- 1Structural Biology and Bioinformatics Division, CSIR-Indian Institute of Chemical Biology, Kolkata, India
- 2Computational Science Division, Saha Institute of Nuclear Physics, Kolkata, India
Biological cell is the fundamental building block of every living system. The plasma membrane, a phospholipid bilayer consisting of two asymmetric leaflets, defines its existence by separating the interior from the exterior. This low dielectric barrier selectively prevents the passage of hydrophilic and charged compounds including small ions. Integral transmembrane proteins span the entire bilayer and take part in small-molecule transport and complex signaling pathways while functioning as receptors and/or ion channels. These proteins carry important biological functions and hence are attractive drug targets. Present review considers the members of two important protein superfamilies that provided the major pharmaceutical drug-targets, viz., Cys-loop pentameric ligand gated ion channels (pLGICs) and class A G-protein-coupled receptors (GPCRs). The crystal structures of integral membrane proteins (IMPs) are difficult to obtain. Their unavailability has limited the structural investigation and associated structure-based drug designing (SBDD). However, recent advancement in crystallographic techniques yielded some important crystal structures. The advancement of computational science guided IMPs study even in the absence of crystal structures through the homology/comparative modeling approaches. These proteins possess multiple ligand binding sites including both orthosteric and allosteric sites. Addressing the multidimensional problem of understanding the structure and dynamics of such big proteins, multisite-protein-ligand complexes is now possible with molecular dynamics simulation approach, enabled with highly enhanced computational power. Overall the discussion highlights the understanding of structure-function relationship that guides SBDD of these interesting and important transmembrane proteins.
Introduction
Lipids are one of the major macromolecules present in the cell which are amphipathic in nature with polar head groups and hydrophobic tails. This amphipathic nature helps the lipid molecules to arrange themselves in bilayers which are about 5 nm thickness in living cells [1]. Various proteins are associated with these membranes which carry out varied functions of the cell including passage of selective molecules inside the cell, molecule recognition, and signal transduction [2]. The functions of the membrane associated proteins are modulated by the biophysical properties of the bilayer membrane which in turn depend on the lipid composition of bilayer. The glycerophospholipids are the major constituent of bilayers along with sphingomyelin and cholesterol [1]. The proteins associated with bilayer membranes are of two types—integral membrane proteins (IMPs) and peripheral proteins. IMPs are embedded directly into the lipid bilayer. Peripheral membrane proteins are not inserted into the lipid bilayer but are attached to the membrane by interactions with the peripheral regions of the bilayer or with integral proteins [2]. The structures of most of the IMPs are α-helical in nature. These α-helical domains are embedded in the membranes by hydrophobic interactions with the bilayer and also by polar interactions with the polar head groups of the phospholipids [3]. Few IMPs have single α-helix such as glycophorin, which in the active state forms dimer [3]. Most of the IMPs have multiple transmembrane helices. The pentameric ligand gated ion channels (pLGICs) have four transmembrane helices contributing per subunit, so in total 20 helices forming the channel pore, while G-protein coupled receptors (GPCRs) have seven helices spanning the entire membrane [3]. Another type of IMPs, such as porins, has different structure as it forms β-barrel. Amino acids of porins are largely polar [3]. The IMPs include transporters, linkers (proteins attached to the cytoplasmic side regulating downstream proteins), channels, receptors, enzymes, structural membrane-anchoring domains, proteins involved in accumulation and transduction of energy and proteins responsible for cell adhesion. Peripheral proteins adhere temporarily to the bilayer membranes. Different peripheral membrane proteins include enzymes, electron carriers, hormones, toxins, carriers of small molecules etc. Membrane proteins thus play huge functional role in any organism. To study the structural biology of these proteins, 3D structural information is needed which are very limited due to difficulty in protein expression and purification process [4]. It is even more difficult to crystalize membrane protein as it needs two types of environment—hydrophobic for the trans-membrane domain (TMD) region and hydrophilic for the extra cellular domain (ECD) region. Till now only 4,193 membrane proteins have been determined by experimental methods [4]. These limitations of experimental structure determination could at least be partially overcome by use of computational methods. This review focusses on the use of such methods in two important classes of transmembrane proteins viz., pLGICs and class A GPCRs.
Ligand Gated Ion Channels (LGICs)
Human brain is center of nervous system containing billions of nerve cells with a mind-boggling complexity. On average, each neuron is connected to other neurons through about thousands of synapses depending on local neuro-anatomy. The speedy electrical signals from pre-synaptic neurons cause the release of neurotransmitters at synaptic cleft. The converted chemical signals of neurotransmitters bind to the ligand gated ion channels (LGICs) embedded in the membrane of post-synaptic neurons. Once activated LGIC superfamily proteins mediate the passage of ions at the post-synaptic membrane. The LGIC receptors mediate specific synaptic transmissions by forming a unique signal transduction system re-converting the chemical signals of neurotransmitters back to the electrical signals. Overall this arrangement delivers fast and timely transmissions needed for responses of complex organisms. The dicarboxylic amino acids like glutamate, aspartate mediate excitatory responses, while monocarboxylic ω-amino acids like γ-amino butyric acid (GABA), glycine, β-alanine, and taurine mediate inhibitory stimuli [5]. There exists a delicate counter-balance between endogenous chemicals to harmonize the optimal brain function. Any imbalance may lead to number of neurological disorders. It makes LGICs as pharmaceutically important drug targets, which can be modulated by both endogenous neurotransmitters and exogenous allosteric modulators. Pharmaceutically important anion selective inhibitory members are GABAA/C-Rs [6, 7], strychnine-sensitive glycine receptors (Gly-Rs) [8, 9] and invertebrate glutamate gated chloride channels (GluCl) [10–12], while cation selective excitatory members are nicotinic acetylcholine receptors (nACh-Rs) [13, 14] and 5-hydroxytryptamine type-3receptors (5-HT3Rs) [15, 16].
G-Protein Coupled Receptors (GPCRs)
G-protein-coupled receptors (GPCRs) are the largest and most diverse group of membrane receptors in eukaryotes and constitute about 40% of the drug targets [17]. GPCRs are single polypeptide chain composed of around 300 amino acids, arranged in 7 transmembrane (TM) helices with alternate intracellular (IC) and extracellular (EC) loops. Upon ligand binding, conformational changes take place in the GPCRs. This conformational information is then transmitted to G proteins and effectors to initiate signal transduction [18]. The various physiological roles of GPCRs include visual sense (rhodopsin), taste, olfactory sense, behavioral regulation (serotonin and glutamate receptors), immune system regulation (chemokine), nervous system transmission, growth and metastasis. Based on phylogenetic criteria, human GPCRs have been divided into 5 families—rhodopsin, glutamate, adhesion, frizzled/taste2, and secretin [19]. Of these rhodopsin is the largest family with highest number of drug targets, hence, this review will focus on the rhodopsin family (class A) of GPCRs which will be discussed in details. The secretin family is another small family of GPCRs consisting of 15 members. Few members of this family are the calcitonin and calcitonin-like receptors, the corticotropin-releasing hormone receptors, the glucagon receptor, the gastric inhibitory polypeptide receptors, the glucagon-like peptide receptors, the growth-hormone-releasing hormone receptor among others. These receptors share 21–67% homology among themselves [19]. The binding site for the ligands consists of both N-terminal domain and EC region of transmembrane domains [19]. Adhesion family of receptors are the second largest family of GPCRs after Rhodopsin family. They are characterized by long N-terminal domain. They play a role in immune system, neuronal development and bone marrow and hematapoietic stem cells interactions. The binding sites of these receptors are located in the N-terminal domains [19]. Frizzled receptors play major roles in cell polarity, embryonic development, formation of neural synapses, cell proliferation, and many other processes in developing and adult organisms [19].
A Brief Evolutionary History of the pLGIC and GPCRs
The pLGIC descended from an early lateral transfer from prokaryotic source before the prokaryote-eukaryote dichotomy [20]. The pLGIC diversity is ancient and for divergent subunits only 10–15% of sequence identity is witnessed, however, multiple sequence alignment across entire superfamily clearly indicates high degree of conservation for important residues [20, 21]. Taken together the pLGIC superfamily shows lack of conservations at sequence level with highly conserved structures [22]. The most conserved regions are the transmembrane region (TM) and ligand-binding region in ECD while the remaining regions are less conserved [22, 23].
The GPCRs are present in all the five kingdoms of life with abundance in mammals. Approximately 4% of the mammalian genes encode for GPCRs [24]. GPCRs play a significant role in inter and intra-communication between organisms. The evolutionary success of GPCRs could be attributed to the fine-tuning of this communication process, and for this fine-tuning structural diversity is essential [25]. Intra-chromosomal gene duplications, entire chromosomal and whole genome duplications have helped GPCRs to evolve through all kingdoms of life [26]. Rhodopsin family, the largest family in GPCRs is considered the most evolved family. The ligand-binding site is located in the TM regions in this family, whereas in other GPCR families, N-terminal region of the receptors also play a crucial role in binding of ligands [25].
Structure-Function Details of pLGICS and Class A GPCRs
The structural assembly of pLGICs uses five subunits and provide regions like ECD, membrane embedded TMD forming ion channel with flanking intracellular domain. The subunit composition determines ion selectivity, neurotransmitter affinity, subcellular localization, gating kinetics, and pharmacology [21]. There exist different stoichiometry and subunit arrangements forming either homopentameric (identical subunits) or heteropentameric (variable subunits) receptors [27].
The depolarizing nACh-Rs and 5-HT3R channels provide excitatory synaptic signals while hyperpolarizing GABAA/C-Rs, Gly-Rs, and GluCl channels provides inhibitory signals. The diversity of subunit isoforms and further mRNA splice variants gives enormous heterogeneity for pentamer formation. The human nACh-Rs carries the repertoire of 16 different subunit subtypes α1−α7, α9, α10, β1−4, γ, δ, and ε [28] and GABAA-R uses 19 different subunit subtypes, α1−6, β1−3, γ1−3, δ, ε, π, θ, and ρ1−3 [6, 29–31]. This review exclusively considers GABAA-R as LGIC representative, thence provides its detailed discussion hereafter. Human brain contains 20–30% GABAergic neurons [32], where GABA mainly acts through ionotropic (GABAA, GABAC) and metabotropic (GABAB) receptors. The native and most abundant GABAA-R form comprises of two α1, two β2, and one γ2 subunits (about in 70–90% of all GABAA-Rs) [33–35]. The homopentamers are mostly limited to ρ and β1/3 subunits [36].
The physiological significance of diversity equips each of the divergent combination having different channel kinetics, affinity for GABA, rate of desensitization and ability for transient chemical modification such as phosphorylation [37]. Hetero-oligomeric channel assembly is known to form more efficiently with added benefits of higher chloride ion passage and possibly at lower GABA concentrations [38].
Each GABAA-R subunit consists of (i) extracellular ligand binding (~200–250 amino acids) N-terminal domain which starts from α-helix and further uses 10 β-strands to fold into a curled β-sandwich of inner and outer β-strands [39]; (ii) hydrophobic trans-membrane domain (TMD) of four α-helices (M1-4) where M2-helix lines ion channel; (iii) flanking intracellular loop domain (ICD); and (iv) a small C-terminal extracellular domain. Almost 10 small ligand binding sites are found at GABAA-R structure [40]. Most of these receptor sites are present at the subunit junctions and are spread all over receptor structure [40]. These allosteric and orthosteric sites couple ECD and TMD regions of GABAA-R covalently and functionally. The change over from closed (switched OFF) to open state starts when two GABA molecules bind at the orthosteric sites present at β+/α- subunit interfaces [41]. The conformational changes started after the binding of neurotransmitter in ECD region reaches to TMD region resulting in the opening of channel pore (switched ON), which further permits the influx of chloride ions and the initiation of an inhibitory postsynaptic potential (IPSP) [32]. Generally, chloride ion influx is from extracellular to intracellular region [35]. However, in developmental stage intracellular concentration exceeds extracellular levels causing ion efflux from inside to outside for neuronal depolarization (excitation) [35, 42]. GABAA-R controls the excitability of the brain to modulate anxiety, feeding and drinking behavior, circadian rhythms and cognition, vigilance, memory and learning [43]. Overall, immense therapeutic opportunities exist that work through GABAergic inhibition.
Out of total 799 members of full length GPCRs, class A GPCR also known as rhodopsin family has around 670 members [44]. The rhodopsin family is highly diversified based on both the basis of its sequence in the N-terminal and the TM regions, and the ligand binding characteristics. The TM region consists of 7 TM helices arranged in anticlockwise pattern. Rhodopsin family is further subdivided in to 4 classes, namely, α, β, γ, and δ [45]. This class of GPCRs is target for various ligands such as peptides, amines and purines. The α group consists of various drug targets such as histamine receptors, dopamine receptors, serotonin receptors, adrenoreceptors, muscarinic receptors, prostanoid receptors, and the cannabinoid receptor. Widely used drugs such as antihistamines, antacids, antipsychotic, and cardiovascular drugs bind to these receptors [46]. The binding site of these receptors is located in the TM region The β subfamily consists of endothelin, gonadotrophin-releasing hormone, and oxytocin receptor. The ligands for these receptors are mainly peptidic ligands with binding site consisting of TM regions, EC loops and N-terminal region. The γ group consists of opioid receptors, somatostatin receptors, and angiotensin receptors [45]. The members of δ subfamily of rhodopsin family have very low sequence homology with rhodopsin protein. However, they have a series of conserved residues in the TM region, which categorize them in the rhodopsin class of receptors [47]. The conserved residues of each of the TM helices [47] are provided in Table 1. The numbering scheme of the residues is followed for class A GPCRs [48] where the first number denotes the helix (1–7) and the second is the residue position relative to the most conserved position, which is assigned the number 50. Both the hydrophobic and polar residues are important for receptor activation [49]. The residue 3.40 stabilizes the receptor in the active state and mutation of this residue in histamine H1 receptor abolishes its activity [50]. The crystallographic structure of β2 adrenergic receptor complexed with G protein shows that during the process of receptor activation, R3.50 interacts with Y5.58 and Y 7.53 [51].
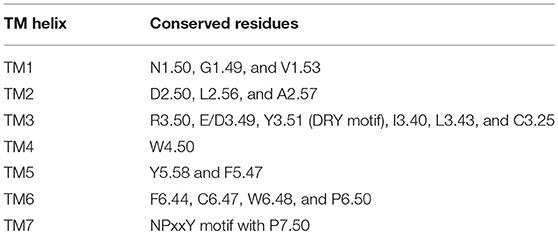
Table 1. Conserved residues in each of the TM helices in rhodopsin family [44].
LGICs and GPCRs: Implication for Drug Discovery
Currently entire mankind is facing the challenge of maintaining the proper balance of immensely operative brain due to extremely competitive and stressful life. The Global Burden of Disease Study 2010 indicated 28.6% contribution of neurological disorders [52] presenting significant disease burden. Considering the desperate need, the drug discovery programs targeting LGICs and GPCRs hold profound potential.
