- 1The Molecular Diagnostics and Clinical Research Unit, Departement of Blood Samples, Biochemistry and Immunology, University Hospital of Southern Denmark, Aabenraa, Denmark
- 2Section for Biostatistics and Evidence-Based Research, The Parker Institute, Bispebjerg and Frederiksberg Hospital, Copenhagen, Denmark
- 3Department of Regional Health Research, University of Southern Denmark, Odense, Denmark
- 4Department of Molecular Medicine, University of Southern Denmark, Odense, Denmark
- 5Research Unit of Rheumatology, Department of Clinical Research, University of Southern Denmark, Odense University Hospital, Odense, Denmark
- 6Department of Cancer and Inflammation Research, Odense University Hospital, Odense, Denmark
- 7Department of Medical Gastroenterology, Odense University Hospital, Odense, Denmark
- 8University Research Clinic for Innovative Patient Pathways, Silkeborg Regional Hospital, Silkeborg, Denmark
- 9Department of Gastroenterology, Slagelse Hospital, Slagelse, Denmark
- 10Department of Dermatology and Allergy Centre, Odense University Hospital, Odense, Denmark
- 11Department of Hepatology and Gastroenterology, Aarhus University Hospital, Aarhus, Denmark
- 12Department of Surgery, University Hospital of Southern Denmark, Aabenraa, Denmark
- 13Department of Research and Learning, University Hospital of Southern Denmark, Aabenraa, Denmark
- 14Department of Gastroenterology, Herlev Hospital, University of Copenhagen, Herlev, Denmark
- 15Department of Gastroenterology, Hospital of Southwest Jutland, Esbjerg, Denmark
- 16Research Unit for Dietary Studies, The Parker Institute, Bispebjerg and Frederiksberg Hospital, Copenhagen, Denmark
- 17Section for General Practice, Department of Public Health, University of Copenhagen, Copenhagen, Denmark
- 18Faculty of Food Science and Nutrition, School of Health Sciences, University of Iceland, Reykjavík, Iceland
- 19Clinical Institute, University of Southern Denmark, Odense, Denmark
- 20Research Unit of Medical Gastroenterology, Department of Clinical Research, University of Southern Denmark, Odense, Denmark
- 21Open Patient Data Explorative Network, Department of Clinical Research, University of Southern, Odense, Denmark
Background: Biologic disease-modifying drugs have revolutionised the treatment of a number of chronic inflammatory diseases (CID). However, up to 60% of the patients do not have a sufficient response to treatment and there is a need for optimization of treatment strategies.
Objective: To investigate if the treatment outcome of biological therapy is associated with the habitual dietary intake of fibre and red/processed meat in patients with a CID.
Methods: In this multicentre prospective cohort study, we consecutively enrolled 233 adult patients with a diagnosis of Crohn's Disease, Ulcerative Colitis, Rheumatoid Arthritis (RA), Axial Spondyloarthritis, Psoriatic Arthritis and Psoriasis, for whom biologic therapy was planned, over a 3 year period. Patients with completed baseline food frequency questionnaires were stratified into a high fibre/low red and processed meat exposed group (HFLM) and an unexposed group (low fibre/high red and processed meat intake = LFHM). The primary outcome was the proportion of patients with a clinical response to biologic therapy after 14–16 weeks of treatment.
Results: Of the 193 patients included in our primary analysis, 114 (59%) had a clinical response to biologic therapy. In the HFLM group (N = 64), 41 (64%) patients responded to treatment compared to 73 (56%) in the LFHM group (N = 129), but the difference was not statistically significant (OR: 1.48, 0.72–3.05). For RA patients however, HFLM diet was associated with a more likely clinical response (82% vs. 35%; OR: 9.84, 1.35–71.56).
Conclusion: Habitual HFLM intake did not affect the clinical response to biological treatment across CIDs. HFLM diet in RA patients might be associated with better odds for responding to biological treatment, but this would need confirmation in a randomised trial.
Trial registration: (clinicaltrials.gov), identifier [NCT03173144].
Introduction
Crohn's disease (CD), Ulcerative Colitis (UC), Rheumatoid Arthritis (RA), Axial Spondyloarthritis (axSpA), Psoriatic Arthritis (PsA), and Psoriasis (PsO) are known as Chronic Inflammatory Diseases (CID). These CID's share pathophysiological pathways, genetics, environmental factors and pharmacological therapies (1). They are prevalent conditions, especially in Westernised, high-income countries with estimated prevalences of 0.25–1.99% (2–6) and the disease burden is predicted to rise (3, 5, 7). CIDs impact education, employability, social and interpersonal functioning negatively, thereby influencing patients' quality of life (8–10). Biologic disease-modifying drugs have revolutionised the treatment of CIDs (1). Unfortunately, up to 60% of the patients have no or only suboptimal response to biological treatment (1, 11, 12). Therefore there is an apparent need to optimise current treatment strategies (1, 13).
Aside pharmacologic treatment, many patients are interested in self-management strategies for their symptoms and often request dietary recommendations. However, sound evidence for such recommendations is lacking, hence, research within this field is imperative. Diet may have anti- or pro-inflammatory effects through its effect on the colonic microbiome which is recognised as important in CID pathogenesis (14–16), e.g., in the gut-joint axis or the gut-skin axis (14, 16), microbiome dysbiosis and abnormal intestinal barrier permeability (14, 16, 17). Short-chain fatty acids, which are products of bacterial fermentation of dietary fibres in the colon, play a crucial role in maintaining colonic epithelial integrity (18, 19). Thus, reduced intake of dietary fibre has been correlated with a thinner colonic mucus (20). Furthermore, end-products of red and processed meat fermentation in the colon have detrimental effects on colonic epithelial health (21). Therefore, a diet rich in dietary fibre and low in red/processed meat may have a protective effect on the gut and reduce systemic inflammation in CID, potentially complementing biological therapy.
The primary objective of this prospective, multicentre cohort study was to compare the proportion of CID patients achieving clinical response to biologics whilst exposed to their habitual diet high in fibre and low in red/processed meat relative to the unexposed CID patients after 14–16 weeks of treatment. Secondary outcomes included changes in health-related quality of life, physician's global assessment and C-reactive protein.
Materials and methods
The study was approved by The Regional Committees on Health Research Ethics for Southern Denmark (S-20160124), the processing of personal data was notified to and approved by the Region of Southern Denmark and listed in the internal record (18/13682) cf. Art 30 of the EU General DATA Protection Regulation. Additionally, the study was registered at Clinical.Trials.gov (NCT03173144) and a protocol published before initiation of the study (22). A statistical analysis plan (SAP) was specified before database closure and the beginning of any statistical analyses (Supplementary File 1). Findings are reported according to the STROBE statement (23).
Study population and setting
The study has been described previously (22). Briefly, we conducted a prospective multicentre cohort study at nine Danish clinical centres with prospectively enrolment of adult patients diagnosed with CID planned to start treatment with a biologic disease-modifying drug. Participants completed questionnaires from home and were examined in the clinics at baseline (prior to initiation of biologic therapy) and after 14–16 weeks of treatment, which is when we expect to observe a potential clinical effect. Patients who did not answer the Food Frequency Questionnaire (FFQ) administered before treatment initiation were excluded. We enrolled patients over a 3-year period from September 21st, 2017 to March 30th, 2020. Enrolment of patients was terminated before reaching the planned number of 320 patients (22) due to the Covid-19 pandemic.
Two patient associations (the Danish Colitis-Crohn's Association and the Danish Psoriasis Association) and three patient representatives diagnosed with RA were involved in developing recruitment plans as well as design of the study and communication about the study to patient members. The results were discussed with patient partners at the Department of Rheumatology at the University Hospital of Odense and an Inflammatory Bowel Disease (IBD) advisory board patient advisory board at the University Hospital of Southern Denmark—Aabenraa.
Data collection
Study data were collected and managed using REDCap (Research Electronic Data Capture) electronic data capture tools (24, 25) hosted at OPEN (Open Patient data Explorative Network), Department of Clinical Research, University of Southern Denmark. Patients were assessed after signing the informed consent at baseline and after a period of 14–16 weeks of treatment. Physicians and study nurses were oriented individually about the study and the standardised data collection forms in REDCap by study personnel. Attending physicians completed all disease activity assessments of patients and CID specific standardised data forms on disease activity at baseline and follow-up (for details, please refer to the SAP): Harvey Bradshaw Index (HBI, CD), Mayo Clinic Score (UC), Simple Clinical Colitis Activity Index (SCCAI, UC), Disease Activity Score 28-CRP (DAS28-CRP, RA and PsA), Simplified Disease Activity Index (SDAI, RA and PsA), 46 joint count (RA), Bath Ankylosing Spondylitis Metrology Index (BASMI, axSpA), Psoriasis Area and Severity Index (PASI, PsO), 66/68 joint count (PsA) as well as a global assessment (all). Physicians or trained study nurses also collected data from medical records about diagnosis, disease localisation, disease duration, history of biological medication, concomitant medication and data on height and weight from clinical visits.
Patients reported smoking status, physical activity and dietary intake via an electronic questionnaire sent to them at baseline and follow-up. Patients also reported on the generic quality of life measures [the Short Form Health Survey-12 (SF-12) and the Short Health Scale] as well as CID specific quality of life measures [RA and PsA; Health Assessment Questionnaire Disability Index (HAQ-DI), PsO; Dermatology Life Quality Index (DLQI)]. Furthermore, patients reported on disease activity scores relevant for axSpA; Bath Ankylosing Spondylitis Functional Index (BASFI) and Bath Ankylosing Spondylitis Disease Activity Index (BASDAI). Details are explained in the SAP (see Supplementary File 1). Data management and study coordination were conducted at the University Hospital of Southern Denmark (Aabenraa), the Molecular Diagnostics and Clinical Research Unit.
Dietary assessment
The FFQ used in the present study was developed and validated in relation to the 2007–2008 Danish Health Examination Survey (26, 27). It was internet-based and administered to the participants prior to initiation of biological therapy with questions covering the habitual dietary intake during the past month. The FFQ contained information from 267 different food groups. For each item, frequency of consumption was evaluated by eight categories ranging from “newer/seldom” to “twice or more per day.” In order to quantify portion sizes for main meals and other main food items, a photographic food atlas consisting of different food and meal series was included at the end of the questionnaire. However, fixed portion sizes were used for some food items with more standardised portion sizes such as fruits. The actual weight in grams for each food item was derived by multiplying the reported frequency of consumption with estimated portion sizes. Thus, total energy intake (Kj/day) and intake of different nutrients including fibres (g/day) was quantified by multiplying the amount consumed of each food item with the amount of energy or nutrient in that food according to the Danish Food Composition Tables (National Food Institute, Technical University of Denmark, https://frida.fooddata.dk/) and aggregating that contribution over all food items in the FFQ. The food group “Red/processed meat” includes beef, veal, pork, lamb, venison, charcuterie, cold cuts and entrails. The Danish Food Composition Tables are maintained and regularly updated by the National Food Institute at the Technical University of Denmark.
Main outcomes
The primary endpoint was the proportion of participants with a clinical response to biologic therapy at the follow-up visit (14–16 weeks from baseline). The specific criteria for clinical response varied across the CID conditions (22):
• Crohn's disease: clinical remission, defined as Harvey-Bradshaw Index of 4 or less;
• Ulcerative colitis: clinical remission, defined as Mayo Clinic Score of 2 or less (with no individual sub score of >1);
• Rheumatoid arthritis: clinical response, defined as at least a 20% improvement according to the criteria of the American College of Rheumatology (ACR20) (28);
• Axial spondyloarthritis: clinical response, defined as at least a 20% improvement according to the Assessment of Spondyloarthritis International Society (ASAS20) (29);
• Psoriatic arthritis: clinical response, defined as at least a 20% improvement according to the criteria of ACR20;
• Psoriasis: clinical response, defined as at least a 75% improvement in Psoriasis Area and Severity Index (PASI 75).
Important secondary (generic) outcomes included changes from baseline to follow-up in measures of health-related quality of life (SF-12; the physical and mental component summaries, the short health scale consists of four components: symptom burden, functional status, disease-related burden and general wellbeing), C-reactive protein, and physician's global assessment. Additionally, the proportion of patients continuing biologic treatment beyond the follow-up period was a secondary outcome measure.
Other secondary non-generic outcomes listed at clinical.trials.gov included changes from baseline to follow-up in disease scores (e.g., ΔHBI score, ΔMayo Clinic score, Δtender joint count, Δswollen joint count, ΔPASI score etc., see SAP).
Statistical analyses
We followed the prespecified statistical analysis plan (Supplementary File 1) based on the analyses outlined in the original protocol (22). The efficacy of treatment was explored in relation to the baseline dietary habits of the participants. Based on their ratio of fibre to red/processed meat intake, participants were divided into an exposed “high fibre low meat” group (=HFLM, consisting of the upper tertile of the study sample) and an unexposed “low fibre high meat” group (=LFHM, consisting of remaining participants, i.e., the lower 66.6% of the study sample). We summarised selected baseline characteristics for HFLM and LFHM by descriptive statistics. We calculated standardised differences to compare the distribution of baseline covariates between groups (30): a standardised difference above 0.5 SD-units was considered indicative of a potential (data-driven) confounder.
The independent contribution of the sub-components to the primary composite outcome were visualised in a forest plot: In the “As Observed” population (i.e., complete data for clinical response), we calculated the ORs and 95% confidence interval (95% CI) of clinical responses in the groups within each CID and pooled the estimates using random-effects meta-analysis (STATA version 16.1, the “metan” package) as we assumed normal distribution of logOR and moreover expected heterogeneity across CIDs.
