- 1Department of Nutrition and Health, Beijing Advanced Innovation Center for Food Nutrition and Human Health, China Agricultural University, Beijing, China
- 2Department of General Practice, The Third Medical Center of Chinese PLA General Hospital, Beijing, China
Background: To assess the association between 12 food groups intake and the risk of urologic cancers.
Methods: We scanned PubMed and Web of Science databases up to April 1st, 2023, and 73 publications met the inclusion criteria in the meta-analysis. We used a random effects model to estimate the summary risk ratios (RRs) and 95% confidence intervals (95% CI).
Results: In the linear dose–response meta-analysis, an inverse association was found between each additional daily 100 g of fruits [RR: 0.89, 95%CI = (0.83, 0.97)], 100 g of vegetables [RR: 0.92, 95%CI = (0.85, 0.99)], 12 g of alcohol [RR: 0.91, 95%CI = (0.88, 0.94)] and 1 cup of coffee [RR: 0.95, 95%CI = (0.83, 0.97)] intake and the risk of renal cell carcinoma. Conversely, each additional daily 100 g of red meat intake was positively associated with renal cell carcinoma [RR: 1.41, 95%CI = (1.03, 2.10)]. Inverse associations were observed between each additional daily 50 g of egg [RR: 0.73, 95%CI = (0.62, 0.87)] and each additional daily 1 cup of tea consumption and bladder cancer risk [RR: 0.97, 95%CI = (0.94, 0.99)]. There were no significant associations for nonlinear dose–response relationships between 12 food groups and urological cancers.
Conclusion: Our meta-analysis strengthens the evidence that appropriate intake of specific food groups, such as fruits, vegetables, alcohol, tea, and coffee, is associated with the risk of renal cell carcinoma or bladder cancer. More studies are required to fill the knowledge gap on the links between various food groups and urologic cancers because the evidence was less credible in this meta-analysis.
Systematic Review Registration: This study was registered on PROSPERO (CRD42022340336).
1. Introduction
Urologic cancers can occur anywhere in the kidney, bladder, renal pelvis, ureter, and urethra. Notably, renal cell carcinoma (RCC) and bladder cancer (BC) are the most common urinary system tumors in both men and women. RCC and BC incidence have been steadily increasing worldwide over the past decades (1). According to GLOBOCAN data, more than 431,000 new cases of RCC were diagnosed and more than 573,000 new cases of BC were recorded worldwide in 2020 (2). Despite the identification of several modifiable lifestyle risk factors, including excess body weight (3), hypertension (4), smoking (5), and physical inactivity (6), there has been little progress in understanding the origin of urologic cancers.
Efforts to find a connection between diet and urologic cancers have a long history in cancer research. Currently, several studies have demonstrated a strong connection between food and the risk of urologic cancers (7–10). Many dietary factors, such as total fruits, total vegetables, processed meat, and alcohol consumption, are thought to influence urologic cancers risk (11–14). However, insights from epidemical studies on the modifying effects of food consumption on urologic cancers are still controversial (15–18). Therefore, more updated and sufficient evidence is needed to address these long-controversial issues. In this meta-analysis, we aim to investigate the associations of 12 food groups with the risk of urologic cancers, evaluate the food groups’ credibility of meta-evidence on their association with urologic cancers risk, and propose optimally effective strategies for the prevention of urologic cancers.
2. Methods
The PROSPERO International Prospective Register of Systematic Reviews has acknowledged the protocol for this meta-analysis (CRD42022340336). This systematic review was developed based on the guidelines of the Preferred Reporting Items for Systematic Reviews and meta-Analyses (PRISMA) (19).
2.1. Search strategy and study selection
To investigate the association between specific food group intake and urologic cancer risk, articles published in PubMed and Web of Science before April 1st, 2023 were searched. The search was restricted to the English language. The keywords used in the search strategy are presented as search terms (Supplementary Appendix S1). To discover pertinent research more comprehensively, the electronic search method also looked through all related earlier reviews. The study inclusion criteria were as follows: (1) prospective cohort studies, case cohorts, nested case–control studies; (2) studies reported the association for at least one of the 12 food groups (fruits, vegetables, legumes, egg, dairy, fish, red meat, processed meat, sugar-sweetened beverages (SSB), alcoholic drinks, coffee, and tea) and risk of urologic cancers; (3) the authors reported the risk ratio (RR) estimates or hazard ratios (HRs) with 95% confidence intervals (CIs) or the number of urologic cancers events. Exclusion criteria were (1) studies did not report relevant exposure; (2) studies did not contain cases of urinary system cancer; (3) reviews, meta-analyses, retrospective studies, non-human studies, studies without sufficient data, case–control studies, cross-sectional designs, and interventional studies.
After screening the titles and abstracts, duplicate papers and those that did not fit the criteria for inclusion had been removed. The full-texts of the remaining records were then assessed for eligibility.
2.2. Data extraction
Our 2 reviewers (S.W. and X.Z.) independently extracted the information as follows: first author’s name, year of publication, country, cohort name, study duration (years of follow-up), sex, age, cases, sample size, exposure assessment method, outcome, type of food groups, quantity of food intake, risk estimate (most adjusted RRs or HRs with 95% CI), and covariates used for adjustment. When the same study appeared to have multiple publications, we selected the version which contains the largest sample and longest follow-up.
2.3. Risk of bias assessment
The Newcastle-Ottawa scale (NOS) was used to assess the methodological quality of prospective cohort studies included (20). It contains 8 categories relating to methodological quality: representativeness of the exposed cohort, selection of non-exposed cohort, ascertainment of exposure, demonstration that the outcome of interest was not present at the start of the study, comparability of the cohorts on the basis of the design or analysis, assessment of outcome, follow-up duration, and adequacy of follow up of cohorts. This scoring system suggests classifying the meta-evidence into three categories: low (0–3 points), moderate (4–6 points), and high (7–9 points).
2.4. Statistical analysis
We used the random effects model to calculate the pooled RR and 95% CI, and linear or non-linear dose–response analysis. The HRs reported in the included studies were considered equal to RRs. We carried out the dose–response meta-analysis using the approach suggested by Greenland and Longnecker et al. (21). The distribution of cases, person-years or non-cases, as well as the RRs with 95% CIs, were required for at least three quantitative exposure categories when we applied this method. In dose–response meta-analysis, the lowest intake category from each study was used as the reference, and the other intake categories were compared to the reference. When the exposure category was reported in the closed interval, consumption was considered as the midpoint of the interval. When the exposure category was open-ended, we assumed that its length was the same as the adjacent category.
Restricted cubic splines for each study with more than 3 quantiles of exposure were calculated to explore possible nonlinear associations. We used three fixed knots through the total range of the reported intake at 10, 50, and 90% (22, 23). Units of exposure were defined as follows: total fruits (100 g/day), total vegetables (100 g/day), legumes (100 g/day), egg (50 g/day), dairy (200 g/day), fish (100 g/day), red meat (100 g/day), processed meat (50 g/day), alcohol (12 g/day), coffee (1 cup/day), tea (1 cup/day) and SSB (1 drink/day). When the studies did not specify the quantitative amount or reported food intake as serving size only, we adopted the WCRF 2017 suggested conversions (Supplementary Table S1).
We performed subgroup analyses of potential influencing factors to discern the source of heterogeneity. If there were an adequate number of studies (n ≥ 5) available for a particular food group in the meta-analysis, subgroup analyses by geography (US, UK, Asia), sex (Male, Female, Male and female), follow-up duration (mean ≥ 10 years vs. <10 years), no of participants (≥100,000 vs. <100,000). Egger’s linear regression tests and visual inspection of funnel plots were used to evaluate publication bias (24, 25). Furthermore, we conducted sensitivity analysis by omitting one study at a time when significant publication bias (p > 0.05) or heterogeneity (I2 ≥ 50%) was detected in the results. All statistical analyses in this systemic review were performed with Stata (version 14; Stata Corp). Two-tailed was used in all tests and value of p of less than 0.05 was considered to indicate statistical significance.
2.5. Quality of meta-evidence
Two independent researchers (J.Q. and D.J.) evaluated the overall quality of the evidence using the NutriGrade scoring system (max 10 points). This tool comprises the following items: (1) risk of bias, study quality, and study limitations; (2) precision; (3) heterogeneity; (4) directness; (5) publication bias; (6) funding bias; (7) effect size; and (8) dose–response (26). Scores between 0 and 3.99, 4–5.99, 6–7.99 were categorized as very low, low, and moderate, and score between 8–10 represents good quality meta-evidence, respectively. Disagreements were settled by conversation until an agreement was achieved.
3. Results
3.1. Study characteristics
The selection of the studies and the outcomes of the literature search were reported in Figure 1. In total, the original search turned up 5,788 articles. Duplicate papers and those that did not fit the criteria for inclusion have been removed. 113 full-text articles from potentially relevant studies were further evaluated. After a full-text review, additional 41 articles were excluded (Supplementary Appendix S2). And one additional record was added through systematic review. At last, 73 publications were included in the meta-analysis. During a mean of 13.6 years of follow-up, 4,903,674 participants were documented, of which 15,666 cases were ascertained (Supplementary Table S2). We evaluated the quality of the studies and yielded an average score of 8.38. Details of quality scores for all included studies are presented in Supplementary Table S3.
3.2. Foods associated with increased urologic cancers risk
3.2.1. Red meat
Comparing extreme intake categories (0 g/d vs. 43 g/d), a positive association was observed between red meat consumption and risk of RCC [RR: 1.24, 95%CI = (1.07, 1.43)] (Figure 2; Supplementary Figure S1). A positive association was found for each additional 100 g/d of red meat consumption and risk of RCC [RR: 1.41, 95%CI = (1.03, 2.10)], but not for BC [RR: 1.09, 95%CI = (0.94, 1.27)] (Figure 3). No nonlinear dose–response relationship was found between red meat consumption and RCC (p = 0.42) (Figure 4E) or BC (p = 0.12) (Figure 5E).
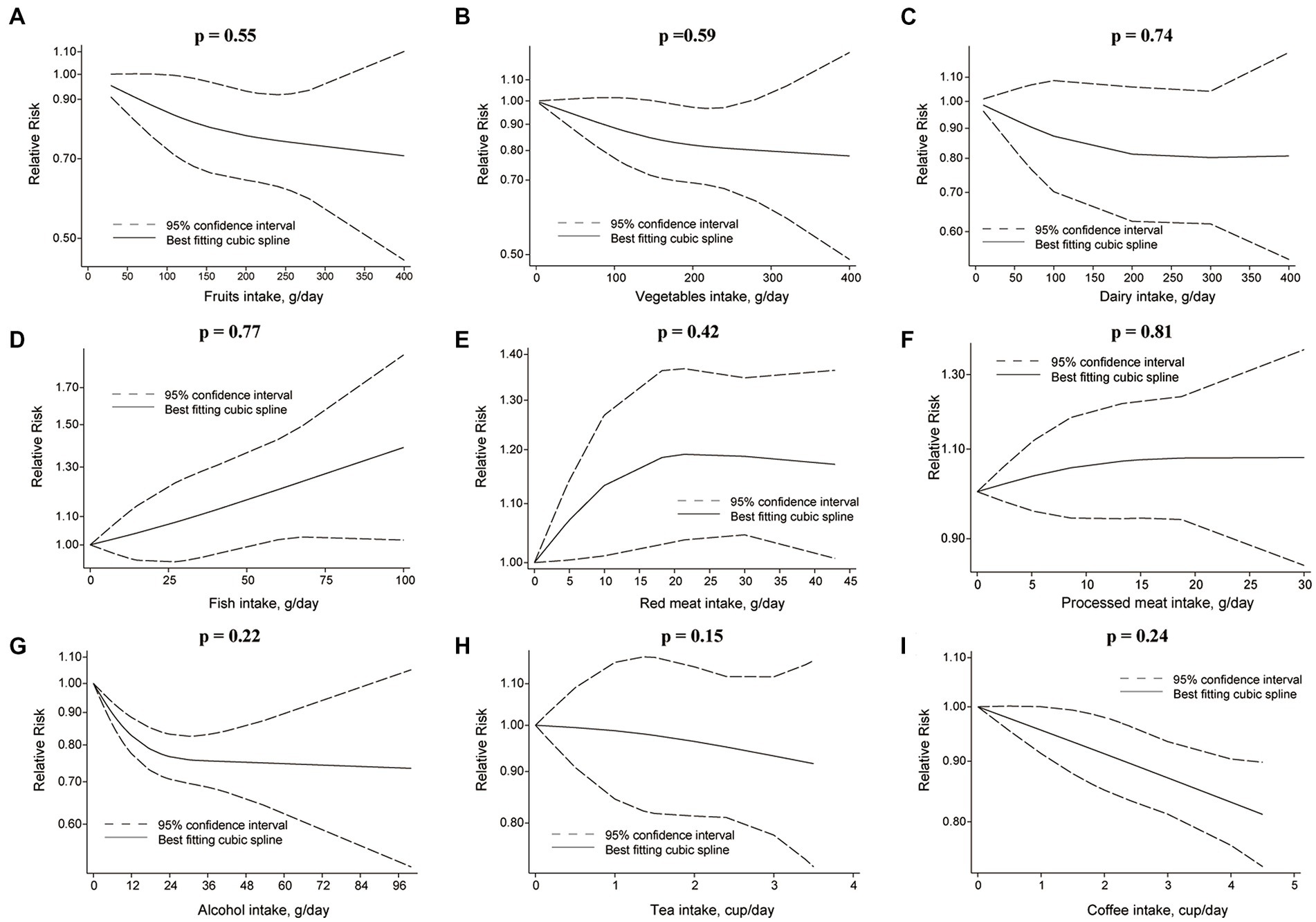
Figure 4. Non-linear dose–response relationship between food groups and risk of RCC. (A) Fruits, (B) vegetables, (C) dairy, (D) fish, (E) red meat, (F) processed meat, (G) alcohol, (H) tea, and (I) coffee.
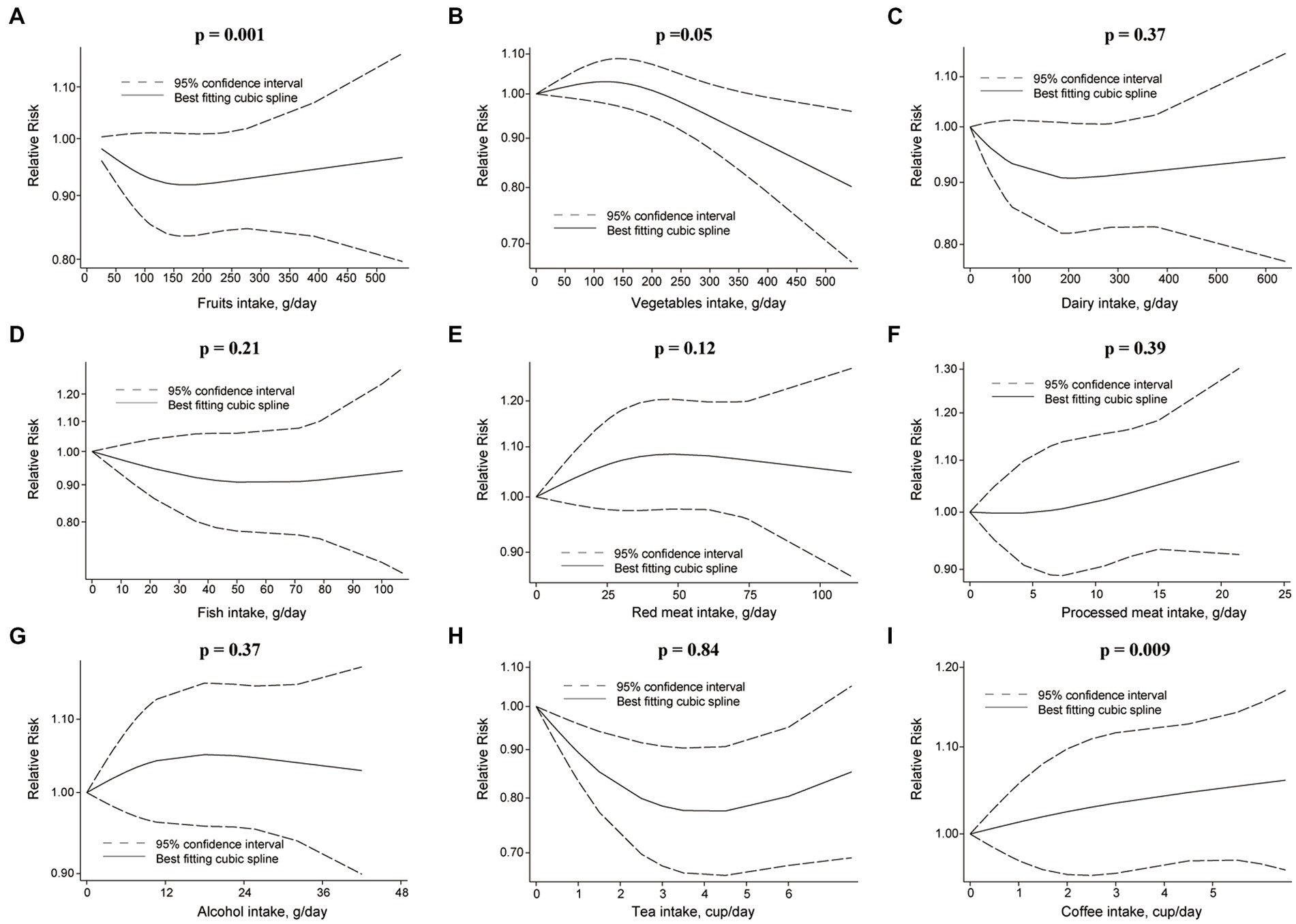
Figure 5. Non-linear dose–response relationship between food groups and risk of BC. (A) Fruits, (B) vegetables, (C) dairy, (D) fish, (E) red meat, (F) processed meat, (G) alcohol, (H) tea, and (I) coffee.
3.3. Foods associated with decreased urologic cancers risk
3.3.1. Fruits
An inverse association was observed between fruits consumption and RCC risk when extreme intake categories were compared [RR: 0.86, 95%CI = (0.77, 0.97)] (Figure 2; Supplementary Figure S2). However, we found that fruit intake did not reduce the risk of BC when comparing the highest and lowest intake categories [RR: 0.89, 95%CI = (0.76, 1.04)] (Figure 2; Supplementary Figure S4).
Each additional 100 g/d of fruits was inversely associated with RCC risk [RR: 0.89, 95%CI = (0.83, 0.97)] (Figure 3), but not for BC risk [RR: 0.98, 95%CI = (0.95, 1.01)]. Nonetheless, fruits intake and RCC risk did not appear to have a nonlinear dose–response relationship (p = 0.55) (Figure 4A). Although fruit intake and risk of RCC did appear to be associated in a non-linear dose–response manner (p = 0.001) (Figure 5A), the CI overlaps with RR = 1, so it is not a statistically significant association.
In a stratified analysis of high-category versus low-category fruit intake and BC risk, there was no indication of heterogeneity between subgroups (I2 < 50%). These differences between the subgroups were not statistically significant (p > 0.05) (Supplementary Table S6).
3.3.2. Vegetables
Comparing the highest to the lowest categories of vegetable intake, increased vegetable consumption was linked to a lower RCC risk [RR: 0.88, 95%CI = (0.79, 0.98)], but not for BC risk [RR: 0.97, 95%CI = (0.84, 1.11)] (Figure 2; Supplementary Figure S3). Additionally, vegetable intake was inversely correlated with the risk of RCC for each additional 100 g consumed daily [RR: 0.92, 95%CI = (0.85, 0.99)] (Figure 3). But each additional 100 g/d vegetable intake [RR: 0.98, 95%CI = (0.96, 1.00)] was not associated with the risk of BC (Figure 3). In stratified studies of vegetable intake and BC risk, there was no indication of heterogeneity between subgroups (Supplementary Table S7).
In the nonlinear dose–response meta-analysis, no association was observed between vegetable intake and RCC risk (p = 0.59) (Figure 4B) or BC risk (p = 0.05) (Figure 5B).
3.3.3. Alcohol
An inverse association between alcohol consumption and risk of RCC was found when comparing the highest to lowest categories [RR: 0.70, 95%CI = (0.64, 0.77)] (Figure 2; Supplemental Figure S4). No correlation between alcohol consumption and the risk of BC was seen when the highest to lowest categories are compared [RR: 1.03, 95%CI = (0.84, 1.27)] (Figure 2; Supplementary Figure S4).
The risk of RCC was inversely correlated with the additional daily 12 g of alcohol intake [RR: 0.91, 95%CI = (0.88, 0.94)] (Figure 3). But no association was found between the additional daily 12 g of alcohol intake [RR: 1.01, 95%CI = (0.97, 1.05)] and the risk of BC (Figure 4). No non-linear dose–response association between alcohol consumption and RCC risk (p = 0.22) or BC risk was found (p = 0.37) (Figure 5).
3.3.4. Tea
Comparing the highest to the lowest categories, no associations between tea intake and risk of RCC [RR: 0.97, 95%CI = (0.91, 1.17)] and BC [RR: 0.90, 95%CI = (0.70, 1.15)] were observed (Figure 2; Supplementary Figure S5).
An inverse association was observed for each additional daily 1 cup of tea and risk of BC [RR: 0.97, 95% CI = (0.94, 0.99)], but not for RCC [RR: 0.98, 95%CI = (0.92 to 1.04)] (Figure 3). There was no evidence of a non-linear dose–response association between tea and RCC risk (p = 0.15) (Figure 4H) or BC risk (p = 0.84) (Figure 5H).
No evidence of heterogeneity was detected between subgroups in stratified analyses on tea and bladder cancer (Supplementary Table S8).
3.3.5. Coffee
While comparing the highest to lowest categories, there was no association between coffee consumption and RCC risk [RR: 0.91, 95%CI = (0.80, 1.03)] or BC risk [RR: 1.04, 95%CI = (0.88, 1.21)] (Figure 2; Supplementary Figure S6). The risk of RCC decreased with each additional daily cup of coffee [RR: 0.95, 95%CI = (0.93, 0.97)] (Figure 3). There was no correlation between the risk of BC and the additional daily cup of coffee [RR: 1.00, 95%CI = (0.99, 1.01)] (Figure 3). No non-linear dose–response association between coffee consumption and BC risk was found (p = 0.009) (Figure 5I). After stratification by Geographic location, heterogeneity was observed, demonstrating a positive association between coffee consumption and incidence of BC only in research conducted in Europe [RR: 1.19, 95%CI = (1.01, 1.39)] (Supplementary Table S5).
3.4. Foods not associated with urologic cancers risk
3.4.1. Legumes
There was no association between legumes intake and risk of RCC [RR: 0.94, 95%CI = (0.69, 1.27)] or risk of BC [RR: 1.56, 95%CI = (0.79, 3.09)] when the highest and lowest categories of legumes intake were compared (Figure 2; Supplementary Figure S7). There was no correlation between each additional daily intake of 100 g of legumes and the risk of RCC [RR: 1.15, 95%CI = (0.49, 2.67)] or BC [RR: 0.80, 95%CI = (0.60, 1.06)] (Figure 3). Due to the limited availability of the data, non-linear dose–response meta-analysis was not applicable.
3.4.2. Egg
No correlation between egg intake and risk of RCC [RR: 1.20, 95%CI = (0.60, 2.40)] or risk of BC [RR: 0.77, 95%CI = (0.57, 1.05)] was observed when comparing the highest to lowest categories of egg consumption (Figure 2; Supplementary Figure S8). An inverse association was found between each additional daily 50 g of egg consumption and the risk of BC [RR: 0.73, 95%CI = (0.62, 0.87)] (Figure 3). Due to the scarcity of data in prospective cohort studies, it was not possible to analyze the non-linear dose–response relationship between egg intake and urological cancers.
3.4.3. Dairy
There was no association between dairy intake and the risk of RCC [RR: 0.96, 95%CI = (0.68, 1.35)], or BC [RR: 0.93, 95%CI = (0.80, 1.07)] when the highest and lowest categories of dairy intake were compared (Figure 2; Supplementary Figure S9). Each additional 200 g of dairy consumption daily did not affect the risk of RCC [RR: 0.87, 95%CI = (0.73, 1.03)] or BC [RR: 0.96, 95%CI = (0.91, 1.01)] (Figure 3). There was also no evidence of a non-linear dose–response relationship between dairy consumption and RCC risk (p = 0.74) (Figure 4C) or BC risk (p = 0.37) (Figure 5C). In stratified analyses of dairy consumption and BC risk, no significant evidence of heterogeneity was found between subgroups (Supplementary Table S9).
3.4.4. Fish
Comparing the highest to the lowest categories, no association between fish intake and RCC risk [RR: 1.11, 95%CI = (0.92, 1.33)] or BC risk [RR: 0.79, 95% CI = (0.56, 1.11)] was observed (Figure 2; Supplementary Figure S10). Each additional daily 100 g of fish intake was not associated with the risk of RCC [RR: 1.37, 95% CI = (0.96, 1.78)] or BC [RR: 0.88, 95% CI = (0.70, 1.11)] (Figure 3).
There was no evidence of a non-linear dose–response association between fish intake and RCC risk (p = 0.77) (Figure 4D), or BC risk (p = 0.21) (Figure 5D). Subgroup analyses showed evidence of heterogeneity, for the prospective cohort studies in America and studies with ≥100,000 participants, a high intake of fish has been linked to a significantly lower risk of BC (Supplementary Table S4).
3.4.5. Processed meat
There was no significant association between processed meat consumption and the risk of RCC [RR: 1.11, 95%CI = (0.96, 1.28)], or BC [RR: 1.05, 95%CI = (0.92, 1.18)] (Figure 2; Supplementary Figure S11). Each additional 50 g of processed meat consumed daily was not associated with a higher risk of RCC [RR: 1.17, 95%CI = (0.83, 1.66)], or BC [RR: 1.20, 95%CI = (0.83, 1.76)] (Figure 3). There was no nonlinear dose–response relationship between processed meat intake and RCC risk (p = 0.81) (Figure 4F), or BC risk (p = 0.39) (Figure 5F).
3.4.6. Sugar-sweetened beverages
There was no association between SSB intake and the risk of RCC [RR: 1.05, 95%CI = (0.89, 1.24)] or BC [RR: 0.79, 95%CI = (0.44, 1.43)] when the highest and lowest categories were compared (Figure 2; Supplementary Figure S12). In addition, dose–response meta-analysis was not possible due to a lack of data availability.
3.5. Publication bias and sensitivity analysis
Based on the funnel plot (Supplementary Figures S13–S17) and Egger’s test, there was no publication bias for alcohol intake and risk of RCC (p = 0.848, n = 12 studies), fruit (p = 0.402, n = 14 studies), vegetables (p = 0.469, n = 13 studies), tea (p = 0.186, n = 10 studies), coffee (p = 0.748, n = 16 studies) intake for BC. In the influence analysis in which we excluded one study from high versus low meta-analysis with high heterogeneity (I2 ≥ 50%), in turn, the summary estimates were not substantially altered for all of the exposures (fruits, vegetables, legumes, dairy, fish, tea, and coffee) (Supplementary Figures S18–S24).
3.6. Quality of evidence
We graded and assessed the quality of meta-evidence regarding the association between food groups and the risk of RCC and BC. In our results, the classification of RCC’s NutriGrade meta-evidence was given as follows: “high” for alcohol, “moderate” for fruits, vegetables, red meat, and coffee, and “low” for the other seven food groups (Table 1). The NutriGrade grading on BC was rated “moderate” for fruits, vegetables, tea, and coffee, and “low” for the 8 other food groups (Table 2).
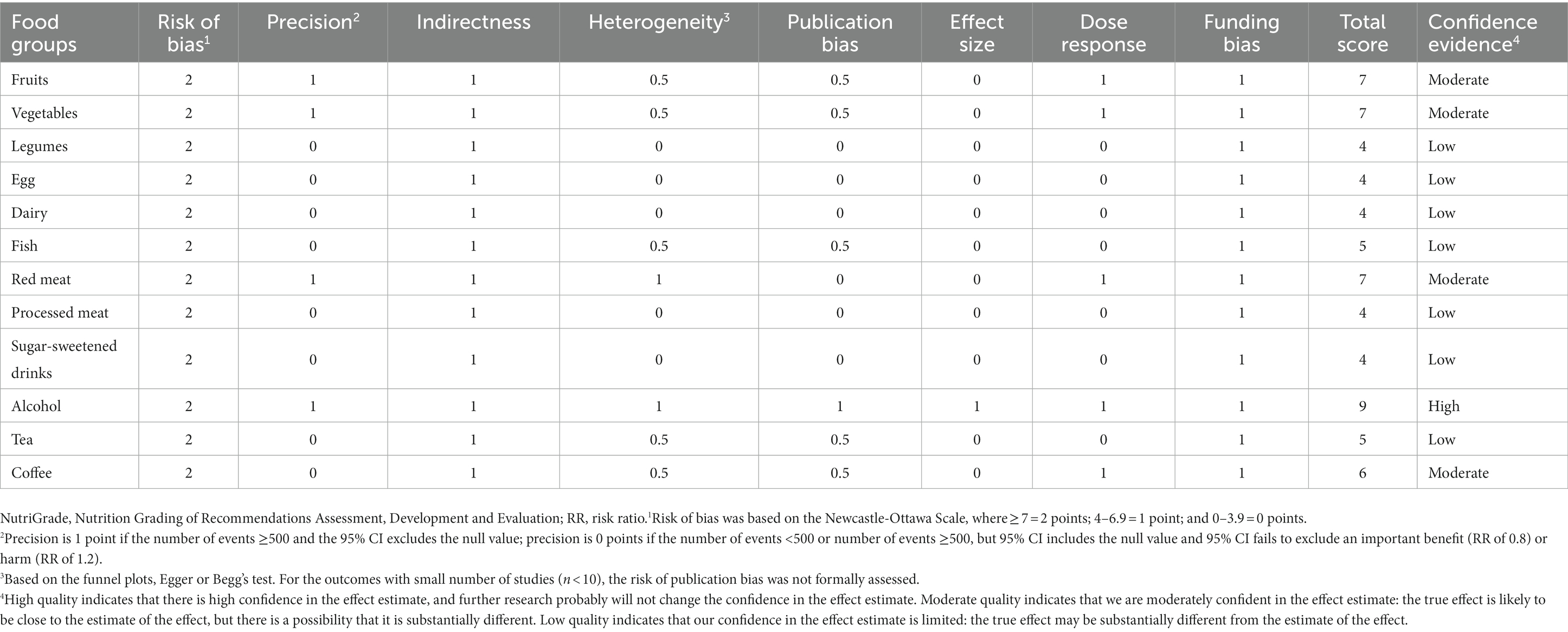
Table 1. NutriGrade assessment of confidence in estimate effect of studies evaluated the association between various food groups and risk of RCC.
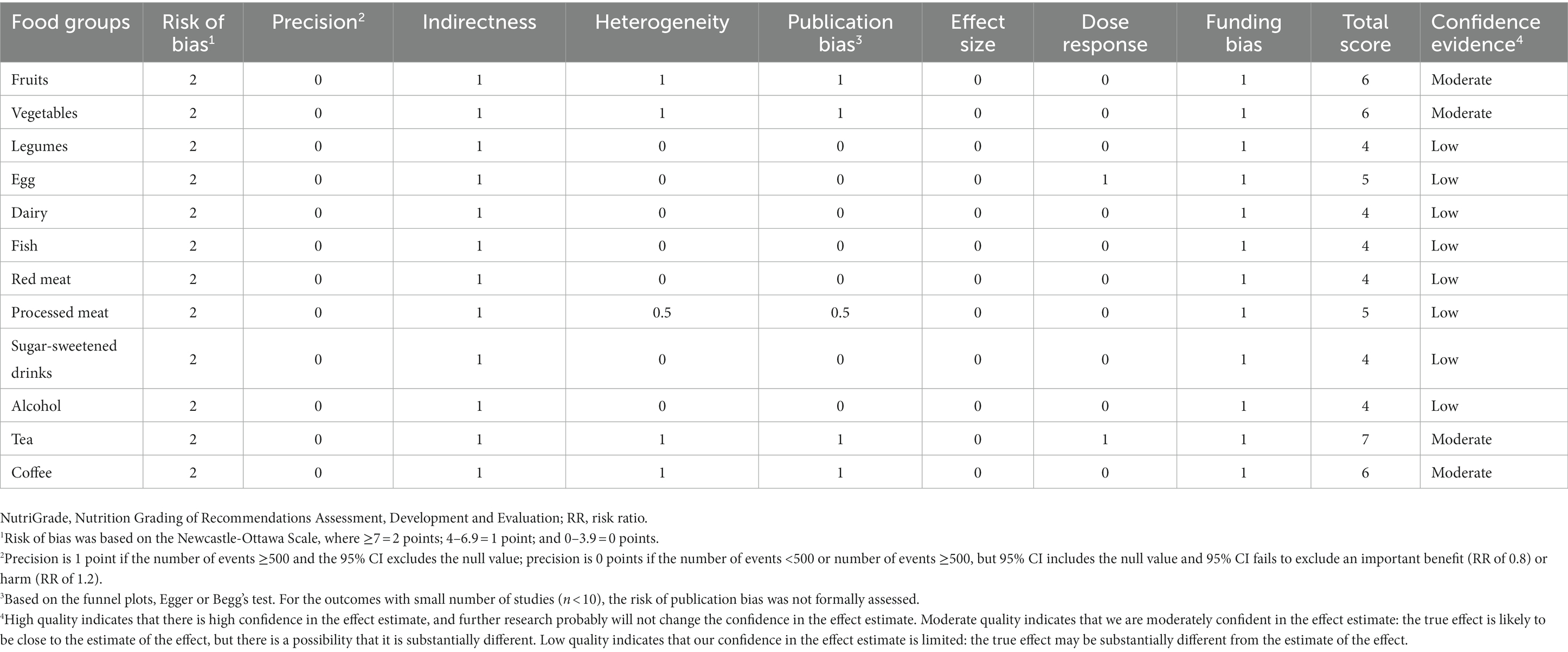
Table 2. NutriGrade assessment of confidence in estimate effect of studies evaluated the association between various food groups and risk of BC.
4. Discussion
4.1. Principal findings
In this study, we evaluated the associations of targeted food groups—fruits, vegetables, legumes, egg, dairy, fish, red meat, processed meat, SSB, alcohol, tea, and coffee—and the risk of RCC and BC. First, we found that fruits, vegetables, and alcohol were associated with a decreased risk of RCC in the high versus low meta-analysis, while red meat was associated with an increased risk of RCC. Second, in the linear dose–response meta-analysis, an inverse association was found between fruits, vegetables, alcohol, coffee intake and risk of RCC, and a positive association was found between red meat intake and RCC. Differently, tea consumption was negatively associated with the risk of BC. At last, there were no indications for nonlinear dose–response relationships between preselected food groups intake and risk of RCC and BC.
The NutriGrade tool suggested a high confidence in the estimate of the alcohol intake and risk of RCC, and moderate confidence in the estimate of the effect of fruits, vegetables, tea, and coffee intake for the risk of RCC. The NutriGrade tool for BC was classified as “moderate” for fruits, vegetables, tea, and coffee, and the confidence for other food groups was lower.
4.2. Strengths of the study
The study has some advantages. First, the article is the first meta-analysis that includes all the available prospective cohort studies to estimate the connection between food groups and the risk of urologic cancers.
Of note, we only included urologic cancers that occur in both men and women, which minimizes gender differences. Furthermore, we performed various types of analyses that enable us to thoroughly identify the associations between food groups and urological cancers and found an ideal intake with the lowest risk. This meta-analysis included prospective studies only, the recall bias was successfully avoided, and the likelihood of selection bias was decreased (27). Additionally, the overall quality of evidence is further ensured by the Newcastle-Ottawa scale assessment (8.38 on average).
4.3. Comparison with other studies
Our findings are consistent with earlier meta-analyses that showed an inverse association between fruits and vegetable intake and RCC risk (28–32). While other research indicates that the incidence of urologic cancers is not associated with overall fruit and vegetable consumption (11–13, 33–37). The base of the dietary pyramid, fruits and vegetables, contain many compounds that may prevent cancers, yet it is challenging to determine the proportional value of each component. Any preventative impact could most likely be attributed to a confluence of actions on many carcinogenesis-related pathways. Numerous antioxidant elements (including carotenoids and vitamin C), minerals, dietary fiber, phenols, flavonoids, and phytochemicals are present in many fruits and vegetables, which may influence the processes governing cell proliferation and death (38). These processes are assumed to be primarily responsible for the association between consuming fruits and vegetables and a decreased risk of urological cancers. For example, cruciferous vegetables have high levels of glucosinolates, which are converted into isothiocyanates by the enzyme myrosinase during food preparation and change the way carcinogens are metabolized, which could decrease the risk of cancers (39).
No association was found in our meta-analysis between dairy consumption and RCC and BC risk. Similarly, previous studies found no association between BC risk and milk consumption (40, 41), whereas some previous meta-analyses suggested dairy (such as milk) consumption is positively associated with the risk of BC (42–44). Calcium, vitamins, and protein are the elements found in dairy, which may benefit human health (45). Suggestions on dairy consumption to urologic cancers cannot yet be made due to conflicting results.
In this meta-analysis, we found a statistically significant positive association between red meat intake and RCC. A positive association has previously been reported between red meat consumption and the risk of RCC (46, 47), while some meta-analyses found no associations between red meat intake and the risk of RCC (48). The difference could be explained by the inclusion of prospective studies only. However, we observed no associations between red meat and processed meat intake and the risk of BC. Meat plays a significant role in human nutrition because it offers high-quality protein as well many vital minerals, including iron, zinc, and vitamin B12 (49). Nevertheless, a high intake of red and processed meat is associated with an increased risk for diseases. A recent study has found the consumption of Neu5Gc (N-Glycolylneuraminic acid) from red meat increases the risk of cancers (50). On the cell surface of the majority of mammals, Neu5Gc exists naturally. Due to the inactivation of the gene encoding CMP-N-acetylneuraminic acid hydroxylase, it is not present in human tissues. Whenever people eat too much red meat, Neu5Gc enters cells, where the immune system recognizes it as a foreign threat and produces antibodies to destroy it. Repeated consumption of these meats will trigger this immune response, leading to long-term chronic inflammation and an increased risk of tumor formation (51, 52).
The association between alcohol intake and the risk of BC is not consistent. A meta-analysis reported that in males, alcohol may increase the risk of BC in a dose-independent manner (53), whereas another study reported that there is no material relationship between high levels of alcohol consumption and BC risk (17). No significant association was found between alcohol consumption and BC risk in our results, which is consistent with previous meta-analyses (54). However, there was a statistically significant and persuasive relationship between alcohol and RCC. The findings from our meta-analysis support the previous hypothesis that alcoholic beverage intake is inversely associated with risk of RCC (17, 55, 56).
There are various theories as to how alcohol may lower the risk of kidney cancer, but the exact processes remain elusive: (1) Moderate alcohol consumption is linked to lower rates of type 2 diabetes and hyperinsulinemia, which may be risk factors for kidney cancer; (2) Antioxidant phenolic substances that can prevent cell cycle progression and reduce oxidative stress may be present in alcoholic beverages (57, 58); (3) The diuretic impact of alcohol, which increases urine volume and shortens exposure times, is another theory that might apply (59). (4) It is worth noting that the relationship between alcohol intake and RCC risk would seem to be influenced by inter-individual germline variation in alcohol-metabolizing genes (60). Taken together, to corroborate our findings, further investigate other particular demographic groups, and identify possible regulator genes or biomarkers based on molecular epidemiology, additional high-quality studies should be carried out.
Our findings did not support the conclusion that tea consumption is related to a decreased risk of RCC, which is consistent with previous studies (61, 62). Our findings of an inverse correlation between tea consumption and BC were indeed consistent with the findings of several previous studies (63, 64). Studies in animals have demonstrated that some tea constituents may have a restraining effect on BC development (65). This inhibitory activity is believed to be primarily due to the antioxidative and possibly antiproliferative effects of polyphenol compounds (such as epigallocatechin gallate), through inhibition of metabolic or signal-transduction pathways (66, 67).
In our meta-analysis, there was no significant statistical association between coffee drinking and BC risk, and no correlation was detected in earlier cohort studies (68–70). Our results support that the consumption of coffee is associated with a reduced risk of RCC. Results from previous analyses provide evidence of the benefit of caffeinated coffee (71), while other studies demonstrate no significant association between coffee consumption and RCC (72). According to epidemiological research, coffee consumption was inversely associated with the risk of several cancers (73). Many studies have suggested mechanisms by which coffee intake reduces RCC risk. For example, the presence of phytochemicals, such as caffeine, chlorogenic acid, and caffeic acid, may be responsible for enhanced insulin sensitivity (74). Furthermore, convincing evidence showed that being overweight may increase the risk of developing RCC (75). However, caffeine may enhance energy balance by suppressing appetites, raising basal metabolic rate, and stimulating food-induced thermogenesis to regulate weight (76). The exact mechanism by which obesity raises the risk of RCC is uncertain, but the accumulation of body fat directly affects insulin levels in the body, thereby elevating the probability of hypertension, both of which are strongly associated with the development of RCC (77, 78).
The high versus low meta-analysis and dose–response analysis revealed no association between legumes and the risk of RCC or BC. To support our findings, additional well-designed prospective studies will be required, taking into account the limitations of the included research (69). In addition to being an excellent source of fiber, legumes also contain certain bioactive substances, which are peptides formed from proteins that have been shown in in vitro experiments to have antioxidant effects (79). We observed an inverse association between egg consumption and BC risk, which is different from previous meta-analyses (80, 81). This inconsistency could be explained by the inclusion of new studies. Egg yolks contain accessible xanthophyll carotenoids, which have anti-inflammatory and antioxidant properties and may be able to prevent cancers (82). Taking into account the limitations of the included studies and the low credibility of meta-evidence confidence, this result should be considered with caution. In our meta-analysis, no association was found between fish consumption and RCC risk or BC risk. Similarly, a previous cohort study has found no association between BC risk and fish consumption (83). On the contrary, an investigation reported a beneficial effect (84). Multiple studies have suggested that ω-3 fatty acids which is abundant in fatty fish may have a reducing influence on the chance of developing cancer (84–86). Further well-designed prospective studies are required to further investigate the impact of fish on RCC and BC due to the dearth of studies in this area.
4.4. Limitations of the study
Unfortunately, this study has some technical and biological limitations. First, substantial heterogeneity exists in some analyses, which could not be further explored due to the limited number of studies. The heterogeneity between studies was not completely omitted after subgroup analysis, and the interpretation of the findings should be done with caution. Second, food frequency questionnaires, which rely on recall are a common source for estimates of food category intake. Therefore, measurement errors seemed inevitable and can lead to the misclassification of exposures. In addition, since the food categories in each food questionnaire were not fully standardized, the food items in our meta-analysis were only counted according to broad categories, and the completeness and credibility of this analysis would be higher if more detailed and standardized food categories were available in the future. Of note, sex is a significant factor in epidemiological studies, but the data were not sufficient to support analyses by sex of the meta-analysis. As a result, some of these results need to be evaluated carefully. Therefore, it is meaningful to design more effective and comprehensive prospective cohort studies to investigate associations between food groups and urological cancers risk.
5. Conclusion
Overall, our meta-analysis collectively tends to show some correlation between food group intake and urological cancers. We found that a high intake of red meat increases the risk of RCC. High fruit, vegetable, alcohol, and coffee intake may play a protective role against RCC. A high intake of tea may decrease the risk of BC. For urethral cancer and renal pelvis carcinoma, the number of related studies is too small to support meta-analysis. In summary, these findings may contribute to developing food-based dietary recommendations for preventing urologic cancers.
Author contributions
JQ: conceptualization, investigation, data curation, software, and writing–original draft. PA: conceptualization and methodology. DJ: data curation and writing–original draft preparation. YJ: visualization and investigation. SW and XZ: software and validation. YL: writing-reviewing and funding acquisition. JL: writing–reviewing and editing. CZ: supervision and project administration. All authors contributed to the article and approved the submitted version.
Funding
This work was supported by the Beijing Advanced Innovation Center for Food Nutrition and Human Health, the National Natural Science Foundation of China (31970717 and 82170429), the Chinese Universities Scientific Fund (2020TC015), and the Beijing Municipal Natural Science Foundation (7222111).
Conflict of interest
The authors declare that the research was conducted in the absence of any commercial or financial relationships that could be construed as a potential conflict of interest.
Publisher’s note
All claims expressed in this article are solely those of the authors and do not necessarily represent those of their affiliated organizations, or those of the publisher, the editors and the reviewers. Any product that may be evaluated in this article, or claim that may be made by its manufacturer, is not guaranteed or endorsed by the publisher.
Supplementary material
The Supplementary material for this article can be found online at: https://www.frontiersin.org/articles/10.3389/fnut.2023.1154996/full#supplementary-material
References
1. Usher-Smith, J, Simmons, RK, Rossi, SH, and Stewart, GD. Current evidence on screening for renal cancer. Nat Rev Urol. (2020) 17:637–42. doi: 10.1038/s41585-020-0363-3
2. Sung, H, Ferlay, J, Siegel, RL, Laversanne, M, Soerjomataram, I, Jemal, A, et al. Global cancer statistics 2020: GLOBOCAN estimates of incidence and mortality worldwide for 36 cancers in 185 countries. CA Cancer J Clin. (2021) 71:209–49. doi: 10.3322/caac.21660
3. Landberg, A, Fält, A, Montgomery, S, Sundqvist, P, and Fall, K. Overweight and obesity during adolescence increases the risk of renal cell carcinoma. Int J Cancer. (2019) 145:1232–7. doi: 10.1002/ijc.32147
4. Makino, T, Izumi, K, Iwamoto, H, Kadomoto, S, Naito, R, Yaegashi, H, et al. The impact of hypertension on the clinicopathological outcome and progression of renal cell carcinoma. Anticancer Res. (2020) 40:4087–93. doi: 10.21873/anticanres.14407
5. Patel, NH, Attwood, KM, Hanzly, M, Creighton, TT, Mehedint, DC, Schwaab, T, et al. Comparative analysis of smoking as a risk factor among renal cell carcinoma histological subtypes. J Urol. (2015) 194:640–6. doi: 10.1016/j.juro.2015.03.125
6. Xiao, Q, Liao, L, Matthews, CE, Chow, W-H, Davis, F, Schwartz, K, et al. Physical activity and renal cell carcinoma among black and white Americans: a case-control study. BMC Cancer. (2014) 14:707. doi: 10.1186/1471-2407-14-707
7. Chyou, P-H, Nomura, AMY, and Stemmermann, GN. A prospective study of diet, smoking, and lower urinary tract cancer. Ann Epidemiol. (1993) 3:211–6. doi: 10.1016/1047-2797(93)90021-U
8. Greenwald, P, Clifford, CK, and Milner, JA. Diet and cancer prevention. Eur J Cancer. (2001) 37:948–65. doi: 10.1016/s0959-8049(01)00070-3
9. Grieb, SMD, Theis, RP, Burr, D, Benardot, D, Siddiqui, T, and Asal, NR. Food groups and renal cell carcinoma: results from a case-control study. J Am Diet Assoc. (2009) 109:656–67. doi: 10.1016/j.jada.2008.12.020
10. Macleod, LC, Hotaling, JM, Wright, JL, Davenport, MT, Gore, JL, Harper, J, et al. Risk factors for renal cell carcinoma in the VITAL study. J Urol. (2013) 190:1657–61. doi: 10.1016/j.juro.2013.04.130
11. Bertoia, M, Albanes, D, Mayne, ST, Männistö, S, Virtamo, J, and Wright, ME. No association between fruit, vegetables, antioxidant nutrients and risk of renal cell carcinoma. Int J Cancer. (2010) 126:1504–12. doi: 10.1002/ijc.24829
12. Rashidkhani, B, Lindblad, P, and Wolk, A. Fruits vegetables and risk of renal cell carcinoma: a prospective study of Swedish women. Int J Cancer. (2005) 113:451–5. doi: 10.1002/ijc.20577
13. Lee, JE, Männistö, S, Spiegelman, D, Hunter, DJ, Bernstein, L, van den Brandt, PA, et al. Intakes of fruit, vegetables, and carotenoids and renal cell cancer risk: a pooled analysis of 13 prospective studies. Cancer Epidemiol Biomark Prev Publ Am Assoc Cancer Res Cosponsored Am Soc Prev Oncol. (2009) 18:1730–9. doi: 10.1158/1055-9965.EPI-09-0045
14. Michaud, DS, Spiegelman, D, Clinton, SK, Rimm, EB, Willett, WC, and Giovannucci, EL. Fruit and vegetable intake and incidence of bladder cancer in a male prospective cohort. JNCI J Natl Cancer Inst. (1999) 91:605–13.
15. Bellocco, R, Pasquali, E, Rota, M, Bagnardi, V, Tramacere, I, Scotti, L, et al. Alcohol drinking and risk of renal cell carcinoma: results of a meta-analysis. Ann Oncol. (2012) 23:2235–44. doi: 10.1093/annonc/mds022
16. Cheng, G, and Xie, L. Alcohol intake and risk of renal cell carcinoma: a meta-analysis of published case-control studies. Arch Med Sci AMS. (2011) 7:648–57. doi: 10.5114/aoms.2011.24135
17. Pelucchi, C, Galeone, C, Tramacere, I, Bagnardi, V, Negri, E, Islami, F, et al. Alcohol drinking and bladder cancer risk: a meta-analysis. Ann Oncol. (2012) 23:1586–93. doi: 10.1093/annonc/mdr460
18. Song, DY, Song, S, Song, Y, and Lee, JE. Alcohol intake and renal cell cancer risk: a meta-analysis. Br J Cancer. (2012) 106:1881–90. doi: 10.1038/bjc.2012.136
19. Moher, D, Liberati, A, Tetzlaff, J, and Altman, DG, PRISMA Group. Preferred reporting items for systematic reviews and meta-analyses: the PRISMA statement. PLoS Med. (2009) 6:e1000097. doi: 10.1371/journal.pmed.1000097
20. Stang, A . Critical evaluation of the Newcastle-Ottawa scale for the assessment of the quality of nonrandomized studies in meta-analyses. Eur J Epidemiol. (2010) 25:603–5. doi: 10.1007/s10654-010-9491-z
21. Greenland, S, and Longnecker, MP. Methods for trend estimation from summarized dose-response data, with applications to meta-analysis. Am J Epidemiol. (1992) 135:1301–9. doi: 10.1093/oxfordjournals.aje.a116237
22. DerSimonian, R, and Laird, N. Meta-analysis in clinical trials. Control Clin Trials. (1986) 7:177–88. doi: 10.1016/0197-2456(86)90046-2
23. Harrell, FE, Lee, KL, and Pollock, BG. Regression models in clinical studies: determining relationships between predictors and response. J Natl Cancer Inst. (1988) 80:1198–202.
24. Higgins, JPT, and Thompson, SG. Quantifying heterogeneity in a meta-analysis. Stat Med. (2002) 21:1539–58. doi: 10.1002/sim.1186
25. Egger, M, Davey Smith, G, Schneider, M, and Minder, C. Bias in meta-analysis detected by a simple, graphical test. BMJ. (1997) 315:629–34. doi: 10.1136/bmj.315.7109.629
26. Schwingshackl, L, Knüppel, S, Schwedhelm, C, Hoffmann, G, Missbach, B, Stelmach-Mardas, M, et al. Perspective: NutriGrade: a scoring system to assess and judge the meta-evidence of randomized controlled trials and cohort studies in nutrition research. Adv Nutr Int Rev J. (2016) 7:994–1004. doi: 10.3945/an.116.013052
27. Colditz, GA . Overview of the epidemiology methods and applications: strengths and limitations of observational study designs. Crit Rev Food Sci Nutr. (2010) 50:10–2. doi: 10.1080/10408398.2010.526838
28. Huang, T, Ding, P, Chen, J, Yan, Y, Zhang, L, Liu, H, et al. Dietary fiber intake and risk of renal cell carcinoma: evidence from a meta-analysis. Med Oncol Northwood Lond Engl. (2014) 31:125. doi: 10.1007/s12032-014-0125-2
29. Liu, B, Mao, Q, Wang, X, Zhou, F, Luo, J, Wang, C, et al. Cruciferous vegetables consumption and risk of renal cell carcinoma: a meta-analysis. Nutr Cancer. (2013) 65:668–76. doi: 10.1080/01635581.2013.795980
30. Tang, L, Zirpoli, GR, Guru, K, Moysich, KB, Zhang, Y, Ambrosone, CB, et al. Consumption of raw cruciferous vegetables is inversely associated with bladder cancer risk. Cancer Epidemiol Biomark Prev Publ Am Assoc Cancer Res Cosponsored Am Soc Prev Oncol. (2008) 17:938–44. doi: 10.1158/1055-9965.EPI-07-2502
31. Zhang, S, Jia, Z, Yan, Z, and Yang, J. Consumption of fruits and vegetables and risk of renal cell carcinoma: a meta-analysis of observational studies. Oncotarget. (2017) 8:27892–903. doi: 10.18632/oncotarget.15841
32. Zhao, J, and Zhao, L. Cruciferous vegetables intake is associated with lower risk of renal cell carcinoma: evidence from a meta-analysis of observational studies. PLoS One. (2013) 8:e75732. doi: 10.1371/journal.pone.0085403
33. Vieira, AR, Vingeliene, S, Chan, DSM, Aune, D, Abar, L, Navarro Rosenblatt, D, et al. Fruits, vegetables, and bladder cancer risk: a systematic review and meta-analysis. Cancer Med. (2015) 4:136–46. doi: 10.1002/cam4.327
34. Xenou, D, Tzelves, L, Terpos, E, Stamatelopoulos, K, Sergentanis, TN, and Psaltopoulou, T. Consumption of fruits, vegetables and bladder cancer risk: a systematic review and meta-analysis of prospective cohort studies. Nutr Cancer. (2021):1–14. doi: 10.1080/01635581.2021.1985146
35. Xu, C, Zeng, X-T, Liu, T-Z, Zhang, C, Yang, Z-H, Li, S, et al. Fruits and vegetables intake and risk of bladder cancer: a PRISMA-compliant systematic review and dose-response meta-analysis of prospective cohort studies. Medicine (Baltimore). (2015) 94:e759. doi: 10.1097/MD.0000000000002357
36. Yu, EY-W, Wesselius, A, Mehrkanoon, S, Goosens, M, Brinkman, M, van den Brandt, P, et al. Vegetable intake and the risk of bladder cancer in the bladder cancer epidemiology and nutritional determinants (BLEND) international study. BMC Med. (2021) 19:56. doi: 10.1186/s12916-021-01931-8
37. Weikert, S, Boeing, H, Pischon, T, Olsen, A, Tjonneland, A, Overvad, K, et al. Fruits and vegetables and renal cell carcinoma: findings from the European prospective investigation into cancer and nutrition (EPIC). Int J Cancer. (2006) 118:3133–9. doi: 10.1002/ijc.21765
38. Sacerdote, C, Matullo, G, Polidoro, S, Gamberini, S, Piazza, A, Karagas, MR, et al. Intake of fruits and vegetables and polymorphisms in DNA repair genes in bladder cancer. Mutagenesis. (2007) 22:281–5. doi: 10.1093/mutage/gem014
39. Riso, P, Brusamolino, A, Moro, M, and Porrini, M. Absorption of bioactive compounds from steamed broccoli and their effect on plasma glutathione S-transferase activity. Int J Food Sci Nutr. (2009) 60:56–71. doi: 10.1080/09637480802089751
40. Acham, M, Wesselius, A, van Osch, FHM, Yu, EY-W, van den Brandt, PA, White, E, et al. Intake of milk and other dairy products and the risk of bladder cancer: a pooled analysis of 13 cohort studies. Eur J Clin Nutr. (2020) 74:28–35. doi: 10.1038/s41430-019-0453-6
41. Li, F, An, S, Zhou, Y, Liang, Z, Jiao, Z, Jing, Y, et al. Milk and dairy consumption and risk of bladder cancer: a meta-analysis. Urology. (2011) 78:1298–305. doi: 10.1016/j.urology.2011.09.002
42. Bermejo, LM, López-Plaza, B, Santurino, C, Cavero-Redondo, I, and Gómez-Candela, C. Milk and dairy product consumption and bladder cancer risk: a systematic review and meta-analysis of observational studies. Adv Nutr Bethesda Md. (2019) 10:S224–38. doi: 10.1093/advances/nmy119
43. Mao, Q-Q, Dai, Y, Lin, Y-W, Qin, J, Xie, L-P, and Zheng, X-Y. Milk consumption and bladder cancer risk: a meta-analysis of published epidemiological studies. Nutr Cancer. (2011) 63:1263–71. doi: 10.1080/01635581.2011.614716
44. Wu, J, Yu, Y, Huang, L, Li, Z, Guo, P, and Xu, YW. Dairy product consumption and bladder cancer risk: a meta-analysis. Nutr Cancer. (2020) 72:377–85. doi: 10.1080/01635581.2019.1637909
45. Mozaffarian, D . Dietary and policy priorities for cardiovascular disease, diabetes, and obesity: a comprehensive review. Circulation. (2016) 133:187–225. doi: 10.1161/CIRCULATIONAHA.115.018585
46. Faramawi, MF, Johnson, E, Fry, MW, Sall, M, Zhou, Y, and Yi, Z. Consumption of different types of meat and the risk of renal cancer: meta-analysis of case-control studies. Cancer Causes Control CCC. (2007) 18:125–33. doi: 10.1007/s10552-006-0104-9
47. Zhang, S, Wang, Q, and He, J. Intake of red and processed meat and risk of renal cell carcinoma: a meta-analysis of observational studies. Oncotarget. (2017) 8:77942–56. doi: 10.18632/oncotarget.18549
48. Lee, JE, Spiegelman, D, Hunter, DJ, Albanes, D, Bernstein, L, van den Brandt, PA, et al. Fat, protein, and meat consumption and renal cell cancer risk: a pooled analysis of 13 prospective studies. JNCI J Natl Cancer Inst. (2008) 100:1695–706. doi: 10.1093/jnci/djn386
49. Geissler, C, and Singh, M. Iron, meat and health. Nutrients. (2011) 3:283–316. doi: 10.3390/nu3030283
50. Bashir, S, Fezeu, LK, Leviatan Ben-Arye, S, Yehuda, S, Reuven, EM, Szabo de Edelenyi, F, et al. Association between Neu5Gc carbohydrate and serum antibodies against it provides the molecular link to cancer: French NutriNet-Santé study. BMC Med. (2020) 18:262. doi: 10.1186/s12916-020-01721-8
51. Wolk, A . Potential health hazards of eating red meat. J Intern Med. (2017) 281:106–22. doi: 10.1111/joim.12543
52. Ziouziou, I, Shariat, SF, Ajdi, F, and Khabbal, Y. Association of processed meats and alcohol consumption with renal cell carcinoma: a worldwide population-based study. Nutr Cancer. (2021) 73:2665–70. doi: 10.1080/01635581.2020.1856388
53. Lao, Y, Li, X, He, L, Guan, X, Li, R, Wang, Y, et al. Association between alcohol consumption and risk of bladder cancer: a dose-response meta-analysis of prospective cohort studies. Front Oncol. (2021) 11:696676. doi: 10.3389/fonc.2021.696676
54. Mao, Q, Lin, Y, Zheng, X, Qin, J, Yang, K, and Xie, L. A meta-analysis of alcohol intake and risk of bladder cancer. Cancer Causes Control CCC. (2010) 21:1843–50. doi: 10.1007/s10552-010-9611-9
55. Antwi, SO, Eckel-Passow, JE, Diehl, ND, Serie, DJ, Custer, KM, Wu, KJ, et al. Alcohol consumption, variability in alcohol dehydrogenase genes and risk of renal cell carcinoma. Int J Cancer. (2018) 142:747–56. doi: 10.1002/ijc.31103
56. Halliwell, B . Free radicals, antioxidants, and human disease: curiosity, cause, or consequence? Lancet Lond Engl. (1994) 344:721–4.
57. Vartolomei, MD, Iwata, T, Roth, B, Kimura, S, Mathieu, R, Ferro, M, et al. Impact of alcohol consumption on the risk of developing bladder cancer: a systematic review and meta-analysis. World J Urol. (2019) 37:2313–24. doi: 10.1007/s00345-019-02825-4
58. Kk, NCS, and Ar, F. Evaluation of dietary, medical and lifestyle risk factors for incident kidney cancer in postmenopausal women. Int J Cancer. (2004) 108:11532. doi: 10.1002/ijc.11532
59. Zhang, H, and Fu, L. The role of ALDH2 in tumorigenesis and tumor progression: Targeting ALDH2 as a potential cancer treatment. Acta Pharm Sin B. (2021) 11:1400–11. doi: 10.1016/j.apsb.2021.02.008
60. Hu, Z-H, Lin, Y-W, Xu, X, Chen, H, Mao, Y-Q, Wu, J, et al. No association between tea consumption and risk of renal cell carcinoma: a meta-analysis of epidemiological studies. Asian Pac J Cancer Prev APJCP. (2013) 14:1691–5. doi: 10.7314/apjcp.2013.14.3.1691
61. Lee, JE, Hunter, DJ, Spiegelman, D, Adami, H-O, Bernstein, L, van den Brandt, PA, et al. Intakes of coffee, tea, milk, soda and juice and renal cell cancer in a pooled analysis of 13 prospective studies. Int J Cancer. (2007) 121:2246–53. doi: 10.1002/ijc.22909
62. Al-Zalabani, AH, Wesselius, A, Yi-Wen, YE, van den Brandt, P, Grant, EJ, White, E, et al. Tea consumption and risk of bladder cancer in the Bladder Cancer Epidemiology and Nutritional Determinants (BLEND) Study: Pooled analysis of 12 international cohort studies. Clin Nutr Edinb Scotl. (2022) 41:1122–30. doi: 10.1016/j.clnu.2022.03.020
63. Wu, S, Li, F, Huang, X, Hua, Q, Huang, T, Liu, Z, et al. The association of tea consumption with bladder cancer risk: a meta-analysis. Asia Pac J Clin Nutr. (2013) 22:128–37. doi: 10.6133/apjcn.2013.22.1.15
64. Xu, X-Y, Zhao, C-N, Cao, S-Y, Tang, G-Y, Gan, R-Y, and Li, H-B. Effects and mechanisms of tea for the prevention and management of cancers: An updated review. Crit Rev Food Sci Nutr. (2020) 60:1693–705. doi: 10.1080/10408398.2019.1588223
65. Zhang, Y-F, Xu, Q, Lu, J, Wang, P, Zhang, H-W, Zhou, L, et al. Tea consumption and the incidence of cancer: a systematic review and meta-analysis of prospective observational studies. Eur J Cancer Prev. (2015) 24:353–62. doi: 10.1097/CEJ.0000000000000094
66. Zhao, J, Blayney, A, Liu, X, Gandy, L, Jin, W, Yan, L, et al. EGCG binds intrinsically disordered N-terminal domain of p53 and disrupts p53-MDM2 interaction. Nat Commun. (2021) 12:986. doi: 10.1038/s41467-021-21258-5
67. Dai, Z-W, Cai, K-D, Li, F-R, Wu, X-B, and Chen, G-C. Association between coffee consumption and risk of bladder cancer in a meta-analysis of 16 prospective studies. Nutr Metab. (2019) 16:66. doi: 10.1186/s12986-019-0390-3
68. Huang, T, Guo, Z, Zhang, X, Zhang, X, Liu, H, Geng, J, et al. Coffee consumption and urologic cancer risk: a meta-analysis of cohort studies. Int Urol Nephrol. (2014) 46:1481–93. doi: 10.1007/s11255-014-0699-9
69. Yu, EYW, Dai, Y, Wesselius, A, van Osch, F, Brinkman, M, van den Brandt, P, et al. Coffee consumption and risk of bladder cancer: a pooled analysis of 501,604 participants from 12 cohort studies in the Bladder Cancer Epidemiology and Nutritional Determinants (BLEND) international study. Eur J Epidemiol. (2020) 35:523–35. doi: 10.1007/s10654-019-00597-0
70. Antwi, SO, Eckel-Passow, JE, Diehl, ND, Serie, DJ, Custer, KM, Arnold, ML, et al. Coffee consumption and risk of renal cell carcinoma. Cancer Causes Control CCC. (2017) 28:857–66. doi: 10.1007/s10552-017-0913-z
71. Wijarnpreecha, K, Thongprayoon, C, Thamcharoen, N, Panjawatanan, P, and Cheungpasitporn, W. Association between coffee consumption and risk of renal cell carcinoma: a meta-analysis. Intern Med J. (2017) 47:1422–32. doi: 10.1111/imj.13621
72. van Dam, RM, Hu, FB, and Willett, WC. Coffee, Caffeine, and Health. Campion EW, editor. N Engl J Med. (2020) 383:369–78. doi: 10.1056/NEJMra1816604
73. van Dam, RM, and Hu, FB. Coffee consumption and risk of type 2 diabetes: a systematic review. JAMA. (2005) 294:97–104. doi: 10.1001/jama.294.1.97
74. Tabrizi, R, Saneei, P, Lankarani, KB, Akbari, M, Kolahdooz, F, Esmaillzadeh, A, et al. The effects of caffeine intake on weight loss: a systematic review and dose-response meta-analysis of randomized controlled trials. Crit Rev Food Sci Nutr. (2019) 59:2688–96. doi: 10.1080/10408398.2018.1507996
75. Rini, BI, Campbell, SC, and Escudier, B. Renal cell carcinoma. Lancet. (2009) 373:14. doi: 10.1016/S0140-6736(09)60229-4
76. Abdullah, A, Peeters, A, de Courten, M, and Stoelwinder, J. The magnitude of association between overweight and obesity and the risk of diabetes: a meta-analysis of prospective cohort studies. Diabetes Res Clin Pract. (2010) 89:309–19. doi: 10.1016/j.diabres.2010.04.012
77. Hall, JE, Brands, MW, and Henegar, JR. Mechanisms of hypertension and kidney disease in obesity. Ann N Y Acad Sci. (1999) 892:91–107. doi: 10.1111/j.1749-6632.1999.tb07788.x
78. Malaguti, M, Dinelli, G, Leoncini, E, Bregola, V, Bosi, S, Cicero, AFG, et al. Bioactive peptides in cereals and legumes: agronomical, biochemical and clinical aspects. Int J Mol Sci. (2014) 15:21120–35. doi: 10.3390/ijms151121120
79. Fang, D, Tan, F, Wang, C, Zhu, X, and Xie, L. Egg intake and bladder cancer risk: A meta-analysis. Exp Ther Med. (2012) 4:906–12. doi: 10.3892/etm.2012.671
80. Li, F, Zhou, Y, Hu, R, Hou, L, Du, Y-J, Zhang, X, et al. Egg consumption and risk of bladder cancer: a meta-analysis. Nutr Cancer. (2013) 65:538–46. doi: 10.1080/01635581.2013.770041
81. Clayton, ZS, Fusco, E, and Kern, M. Egg consumption and heart health: A review. Nutr Burbank Los Angel Cty Calif. (2017) 37:79–85. doi: 10.1016/j.nut.2016.12.014
82. Li, Z, Yu, J, Miao, Q, Sun, S, Sun, L, Yang, H, et al. The association of fish consumption with bladder cancer risk: a meta-analysis. World J Surg Oncol. (2011) 9:107. doi: 10.1186/1477-7819-9-107
83. Dianatinasab, M, Wesselius, A, de Loeij, T, Salehi-Abargouei, A, Yu, EYW, Fararouei, M, et al. The association between meat and fish consumption and bladder cancer risk: a pooled analysis of 11 cohort studies. Eur J Epidemiol. (2021) 36:781–92. doi: 10.1007/s10654-021-00762-4
84. Fernandez, E, Chatenoud, L, La Vecchia, C, Negri, E, and Franceschi, S. Fish consumption and cancer risk. Am J Clin Nutr. (1999) 70:85–90. doi: 10.1093/ajcn/70.1.85
85. Ullah, H, Liu, G, Yousaf, B, Ali, MU, Abbas, Q, Munir, MAM, et al. Developmental selenium exposure and health risk in daily foodstuffs: a systematic review and meta-analysis. Ecotoxicol Environ Saf. (2018) 149:291–306. doi: 10.1016/j.ecoenv.2017.11.056
Keywords: urologic cancers, foods, prospective cohort, meta-analysis, dose–response
Citation: Qi J, An P, Jin D, Ji Y, Wan S, Zhang X, Luo Y, Luo J and Zhang C (2023) Food groups and urologic cancers risk: a systematic review and meta-analysis of prospective studies. Front. Nutr. 10:1154996. doi: 10.3389/fnut.2023.1154996
Edited by:
Raul Zamora-Ros, Institut d'Investigacio Biomedica de Bellvitge (IDIBELL), SpainReviewed by:
Alicia Heath, Imperial College London, United KingdomCaroline Sigman, CCS Associates, United States
Copyright © 2023 Qi, An, Jin, Ji, Wan, Zhang, Luo, Luo and Zhang. This is an open-access article distributed under the terms of the Creative Commons Attribution License (CC BY). The use, distribution or reproduction in other forums is permitted, provided the original author(s) and the copyright owner(s) are credited and that the original publication in this journal is cited, in accordance with accepted academic practice. No use, distribution or reproduction is permitted which does not comply with these terms.
*Correspondence: Yongting Luo, bHVvLnlvbmd0aW5nQGNhdS5lZHUuY24=; Junjie Luo, bHVvampAY2F1LmVkdS5jbg==; Chengying Zhang, emhhbmdjaHkxOTY5QDEyNi5jb20=
†These authors have contributed equally to this work