- Nutrition and Health Research Group, Department of Precision Health, Luxembourg Institute of Health, Strassen, Luxembourg
Background: Metabolic syndrome (MetS) is a major risk factor for cardiometabolic complications. Certain dietary patterns play a pivotal role in improving MetS components. The aim of this investigation was to study associations between the Mediterranean Diet Score (MDS) and the Alternative Healthy Eating Index (AHEI) and the odds of MetS and its components in adults living in Luxembourg.
Methods: Data from 1,404 adults participating in the cross-sectional ORISCAV-LUX2 study were analyzed by a self-reported questionnaire, anthropometric measures, a food frequency questionnaire (174 items), and blood/urine samples.
Results: A significant association of dietary indices and MetS was not found except when expressing MetS as a score (continuous variable, log-transformed), based on the weighting of compounds using exploratory factor analysis with the MDS (β = −0.118, 95% CI: −0.346, −0.120) and AHEI (β = −0.133, 95% CI: −0.059, −0.019). Fully adjusted linear regression models further showed significant inverse associations between components of MetS and MDS (all as log-transformed variables), including body mass index (BMI) (β = −0.0067, 95% CI: −0.0099, −0.0036), waist-circumference (WC) (β = −0.0048, 95% CI: −0.0072, −0.0024), systolic blood pressure (SBP) (β = −0.0038, 95% CI: −0.0061, −0.0016), and diastolic blood pressure (DBP) (β = −0.0035, 95% CI: −0.0060, −0.0009). Similarly, significant inverse associations between AHEI and components of MetS (log-transformed) included BMI (β = −0.0001, 95% CI: −0.0016, −0.0002), WC (β = −0.0007, 95% CI: −0.0011, −0.0002), SBP (β = −0.0006, 95% CI: −0.0010, −0.0002), and DBP (β = −0.0006, 95% CI: −0.0011, −0.0001).
Conclusion: Higher adherence to a Mediterranean diet and following healthy eating guidelines were associated with reduced odds of MetS and several of its components in Luxembourgish residents, highlighting that balanced and healthy eating patterns are a crucial cornerstone in the fight against MetS.
Introduction
Metabolic syndrome (MetS) is a metabolic and public health-related condition that burdens the life of people and also the healthcare system globally. It is a major risk factor for cardiometabolic complications, affecting over a billion people worldwide (1), with a prevalence of 28% in adults in Luxembourg (2). MetS is a multi-faceted disorder characterized by a cluster of interrelated risk factors for coronary heart disease (CHD), cardiovascular diseases (CVD), and type 2 diabetes (T2D) (1). There are various definitions and diagnostic criteria for MetS. The most generally used and documented criteria are based on the National Cholesterol Education Program Adult Treatment Panel III (NCEP ATP III) (3). MetS is diagnosed when three or more of the following five main components are fulfilled: dyslipidemia, elevated arterial blood pressure (BP), dysregulated glucose homeostasis, abdominal obesity, and/or insulin resistance (4).
One of the most important aspects of MetS is that it aids in screening individuals at high risk of developing CVD, T2D, hypertension, and atherosclerosis, together with increased disease mortality (5). MetS is linked to a 1.5-fold increase in all-cause mortality and a 2-fold elevation of CVD risk (6). In order to decrease the risk of CVD, T2D, and other complications, drug therapy for metabolic components is considered in some cases (7). However, early prevention measures for this condition are more effective when employed on a population-wide scale rather than drug therapy treatment. Nutrition is among the most significant modifying lifestyle factors influencing cardio-metabolic health (8). Modest weight reduction by lifestyle modification, including a healthy diet and sufficient physical activity, are a practical and effective means that can improve all five components of MetS, as lifestyle modification controls the entire metabolic profile of individuals with MetS and selectively mobilizes the abdominal visceral fat (9, 10).
Despite the widespread occurrence and significance of MetS, there is no consensus about the contribution of individual dietary risk factors to MetS. However, several meta-analyses investigating risk factors for MetS have shown that a healthy diet can significantly reduce the (pooled relative) risk of developing MetS (by 15%) and CVD (by 31%) (8, 11, 12). For example, it has been shown that dietary patterns such as a diet low in saturated fat (13), low in simple sugars (14), and reduced meat consumption (15) but high in fruits and vegetables (16) can reduce the risk of MetS. However, rather than focusing only on individual dietary aspects, more integrative and comprehensive approaches that investigate the entirety of food items consumed are sought that characterize dietary properties in a more holistic approach. Such approaches have the primary goal of synthesizing a significant quantity of dietary data (a food item or a nutrient) into an overall score that can be used to evaluate potential risk factors or relate to a health outcome. Two such prominent indices include the Alternative Healthy Eating Index (AHEI) and the Mediterranean Diet Score (MDS).
The AHEI is an index developed by researchers at the Harvard School of Public Health as an alternative measure of diet quality, focused on absolute food intake rather than nutrient density, to identify the future risk of diet-related chronic disease. The AHEI is a valuable tool that measures adherence to dietary guidelines and evidence-based recommendations for nine food groups or aspects, including vegetables, fruits, nuts, and soy protein, the ratio of white to red meat, fiber, trans-fatty acids, the ratio of PUFA/SFA, multivitamin supplement intake, and alcohol (17). A multiethnic Cohort found that better diet quality—as assessed by AHEI, was associated with an 18–26% lower risk of all-cause and CVD mortality; meta-analyses also confirmed these results (18, 19). Several mechanisms for how a higher AHEI may relate to cardiometabolic diseases have been described, such as the direct effects of fibers in reducing cholesterol absorption, the impact of polyphenols in reducing oxidative stress and inflammation, as well as their indirect effects through gut microbiota (20). The MDS is based on solid epidemiologic evidence and evaluates adherence to the Mediterranean diet (MD) based on the consumption of selected foods, especially considering fish intake in addition to legumes, vegetables, fruits, and grains as an advantageous group, and dairy products, red meat, lipids, and alcohol as an adverse health food group (21). High MDS (indicating adherence to an MD) is characterized by high consumption of fish and seafood, olive oil as the primary fat, vegetables, fruits, whole grains, legumes, and nuts, with a high MUFA/SFA ratio; and low consumption of red meat and meat products is related to a lower risk of cardiometabolic diseases. The high amounts of unsaturated fats (MUFA and PUFA), fiber, and polyphenols in this diet and other factors, such as the low intake of SFA, contribute to its beneficial effects in reducing the risk of such diseases, including MetS (22). In addition, recently, the regulatory role of nutritional components of the MD on the gut microbiota and the immune system and the relation to non-communicable diseases, including obesity, T2D, CVD, MetS, and some types of cancer, has been emphasized (23). Evidence suggests that MD adherence was able to modulate the gut microbiota and increases its diversity, which has been related to increased short-chain fatty acid (SCFA) production, among others (24).
It has been shown that assessing overall diet quality, rather than specific nutrients or food components, is more successful in predicting a relationship between diet and disease (25). According to our knowledge, no previous study has used MDS and AHEI together for estimating the risk of MetS. Since these two indicators somehow complement each other (in terms of components) and surveys using them together are limited, a combined investigation was felt appropriate. In addition, no association between dietary indices such as MDS and AHEI with MetS and its components among residents of Luxembourg, a country characterized by a high rate of risk factors such as obesity and high blood pressure (26) and quite diverse dietary habits/culinary landscape (due its almost 50% foreign residents), has been reported on thus far. Therefore, it is important to shed light on this area with the aim of better controlling MetS. This study strives to derive a more recent estimation of this association by determining the relation between MDS, AHEI score, and MetS and its components among residents of Luxembourg.
Materials and methods
Study protocol and design
This analysis is based on the national survey “Observation of Cardiovascular Risk Factor in Luxembourg (ORISCAV-LUX 2),” which is the second wave of a cross-sectional study, carried out between January 2016 and January 2018 to assess risk factors of CVD in the Luxembourgish adult population (27). In the original ORISCAV-LUX survey (2007–2008), n = 1,432 participants were included by a systematic random sampling procedure. In the following ORISCAV-LUX 2 survey, participants were retained by an initial baseline and complimentary sampling. A total of 660 individuals participated in both studies. In the present analysis, the participants were randomly selected based on sociodemographic attributes, including the district of residence, age, and gender. A full description of the sampling procedure in the first and second waves has already been published elsewhere (28). This study was approved by the National Research Ethics Committee (CNER, No 201-505/12) and the National Commission for Data Protection (CNPD).