For the Intention-to-Treat (ITT) population, differences in the proportions of participants with clinical responses between groups were analysed in two logistic regression models: (i) in the “crude model,” we adjusted only for CID; whereas, in the adjusted model (ii) we adjusted for CID, sex, age, and smoking status (ordinal scale: never, former, occasional and current), which were a priori considered potential confounding variables. Initially, clinical centre was also included as a covariate in the model but excluded in the best fitting model. Missing values were imputed by Markov Chain Monte Carlo multiple imputations using the SAS PROC MI procedure (31), assuming that the data were missing at random. We conducted five rounds of multiple imputations. For the dichotomous outcomes, we combined the estimates of the logistic regression analyses of each imputed dataset using Rubin's Rule (32). The multiple imputation datasets for continuous outcomes were combined using linear mixed effect analyses with the inclusion of patient ID as random effect and imputation as a fixed factor variable. The estimates for the continuous outcomes are reported as least-squares means with standard errors. P values were two-sided, with P < 0.05 considered potentially statistically significant. We did not explicitly adjust for multiple comparisons, however due to the implied issues of multiplicity, the secondary outcomes were interpreted with caution based on the Hochberg sequential procedure (33). These analyses where repeated when we (exploratively) analysed two secondary predictors of interest; (1) the upper tertile vs. the two lower tertiles of the study sample with regard to fibre intake [high fibre (HF) vs. low fibre (LF)] and (2) the lower tertile vs. the two upper tertiles of the study sample with regard to red/processed meat intake [low meat (LM) vs. high meat (HM)]. However, beside the previous covariates we also adjusted for red/processed meat intake in the first comparison and fibre intake in the second comparison.
To assess the predictive value of dietary exposure on clinical response on a continuous scale (i.e., independent of arbitrary tertile thresholds), we performed ROC curve analyses for the ratio of fibre to red/processed meat intake and for fibre and red/processed meat intake separately by calculating the sensitivity and specificity for each exposure variable on predicting clinical response and thereafter plotting sensitivity against 1-specificity.
To test the robustness of our results, we carried out six sensitivity analyses on the primary outcome and selected key secondary outcomes; complete case analyses (Supplementary Table S2), per protocol analysis (Supplementary Table S3), non-responder imputation analysis (Supplementary Table S4), an analysis on the subset of the study population fulfilling the original eligibility criteria (i.e., naive to biologic therapy and commencing TNF inhibitor treatment) (Supplementary Table S5), a statistical test for interaction comparing the biologic naive subgroup vs. the bio-experienced subgroup (Supplementary Figure S1) (34, 35) and an analysis with the further inclusion of BMI as covariate (Supplementary Table S8). Moreover, we carried out a propensity score analysis with full optimal matching to assess the potential confounding effect of baseline characteristic differences between the groups. Propensity score analysis was carried out in R (version 4.0.2) using the “MatchIt” package. Propensity scores were calculated by a logistic regression model including the following covariates: age, sex, CID diagnosis, smoking status, number of previous biological medication used, and disease duration. After assessing covariate balance, the marginal estimate of the effect of exposure was calculated.
Results
We enrolled 233 participants, representing ~50% of the patients screened for eligibility (Figure 2). Many of the IBD patients screened did not enter the study. The main reasons were hospitalisation, unwillingness to participate or that treatments were initiated too fast for patients to be included in the study. 40 participants were excluded due to missing or incomplete FFQ data. Of the remaining 193 participants (representing our ITT population), 153 (81% of the study population) were naive to biologic treatment, including almost all rheumatologic patients (except four). According to the fibre/red meat ratio exposure, patients were stratified into a HFLM group (n = 64) and a LFHM group (n = 129). A total of 17 patients (8.8%) were lost to follow-up with regard to the primary outcome [HFLM: 5, 7.8% and LFHM: 12, 9.3%, P = 1.00 (Fischer's exact test)]. Table 1 shows the baseline characteristics; there were more women in the HFLM group (81%) compared to the LFHM group (52%). All other covariates except the intake of fibre and red/processed meat had an SMD of <0.5 SD-units i.e., they were relatively balanced between the groups.
A total of 108 patients out of the 176 (61.4%) from whom we had data on regarding clinical response (i.e., the “As observed” population) responded to the biologics at follow-up according to our specified criteria for clinical response. On the “As observed population,” we included the absolute number of clinical response (events) for HFLM and LFHM in a meta-analysis of the included CIDs (Figure 1). There was no difference between groups in the primary (combined) analysis (OR 1.14, 95% CI: 0.41, 3.15) but the I2 of 50.7% indicated moderate to substantial heterogeneity across CIDs. When looking at the individual strata, the OR for RA patients was in favour of the HFLM group (OR 8.56, 95% CI: 1.74–42.17). Based on logistic regression models on the ITT population, 64% responded to biologics in the HFLM exposed group compared to 56% in the LFHM group (Table 2, OR 1.39, 95% CI: 0.72–2.69). After adjusting for sex, age, CID, and smoking status, the OR was 1.48 (Table 2, 0.72–3.05). For RA patients, the OR when comparing HFLM to LFHM in the adjusted model was 9.84 (1.35–71.56). Changes in health-related quality of life (SF-12 PCS, SF-12 MCS, and the Short Health Scale), physician's global assessment and C-reactive protein were similar between groups (Table 2). Likewise, the proportion of patients continuing biologic treatment beyond the 14–16 weeks was similar between groups.
