F), which was verified in the test set GSE37587 (Figures 3G,H). Taken together, the 21 ferroptosis-related genes were highly heterogeneous between normal and IS tissues, and their expression changes may play a vital role in the initiation and development of IS.
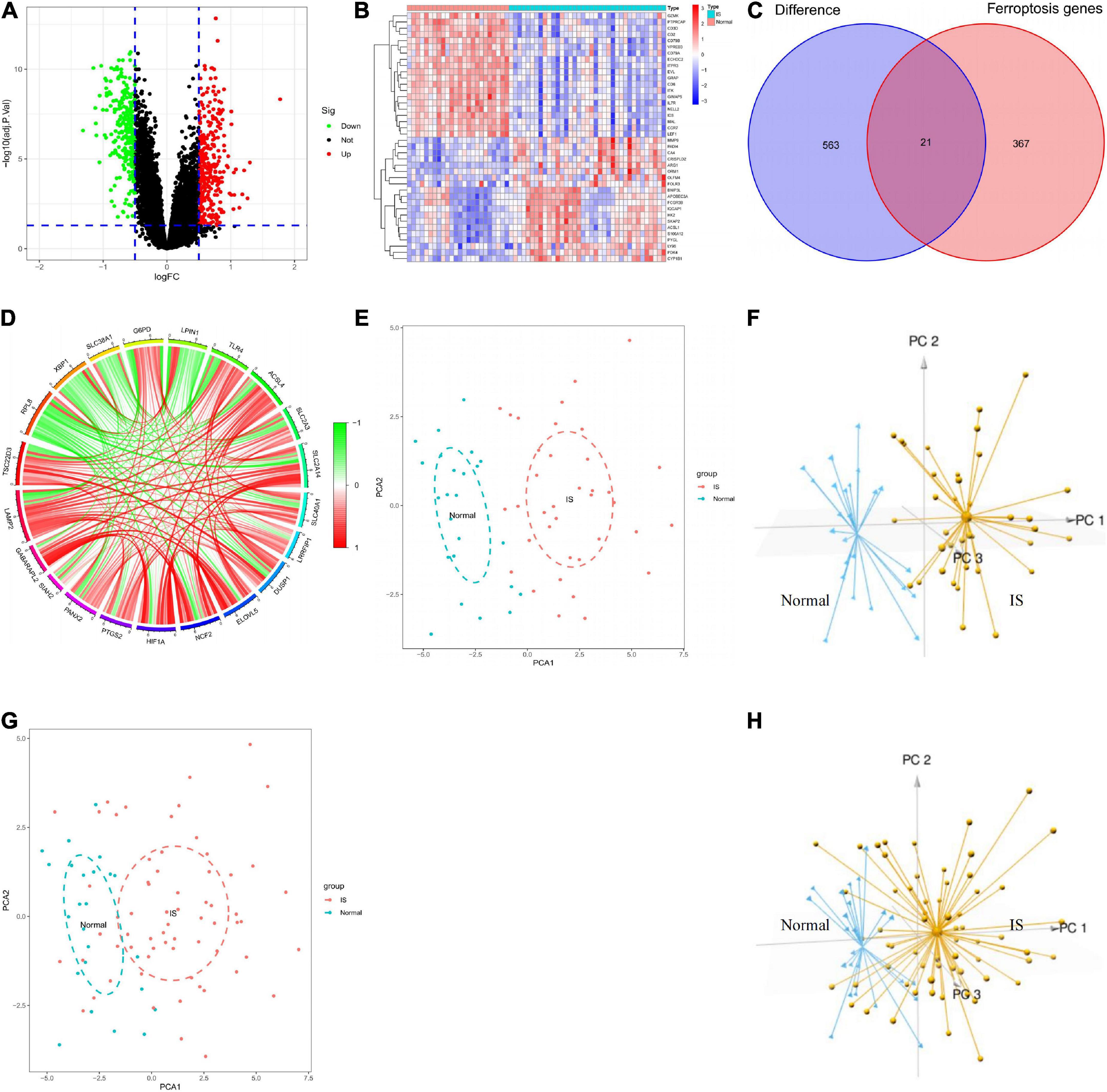
Figure 3. Analysis of differentially expressed genes. (A) Screening of DEGs shown by volcano plot. (B) Heatmap of the 40 genes expressed differently in IS samples compared to normal samples. (C) Venn diagram showing 21 ferroptosis-related DEGs. (D) Circle diagram showing the correlation of 21 ferroptosis-related DEGs. (E,F) 2D and 3D PCA plots showing PCA analysis based on 21 ferroptosis-related genes in GSE16561. (G,H) 2D and 3D PCA plots showing PCA analysis based on 21 ferroptosis-related genes in GSE37587. DEGs, differentially expressed genes; IS, ischemic stroke; PCA, principal components analysis.
Enrichment analysis
The box plot described the expression pattern of the 21 ferroptosis-related DEGs in IS and normal samples. In the two datasets, most of the 21 genes exhibited upregulation in IS tissues vs. normal tissues, except for LPIN1, RPL8, SLC38A1, and XBP1, which showed down-regulated expression (Figures 4A,B). Among 21 genes examined by enrichment analysis, monocarboxylic acid metabolism, iron ion responses, and ferroptosis were primarily enriched (Figure 4C).
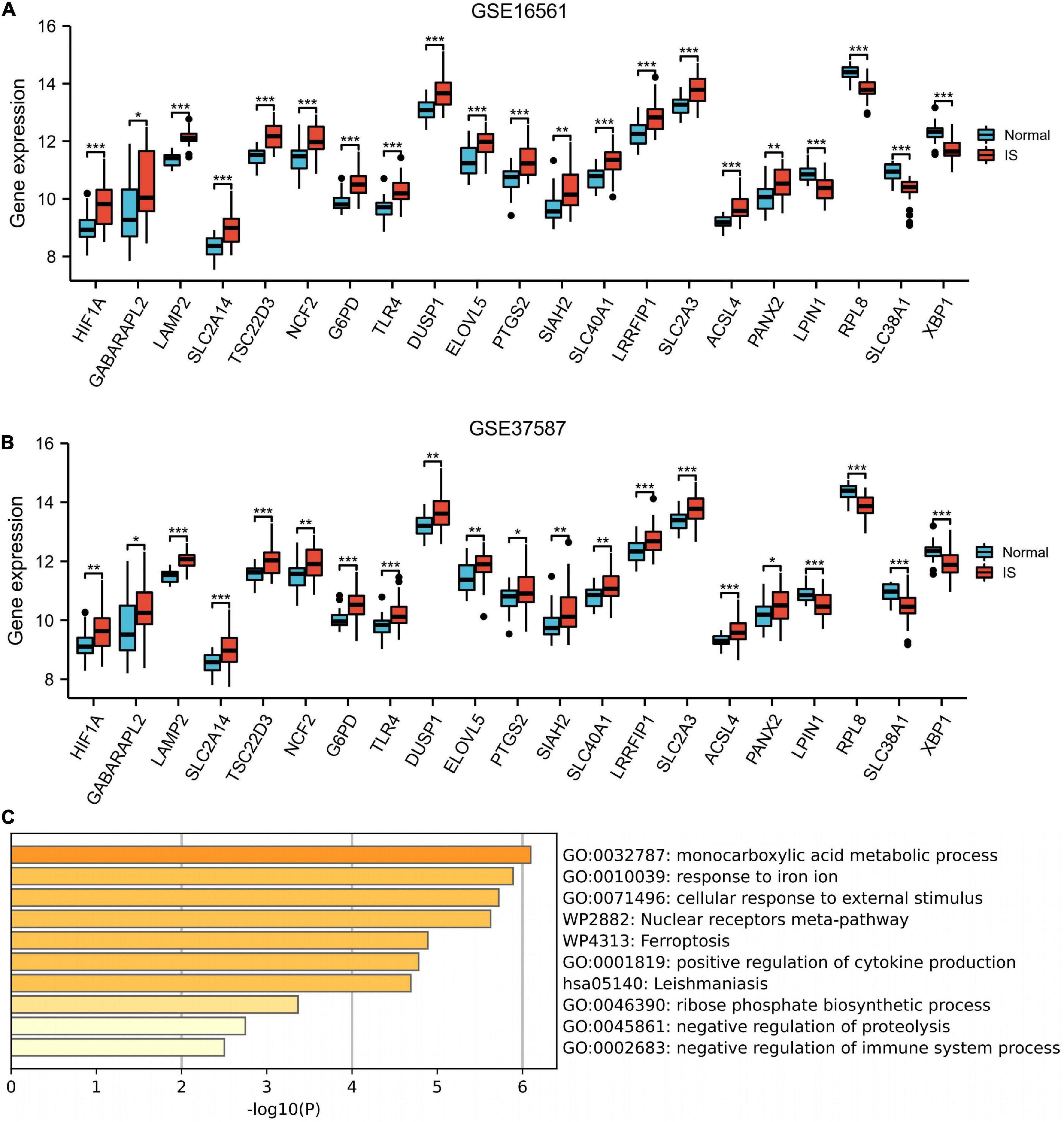
Figure 4. Enrichment analysis. Box plot described the expression pattern of the 21 ferroptosis-related genes between IS and normal samples in GSE16561 (A) and GSE37587 (B). (C) 21 ferroptosis-related genes enrichment analysis. IS, ischemic stroke. *p < 0.05, **p < 0.01, and ***p < 0.001.