A total of 1,558 Luxembourg residents between the ages of 25 and 81 were recruited for the ORISCAV-LUX2 study (27). Of the 1,558 participants, 127 were excluded from the analysis due to missing FFQ information or missing data, 26 due to receiving extreme values of energy, and 1 due to age over 80 years. Overall, 1,404 participants were included in our research question (descriptive analyses), with a complete dataset of nutritional characteristics and having at least one of the MetS components. When crucial data for our analysis, such as results of the five main components of MetS, were missing, it was considered an incomplete metabolic profile; thus, these cases (60 participants) were excluded. Therefore, participants with incomplete metabolic profiles were excluded from correlation and regression analyses, and 1,344 cases were considered for such analysis, as represented in the flowchart in Figure 1.
Data collection
Participation in ORISCAV-LUX 2 research entailed three primary steps: completing a self-reported questionnaire (including socio-demographic variables), clinical and anthropometric measures by trained nurses per the standardized operating procedures, and collecting blood, urine, and hair samples in a commercial accredited Laboratory (Ketterthill, Luxembourg).
The data were obtained from questionnaires related to sociodemographic and anthropometric aspects, lifestyle, and self-reported health conditions, including a validated food frequency questionnaire (FFQ) with 174 items (29). The participants, with the assistance of a trained nurse, indicated the frequency and portion size of all consumed food items and beverages on a scale ranging from “never or rarely,” “two or more times/day,” “once a day,” “3–5 times/week,” “1–2 times/week,” and “1–3 times/month” over the previous year. The macro- and micronutrient intake was calculated by multiplying each food item’s consumption frequency by the specific nutrient content of each portion. A photo album covering portion size images of all the consumed food and beverage items was used to accurately identify the portion sizes and determine the amount of intake. The macronutrient and micronutrient intakes were calculated by translating foods and beverages into nutrients using the French Ciqual food database, which lists nutritional information for over 3,100 food items (30). Furthermore, The MDS score and its quartiles were calculated based on the FFQ and Ciqual database; the same was done regarding the AHEI. Demographics, socioeconomic factors, and details about the anthropometric and clinical measurement are all explained in more detail previously, similar to the dietary assessment (27).
Calculation of MetS scores
The MetS as a categorical variable was defined based on the NCEP ATP III definition (Supplementary Table 1). Accordingly, the participant was considered to have MetS if he/she had three or more of the following main components: dyslipidemia, elevated arterial BP, dysregulated glucose homeostasis, abdominal obesity, and/or insulin resistance, according to the NCEP ATP III criteria (3).
However, as there is no validated tool to calculate the MetS score as a continuous variable, previous research reports have employed several methods to quantify it. We used three different standard methods in our approach to quantify the MetS.
First, we calculated the MetS score based on the weighting of its component by using the exploratory factor analysis (EFA). The scoring MetS based on EFA (MetSEFA) was calculated by subtracting the total mean of each component of MetS from values for each person and then dividing it by the total SD multiplied by the EFA coefficient for each component (31). MetSEFA = [(total mean of FBG-individuals FBG/total SD) * EFA coefficient for FBG] + [(total mean of triglyceride (TG)-individuals TG/total SD) * EFA coefficient for TG] + [(total mean of waist circumference (WC)-individuals WC/total SD) * EFA coefficient for WC] + [(total mean of SBP-individuals SBP/total SD) * EFA coefficient for SBP] + [(total mean of HDL-individuals HDL/total SD) * EFA coefficient for HDL], with FBG being fasting blood glucose, SD being standard deviation, and SBP being systolic blood pressure.
The second method (siMS score) was based on a previously published method (32), with the following equation: (2 * WC/height) + (FBG/5.6) + (TG/1.7) + (SBP/130) − (HDL/1.28 or 1.02 for women or men) with values for TG, HDL, and FBG in mmol/L, height and WC in cm.
The third quantifying method was calculated based on regression modules (MetSR) using logistic regression, and the final equation was: −19.94 + (WC * 0.072) + (FBG * 0.052) + (TG * 0.008) + (HDL * − 0.037) + (SBP * 0.025) + (gender * 1.188 or −1.188 for men or women, respectively) + (age * 0.009 or * 0.067 for men or women, respectively), with values for HDL, TG, and FBG in mg/dL.
Calculation of Mediterranean diet score and alternative healthy eating index
Mediterranean diet score
The MDS was chosen in this study to assess the association of the MD diet with MetS. To calculate the MDS for this study, the intake of required food groups was obtained from the completed FFQ data. Then, the median intake of the entire population investigated was found for each food group, and after that, each participant’s food consumption was compared with the median. The MDS ranged from zero (low adherence to MD) to nine (high adherence to MD) and was calculated by combining all component ratings (33). In the end, MDS quartiles were calculated.
Alternative healthy eating index
The AHEI was used in this study to assess the association between a healthy diet and MetS. The AHEI uses nine nutritional components to assess food quality and may be used to offer dietary advice for healthy eating (Supplementary Table 2). Moreover, it is considered more detailed and specific compared to its predecessor, the healthy eating index (HEI), because it includes more items than the HEI, such as protein sources, red-to-white meat ratio, polyunsaturated to saturated fat, and fibers, rather than the broader categories, such as grains and all meats combined as used in the HEI. In addition, attention to the duration of multivitamin usage is also one element that distinguishes the AHEI from the original HEI and other indices (17), though we could not include it here. To construct the AHEI score for this study, relevant food items on the FFQ were allocated to their relevant food categories of each completed FFQ. The nutritional database CIQUAL was used to compute the ratio of PUFA to SFA and cereal fiber intake. The trans-fatty acid information was not available. Therefore, it was not considered in the AHEI scoring, and the AHEI was calculated for this study with only eight components. The amount of each food group was calculated in grams, and grading was done by using the highest-scoring point as a cut-off point. Each component is worth a minimum of zero and a maximum of 10 points, except for the multivitamin component (5 points is the maximum). A total AHEI score, ranging from zero (worst/unhealthy) to 75 (best/healthy), was calculated by combining all component ratings (Supplementary Table 2). In the end, the four AHEI quartiles were calculated.
Statistical analyses
Descriptive and correlations analyses
Statistical analyses were performed using SPSS (IBM, Chicago, IL, USA) v. 25. The normality of data distribution and equality of variance was tested by both Q-Q normality plots and the Kolmogorov–Smirnov test. Log-transformation was undertaken to obtain normal distribution when original data did not meet the normality criteria. These values were reported as median and interquartile ranges (IR), and the categorical variables were reported as numbers and percentages. For continuous data, t-tests were utilized (log-transformation was used for all the continuous variables), while the chi-square test was employed for categorical variables. Distributions of demographics, anthropometric and socioeconomic characteristics of participants based on gender were reported, also based on the MDS and its quartiles and the AHEI and its quartiles. In addition, the distribution of the MDS, the AHEI, and the selected nutrients of participants were reported based on gender and metabolic syndrome status. The p-values below 0.05 (2-tailed) were considered statistically significant.
For correlations, as our data were not normally distributed, the non-parametric correlation was performed using Spearman correlations (bivariate correlations) to assess the correlation of the dietary indices MDS and AHEI with cardiometabolic biomarkers.
Linear regression models were performed to assess the association of each component of MetS with MDS and AHEI. The dietary indices’ scores and the quartiles were analyzed in models A, B, and C.
Model A: the dependent variable was one of the components, and the independent variable was one of the indices, either as the continuous score or as quartiles.
Model B: Model A + adjusted for age groups and gender as confounding factors.
Model C: Model B + additionally adjusted for all sociodemographic variables and selected anthropometric variables (education, job, income, marital status, country of birth, physical activity, currently smoking, and total energy intake).
Logistic regression models were also performed to analyze the association of MetS (as a categorical variable) with the MDS and AHEI with three models (all dependent variables were entered in the regression models log-transformed):
Model A: the dependent variable was MetS as a categorical variable, and the independent variable is one of the indices (AHEI score, AHEI quartiles, MDS score, MDS quartiles).
Model B: Model A + adjusted for age groups and gender as confounding factors.
Model C: Model B + additionally adjusted for all sociodemographic variables and selected anthropometric variables (education, job, income, marital status, country of birth, physical activity, currently smoking, and total energy intake).
In addition, linear regression models were performed to assess MetS scores’ (as a continuous variable) association with MDS and AHEI. The score and the dietary indices’ quartiles were analyzed in three models A, B, and C, as the following (all dependent variables were entered in the regression models log-transformed):
Model A: the dependent variable was one of MetS scores (MetSEFA, siMS score, and MetSR), and the independent variable was one of the indices (MDS and AHEI), either as the continuous score or as quartiles.
Model B: Model A + adjusted for age groups and gender as confounding factors.
Model C: Model B + additionally adjusted for all sociodemographic variables and selected anthropometric variables (education, job, income, marital status, country of birth, physical activity, currently smoking, and total energy intake).
Results
Descriptive analyses
Demographic and socioeconomic distribution analyses are given in Table 1. There were significant differences between participants with MetS and participants without MetS regarding age, BMI, education, job, income, and marital status (Table 1).
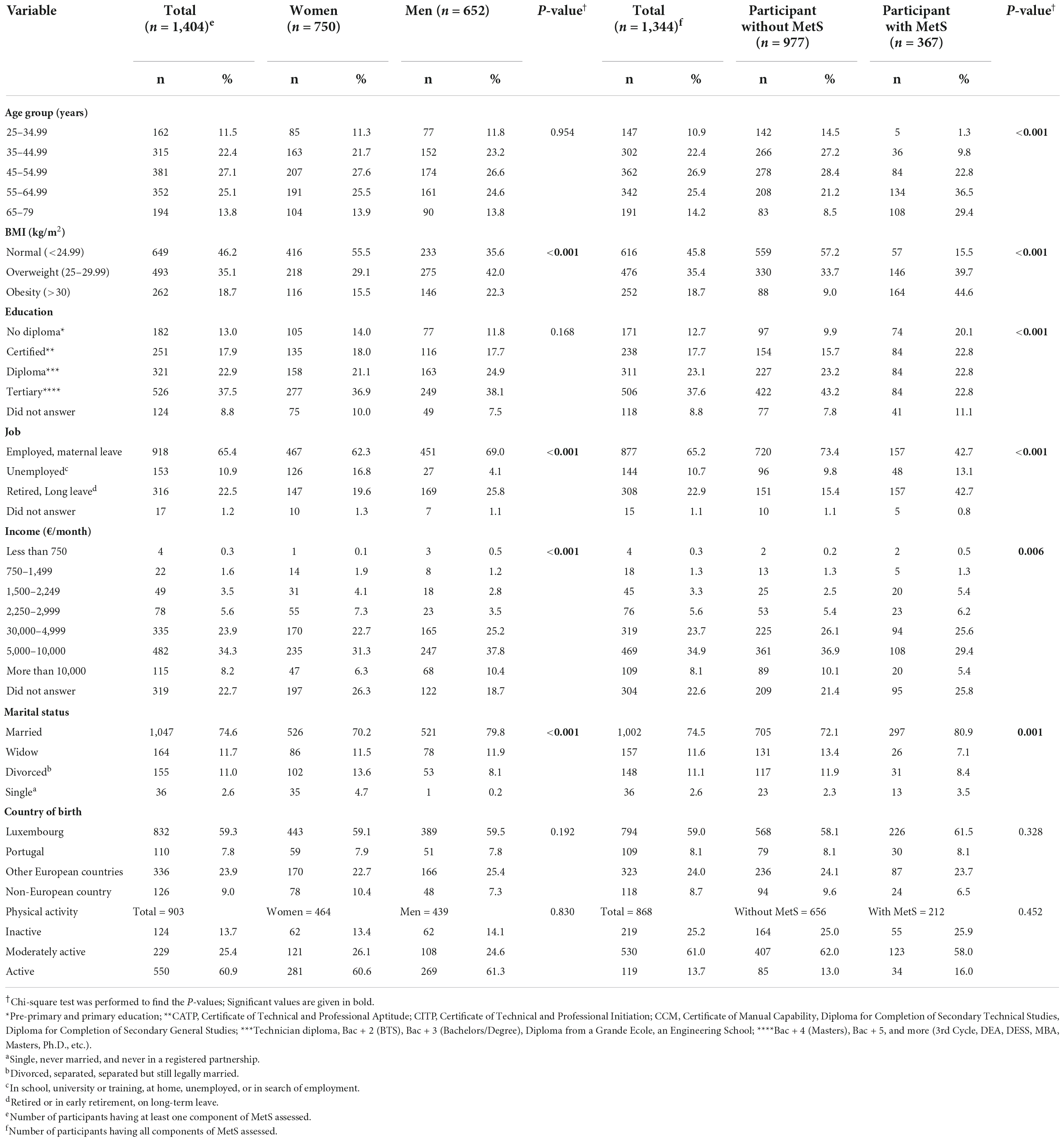
Table 1. Distribution of demographics, anthropometric and socioeconomic characteristics of participants based on gender and MetS status, presented as frequency, percentage, and P-values, comparing women vs. men and participants without MetS vs. participants with MetS.
Also, there were significant differences in dietary patterns between the participants who did have MetS and participants who did not have MetS, according to plant-based protein, docosapentaenoic acid (DPA), carbohydrates, sugar, salt, zinc, sodium, potassium, dairy products, fruits, red meat, nuts and soy products and starchy vegetables (Table 2).
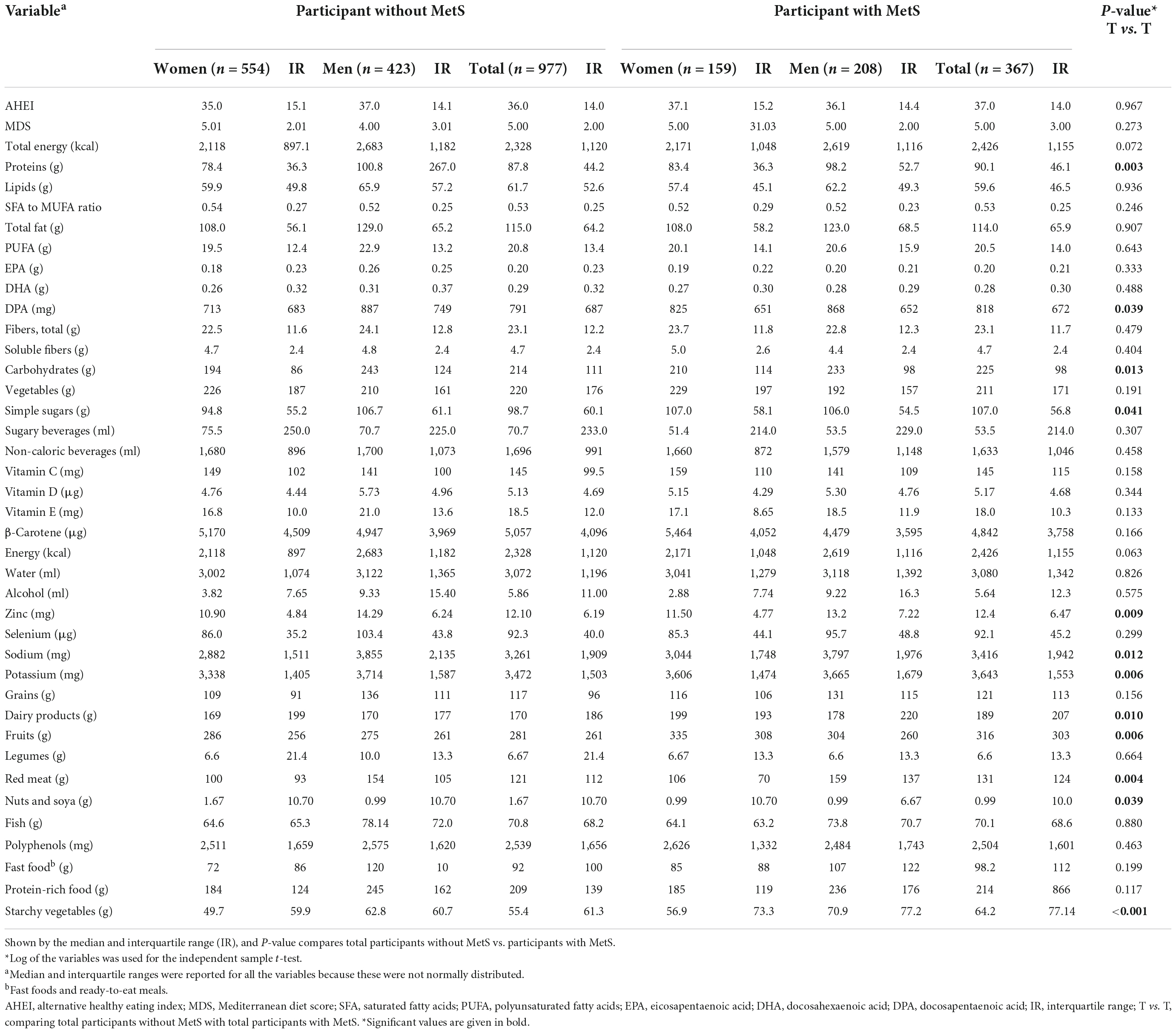
Table 2. Distribution of AHEI, MDS, and selected nutrient intakes of participants per day based on gender and MetS status.