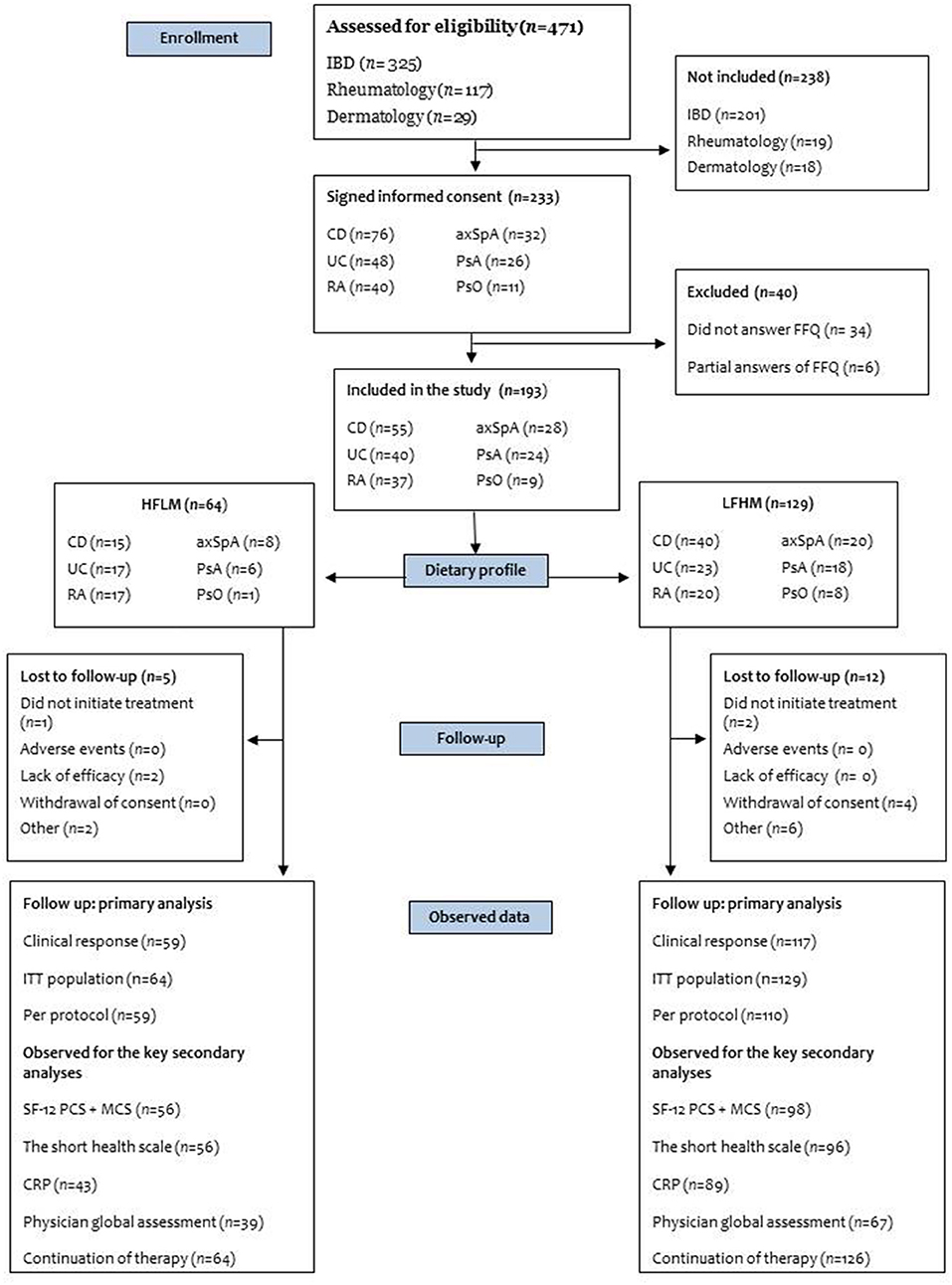
Figure 1. Flow chart of the enrolment of participants. Number of Chronic Inflammatory Disease patients who were screened, included in the study and included in the analysis. Patients screened for eligibility were not explicitly recorded in the study. For CD, UC, and PsO we estimated a number based on the mean inclusion rate from two clinics that kept a pre-screening log. For RA, axSpA, and PsA, the number is not an estimate but the total number of patients that initiated biologic treatment in the clinic during enrolment. IBD, Inflammatory bowel disease (Crohn's disease and Ulcerative colitis); CD, Crohn's disease; UC, Ulcerative colitis; RA, Rheumatoid arthritis; axSpA, Axial spondyloarthritis; PsA, Psoriatic arthritis; PsO, Psoriasis; FFQ, Food Frequency Questionnaire; HFLM, high fibre/low meat; LFHM, low fibre/high meat; ITT, intention-to-treat; SF-12 PCS + MCS, short form health survey the physical and mental component summary, The short health scale includes four health dimensions (symptom burden, functional status, disease-related burden and general wellbeing); CRP, C-reactive protein.
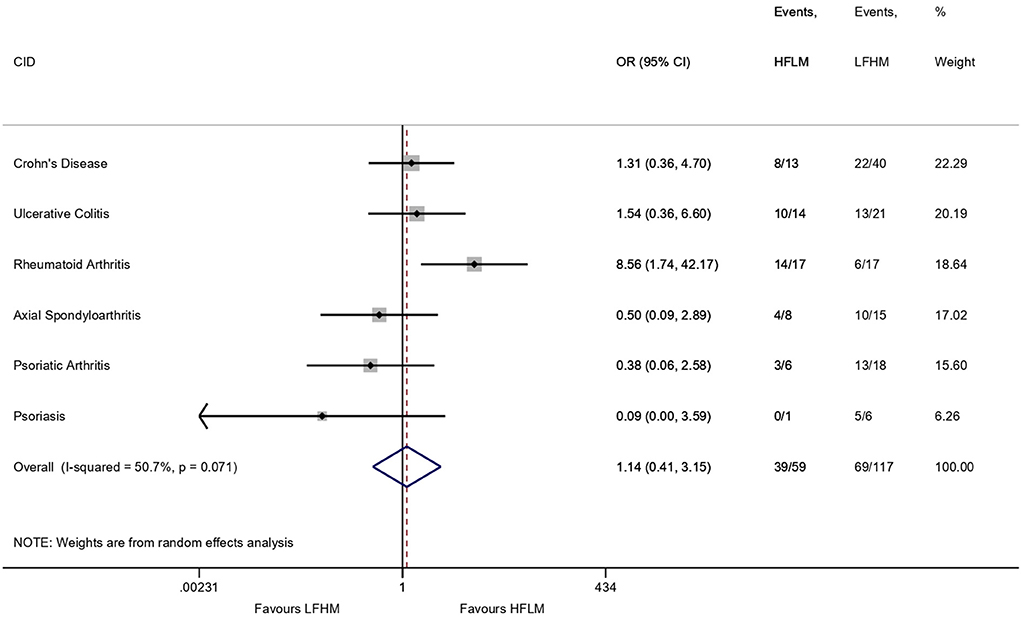
Figure 2. Meta-analysis (random effects model) of the included Chronic Inflammatory Diseases (CIDs) on the “As Observed” population comparing clinical response to biologics in patients with a high intake of fibre and low intake of red/processed meat (HFLM) vs. patients with a low intake of fibre and high intake of red/processed meat (LFHM). The horizontal lines represent the odds ratio (OR) ± 95% confidence interval. Event = clinical response according to the specified criteria for each CID, i.e., the number shows how many out of the total number of participants in the group, that have had a clinical response. The “As Observed” populations means patients with complete data for clinical response (i.e., 17 patients are excluded).
The results of the sensitivity analyses (Supplementary Tables S2–S5, S8 and Supplementary Figure S1) are essentially in agreement with the primary analysis. However, in Supplementary Table S5, when analysing the subset of the study population that were naive to biological treatment and treated with a TNF inhibitor, the OR of clinical response was 2.61 higher in the HFLM group (1.09–6.18). Thus, we carried out a subgroup analysis to test for interaction between naive and bio-experienced patients, but found no evidence to support a different impact of the diet groups on clinical response, with an estimated interaction effect (ratio of odds ratio) of 1.58 (0.74–3.35, P = 0.1). The propensity score analysis was also in agreement with the primary analysis (results not shown).
Finally, we investigated if fibre intake and red/processed meat where independently associated with clinical response in explorative analyses. There was no significant difference between the groups in either comparison (Supplementary Tables S6, S7).
In order to fully explore whether other thresholds of fibre and meat intake could change the prognostic utility of these measures, Figure 3 represents the ROC curves which supports the finding of no association between fibre and meat intake and clinical response. There was no predictive value of the diet on clinical response for neither the ratio or the isolated intake of fibre and red/processed meat as all three curves overlap the reference line (Figure 3). Other secondary outcomes are presented in Supplementary Table S1.
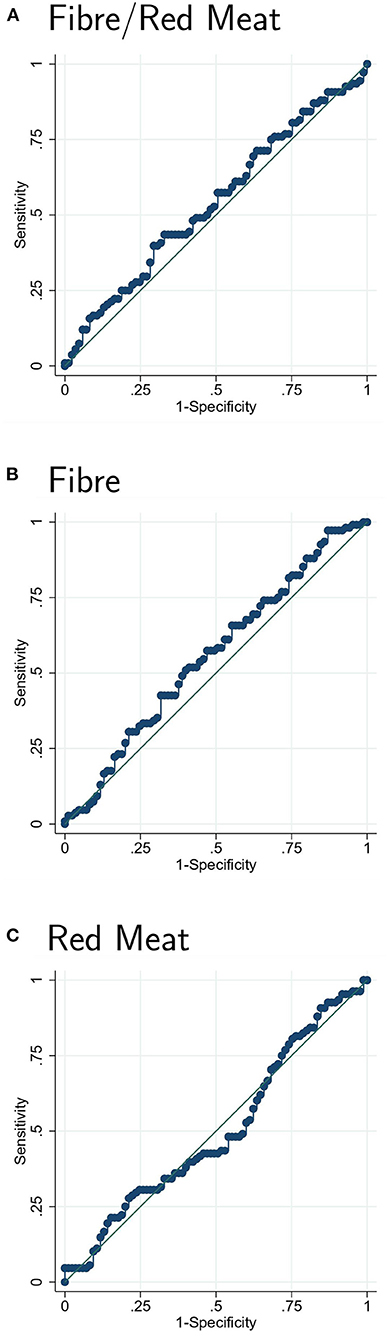
Figure 3. Receiver operating characteristics curve analysis for the predictive value of the ratio of fibre and red/processed meat intake (A), fibre intake (B) and red/processed meat intake (C) on clinical response. The ROC curves were constructed by calculating the sensitivity and specificity for prediction of clinical response for (A) the ratio of fibre to red/processed meat intake, (B) fibre intake, and (C) red/processed meat intake. Thereafter the sensitivity was plotted against 1-specificity. ROC, receiver operating characteristics.
Discussion
Principal findings
Our study evaluated if a habitual high intake of fibre and low intake of red/processed meat vs. low intake of fibre and high intake of red/processed meat was associated with clinical response to biological treatment in prospectively enrolled patients with one of six diagnoses of CID. There was no significant difference in the proportion of patients with a clinical response between groups across the CID conditions or in any of the secondary outcomes. However, our results indicate that a high habitual dietary intake of fibre and low intake of red/processed meat can complement the biologic treatment for RA patients as the odds of clinical response were almost 10 times higher in the HFLM group compared to LFHM.
Comparison with other studies
It has been suggested that the high prevalence of CIDs in Westernised countries relates to dietary habits and lifestyle. While dietary habits may be relevant in the development (and therefore prevention) of CIDs, diet as a supporting factor for pharmacologic treatment has not received much attention. Nevertheless, a supporting effect of diet on pharmacologic treatment is possible. For instance, a meta-analysis found that enteral nutrition therapy in combination with infliximab therapy increases the efficacy for CD (36). Also, an interventional but uncontrolled study found a remission rate of 100% among newly diagnosed CD patients on infliximab (TNFi) therapy in combination with a semi-vegetarian (high fibre) diet (37).
High consumption of red/processed meat and low consumption of dietary fibres is typical of a Westernised diet, while the opposite is characteristic for a Mediterranean Diet (38). The Mediterranean diet is considered a model for healthy eating, and adherence to it is associated with reduced risk of overall mortality, occurrence of chronic diseases (39) and symptomatic improvement of RA (40). Nevertheless, the diet of the HFLM group does not represent a healthy eating pattern in terms of fibre and red/processed meat intake per se, but rather a healthier (or less unhealthy) diet in contrast to the LFHM group. The estimated daily intake of fibre in the HFLM group of 22 g/day is below the recommendations from most countries of 25–35 g/day (41) but in line with the estimated average intake of dietary fibre for Danes (22 g/day) (42) and Europeans (men: 18–24 g/day, women: 16–20 g/day) (41). On the other hand, the average intake of red/processed meat in HFLM was 51 g/day (LFHM: 118 g/day), which is above the EAT-Lancet Commission's proposal of a healthy dietary pattern (14 g/day) (43). However, the intake corresponds well with estimates of mean intakes of red and processed meat among European countries (71–93 g/day) (44). Yet, the intake in our study is low in comparison with a report of the Danes dietary habits from 2011–2013 (134 g/day) (42).
An explanation for the potential benefit of the HFLM diet for RA patients, that we observe in our study in contrast to the other CIDs, may lie in the different positions of the CIDs on the immunological disease continuum extending from “pure” autoinflammatory diseases to “pure” autoimmune diseases (45–47). Although there are mixed autoinflammatory and autoimmune patterns among the CIDs, RA is classified as an autoimmune disease in the classical sense whereas the other CIDs are more classified as autoinflammatory (45, 48). Thus, it can be speculated that the adaptive immune system may be more susceptible to dietary influences. Another explanation may be the fact that almost all RA patients in our study were naive to biologics. Studies have found that rheumatic patients naive to TNFi are more likely to respond to the treatment compared to biologic experienced patients (49–51). The significant differences between the groups among the RA patients in the primary analysis suggests that this likelihood of a positive response to treatment is emphasised when adding dietary habits to the equation. However, this was not the case for CIDs as a group as we found no evidence to support a different impact of the diet groups on clinical response when comparing biologic naive with biologic-experienced patients. We also looked into whether there were large differences in fibre and meat intake across the CIDs that could perhaps be part of the explanation (see Supplementary Table S9). However, the RA patients did not stand out as an extreme.
Strengths and limitations of the study
Our study is subject to certain limitations inherent in its design. Measurement of the exposure is connected to some imprecision as it is based on FFQs evaluating the past month before treatment initiation. The FFQ is a self-reporting assessment instrument and is therefore prone to measurement error by risk of recall bias and under- or over-reporting of dietary intake. This imprecision is likely to bias the results towards unity (52). Another limitation of our study is the limited number of participants. The wide 95% confidence interval of our primary outcome indicates a risk for type 2 errors, i.e., we cannot conclude that there truly is no difference between the groups across the CID diagnoses because of insufficient power. Furthermore, perhaps the two diet groups were not sufficiently separated (i.e., indifferent) in relation to exposure and it would be more correct to name the LFHM group “non-high fibre/ non-low meat.” If we had the power, potential differences would be more obvious if we compared the upper tertile with the lower tertile as the middle tertile may blur the contrast.
Dietary assessment (i.e., the FFQ) was only carried out at baseline. Thus, our analyses are based on the assumption that the habitual dietary intake will not change over time, but this may not be accurate for especially CD and UC. It is known that IBD patients eat differently during active and inactive disease (53), thus, the dietary intake for this particular group may change during the treatment period. Nevertheless, specifically fibre intake has been observed to be the same in IBD patients with active and inactive disease (53), although lower compared to healthy controls (53, 54). Thus, the intake of fibres may be relatively stable. However, if the intake of fibre and red/processed meat have changed during the study period for the study participants, there is a risk of misclassification bias, which can have unpredictable results as it can either increase or decrease the association. Furthermore, intake of fibres and red/processed meat may very well be associated with intake of other macro- and micro nutrients and foods. Thus, the diet other than fibre and meat intake will probably vary considerably between HFLM and LFHM (55), which may be partly responsible for the observed effect or lack of.
The eligibility criteria for the study were changed after protocol development and initiation of the study (i.e., bio-experienced patients and patients initiating other biologics than TNFi were also made eligible), which can sometimes create bias. However, the amendment was not made based on looking at the data but to increase the sample size. If anything, the heterogeneity of the study sample, which was increased due to the change, may have weakened a possible association. Indeed, a sensitivity analysis of the subgroup fulfilling the original eligibility criteria showed differences between the diet groups.
The study also has several strengths, including its prospective study design and rigorous study protocol (22). In addition, it is a real-life pragmatic cohort that strengthens the external validity of the study. Furthermore, participants were unaware of the specific exposure (fibre and red/processed meat intake) being studied. Finally, our results were robust to multiple sensitivity analyses, including applying an alternative approach (propensity score analysis), except for the above mentioned which found a difference between groups.
Conclusion
In conclusion, we found no difference in clinical response to treatment with biologics among CID patients exposed to a habitual HFLM diet compared to a LFHM diet. Yet, our results suggest a potential benefit of the HFLM diet among RA patient, however, due to the imprecision of the exposure, the observational study design, and because the analysis is based on a relatively small number of patients, more research on the subject is needed—preferably from interventional studies.
Data availability statement
The raw data supporting the conclusions of this article will be made available upon request pending application and approval, contact: dmFAcnN5ZC5kaw==.
Ethics statement
The studies involving human participants were reviewed and approved by the Regional Committees on Health Research Ethics for Southern Denmark, Vejle, Denmark. The patients/participants provided their written informed consent to participate in this study.
Author contributions
VA and RC conceptualised the study and drafted the study protocol. SSø, HM, AN, HG, LH, JD, MJ, KA, ON, JB, BH, UH, AB, TE, JK, RC, and VA contributed to protocol development and methodology. VA and SO led the funding acquisition. HM, AB, HG, RH, TG, NP, SSa, LH, JD, CH, MJ, KA, ON, FB, JB, AB, TE, JK, SO, and SSø, participated in recruitment of patients and data collection. KA, MJ, SSø, and SO developed the data collection tool in REDCap. SSø, VA, and SO led the study co-ordination and VA the project administration. SO and RC drafted the statistical analysis plan. SO, AP, and RC performed the statistical analyses. TH analysed the FFQ. SO and RC had full access to all the data in the study and take responsibility for the integrity of the data and the accuracy of the data analysis. SO drafted the manuscript and attests that all listed authors meet authorship criteria and that no others meeting the criteria have been omitted. VA, RC, TE, JK, and SO are guarantors of the study. All authors contributed to and approved the statistical analysis plan and critically revised the manuscript for scientific content and approved the final version of the manuscript.
Funding
This project has received funding from the European Union's Horizon 2020 research and Innovation programme under grant agreement No. 733100 (https://www.syscid.eu/). The Parker Institute, Bispebjerg and Frederiksberg Hospital is supported by a core grant from the Oak Foundation (OCAY-18-774-OFIL). The project has furthermore received funding from IBD-Care (Improved diagnosis and treatment in the Region of Southern Denmark, 17/18561), the Region of Southern Denmark, University Hospital of Southern Denmark and the memorial fund of Knud and Edith Eriksen.
Acknowledgments
We thank the patients who participated in the study. We thank Caroline Margaret Moos for English proofreading of the manuscript. We also thank Nathalie Fogh Rasmussen and Laura Gregersen for proofreading and comments. We thank OPEN (Open Patient data Explorative Network, Odense University Hospital, Region of Southern Denmark) for access to OPEN Biobank and REDCap.
Conflict of interest
Author CH has received speaker fee from Takeda Pharma and Tillotts Pharma (unrelated to the present work).
The remaining authors declare that the research was conducted in the absence of any commercial or financial relationships that could be construed as a potential conflict of interest.
Publisher's note
All claims expressed in this article are solely those of the authors and do not necessarily represent those of their affiliated organizations, or those of the publisher, the editors and the reviewers. Any product that may be evaluated in this article, or claim that may be made by its manufacturer, is not guaranteed or endorsed by the publisher.
Supplementary material
The Supplementary Material for this article can be found online at: https://www.frontiersin.org/articles/10.3389/fnut.2022.985732/full#supplementary-material
References
1. Li P, Zheng Y, Chen X. Drugs for autoimmune inflammatory diseases: from small molecule compounds to anti-TNF biologics. Front Pharmacol. (2017) 8:460. doi: 10.3389/fphar.2017.00460
2. Sieper J, Poddubnyy D. Axial spondyloarthritis. Lancet. (2017) 390:73–84. doi: 10.1016/S0140-6736(16)31591-4
3. Ng SC, Shi HY, Hamidi N, Underwood FE, Tang W, Benchimol EI, et al. Worldwide incidence and prevalence of inflammatory bowel disease in the 21st century: a systematic review of population-based studies. Lancet. (2018) 390:2769–78. doi: 10.1016/S0140-6736(17)32448-0
4. Ogdie A, Weiss P. The epidemiology of psoriatic arthritis. Rheum Dis Clin North Am. (2015) 41:545–68. doi: 10.1016/j.rdc.2015.07.001
5. Parisi R, Iskandar IYK, Kontopantelis E, Augustin M, Griffiths CEM, Ashcroft DM. National, regional, and worldwide epidemiology of psoriasis: systematic analysis and modelling study. BMJ. (2020) 369:m1590. doi: 10.1136/bmj.m1590
6. Alamanos Y, Drosos AA. Epidemiology of adult rheumatoid arthritis. Autoimmun Rev. (2005) 4:130–6. doi: 10.1016/j.autrev.2004.09.002
7. Cross M, Smith E, Hoy D, Carmona L, Wolfe F, Vos T, et al. The global burden of rheumatoid arthritis: estimates from the global burden of disease 2010 study. Ann Rheum Dis. (2014) 73:1316–22. doi: 10.1136/annrheumdis-2013-204627
8. Knowles SR, Graff LA, Wilding H, Hewitt C, Keefer L, Mikocka-Walus A. Quality of life in inflammatory bowel disease: a systematic review and meta-analyses—part I. Inflamm Bowel Dis. (2018) 24:742–51. doi: 10.1093/ibd/izx100
9. Ritchlin CT, Colbert RA, Gladman DD. Psoriatic arthritis. N Engl J Med. (2017) 376:957–70. doi: 10.1056/NEJMra1505557
10. Stern RS, Nijsten T, Feldman SR, Margolis DJ, Rolstad T. Psoriasis is common, carries a substantial burden even when not extensive, and is associated with widespread treatment dissatisfaction. J Investig Dermatol Symp Proc. (2004) 9:136–9. doi: 10.1046/j.1087-0024.2003.09102.x
11. Aletaha D, Smolen JS. Diagnosis and management of rheumatoid arthritis: a review. JAMA. (2018) 320:1360–72. doi: 10.1001/jama.2018.13103
12. Digby-Bell JL, Atreya R, Monteleone G, Powell N. Interrogating host immunity to predict treatment response in inflammatory bowel disease. Nat Rev Gastroenterol Hepatol. (2020) 17:9–20. doi: 10.1038/s41575-019-0228-5
13. Nielsen OH, Ainsworth MA. Tumour necrosis factor inhibitors for inflammatory bowel disease. N Engl J Med. (2013) 369:754–62. doi: 10.1056/NEJMct1209614
14. Zaiss MM, Joyce Wu HJ, Mauro D, Schett G, Ciccia F. The gut-joint axis in rheumatoid arthritis. Nat Rev Rheumatol. (2021) 17:224–37. doi: 10.1038/s41584-021-00585-3
15. Neurath MF. Host-microbiota interactions in inflammatory bowel disease. Nat Rev Gastroenterol Hepatol. (2020) 17:76–7. doi: 10.1038/s41575-019-0248-1
16. Sikora M, Stec A, Chrabaszcz M, Knot A, Waskiel-Burnat A, Rakowska A, et al. Gut microbiome in psoriasis: an updated review. Pathogens. (2020) 9:463–77. doi: 10.3390/pathogens9060463
17. Kanda N, Hoashi T, Saeki H. Nutrition and psoriasis. Int J Mol Sci. (2020) 21:5405–24. doi: 10.3390/ijms21155405
18. Campos-Perez W, Martinez-Lopez E. Effects of short chain fatty acids on metabolic and inflammatory processes in human health. Biochim Biophys Acta Mol Cell Biol Lipids. (2021) 1866:158900. doi: 10.1016/j.bbalip.2021.158900
19. Couto MR, Gonçalves P, Magro F, Martel F. Microbiota-derived butyrate regulates intestinal inflammation: focus on inflammatory bowel disease. Pharmacol Res. (2020) 159:104947. doi: 10.1016/j.phrs.2020.104947
20. Desai MS, Seekatz AM, Koropatkin NM, Kamada N, Hickey CA, Wolter M, et al. A dietary fibre-deprived gut microbiota degrades the colonic mucus barrier and enhances pathogen susceptibility. Cell. (2016) 167:1339–1353.e21. doi: 10.1016/j.cell.2016.10.043
21. Yao CK, Muir JG, Gibson PR. Review article: insights into colonic protein fermentation, its modulation and potential health implications. Aliment Pharmacol Ther. (2016) 43:181–96. doi: 10.1111/apt.13456
22. Christensen R, Heitmann BL, Andersen KW, Nielsen OH, Sorensen SB, Jawhara M, et al. Impact of red and processed meat and fibre intake on treatment outcomes among patients with chronic inflammatory diseases: protocol for a prospective cohort study of prognostic factors and personalised medicine. BMJ Open. (2018) 8:e018166. doi: 10.1136/bmjopen-2017-018166
23. Vandenbroucke JP, von Elm E, Altman DG, Gøtzsche PC, Mulrow CD, Pocock SJ, et al. Strengthening the reporting of observational studies in epidemiology (STROBE): explanation and elaboration. Ann Intern Med. (2007) 147:W163–94. doi: 10.7326/0003-4819-147-8-200710160-00010-w1
24. Harris PA, Taylor R, Thielke R, Payne J, Gonzalez N, Conde JG. Research electronic data capture (REDCap)—a metadata-driven methodology and workflow process for providing translational research informatics support. J Biomed Inform. (2009) 42:377–81. doi: 10.1016/j.jbi.2008.08.010
25. Harris PA, Taylor R, Minor BL, Elliott V, Fernandez M, O'Neal L, et al. The REDCap consortium: building an international community of software platform partners. J Biomed Inform. (2019) 95:103208. doi: 10.1016/j.jbi.2019.103208
26. Eriksen L, Grønbæk M, Helge JW, Tolstrup JS, Curtis T. The Danish health examination survey 2007–2008 (DANHES 2007–2008). Scand J Public Health. (2011) 39:203–11. doi: 10.1177/1403494810393557
27. Køster-Rasmussen R, Siersma V, Halldorsson TI, de Fine Olivarius N, Henriksen JE, Heitmann BL. Missing portion sizes in FFQ—alternatives to use of standard portions. Public Health Nutr. (2015) 18:1914–21. doi: 10.1017/S1368980014002389
28. Felson DT, Anderson JJ, Boers M, Bombardier C, Furst D, Goldsmith C, et al. American college of rheumatology. Preliminary definition of improvement in rheumatoid arthritis. Arthritis Rheum. (1995) 38:727–35. doi: 10.1002/art.1780380602
29. Sieper J, Rudwaleit M, Baraliakos X, Brandt J, Braun J, Burgos-Vargas R, et al. The assessment of spondyloarthritis international Society (ASAS) handbook: a guide to assess spondyloarthritis. Ann Rheum Dis. (2009) 68 Suppl 2:ii1–44. doi: 10.1136/ard.2008.104018
30. Austin PC. Balance diagnostics for comparing the distribution of baseline covariates between treatment groups in propensity-score matched samples. Stat Med. (2009) 28:3083–107. doi: 10.1002/sim.3697
31. Berglund P, Heeringa S. Multiple Imputation of Missing Data Using SAS. Cary, NC: SAS Institute Inc (2014).
32. Rubin DB. Multiple Imputation for Nonresponse in Surveys. Wiley Series in Probability and Mathematical Statistics. Applied Probability and Statistics. New York, NY: Wiley (1987). p. 253. doi: 10.1002/9780470316696
33. Cao J, Zhang S. Multiple comparison procedures. JAMA. (2014) 312:543–4. doi: 10.1001/jama.2014.9440
34. Altman DG, Bland JM. Interaction revisited: the difference between two estimates. BMJ. (2003) 326:219. doi: 10.1136/bmj.326.7382.219
35. Christensen R, Bours MJL, Nielsen SM. Effect modifiers and statistical tests for interaction in randomised trials. J Clin Epidemiol. (2021) 134:174–7. doi: 10.1016/j.jclinepi.2021.03.009
36. Nguyen DL, Palmer LB, Nguyen ET, McClave SA, Martindale RG, Bechtold ML. Specialised enteral nutrition therapy in Crohn's disease patients on maintenance infliximab therapy: a meta-analysis. Therap Adv Gastroenterol. (2015) 8:168–75. doi: 10.1177/1756283X15578607
37. Chiba M, Tsuji T, Nakane K, Komatsu M. High amount of dietary fibre not harmful but favourable for Crohn's disease. Perm J. (2015) 19:58–61. doi: 10.7812/TPP/14-124
38. García-Montero C, Fraile-Martínez O, Gómez-Lahoz AM, Pekarek L, Castellanos AJ, Noguerales-Fraguas F, et al. Nutritional components in western diet vs. mediterranean diet at the gut microbiota-immune system interplay. Implications for health and disease. Nutrients. (2021) 13:699–48. doi: 10.3390/nu13020699
39. Sofi F, Cesari F, Abbate R, Gensini GF, Casini A. Adherence to mediterranean diet and health status: meta-analysis. BMJ. (2008) 337:a1344. doi: 10.1136/bmj.a1344
40. Guerreiro CS, Calado Â, Sousa J, Fonseca JE. Diet, microbiota, and gut permeability-the unknown triad in rheumatoid arthritis. Front Med. (2018) 5:349. doi: 10.3389/fmed.2018.00349
41. Stephen AM, Champ MM, Cloran SJ, Fleith M, van Lieshout L, Mejborn H, et al. Dietary fibre in Europe: current state of knowledge on definitions, sources, recommendations, intakes and relationships to health. Nutr Res Rev. (2017) 30:149–90. doi: 10.1017/S095442241700004X
42. Pedersen AN, Christensen T, Matthiessen J, Knudsen VK, Rosenlund-Sørensen M, Biltoft-Jensen A, et al. Danskernes kostvaner 2011–2013. DTU National Food Institute (2015). Available online at: www.food.dtu.dk
43. Willett W, Rockström J, Loken B, Springmann M, Lang T, Vermeulen S, et al. Food in the anthropocene: the EAT-lancet commission on healthy diets from sustainable food systems. Lancet. (2019) 393:447–92. doi: 10.1016/S0140-6736(18)31788-4
44. Cocking C, Walton J, Kehoe L, Cashman KD, Flynn A. The role of meat in the European diet: current state of knowledge on dietary recommendations, intakes and contribution to energy and nutrient intakes and status. Nutr Res Rev. (2020) 33:181–9. doi: 10.1017/S0954422419000295
45. McGonagle D, McDermott MF. A proposed classification of the immunological diseases. PLoS Med. (2006) 3:e297. doi: 10.1371/journal.pmed.0030297
46. Szekanecz Z, McInnes IB, Schett G, Szamosi S, Benko S, Szucs G. Autoinflammation and autoimmunity across rheumatic and musculoskeletal diseases. Nat Rev Rheumatol. (2021) 17:585–95. doi: 10.1038/s41584-021-00652-9
47. Sarkar MK, Tsoi LC, Gudjonsson JE. Psoriasis: a mixed autoimmune and autoinflammatory disease. Curr Opin Immunol. (2017) 49:1–8. doi: 10.1016/j.coi.2017.07.007
48. McGonagle D, Watad A, Savic S. Mechanistic immunological based classification of rheumatoid arthritis. Autoimmun Rev. (2018) 17:1115–23. doi: 10.1016/j.autrev.2018.06.001
49. Mease PJ, Karki C, Liu M, Li Y, Gershenson B, Feng H, et al. Discontinuation and switching patterns of tumour necrosis factor inhibitors (TNFis) in TNFi-naive and TNFi-experienced patients with psoriatic arthritis: an observational study from the US-based corrona registry. RMD Open. (2019) 5:e000880. doi: 10.1136/rmdopen-2018-000880
50. Pope J, Thorne JC, Haraoui BP, Psaradellis E, Sampalis J. Do patients with active RA have differences in disease activity and perceptions if anti-TNF naive vs. anti-TNF experienced? Baseline results of the optimization of adalimumab trial. Med Sci Monit. (2012) 18:Pi17–20. doi: 10.12659/MSM.883250
51. Gomez-Reino JJ, Carmona L. Switching TNF antagonists in patients with chronic arthritis: an observational study of 488 patients over a 4-year period. Arthritis Res Ther. (2006) 8:R29. doi: 10.1186/ar1881
52. Vandenbroucke JP, von Elm E, Altman DG, Gøtzsche PC, Mulrow CD, Pocock SJ, et al. Strengthening the reporting of observational studies in epidemiology (STROBE): explanation and elaboration. Int J Surg. (2014) 12:1500–24. doi: 10.1016/j.ijsu.2014.07.014
53. Cox SR, Clarke H, O'Keeffe M, Dubois P, Irving PM, Lindsay JO, et al. Nutrient, fibre, and FODMAP intakes and food-related quality of life in patients with inflammatory bowel disease, and their relationship with gastrointestinal symptoms of differing aetiologies. J Crohns Colitis. (2021) 15:2041–53. doi: 10.1093/ecco-jcc/jjab116
54. Principi M, Losurdo G, Iannone A, Contaldo A, Deflorio V, Ranaldo N, et al. Differences in dietary habits between patients with inflammatory bowel disease in clinical remission and a healthy population. Ann Gastroenterol. (2018) 31:469–73. doi: 10.20524/aog.2018.0273
Keywords: chronic inflammatory disease, biologic therapy, diet, red meat, fibre, processed meat, rheumatoid arthritis, inflammatory bowel disease
Citation: Overgaard SH, Sørensen SB, Munk HL, Nexøe AB, Glerup H, Henriksen RH, Guldmann T, Pedersen N, Saboori S, Hvid L, Dahlerup JF, Hvas CL, Jawhara M, Andersen KW, Pedersen AK, Nielsen OH, Bergenheim F, Brodersen JB, Heitmann BL, Halldorsson TI, Holmskov U, Bygum A, Christensen R, Kjeldsen J, Ellingsen T and Andersen V (2022) Impact of fibre and red/processed meat intake on treatment outcomes among patients with chronic inflammatory diseases initiating biological therapy: A prospective cohort study. Front. Nutr. 9:985732. doi: 10.3389/fnut.2022.985732
Received: 04 July 2022; Accepted: 20 September 2022;
Published: 13 October 2022.
Edited by:
Michele Barone, University of Bari Aldo Moro, ItalyReviewed by:
Pugazhendhi Srinivasan, University of Kansas Medical Center, United StatesGiuseppe Losurdo, University of Bari Medical School, Italy
Copyright © 2022 Overgaard, Sørensen, Munk, Nexøe, Glerup, Henriksen, Guldmann, Pedersen, Saboori, Hvid, Dahlerup, Hvas, Jawhara, Andersen, Pedersen, Nielsen, Bergenheim, Brodersen, Heitmann, Halldorsson, Holmskov, Bygum, Christensen, Kjeldsen, Ellingsen and Andersen. This is an open-access article distributed under the terms of the Creative Commons Attribution License (CC BY). The use, distribution or reproduction in other forums is permitted, provided the original author(s) and the copyright owner(s) are credited and that the original publication in this journal is cited, in accordance with accepted academic practice. No use, distribution or reproduction is permitted which does not comply with these terms.
*Correspondence: Silja H. Overgaard, U2lsamEuaHZpZC5vdmVyZ2FhcmRAcnN5ZC5kaw==
†These authors share last authorship