In mammals, GABA is present in more than 30% of the central nervous system (CNS) neurons [53]. Excessive GABA-mediated inhibition may lead to coma, depression, low blood pressure, sedation, whereas excessive excitation may result in convulsions, anxiety, high blood pressure, restlessness, and insomnia [54]. The neuropharmacology of GABAA-R is vital as it is a major therapeutic target for anxiety disorders, cognitive disorders, epilepsies, mood disorders, schizophrenia, and sleep disorders [54]. The widespread distribution and broad effects made them important drug targets [55]. The latest study indicates that the mental health disorders cost for not treating the depression and anxiety affects the global economy nearly by $1trillion every year [56]. The investment cost needed over the period of 2016–30 to substantially scale up effective treatment coverage for depression and anxiety disorders is estimated to be US$147 billion [56]. Another level of consideration for CNS drug discovery is coupling of neurotransmitter (NT) receptors to ion channels in the brain [57]. The convergence and divergence of NT actions give rise to diversity in neuronal signaling [57]. Apart from activating or deactivating the channels, NTs can simultaneously alter the properties of voltage-dependent ion channel conductance thus controlling cell excitability [58]. The few interesting coupling examples to understand while perusing CNS drug discovery are: (i) opioid inhibits GABA-mediated synaptic currents by controlled presynaptic voltage-dependent potassium conductance, [59]; (ii) Kir3 potassium channels coupling with metabotropic GABAB-R controls neuronal excitation [60], and (iii) GABAB-Rs inhibit many voltage-sensitive calcium channel (VSCC) subtypes and may control Ca-dependent neuronal processes [61] etc. Thus, different receptor types modulate the same ion channel either using shared second messenger system or separate systems viz., NTs like GABA, serotonin, and adenosine act on different receptors but activate same G protein to open a common K+ channel [57]. Overall the considerations of (i) amount of NT released and (ii) type of receptor and coupling mechanism determines the complexity in neuronal signaling [57].
Drug Designing Hierarchy Considerations: Subtypes, States, and Sites of Receptors
Taking into account the different subtypes forming the pentamer (for selective actions), states of receptor (for functional reasons) and binding sites (targeted binding), there are three critical factors to be considered for drug discovery (Figure 1B).
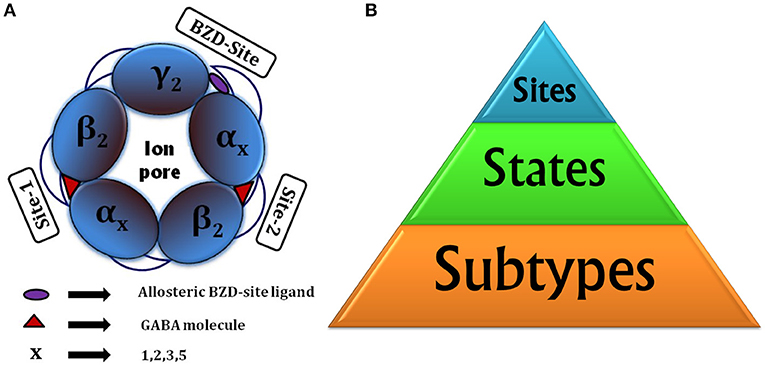
Figure 1. (A) Animated top view of GABAA-R showing (i) The subunit arrangement and channel pore; (ii) Location of orthosteric site-2 and site-1 with bound GABA having capped C-loop; (iii) allosteric BZD-site. (B) Drug designing hierarchy: The major consideration is subtypes to target, next come the “states” of receptor it brings responsibility of finding functionally important sites or hidden allosteric sites from the ensemble of conformations, and finally the sites to be targeted, whether orthosteric or allosteric.
In GABAA-Rs the molecular genetics and pharmacological data indicated that α2/3-subunit containing GABAA-Rs show “anxiolytic” action, whereas α1-subunit containing show “sedative” actions [62]. Thus, a compound selective for α2/α3-GABAA-Rs and having no activity at α1-GABAA-Rs would provide benefits from non-sedating anxiolytic actions and its less addictive nature.
The receptor composition having α1, α2, α3, or α5 subunits in addition to β- and γ- subunits are benzodiazepine (BZD)-sensitive [63, 64]. They are largely located synaptically and mediate phasic inhibition in the brain [65]. The BZDs acting through BZD site, present at α (+)/γ (–) subunit interface, affect the preactivation step and cause global conformational rearrangements of GABAA-R structure [66]. Overall it shifts the equilibrium between the ligand bound resting and preactivated states before channel opening [66]. The pentamers consisting α4- or α6-subunits together with β and δ subunits, located extrasynaptically, mediate tonic inhibition and are insensitive to BZD modulation [67]. The diverse subtype combinations with different regional, cellular and sub-cellular distribution [35] provides opportunity to modulate different neuronal circuits entitled with the novel selective actions, viz., selective affinity or efficacy.
Apart from already discussed closed and open states, there exist other receptor states like uncapped state with GABA-unbound or antagonist bound states [68–72], desensitized state [73] and doubly/singly GABA bound states having unique role to play in mediating fast inhibitory neurotransmissions.
Structurally, LGICs possess five subunit junctions with ligand binding possibilities, out of which three sites in ECD are important in case of nACh-R [74] and GABAA-R (two GABA binding sites and one BZD-site, shown in Figure 1A).
The GABA binding site is located just beneath the C-loop, hence, its positioning can be considered as a main barrier for GABA entry [68]. The GABA unbound intermediate “Uncapped receptive state” originates form closed state and provides accessibility path for GABAs to enter and bind. The extra C-loop opening at site-2 offers more time and space for GABA binding than the less opened C-loop of site-1, making site-2 as favored GABA binding site [68, 75]. This uncapped state controls the subsequent formation of doubly and/or singly bound states. The projected journey centered on uncapped receptive state [68] with GABAA-R opening-closing cycle is shown in Figure 2.
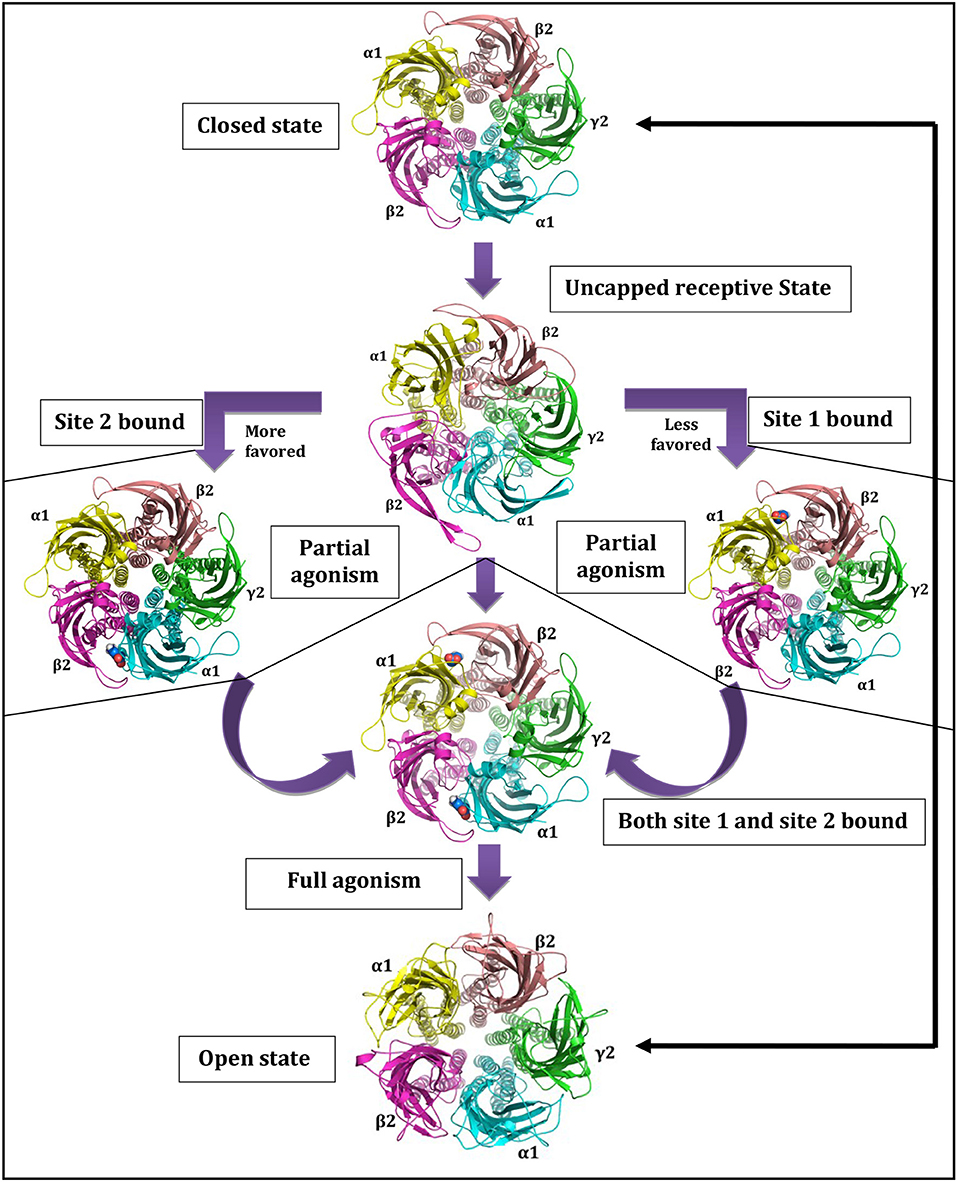
Figure 2. Central role of uncapped receptive state in governing state as well as site dependant characteristics of GABAA-Rs. Reprinted by permission of Taylor & Francis Ltd, http://www.tandfonline.com [68].
The exploration of third (allosteric) site is needed when there is decrease in apparent affinity or concentration of GABA to elicit chloride ion currents [76]. The restoration of neuronal balance can be achieved through therapeutic modulation through BZD-site. So, what happens to the cross-talk between two cooperative orthosteric GABA sites when we bring third allosteric BZD-site into consideration? And also, how it is connected with subtype selective modulations? These are the required questions to be addressed for subtype selective drug designing. In summary, the critical factors to be considered for drug designing are the subtype composition, states (bound, unbound, desensitized, intermediate etc.) and sites (orthosteric and allosteric) of the receptor.
GPCRs: Implication for Drug Discovery
Various GPCR proteins are targets for diseases associated with CNS. Positive and negative allosteric modulators could help in modulating these GPCRs [77]. P2Y, a purinergic GPCR is a target for Alzheimer's disease and is distributed throughout the brain. An allosteric binding site for this receptor has been deciphered by crystallography. Negative allosteric modulators could be identified using the binding site inhibition [78]. Muscarinic acetylcholine receptors (mAchRs) are involved in cognitive, memory and motor functions [77]. M1 and M4 mAchRs subclasses are considered as important targets for schizophrenia, Alzheimer's disease and Parkinson's disease [79]. Positive allosteric modulators could help in increasing the reduced cholinergic transmission [80]. Various subtype selective positive allosteric modulators of mAchRs have been undergoing clinical trials [77]. Metabotropic glutamate (mGlu) receptors are class C GPCRs which have a large extracellular domain for binding glutamate and have been divided into eight subtypes [77]. They are targets for Parkinson disease (mGlu4; mGlu5); cerebellar ataxia (mGlu1) and Alzheimer's disease (mGlu2, mGlu5) [77]. The orthosteric sites for the different subtypes are highly conserved, so it is very difficult to identify subtype selective ligands. Role of GPCRs' structural data for drug discovery of neurodegenerative process have been reviewed thoroughly [78]. Recent elucidation of various crystallographic structures of GPCRs have helped in better understanding of structural biology at the orthosteric site. Studies on β1 adrenergic receptor and β2 adrenergic receptor elucidates that the substrate selectivity is due to specific regions in the EC loop of each receptors [79]. Likewise, crystallographic structures of other GPCRs have shed insights into the subtype selectivity of ligands at the orthosteric sites [78]. Another important aspect for understanding the structural biology of GPCRs is their conformations in the lipid environment. The composition of lipid bilayer specifically the concentration of cholesterol modifies the conformational states and activation of these receptors [80]. Another aspect of GPCR structural biology is the presence of homo/hetero dimerization of the receptors which also modulates the binding of ligands and the signal transduction process [81, 82]. Various conformational states of GPCRs are present which are in between active and inactive states and conformational stabilization of these states could not be explained only by the presence of orthosteric ligand-binding sites. On the other hand, allosteric modulators could potentially target various subtypes. These allosteric binding sites are present in EC domain and help in stabilization of intermediate states and ligand binding which promote alternate signaling pathways [78, 83]. The biggest advantage of allosteric site is that it is under less evolutionary pressure and thus it may easier to develop subtype selective ligands. A list of available subtype selective modulators of class A GPCRs have been summarized [83]. Allosteric modulators of mGlu receptors have been found to demonstrate symptomatic treatment potential in preclinical studies in mouse models [77]. Thus, identification of allosteric modulators of GPCRs involved in CNS diseases appears to be promising in subtype modulation of these receptors and is a therapeutically major area of research.
Recent Three-Dimensional Structures
Recent Three-Dimensional Structures of GABAA-Rs and Structural Homologs
First high-resolution structure of membrane protein was for soluble acetylcholine binding protein (AChBP) containing extracellular region [84]. Initially, the structure of apo AChBP from Aplysia Californica [85] served as template for many modeling works restricted to only the ligand binding domain (LBD) of receptor. The first complete structure was from heteromeric nAChR of Torpedo marmorata electric organ at 4Å resolution [86, 87]. The resolved crystal structures of two bacterial homologs from Erwinia chrysanthemii (ELIC) [88] and Gloeobacter violaceus (GLIC) [89] provided understanding about the closed and open states, respectively. By serving as representative templates, these biologically important states provided valuable understanding of GABAA-R structure and function [23, 68]. The first resolved structure of an anion-selective glutamate-gated chloride ion channel homopentamer (GluClα) was from Caenorhabditis elegans [90, 91]. These homologous proteins opened the doors to understand the structure of GABAA-Rs. Recent disclosure of human β3-homopentamer [39] provided opportunity to understand the structural details of GABAA-R, however, its desensitized state nature limited its utility for modeling other states of receptors. The latest structures are apo GluCl from Caenorhabditis elegans [92] and agonist-bound form [90], nanobody-bound mouse 5HT3R [93], agonist-bound human GlyRα3 [94], and ivermectin bound form [95] further providing the structure-function understanding.
Recent Three-Dimensional Structures of GPCRs
The first crystallographic structure of GPCR was that of bovine rhodopsin in 2000 [96]. The structure of squid rhodopsin was deposited in 2008 [97]. The first crystallographic structure of human β2-adrenergic-receptor complexed with inverse agonist was deposited in 2007 [98] and thereafter structures bound to antagonist [99] and irreversible agonist were deciphered [100]. The structures of Turkey β1-Adrenergic-aceptor complexed with antagonist [79], partial agonist and agonist were deciphered [101]. Thereafter the crystallographic structures of human Alpha2 Adenosine receptor bound to antagonist [102] and agonist [103] were deciphered. These structures paved the way for better understanding of structural basis of receptor-ligand interaction in GPCRs. These structures also served as new templates for homology modeling of other GPCRs. Crystallographic structures of all three opioid receptors bound to antagonists were deciphered in 2012 [104–106]. All available crystallographic structures of GPCRs are listed in the following database (https://zhanglab.ccmb.med.umich.edu/GPCR-EXP/).