Clinical correlation analysis
The expression levels of HIF1A, GABARAPL2, LAMP2, SLC2A14, NCF2, ELOVL5, ACSL4, and XBP1 in patients ≥78 years old were significantly higher than those in patients < 78 years old in both the GSE16561 and GSE37587 datasets (Figures 5A,B). In the GSE16561 cohort, as compared to female patients, male patients displayed remarkably higher expression of HIF1A, GABARAPL2, SLC2A1, NCF2, and ELOVL5, while lower expression of TLR4 and SLC40A1 (Figure 5C). In the test set GSE37587, higher expression of HIF1A, GABARAPL2, SLC2A14, and NCF2 was also observed in male patients vs. female patients (Figure 5D). Therefore, the expression of these ferroptosis-related genes was interrelated with the age and gender of IS patients.
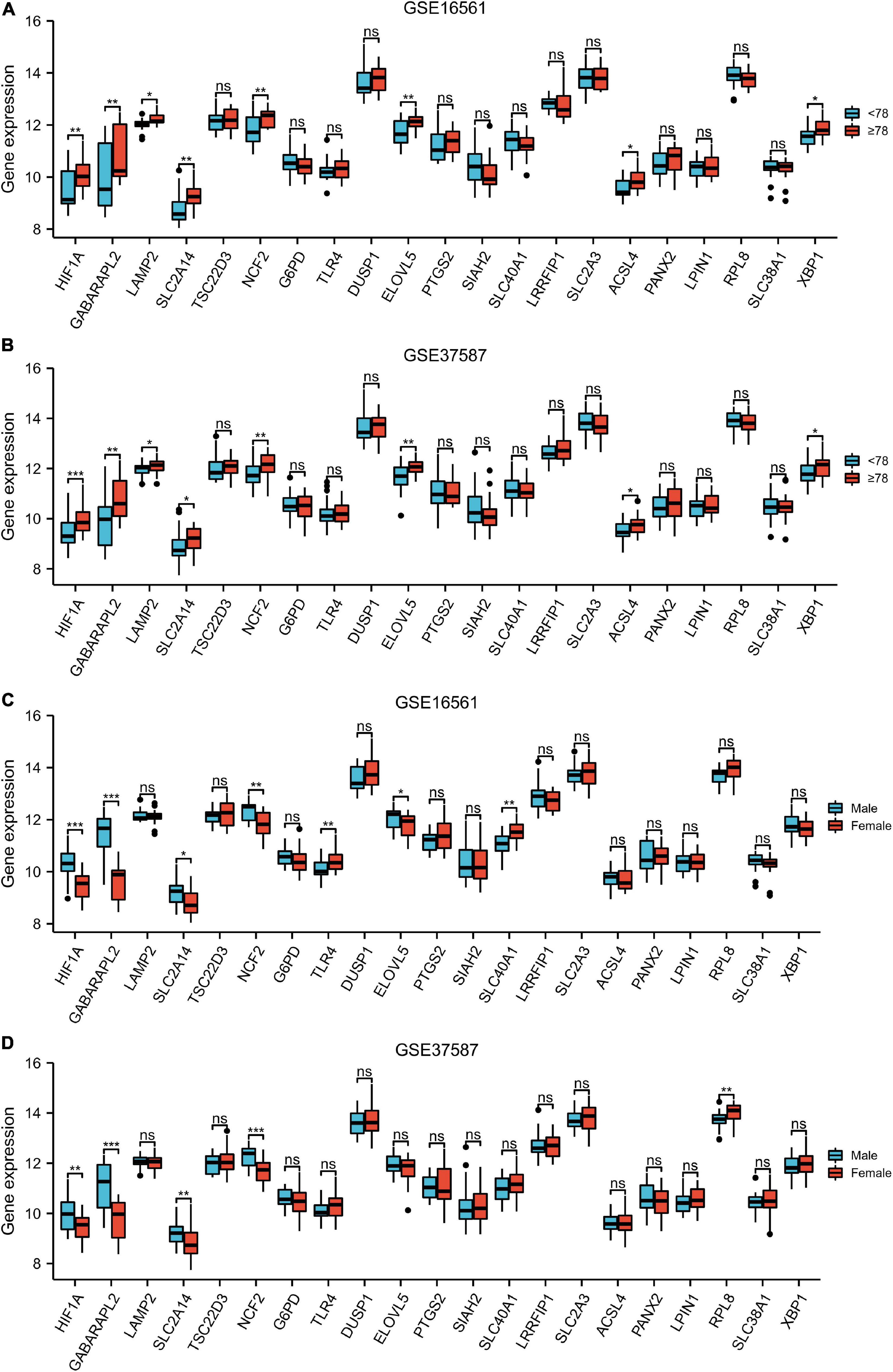
Figure 5. Clinical correlation analysis. Box plot showing the expression pattern of the 21 ferroptosis-related genes between <78 and ≥78 patients in GSE16561 (A) and GSE37587 (B). Box plot showing the expression pattern of the 21 ferroptosis-related genes between male and female patients in GSE16561 (C) and GSE37587 (D). ns: p ≥ 0.05, *p < 0.05, **p < 0.01, and ***p < 0.001.
Receiver operating feature analysis
The ROC curves of the GSE16561 dataset revealed excellent accuracy of LAMP2 (AUC = 0.98), TSC22D3 (AUC = 0.90), SLC38A1 (AUC = 0.89), and RPL8 (AUC = 0.89) in distinguishing between the outcomes of normal and IS groups (Figures 6A–C). The ROC curves of the test set GSE37587 manifested moderate accuracy of LAMP2 (AUC = 0.92), RPL8 (AUC = 0.85), SLC38A1 (AUC = 0.80), and TSC22D3 (AUC = 0.78) in terms of differentiating between the outcomes of normal and IS groups (Figures 6D–F). In the GSE16561 dataset, based on lasso regression, we constructed a model composed of seven genes (LAMP2, LPIN1, TLR4, SLC2A3, LRRFIP1, PANX2, and GABARAPL2) to distinguish healthy subjects from IS patients (Supplementary Figures 2A,B). The AUC of GSE16561 was 1.000 in the training set, and the values of GSE37587 and GSE58294 AUC in the verification set were 0.961 and 0.730, respectively (Supplementary Figures 2C–E). Overall, these ferroptosis-related genes had remarkable diagnostic significance for IS patients.
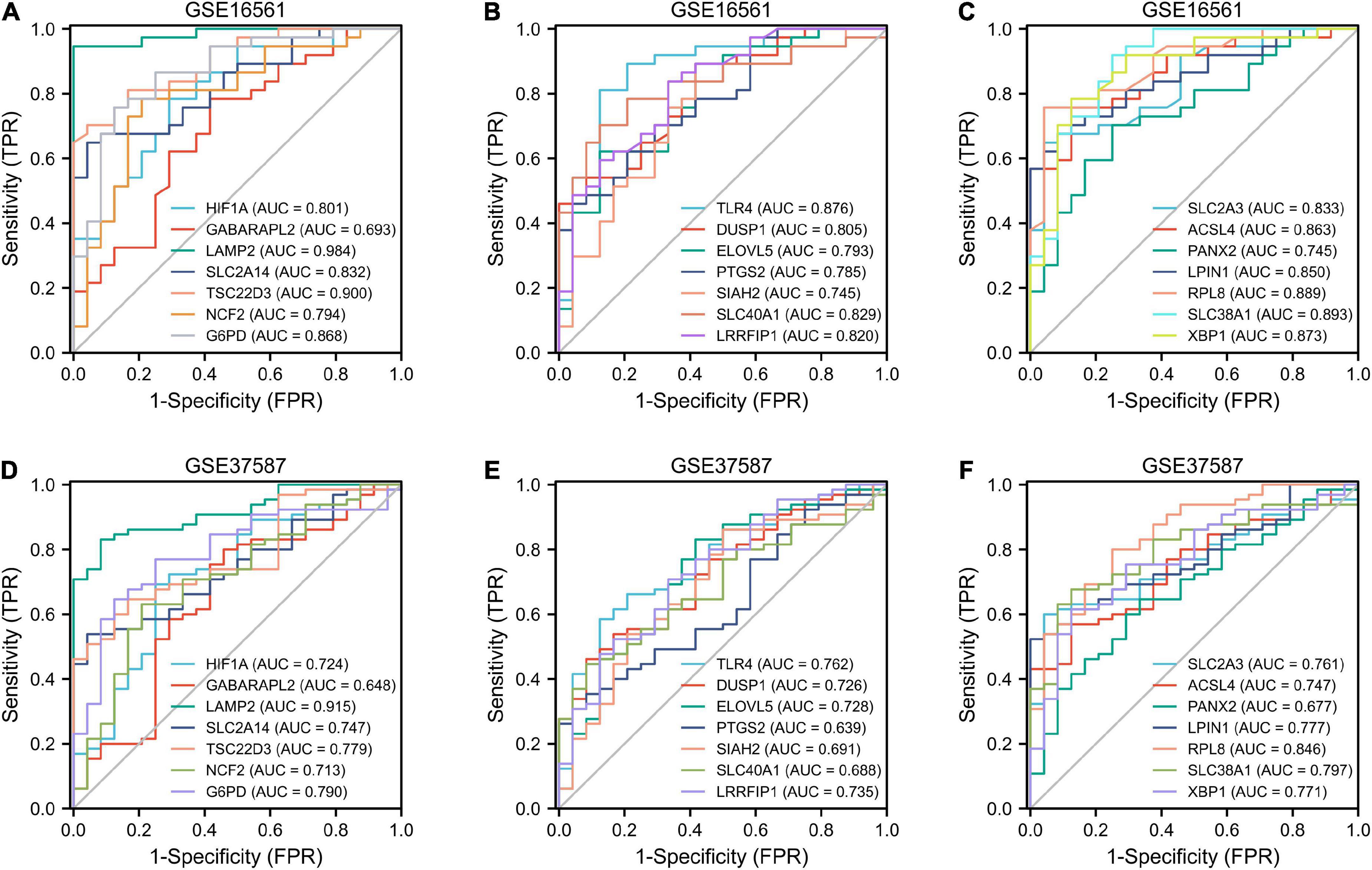
Figure 6. ROC analysis. Diagnostic ROC analysis of 21 ferroptosis-related genes in GSE16561 (A–C) and GSE37587 (D–F). ROC, receiver operating characteristic; AUC, area under curve; FPR, false positive rate; TPR, true positive rate.
Transcript factor, upstream miRNA, and drug prediction
The transcription factors, upstream miRNAs, and related drugs of the 21 ferroptosis-related genes were predicted via the Enrichr platform. STAT6, IRF8, and HMGA1 were the main transcription factors retrieved from the TRRUST database (Table 1). Hsa-miR-548ag, hsa-miR-329-5p and hsa-miR-625-3p were the major upstream miRNAs according to the miRTarBase database (Table 2). Vandetanib, FERRIC CITRATE, etc., were the primary drugs predicted from the DSigDB database (Table 3). The identified transcription factors and miRNAs might be of prominent importance in the development of IS, and the predicted drugs can serve as potential drugs for IS.
Hub gene analysis
Based on the 21 ferroptosis-related genes, a PPI network was generated based on the STRING online database (Figure 7A). Hub genes were analyzed using the plug-in cytoNCA. The top 11 hub genes were identified by calculating the Betweenness, Closeness, and Degree (Figures 7B,C). Based on enrichment analysis, the top 11 hub genes were highly enriched in fatty acid metabolism, hypoxia response, and angiogenesis (Figure 7D).
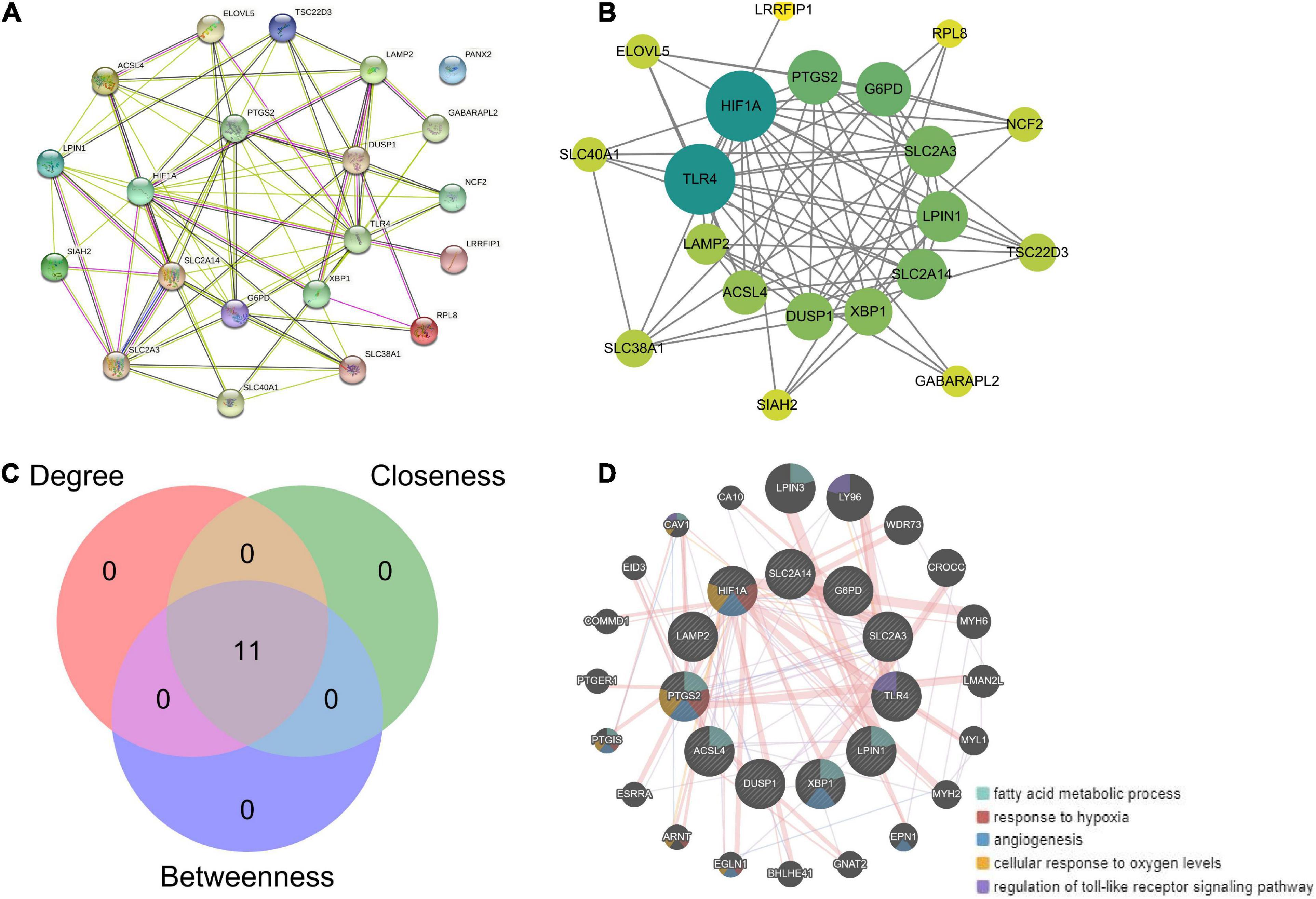
Figure 7. Hub gene analysis. (A) Based on the 21 ferroptosis-related genes, a PPI network was generated based on the STRING online database. (B) Hub genes were analyzed using the plug-in cytoNCA. (C) The top 11 hub genes were identified by calculating the betweenness, closeness, and degree. (D) Enrichment analysis of 11 hub genes by GeneMANIA online database.
Clustering analysis based on hub genes
We performed an unsupervised consistency clustering analysis on IS samples based on 11 hub genes (Supplementary Figures 3A–C). According to the average consistency evaluation within the cluster group, we choose the number of clusters as K = 2 (Figures 8A,B). We named these two subtypes C1 and C2, respectively. PCA analysis revealed significant differences between subtypes (Figure 8C). There was significant heterogeneity in the expression of 11 hub genes between subtypes (Figure 8D), and there was some association between subtypes and the age and sex of IS patients (Figures 8E,F).
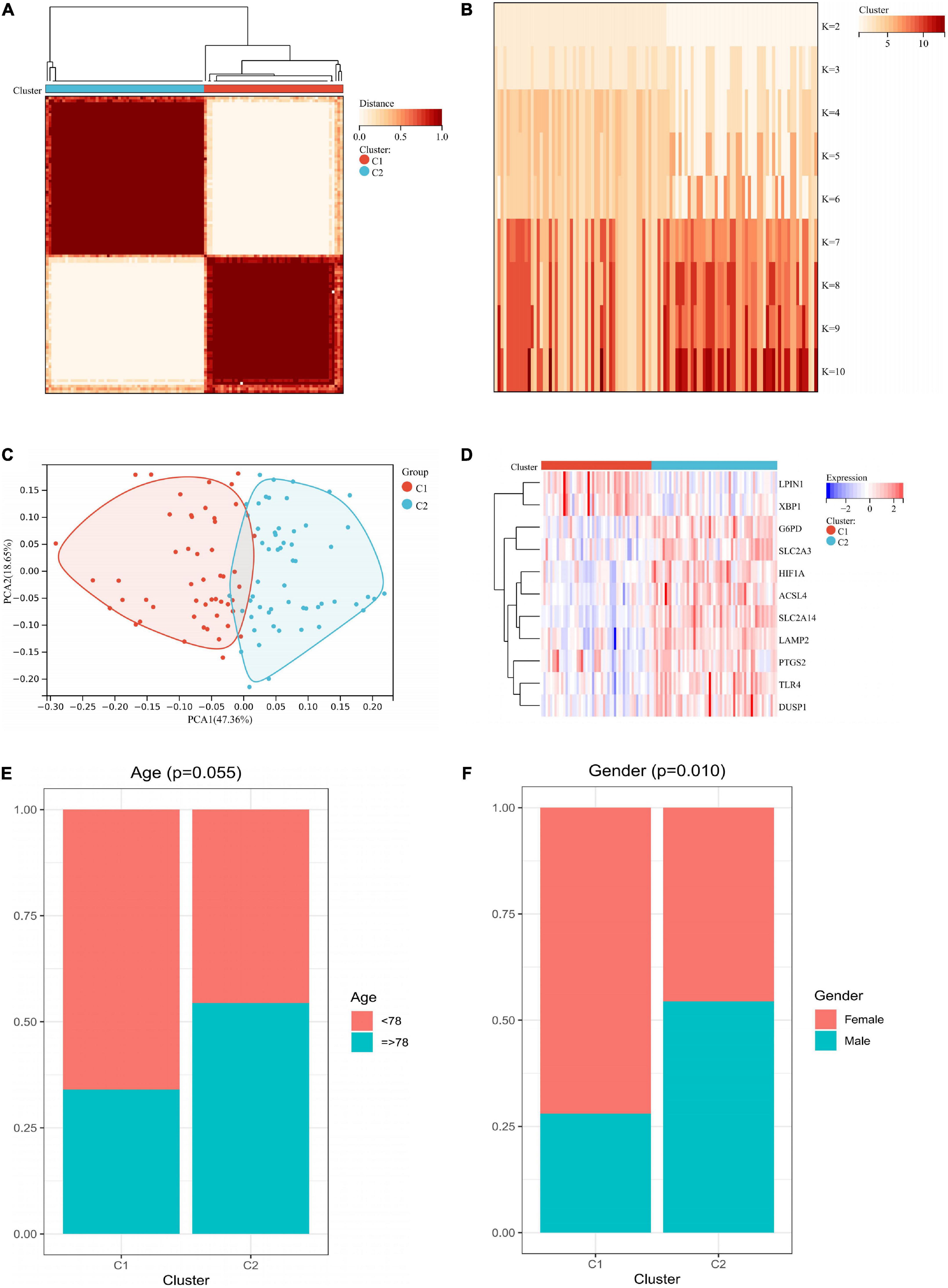
Figure 8. Unsupervised clustering of 11 hub genes. (A) Consensus matrix heatmap when k = 2. (B) Tracking plot showing the sample classification when k = 2–10. (C) PCA plots showing a remarkable difference in transcriptome between two subtypes. (D) Heatmap showing the expression of 11 hub genes in two subtypes. (E) Age ratio distribution in the two subtypes. (F) Gender ratio distribution in the two subtypes. PCA, principal components analysis.
Characteristics of immune microenvironment in different subtypes
Figure 9A showed that most of the hub genes were expressed at higher levels in C1 subtype than in C2. Most of the immune checkpoints and HLA genes were significantly upregulated in C1 compared to C2 subtype (Figures 9B,C). The C2 subtype was more immunoactive (p53 pathway, complement, IL6-JAK-STAT3 signaling, TNFA signaling via NFKB, chemokine signaling pathway, etc.) than the C1 subtype, as shown in Supplementary Figure 4. Based on the results of the ssGSEA algorithm, most immune cell infiltration levels differed significantly between C1 and C2 subtypes (Figure 9D). And the CIBERSORT algorithm analysis revealed a significant difference between C1 and C2 subtypes in terms of T cell infiltration (Supplementary Figures 5A,B).
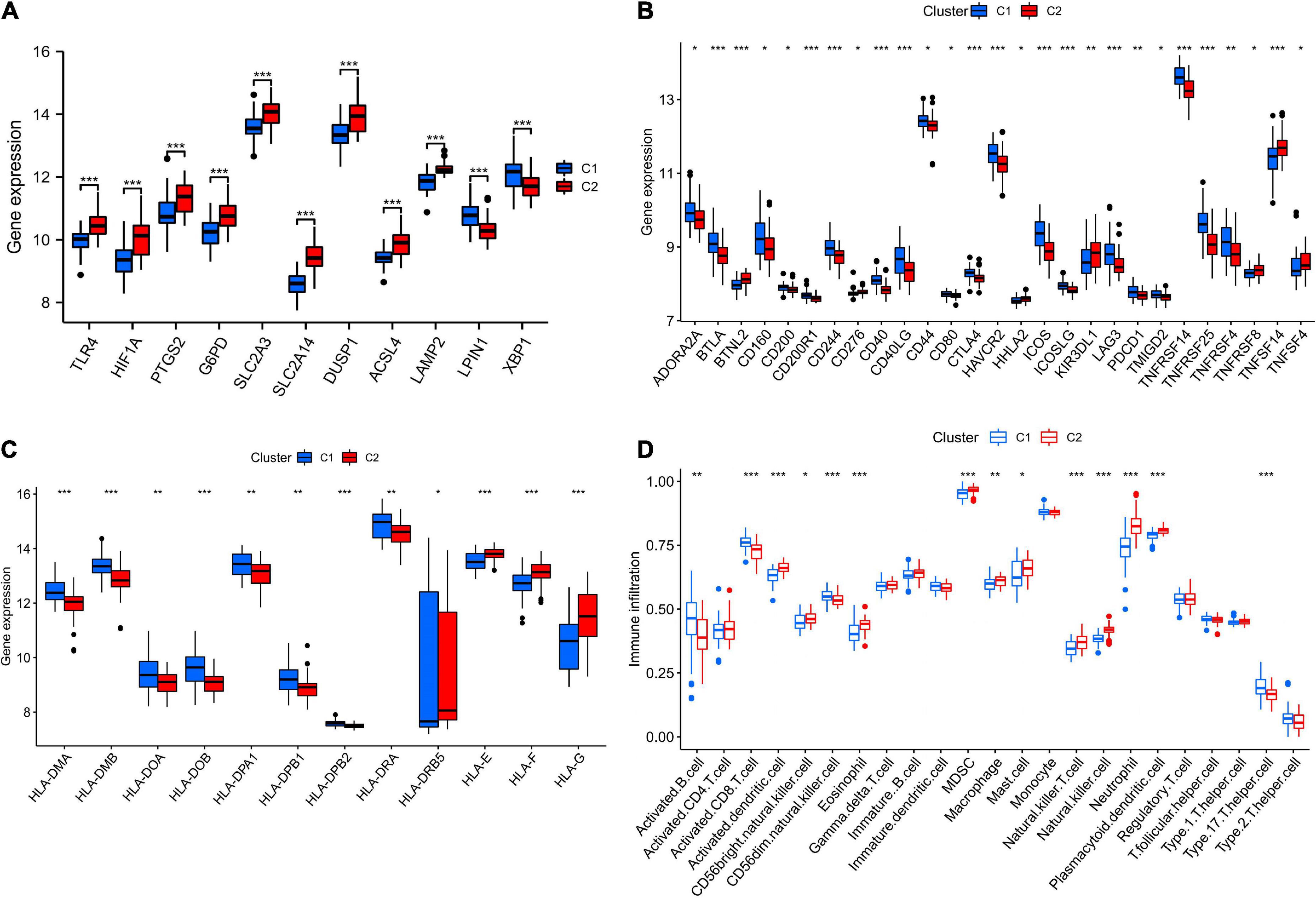
Figure 9. The features of the immunological microenvironment differ between subtypes. Box plots showing that there were differences in hub genes (A), immune checkpoints (B), HLA genes (C), and immune cell infiltration (D) between the two subtypes. *p < 0.05, **p < 0.01, and ***p < 0.001.