In addition, a comparison of dietary intakes based on categories of MetS components are represented in Table 3. The results of the in-depth investigation showed that participants with SBP higher than the ATP III cut-off (≥130) significantly consumed more energy, red meat, grains, starchy vegetables, total fat, SFA, sodium, simple sugars, alcohol, and less fish and DHA than participants with SBP lower than the cut-off (<130). Similar findings were encountered for DBP. Regarding other components, participants with WC higher than the cut-off [≥102 (men) and ≥88 (women)] consumed significantly more red meat, starchy vegetables, dairy products, cholesterol, sodium, and alcohol comparing participants with WC lower than the cut-off [<102 (men) and <88 (women)]. Similar results were obtained comparing participants based on FBG and TG and in the reverse for HDL categories.
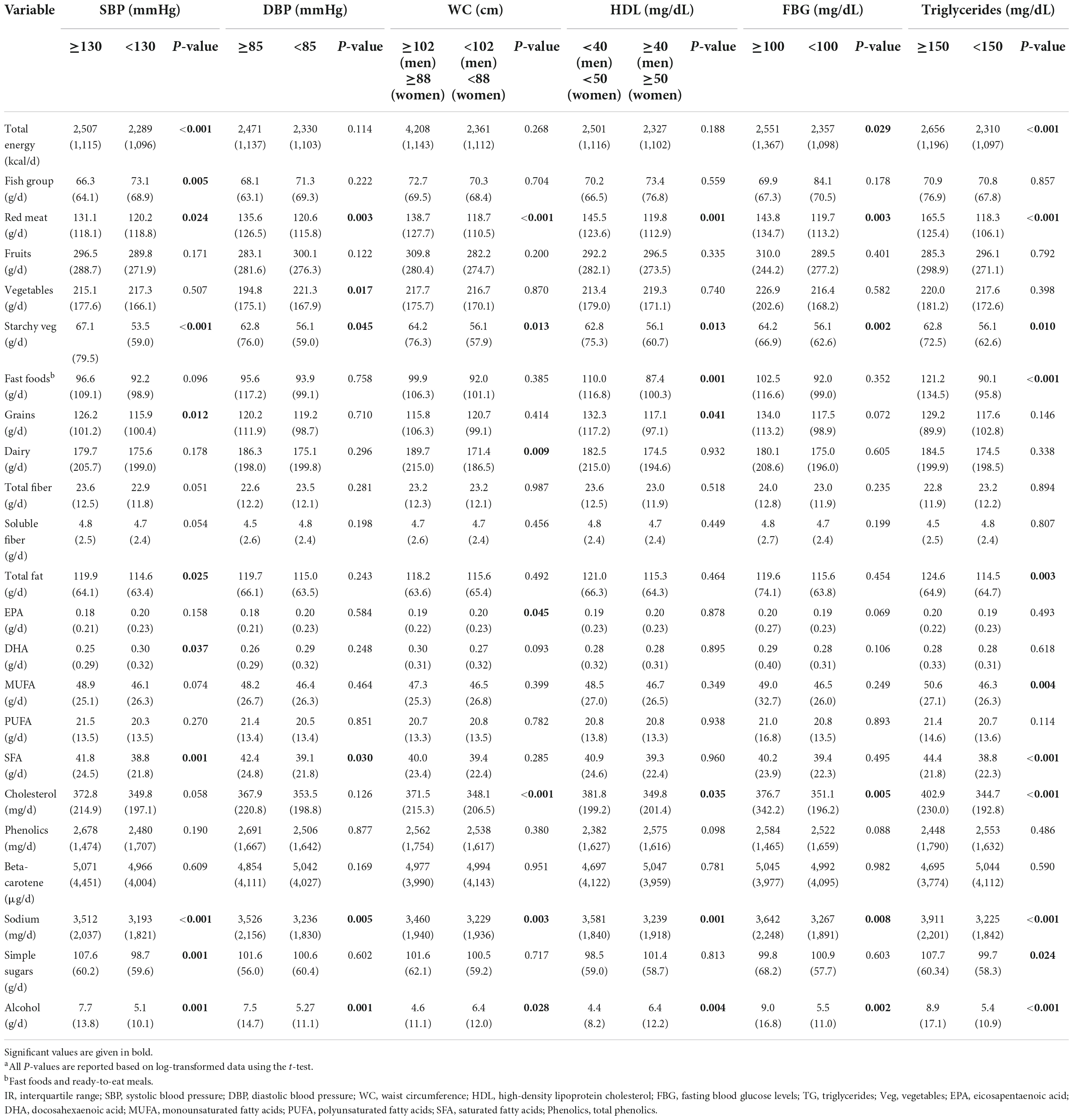
Table 3. Comparison (median and IR) of intake of food groups and selected nutrients based on MetS components.a
Correlation analyses
The correlation analyses between MDS and cardiometabolic markers revealed statistically significant inverse correlations with BMI, waist circumference (WC), TG, SBP, and DBP. Furthermore, a moderate positive correlation was observed between the MDS and the AHEI. The AHEI showed no statistically significant correlation with cardiometabolic markers (Table 4); however, significant results were observed in regression models adjusted for confounding factors.
Regression models
Linear regression analyses of MDS and AHEI with MetS components in final models revealed statistically significant negative associations between MDS and BMI, WC, SBP, and DBP (Table 5, model C). Similar findings were observed for MDS when investigated by quartiles. Regarding the AHEI score, negative associations were observed with the BMI, WC, fasting blood glucose (FBG), SBP, and DBP. For the AHEI quartiles, negative associations were found with BMI, WC, and SBP only (Supplementary Table 3).
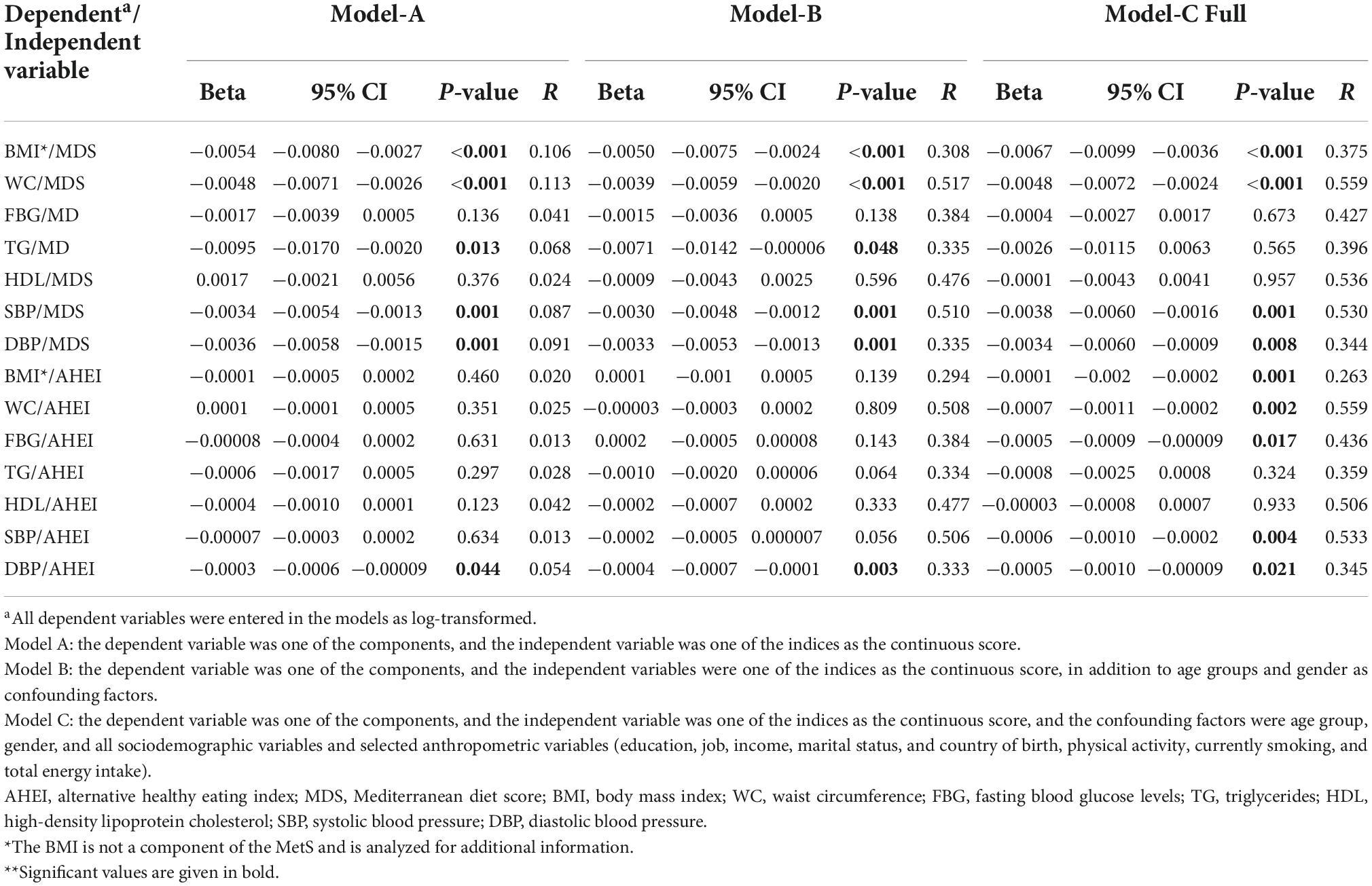
Table 5. Association of the dietary indices MDS and AHEI with MetS components, as derived by linear regression (beta non-standardized, 95% CI, P-value**, and strength of linear association R).
The final model (C) of logistic regression analysis revealed no statistically significant association between MetS and the dietary indices MDS and AHEI, neither when presented as scores nor as quartiles (Supplementary Table 4).
Linear regression analyses of MetS scores (based on the EFA scoring model; MetSEFA) with MDS (as a score and as quartiles) in the three models revealed statistically significant negative associations; similar findings were observed regarding MetS scores (based on the regression scoring model; MetSR) and MDS. Moreover, linear regression analyses of the MetS score presented as the siMS score with the MDS revealed statistically significant negative associations only in model A (Table 6).
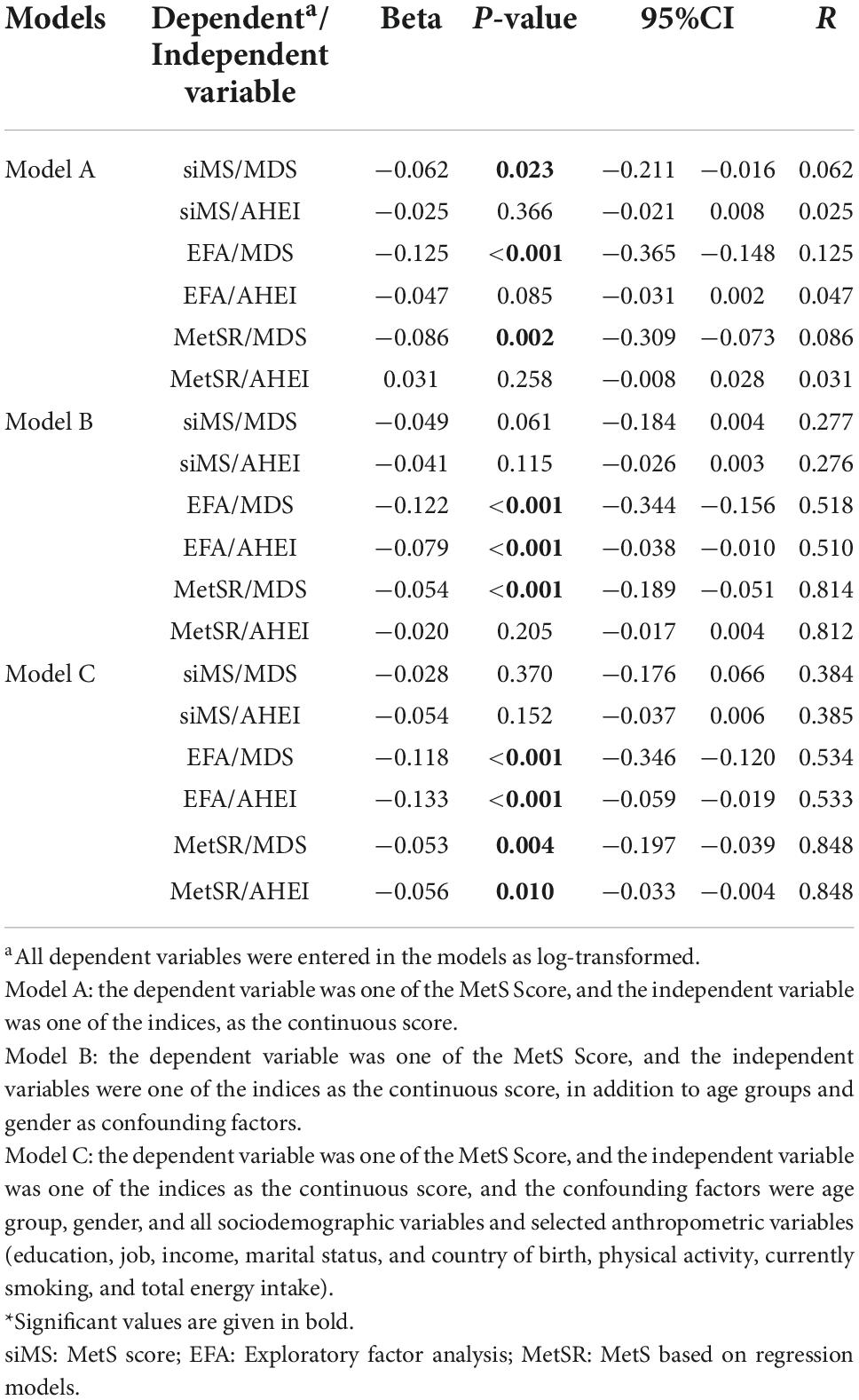
Table 6. Association of MetS scores with MDS and AHEI, as calculated by linear regression (beta non-standardized, 95% CI, P-value*, and strength of linear association R).
The linear regression analyses between the MetSEFA score and the AHEI (both the score and the quartiles) in model B and C show a significant negative association. Regarding the MetSR, there was a significant negative association with the AHEI in model C. However, there was no significant association between AHEI and MetS score as a siMS score with the AHEI (Table 6; Supplementary Table 5).
Discussion
In this nationwide cross-sectional study, we investigated the association between two prominent dietary indices, the MDS and AHEI, with MetS (both as a categorical and continuous outcome) and its components. Logistic regression models did not reveal significant associations between the MDS or AHEI and the odds of MetS (as a categorical variable). However, when employing MetS as a continuous variable, inverse associations between MDS and AHEI and MetS were found partly in the fully adjusted models. Furthermore, based on the final adjusted linear regression models, the MetS components BMI, WC, SBP, and DBP were significantly inversely associated with the MDS, and BMI, WC, SBP, DBP, and FBG with the AHEI.
The inverse associations of the MDS with BMI and WC are well-aligned with the findings from previous studies and with meta-analyses (3, 34). As noted earlier, MD has been reported to positively affect central adiposity in persons with MetS (3, 34). The MD’s high intake of fruits, vegetables, fibers, whole grains, and unsaturated fatty acids (MUFA and PUFA) characteristics has been reported to decrease adiposity, leading to improvements in BMI and, thus also, WC. The mechanistic aspects involved may include antioxidant, anti-inflammatory, and also prebiotic-like effects (35), all of which having metabolic advantages on the cardiovascular system. The influence of the MD on the human gut microbiota has been met recently with increased interest, suggesting that the gut microbiota of people following a Mediterranean-type diet was significantly different in terms of diversity and richness (e.g., F. prausnitzii and B. cellulosilyticus) from people on a Western-type diet (36). Suggested possible mechanisms included bacterial metabolic effects in the colon, including plant-derived polysaccharide degradation, SCFA production out of dietary fiber, and increased excretion of secondary bile acids (36). However, our present knowledge of the cause-effect associations between gut microbiota and the risk of diseases is incomplete and requires further controlled intervention trials to understand the causal relationship between gut microbiota and dietary patterns, including the MD. In addition, direct effects via reduced energy intake are also plausible. For example, due to their high protein content, the nuts included in the MD may positively affect satiety, leading to a decreased caloric intake and, thus, a reduction in BMI (37). In the present study, participants with MetS vs. without MetS–in addition to consuming fewer nuts–also consumed more salt, red meat, and simple sugars (Table 2), which are all characteristics of a more Western-type diet but are typically low in the MD (14), and which could negatively affect MetS (8, 14). Especially simple sugars, due to their pro-inflammatory aspects and impacting insulin sensitivity (38, 39), have been related to the risk of MetS (40), and red meat, due to its high heme-iron content and less favorable fatty acid profile, have also been related to MetS (41). However, it was also observed that persons with MetS consumed more fruits and starchy vegetables (mostly potatoes) than persons without MetS, but of course, reverse causality (persons with MetS being more prudent with their dietary habits) cannot be excluded from our study.
