- 1Department of Physics, Norwegian University of Science and Technology, Trondheim, Norway
- 2Geosciences Rennes (UPR 4661), Centre National de la Recherche Scientifique, Universite de Rennes 1, Rennes, France
- 3Polytec Research Institute, Haugesund, Norway
We propose a mapping from fracture systems consisting of intersecting fracture sheets in three dimensions to an abstract network consisting of nodes and links. This makes it possible to analyze fracture systems with the methods developed within modern network theory. We test the mapping for two-dimensional geological fracture outcrops and find that the equivalent networks are small-world and dissasortative. By analyzing the Discrete Fracture Network model, which is used to generate artificial fracture outcrop networks, we also find small world networks. However, the networks turn out to be assortative.
1. Introduction
Topological analysis of networks has had an explosive growth over the last decade (Barabási and Stanley, 2003). A large number of new concepts and quantitive tools for describing networks have been introduced, making it possible to describe and classify complex network structures at a level that never earlier has been achieved (Albert and Barabási, 2002; Boccaletti et al., 2006). There is one class, though, of networks that has resisted this kind of analysis: Fracture networks. These consist of intersecting fracture sheets, making both the concepts of links and nodes far from obvious. Fracture networks, however, are extremely important from a technological point of view. For example, in carbonate petroleum reservoirs, the oil is transported through fracture networks as the permeability of the porous matrix is too low (Van Golf-Racht, 2007). Another example is the extraction of shale gas though hydrofracturing (Mooney, 2011).
We propose a transformation from fracture network to an equivalent network consisting of nodes and links. This makes it possible to qualitatively and quantitatively characterize the topology of fracture networks.
An important consequence of this is that it is possible to compare models that generate artificial networks with real networks quantitatively.
Fracture outcrop networks have been studied from a network point of view by Valentini et al. (2007a,b). Fracture outcrops are fracture lines visible on the surfaces of geological formations. The outcrop fracture lines are one-dimensional cuts through the two-dimensional fracture sheets. Valentini et al. treats fracture lines as links and their crossing points as nodes. This gives a more narrow degree distribution than the transform proposed in this paper. However, Valentini et al. also conclude that fracture networks are small-world networks (Valentini et al., 2007a). In three dimensions where the fractures are sheets, the transformation we propose is necessary to define the topology network.
Our analysis is somewhat related to the information measure for cities introduced by Rosvall et al. (2005).
We analyze in the following fracture data from eight outcrops found in south-east Sweden. A detailed description of the bedrock composition and geological history are given in (Darcel et al., 2006; Åström, 2007; Ström et al., 2008). We show one of the outcrop fracture networks in Figure 1A. As we shall see, the equivalent network (shown in Figure 1B) constructed from the original network has small-world character. Furthermore, it is disassortative.
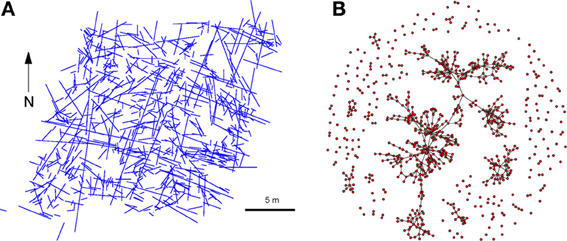
Figure 1. (A) Fracture network of outcrop AMS000025 (B) Equivalent network based on the original network shown in (A).
We then go on to analyze artificial fracture networks generated with the Discrete Fracture Network (DFN) model (Darcel et al., 2003). Examples of DFN generated fracture systems are given in Figure 2. The equivalent networks constructed from the original networks generated by this model also show small-world behavior. However, they are assortative.
2. Method
The eight outcrops covers between 250 and 600 m2. All visible fractures with length over 0.5 m have been recorded in the data sets. We prepare the data sets as follows. When tracing the fracture lines, they may appear disconnected or doubled due to topography or ground weathering. An illustration of a outcrop is shown in Figure 3A. We therefore use a reconnection procedure (Darcel et al., 2009). That is, we first project fracture traces on a flat surface to reduce the perturbation due to rock surface topography. Then scattered segments that are likely to belong to the same trace are reconnected to one single segment accounting for orientation and distance consistency. We focus on traces with a dashed-line, disconnected step or layered patterns. We then straighten all the fractures lines. The result is shown in Figure 3B.