Functional enrichment analysis between different subtypes
Two hundred seventy-two DEGs were obtained from Cluster 1 and Cluster 2, of which 49 DEGs were upregulated in Cluster 1 and 223 DEGs were downregulated in Cluster 1 (Figure 10A). According to the heat map, two molecular subtypes could be distinguished by these DEGs (Figure 10B). Afterward, we analyzed DEGs among the two subtypes. As illustrated in Figures 10C,D, these DEGs were mainly associated with immune responses (immune system process, cell activation, leukocyte activation, etc.). Based on KEGG enrichment analysis, these DEGs were mainly associated with ribosomes, osteoclast differentiation, and autophagy (Figures 10E,F).
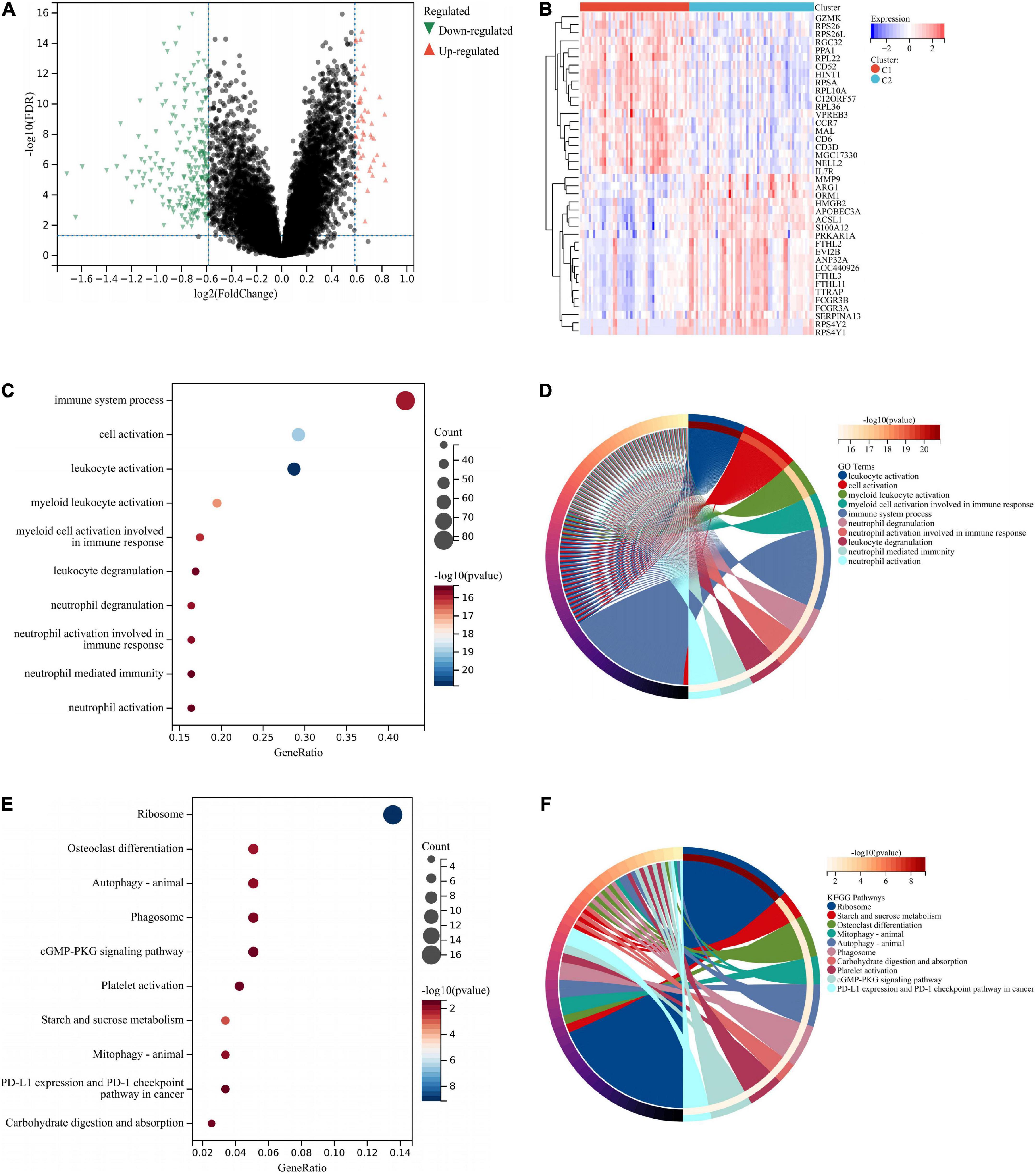
Figure 10. Functional analysis between two different subtypes. The DEGs were shown by volcano plot (A) and heat map (B) between two subtypes. (C) GO enrichment analysis was performed on the DEGs. GO terms are represented on the y-axis, gene ratios are shown on the x-axis, circle sizes refer to gene numbers, and colors represent p-values. (D) GO enrichment analysis of the DEGs. Different colors represent various significant GO terms and related enriched genes. (E) KEGG pathway analysis was performed on the DEGs. The y-axis represents different pathways, gene ratios enriched in relative pathways by the x-axis, circles represent gene numbers, and colors represent p-values. (F) KEGG pathway analysis of the DEGs. Different colors represent various significant pathways and related enriched genes. DEGs, differentially expressed genes; KEGG, Kyoto Encyclopedia of Genes and Genomes; GO, Gene Ontology.
External dataset validation
At the same time, we use GSE58294 to verify our analysis results and get similar results. First, we normalized the GSE58294 data set (Supplementary Figures 6A–C). Most 21 genes exhibited upregulation in IS tissues vs. normal tissues (Supplementary Figure 6D). The ROC curves also revealed excellent accuracy of LAMP2 (AUC = 0.94), TSC22D3 (AUC = 0.80), and SLC38A1 (AUC = 0.82) in distinguishing between the outcomes of normal and IS groups (Supplementary Figures 6E–G). We performed an unsupervised consistency clustering analysis on IS samples based on 11 hub genes and divided IS samples into two subtypes, C1 and C2 (Supplementary Figure 7A). Most of the immune checkpoints and HLA genes were significantly upregulated in C1 compared to C2 subtype (Supplementary Figures 7B,C). Most immune cell infiltration levels differed significantly between C1 and C2 subtypes (Supplementary Figure 7D). The C2 subtype was more immunoactive (complement, B cell receptor signaling pathway, VEGF signaling pathway, chemokine signaling pathway, etc.) than the C1 subtype (Supplementary Figure 7E).
Discussion
Ferroptosis is a unique type of programmed cell death involved in metabolism, redox biology, and various diseases (33), such as degenerative disorders, carcinogenesis, stroke, and traumatic brain injury. A recent study demonstrated that ferroptosis is critical for the progress of cerebral stroke (34). By understanding the association between ferroptosis and IS, new biomarkers and approaches to diagnosis and treatment can be developed.
In the present study, using GSE16561 dataset, 21 ferroptosis-related DEGs were identified. IS-related pathways like monocarboxylic acid metabolism, iron ion response, and ferroptosis were enriched. Monocarboxylic acids such as lactic acid (35), pyruvate (36), and ketone body (37) are closely related to IS. ACSL4 was reported to be a potential therapeutic target for IS, as it could aggravate IS by promoting ferroptosis (38). Therefore, the 21 ferroptosis-related DEGs identified in this study may contribute significantly to IS through these pathways.
Furthermore, clinical correlation analysis indicated that the expression of DEGs was related to patients’ age and gender. It has been long recognized that stroke incidence is higher in men than in women globally (39). Moreover, men have a higher age-adjusted incidence of stroke than women (40).
Further analysis revealed that LAMP2, RPL8, and SLC38A exhibited excellent diagnostic performance for IS patients in both the GSE16561 and GSE37587 datasets. Tao et al. (41) revealed that miR-207 mediated the ischemic injury and spontaneous recovery by participating in the lysosome pathway via regulating LAMP2. After intracerebral hemorrhage, human brain RPL8 mRNA expression increased, suggesting it may be a therapeutic target (42). To conclude, the 21 ferroptosis-related DEGs might be critical to IS.
The transcription factors, upstream miRNA, and drugs that correspond to the 21 ferroptosis-related DEGs were also confirmed in this study. The transcription factors identified mainly were STAT6, IRF8, and HMGA1. STAT6/Arg1 promoted microglia/macrophage efferocytosis and inflammation resolution in stroke mice (43); IRF8 protected against cerebral ischemic-reperfusion injury (44); has-miR-196a alleviated ischemic brain injury in mice by directly targeting HMGA1 (45). The three miRNAs identified were hsa-miR-548ag, hsa-miR-329-5p, and hsa-miR-625-3p. The expression of hsa-miR-625-3p was correlated with cholesterol levels (46) and hsa-miR-625-3p was reported to be interrelated with cerebral infarction (47). Given these findings, the identified transcription factors and miRNAs were essential to the ferroptosis dysfunction in IS. Among the drugs, vandetanib could be used to treat thyroid and non-small cell lung cancer (48), and it might serve as a potential drug for IS.
Protein-protein interaction analysis identified 11 hub genes out of the 21 ferroptosis-related genes, which were majorly enriched in fatty acid metabolic process, response to hypoxia, and angiogenesis. Research showed that the fatty acid metabolic process was closely correlated with stroke (49). Hypoxia could induce IS (50), while angiogenesis-associated factors could act as biomarkers for IS patients (51). Therefore, the 11 hub genes are presumably of vital importance in IS.
In this study, using consistent clustering, we identified two subtypes (C1 and C2) in IS samples based on 11 ferroptosis-related genes. C1 contained 50 samples, and C2 contained 57 samples. Significant heterogeneity between the two subgroups was confirmed by immunoassay and enrichment analysis. At the same time, we use GSE58294 to verify our analysis results and get similar results. According to many studies, ferroptosis plays a vital role in immunity (52, 53). It is thought that ferroptotic cells activate innate immunity and release pro-inflammatory factors in various diseases, attracting many different immune cells to the area (54). In IS, BBB breaks down, allowing immune cells to flood into the central nervous system. Our findings suggested that NK and mast cells infiltrated less in C1 than in C2. Kong et al. (55) reported that the number of NK cells was reduced in IS patients. The mast cells contributed to the development of IS by speeding up BBB disruption and magnifying neuroinflammation by releasing cytokines (56). Two limitations in this study warrant mention. The ferroptosis-related DEGs with significance in IS might not be comprehensively included. Moreover, validations are required in further in vivo and in vitro experiments.
Conclusion
The current study identified 21 ferroptosis-related DEGs in IS, which were pertinent to the age and gender of IS patients and had an excellent diagnostic performance. Vandetanib, FERRIC CITRATE, etc., were identified as potential drugs for IS. In addition, we proposed a molecular classification based on ferroptosis-related genes, namely C1 and C2 subtypes in IS. In conclusion, our findings may help to design immunotherapies for IS patients.
Data availability statement
The datasets presented in this study can be found in online repositories. The names of the repository/repositories and accession number(s) can be found in the article/Supplementary material.
Author contributions
YJZ, BL, and ZP designed the study, performed data analysis, and wrote the manuscript. YSZ, RY, XH, LZ, and XZ gathered clinical and expression data. All authors contributed to the article and approved the submitted version.
Conflict of interest
The authors declare that the research was conducted in the absence of any commercial or financial relationships that could be construed as a potential conflict of interest.
Publisher’s note
All claims expressed in this article are solely those of the authors and do not necessarily represent those of their affiliated organizations, or those of the publisher, the editors and the reviewers. Any product that may be evaluated in this article, or claim that may be made by its manufacturer, is not guaranteed or endorsed by the publisher.
Supplementary material
The Supplementary Material for this article can be found online at: https://www.frontiersin.org/articles/10.3389/fnut.2022.1010918/full#supplementary-material
Supplementary Figure 1 | GSE37587 data set preprocessing. (A) Box plots showing gene expression levels between different samples after normalization. 2D and 3D PCA plots demonstrated the distribution of samples before (B,C) and after (D,E) pretreatment. PCA, principal components analysis.
Supplementary Figure 2 | Construct a diagnostic model of 21 IS-associated ferroptosis genes. (A) Least absolute shrinkage and selection operator (LASSO) coefficient profiles of 21 IS-related ferroptosis genes. (B) Plots of the 10-fold cross-validation error rates. (C–E) The model’s discrimination ability for healthy and IS samples was analyzed by ROC curve and evaluated by AUC value. IS, ischemic stroke; ROC, receiver operating characteristic; AUC, area under curve; FPR, false positive rate; TPR, true positive rate.
Supplementary Figure 3 | Cluster parameter analysis. (A) Cumulative distribution curve when k = 2–10. (B) Relative alterations in the area under CDF curve. (C) Sample clustering consistency when k = 2–10.
Supplementary Figure 4 | Two subtypes differ in biological function. Heatmap showing the enrichment levels of Hallmark (A) and KEGG (B) gene sets in two subtypes.
Supplementary Figure 5 | CIBERSORT to assess immune cell infiltration. (A) Bar plot showing the proportion of 22 immunocytes in two subtypes. (B) Violin plot showing the ratio of immune cells between two subtypes.
Supplementary Figure 6 | The expression level and diagnostic value of 21 IS-associated ferroptosis genes were verified in the GSE58294 data set. (A) Box plot showing the gene expression level between different samples after normalization. (B,C) 2D and 3D PCA plots demonstrated the distribution of samples after pretreatment. (D) Box plot described the expression pattern of the 21 ferroptosis-related genes between IS and normal samples. (E–G) Diagnostic ROC analysis of 21 ferroptosis-related genes in GSE58294. PCA, principal components analysis; IS, ischemic stroke; ROC, receiver operating characteristic; AUC, area under curve; FPR, false positive rate; TPR, true positive rate. Ns: p ≥ 0.05, *p < 0.05, **p < 0.01, and ***p < 0.001.
Supplementary Figure 7 | The unsupervised cluster analysis of 11 hub genes was verified in the GSE58294 data set. (A) Based on 11 hub gene expression levels, IS samples were divided into two subtypes, C1 and C2. Box plots showing that there were differences in immune checkpoints (B), HLA genes (C), and immune cell infiltration (D) between the two subtypes. (E) Heatmap showing the enrichment levels of KEGG gene sets in two subtypes. KEGG, Kyoto Encyclopedia of Genes and Genomes. *p < 0.05, **p < 0.01, and ***p < 0.001.
Footnotes
- ^ http://www.ncbi.nlm.nih.gov/geo
- ^ http://www.zhounan.org/ferrdb/current/
- ^ https://bioconductor.org/packages/sva/
- ^ https://bioconductor.org/packages/limma/
- ^ https://CRAN.R-project.org/package=pheatmap
- ^ https://CRAN.R-project.org/package=venn
- ^ https://CRAN.R-project.org/package=corrplot
- ^ https://CRAN.R-project.org/package=circlize
- ^ http://amp.pharm.mssm.edu/Enrichr/
- ^ https://CRAN.R-project.org/package=pROC
- ^ https://CRAN.R-project.org/package=ggplot2
- ^ http://genemania.org/
References
1. Chehaibi K, Trabelsi I, Mahdouani K, Slimane MN. Correlation of oxidative stress parameters and inflammatory markers in ischemic stroke patients. J Stroke Cerebrovasc Dis. (2016) 25:2585–93. doi: 10.1016/j.jstrokecerebrovasdis.2016.06.042
2. Qi Z, Zhao Y, Su Y, Cao B, Yang JJ, Xing Q. Serum extracellular vesicle-derived miR-124-3p as a diagnostic and predictive marker for early-stage acute ischemic stroke. Front Mol Biosci. (2021) 8:685088. doi: 10.3389/fmolb.2021.685088
3. Tang X, Fang M, Cheng R, Zhang Z, Wang Y, Shen C, et al. Iron-Deficiency and estrogen are associated with ischemic stroke by up-regulating transferrin to induce hypercoagulability. Circ Res. (2020) 127:651–63. doi: 10.1161/CIRCRESAHA.119.316453
4. Li J, Cao F, Yin HL, Huang ZJ, Lin ZT, Mao N, et al. Ferroptosis: past, present and future. Cell Death Dis. (2020) 11:88. doi: 10.1038/s41419-020-2298-2
5. Xu T, Ding W, Ji X, Ao X, Liu Y, Yu W, et al. Molecular mechanisms of ferroptosis and its role in cancer therapy. J Cell Mol Med. (2019) 23:4900–12. doi: 10.1111/jcmm.14511
6. Weiland A, Wang Y, Wu W, Lan X, Han X, Li Q, et al. Ferroptosis and Its role in diverse brain diseases. Mol Neurobiol. (2019) 56:4880–93. doi: 10.1007/s12035-018-1403-3
7. Du X, Zhang Y. Integrated analysis of immunity- and ferroptosis-related biomarker signatures to improve the prognosis prediction of hepatocellular carcinoma. Front Genet. (2020) 11:614888. doi: 10.3389/fgene.2020.614888
8. Rui T, Li Q, Song S, Gao Y, Luo C. Ferroptosis-relevant mechanisms and biomarkers for therapeutic interventions in traumatic brain injury. Histol Histopathol. (2020) 35:1105–13. doi: 10.14670/HH-18-229
9. Shi K, Wood K, Shi FD, Wang X, Liu Q. Stroke-induced immunosuppression and poststroke infection. Stroke Vasc Neurol. (2018) 3:34–41. doi: 10.1136/svn-2017-000123
10. Wong CH, Jenne CN, Lee WY, Leger C, Kubes P. Functional innervation of hepatic iNKT cells is immunosuppressive following stroke. Science. (2011) 334:101–5. doi: 10.1126/science.1210301
11. Fu Y, Liu Q, Anrather J, Shi FD. Immune interventions in stroke. Nat Rev Neurol. (2015) 11:524–35. doi: 10.1038/nrneurol.2015.144
12. Amantea D, Bagetta G. Drug repurposing for immune modulation in acute ischemic stroke. Curr Opin Pharmacol. (2016) 26:124–30. doi: 10.1016/j.coph.2015.11.006
13. Barrett T, Wilhite SE, Ledoux P, Evangelista C, Kim IF, Tomashevsky M, et al. NCBI GEO: archive for functional genomics data sets–update. Nucleic Acids Res. (2013) 41:D991–5. doi: 10.1093/nar/gks1193
14. Barr TL, Conley Y, Ding J, Dillman A, Warach S, Singleton A, et al. Genomic biomarkers and cellular pathways of ischemic stroke by RNA gene expression profiling. Neurology. (2010) 75:1009–14. doi: 10.1212/WNL.0b013e3181f2b37f
15. Barr TL, VanGilder R, Rellick S, Brooks SD, Doll DN, Lucke-Wold AN, et al. A genomic profile of the immune response to stroke with implications for stroke recovery. Biol Res Nurs. (2015) 17:248–56. doi: 10.1177/1099800414546492
16. Stamova B, Jickling GC, Ander BP, Zhan X, Liu D, Turner R, et al. Gene expression in peripheral immune cells following cardioembolic stroke is sexually dimorphic. PLoS One. (2014) 9:e102550. doi: 10.1371/journal.pone.0102550
17. Zhou N, Bao J. FerrDb: a manually curated resource for regulators and markers of ferroptosis and ferroptosis-disease associations. Database (Oxford). (2020) 2020:baaa021. doi: 10.1093/database/baaa021
18. Ritchie ME, Phipson B, Wu D, Hu Y, Law CW, Shi W, et al. limma powers differential expression analyses for RNA-sequencing and microarray studies. Nucleic Acids Res. (2015) 43:e47. doi: 10.1093/nar/gkv007
19. Gu Z, Gu L, Eils R, Schlesner M, Brors B. circlize Implements and enhances circular visualization in R. Bioinformatics. (2014) 30:2811–2. doi: 10.1093/bioinformatics/btu393
20. Zhou Y, Zhou B, Pache L, Chang M, Khodabakhshi AH, Tanaseichuk O, et al. Metascape provides a biologist-oriented resource for the analysis of systems-level datasets. Nat Commun. (2019) 10:1523. doi: 10.1038/s41467-019-09234-6
21. Kuleshov MV, Jones MR, Rouillard AD, Fernandez NF, Duan Q, Wang Z, et al. Enrichr: a comprehensive gene set enrichment analysis web server 2016 update. Nucleic Acids Res. (2016) 44:W90–7. doi: 10.1093/nar/gkw377
22. Robin X, Turck N, Hainard A, Tiberti N, Lisacek F, Sanchez JC, et al. pROC: an open-source package for R and S+ to analyze and compare ROC curves. BMC Bioinform. (2011) 12:77. doi: 10.1186/1471-2105-12-77
23. Villanueva RAM, Chen ZJ. ggplot2: Elegant Graphics for Data Analysis, 2nd edition. Measurement-Interdisciplinary Res Perspect. (2019) 17:160–7. doi: 10.1080/15366367.2019.1565254
24. Szklarczyk D, Franceschini A, Wyder S, Forslund K, Heller D, Huerta-Cepas J, et al. STRING v10: protein-protein interaction networks, integrated over the tree of life. Nucleic Acids Res. (2015) 43:D447–52. doi: 10.1093/nar/gku1003
25. Tang Y, Li M, Wang J, Pan Y, Wu FX. CytoNCA: a cytoscape plugin for centrality analysis and evaluation of protein interaction networks. Biosystems. (2015) 127:67–72. doi: 10.1016/j.biosystems.2014.11.005
26. Shannon P, Markiel A, Ozier O, Baliga NS, Wang JT, Ramage D, et al. Cytoscape: a software environment for integrated models of biomolecular interaction networks. Genome Res. (2003) 13:2498–504. doi: 10.1101/gr.1239303
27. Warde-Farley D, Donaldson SL, Comes O, Zuberi K, Badrawi R, Chao P, et al. The GeneMANIA prediction server: biological network integration for gene prioritization and predicting gene function. Nucleic Acids Res. (2010) 38:W214–20. doi: 10.1093/nar/gkq537
28. Wilkerson MD, Hayes DN. ConsensusClusterPlus: a class discovery tool with confidence assessments and item tracking. Bioinformatics. (2010) 26:1572–3. doi: 10.1093/bioinformatics/btq170
29. Newman AM, Steen CB, Liu CL, Gentles AJ, Chaudhuri AA, Scherer F, et al. Determining cell type abundance and expression from bulk tissues with digital cytometry. Nat Biotechnol. (2019) 37:773–82. doi: 10.1038/s41587-019-0114-2
30. Hanzelmann S, Castelo R, Guinney J. GSVA: gene set variation analysis for microarray and RNA-seq data. BMC Bioinform. (2013) 14:7. doi: 10.1186/1471-2105-14-7
31. Liberzon A, Subramanian A, Pinchback R, Thorvaldsdottir H, Tamayo P, Mesirov JP. Molecular signatures database (MSigDB) 3.0. Bioinformatics. (2011) 27:1739–40. doi: 10.1093/bioinformatics/btr260
32. Yu G, Wang LG, Han Y, He QY. clusterProfiler: an R package for comparing biological themes among gene clusters. OMICS. (2012) 16:284–7. doi: 10.1089/omi.2011.0118
33. Stockwell BR, Friedmann Angeli JP, Bayir H, Bush AI, Conrad M, Dixon SJ, et al. Ferroptosis: A regulated cell death nexus linking metabolism, redox biology, and disease. Cell. (2017) 171:273–85. doi: 10.1016/j.cell.2017.09.021
34. Zhang Y, Lu X, Tai B, Li W, Li T. Ferroptosis and its multifaceted roles in cerebral stroke. Front Cell Neurosci. (2021) 15:615372. doi: 10.3389/fncel.2021.615372
35. Pilarczyk M, Krasinska-Czerlunczakiewicz H, Stelmasiak Z. [Evaluation of lactic acid levels in blood of patients with ischemic stroke in the earliest stage of the disease]. Neurol Neurochir Pol. (1999) 32(Suppl 6):109–11.
36. Krasinska-Czerlunczakiewicz H, Pilarczyk M, Stelmasiak Z. [Evaluation of pyruvic acid concentration in blood of patients with ischemic stroke in the earliest stage of the disease]. Neurol Neurochir Pol. (1999) 32(Suppl 6): 113–5.
37. You S, Xu J, Ou Z, Zhong C, Han Q, Chen J, et al. Prognostic significance of urinary protein and urinary ketone bodies in acute ischemic stroke. Nutr Metab Cardiovasc Dis. (2021) 31:3152–60. doi: 10.1016/j.numecd.2021.07.010
38. Cui Y, Zhang Y, Zhao X, Shao L, Liu G, Sun C, et al. ACSL4 exacerbates ischemic stroke by promoting ferroptosis-induced brain injury and neuroinflammation. Brain Behav Immun. (2021) 93:312–21. doi: 10.1016/j.bbi.2021.01.003
39. Bushnell CD, Chaturvedi S, Gage KR, Herson PS, Hurn PD, Jimenez MC, et al. Sex differences in stroke: Challenges and opportunities. J Cereb Blood Flow Metab. (2018) 38:2179–91. doi: 10.1177/0271678X18793324
40. Benjamin EJ, Virani SS, Callaway CW, Chamberlain AM, Chang AR, Cheng S, et al. Heart disease and stroke statistics-2018 update: A report from the american heart association. Circulation. (2018) 137:e67–492. doi: 10.1161/CIR.0000000000000558
41. Tao J, Liu W, Shang G, Zheng Y, Huang J, Lin R, et al. MiR-207/352 regulate lysosomal-associated membrane proteins and enzymes following ischemic stroke. Neuroscience. (2015) 305:1–14. doi: 10.1016/j.neuroscience.2015.07.064
42. Chen B, Chen Z, Liu M, Gao X, Cheng Y, Wei Y, et al. Inhibition of neuronal ferroptosis in the acute phase of intracerebral hemorrhage shows long-term cerebroprotective effects. Brain Res Bull. (2019) 153:122–32. doi: 10.1016/j.brainresbull.2019.08.013
43. Cai W, Dai X, Chen J, Zhao J, Xu M, Zhang L, et al. STAT6/Arg1 promotes microglia/macrophage efferocytosis and inflammation resolution in stroke mice. JCI Insight. (2019) 4:e131355. doi: 10.1172/jci.insight.131355
44. Xiang M, Wang L, Guo S, Lu YY, Lei H, Jiang DS, et al. Interferon regulatory factor 8 protects against cerebral ischaemic-reperfusion injury. J Neurochem. (2014) 129:988–1001. doi: 10.1111/jnc.12682
45. Zhang DL, Liu X, Wang Q, Li N, Wu SH, Wang C. Downregulation of microRNA-196a attenuates ischemic brain injury in rats by directly targeting HMGA1. Eur Rev Med Pharmacol Sci. (2019) 23:740–8. doi: 10.26355/eurrev_201901_16888
46. Raitoharju E, Seppala I, Oksala N, Lyytikainen LP, Raitakari O, Viikari J, et al. Blood microRNA profile associates with the levels of serum lipids and metabolites associated with glucose metabolism and insulin resistance and pinpoints pathways underlying metabolic syndrome: the cardiovascular risk in Young Finns Study. Mol Cell Endocrinol. (2014) 391:41–9. doi: 10.1016/j.mce.2014.04.013
47. Qi X, Lin H, Hou Y, Su X, Gao Y. Comprehensive Analysis of Potential miRNA-Target mRNA-Immunocyte Subtype Network in Cerebral Infarction. Eur Neurol. (2022) 85:148–61. doi: 10.1159/000518893
48. Morabito A, Piccirillo MC, Costanzo R, Sandomenico C, Carillio G, Daniele G, et al. Vandetanib: An overview of its clinical development in NSCLC and other tumors. Drugs Today (Barc). (2010) 46:683–98. doi: 10.1358/dot.2010.46.9.1516989
49. Zhang W, Chen R, Yang T, Xu N, Chen J, Gao Y, et al. Fatty acid transporting proteins: Roles in brain development, aging, and stroke. Prostaglandins Leukot Essent Fatty Acids. (2018) 136:35–45. doi: 10.1016/j.plefa.2017.04.004
50. Ranjbar Taklimie F, Gasterich N, Scheld M, Weiskirchen R, Beyer C, Clarner T, et al. Hypoxia induces astrocyte-derived lipocalin-2 in ischemic stroke. Int J Mol Sci. (2019) 2:1271. doi: 10.3390/ijms20061271
51. Alrafiah A, Alofi E, Almohaya Y, Hamami A, Qadah T, Almaghrabi S, et al. Angiogenesis biomarkers in ischemic stroke patients. J Inflamm Res. (2021) 14:4893–900. doi: 10.2147/JIR.S331868
52. Stockwell BR, Jiang X. A physiological function for ferroptosis in tumor suppression by the immune system. Cell Metab. (2019) 30:14–5. doi: 10.1016/j.cmet.2019.06.012
53. Xu X, Lin D, Tu S, Gao S, Shao A, Sheng J. Is ferroptosis a future direction in exploring cryptococcal meningitis? Front Immunol. (2021) 12:598601. doi: 10.3389/fimmu.2021.598601
54. Li W, Feng G, Gauthier JM, Lokshina I, Higashikubo R, Evans S, et al. Ferroptotic cell death and TLR4/Trif signaling initiate neutrophil recruitment after heart transplantation. J Clin Invest. (2019) 129:2293–304. doi: 10.1172/JCI126428
55. Kong Y, Li S, Cheng X, Ren H, Zhang B, Ma H, et al. Brain ischemia significantly alters microRNA expression in human peripheral blood natural killer cells. Front Immunol. (2020) 11:759. doi: 10.3389/fimmu.2020.00759
Keywords: ferroptosis, ischemic stroke, biomarkers, immune microenvironment, subtypes
Citation: Zhang Y, Zhang Y, Yao R, He X, Zhao L, Zuo X, Lu B and Pang Z (2022) Ferroptosis-related differentially expressed genes serve as new biomarkers in ischemic stroke and identification of therapeutic drugs. Front. Nutr. 9:1010918. doi: 10.3389/fnut.2022.1010918
Received: 12 September 2022; Accepted: 26 October 2022;
Published: 10 November 2022.
Edited by:
Yulong Li, University of Nebraska Medical Center, United StatesReviewed by:
Xiaodong Chen, Huazhong Agricultural University, ChinaHuiyin Tu, Zhengzhou University, China
Copyright © 2022 Zhang, Zhang, Yao, He, Zhao, Zuo, Lu and Pang. This is an open-access article distributed under the terms of the Creative Commons Attribution License (CC BY). The use, distribution or reproduction in other forums is permitted, provided the original author(s) and the copyright owner(s) are credited and that the original publication in this journal is cited, in accordance with accepted academic practice. No use, distribution or reproduction is permitted which does not comply with these terms.
*Correspondence: Zongran Pang, zrpang@163.com; Binan Lu, binanlu@muc.edu.cn