Regarding the association of the odds of MetS and the MDS and the AHEI, the logistic regression results were surprising, as they revealed a non-significant association between MetS and the MDS or the AHEI, even in the final adjusted models. This may be due to the fact that MetS was reported as a categorical variable with a bivariate outcome (yes, no), which likely decreased statistical power compared to continuous variables such as the individual components. Accordingly, in future analyses, it would be recommendable to extend analyses and to study the effects of dietary indices on a “MetS score,” e.g., the Metabolic Syndrome Severity Score as a continuous variable that allows for better powered statistical analyses. Indeed, such MetS scores have been proposed (32, 42); however, no validated or globally accepted, or recommended single score exists. When using MetS as a continuous variable, the EFA-based score and especially the MetSR score resulted in a significant inverse association with a high regression coefficient (Table 6) between both the MDS and the AHEI and MetS, which is more in line with the results of the MetS components. Both the MDS and AHEI were similarly associated with MetS regarding the regression coefficient and beta.
Our findings regarding blood pressure (BP) measurements are in line with previous research studies and meta-analysis outcomes that also showed an inverse association of the MDS with both SBP and DBP (43, 44). The effects of MD on BP may have several reasons. For instance, the MD is rich in polyphenols such as flavonoids that can improve the formation of nitric oxide, which can enhance vasodilation and endothelial function (45). Carotenoids, another group of secondary plant metabolites, could have anti-inflammatory factors that are also important for vessel health (46). In addition to acting in an antioxidant and anti-inflammatory fashion via transcription factors, this can also improve vessel health (47). The intake of fruits and vegetables and less processed foods lowers the burden of sodium intake, which has been strongly associated with BP (48). As in our study, polyphenol and also carotenoid intake did not differ between persons with and without MetS; perhaps the higher salt intake in persons with MetS could have rather been playing a more important role. Though not all studies found significant effects of MD on BP, e.g., in the study of Thomopoulos et al., it was argued that even a marginal effect could contribute to improved CVD risk, as 1 mm Hg improvement in BP can lead to a 2% improvement of CVD risk (49).
Regarding blood lipids, such as triglycerides and HDL-C, our results did not reveal an inverse association in our final adjusted model. Our finding is in line with the results by Godos et al., who found a non-significant association between MDS and TG (3). Other studies, though, have shown a significant inverse association between adherence to MD and TG (34). However, a systematic review concluded that the MD significantly influences HDL-C functionality (antioxidant and anti-inflammatory properties), size, and composition. Specifically, there was an expressed need to clarify MD-derived modulations of HDL-C regarding the determination of HDL-C subgroups, some of which are rather pro- (e.g., HDL2) and some anti-inflammatory (e.g., HDL3) (50). Therefore, our study’s lack of significant results could be due to the lack of insights into relevant HDL-C subgroups, differing by size and lipid composition. In addition, it is likely that population differences and additional possible confounders, such as genetic background or environmental variables, might account for these differences in findings.
Fasting blood glucose, another hallmark component of MetS, did also not associate significantly with MD. This outcome is, on one side, similar to the findings of previous meta-analyses (3, 34). On the other side, Shah et al., in their meta-analysis, described the MD as the best anti-diabetic diet, as it significantly decreased the risk of T2D (51), which has been emphasized in the general literature, as reviewed previously (20). It is possible that FBG exhibited too high variability in the present study, and other markers, such as HbA1C, would have been a more suitable predictor of blood glucose status. FBG is also strongly influenced by physical activity (51), as are blood lipids; however, as we took it into account as a possible confounder, we do not think this perturbed our analysis.
Regarding the AHEI, our investigations found significant inverse associations between the AHEI and BMI, WC, FBG, and BP. These results are largely in accordance with previous findings from meta-analyses and research studies (52–56). Such results are not surprising because increasing the intake of healthy food groups, including fruits, vegetables, and unsaturated fat, and avoiding unhealthy food groups, such as red meat and saturated fat, can supply the body with vitamins and secondary plant metabolites such as polyphenols (e.g., flavonoids) and carotenoids that can improve vascular function, insulin sensitivity, thrombosis, and inflammation (57).
Comparing the association of the MDS and the AHEI with MetS components, it was found that the MDS had a similar association with all MetS components than the AHEI. For instance, improving both scores by one-third, i.e., 3 units in MDS score would reduce WC by 1.43%, and an increase in 25 units of AHEI would reduce WC by 1.74%. However, there are some differences in the scoring algorithm between MDS and the AHEI, such as the fish group that only exists in the MDS. Fish is rich in anti-inflammatory omega-3 fatty acids, which can improve the MetS and its components (58), while the AHEI scoring algorithm reflects the fatty acid as a ratio of MUFA to SFA. In this case, MDS may be more capable of capturing the benefit of fatty acids regarding the MetS thanks to its scoring algorithm that includes the fish group. Although fish intake did not differ between persons with MetS and those without, this differs when focusing on components of MetS. For example, participants with SBP higher than the ATP III cut-off (≥130) significantly consumed less fish and DHA than participants with SBP lower than the ATP III cut-off (<130) (Table 3). However, the intake of some food groups, such as fruits and vegetables, and in line with these, the intake of beta-carotene and polyphenols between people with and without MetS, as well as its components, were not significantly different.
The present study has limitations. First, it was a cross-sectional study; thus, no causality can be inferred from such epidemiological studies. This may be overcome in the future by prospective cohort studies or even randomized controlled trials. Second, our data was not fully representative of the Luxembourg population regarding age, BMI, and place of residence. Accordingly, the results cannot be generalized to the general population. This may be amended by future larger trials with superior stratification designs.
Moreover, Luxembourg’s geographical location may influence the MD’s operational definition and whether it can accurately reflect the typical Mediterranean dietary pattern in its original location. Therefore, there may be a need for an adapted MDS for non-Mediterranean countries (59). Last but not least, diet scores that adapt for energy as part of the scoring algorithm, namely “the relative MDS,” may provide a stronger insight into how MD diet composition is related to body weight, which is—via WC—a core component of MetS. Still, we have selected to assess the more commonly used unadjusted scores. The same applies to the AHEI.
Strengths of the study include that a trained nurse collected the FFQ, which was not a self-ported questionnaire; thus, measurement bias was reduced. Moreover, using the FFQ as a tool for reporting dietary intake is more appropriate and precise than some other techniques, such as food records. The FFQ also contained a large number of food items (174 items), and it was matched with a local food composition database (Ciqual from France) to estimate nutrient intake. Finally, we had anthropometric measurements rather than relying on self-reported measures often used in similar large data sets, and a commercially accredited laboratory in Luxembourg carried out the biological measurements.
Conclusion
According to our knowledge, this is the first study to observe an association of the MDS and the AHEI with MetS and its components across Luxembourgish residents. Our study found significant inverse associations between MDS and BMI, WC, and blood pressure as components of MetS and, similarly, inverse associations between the AHEI and MetS components (BMI, WC, FBG, and blood pressure). It was also shown that a MetS severity score that is continuous was more aligned with findings from the components than the classical categorical definition. Accordingly, results support that adherence to the MD or healthy eating patterns can improve cardiovascular disease risk factors, including MetS. Further research is needed to examine the association of MDS and AHEI in more detail and focus on additional biomarkers of MetS components, such as markers of inflammation and/or oxidative stress. Finally, to validate that the MD and the AHEI might be taken as a first option for the primary prevention of MetS, more research on high-risk patients is needed, preferably within the frame of intervention studies.
Data availability statement
The original contributions presented in this study are included in this article/Supplementary material, further inquiries can be directed to the corresponding author.
Ethics statement
The studies involving human participants were reviewed and approved by CNER–Comité National d’Ethique de Recherche. The patients/participants provided their written informed consent to participate in this study.
Author contributions
FV and KA performed the statistical analyses, interpreted the data, and drafted the manuscript. FV and TB provided expertise and oversight on the intellectual content. TB was involved in critically reviewing the manuscript. All authors read and agreed to the published version of the manuscript.
Funding
The ORISCAV-LUX 2 data collection was funded by the LIH (Ministry of Higher Education and Research funding).
Acknowledgments
We appreciate the dedication of all participants who take part in the ORISCAV-LUX and ORISCAV-LUX 2 studies. We thank the research nurses involved in the ORISCAV-LUX 2 study. We are finally very much indebted to all members of the ORISCAV-Working Group who contributed to the planning and conducting of the ORISCAV-LUX studies: Ala’a Alkerwi, Stephanie Noppe, Charles Delagardelle, Jean Beissel, Anna Chioti, Saverio Stranges, Jean-Claude Schmit, Marie-Lise Lair, Marylène D’Incau, Jessica Pastore, Gloria Aguayo, Gwenaëlle Le Coroller, Michel Vaillant, Hanen Samouda, Brice Appenzeller, Laurent Malisoux, Sophie Couffignal, Manon Gantenbein, Yvan Devaux, Laetitia Huiart, Dritan Bejko, Guy Fagherazzi, Magali Perquin, Maria Ruiz-Castell, and Isabelle Ernens.
Conflict of interest
The authors declare that the research was conducted in the absence of any commercial or financial relationships that could be construed as a potential conflict of interest.
Publisher’s note
All claims expressed in this article are solely those of the authors and do not necessarily represent those of their affiliated organizations, or those of the publisher, the editors and the reviewers. Any product that may be evaluated in this article, or claim that may be made by its manufacturer, is not guaranteed or endorsed by the publisher.
Supplementary material
The Supplementary Material for this article can be found online at: https://www.frontiersin.org/articles/10.3389/fnut.2022.1087985/full#supplementary-material
References
1. Saklayen M. The global epidemic of the metabolic syndrome. Curr Hypert Rep. (2018) 20:1–8. doi: 10.1007/s11906-018-0812-z
2. Alkerwi A, Donneau A, Sauvageot N, Lair M, Scheen A, Albert A, et al. Prevalence of the metabolic syndrome in Luxembourg according to the Joint Interim Statement definition estimated from the ORISCAV-LUX study. BMC Publ Health. (2011) 11:4. doi: 10.1186/1471-2458-11-4
3. Godos J, Zappalà G, Bernardini S, Giambini I, Bes-Rastrollo M, Martinez-Gonzalez M. Adherence to the Mediterranean diet is inversely associated with metabolic syndrome occurrence:a meta-analysis of observational studies. Int J Food Sci Nutr. (2017) 68:138–48. doi: 10.1080/09637486.2016.1221900
4. Detection NCEPEPo, Adults ToHBCi. Third report of the national cholesterol education program (NCEP) expert panel on detection, evaluation, and treatment of high blood cholesterol in adults (adult treatment panel III): the program. Circulation. (2002) 106:3143–421. doi: 10.1161/circ.106.25.3143
5. Alberti K, Zimmet P, Shaw J. The metabolic syndrome—a new worldwide definition. Lancet. (2005) 366:1059–62. doi: 10.1016/S0140-6736(05)67402-8
6. Mottillo S, Filion K, Genest J, Joseph L, Pilote L, Poirier P, et al. The metabolic syndrome and cardiovascular risk:a systematic review and meta-analysis. J Am Coll Cardiol. (2010) 56:1113–32. doi: 10.1016/j.jacc.2010.05.034
7. Bianchi C, Penno G, Romero F, Del Prato S, Miccoli R. Treating the metabolic syndrome. Exp Rev Cardiov Ther. (2007) 5:491–506. doi: 10.1586/14779072.5.3.491
8. Fabiani R, Naldini G, Chiavarini M. Dietary patterns and metabolic syndrome in adult subjects:a systematic review and meta-analysis. Nutrients. (2019) 11:2056. doi: 10.3390/nu11092056
9. Chartrand D, Murphy-Després A, Alméras N, Lemieux I, Larose E, Després J. Overweight, obesity, and CVD risk:a Focus on visceral/ectopic fat. Curr Atheroscl Rep. (2022) 24:185–95. doi: 10.1007/s11883-022-00996-x
10. Després J, Lemieux I. Abdominal obesity and metabolic syndrome. Nature. (2006) 444:881–7. doi: 10.1038/nature05488
11. van Namen M, Prendergast L, Peiris C. Supervised lifestyle intervention for people with metabolic syndrome improves outcomes and reduces individual risk factors of metabolic syndrome:a systematic review and meta-analysis. Metabolism. (2019) 101:153988. doi: 10.1016/j.metabol.2019.153988
12. Rodríguez-Monforte M, Flores-Mateo G, Sánchez E. Dietary patterns and CVD:a systematic review and meta-analysis of observational studies. Br J Nutr. (2015) 114:1341–59. doi: 10.1017/S0007114515003177
13. Harrison S, Couture P, Lamarche B. Diet quality, saturated fat and metabolic syndrome. Nutrients. (2020) 12:3232. doi: 10.3390/nu12113232
14. Martínez-González M, Martín-Calvo N. The major European dietary patterns and metabolic syndrome. Rev Endocr Metabol Dis. (2013) 14:265–71. doi: 10.1007/s11154-013-9264-6
15. Luan D, Wang D, Campos H, Baylin A. Red meat consumption and metabolic syndrome in the costa rica heart study. Eur J Nutr. (2020) 59:185–93. doi: 10.1007/s00394-019-01898-6
16. Tian Y, Su L, Wang J, Duan X, Jiang X. Fruit and vegetable consumption and risk of the metabolic syndrome:a meta-analysis. Publ Health Nutr. (2018) 21:756–65. doi: 10.1017/S136898001700310X
17. McCullough M, Feskanich D, Stampfer M, Giovannucci E, Rimm E, Hu F, et al. Diet quality and major chronic disease risk in men and women:moving toward improved dietary guidance. Am J Clin Nutr. (2002) 76:1261–71. doi: 10.1093/ajcn/76.6.1261
18. Shan Z, Li Y, Baden M, Bhupathiraju S, Wang D, Sun Q, et al. Association between healthy eating patterns and risk of cardiovascular disease. JAMA Int Med. (2020) 180:1090–100. doi: 10.1001/jamainternmed.2020.2176
19. Harmon B, Boushey C, Shvetsov Y, Ettienne R, Reedy J, Wilkens L, et al. Associations of key diet-quality indexes with mortality in the multiethnic cohort:the dietary patterns methods project. Am J Clin Nutr. (2015) 101:587–97. doi: 10.3945/ajcn.114.090688
20. Bohn T, Samouda H, Alkerwi A. Dietary patterns and type 2 diabetes—relationship to metabolic syndrome and inflammation. In: Hébert J, Hofseth L editors. Diet, inflammation, and health. (Chap. 7), Cambridge, MA: Academic Press (2022). p. 261–366. doi: 10.1016/B978-0-12-822130-3.00014-4
21. Trichopoulou A, Costacou T, Bamia C, Trichopoulos D. Adherence to a Mediterranean diet and survival in a greek population. New Engl J Med. (2003) 348:2599–608. doi: 10.1056/NEJMoa025039
22. Rosato V, Temple N, La Vecchia C, Castellan G, Tavani A, Guercio V. Mediterranean diet and cardiovascular disease:a systematic review and meta-analysis of observational studies. Eur J Nutr. (2019) 58:173–91. doi: 10.1007/s00394-017-1582-0
23. García-Montero C, Fraile-Martínez O, Gómez-Lahoz A, Pekarek L, Castellanos A, Noguerales-Fraguas F, et al. Nutritional components in western diet versus mediterranean diet at the gut microbiota–immune system interplay. implications for health and disease. Nutrients. (2021) 13:699. doi: 10.3390/nu13020699
24. Merra G, Noce A, Marrone G, Cintoni M, Tarsitano M, Capacci A, et al. Influence of Mediterranean diet on human gut microbiota. Kompass Nutr Diet. (2022) 2:19–25. doi: 10.1159/000523727
25. Asghari G, Mirmiran P, Yuzbashian E, Azizi F. A systematic review of diet quality indices in relation to obesity. Br J Nutr. (2017) 117:1055–65. doi: 10.1017/S0007114517000915
26. Alkerwi A, Bahi I, Stranges S, Beissel J, Delagardelle C, Noppe S, et al. Geographic variations in cardiometabolic risk factors in Luxembourg. Int J Environm Res Publ Health. (2017) 14:648. doi: 10.3390/ijerph14060648
27. Vahid F, Brito A, Le Coroller G, Vaillant M, Samouda H, Bohn T, et al. Dietary intake of adult residents in Luxembourg taking part in two cross-sectional studies—ORISCAV-LUX (2007-2008) and ORISCAV-LUX 2 (2016-2017). Nutrients. (2021) 13:4382. doi: 10.3390/nu13124382
28. Alkerwi A, Pastore J, Sauvageot N, Coroller G, Bocquet V, d’Incau M, et al. Challenges and benefits of integrating diverse sampling strategies in the observation of cardiovascular risk factors (ORISCAV-LUX 2) study. BMC Med Res Methodol. (2019) 19:27.
29. Alkerwi A, Pastore J, Sauvageot N, Coroller G, Bocquet V, D’Incau M, et al. Challenges and benefits of integrating diverse sampling strategies in the observation of cardiovascular risk factors (ORISCAV-LUX 2) study. BMC Med Res Methdol. (2019) 19:1–10.
30. French Agency for Food EaOHS,. ANSES-CIQUAL French Food Composition Table for Nutritional Intakes Calculation CALNUT. Available online at: https://ciqual.anses.fr/.
31. Shah S, Novak S, Stapleton L. Evaluation and comparison of models of metabolic syndrome using confirmatory factor analysis. Eur J Epidemiol. (2006) 21:343–9. doi: 10.1007/s10654-006-9004-2
32. Sebekova K, Sebek J. Continuous metabolic syndrome score (siMS) enables quantification of severity of cardiometabolic affliction in individuals not presenting with metabolic syndrome. Bratislavské Lekárske Listy. (2018) 119:675–8. doi: 10.4149/BLL_2018_121
33. Trichopoulou A, Kouris-Blazos A, Wahlqvist M, Gnardellis C, Lagiou P, Polychronopoulos E, et al. Diet and overall survival in elderly people. BMJ. (1995) 311:1457–60. doi: 10.1136/bmj.311.7018.1457
34. Bakaloudi D, Chrysoula L, Kotzakioulafi E, Theodoridis X, Chourdakis M. Impact of the level of adherence to Mediterranean diet on the parameters of metabolic syndrome:a systematic review and meta-analysis of observational studies. Nutrients. (2021) 13:1514. doi: 10.3390/nu13051514
35. Nani A, Murtaza B, Sayed Khan A, Khan N, Hichami A. Antioxidant and anti-inflammatory potential of polyphenols contained in Mediterranean diet in obesity:molecular mechanisms. Molecules. (2021) 26:985. doi: 10.3390/molecules26040985
36. Wang D, Nguyen L, Li Y, Yan Y, Ma W, Rinott E, et al. The gut microbiome modulates the protective association between a Mediterranean diet and cardiometabolic disease risk. Nature Med. (2021) 27:333–43. doi: 10.1038/s41591-020-01223-3
37. Garcia-Lorda P, Megias Rangil I, Salas-Salvado J. Nut consumption, body weight and insulin resistance. Eur J clin Nutr. (2003) 57:S8–11. doi: 10.1038/sj.ejcn.1601802
38. Koebnick C, Black M, Wu J, Shu Y, MacKay A, Watanabe R, et al. A diet high in sugar-sweetened beverage and low in fruits and vegetables is associated with adiposity and a pro-inflammatory adipokine profile. Br J Nutr. (2018) 120:1230–9. doi: 10.1017/S0007114518002726
39. MacDonald IA. A review of recent evidence relating to sugars, insulin resistance and diabetes. Eur J Nutr. (2016) 55:17–23. doi: 10.1007/s00394-016-1340-8
40. Sun S, Anderson G, Flickinger B, Williamson-Hughes P, Empie M. Fructose and non-fructose sugar intakes in the US population and their associations with indicators of metabolic syndrome. Food Chem Toxicol. (2011) 49:2875–82. doi: 10.1016/j.fct.2011.07.068
41. Guo H, Ding J, Liang J, Zhang Y. Association of red meat and poultry consumption with the risk of metabolic syndrome:a meta-analysis of prospective cohort studies. Front Nutr. (2021) 8:691848. doi: 10.3389/fnut.2021.691848
42. Hosseini M, Sarrafzadegan N, Kelishadi R, Monajemi M, Asgary S, Vardanjani H. Population-based metabolic syndrome risk score and its determinants:the Isfahan healthy heart program. J Res Med Sci. (2014) 19:1167.
43. Cowell O, Mistry N, Deighton K, Matu J, Griffiths A, Minihane A, et al. Effects of a mediterranean diet on blood pressure:a systematic review and meta-analysis of randomized controlled trials and observational studies. J Hypert. (2021) 39:729–39. doi: 10.1097/HJH.0000000000002667
44. Filippou C, Thomopoulos C, Kouremeti M, Sotiropoulou L, Nihoyannopoulos P, Tousoulis D, et al. Mediterranean diet and blood pressure reduction in adults with and without hypertension:a systematic review and meta-analysis of randomized controlled trials. Clinical Nutr. (2021) 40:3191–200. doi: 10.1016/j.clnu.2021.01.030
45. Medina-Remón A, Tresserra-Rimbau A, Pons A, Tur J, Martorell M, Ros E, et al. Effects of total dietary polyphenols on plasma nitric oxide and blood pressure in a high cardiovascular risk cohort. the PREDIMED randomized trial. Nutr Metab Card Dis. (2015) 25:60–7. doi: 10.1016/j.numecd.2014.09.001
46. Bohn T. Carotenoids and markers of oxidative stress in human observational studies and intervention trials:implications for chronic diseases. Antioxidants. (2019) 8:179. doi: 10.3390/antiox8060179
47. Upadhyay S, Dixit M. Role of polyphenols and other phytochemicals on molecular signaling. Ox Med Cell Longev. (2015) 2015:504253. doi: 10.1155/2015/504253
48. De Pergola G, D’Alessandro A. Influence of mediterranean diet on blood pressure. Nutrients. (2018) 10:1700. doi: 10.3390/nu10111700
49. Thomopoulos C, Parati G, Zanchetti A. Effects of blood pressure lowering on outcome incidence in hypertension:7. effects of more vs. less intensive blood pressure lowering and different achieved blood pressure levels–updated overview and meta-analyses of randomized trials. J Hypert. (2016) 34:613–22. doi: 10.1097/HJH.0000000000000881
50. Grao-Cruces E, Varela L, Martin M, Bermudez B, Montserrat-de la Paz S. High-density lipoproteins and mediterranean diet:a systematic review. Nutrients. (2021) 13:955. doi: 10.3390/nu13030955
51. Shah S, Karam J, Zeb A, Ullah R, Shah A, Haq I, et al. Movement is improvement:the therapeutic effects of exercise and general physical activity on glycemic control in patients with type 2 diabetes mellitus:a systematic review and meta-analysis of randomized controlled trials. Diabetes Ther. (2021) 12:707–32. doi: 10.1007/s13300-021-01005-1
52. Fallaize R, Livingstone K, Celis-Morales C, Macready A, San-Cristobal R, Navas-Carretero S, et al. Association between diet-quality scores, adiposity, total cholesterol and markers of nutritional status in European adults:findings from the Food4Me study. Nutrients. (2018) 10:49. doi: 10.3390/nu10010049
53. Saraf-Bank S, Haghighatdoost F, Esmaillzadeh A, Larijani B, Azadbakht L. Adherence to healthy eating index-2010 is inversely associated with metabolic syndrome and its features among Iranian adult women. Eur J Clin Nutr. (2017) 71:425–30. doi: 10.1038/ejcn.2016.173
54. Alhazmi A, Stojanovski E, McEvoy M, Garg M. The association between dietary patterns and type 2 diabetes:a systematic review and meta-analysis of cohort studies. J Hum Nutr Diet. (2014) 27:251–60. doi: 10.1111/jhn.12139
55. Jannasch F, Kröger J, Schulze M. Dietary patterns and type 2 diabetes:a systematic literature review and meta-analysis of prospective studies. J Nutr. (2017) 147:1174–82. doi: 10.3945/jn.116.242552
56. Streppel M, Arends L, van ’t Veer P, Grobbee D, Geleijnse J. Dietary fiber and blood pressure:a meta-analysis of randomized placebo-controlled trials. Arch Int Med. (2005) 165:150–6. doi: 10.1001/archinte.165.2.150
57. Siri-Tarino P, Chiu S, Bergeron N, Krauss R. Saturated fats versus polyunsaturated fats versus carbohydrates for cardiovascular disease prevention and treatment. Ann Rev Nutr. (2015) 35:517. doi: 10.1146/annurev-nutr-071714-034449
58. de Camargo Talon L, de Oliveira E, Moreto F, Portero-McLellan K, Burini R. Omega-3 fatty acids supplementation decreases metabolic syndrome prevalence after lifestyle modification program. J Funct Foods. (2015) 19:922–8. doi: 10.1016/j.jff.2015.01.022
Keywords: inflammation, oxidative stress, heart disease, type 2 diabetes, hypertension, dietary indices
Citation: Al Kudsee K, Vahid F and Bohn T (2022) High adherence to the Mediterranean diet and Alternative Healthy Eating Index are associated with reduced odds of metabolic syndrome and its components in participants of the ORISCAV-LUX2 study. Front. Nutr. 9:1087985. doi: 10.3389/fnut.2022.1087985
Received: 04 November 2022; Accepted: 23 November 2022;
Published: 13 December 2022.
Edited by:
Sofi G. Julien, Holy Spirit University of Kaslik, LebanonReviewed by:
Uma Tiwari, Technological University Dublin, IrelandAida Turrini, Independent Researcher, Rome, Italy
Copyright © 2022 Al Kudsee, Vahid and Bohn. This is an open-access article distributed under the terms of the Creative Commons Attribution License (CC BY). The use, distribution or reproduction in other forums is permitted, provided the original author(s) and the copyright owner(s) are credited and that the original publication in this journal is cited, in accordance with accepted academic practice. No use, distribution or reproduction is permitted which does not comply with these terms.
*Correspondence: Torsten Bohn, VG9yc3Rlbi5ib2huQGxpaC5sdQ==
†These authors have contributed equally to this work and share first authorship