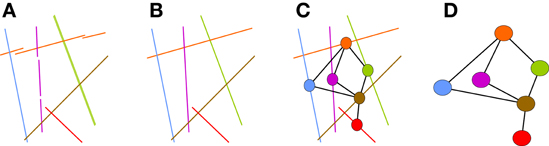
Figure 3. Clock-wise from upper left. (A) Representation of fracture outcrop network. (B) Reconnected fracture network. (C) Equivalent network placed on top of fracture outcrop network. (D) Equivalent network representation of (B).
We have now come to the central idea of this paper. In Figure 3C, each fracture line has been associated with a node. Whenever two fracture lines cross, we place a link between the nodes representing the two fracture lines. In Figure 3D, we show the equivalent network consisting of nodes representing the fracture lines and links representing crossing fracture lines (Andresen, 2008).
We note that this equivalent network is as simple to construct in a three-dimensional system of fracture sheets: each fracture sheet is represented by a node and whenever two sheets cross each other, a link is placed between the equivalent nodes.
3. Results
Arguably the most central property of any complex network is the degree distribution P(k). The degree, k, of a node is the number of other nodes that it is linked to. The equivalent networks generated from the outcrop networks show a broad degree distribution. We plot the cumulative distribution, P(>k) in Figure 4. When P(k) follows a power-law the network is scale free (Albert and Barabási, 2002). We plot the data on a log-log scale and fit the average to the power law P(k) ~ k−2.2. The scaling ensues over one decade. We note, however, as shown in the insert, that with such a short range, an exponential fit would also match. In the case of the DFN data the goodness of the power law fit is dependent on model parameters.
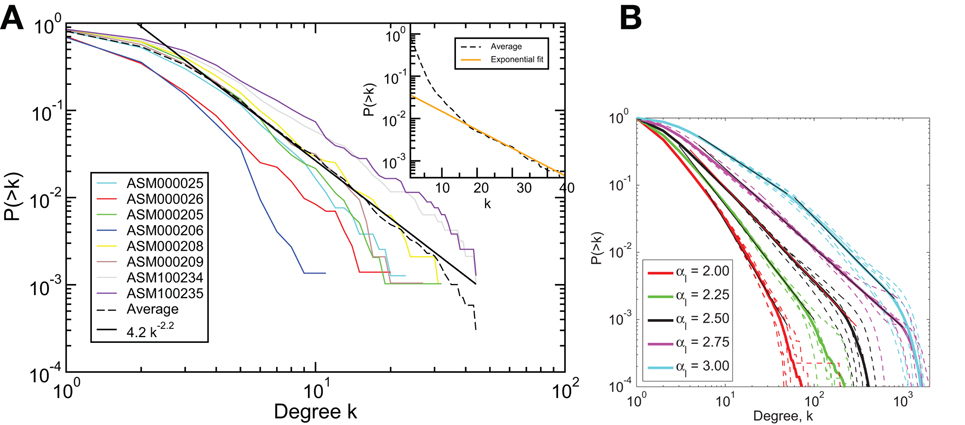
Figure 4. Cumulative degree distribution P(>k) for: (A) Networks generated from the eight outcrop data sets. Insert shows the average compared to a power law fit (exponent −2.3) and an exponential fit. (B) DFN model. Values for the expoents of the fits are given in Table 2.
The clustering is a local measure of how well a network is connected on a local neighbor-to-neighbor scale. The global clustering coefficient, C, is defined (Watts and Strogatz, 1998; Newman, 2003) as the average over all the local clustering coefficients, Ci, for each node
where ki is the degree of node i, N is the total number of nodes and ENN, i is the number of links between the nearest neighbors of node i. The clustering coefficient falls in the interval 0 ≤ C ≤ 1, and a high value indicates that there is a high chance that two neighbors of a node is connected to each other. This makes the network highly connected on a local scale, making it easy for nodes to efficiently interact on this scale.
In order to determine whether the clustering coefficients found for the networks are large for their number of nodes and links, we compare them to rewired and random versions of the same networks. In rewiring (Mathias and Gopal, 2001) two pairs of connected nodes are selected at random, and the links interchanged so that two new pairs of connected nodes are created. The procedure is repeated until all links are moved. This preserves the degree distribution since all nodes retain their initial degree, but it removes any correlation between the degrees of the connected nodes. For the random version all links are removed and redistributed randomly between the nodes. This produces a new degree distribution that is generally not broad. In all cases the quoted values for these networks are averaged over 1000 realizations. As can be seen from Table 1, the equivalent networks have an average clustering coefficient of 0.18 which is more than an order of magnitude larger than for comparable rewired networks, and two orders of magnitude larger than for purely random versions. Hence, they are well connected on a local scale.
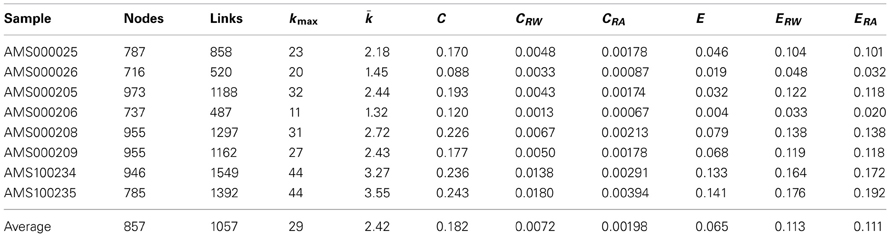
Table 1. List of the number of nodes (fractures), links, maximum degree kmax, average degree , clustering coefficient C, clustering coefficient for rewired networks CRW, clustering coefficient for random networks CRA, efficiency E, efficiency for rewired networks ERW, and efficiency for random networks ERA for all the outcrop samples.
The efficiency, E, is a global measure for how well the different parts of the network are connected, and how easily nodes in different parts of the network can interact. The measure is defined using the shortest distance, dij, between two nodes i and j (Boccaletti et al., 2006)
where dij = ∞ if node i and j are not connected. E falls in the interval 0 ≤ E ≤ 1, and a high value indicates that it is easy for nodes far apart in the network to interact since there on average is just a few links between any two nodes.
In Table 1 we present E for all the equivalent networks and their average is 0.065, which is smaller than for the rewired (ERW) and random (ERA) versions both having an average of 0.11. However the efficiency (E) is only smaller by a factor of about 2, making E and ERW/RA of the same order. We would expect the rewired and random networks to have a high efficiency, several orders of magnitude larger than ordered networks, because they have a large portion of long-range links. The fact that the equivalent networks have an efficiency comparable to that of the rewired and random versions means that compared to ordered networks they have a large efficiency. We will discuss the impact of C and E for the equivalent networks in more detail below.
It is also interesting to study any correlations between the degrees of linked nodes. Does high degree nodes link predominantly to low degree nodes or high degree nodes? (Maslov and Sneppen, 2002) introduced a correlation matrix
where P(k1, k2) is the probability that a node of degree k1 is linked to a node of degree k2 for the network to be investigated. PR(k1, k2) is the same probability of a rewired version of the network. If C(k1, k2) = 1 for all (k1, k2) then there is no degree correlations in the linking between nodes. If C(k1, k2) > 1 for some values of (k1, k2) then there is an over-representation of links between nodes of degree k1 and k2 in the investigated network compared to that of a rewired version of the network. If C(k1, k2) < 1 there is an under-representation. Note that the matrix C(k1, k2) is symmetric.
In Figure 5 we have plotted the average of the matrix C(k1, k2) for all outcrops, where PR(k1, k2) is averaged over 10000 realizations. We observe an over-representation of small degree nodes linking to higher degree nodes, and an under-representation of equal degree nodes linking to each other. Such networks are disassortative, and are abundant in naturally occurring networks (Amaral et al., 2004; Hansen and Hansen, 2007).