Computational Structure-Based Drug Designing Approaches in Solving LGIC and Class A GPCR Structures and Protein-Ligand Recognition
Traditional vs. Modern Drug Discovery
The unfulfilled clinical need accompanied by disease or clinical condition is the mother of all drug discovery program initiatives. One of the costliest, time consuming, and complex ideas to explore is dreaming about a drug molecule from the pool of billions of chemical compounds. It takes arduous input of almost 12–15 years and expense of more than $ 1 billion [107]. Traditional drug discovery was mainly driven by combinatorial chemistry taking chances in a trial and-error manner. Also, the strategy of one size-fits-all-diseases does not follow while identifying and validating drug candidate. It is impractical to conduct experiments on biological screening of publicly accessible billions of compounds. Hence, the current drug discovery program is an interdisciplinary endeavor that considers input from all the essential disciplines to avoid end stage drug failures saving huge loss of time, money and resources.
The rational drug designing follows “one gene, one drug, one disease” paradigm [108], which arose from the agreement between genetic reductionism and latest molecular biology techniques helping in characterization of disease-causing genes. The most widely used “reductionistic” target-based approach [109] focuses on identification and validation of small molecule compounds against a specific protein target. The chosen target function must be essential for disease-phenotype. The process of choosing a drug-target for a costly drug discovery program is pivotal. The key preclinical stages of the drug discovery process are as follows: (i) initial target identification and validation; (ii) thorough assay development; (iii) high throughput screening; (iv) hit identification; (v) lead optimization and final selection of candidate molecule for clinical development [107]. The drug discovery and development pipeline is shown in Figure 3.
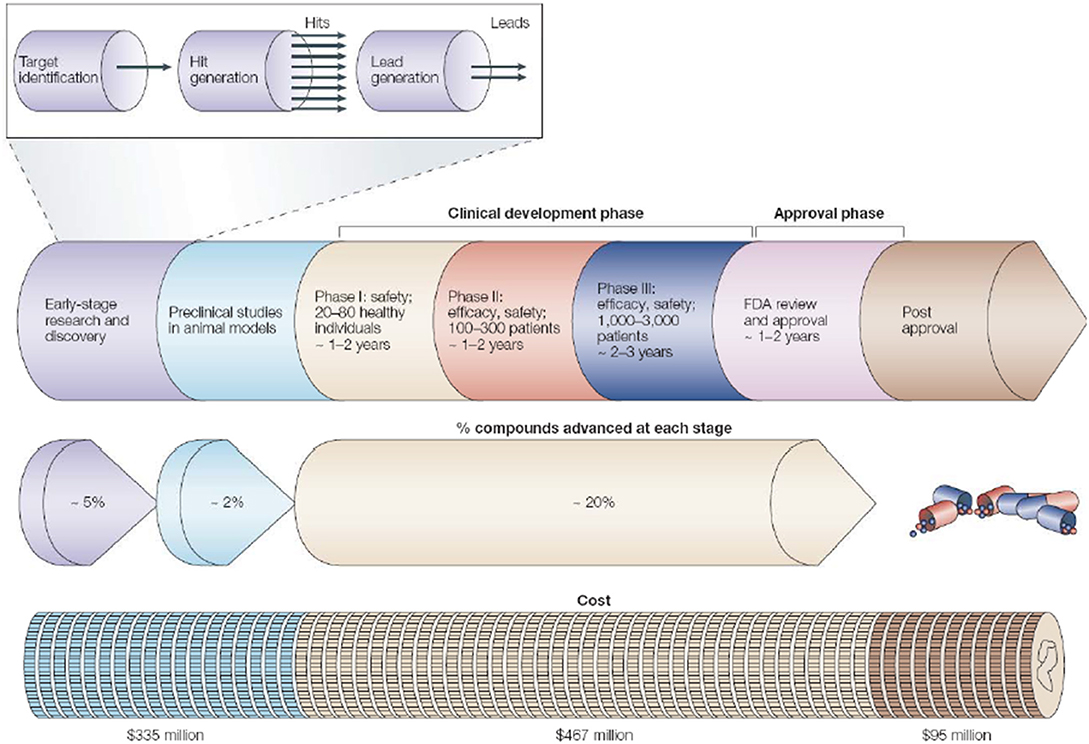
Figure 3. The pipeline for drug discovery and development. The long process starting from initial idea to a marketed drug takes ~12–15 years and success rate of finally reaching 1 in 5,000 compounds to the market (may vary depending on the disease area). The preclinical stage gives most failures so need to detect it as early as possible. Pipeline follows hit-to-lead process by assessing “hits” for relevant biological and drug-like properties (Reprinted by permission from Macmillan Publishers, Ltd.: Nature Horizon, ref. 110, copyright 2004).
One of the first drugs based on rational designing approach was Relenza [111], used to treat influenza. Computational methodologies form the essential part of modern drug discovery programs [112] assisting with enormous inputs. The inputs assist at every level of drug designing process including the use in high throughput screening [113]. Overall it has tremendously fostered the process of drug designing saving up to 50% of total drug design cost [114].
The era of 1990s favored and extensively followed “reductionistic” target-based approach. In the present postgenomic era, its sole follow up yielded persistent failures [115]. More holistic approach involves the screening of test compounds to determine the ability to elicit phenotypic changes in mammalian cells/animal model [109]. This phenotype-based approach is not limited to individual genes or proteins, however, it covers the investigation of signaling pathways in a systems-based manner [116]. This approach aims at providing the chemical tools against every protein encoded by the genome. The phenotype-based versus target-based drug discovery pipeline follow up is given in Figure 4.
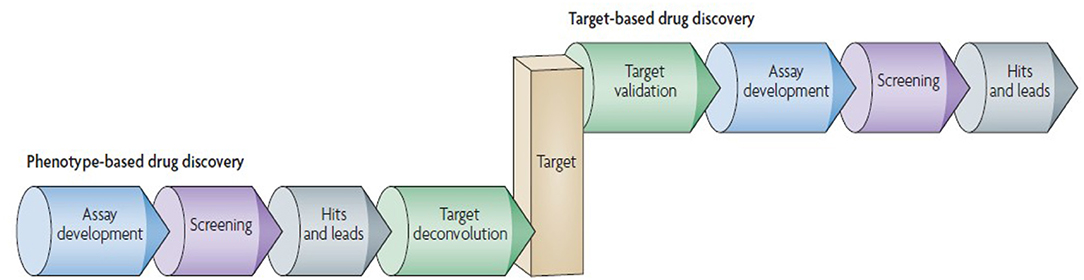
Figure 4. Phenotype-based vs. target-based drug discovery. The figure demonstrates the identification of molecular target and lead molecules during early phase drug discovery process. The phenotype-based approach first obtains lead molecules, and then proceeds with target deconvolution for identifying the targets that highlights observed phenotypic effects. In target-based approach identification and validation of molecular targets takes place before starting the actual lead discovery (Reprinted by permission from Macmillan Publishers, Ltd.: Nature Reviews Drug Discovery, ref. 109, copyright 2007).
In structure-based drug design, 3D-structure of a drug target interacting with small molecules is used to guide drug discovery [117]. As mentioned by Raymond Stevens, “We're in a very target-rich but lead-poor post-genomics era for drug discovery” [117]. The source of structural information can be achieved through X-ray crystallography, nuclear magnetic resonance (NMR) spectroscopy or cryo-electron microscopy (cryo-EM).
In the new era of molecular biology, the determination of large, fragile, or flexible ion channel structures can be achieved through the resolution revolution in cryo-EM method [118]. Without protein crystallization this technique can capture full conformational states [119]. The resolution for most of the cryo-EM ion channels is in the modest range of 3–4 Å [119]. By combining cryo-EM and MDS studies significant insights about drug binding to ion channels can be achieved [119] for such structures. Latest (2018) cryo-EM structure of pLGIC member 5-HT3A serotonin receptor in its apo-sate [120] is the best example to mention here. 3D structures of GPCRs such as calcitonin receptor complexed with peptide ligand and heterotrimeric Gαsβγ protein [121] and glucagon-like peptide receptor complexed with Gs [122] have been determined recently using cryo-EM technology.
The site-directed spin labeling and electron paramagnetic resonance (SDSL-EPR) spectroscopy provides another approach for studying structure-functions details of ion channels. The study on K+ channel KcsA outer vestibule is an excellent example where authors used combined SDSL-EPR and restrained ensemble (RE) simulations to gain insights on gating-induced structural dynamics [123]. The combined approach of using EPR-spectroscopy and computer simulations carries a potential to answer problems on ion channels [123, 124].
Focused combinatorial chemistry is the best thing that ever happened to structure-based drug design [117]. Target knowledge based combinatorial chemistry could guide the rapid generation of numerous compounds. Michael Milburn mentioned, “Structure is a really good way of quickly getting a handle on how the lead compound binds to the target of interest and what one might be able to do with chemistry to modify the molecule to get the desired properties” [117]. So, when we know about the binding profile of any compound we also know where it can possibly be modified to improve its druggability. When 3D-structures of the targets are unavailable the path of structure based drug designing could be nicely guided by homology/comparative modeling of the target.
Homology Modeling and Molecular Docking
Structure based studies without experimentally determined three-dimensional structure availability can be completed with the help of homology modeling technique. The obvious reason to perform modeling is rightly stated by Henry A. Bent, “A model must be wrong, in some respect, else it would be the thing itself. The trick is to see where it is right” [125].
Homology modeling predicts the tertiary structure of an unknown protein using known 3D-structure of protein(s) with homologous sequence as template(s) [126, 127]. Unfortunately, the 3D-structures of many pharmaceutically important drug targets like GPCRS and ion channels are not available as structure elucidation is often hampered by difficulties in isolating pure protein, diffracting crystals and many other technical aspects. Homology modeling is based on two major observations: (1) the structure of a protein is uniquely determined by its amino acid sequence; and (2) during evolution, the structure changes much slowly than the associated sequence, hence, similar sequences adopt identical structures and distantly related sequences fold into similar structures [128, 129]. One of the most successful and largely used tools for homology modeling is MODELLER [126, 130, 131]. The first step of homology modeling is to select an appropriate template for the query sequence. Alignment is reliable when a structure with high sequence homology from PDB [132] (>50%) is available. As identity continues to decrease below 30%, the task of recognizing the appropriate template becomes increasingly difficult [133]. Methods such as BLAST [134] and PSI-BLAST [135] are often used for finding templates. After identification of the best template for comparative modeling, an optimal alignment must be made, which plays a crucial role in determining the quality of the model. Methods like Smith Waterman algorithm [136] Clustal X [137], Dialign [138], Fugue [139], and PROMALS-3D [140] are routinely employed for sequence alignment. The issue of low template-target sequence identity is common in membrane protein modeling. Despite this, it is well accepted that the members of pLGIC super family adopt similar 3D-structures. Suitable models can be obtained even with low sequence identity [23]. Consequently, the use of profile-based sequence-structure methods like Fugue and PROMALS-3D are recommended for obtaining reliable IMP models. It is advised to use multiple templates to increase the sequence coverage for such cases. Additionally, knowledge of experimental data from biochemical and pharmacological experiments can be used to guide the process of model generation by applying structural restrains. Once a proper target-template alignment is ready, the model building starts with generation of 3D coordinates of backbone. After the initial model building, the model should be optimized using either energy minimization or molecular dynamics simulation methods. Validation of homology models is carried out for stereochemical accuracy, fold reliability and packing quality. Various programs and servers like WhatIf [141], Procheck [142], Prosa [143], Verify3D [144], and Molprobity [145] are routinely employed to check the reliability of the models. A reliable measure of model quality assessment is Cα-RMSD values obtained after RMSD fitting of model with native state template. Homology modeling methods are applicable for membrane protein modeling as it is to water-soluble proteins [146]. The acceptable models can be obtained with Cα-RMSD values to the native of 2 Å or less in the transmembrane regions at 30% or higher sequence identity [146]. Details of binding modes for the known compounds and presence of reported interactions can provide important criteria to judge the quality of obtained model and to confirm the proper modeling of active-sites. Mostly obtained models represent the unliganded state and hence post-modeling confirmation is required. With this approach accurate homology models of IMPs have been obtained [23]. However, it can also be taken care during modeling process by implying ligand-aware modeling approaches [147]. It treats ligands as an integral part of a model throughout the entire modeling process like ligand steered modeling (LSM) which heavily relies on correct ligand placement. The binding site remodeling approach applies ligand restraints on the initial model to build a second refined model [148], such approach showed success rate of 70% in producing near-native binding-site geometries (rmsd < 2.0Å) with MODELLER [148]. For mutagenesis studies low-accuracy models can be completely sufficient while structure-based virtual screening requires greater accuracy [149].
To understand the world of protein, it is essential to know the different ways of communication operating through the molecular interactions at its functional sites. Both transient and long-lasting interactions between macromolecules and their molecular partners are fundamentally important for all the biological mechanisms and it lies at the conceptual heart of protein function [150]. The protein binding site is of prime importance and involves in the binding events. The 3D-arrangement of a binding site consists of specific amino acids, which confers characteristic (i) geometry: the size and shape and (ii) physicochemical properties: hydrophobic or polar, for molecular binding [1]. The event of molecular recognition between protein and any small molecule considers the complementarity between the two binding partners [151]. Adequate steric complementarity, large interaction interfaces and specific geometric constrains are necessary for proper binding.
Docking is a method of computationally predicting conformations of a ligand and its proper orientation into the binding site of the protein of interest using different search algorithms. It is used for predicting protein-ligand interactions and binding affinity. Protein-ligand docking is a tool to identify novel ligands using structure-based virtual screening approach. The basic requirements for docking are 3D-structures of the protein and ligands and a docking tool with validated search algorithm and scoring function [152]. The origin of degrees of freedom to consider comes from the relative orientation of two binding partners (i.e., three rotations and three translations) as well as their conformational flexibility (rotatable torsion or dihedral angles). Given a binding site, ligand sampling algorithm generates putative ligand orientations called as poses. Search algorithms for sampling the conformations of the ligand can be broadly divided into three categories, namely, systematic methods (incremental construction), random or stochastic methods (Monte Carlo, genetic algorithms), and simulation methods [152].
Docking procedures can be classified into three levels by their degree of flexibility [152]: (1) Rigid body docking approach which uses conventional lock and key model and treats both protein and ligand as rigid solid bodies. (2) Semi-flexible docking approach, where one of the two molecules, usually the smaller ligand is considered as flexible, while the receptor is regarded as rigid. (3) Flexible docking approach, in which both ligand and protein are treated as flexible.
Scoring functions are mathematical methods, which are used to predict the strength of the binding affinity of protein-ligand complex after docking. Different types of scoring functions, used in docking are [152]:
(i) Force field-based methods use non-bonded interaction energies from existing force fields to estimate binding affinity. These methods approximate the binding free energy of protein-ligand complexes by summing the van der Waals and electrostatic interactions.
(ii) In empirical scoring functions, the binding affinity is decomposed into sum of few chemically acceptable interactions. These scoring functions are made up of terms accounting for hydrogen bonds, ionic interactions, hydrophobic interactions, and entropic contributions.
(iii) The knowledge-based scoring functions model the protein-ligand affinity by means of statistical analysis of structural data of different protein-ligand complexes. The frequency of occurrence of a particular combination of atom types is a measure of its contribution to binding affinity.
Few popular docking algorithms are LigandFit [153], FlexX [154], DOCK [155], GOLD [156], AutoDock [157], Glide [158], and InducedFit. LigandFit considers protein as rigid molecule. FlexX, AutoDock, DOCK, and Glide consider partial flexibility of protein. GOLD involves flexibility of side chains of protein. InducedFit algorithm uses more realistic approach that considers the flexibility of both the binding partners adding induced protein flexibility.
Homology Modeling of GABAA-Rs and Docking of Ligands
The structural homology across the pLGIC superfamily has been explored for advancing the knowledge of members difficult to crystalize, using homology modeling approaches. Continuous efforts have been made to build better homology models of GABAA-Rs [23, 159–169]. Most of the available crystal structures used for LGIC modeling are homopentameric while the structures to be modeled are heteropentameric in nature. The use of different templates for modeling is discussed under recent 3-Dimentional structures of LGIC heading. The issue of homo for hetero needs to be addressed for using these templates properly. The following parameters need to be carefully considered and addressed during modeling: (a) the sequence coverage between target-template sequence; (b) maximizing evolutionary important conservations with sequence-structure based alignment across all the subunits; (c) subunit order whether clockwise or anti-clockwise to obtain biologically relevant subunit arrangement; (d) dealing with the unaligned intra-cellular regions; (e) considering multiple templates to combat low sequence identity issue; and (f) the state of the considered template structure whether (apo, holo, closed, or open etc.). The very common observation about all the modeling studies is that they are successful in providing valuable information based on where the model is right. This skillful utilization of modeling technique is encouraged where obtaining a crystal structure is difficult. The homology modeling has helped in understanding the GABAA-R structures and is still serving the purpose. The recently available templates carry more sequence identity and are contributing for model building depending on their states for addressing problems within scope.
In normal physiological conditions, two orthosteric GABA binding sites of GABAA-R function to open the channel for IPSPs. These sites consist of residues Phe64, Arg66, Arg119, Arg131 from α (–) subunit and Glu155, Tyr157, Phe200, Tyr205, Thr202 from β (+) subunit [161, 169–171] (see Figure 1A). Understanding the binding of very small and flexible GABA molecule with so many reported binding site residues is difficult task. Previous studies have explained the molecular recognition of GABA and most of them are in accord with literature findings pinpointing not all but important binding residues at the respective models [23, 68, 169]. The binding pockets of such multimeric protein involves the junctions between two subunits, hence, to cover their contribution the ligand flexibility alone cannot be sufficient. So, to obtain reliable binding solution the flexibility of both binding partners needs to be considered for huge proteins like GABAA-Rs. Next question to address for multisite binding problem is: which of the two orthosteric binding sites to consider for first docking experiment, which for the second and why? The earlier literature suggests that site-2 is favored GABA binding site over site-1 [68, 75], these observations address this issue of binding site prioritization for docking experiment. It can also be quickly tracked by performing IFD experiment separately on each of the sites and comparing their scores finally to go with the highest scoring site. To combat with the diseased situations, exploring positive allosteric modulatory (PAM) effects by targeting BZD-site remains the choice of need. The allosteric BZD-site is present at the subunit junction of α(1,2,3,5)(+)/γ2(−). As a whole, BZD-site is formed by the contribution of α-subunit residues Tyr159, Ser204, Tyr209, and His101, and γ2-subunit residues Phe77, Met130, and Thr142 residues [169, 172, 173]. Compared to endogenous neurotransmitters the allosteric binders are larger in size and mostly more stable/less flexible. Their binding with α1-subunit containing GABAA-R shows type I BZD-binding profile with sedation and α2/α3-and α5-subunit containing receptors shows type II BZD-binding profile without sedation [174]. There are lot of studies which have shown the binding of allosteric modulators at BZD-site [161–164, 169]. In common agreement they are able to pose allosteric modulators at the binding site.
Homology Modeling of Class A GPCRs and Docking of Ligands
Class A GPCRs (rhodopsin family) include hormone, neurotransmitter, and light receptors, altogether representing the largest family of drug targets. These are characterized by 7 transmembrane helices with extracellular N-terminal region and intracellular C-terminal region. The basic step of homology modeling is to identify a proper template. Earlier in the 1990s, the homology models of GPCRs were generated using bacteriorhodopsin as template [175]. But it has <20% sequence similarity with the GPCRs. From the analysis of hydropathy profiles and sequence alignments of 39 GPCRs, it was found that there is high degree of homology between the 7 transmembrane helices of GPCRs [176]. 3D-models of various neurotransmitter receptors (serotonergic 5-HT2, dopaminergic D2, muscarinic m2, adrenergic α2 and β2) were generated based on the bacteriorhodopsin as template. The binding pockets of these receptors were also identified. Negatively charged Aspartic acid of TM3 and hydrophobic residues of TM4, TM5, TM6, and TM7 were found to be the part of ligand binding site. 3D-structure of melanocortin-1 receptors complexed with MSH peptide was generated using bacteriorhodopsin [177]. This peptide also formed salt bridge with Asp of TM3. Crystal structure of bovine rhodopsin was deposited in the year 2000 [96], since then bovine rhodopsin has been used as a template for modeling GPCRs [178]. The crystallographic structure of bovine rhodopsin was the first real template for GPCR modeling. Homology model of 5-HT1A serotonin receptor was also generated using bovine rhodopsin as template [179]. The model was improved by docking of ligands to the initial structure. With this model, crucial interactions of arylpiperazine derivatives were identified which corroborated with the experimental binding affinity data. Homology models of mu, delta, and kappa opioid receptors have been generated using bovine rhodopsin as template [180, 181]. 3D-structure of α1a adrenergic receptor was generated using homology modeling with bovine rhodopsin as template [182]. A known agonist norepinephrine and antagonist WB-4101 were docked to the homology model. Specific interactions for agonist and antagonist binding were determined which corroborated with experimental mutagenesis studies. A homology model of P2Y purinergic receptor was also generated using bovine rhodopsin as template [183]. The transmembrane helices of this receptor were modeled based on the template sequence and then the homology model was energy optimized. The residues found to be critical for ligand binding corroborated with the experimentally determined important residues for binding. They have also found few other residues near the extracellular interface to be crucial for ligand binding. Another study which attempted to model cholecystokinin (CCK) receptor [184] suggested that bovine rhodopsin may not be well suited for modeling different conformational states of all GPCRs. Bovine rhodopsin as a template is good for dopamine D2, muscarinic M1 and histamine H4 receptors and for all GPCRs which have structural divergences. Few years later, new crystallographic structures of β1-adrenergic receptor (β1AR), β2-adrenergic receptor (β2AR), A2A-adenosine receptor (A2AR), and squid rhodopsin [97, 185, 186] were deposited which paved the way for realistic generation of homology models of different GPCRs. It was also shown that use of multiple templates resulted in better homology models in case of low sequence identity [187, 188]. Opioid receptors have <30% identity with the new available templates. Homology models of all the three opioid receptors (μ, δ, κ) were generated using multiple templates [189]. The use of multiple templates significantly improved the sequence similarity of the transmembrane regions and overall structural quality of the homology models [189].
Use of Molecular Dynamics Simulations for Structure-Based Drug Designing
Richard Feynman in 1963 nicely described wiggling and jiggling of atoms as, “In an attempt to understand life, it is that, all things are made of atoms, and that everything that living things do can be reduced to wiggling and jiggling of atoms.” The component rigidity is required to maintain the structure, while flexibility is needed to perform the function, accordingly, we need the method that properly make us understand both of these. Molecular dynamics simulations (MDS) are used to understand the inherent properties of the molecular systems, e.g., proteins, where the time evolution motions of a set of interacting atoms are followed by integrating their equations of motions using the Newton's laws [190]. Monitoring the time dependent evolution of molecular systems allows studying their structural, dynamic, and thermodynamic properties. First molecular dynamics of protein was performed on Bovine pancreatic trypsin inhibitor in 1977 [191]. This study was fundamental in proving that proteins are not rigid and are dynamic in nature. Since then molecular dynamics simulation technique has been used in studying the protein folding problem and the impact of internal motions of proteins in ligand binding. The motions of a system are simulated under the influence of a specified force field according to Newton's equation of motion. The total force on each particle in the system at a time t is calculated as the vector sum of its interactions with other particles. From the force, the accelerations of the particles can be determined, which are then combined with the positions and velocities at a time t to calculate the positions and velocities at a time t + δt, where δt is the increment in time (1 to 2 × 10−15 s or 1–2 fs).
For simulating molecules either explicit solvent or implicit solvent can be used. Periodic boundary conditions (PBC) are employed to mimic a bulk phase in which one side of the simulation loops back to the opposite side. The commonly employed MD packages for simulations of proteins are AMBER1, CHARMM 2, GROMACS 3, and NAMD 4.
Molecular dynamics simulation may be useful for pharmacology in different ways. First, it may help in enabling the analysis of communication within the protein-protein complexes with a future aim of enabling the development of drugs that may disrupt the communication [192]. In one such example [193], oligomerization process was studied in a dimeric complex of tryparedoxin peroxidase for a very small time-scale of 10 ns. Second, the analysis of the effects of known ligands on protein dynamics will elucidate the effects of ligand binding as in the all-atom molecular dynamics simulation study of neuraminidases of H1N1 bound with Relenza and Tamiflu for 20 ns [194]. Third, finding of different conformations associated with specific cellular function to enable the discovery of conformationally selective ligands for example all-atom molecular dynamics simulation study of crystallographic structure of Escherichia coli MutS complexed with DNA for 10 ns [195].
MDS requires sample preparation, here it is system setup which determines the success or failure of the simulation experiment. MDS is also used in the determination of structures from x-ray crystallography and from NMR experiments.
Molecular Dynamics (MD) Simulation Studies on LGICs
Transmembrane (TM) α-helices form an important structural organization of IMP that functions as membrane embedded channel. Proper system setup of LGICs is challenging task as it explicitly consists protein, lipid bilayer, waters and ions forming a huge system (Figure 5) [68]. The physiologically relevant and friendly cross-talk between all these constituents is mandatory for successful simulation studies. Difficulties in obtaining the LGIC protein crystal structures, progress of MDS force-fields/methods and computational power altogether compensated for delayed use of computer simulations, particularly for IMPs. The earliest reported simulation study was on pore-lining M2δ helix of the nACh-Rs [196].
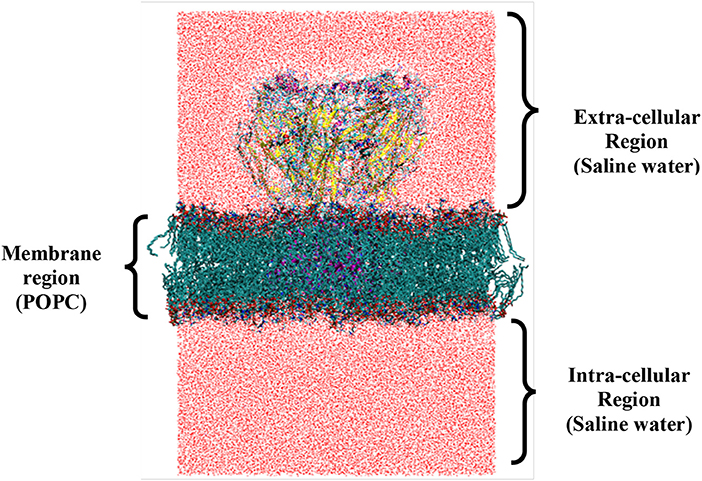
Figure 5. System setup of full length ionophore complex for molecular dynamics simulation (closed state). The regions specify the different cellular compartments that are divided by the presence of membrane. At the center of the membrane, protein is embedded considering the proper placement of structural regions like ECD, TMD, and ICD to form the final setup. The number of atoms for (i) protein (15,688); (ii) lipids (25,896); (iii) waters (207,033); and (iv) ions (140) of system shown.
Initially when complete structures were unavailable, studies focused on ECD region for understanding the ligand binding without bilayer [169, 173]. Meanwhile with the availability of complete ion channel structures full length setups with complete ionophore complex were studied [88, 89]. However, for more complex (heteropentameric) and still unavailable structures like GABAA-Rs, bacterial homolog-based models were used for MDS studies [23, 68]. The preliminary (solvent)-(protein-membrane)-(solvent) set up can be tailored to answer complex biological problems. To study gradient based ion passage, set up with different ion gradient across the bilayer is required. Based on the considerations of multiple factors like, (i) mixed lipid bilayers; (ii) multiple-ligand bound complexes; (iii) ion pulling; (iv) dummy spin labeled atoms [197]; (v) ligand binding pathway search; and (vi) Mutations etc., system set up may vary. The selection of proper simulation method depending on the problem to be addressed and the time length of simulation are two critical factors to be considered for LGIC MD simulations.
The simulations on endogenous GABA bound complexes viz. singly and doubly, for ~100-ns run provided answers for closed to open state transitions of GABAA-R [68]. The positive cooperativity of two GABA binding sites was evident highlighting (i) singly bound states possess less GABA residence and (ii) GABA binding at one site affects the nature of C-loop changes at the other site, controlling ligand residence/accessibility. The MD simulations can also provide insights on the flexibility issue underlining the contribution of “clamping residues” which participates in late ligand binding events. As rightly mentioned by David Colquhoun in 1998 “when the receptor is in its active conformation, several amino acids now clamp down onto the ligand and form the part of its binding pocket and these residues affect the extent of ligand binding.” [198]. These residues might not show any binding initially, however during dynamics they may clamp down to form the part of binding pocket. Our study on GABAA-R showed that the residue Thr202 was lately involved in GABA binding event and its involvement was there till the detachment of GABA from the pocket [68].
Molecular Dynamics (MD) Simulation Studies on GPCRs
Very early MD simulation studies on GPCRs were performed on a 3D-model of transmembrane helices of 5-HT2 receptor [199]. The 3D-model was generated based on bacteriorhodopsin as template [199]. MD simulations of 125-ps were performed with agonist and antagonist bound structures where agonist bound structure showed some structural changes in the binding site where as no change was observed in the antagonist bound structure. Homology models of three opioid receptors (μ, δ, κ) were built using bacteriorhodopsin as template and MD simulations were performed for 2-ns [180]. The MDS studies could differentiate between the dynamics of ligand-binding sites of the three different subtypes. They also categorized motions sensitive to ligand binding. CXC chemokine receptor 4 (CXCR4) was modeled based on bovine rhodopsin as template and then 2.5-ns MD simulation was carried out [200]. MD simulation resulted in minimized structure and provided many structural details about the receptor. It resulted in opening of a “mouth shaped void” and gradual closing of that void. It also resulted in formation of salt bridge between Arg188-Glu277, which is crucial for active state of the receptor. After the deposition of crystallographic structure of bovine rhodopsin, MD simulation was carried out on the crystallographic structure, which provided useful insights about the conformational changes in the protein backbone leading to the signal transduction. The results suggested molecular mechanism of intramolecular signal transduction in GPCRs [201]. Other MDS studies have also been performed on bovine rhodopsin in an explicit dioleoyl-phosphatidylcholine bilayer which showed that the retinal rhodopsin protein undergoes a conformational change [202]. This conformational change results in local changes, which further leads to global conformational changes in the protein. These large-scale motions eventually activate the G-proteins. MD simulation studies were performed on a homology model of adenosine A3 receptor, based on bovine rhodopsin as template [203]. MD simulations provided with the insights into how the receptor responds on binding of agonist and inverse agonist. Molecular dynamics simulation of human histamine H4 receptor was carried out in explicit membrane complexed with its endogenous activator histamine and with selective hH4R antagonist JNJ7777120 [204]. Studies corroborated with experimental data on GPCR activation. Histamine formed an interaction with Asn147 which is important for hH4R activation. Also, the intracellular side of TM helix 6 moved significantly away from TM helices 3 and 7 whereas in JNJ7777120-hH4R complex the intracellular side of TM6 moved toward the TM3. With the advancement of computational resources and availability of new crystallographic structures as templates, simulations with much longer timescales were performed, which could reveal the mechanisms of receptor activation and drug binding. Microsecond level simulations have been performed on β1- and β2-adrenergic receptors complexed with three antagonists and one agonist [205]. To identify the mechanism of drug binding they haven't docked the ligands to the receptor, instead placed the ligands 15 Å away from the receptor. In majority of the simulation runs drug entered the binding pocket through similar pathway and the receptor had undergone similar intermediate states. The drug first passed through the EC loop 2 and 3 contacting with hydrophobic residues Tyr308, Phe193, Ala200, His296, and Val297 and then it passes through the crevice between EC loop 2 and TM 5, 6, and 7 to reach the binding pocket. Authors also suggested that dehydration of both ligand and receptor facilitates the drug entry to the binding pocket. Their unbiased simulation and drug binding identified some metastable drug binding sites which could delineate the binding site for allosteric modulators. Using accelerated molecular dynamics simulation approach, activation of inactive state of M2 muscarinic receptor was achieved [206]. The study found that formation of hydrogen bond between Tyr206-Tyr440 and tilting of cytoplasmic end of TM6 characterize the receptor activation. Also, the residue Tyr430 is flipped away from the binding site. Communication in the intracellular domains gets weakened as a result of activation of the receptor. They also found that motions of residues in ligand binding site and G-protein coupling sites are correlated. Several other studies have reviewed MD simulations of GPCRs [207, 208].
Time-Scales and Methodological Basis for Using MD Simulations to Address Structure-Based Drug Designing
Understanding of molecular motions is essential for perusing successful drug designing. Starting from early motionless “lock and key” MWC (Monod-Wyman-Changeux) model [209], “induced-fit” KNF (Koshland-Nemethy-Filmer) model [210] to modern conformational ensemble model [211–218], it is evident that nature uses full spectrum of conformational heterogeneity [219] for executing allosteric mechanisms. Thus, time length of simulations is an important criterion for capturing the relevant dynamics of rigid body, side-chains, backbones, local unfolding, and intrinsic disorder of proteins [218] covering full conformational space with increasing disorderness. Longer the simulations, - greater will be the chance to observe large-scale conformational changes. The nanosecond scale simulations are appropriate for observing local-fluctuations covering the coordinated motions of nearby residues and fast allosteric signals [220].
Large scale-unbiased simulations offer useful sampling approach to obtain diverse protein conformations involved in protein allostery. They are well suited to study many large-scale conformational changes [220], finding of hidden allosteric or cryptic sites [221] and complete ligand binding-unbinding events. The very recent 11 min MD simulation depicted the unbinding process of inhibitor TPPU at enzyme target soluble epoxide hydrolase [222]. They achieved pharmacologically relevant time scales using weighted ensemble-based algorithm WExplore. Large-scale unbiased simulations were used to study the spontaneous binding of beta blockers at β2-adrenergic receptors by placing them away from orthosteric binding sites [205]. The blockers bound similarly as the crystallographic poses. Their binding pathway also scanned low-sampled conformations finding hidden allosteric sites in extracellular vestibule to be targeted further. These computational studies are very expensive and not all research endeavors can avail such facilities.
Under such circumstances accelerated MD simulations (aMD) comes as handy approach. The aMD methods are very useful for proteins with slow timescale dynamics [223]. The aMD methods [224, 225] modify the potential energy landscape to escape the energy minima thus allowing state transitions normally unavailable through conventional simulations. The stochastic conformational sampling approach of Mote-Carlo simulations uses slight modification of starting conformation to obtain new one. The acceptance or rejection of obtained conformation depends on the fulfillment of potential energy criteria [220, 226]. The limitation is time-scale information connecting two conformations remains unrevealed.
MD-based Markov State Models (MSMs) uses stochastic kinetic model to describe the transition probabilities between the discrete states at a fixed time interval [220, 227–229]. To build a model it requires multiple independent simulation trajectories to enable proper sampling of entire conformational space. To understand the ligand modulation of β2-adrenergic receptor activation pathways, generation of numerous trajectories was achieved through Google cloud computing facility [230] followed with MSM based analysis. Authors found intermediate states, which were further utilized for successful virtual screening studies.
To capture structural fluctuations Normal Mode Analysis (NMA) uses harmonic approximations that decompose harmonic orthogonal modes near equilibrium into low-frequency modes having multi-atom motions [231, 232]. The use of NMA based Elastic Network Model (ENM) [233] starting from open and closed states of GABAA-Rs indicated “Twist to Turn” global motions where ECD rotates counterclockwise and TMD rotates clockwise around the central axis [23] along the transition pathway.
When all-atom modeling is unable to answer the posed biological problem due to computational constrains the coarse-grained (CG) modeling with reduced representation of biomolecule is preferred. With the use of coarse-grained (CG) simulation model increase in time length of simulations (from nano-scale to micro-scale level) can be secured by modifying all-atom membrane protein model to CG-model [234]. The CG-simulation has also been employed for the proper immersion of protein in a phospholipid bilayer [235]. CG models have been developed for detergents and lipid bilayers and CG simulations have been performed to study the interactions of membrane protein and peptides with the lipid bilayer [236]. Accurate positions of proteins of varied sizes and architecture in the lipid bilayer have also been studied with CG simulations [237]. Both all-atom and CG simulation approaches and associated problems for ion-channel proteins have been extensively reviewed by Tieleman et al. [238].
Overall, while applying conformational sampling techniques to understand modern allostery for drug designing the proper follow up of drug designing hierarchy (Figure 1B) is mandatory. After choosing subtype of functional interest the second level hierarchy of states carries immense potential as these “conformational states” can be of functional importance or hidden allosteric sites. Exploration of these states can guide the path leading us to the final level for targeting of “novel or existing sites” for structure-based drug designing with increased success rate.
Energy Calculations Using Simulations
Free energy is a state function and depends on the event of energy change from afore state to after event state irrespective of the path of energy change. To calculate drug binding affinity one can use technique called alchemical transformations [239]. In MDS the non-bonding forces from ligand are gradually turned off without incorporating any errors, practically allowing ligand to disappear from simulation [240]. This vanishing of bound ligand takes care of both protein as well as solution interactions. This indirect measurement of drugs potency requires running of two simulations to study ligand detachment from: (a) protein-ligand complex and (b) solvent-ligand complex [240]. The calculation considers ΔG bind + ΔG protein – ΔG water = 0, thus ΔG bind = ΔG water – ΔG protein gives us the free energy of binding [240]. This method has been accurately used to calculate ligand binding [241], but they need to be used cautiously for their sensitivity to incomplete sampling [242].
Other Drug-Designing Approaches
Pharmacophore Modeling and Virtual Screening
Pharmacophore modeling
To find new ligands for a protein, it is necessary to find out compounds which share a set of common three-dimensional features with the already known ligands. This set of common features in 3D space that interact with a set of complementary sites on the biological target is known as pharmacophore. The IUPAC defines a pharmacophore to be “an ensemble of steric and electronic features that is necessary to ensure the optimal supramolecular interactions with a specific biological target and to trigger (or block) its biological response” [243]. Pharmacophore key elements might be a group of atoms, a part of the volume of the molecule, “classical” pharmacophoric features like H-bond acceptors (HBA) and donors (HBD), charged or ionizable groups, hydrophobic (HY), and/or aromatic rings (RA) together with geometrical constraints like distances, angles, and dihedral angles [244]. The set of these features is known as pharmacophore model.
Virtual screening
One of the key steps in drug design process is virtual screening, which includes the identification of chemical compounds (hits) that display the desired pharmacological properties toward the specific biological target [245]. It is used as a lead identification technique and has been applied largely in recent years. Virtual screening is a complementary technique to high throughput screening (HTS) [113]. It has several advantages over experimental HTS as a lead identification method: higher number of compounds can be screened within a reasonable amount of time than traditional HTS. Second, compounds with predicted activity against the biological target must be tested in vitro, thus reducing the cost at a considerable amount. Third, compounds can be built into virtual libraries for screening that have not yet been synthesized, saving the considerable time and expense of building a screening library for high-throughput screening. Virtual screening can be classified into two types: ligand-based approaches which do not utilize the structure of the biological target in screening, and structure-based approaches, which utilize the structure of the biological target. Different approaches for virtual screening have been identified which involve searching of existing databases using pharmacophore and other ligand and structure-based information [245]. The pharmacophore based virtual screening can be very useful for finding new ligands with different chemical scaffolds. The schematic representation of pharmacophore-based screening approach in shown in Figure 6 [244].
Use of Drug Designing Techniques for GPCR Drug Discovery
The binding site of class A GPCRs is located between TM3-TM6. The major binding requirement of GPCRs is fulfilled by aspartic acid of TM3 which forms salt bridge with a protonated nitrogen of the ligands. However, compounds with non-protonated nitrogen have been identified to be active toward κ opioid receptor (class A GPCR) [246] and also the binding requirements of such compounds have been identified using docking studies. The structure of the active compound is shown in Figure 7 [247]. The other interactions are mainly hydrophobic in nature formed with residues from TM4, TM5, and TM6. Several computational studies have been performed to identify the binding sites, generating a pharmacophore based on the binding site and designing new ligands for specific receptors. A pharmacophore derived for opioid class of receptors (class A GPCRs) show four minimum features as binding requirements of ligands to the receptors [248] comprising of one positive charge, one aromatic interaction, and two hydrophobic interactions (Figure 8). Hits for both μ and κ opioid receptors were predicted using integrated computational approach involving shape based virtual screening methodology [248]. Quantitative structure-activity relationship studies (QSAR), which correlate various physicochemical, topological, and structural properties of compounds with their biological activities, have been used to predict the activities of the new hits leading to the enrichment of hits [248].
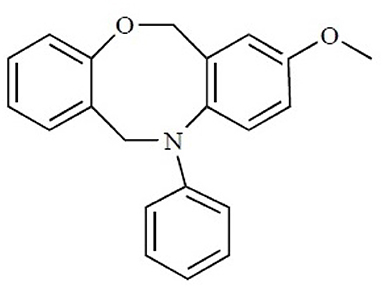
Figure 7. The chemical structure of the non-protonated ligand showing activity toward κ-opioid receptor. Reproduced with permission from Bentham Science Publishers [247].
The structure of the binding sites and the important interactions with the ligands were derived for various GPCR receptors such as human D2 DR, human β2 AR, human M1 MAR, human CCR1 chemokine receptor, and mouse MrgC11 receptors [249]. They have docked ligands using HierDock procedure [250] or MSCDock [251]. This study was able to identify difference between the agonist and antagonist binding for the different receptors. The antagonists of α1A adrenergic receptor are discovered using virtual screening [252], which was guided by 3D pharmacophoric model. The identified hits were docked to the homology model of α1A adrenergic receptor using GOLD. The top ranked hits were evaluated using radioligand binding assay. Thirty-seven compounds were identified with better Ki values. In another study structure-based pharmacophore was used to identify antagonists for cannabinoid receptor [253]. In this study authors have first generated an antagonist bound complex of the cannabinoid receptor and then used MD simulations to optimize the system. The binding requirements of the antagonist were analyzed, and they have further refined the binding pocket using docking of known antagonists to the receptor and developed a consensus scoring which could differentiate between potential antagonist and any random compound. The X-ray structure of the β2 adrenergic receptor was identified in the ground state which was not sufficient to distinguish between binding of agonists and antagonists. A study aimed to generate correct conformational states of β2 adrenergic receptor was carried out for binding of agonists and antagonists [254]. Novel compounds as potent ligands for Dopamine 3 (D3) subtype receptor were identified using pharmacophore based virtual screening [255]. Structure-based pharmacophore modeling and virtual screening of large databases were used for identification of novel D3 ligands. Finally, eight promising compounds were identified after D3 binding assay. Selective agonists of κ-opioid receptor and allosteric modulators of μ-opioid receptor have been identified using structure and ligand-based computational approaches [256, 257].
Applicability and availability of various ligand-based and structure-based computational techniques for identification of drug candidates in GPCRs have been thoroughly reviewed by Basith et al [258]. The virtual screening strategy has been successful in identifying hits for different GPCR targets. Various ligands for both orthosteric and allosteric sites have been identified in the past few years [259, 260]. GPCR focused libraries have also been made available in database such as Chembridge, ChemDiv, Asinex among others [258]. However, several limitations exist which effect the success rates of these strategies. One of the major limitation is the error in the model development based on cheminformatics tools. These errors may be due to flaws in the statistical models or due to experimental data which are taken into account while developing the model [258, 261, 262]. In spite of availability of large no. of databases, the entries in the databases are often erroneous due to errors in ligand structures, bioactivities, activity types [263, 264]. Another major limitation is the unavailability of accurate approximation of the energy terms which result in false prediction of hits [258]. Though there has been significant increase in the computational resources, calculation of energetic terms, and free energy calculations are still not accurate. And finally, though the number of crystallographic structures of GPCRs has increased recently, huge number of GPCR structures still remain unresolved. Also, since it is now known that there exist several intermediate states in between active and inactive states, these intermediate conformations need to be captured either by experimental or theoretical molecular simulation approaches. These states play a crucial role in alternate signal transduction pathways and subtype selectivity of GPCRs.
Conclusion
Drug discovery is difficult task but when it comes to CNS drug discovery it is even more challenging and complex. The underlining obvious reason is the enormous complexity of highly operative brain. The speedy intercellular neurotransmissions commence through the IMPs. Understanding the structural biology of membrane proteins is limited due to inherent difficulties to crystalize them and their heteromeric nature. To understand normal CNS mechanisms vs. abnormal CNS pathophysiological conditions computational approaches have greatly assisted. In this review, we have mentioned the use of important computational methods which have helped CNS drug discovery program over the time, irrespective of the hurdles for studying it with experimental methods. The methods used and their progress have exclusively been focused on LGIC representative GABAA-R and GPCR representative class A GPCRs. The observations from computational studies need to be linked with experimental studies for further validation/support with the recent technology development. This interdisciplinary approach increases the chances of reaching success in CNS drug discovery endeavors by several folds. In this era of technological development, both methods complement each other and they are inseparable. In summary, the success in simultaneous and resourceful utilization of these two approaches can fill the gap in between, which is acting as bottleneck. The hierarchical considerations of subtypes (functionally selective), then states (captured from ensemble of conformations) and finally the sites (orthosteric and allosteric) are the roadmap to follow for efficient structure-function understanding. The modern interdisciplinary approaches hold the potential to hunt and couple normal vs. abnormal (patho-) physiology of CNS for the benefit of drug discovery-oriented neuro-pharmacological research.
Author Contributions
All authors listed have made a substantial, direct and intellectual contribution to the work, and approved it for publication.
Conflict of Interest Statement
The authors declare that the research was conducted in the absence of any commercial or financial relationships that could be construed as a potential conflict of interest.
Acknowledgments
This work was supported by the Council of Scientific and Industrial Research (CSIR), New Delhi under E.S. grant [sanction letter no. 21(0918)/12/EMR-II]. PP thanks Council of Scientific and Industrial Research (CSIR) for providing Senior Research Fellowship under E.S. scheme of NG.
Abbreviations
pLGICS, pentameric ligand gated ion channels; GPCRs, G-protein-coupled receptors; SBDD, structure-based drug designing; IMPs, integral membrane proteins; TMD, trans-membrane domain; ECD, extra cellular domain; IC, intra-cellular; TM, transmembrane; GABA-Rs, γ-amino butyric acid receptors; IPSP, inhibitory postsynaptic potential; CNS, central nervous system; NT, neurotransmitter; IFD, induced fit docking; VSCC, voltage-sensitive calcium channel; BZD, benzodiazepine; GLIC, Gloeobacter violaceus; CCK, cholecystokinin; β1AR, β1-adrenergic receptor; β2AR, β2-adrenergic receptor, A2A-adenosine receptor; MDS, Molecular dynamics simulations; POPC, 1-palmitoyl-2-oleoyl-sn-glycero-3-phosphocholine; CXCR4, chemokine receptor 4; aMD, accelerated molecular dynamics simulations; MSMs, Markov State Models; NMA, normal mode analysis; ENM, Elastic Network Model; CG, coarse-grained; HBA, H-bond acceptors; HBD, H-bond donors; HY, hydrophobic; RA, aromatic rings; HTS, high throughput screening; QSAR, Quantitative structure-activity relationship; RMSD, root mean-square deviation; nACh-Rs, nicotinic acetylcholine receptors; cryo-EM, cryo-electron microscopy.
Footnotes
References
1. Alberts B, Johnson A, Lewis J, Raff M, Roberts K, Walter P. Molecular Biology of the Cell. New York, NY: Garland Science (2002).
3. Lodish H, Berk A, Zipursky SL, Matsudaira P, Baltimore D, Darnell J. Molecular Cell Biology. Section 3.4, Membrane Proteins. New York, NY: W. H. Freeman (2000).
4. Almeida JG, Preto AJ, Koukos PI, Bonvin AM, Moreira IS. Membrane proteins structures: a review on computational modeling tools. Biochim Biophys Acta (2017) 1859:2021–39. doi: 10.1016/j.bbamem.2017.07.008.
5. Bloom FE. Neurotransmission and the central nervous system. In: Brunton LL, editor. Goodman Gilman's the Pharmacological Basis of Therapeutics. New York, NY: McGraw-Hill (2006). p. 330–31.
6. Olsen RW, Sieghart W. International Union of Pharmacology. LXX. Subtypes of gamma-aminobutyric acid(A) receptors: classification on the basis of subunit composition, pharmacology, and function. Pharmacol Rev. (2008) 60:243–60. doi: 10.1124/pr.108.00505
7. Belelli D, Harrison NL, Maguire J, Macdonald RL, Walker MC, Cope DW. Extrasynaptic GABAA receptors: form, pharmacology, and function. J Neurosci. (2009) 29:12757–63. doi: 10.1523/JNEUROSCI.3340–09.2009
8. Lynch JW. Native glycine receptors and their physiological roles. Neuropharmacology (2009) 56:303–9. doi: 10.1016/j.neuropharm.2008.07.034
9. Yevenes GE, Zeilhofer HU. Allosteric modulation of glycine receptors. Br J Pharmacol. (2011) 164:224–36. doi: 10.1111/j.1476–5381.2011.01471.x
10. Corringer PJ, Baaden M, Bocquet N, Delarue M, Dufresne V, Nury H, et al. Atomic structure dynamics of pentameric ligand-gated ion channels: new insight from bacterial homologues. J. Physiol. (2010) 588:565–72. doi: 10.1113/jphysiol.2009.183160
11. Thompson AJ, Lester HA, Lummis SC. The structural basis of function in Cys-loop receptors. Q Rev Biophys. (2010) 43:449–99. doi: 10.1017/S0033583510000168
12. Miller PS, Smart TG. Binding, activation and modulation of Cys-loop receptors. Trends Pharmacol Sci. (2010) 31:161–74. doi: 10.1016/j.tips.2009.12.005
13. Millar NS, Gotti C. Diversity of vertebrate nicotinic acetylcholine receptors. Neuropharmacology (2009) 56:237–46. doi: 10.1016/j.neuropharm.2008.07.041
14. Changeux JP. Allosteric receptors: from electric organ to cognition. Annu Rev Pharmacol Toxicol. (2010) 50:1–38. doi: 10.1146/annurev.pharmtox.010909.105741
15. Barnes NM, Hales TG, Lummis SCR, Peters JA. The 5-HT3 receptor – the relationship between structure and function. Neuropharmacology (2009) 56:273–84. doi: 10.1016/j.neuropharm.2008.08.003
16. Walstab J, Rappold G, Niesler B. 5-HT3 receptors: role in disease and target of drugs. Pharmacol Ther. (2010) 128:146–69. doi: 10.1016/j.pharmthera.2010.07.001
17. Overington JP, Al-Lazikani B, Hopkins AL. How many drug targets are there? Nat. Rev. Drug. Discov. (2006) 5:993–6. doi: 10.1038/nrd2199
18. Lundstrom K. An overview on GPCRs and drug discovery: structure-based drug design and structural biology on GPCRs. In: Leifert WR, editor. G Protein-Coupled Receptors in Drug Discovery. Boca Raton, FL: Springer Verlag Publication (2009). p. 51–66.
19. Lagerström MC, Schiöth HB. Structural diversity of G protein-coupled receptors and significance for drug discovery. Nat Rev Drug Discov. (2008) 7:339–57. doi: 10.1038/nrd2518
20. Tasneem A, Iyer LM, Jakobsson E, Aravind L. Identification of the prokaryotic ligand-gated ion channels their implications for the mechanisms origins of animal Cys-loop ion channels. Genome Biol. (2005) 6:R4. doi: 10.1186/gb-2004-6-1-r4
21. Dent JA. Evidence for a diverse Cys-loop ligand-gated ion channel superfamily in early bilateria. J Mol Evol. (2006) 62:523–35. doi: 10.1007/s00239–005-0018–2
22. Dent JA. The evolution of pentameric ligand-gated ion channels. Adv Exp Med Biol. (2010) 683:11–23. doi: 10.1007/978–1-4419–6445-8_2
23. Vijayan RS, Trivedi N, Roy SN, Bera I, Manoharan P, Payghan PV, et al. Modeling the closed and open state conformations of the GABA(A) ion channel—plausible structural insights for channel gating. J Chem Inf Model. (2012) 52:2958–69. doi: 10.1021/ci300189a
24. Bjarnadóttir TK, Gloriam DE, Hellstr SH, Kristiansson H, Fredriksson R, Schiöth HB. Comprehensive repertoire and phylogenetic analysis of the G protein-coupled receptors in human mouse. Genomics (2006) 88:263–73. doi: 10.1016/j.ygeno.2006.04.001
25. Perez DM. The evolutionarily triumphant G-protein-coupled receptor. Mol Pharmacol. (2003) 63:1202–5. doi: 10.1124/mol.63.6.1202
26. Römpler H, Stäubert C, Thor D, Schulz A, Hofreiter M, Schöneberg T. G protein-coupled time travel: evolutionary aspects of GPCR research. Mol Intervent. (2007) 7:17–25. doi: 10.1124/mi.7.1.5
27. Lemoine D, Jiang R, Taly A, Chataigneau T, Specht A, Grutter T. Ligand-gated ion channels: new insights into neurological disorders and ligand recognition. Chem Rev. (2012) 112:6285–318. doi: 10.1021/cr3000829
28. Collingridge GL, Olsen RW, Peters J, Spedding M. A nomenclature for ligand-gated ion channels. Neuropharmacology (2009) 56:2–5. doi: 10.1016/j.neuropharm.2008.06.063
29. Smith GB, Olsen RW. Functional domains of GABAA receptors. Trends Pharmacol Sci. (1995) 16:162–8. doi: 10.1016/S0165–6147(00)89009–4
30. DeLorey TM, Olsen RW. Gamma-aminobutyric acidA receptor structure and function. J Biol Chem. (1992) 267:16747–50.
31. Unwin N. Acetylcholine receptor channel imaged in the open state. Nature (1995) 373:37–43. doi: 10.1038/373037a0
32. Rudolph U, Knoflach F. Beyond classical benzodiazepines: novel therapeutic potential of GABAA receptor subtypes. Nat Rev Drug Discov. (2011) 10:685–97. doi: 10.1038/nrd3502
33. Sieghart W, Sperk G. Subunit composition, distribution and function of GABA(A) receptor subtypes. Curr Top Med Chem. (2002) 2:795–816. doi: 10.2174/1568026023393507
34. Mohler H, Fritschy JM, Rudolph U. A new benzodiazepine pharmacology. J Pharmacol Exp Ther. (2002) 300:2–8. doi: 10.1124/jpet.300.1.2
35. Sieghart W. Subunit composition and structure of GABAA receptor subtypes. In: Enna SJ, Möhler H, editors. The GABA Receptors. Totowa, NJ: Humana Press Inc. (2007). p. 69–86.
36. Connolly CN, Krishek BJ, McDonald BJ, Smart TG, Moss SJ. Assembly and cell surface expression of heteromeric and homomeric gamma-aminobutyric acid type A receptors. J Biol Chem. (1996) 271:89–96. doi: 10.1074/jbc.271.1.89
37. Enna SJ. The GABA receptors. In: Enna SJ, Möhler H, editors. The GABA Receptors. Totowa, NJ: Humana Press Inc. (2007). p. 1–15.
38. Sieghart W. Structure and pharmacology of gamma-aminobutyric acidA receptor subtypes. Pharmacol Rev. (1995) 47:181–234.
39. Miller PS, Aricescu AR. Crystal structure of a human GABAA receptor. Nature (2014) 512:270–5. doi: 10.1038/nature13293
40. Puthenkalam R, Hieckel M, Simeone X, Suwattanasophon C, Feldbauer RV, Ecker GF, et al. Structural studies of GABAA receptor binding sites: which experimental structure tells us what? Front Mol Neurosci. (2016) 9:44. doi: 10.3389/fnmol.2016.00044
41. Smith GB, Olsen RW. Identification of a [3H] muscimol photo affinity substrate in the bovine γ-aminobutyric acid A receptor α subunit. J Biol Chem. (1994) 269:20380–7.
42. Cherubini E, Gaiarsa JL, Ben-Ari Y. GABA: an excitatory transmitter in early postnatal life. Trends Neurosci. (1991) 14:515–9. doi: 10.1016/0166–2236(91)90003-D
43. Sieghart W, Ernst M. Heterogeneity of GABAA receptors: revived interest in the development of subtype-selective drugs. Curr Med Chem. (2005) 5:217–42. doi: 10.2174/1568015054863837
44. Gloriam DE, Fredriksson R, Schioth HB. The G protein-coupled receptor subset of the rat genome. BMC Genomics (2007) 8:338. doi: 10.1186/1471–2164-8–338
45. Fredriksson R, Lagerstrom MC, Lundin LG, Schioth HB. The G protein coupled receptors in the human genome form five main families. Phylogenetic analysis, paralogon groups, and fingerprints. Mol Pharmacol. (2003) 63:1256–72. doi: 10.1124/mol.63.6.1256
46. Tyndall JD, Sandilya R. GPCR agonists and antagonists in the clinic. Med Chem. (2005) 1:405–21. doi: 10.2174/1573406054368675
47. Ballesteros J, Palczewski K. G protein-coupled receptor drug discovery: implications from the crystal structure of rhodopsin. Curr Opin Drug Discov Dev. (2001) 4:561–74.
48. Ballesteros JA, Weinstein H. Integrated methods for the construction of three-dimensional models computational probing of structure-function relations in G protein-coupled receptors. Methods Neurosci. (1995) 25:366–428. doi: 10.1016/S1043–9471(05)80049–7
49. Caltabiano G, Gonzalez A, Cordomí A, Campillo M, Pardo L. The role of hydrophobic amino acids in the structure and function of the rhodopsin family of G protein-coupled receptors. Methods Enzymol. (2013) 520:99–115. doi: 10.1016/B978–0-12–391861-1.00005–8
50. Sansuk K, Deupi X, Torrecillas IR, Jongejan A, Nijmeijer S, Bakker RA, et al. A structural insight into the reorientation of transmembrane domains 3 and 5 during family A G protein-coupled receptor activation. Mol Pharmacol. (2011) 79:262–9. doi: 10.1124/mol.110.066068
51. Rasmussen SG, DeVree BT, Zou Y, Kruse AC, Chung KY, Kobilka TS, et al. Crystal structure of the beta2 adrenergic receptor-Gs protein complex. Nature (2011) 477:549–55. doi: 10.1038/nature10361
52. Whiteford HA, Ferrari AJ, Degenhardt L, Feigin V, Vos T. The global burden of mental, neurological and substance use disorders: an analysis from the Global Burden of Disease Study 2010. PLoS ONE (2015) 10:e0116820. doi: 10.1371/journal.pone.0116820
53. Bloom FE, Iversen LL. Localizing 3H-GABA in nerve terminals of rat cerebral cortex by electron microscopic autoradiography. Nature (1971) 229:628–30. doi: 10.1038/229628a0
54. Johnston G.A. GABAA receptor channel pharmacology. Curr Pharm Des. (2005) 11:1867–85. doi: 10.2174/1381612054021024
55. Sieghart W. Structure, pharmacology, and function of GABAA receptor subtypes. Adv Pharmacol. (2006) 54:231–63. doi: 10.1016/S1054–3589(06)54010–4
56. Chisholm D, Sweeny K, Sheehan P, Rasmussen B, Smit F, Cuijpers P, et al. Scaling-up treatment of depression and anxiety: a global return on investment analysis. Lancet Psychiatry (2016) 3:415–24. doi: 10.1016/S2215–0366(16)30024–4
57. Nicoll RA. The coupling of neurotransmitter receptors to ion channels in the brain. Science (1988) 241:545–51. doi: 10.1126/science.2456612
58. Kandel ER, Schwartz JH. Molecular biology of learning: modulation of transmitter release. Science (1982) 218:433–43. doi: 10.1126/science.6289442
59. Vaughan CW, Ingram SL, Connor MA, Christie MJ. How opioids inhibit GABA-mediated neurotransmission. Nature (1997) 390:611–4. doi: 10.1038/37610.
60. Kulik A, Vida I, Fukazawa Y, Guetg N, Kasugai Y, Marker CL, et al. Compartment-dependent colocalization of Kir3.2-containing K+ channels and GABAB receptors in hippocampal pyramidal cells. J Neurosci. (2006) 26:4289–97. doi: 10.1523/JNEUROSCI.4178–05.2006
61. Mintz IM, Bean BP. GABAB receptor inhibition of P-type Ca2+ channels in central neurons. Neuron (1993) 10:889–98. doi: 10.1016/0896–6273(93)90204–5
62. Atak JR. GABAA receptor subtype–selective modulators. I. α2/α3-selective agonists as non-sedating anxiolytics. Curr Top Med Chem. (2011) 11:1176–202. doi: 10.2174/156802611795371350
63. Korpi ER, Mattila MJ, Wisden W, Lüddens H. GABA(A)-receptor subtypes: clinical efficacy and selectivity of benzodiazepine site ligands. Ann Med. (1997) 29:275–82. doi: 10.3109/07853899708999348
64. Sigel E, Lüscher BP. A closer look at the high affinity benzodiazepine binding site on GABAA receptors. Curr Top Med Chem. (2011) 11:241–6. doi: 10.2174/156802611794863562
65. Rudolph U, Mohler H. Analysis of GABAA receptor function and dissection of the pharmacology of benzodiazepines general anaesthetics through mouse genetics. Annu Rev Pharmacol Toxicol. (2004) 44:475–98. doi: 10.1146/annurev.pharmtox.44.101802.121429
66. Gielen MC, Lumb MJ, Smart TG. Benzodiazepines modulate GABAA receptors by regulating the preactivation step after GABA binding. J Neurosci. (2012). 32:5707–15. doi: 10.1523/JNEUROSCI.5663–11.2012.
67. Brunig I, Scotti E, Sidler C, Fritschy JM. Intact sorting, targeting, clustering of γ-aminobutyric acid A receptor subtypes in hippocampal neurons in vitro. J Comp Neurol. (2002) 443:43–55. doi: 10.1002/cne.10102
68. Payghan PV, Bera I, Bhattacharyya D, Ghoshal N. Capturing state-dependent dynamic events of GABAA-receptors: a microscopic look into the structural and functional insights. J Biomol Struct Dyn. (2016) 34:1818–37. doi: 10.1080/07391102.2015.1094410
69. Grutter T, Bertr S, Kotzyba-Hibert F, Bertr D, Goeldner M. Structural reorganization of the acetylcholine binding site of the torpedo nicotinic receptor as revealed by dynamic photoaffinity labeling. ChemBioChem (2002) 3:652–8. doi: 10.1002/1439-7633(20020703)3:7<652::AID-CBIC652>3.0.CO;2-L
70. Celie PH, Kasheverov IE, Mordvintsev DY, Hogg RC, van Nierop P, et al. Crystal structure of nicotinic acetylcholine receptor homolog AChBP in complex with an alpha-conotoxin PnIA variant. Nat Struct Mol Biol. (2005) 12:582–8. doi: 10.1038/nsmb951
71. Bourne Y, Talley TT, Hansen SB, Taylor P, Marchot P. Crystal structure of a Cbtx–AChBP complex reveals essential interactions between snake α-neurotoxins nicotinic receptors. EMBO J. (2005) 24:1512–22. doi: 10.1038/sj.emboj.7600620
72. Venkatachalan SP, Czajkowski C. A conserved salt bridge critical for GABAA receptor function loop C dynamics. Proc Natl Acad Sci USA. (2008) 105:13604–9. doi: 10.1073/pnas.0801854105
73. Mozrzymas JW, Barberis A, Mercik K, Zarnowska ED. Binding sites, singly bound states, conformation coupling shape GABA-evoked currents. J Neurophysiol. (2003) 89:871–83. doi: 10.1152/jn.00951.2002
74. Indurthi DC, Lewis TM, Ahring PK, Balle T, Chebib M, Absalom NL, et al. Ligand binding at the α4-α4 agonist-binding site of the α4β2 nAChR Triggers receptor activation through a pre-activated conformational state. PLoS ONE (2016) 11:e0161154. doi: 10.1371/journal.pone.0161154
75. Baumann SW, Baur R, Sigel E. Individual properties of the two functional agonist sites in GABA(A) receptors. J Neurosci. (2003) 23:11158–66. doi: 10.1523/JNEUROSCI.23–35-11158.2003
76. Baur R, Sigel E. Benzodiazepines affect channel opening of GABAA receptors induced by either agonist binding site. Mol Pharmacol. (2005) 67:1005–8. doi: 10.1124/mol.104.008151
77. Lütjens R, Rocher JP. Recent advances in drug discovery of GPCR allosteric modulators for neurodegenerative disorders. Curr Opin Pharmacol. (2017) 32:91–5. doi: 10.1016/j.coph.2017.01.001
78. Dalet FG, Guadalupe TF, Del Carmen CH, Humberto GA, Antonio SU. Insights into the structural biology of G-protein coupled receptors impacts drug design for central nervous system neurodegenerative processes. Neural Regen Res. (2013) 8:2290–302. doi: 10.3969/j.issn.1673-5374.2013.24.009
79. Warne T, Serrano-Vega MJ, Baker JG, Moukhametzianov R, Edwards PC, et al. Structure of a beta1-adrenergic G-protein-coupled receptor. Nature (2008) 454:486–91. doi: 10.1038/nature07101.
80. Zocher M, Zhang C, Rasmussen SG, Kobilka BK, Müller DJ. Cholesterol increases kinetic, energetic, and mechanical stability of the human β2-adrenergic receptor. Proc Natl Acad Sci USA. (2012) 109: E3463–72. doi: 10.1073/pnas.1210373109
81. Fuxe K, Borroto-Escuela DO, Marcellino D, Romero-Fernandez W, Frankowska M, Guidolin D, et al. GPCR heteromers and their allosteric receptor-receptor interactions. Curr Med Chem. (2012) 19:356–63. doi: 10.2174/092986712803414259
82. Franco R, Casadó V, Cortés A, Ferrada C, Mallol J, Woods A, et al. Basic concepts in G-protein-coupled receptor homo- and heterodimerization. Sci World J. (2007) 7:48–57. doi: 10.1100/tsw.2007.197
83. Melancon BJ, Hopkins CR, Wood MR, Emmitte KA, Niswender CM, Christopoulos A, et al. Allosteric modulation of seven transmembrane spanning receptors: theory, practice, and opportunities for central nervous system drug discovery. J Med Chem. (2012) 55:1445–64. doi: 10.1021/jm201139r
84. Brejc K, van Dijk WJ, Klaassen RV, Schuurmans M, van Der Oost J, Smit AB, et al. Crystal structure of an ACh-binding protein reveals the ligand-binding domain of nicotinic receptors. Nature (2001) 411:269–76. doi: 10.1038/35077011
85. Hansen SB, Sulzenbacher G, Huxford T, Marchot P, Taylor P, Bourne Y. Structures of Aplysia AChBP complexes with nicotinic agonists antagonists reveal distinctive binding interfaces conformations. EMBO J. (2005) 24:3635–46. doi: 10.1038/sj.emboj.7600828
86. Miyazawa A, Fujiyoshi Y, Unwin N. Structure gating mechanism of the acetylcholine receptor pore. Nature (2003) 423:949–55. doi: 10.1038/nature01748
87. Unwin N. Refined structure of the nicotinic acetylcholine receptor at 4 Å resolution. J Mol Biol. (2005) 346:967–89. doi: 10.1016/j.jmb.2004.12.031
88. Hilf RJC, Dutzler R. X-ray structure of a prokaryotic pentameric ligand-gated ion channel. Nature (2008) 452:375–9. doi: 10.1038/nature06717
89. Bocquet N, Nury H, Baaden M, Le Poupon C, Changeux JP, Delarue M, et al. X-ray structure of a pentameric ligand-gated ion channel in an apparently open conformation. Nature (2009) 457:111–4. doi: 10.1038/nature07462
90. Hibbs RE, Gouaux E. Principles of activation permeation in an anion-selective Cys-loop receptor. Nature (2011) 474:54–60. doi: 10.1038/nature10139
91. Nury H, Van Renterghem C, Weng Y, Tran A, Baaden M, Dufresne V, et al. X-ray structures of general anaesthetics bound to a pentameric ligand-gated ion channel. Nature (2011) 469:428–31. doi: 10.1038/nature09647
92. Althoff T, Hibbs RE, Banerjee S, Gouaux E. X-ray structures of GluCl in apo states reveal a gating mechanism of Cys-loop receptors. Nature (2014) 512:333–7. doi: 10.1038/nature13669
93. Hassaine G, Deluz C, Grasso L, Wyss R, Tol MB, Hovius R, et al. X-ray structure of the mouse serotonin 5-HT3 receptor. Nature (2014) 512:276–81. doi: 10.1038/nature13552
94. Huang X, Chen H, Michelsen K, Schneider S, Shaffer PL. Crystal structure of human glycine receptor-α3 bound to antagonist strychnine. Nature (2015) 526:277–80. doi: 10.1038/nature14972
95. Huang X, Chen H, Shaffer PL. Crystal structures of human GlyRα3 bound to ivermectin. Structure (2017) 25:945–950.e2. doi: 10.1016/j.str.2017.04.007
96. Palczewski K, Kumasaka T, Hori T, Behnke CA, Motoshima H, Fox BA, et al. Crystal structure of rhodopsin: A G protein-coupled receptor. Science (2000) 289:739–45. doi: 10.1126/science.289.5480.739
97. Murakami M, Kouyama T. Crystal structure of squid rhodopsin. Nature (2008) 453:363–7. doi: 10.1038/nature06925
98. Cherezov V, Rosenbaum DM, Hanson MA, Rasmussen SG, Thian FS, Kobilka TS, et al. High-resolution crystal structure of an engineered human beta2-adrenergic G protein-coupled receptor. Science (2007) 318:1258–65. doi: 10.1126/science.1150577
99. Wacker D, Fenalti G, Brown MA, Katritch V, Abagyan R, Cherezov V, et al. Conserved binding mode of human beta2 adrenergic receptor inverse agonists antagonist revealed by X-ray crystallography. J Am Chem Soc. (2010) 132:11443–5. doi: 10.1021/ja105108q
100. Rosenbaum DM, Zhang C, Lyons JA, Holl R, Aragao D, Arlow DH, et al. Structure and function of an irreversible agonist-beta2 adrenoceptor complex. Nature (2011) 469:236–40. doi: 10.1038/nature09665
101. Warne T, Moukhametzianov R, Baker JG, Nehme R, Edwards PC, Henderson R, et al. Structure of a beta1-adrenergic G-protein-coupled receptor. Nature (2011) 469:241–4. doi: 10.1038/nature09746
102. Jaakola VP, Griffith MT, Hanson MA, Cherezov V, Chien EY, Lane JR, et al. The 2.6 angstrom crystal structure of a human A2A adenosine receptor bound to an antagonist. Science (2008) 322:1211–7. doi: 10.1126/science.1164772
103. Xu F, Wu H, Katritch V, Han GW, Jacobson KA, Gao ZG, Cherezov V, et al. Structure of an agonist-bound human A2A adenosine receptor. Science (2011) 332:322–7. doi: 10.1126/science.1202793
104. Wu H, Wacker D, Mileni M, Katritch V, Han GW, Vardy E, et al. Structure of the human kappa-opioid receptor in complex with JDTic. Nature (2012) 485:327–32. doi: 10.1038/nature10939
105. Manglik A, Kruse AC, Kobilka TS, Thian FS, Mathiesen JM, Sunahara RK, et al. Crystal structure of the mu-opioid receptor bound to a morphinan antagonist. Nature (2012) 485:321–6. doi: 10.1038/nature10954
106. Granier S, Manglik A, Kruse AC, Kobilka TS, Thian FS, Weis WI, et al. Structure of the delta-opioid receptor bound to naltrindole. Nature (2012) 485:400–4. doi: 10.1038/nature11111
107. Hughes JP, Rees S, Kalindjian SB, Philpott KL. Principles of early drug discovery. Br J Pharmacol. (2011) 162:1239–49. doi: 10.1111/j.1476–5381.2010.01127.x
108. Drews J. Drug discovery: a historical perspective. Science (2000) 287:1960–4. doi: 10.1126/science.287.5460.1960
109. Terstappen GC, Schlüpen C, Raggiaschi R, Gaviraghi G. Target deconvolution strategies in drug discovery. Nat Rev Drug Discov. (2007) 6:891–903. doi: 10.1038/nrd2410
110. O'Driscoll C. “A virtual space odyssey,”, in Charting Chemical Space: Finding New Tools to Explore Biology. New York, NY: Nature Publishing Group (2004). p. 1–4.
111. von Itzstein M, Wu WY, Kok GB, Pegg MS, Dyason JC, Jin B, et al. Rational design of potent sialidase-based inhibitors of influenza virus replication. Nature (1993) 363:418–23. doi: 10.1038/363418a0
112. Jorgensen WL. The many roles of computation in drug discovery. Science (2004) 303:1813–8. doi: 10.1126/science.1096361
113. Bajorath J. Integration of virtual and high-throughput screening. Nat Rev Drug Discov. (2002) 1:882–94. doi: 10.1038/nrd941
114. Stahl M, Guba W, Kansy M. Integrating molecular design resources within modem drug discovery research: the Roche experience. Drug Discov Today (2006) 11:326–33. doi: 10.1016/j.drudis.2006.02.008
115. Sams-Dodd F. Target-based drug discovery: is something wrong? Drug Discov Today (2005) 10:139–47. doi: 10.1016/S1359–6446(04)03316–1
116. Butcher EC. Can cell systems biology rescue drug discovery? Nat Rev Drug Discov. (2005) 4:461–7. doi: 10.1038/nrd1754
117. Henry CM. Structure-based drug design. Chem Eng News (2001) 79:69–78. doi: 10.1021/cen-v079n023.p069
118. Kuhlbrandt W. Biochemistry. The resolution revolution. Science (2014) 343:1443–4. doi: 10.1126/science.1251652
119. Lau C, Hunter MJ, Stewart A, Perozo E, Vandenberg JI. Never at rest: insights into the conformational dynamics of ion channels from cryo-electron microscopy. J Physiol. (2018) 596:1107–19. doi: 10.1113/JP274888
120. Basak S, Gicheru Y, Samanta A, Molugu SK, Huang W, Fuente M, et al. Cryo-EM structure of 5-HT3A receptor in its resting conformation. Nat Commun. (2018) 9:514. doi: 10.1038/s41467–018-02997–4
121. Liang YL, Khoshouei M, Glukhova A, Furness SG, Zhao P, Clydesdale L, et al. Phase-plate cryo-EM structure of a biased agonist-bound human GLP-1 receptor–Gs complex. Nature (2018) 555:121–5. doi: 10.1038/nature25773
122. Zhang Y, Sun B, Feng D, Hu H, Chu M, Qu Q, et al. Cryo-EM structure of the activated GLP-1 receptor in complex with a G protein. Nature (2017) 546:248–53. doi: 10.1038/nature22394
123. Raghuraman H, Islam SM, Mukherjee S, Roux B, Perozo E. Dynamics transitions at the outer vestibule of the KcsA potassium channel during gating. Proc Natl Acad Sci USA. (2014) 111:1831–6. doi: 10.1073/pnas.1314875111
124. Raghuraman H, Cordero-Morales JF, Jogini V, Pan AC, Kollewe A, Roux B, et al. Mechanism of Cd2+ coordination during slow inactivation in potassium channels. Structure (2012) 20:1332–42. doi: 10.1016/j.str.2012.03.027
125. Höltje H, Sippl W, Rognan D, Folkers G. Molecular Modeling: Basic Principles and Applications. Weinheim, DE: Wiley-VCH (2003).
126. Blundell TL, Siba BL, Sternberg MJE, Thornton JM. Knowledge-based prediction of protein structures the design of novel molecules. Nature (1987) 326:347–52. doi: 10.1038/326347a0
127. Martí-Renom MA, Stuart AC, Fiser A, Sánchez R, Melo F, Šali A. Comparative protein structure modeling of genes genomes. Annu Rev Biophys Biomol Struct. (2000) 29:291–325. doi: 10.1146/annurev.biophys.29.1.291
128. Lesk AM, Chothia C. How different amino acid sequences determine similar protein structures: the structure evolutionary dynamics of the globins. J Mol Biol. (1980) 136: 225–230. doi: 10.1016/0022-2836(80)90373-3
129. Chothia C, Lesk AM. The relation between the divergence of sequence and structure in proteins. EMBO J. (1986) 5:823–6.
130. Fiser A Šali A. Modeller: generation and refinement of homology-based protein structure models. Methods Enzymol. (2003) 374:461–91. doi: 10.1016/S0076–6879(03)74020–8
131. Webb B, Sali A. Protein structure modeling with MODELLER. Protein Struct. Predict. (2014) 426:145–59. doi: 10.1007/978–1-4939–0366-5_1
132. Berman H, Westbrook J, Feng Z, Gillil G, Bhat T, Weissig H, et al. The Protein data bank. Nucleic Acids Res. (2000) 28:235–42. doi: 10.1093/nar/28.1.235
133. Rost B. Twilight zone of protein sequence alignments. Protein Eng. (1999) 12:85–94. doi: 10.1093/protein/12.2.85
134. Altschul S, Gish W, Miller W, Myers E, Lipman D. Basic local alignment search tool. J Mol Biol. (1990) 215:403–10. doi: 10.1016/S0022–2836(05)80360–2
135. Altshcul S, Madden T, Schaffer A, Zhang J, Zhang Z, Miller W, et al. Gapped BLAST PSI-BLAST: a new generation of protein database search programs. Nucleic Acids Res. (1997) 25:3389–402. doi: 10.1093/nar/25.17.3389
136. Smith TF, Waterman MS. Identification of common molecular subsequence. J Mol Biol. (1981) 147:195–7. doi: 10.1016/0022–2836(81)90087–5
137. Larkin MA, Blackshields G, Brown NP, Chenna R, McGettigan PA, McWilliam H, et al. Clustal W Clustal X version 2. Bioinformatics (2007) 23:2947–8. doi: 10.1093/bioinformatics/btm404
138. Subramanian AR, Menkhoff JW, Kaufmann M, Morgenstern B. DIALIGN-T: an improved algorithm for segment-based multiple sequence alignment. BMC Bioinformatics (2005) 6:66. doi: 10.1186/1471–2105-6–66.
139. Shi J, Blundell TL, Mizuguchi K. FUGUE: sequence-structure homology recognition using environment-specific substitution tables structure-dependent gap penalties. J Mol Biol. (2001) 310:243–57. doi: 10.1006/jmbi.2001.4762
140. Pei J, Kim BH, Grishin NV. PROMALS3D: a tool for multiple protein sequence structure alignments. Nucleic Acids Res. (2008) 36:2295–300. doi: 10.1093/nar/gkn072
141. Vriend G. WHAT IF: a molecular modeling and drug design program. J Mol Graph. (1990) 8:52–6. doi: 10.1016/0263–7855(90)80070-V
142. Laskowski RA, MacArthur MW, Moss DS, Thornton JM. PROCHECK - a program to check the stereochemical quality of protein structures. J Appl Cryst. (1993) 26:283–91. doi: 10.1107/S0021889892009944
143. Sippl MJ. Recognition of errors in three-dimensional structures of proteins. Proteins (1993) 17:355–62. doi: 10.1002/prot.340170404
144. Bowie JU, Luthy R, Eisenberg D. A method to identify protein sequences that fold into a known three-dimensional structure. Science (1991) 253:164–70. doi: 10.1126/science.1853201
145. Chen VB, Arendall WB, Headd JJ, Keedy DA, Immormino RM, Kapral GJ, et al. MolProbity: all-atom structure validation for macromolecular crystallography. Acta Crystallogr D (2010) 66:12–21. doi: 10.1107/S0907444909042073
146. Forrest LR, Tang CL, Honig B. On the accuracy of homology modeling and sequence alignment methods applied to membrane proteins. Biophys J. (2006). 91:508–17. doi: 10.1529/biophysj.106.082313
147. Schmidt T, Bergner A, Schwede T. Modelling three-dimensional protein structures for applications in drug design. Drug Discov Today (2014). 19:890–7. doi: 10.1016/j.drudis.2013.10.027
148. Evers A, Gohlke H, Klebe G. Ligand-supported homology modelling of protein binding-sites using knowledge-based potentials. J Mol Biol. (2003). 334:327–45. doi: 10.1016/j.jmb.2003.09.032
149. Fan H, Irwin JJ, Webb BM, Klebe G, Shoichet BK, Sali A. Molecular docking screens using comparative models of proteins. J Chem Inf Model. (2009) 49:2512–27. doi: 10.1021/ci9003706
150. Nooren IM, Thornton JM. Diversity of protein-protein interactions. EMBO J. (2003) 22:3486–92. doi: 10.1093/emboj/cdg359
151. Basu S, Bhattacharyya D, Banerjee R. Self-complementarity within proteins: bridging the gap between binding and folding. Biophys J. (2012) 102:2605–14. doi: 10.1016/j.bpj.2012.04.029
152. Dias R, Azevedo WF. Molecular docking algorithms. Curr Drug Targets (2008) 9:1040–7. doi: 10.2174/138945008786949432
153. Venkatachalam CM, Jiang X, Oldfield T, Waldman M. LigandFit: a novel method for the shape-directed rapid docking of ligands to protein active sites. J Mol Graph Model. (2003) 4:289–307. doi: 10.1016/S1093–3263(02)00164-X
154. Rarey M, Kramer B, Lengauer T, Klebe G. A fast-flexible docking method using an incremental construction algorithm. J Mol Biol. (1996) 261:470–489. doi: 10.1006/jmbi.1996.0477
155. Ewing TJ, Makino S, Skillman AG, Kuntz ID. DOCK 4.0: search strategies for automated molecular docking of flexible molecule databases. J Comput Aided Mol Des. (2001) 15:411–28. doi: 10.1023/A:1011115820450
156. Jones G, Willett P, Glen RC, Leach AR, Taylor R. Development and validation of a genetic algorithm for flexible docking. J Mol Biol. (1997) 267:727–48. doi: 10.1006/jmbi.1996.0897
157. Morris GM, Goodsell DS, Halliday RS, Huey R, Hart WE, Belew RK, et al. Automated docking using a lamarckian genetic algorithm and empirical binding free energy function. J Comput Chem. (1998) 19:1639–62.
158. Friesner RA, Banks JL, Murphy RB, Halgren TA, Klicic JJ, Mainz DT, et al. Glide: A new approach for rapid, accurate docking and scoring. 1. Method and assessment of docking accuracy. J Med Chem. (2004) 47:1739–49. doi: 10.1021/jm0306430
159. Chupakhin VI, Palyulin VA, Zefirov NS. Modeling the open and closed forms of GABAA receptor: analysis of ligand-receptor interactions for the GABA-binding site. Dokl Biochem Biophys. (2006) 408:169–74. doi: 10.1134/S1607672906030173
160. Ernst M, Bruckner S, Boresch S, Sieghart W. Comparative models of GABAA receptor extracellular transmembrane domains: important insights in pharmacology function. Mol Pharmacol. (2005) 68:1291–300. doi: 10.1124/mol.105.015982
161. Bergmann R, Kongsbak K, Sørensen PL, Sander T, Balle T. A unified model of the GABAA receptor comprising agonist benzodiazepine binding sites. PLoS ONE (2013) 8:e52323. doi: 10.1371/journal.pone.0052323
162. Richter L, de Graaf C, Sieghart W, Varagic Z, Mörzinger M, de Esch IJ, et al. Diazepam-bound GABAA receptor models identify new benzodiazepine binding-site ligands. Nat Chem Biol. (2012) 8:455–64. doi: 10.1038/nchembio.917
163. Berezhnoy D, Gibbs TT, Farb DH. Docking of 1 4-benzodiazepines in the α1/γ2 GABAA receptor modulator site. Mol Pharmacol. (2009) 76:440–50. doi: 10.1124/mol.109.054650
164. Ci SQ, Ren TR, Ma CX, Su ZG. Modeling of αk/γ2 (k = 1, 2, 3 5) interface of GABAA receptor docking studies with zolpidem: implications for selectivity. J Mol Graph Model. (2007) 26:537–45. doi: 10.1016/j.jmgm.2007.03.007
165. Sancar F, Ericksen SS, Kucken AM, Teissere JA, Czajkowski C. Structural determinants for high-affinity zolpidem binding to GABA-A receptors. Mol Pharmacol. (2007) 71:38–46. doi: 10.1124/mol.106.029595
166. O'Mara M, Cromer B, Parker M, Chung SH. Homology model of the GABAA receptor examined using Brownian dynamics. Biophys J. (2005) 88:3286–99. doi: 10.1529/biophysj.104.051664
167. Cheng J, Ju XL. Homology modeling atomic level binding study of GABA(A) receptor with novel enaminone amides. Eur J Med Chem. (2010) 45:3595–3600. doi: 10.1016/j.ejmech.2010.05.004
168. Law RJ, Lightstone FC. Modeling neuronal nicotinic GABA receptors: important interface salt-links protein dynamics. Biophys J. (2009) 97:1586–94. doi: 10.1016/j.bpj.2009.06.044
169. Cromer BA, Morton CJ, Parker MW. Anxiety over GABAA receptor structure relieved by AChBP. Trends Biochem Sci. (2002) 27:280–7. doi: 10.1016/S0968–0004(02)02092–3
170. Goldschen-Ohm MP, Wagner DA, Jones MV. Three arginines in the GABAA receptor binding pocket have distinct roles in the formation and stability of agonist- versus antagonist-bound complexes. Mol Pharmacol. (2011) 80:647–56. doi: 10.1124/mol.111.072033
171. Wagner DA, Czajkowski C. Structure dynamics of the GABA binding pocket: a narrowing cleft that constricts during activation. J Neurosci. (2001) 21:67–74. doi: 10.1523/JNEUROSCI.21–01-00067.2001
172. Sigel E, Buhr A. The benzodiazepine binding site of GABAA receptors. Trends Pharmacol Sci. (1997) 18:425–9. doi: 10.1016/S0165–6147(97)90675–1
173. Vijayan RS, Bhattacharyya D, Ghoshal N. Deciphering the binding mode of Zolpidem to GABAA α1 receptor – Insights from molecular dynamics simulation. J Mol Model. (2012) 18:1345–54. doi: 10.1007/s00894–011-1142–0
174. Smith TA. Type A gamma-aminobutyric acid (GABAA) receptor subunits and benzodiazepine binding: significance to clinical syndromes and their treatment. Br J Biomed Sci. (2001) 58:111–21.
175. Henderson R, Baldwin JM, Ceska TA, Zemlin F, Beckmann EA, Downing KH. Model for the structure of bacteriorhodopsin based on high-resolution electron cryo-microscopy. J Mol Biol. (1990) 213:899–29. doi: 10.1016/S0022–2836(05)80271–2
176. Trumpp-Kallmeyer S, Hoflack J, Bruinvels A, Hibert M. Modeling of G-protein-coupled receptors: application to dopamine, adrenaline, serotonin, acetylcholine, and mammalian opsin receptors. J Med Chem. (1992) 35:3448–62. doi: 10.1021/jm00097a002
177. Prusis P, Fr berg PA, Muceniece R, Kalvinsh I, Wikberg JE. A three-dimensional model for the interaction of MSH with the melanocortin-1 receptor. Biochem Biophys Res Commun. (1995) 210:205–10. doi: 10.1006/bbrc.1995.1647
178. Niv MY, Skrabanek L, Filizola M, Weinstein H. Modeling activated states of GPCRs: the rhodopsin template. J Comput Aided Mol Des. (2006) 20:437–48. doi: 10.1007/s10822–006-9061–3
179. Nowak M, Kołaczkowski M, Pawłowski M, Bojarski AJ. Homology modeling of the serotonin 5-HT1A receptor using automated docking of bioactive compounds with defined geometry. J Med Chem. (2006) 49:205–214. doi: 10.1021/jm050826h
180. Strahs D, Weinstein H. Comparative modeling and molecular dynamics studies of the delta, kappa mu opioid receptors. Protein Eng. (1997) 10:1019–38. doi: 10.1093/protein/10.9.1019
181. Aburi M, Smith PE. Modeling and simulation of the human δ opioid receptor. Protein Sci. (2004) 13:1997–2008. doi: 10.1110/ps.04720304
182. Pedretti A, Silva ME, Villa L, Vistoli G. Binding site analysis of full-length α 1a adrenergic receptor using homology modelling and molecular docking. Biochem Biophys Res Commun. (2004) 319:493–500. doi: 10.1016/j.bbrc.2004.04.149
183. Van Rhee AM, Fischer B, Van Galen PJ, Jacobson KA. Modelling the P2Y purinoceptor using rhodopsin as template. Drug Des Discov. (1995) 13:133–54.
184. Archer E, Maigret B, Escrieut C, Pradayrol L, Fourmy D. Rhodopsin crystal: new template yielding realistic models of G-protein-coupled receptors? Trends Pharmacol Sci. (2003) 24:36–40. doi: 10.1016/S0165–6147(02)00009–3
185. Warne T, Edwards PC, Leslie AG, Tate CG. Crystal structures of a stabilized β1-adrenoceptor bound to the biased agonists bucindolol and carvedilol. Structure (2012) 20:841–9. doi: 10.1016/j.str.2012.03.014
186. Rasmussen SG, Choi HJ, Rosenbaum DM, Kobilka TS, Thian FS, Edwards PC, et al. Crystal structure of the human beta2 adrenergic G-protein-coupled receptor. Nature (2007) 450:383–7. doi: 10.1038/nature06325
187. Kneissl B, Leonhardt B, Hildebrandt A, Tautermann CS. Revisiting automated G-protein coupled receptor modeling: the benefit of additional template structures for a neurokinin-1 receptor model. J Med Chem. (2009) 52:3166–73. doi: 10.1021/jm8014487
188. Mobarec JC, Sanchez R, Filizola M. Modern homology modeling of G-protein coupled receptors: Which structural template to use? J Med Chem. (2009) 52:5207–16. doi: 10.1021/jm9005252
189. Bera I, Laskar A, Ghoshal N. Exploring the structure of opioid receptors with homology modeling based on single multiple templates subsequent docking: a comparative study. J Mol Model. (2011) 17:1207–21. doi: 10.1007/s00894–010-0803–8
190. Leach AR. