- 1Department of Public Health, Adama Hospital Medical College, Adama, Ethiopia
- 2Cardiac Primary Prevention Research Center, Cardiovascular Diseases Research Institute, Tehran University of Medical Sciences, Tehran, Iran
- 3Universal Scientific Education and Research Network (USERN), Addis Ababa, Ethiopia
- 4Ethiopian Public Health Institute, Addis Ababa, Ethiopia
- 5Department of Clinical Cardiac Electrophysiology, Tehran Heart Center, Tehran University of Medical Sciences, Tehran, Iran
Background: As defined by the Controlling Nutrition Status (CONUT) score, the prognostic significance of nutritional status has attracted attention in patients with cardiovascular disease. This meta-analysis aimed to determine the importance of CONUT score for prediction of all-cause mortality and major adverse cardiovascular events (MACE) in adult patients with coronary artery disease (CAD).
Methods: Observational studies conducted to evaluate the association of CONUT score with adverse clinical outcomes in patients with CAD were included. We searched MEDLINE, Embase, Scopus, Cochrane library, Google scholar, medRxiv pre-print as well as Science Direct search engine for studies published from the inception of each database until March 21, 2022. Studies reporting the utility of CONUT score in prediction of all-cause mortality and MACE among patients with CAD were eligible. Predictive potential of the CONUT score were summarized by pooling the multivariable adjusted hazard ratio (aHR) with 95% CI for the malnourished vs. normal nutritional status or per point CONUT score increase.
Results: Of 2,547 screened citation, nine observational studies involving 81,257 patients with CAD were analyzed. Malnutrition defined by the CONUT score was associated with significantly increased risk of all-cause mortality when compared with the normal nutritional state (aHR for mild, moderate, and severe malnutrition, respectively: (1.21 [95% CI: 1.15–1.27], I2 = 0%), (1.53 [95% CI: 1.26–1.84], I2 = 84%), and (2.24 [95% CI: 1.57–3.19], I2 = 77%). Similarly, moderate (aHR 1.71 [95% CI: 1.44–2.03], I2 = 0%) and severe (aHR 2.66 [95% CI: 1.82–3.89], I2 = 0%) malnutrition was associated with a significantly higher risk of MACE compared with the normal nutritional state. Additionally, per point increase in the CONUT score was correlated with 20 and 23% additional risk of all-cause mortality and MACE, respectively.
Conclusion: As defined by the CONUT score, malnutrition is an independent predictor of all-cause mortality and MACE in CAD patients. Nutritional assessment with CONUT score could allow clinicians to identify patients with CAD at high risk for adverse clinical outcomes.
Introduction
Coronary artery disease (CAD) remains the leading single cause of death worldwide (1, 2). Since the dramatic development of pharmacological and interventional therapies, the prognosis of CAD patients has improved. However, mortality in CAD patients remains high, and CAD patients are at risk for developing major adverse cardiovascular events (MACE) (3, 4). Therefore, it is particularly important to choose a reasonable preventive strategy for CAD and identify the risk factors leading to poor outcomes.
Malnutrition is known to be associated with poor clinical outcomes in a variety of diseases (5–8). In patients with cardiovascular diseases, evidence indicates that malnutrition is associated with increased in-hospital mortality, mid-to-long-term mortality, and cardiovascular events (9–12). Although nutritional assessment could be a useful predictor of clinical outcome in patients with cardiovascular disease, the lack of a unified definition and gold standard methods for evaluating nutritional status makes it difficult to diagnose malnutrition. Albumin, body weight, body mass index, cholesterol, and other indices are often used to measure nutritional status, although they are often inaccurate (13). Several tools for assessing nutritional status have been developed, including the Geriatric Nutritional Risk Index (GNRI), Prognostic Nutritional Index (PNI), Controlling Nutritional Status (CONUT) score, and Mini Nutritional Assessment (MNA). These nutritional assessment tools showed prognostic value in patients with malignancy, CAD, heart failure and, peripheral arterial diseases (8, 9, 11, 14, 15).
The controlling Nutritional Status (CONUT) score has been reported to be a simple and efficient screening tool for evaluating malnutrition status, especially for early detection and ongoing monitoring of nutritional status in hospitalized patients (16). Current studies suggest that CONUT is associated with short- and long-term prognoses in some diseases (9, 14, 15, 17). The CONUT score is calculated based on the parameters of serum albumin, total cholesterol and, total lymphocyte levels, consisting of an immune-nutritional index reflecting protein/lipid metabolism and immunocompetence (16). According to the CONUT score, individuals with a CONUT score of 0–1 have a normal nutritional status, those with a CONUT score of 2–4 have a mild a moderate degree of malnutrition, and those with a CONUT score of 9–12 have a severe degree of malnutrition (16). The lower level of the laboratory variables used to calculate the CONUT score has shown to be associated with disease progression and mortality in patients with CAD (18–23). Furthermore, when compared to other nutrition-related measures in patients with CAD, recent studies have demonstrated that the CONUT score has the highest predictive ability for mortality and MACE (24, 25). However, no previous meta-analysis has specifically addressed the predictive value of CONUT score for all-cause mortality and MACE in this population group. Therefore, we sought to conduct a meta-analysis to assess the association of malnutrition defined by the CONUT score with all-cause mortality and MACEs in patients with CAD.
Methods
Search Strategy and Study Selection
In reporting the present study, we followed the recommendation of Meta-analysis of Observational Studies in Epidemiology guideline (MOOSE) (26) and the preferred Reporting Items for Systematic Reviews and Meta-Analyses 2020 (PRISMA) 2020 statement (27). We conducted the literature search by screening MEDLINE, Embase, Scopus, Cochrane library, Google scholar, medRxiv pre-print as well as Science Direct search engine. Hand search was done in publishers and journals websites databases in March 2022 for studies published from the inception of each database until March 21, 2022. The following keywords in combination were applied for literature search: “Controlling nutritional status” OR “CONUT” OR “Malnutrition” AND “coronary artery disease” OR “coronary heart disease” OR “acute coronary syndrome” OR “myocardial infarction” OR “unstable angina pectoris” (Supplementary Table 1). The search was run a second time before finalizing the retrieved articles and incorporating any additional identified studies. In addition, we checked the reference list of related articles to identify potentially missing studies. Only articles published in English were considered.
We included observational studies conducted to assess the association of CONUT score with all-cause mortality or MACEs in adult patients with coronary artery disease. No restriction was implemented regarding the sex of the patients. The exclusion criteria included studies that lack a detailed risk summary for the outcomes or reporting unadjusted risk estimates. According to the inclusion and exclusion criteria, GA and AGA independently checked titles and abstracts of search results retrieved from the databases. The same researchers independently reviewed full text articles. The same researchers independently reviewed full text articles. All reviewed and excluded articles were documented on an excel spreadsheet with annotations for reasons of exclusion. In case of discrepancies, they were resolved by inter-researcher discussion with SHM and AVF.
Data Extraction and Assessment of Quality of the Included Studies
GA and AGA extracted data from the final set of included studies for study characteristics, baseline population characteristics, and results in a standardized evidence table. SHM checked these data for accuracy. Disagreements were managed through discussion between researchers. We assessed the methodological quality of the included studies using the Quality in Prognostic Factor Studies (QUIPS) checklist (28). The ratings for individual study were compared between the two researchers and differences were resolved by consensus.
Statistical Analysis
The main analysis was to determine the predictive value of baseline CONUT on all-cause mortality and MACE. The hazard ratio (HR) was used to summarize the estimates representing the risk of all-cause mortality and MACE. The I2 -statistic and the Cochrane Q test were used to assesses the heterogeneity between studies, with statistical significance set at I2 ≥ 50% or p < 0.10. We used a random effects model and the generic inverse variance method to calculate the summary of HR in case of significant heterogeneity. Otherwise, a fixed-effect model was selected. Each study was excluded from the meta-analysis one at a time, and a sensitivity analysis was performed to examine the effect of each study by assessing the degree of change in the magnitude and significance of the effects of exposure. We used CMA 3 and RevMan 5.4. A 2-sided P-value at 5% level is considered significant.
Results
Study Selection
The literature search and selection process are depicted in the PRISMA flow diagram (Figure 1). A total of 2,547 references were retrieved from the database using the described methodology. Of these, 184 duplicate publications and 2,311 references were excluded after scanning the titles and abstracts. The full texts of 57 references were evaluated. After a more detailed review,48 references were excluded for different reasons. For the final analysis, nine (13, 24, 25, 29–34) observational studies were included.
Study Characteristics
The relevant characteristics of the studies included in the meta-analysis are in detailed in Table 1. The included studies were published between 2016 and 2022 and were conducted in Italy, China, Turkey, Taiwan, Japan, and Spain. Seven were retrospective studies (13, 25, 30–34) and two were prospective (24, 29). The number of patients in the eligible studies ranged from 253 to 46,485, for a total of 81,257 CAD patients. The mean age of the participants was 68.6 years old and 71% were male. The mean follow-up period was 4.37 years. The quality assessment of reviewed studies is presented in Supplementary Table 2. The assessment suggested a low-moderate risk of bias for each item. The most common reason that studies have a moderate risk of bias is an inadequate adjustment for confounding factors.
Categorical Analysis of Controlling Nutrition Status Score on All-Cause Mortality and Major Adverse Cardiovascular Events
Four studies (13, 25, 32, 34) provided the categorical analysis of the CONUT score. As shown in Table 2, the meta-analysis indicated malnutrition defined by the CONUT score was significantly associated with higher risk for all-cause mortality when compared with the normal nutritional state (adjusted hazard ratio (aHR) for mild, moderate, and severe degrees of malnutrition, respectively: [1.20 (95% CI: 1.14–1.26), I2 = 0%], [1.53 (95% CI: 1.26–1.84), I2 = 84%], and [2.24 (95% CI: 1.57–3.19), I2 = 77%]. Similarly, moderate [aHR 1.71 (95% CI: 1.44–2.03), I2 = 0%] and severe [aHR 2.66 (95% CI: 1.82–3.890, I2 = 0%] degrees of malnutrition were significantly increased risk of MACE when compared with the normal nutritional status. Statistically, a non-significant association was observed for mild [aHR 1.05 (95% CI: 0.92–1.20), I2 = 0%] degree of malnutrition when compared with the normal nutritional state for MACE. There was no evidence of heterogeneity for the meta-analysis of the association of categorical CONUT score and MACE.
Continuous Analysis of Controlling Nutrition Status Score on All-Cause Mortality and Major Adverse Cardiovascular Events
Eight studies reported the predictive value of the CONUT scores by continuous analysis. As shown in Figure 2, a random-effect meta-analysis indicated that the pooled aHR of all-cause mortality and MACE was (1.20 [95% CI: 1.09–1.33], I2 = 96%) and (1.23 [95% CI: 1.13–1.34] I2 = 87%), respectively, for an increase CONUT score per point, with high heterogeneity. Sensitivity analyses showed that the overall effects remained statistically significant when the individual studies were omitted from the effect size calculation for MACEs. However, for all-cause mortality, the effect reached non-significance in one case when a study by Raposeiras Roubín et al. (25) was omitted (Figure 3).
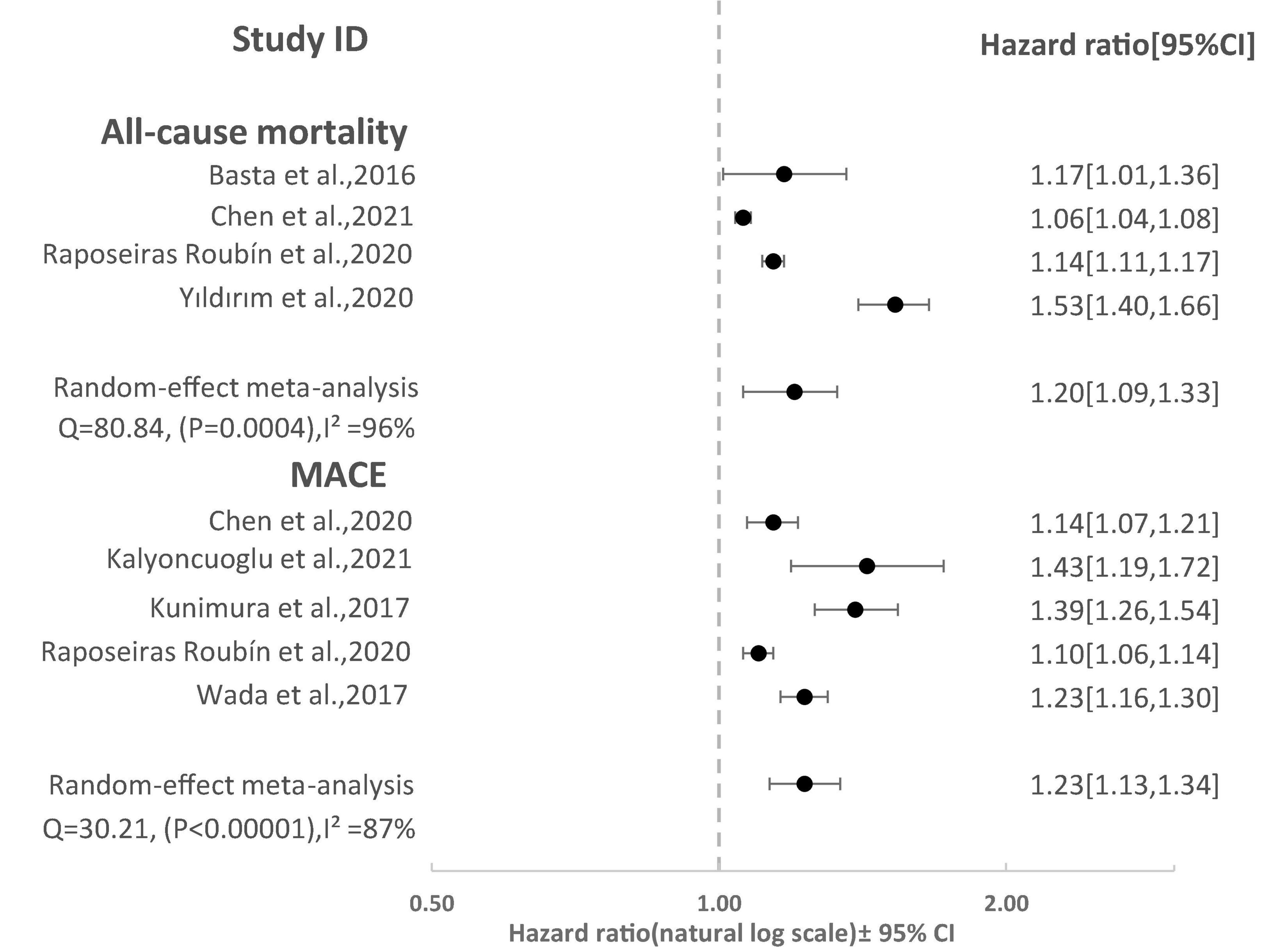
Figure 2. Forest Plot showing pooled hazard ratio with 95% CI of all-cause mortality and major adverse cardiovascular events (MACE) for per point increase in CONUT score.
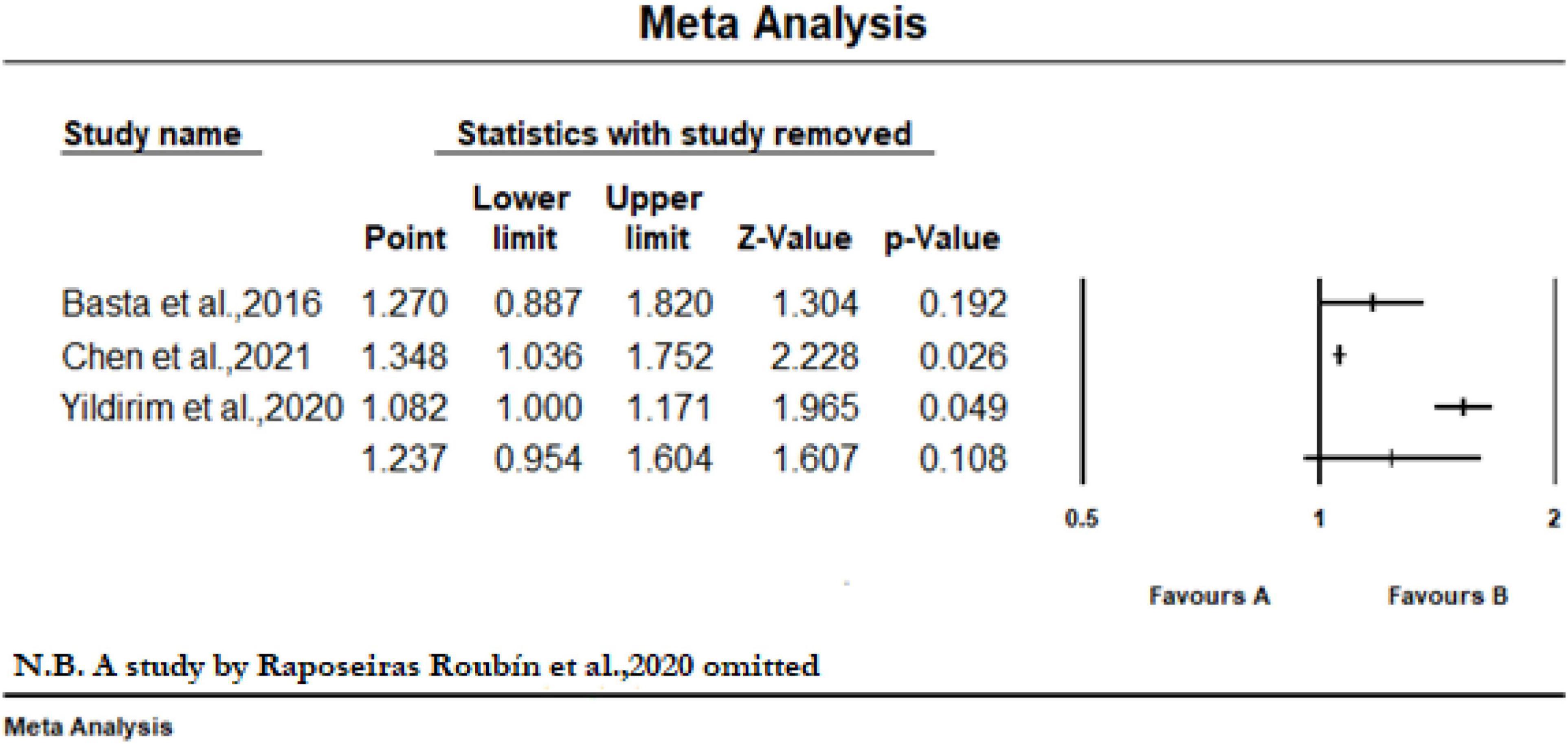
Figure 3. Sensitivity analysis of included studies to determine the pooled hazard ratio with 95% CI of all-cause mortality for per point increase in CONUT score when a study by Raposeiras Roubín et al. (25) was omitted.
Publication Bias
We did not construct the funnel plots or preformed the Begg’s test and Egger’s test to assess the publication bias due to the less than recommended arbitrary minimum number of studies (35).
Discussion
Our meta-analysis showed that malnutrition, as defined by the CONUT, was associated with higher risk of all-cause mortality and MACE in CAD patients. CAD patients with a mild, moderate, and severe degree of malnutrition had a 1. 25-, 1. 62-, and 2.49-fold exaggerated risk of all-cause mortality, respectively, when compared with the normal nutritional state. Likewise, CAD patients with a moderate and severe degree of malnutrition had 1.71- and 2.66-fold increased risk of MACEs, respectively. Moreover, per point increase in CONUT score was associated with 20 and 23% higher risk of all-cause mortality and MACE, respectively. The current meta-analysis suggests that malnutrition can affect the trajectory of CAD prognosis. These findings also confirm the availability of evidence of nutritional assessment by CONUT score in risk stratification of CAD patients.
Malnutrition is common among patients with CAD. Its prevalence varies with various malnutrition indices. Raposeiras Roubín et al. (25) reported that the prevalence of undernutrition in patients with the acute coronary syndrome (ACS) ranged from 9% for the Prognostic Nutritional Index, 50% for the CONUT, and up to 60% for the Nutritional Risk Index. Similarly, Tonet et al. (36) showed that the percentage of ACS patients with malnutrition was 44% using the Mini Nutritional Assessment (MNA). Another recent study conducted to assess the magnitude of malnutrition in diabetic patients with CAD using the CONUT score demonstrated that 60.5% of patients with both CAD and diabetes suffered from malnutrition (37). Moreover, in concordant with our findings, malnutrition determined by the various malnutrition indices was significantly associated with adverse clinical outcomes in patients with CAD (11, 38–40). Therefore, clinicians need to integrate malnutrition detection into their daily practice.
The exact mechanisms of predictive values of CONUT score in CAD remain unclear. CONUT is a simple and efficient screening tool that reflects the nutritional status and immune function of the body (41). The CONUT is based on total lymphocyte count, total cholesterol, and serum albumin (16). The lower level of these laboratory variables has shown to be associated with disease progression and mortality in patients with CAD (18–23). The synthesis of albumin is affected by both nutritional intake and systemic inflammation (19). Several studies have reported that the link between low serum albumin levels and adverse clinical outcomes in patients with heart failure and inflammation has been suggested as a major etiology of low albumin levels (42). Therefore, serum of albumin may indicate both systemic inflammation and nutritional status. Inflammation plays a crucial role in the initiation and progression of atherosclerosis and its acute clinical manifestations (43). Moreover, hypoalbuminemia may indicate persistent injury to the arteries and the progression of atherosclerosis and thrombosis (19). Disruption of cholesterol homeostasis, which occurs as part of the innate immune response, can exacerbate the inflammatory response that causes atherosclerosis (44, 45). The correlation between elevated serum cholesterol levels and cardiovascular disease is well established (46). However, the predictive value of total cholesterol is surprising due to the inverse relationship with adverse clinical outcomes (47). These relationships corroborate the idea of reverse epidemiology, wherein low level of conventional risk factors can seem deleterious (48, 49). Though the cause of the lipid paradox remains unclear, beside other possible explanations, cholesterol-lowering effect of systemic inflammation and malnutrition has been strongly suggested (13, 50, 51). Furthermore, patients with low cholesterol may not receive aggressive statin treatment, and relatively low statin use perhaps may be responsible for high risk within the high-COUNT score group. Malnutrition has been introduced as the most prevalent cause of immunodeficiency (52). Lymphocyte count reduction was shown to be independently and significantly associated with adverse clinical outcomes in CAD (22, 23). Low total lymphocyte level was also associated with higher mortality and was a predictor of prognosis (53, 54). These three laboratory variables contribute to the pathophysiology of CAD (55). Considering the above-mentioned pieces of evidence, the CONUT score may be a reasonable tool for evaluating the nutritional status of the CAD population. Previously published studies have shown that the CONUT score predicts adverse clinical outcome in a population with heart failure (9), stroke (56, 57), hypertension (41), and cancers (58–60).
Despite the increasing number of studies showing the risk of malnutrition, malnutrition is often not listed as a comorbidity of CAD. Our findings strongly support the need for clinicians to practice early identification of malnutrition in high-risk populations. This improves risk stratification and guides subsequent secondary preventive interventions. The variables required to calculate the CONUT score are widely available in routine laboratory tests, malnutrition can be systematically screened in CAD settings regardless of the stage (acute or stable). Malnutrition is a modifiable risk factor. CAD patients with malnutrition should be given meaningful and effective nutrition guidance. However, clinical studies have not shown whether nutritional interventions can improve the prognosis of CAD patients with malnutrition.
Our study should be viewed in the context of certain important limitations. First, we combined only observational studies. All limitations of observations must be taken into account. Second, total cholesterol—a specific variable of the CONUT—might be affected by the use of statins therapy, which could have confounded the assessment of nutritional status using the CONUT score. Third, low to high-degree heterogeneity was observed in the included studies. Different subtypes of patients with CAD, definitions of MACEs, or intervals of follow-up duration may be correlated to the observed heterogeneity. Therefore, the analysis should be interpreted cautiously. Fourth, none of the included studies were able to provide information on preventive medication and adherence to medications given during follow-up. Therefore, we were unable to evaluate the effectiveness of prophylaxis in preventing adverse clinical outcomes. Finally, although we intended to assess potential publication bias and perform a subgroup analysis based on CAD subtype (i.e., ACS and chronic CAD) combined in the meta-analysis, it was not possible due to the less than recommended arbitrary minimum number of studies which under power any of these methods.
Conclusion
As defined by the Controlling Nutrition Status (CONUT) score, malnutrition is an independent predictor of all-cause mortality and MACE in CAD patients. Nutritional assessment with CONUT score could allow clinicians to identify patients with CAD at high risk for adverse clinical outcomes.
Data Availability Statement
The original contributions presented in the study are included in the article/Supplementary Material, further inquiries can be directed to the corresponding author/s.
Author Contributions
GA, AV-F, AA, and SM: study conception, design, and revising the article critically for important intellectual content. GA and AA: acquisition of data, analysis, and interpretation of data, and statistical analysis. GA: drafting the article. All authors read and approved the final version of the study to be published.
Conflict of Interest
The authors declare that the research was conducted in the absence of any commercial or financial relationships that could be construed as a potential conflict of interest.
Publisher’s Note
All claims expressed in this article are solely those of the authors and do not necessarily represent those of their affiliated organizations, or those of the publisher, the editors and the reviewers. Any product that may be evaluated in this article, or claim that may be made by its manufacturer, is not guaranteed or endorsed by the publisher.
Supplementary Material
The Supplementary Material for this article can be found online at: https://www.frontiersin.org/articles/10.3389/fnut.2022.850641/full#supplementary-material
References
1. Chen G, Levy D. Contributions of the framingham heart study to the epidemiology of coronary heart disease. Jama Cardiol. (2016).1:825–30.
2. Wong ND. Epidemiological studies of CHD and the evolution of preventive cardiology. Nat Rev Cardiol. (2014) 11:276–89. doi: 10.1038/nrcardio.2014.26
3. Fox KA, Metra M, Morais J, Atar D. The myth of ‘stable’coronary artery disease. Nat Rev Cardiol. (2020) 17:9–21.
4. Piironen M, Ukkola O, Huikuri H, Havulinna AS, Koukkunen H, Mustonen J, et al. Trends in long-term prognosis after acute coronary syndrome. Eur J Prev Cardiol. (2017) 24:274–80. doi: 10.1177/2047487316679522
5. Datema FR, Ferrier MB, de Jong RJB. Impact of severe malnutrition on short-term mortality and overall survival in head and neck cancer. Oral Oncol. (2011) 47:910–4. doi: 10.1016/j.oraloncology.2011.06.510
6. Knappe-Drzikova B, Maasberg S, Vonderbeck D, Krafft TA, Knüppel S, Sturm A, et al. Malnutrition predicts long-term survival in hospitalized patients with gastroenterological and hepatological diseases. Clin Nutr ESPEN. (2019) 30:26–34. doi: 10.1016/j.clnesp.2019.02.010
7. Zhang X, Tang T, Pang L, Sharma SV, Li R, Nyitray AG, et al. Malnutrition and overall survival in older adults with cancer: a systematic review and meta-analysis. J Geriatr Oncol. (2019) 10:874–83. doi: 10.1016/j.jgo.2019.03.002
8. Luo H, Yang H, Huang B, Yuan D, Zhu J, Zhao J. Geriatric nutritional risk index (GNRI) independently predicts amputation inchronic criticallimb ischemia (CLI). PLoS One. (2016) 11:e0152111. doi: 10.1371/journal.pone.0152111
9. Li H, Zhou P, Zhao Y, Ni H, Luo X, Li J. Prediction of all-cause mortality with malnutrition assessed by controlling nutritional status score in patients with heart failure: a systematic review and meta-analysis. Public Health Nutr (2021) 24:1–8. doi: 10.1017/S1368980021002470
10. Komici K, Vitale DF, Mancini A, Bencivenga L, Conte M, Provenzano S, et al. Impact of malnutrition on long-term mortality in elderly patients with acute myocardial infarction. Nutrients. (2019) 11:224. doi: 10.3390/nu11020224
11. Fan Y, He L, Zhou Y, Man C. Predictive value of geriatric nutritional risk index in patients with coronary artery disease: a meta-analysis. Front Nutr. (2021) 694:736884. doi: 10.3389/fnut.2021.736884
12. Raposeiras-Roubín S, Abu-Assi E, Paz RC, Rosselló X, Barreiro Pardal C, Piñón Esteban M, et al. Impact of malnutrition in the embolic–haemorrhagic trade-off of elderly patients with atrial fibrillation. Europace. (2020) 22:878–87. doi: 10.1093/europace/euaa017
13. Chen S-C, Yang Y-L, Wu C-H, Huang S-S, Chan WL, Lin S-J, et al. Association between preoperative nutritional status and clinical outcomes of patients with coronary artery disease undergoing percutaneous coronary intervention. Nutrients. (2020) 12:1295. doi: 10.3390/nu12051295
14. Kheirouri S, Alizadeh M. Prognostic potential of the preoperative controlling nutritional status (CONUT) score in predicting survival of patients with cancer: a systematic review. Adv Nutr. (2021) 12:234–50. doi: 10.1093/advances/nmaa102
15. Zhang C, Li X-K, Cong Z-Z, Zheng C, Luo C, Xie K, et al. Controlling nutritional status is a prognostic factor for patients with lung cancer: a systematic review and meta-analysis. Ann Palliat Med (2021) 10:3896–905. doi: 10.21037/apm-20-2328
16. De Ulíbarri JI, González-Madroño A, de Villar NG, González P, González B, Mancha A, et al. CONUT: a tool for controlling nutritional status. first validation in a hospital population. Nutr Hosp. (2005) 20:38–45.
17. Liu C, Zhu M, Yang X, Cui H, Li Z, Wei J. Controlling nutritional status score as a predictive marker of in-hospital mortality in older adult patients. Front Nutr. (2021) 8:738045. doi: 10.3389/fnut.2021.738045
18. Suzuki S, Hashizume N, Kanzaki Y, Maruyama T, Kozuka A, Yahikozawa K. Prognostic significance of serum albumin in patients with stable coronary artery disease treated by percutaneous coronary intervention. PLoS One. (2019) 14:e0219044. doi: 10.1371/journal.pone.0219044
19. Kuller LH, Eichner JE, Orchard TJ, Grandits GA, McCallum L, Tracy RP, et al. The relation between serum albumin levels and risk of coronary heart disease in the multiple risk factor intervention trial. Am J Epidemiol. (1991) 134:1266–77. doi: 10.1093/oxfordjournals.aje.a116030
20. Singh RB, Rastogi V, Niaz MA, Ghosh S, Sy RG, Janus ED. Serum cholesterol and coronary artery disease in populations with low cholesterol levels: the Indian paradox. Int J Cardiol. (1998) 65:81–90. doi: 10.1016/s0167-5273(98)00099-0
21. Chen Z, Peto R, Collins R, MacMahon S, Lu J, Li W. Serum cholesterol concentration and coronary heart disease in population with low cholesterol concentrations. Br Med J. (1991) 303:276–82. doi: 10.1136/bmj.303.6797.276
22. Ommen SR, Gibbons RJ, Hodge DO, Thomson SP. Usefulness of the lymphocyte concentration as a prognostic marker in coronary artery disease. Am J Cardiol. (1997) 79:812–4. doi: 10.1016/s0002-9149(96)00878-8
23. Bian C, Wu Y, Shi Y, Xu G, Wang J, Xiang M, et al. Predictive value of the relative lymphocyte count in coronary heart disease. Heart Vessels. (2010) 25:469–73. doi: 10.1007/s00380-010-0010-7
24. Kalyoncuoğlu M, Katkat F, Biter HI, Cakal S, Tosu AR, Can MM. Predicting one-year deaths and major adverse vascular events with the controlling nutritional status score in elderly patients with non–st-elevated myocardial infarction undergoing percutaneous coronary intervention. J Clin Med. (2021) 10:2247. doi: 10.3390/jcm10112247
25. Raposeiras Roubín S, Abu Assi E, Cespón Fernandez M, Barreiro Pardal C, Lizancos Castro A, Parada JA, et al. Prevalence and prognostic significance of malnutrition in patients with acute coronary syndrome. J Am Coll Cardiol. (2020) 76:828–40. doi: 10.1016/j.jacc.2020.06.058
26. Stroup DF, Berlin JA, Morton SC, Olkin I, Williamson GD, Rennie D, et al. Meta-analysis of observational studies in epidemiology: a proposal for reporting. Jama. (2000) 283:2008–12. doi: 10.1001/jama.283.15.2008
27. Page MJ, McKenzie JE, Bossuyt PM, Boutron I, Hoffmann TC, Mulrow CD, et al. The PRISMA 2020 statement: an updated guideline for reporting systematic reviews. Int J Surg. (2021) 88:105906.
28. Hayden JA, van der Windt DA, Cartwright JL, Côté P, Bombardier C. Assessing bias in studies of prognostic factors. Ann Intern Med. (2013) 158:280–6. doi: 10.7326/0003-4819-158-4-201302190-00009
29. Basta G, Chatzianagnostou K, Paradossi U, Botto N, Del Turco S, Taddei A, et al. The prognostic impact of objective nutritional indices in elderly patients with ST-elevation myocardial infarction undergoing primary coronary intervention. Int J Cardiol. (2016) 221:987–92. doi: 10.1016/j.ijcard.2016.07.039
30. Kunimura A, Ishii H, Uetani T, Aoki T, Harada K, Hirayama K, et al. Impact of nutritional assessment and body mass index on cardiovascular outcomes in patients with stable coronary artery disease. Int J Cardiol. (2017) 230:653–8. doi: 10.1016/j.ijcard.2017.01.008
31. Wada H, Dohi T, Miyauchi K, Doi S, Konishi H, Naito R, et al. Prognostic impact of nutritional status assessed by the Controlling Nutritional Status score in patients with stable coronary artery disease undergoing percutaneous coronary intervention. Clin Res Cardiol. (2017) 106:875–83. doi: 10.1007/s00392-017-1132-z
32. Chen L, Huang Z, Lu J, Yang Y, Pan Y, Bao K, et al. Impact of the malnutrition on mortality in elderly patients undergoing percutaneous coronary intervention. Clin Interv Aging. (2021) 16:1347–56. doi: 10.2147/CIA.S308569
33. Yıldırım A, Kucukosmanoglu M, Koyunsever NY, Cekici Y, Belibagli MC, Kılıc S. Combined effects of nutritional status on long-term mortality in patients with non-st segment elevation myocardial infarction undergoing percutaneous coronary intervention. Rev Assoc Med Bras. (2021) 67:235–42. doi: 10.1590/1806-9282.67.02.20200610
34. Liu J, Huang Z, Huang H, He Y, Yu Y, Chen G, et al. Prevalence and mortality of malnutrition in patients with coronary artery disease: A 46,485-Chinese cohort study. Nutr Metab Cardiovasc Dis. (2022) 0939-4753:601–3. doi: 10.1016/j.numecd.2021.12.023
35. Lau J, Ioannidis JP, Terrin N, Schmid CH, Olkin I. The case of the misleading funnel plot. BMJ. (2006) 333:597–600. doi: 10.1136/bmj.333.7568.597
36. Tonet E, Campo G, Maietti E, Formiga F, Martinez-Sellés M, Pavasini R, et al. Nutritional status and all-cause mortality in older adults with acute coronary syndrome. Clin Nutr. (2020) 39:1572–9. doi: 10.1016/j.clnu.2019.06.025
37. Wei W, Zhang L, Li G, Huang Z, Liu J, Wu Z, et al. Prevalence and prognostic significance of malnutrition in diabetic patients with coronary artery disease: a cohort study. Nutr Metab (Lond). (2021) 18:1–10. doi: 10.1186/s12986-021-00626-4
38. Wada H, Dohi T, Miyauchi K, Jun S, Endo H, Doi S, et al. Relationship between the prognostic nutritional index and long-term clinical outcomes in patients with stable coronary artery disease. J Cardiol. (2018) 72:155–61. doi: 10.1016/j.jjcc.2018.01.012
39. Doi S, Iwata H, Wada H, Funamizu T, Shitara J, Endo H, et al. A novel and simply calculated nutritional index serves as a useful prognostic indicator in patients with coronary artery disease. Int J Cardiol. (2018) 262:92–8. doi: 10.1016/j.ijcard.2018.02.039
40. Maruyama S, Ebisawa S, Miura T, Yui H, Kashiwagi D, Nagae A, et al. Impact of nutritional index on long-term outcomes of elderly patients with coronary artery disease: sub-analysis of the SHINANO 5 year registry. Heart Vessels. (2021) 36:7–13. doi: 10.1007/s00380-020-01659-0
41. Sun X, Luo L, Zhao X, Ye P. Controlling Nutritional Status (CONUT) score as a predictor of all-cause mortality in elderly hypertensive patients: a prospective follow-up study. BMJ Open. (2017) 7:e015649. doi: 10.1136/bmjopen-2016-015649
42. Horwich TB, Kalantar-Zadeh K, MacLellan RW, Fonarow GC. Albumin levels predict survival in patients with systolic heart failure. Am Heart J. (2008) 155:883–9. doi: 10.1016/j.ahj.2007.11.043
43. Balanescu S, Calmac L, Constantinescu D, Marinescu M, Onut R, Dorobantu M. Systemic inflammation and early atheroma formation: are they related? Maedica. (2010) 5:292.
44. Tall AR, Yvan-Charvet L. Cholesterol, inflammation and innate immunity. Nat Rev Immunol. (2015) 15:104–16. doi: 10.1038/nri3793
45. Artigas A, Wernerman J, Arroyo V, Vincent J-L, Levy M. Role of albumin in diseases associated with severe systemic inflammation: pathophysiologic and clinical evidence in sepsis and in decompensated cirrhosis. J Crit Care. (2016) 33:62–70. doi: 10.1016/j.jcrc.2015.12.019
46. Gotto AM Jr. Jeremiah Metzger Lecture: cholesterol, inflammation and atherosclerotic cardiovascular disease: is it all LDL? Trans Am Clin Climatol Assoc. (2011) 122:256.
47. Horwich TB, Hernandez AF, Dai D, Yancy CW, Fonarow GC. Cholesterol levels and in-hospital mortality in patients with acute decompensated heart failure. Am Heart J. (2008) 156:1170–6. doi: 10.1016/j.ahj.2008.07.004
48. Rauchhaus M, Clark AL, Doehner W, Davos C, Bolger A, Sharma R, et al. The relationship between cholesterol and survival in patients with chronic heart failure. J Am Coll Cardiol. (2003) 42:1933–40. doi: 10.1016/j.jacc.2003.07.016
49. Güder Gl, Frantz S, Bauersachs J, Allolio B, Wanner C, Koller MT, et al. Reverse epidemiology in systolic and nonsystolic heart failure: cumulative prognostic benefit of classical cardiovascular risk factors. Circ Heart Fail. (2009) 2:563–71. doi: 10.1161/CIRCHEARTFAILURE.108.825059
50. Liu Y, Coresh J, Eustace JA, Longenecker JC, Jaar B, Fink NE, et al. Association between cholesterol level and mortality in dialysis patients: role of inflammation and malnutrition. Jama. (2004) 291:451–9. doi: 10.1001/jama.291.4.451
51. Nakagomi A, Seino Y, Noma S, Kohashi K, Kosugi M, Kato K, et al. Relationships between the serum cholesterol levels, production of monocyte proinflammatory cytokines and long-term prognosis in patients with chronic heart failure. Intern Med. (2014) 53:2415–24. doi: 10.2169/internalmedicine.53.2672
52. Cereda E, Pusani C, Limonta D, Vanotti A. The association of geriatric nutritional risk index and total lymphocyte count with short-term nutrition-related complications in institutionalised elderly. J Am Coll Nutr. (2008) 27:406–13. doi: 10.1080/07315724.2008.10719718
53. Saito H, Kono Y, Murakami Y, Shishido Y, Kuroda H, Yamamoto M, et al. Prognostic significance of pre-and postoperative lymphocyte counts in patients with gastric cancer. Dig Surg. (2019) 36:137–43. doi: 10.1159/000486581
54. Acanfora D, Scicchitano P, Carone M, Acanfora C, Piscosquito G, Maestri R, et al. Relative lymphocyte count as an indicator of 3-year mortality in elderly people with severe COPD. BMC Pulm Med. (2018) 18:116. doi: 10.1186/s12890-018-0685-6
55. Hansson GK. Inflammation, atherosclerosis, and coronary artery disease. N Engl J Med. (2005) 352:1685–95.
56. Naito H, Nezu T, Hosomi N, Aoki S, Kinoshita N, Kuga J, et al. Controlling nutritional status score for predicting 3-mo functional outcome in acute ischemic stroke. Nutrition. (2018) 55:1–6. doi: 10.1016/j.nut.2018.02.025
57. Lopez Espuela F, Roncero-Martín R, Zamorano JDP, Rey-Sanchez P, Aliaga-Vera I, Portilla Cuenca JC, et al. Controlling nutritional status (CONUT) score as a predictor of all-cause mortality at 3 months in stroke patients. Biol Res Nurs. (2019) 21:564–70. doi: 10.1177/1099800419860253
58. Peng L, Meng C, Li J, You C, Du Y, Xiong W, et al. The prognostic significance of controlling nutritional status (CONUT) score for surgically treated renal cell cancer and upper urinary tract urothelial cancer: a systematic review and meta-analysis. Eur J Clin Nutr. (2021). doi: 10.1038/s41430-021-01014-0
59. Takagi K, Domagala P, Polak WG, Buettner S, Ijzermans JN. Prognostic significance of the controlling nutritional status (CONUT) score in patients undergoing hepatectomy for hepatocellular carcinoma: a systematic review and meta-analysis. BMC Gastroenterol. (2019) 19:211. doi: 10.1186/s12876-019-1126-6
Keywords: coronary artery disease, mortality, cardiovascular events, meta-analysis, controlling nutritional status score
Citation: Arero G, Arero AG, Mohammed SH and Vasheghani-Farahani A (2022) Prognostic Potential of the Controlling Nutritional Status (CONUT) Score in Predicting All-Cause Mortality and Major Adverse Cardiovascular Events in Patients With Coronary Artery Disease: A Meta-Analysis. Front. Nutr. 9:850641. doi: 10.3389/fnut.2022.850641
Received: 07 January 2022; Accepted: 30 March 2022;
Published: 09 May 2022.
Edited by:
Lidia Santarpia, University of Naples Federico II, ItalyReviewed by:
Henry Sutanto, SUNY Downstate Medical Center, United StatesJin Liu, Guangdong Provincial People’s Hospital, China
Copyright © 2022 Arero, Arero, Mohammed and Vasheghani-Farahani. This is an open-access article distributed under the terms of the Creative Commons Attribution License (CC BY). The use, distribution or reproduction in other forums is permitted, provided the original author(s) and the copyright owner(s) are credited and that the original publication in this journal is cited, in accordance with accepted academic practice. No use, distribution or reproduction is permitted which does not comply with these terms.
*Correspondence: Godana Arero, Z2FyZXJvMjAxNUBnbWFpbC5jb20=