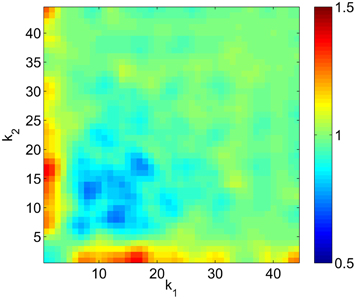
Figure 5. Plot of the correlation matrix C(k1, k2) based on the equivalent networks generated from the eigth outcrop fracture data sets.
The characteristic path length, L is defined as the average distance between any pair of nodes of a network,
Having a large clustering coefficient indicates a large local connectivity, and a small characteristic path length indicates a large global connectivity. When both of these criteria are fulfilled, we have a small-world network (Watts and Strogatz, 1998). Networks consisting of more than one disjoint part will have dij = ∞ for at least one pair of nodes. Hence, the characteristic path length is not a good measure for the global connectivity of such networks. However a small value of dij for most pairs of nodes will give a large average value for 1/dij which is measured by the efficiency. Therefore a large E is comparable to a small L for describing the global connectedness. Since the fracture networks found in the outcrops have been shown to have a clustering coefficient significantly larger than rewired and random versions, and an efficiency of the same order as the rewired and random networks we conclude that these are small-world networks.
We now turn to analyzing the DFN model (Darcel et al., 2003). It is based on the observation that the length of fracture lines in outcrops, l, are distributed according to a power law (Renshaw, 1999; Bonnet et al., 2001)
with αl typically in the range 1.7–3.2 (Bonnet et al., 2001). The outcrops can be divided into two groups: one with αl = 3 (ASM000205 and ASM000206) and one with αl = 2.3± 0.2 (the rest) (Davy et al., 2010). The angular distribution of the directions of the fractures depends on stress history of the fracture system. We assume here the simplest, i.e., a uniform distribution. (Åström, 2007) has measured the angle distribution for some of the data sets we consider here. They are not uniformly distributed. However, there are clear correlations between fracture length and direction in the data sets. This is not captured by a single angle distribution function. Introducing a non-uniform angle distribution in the DFN model does not have a significant impact. Lastly, the position of the fractures must be specified. The DFN model uses a hierarchical construction (Schertzer and Lovejoy, 1987; Meakin, 1991) to place the midpoints of the fractures on a fractal set characterized by a fractal dimension D2. D2 lies for natural fracture in the range 1.5 to 2.0 (Bonnet et al., 2001). The outcrop data has D2 ≈ 2 Åström (2007). In order to generate the fractal set on which the midpoints of the fracture lines are placed, one uses the hierarchical algorithm described by Schertzer and Lovejoy (1987) and (Meakin, 1991): Starting with a square, this is divided into l2 subsections. Each subsection is assigned a probability Pi (i.e. a number between 0 and 1) where i runs from 1 to l2. This procedure is then repeated for each subsection, which is divided into l2 sub-subsections, and new probabilities Pi are assigned to each sub-subsection. Repeating this procedure n times splits the original square into l2n subsquares, each chracterized by Pi1Pi2… Pin giving the probability that a fracture line midpoint is placed in that subsquare. By tuning the probabilitites Pi, the desired fractal dimension D2 is set, see (Davy et al., 2010) for details.
The results of analyzing the equivalent networks of the DFN model networks are given in Table 2. The data are based on 1000 networks of comparable size to those in the outcrop fracture data sets. From the table, we see the same trends as those observed in Table 1 for the eight Outcrop fracture data sets and it is possible to find a combination of αl and D2 to make match between them. However, we show in Figure 6 the averaged degree correlation matrix. This indicates an assortative network structure: nodes of equal coordination number tend to be connected. This is the opposite of what is observed for the outcrop data sets, see Figure 5. Hence, the topology of the artificial networks is quite different from the natural ones. This implies that the topology of the fracture network themselves, artificial and real, are quite different. This difference is not visible from direct observation.

Table 2. List of degree distribution power-law exponents αk, clustering coefficient C, clustering coefficient for rewired networks CRW, clustering coefficient for comparable random networks CRA, efficiency E, efficiency for rewired networks ERW, and efficiency for comparable random networks ERA for various fracture length power-law exponents αl.
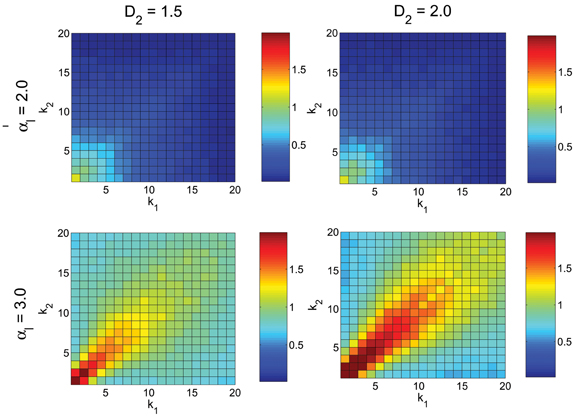
Figure 6. The degree correlation matrix C(k1, k2) for different DFN model parameters αl and D2. This figure should be compared with Figure 5.
In the study by Åström (2007), the outcrop data set is compared with models based on the physical process behind the network. The analysis is performed not on the dual network, but on the fracture network directly. Åström concludes that there are significant discrepancies between the models and the data. These discrepancies are geometrical rather than topological in contrast to the present analysis.
Hence, by constructing the equivalent networks, we have access to the entire analysis toolbox of modern network theory for fracture networks. As we have shown in the analysis presented, this makes it possible to test fracture network models on a quantitative level beyond what has been possible earlier.
Conflict of Interest Statement
The authors declare that the research was conducted in the absence of any commercial or financial relationships that could be construed as a potential conflict of interest.
Acknowledgments
We thank Svensk Kärnbränslehantering AB for outcrop data and H. F. Hansen and E. Skjetne for discussions. Christian André Andresen and Alex Hansen thank Statoil and The Norwegian Academy of Science and Letters for funding through their VISTA program. Sigmund Mongstad Hope and Alex Hansen thank the Norwegian Research Council for funding through the CLIMIT program, grant no. 199970. We also thank the referees for having improved our paper considerably.
Footnotes
- ^A kink in the slope around k = 60 gives 0.9 when fitting for smaller values of k and 1.3 for larger values.
References
Albert, R., and Barabási, A. L. (2002). Statistical mechanics of complex networks. Rev. Mod. Phys. 74:47. doi: 10.1103/RevModPhys.74.47
Amaral, L. A. N., Barrat, A., Barabasi, A. L., Caldarelli, G., De Los Rios, P., Erzan, A., Kahng, B., Mantegna, R., Mendes, J. F. F., Pastor-Satorras, R., Vespignani, A., (2004). Virtual round table on ten leading questions for network research. Eur. Phys. J. B 38, 143�145. doi: 10.1140/epjb/e2004-00109-x
Andresen, C. A. (2008). Properties of Fracture Networks and Other Network Systems. Ph.D. thesis, NTNU, Trondheim.
Åström, J. A. (2007). Statistical analysis of shear cracks on rock surfaces. Eur. Phys. J. B 56, 291–295. doi: 10.1140/epjb/e2007-00138-y
Boccaletti, S., Latora, V., Moreno, Y., Chavez, M., and Hwang, D. U. (2006). Complex networks: structure and dynamics. Phys. Rep. 424, 175–308. doi: 10.1016/j.physrep.2005.10.009
Bonnet, E., Bour, O., Odling, N. E., and Davy, P. (2001). Scaling of fracture systems in geological data. Rev. Geophys. 39, 347–383. doi: 10.1029/1999RG000074
Darcel, C., Bour, O., Davy, P., and de Dreuzy, J. R. (2003). Connectivity properties of two-dimensional fracture networks with stochastic fractal correlation. Water Res. 39:1272. doi: 10.1029/2002WR001628
Darcel, C., Davy, P., Bour, O., and de Dreuzy, J. R. (2006). Discrete fracture network for the forsmark site. Swed. Nucl. Fuel Waste Manage. R-06-79.
Darcel, C., Davy, P., Le Goc, R., de Dreuzy, J. R., and Bour, O. (2009). Statistical methodology for discrete fracture model-including fracture size, orientation uncertainty together with intensity uncertainty and variability. Swed. Nucl. Fuel Waste Manage. R-09-38.
Davy, P., Le Goc, R., Darcel, C., Bour, O., de Dreuzy, J. R., and Munier, R. (2010). A likely universal model of fracture scaling and its consequence for crustal hydromechanics. J. Geophys. Res. 115:B10411. doi: 10.1029/2009JB007043
Hansen, H. F., and Hansen, A. (2007). A monte carlo model for networks between professionals and society. Phys. A 377, 698–708. doi: 10.1016/j.physa.2006.11.064
Maslov, S., and Sneppen, K. (2002). Specificity and stability in topology of protein networks. Science 296, 910–913. doi: 10.1126/science.1065103
Mathias, N., and Gopal, V. (2001). Small worlds: how and why. Phys. Rev. E 63:021117. doi: 10.1103/PhysRevE.63.021117
Meakin, P. (1991). Invasion percolation on substrates with correlated disorder. Phys. A 173, 305–324. doi: 10.1016/0378-4371(91)90366-K
Newman, M. E. J. (2003). The structure and function of complex networks. SIAM Rev. 45, 167–256. doi: 10.1137/S003614450342480
Renshaw, C. E. (1999). Connectivity of joint networks with power law length distributions. Water Res. Res. 35, 2661–2670. doi: 10.1029/1999WR900170
Rosvall, M., Trusina, A., Minnhagen, P., and Sneppen, K. (2005). Networks and cities: an information perspective. Phys. Rev. Lett. 94:028701. doi: 10.1103/PhysRevLett.94.028701
Schertzer, D., and Lovejoy, S. (1987). Physical modeling and analysis of rain and clouds by anisotropic scaling multiplicative processes. J. Geophys. Res. 92, 9693–9714. doi: 10.1029/JD092iD08p09693
Ström, A., Andersson, J., Skagius, K., and Winberg, A. (2008). Site descriptive modelling during characterization for a geological repository for nuclear waste in sweden. Appl. Geochem. 23, 1747–1760. doi: 10.1016/j.apgeochem.2008.02.014
Valentini, L., Perugini, D., and Poli, G. (2007a). The “Small-World” topology of rock fracture networks. Phys. A 377, 323–328. doi: 10.1016/j.physa.2006.11.025
Valentini, L., Perugini, D., and Poli, G. (2007b). The “Small-World” nature of fracture/conduit networks: possible implications for disequilibrium transport of magmas beneath mid-ocean ridges. J. Volcanol. Geotech. Res. 159, 355–365. doi: 10.1038/30918
Keywords: fractures, fracture networks, network topology, dual networks, network analysis
Citation: Andresen CA, Hansen A, Le Goc R, Davy P and Hope SM (2013) Topology of fracture networks. Front. Physics 1:7. doi: 10.3389/fphy.2013.00007
Received: 28 May 2013; Paper pending published: 07 June 2013;
Accepted: 30 July 2013; Published online: 08 August 2013.
Edited by:
Ferenc Kun, University of Debrecen, HungaryReviewed by:
Jan Anders Åström, CSC - IT-center for science, FinlandZoltan Neda, Babes-Bolyai University, Romania
Copyright © 2013 Andresen, Hansen, Le Goc, Davy and Hope. This is an open-access article distributed under the terms of the Creative Commons Attribution License (CC BY). The use, distribution or reproduction in other forums is permitted, provided the original author(s) or licensor are credited and that the original publication in this journal is cited, in accordance with accepted academic practice. No use, distribution or reproduction is permitted which does not comply with these terms.
*Correspondence: Alex Hansen, Department of Physics, Norwegian University of Science and Technology, N–7491 Trondheim, Norway e-mail:YWxleC5oYW5zZW5AbnRudS5ubw==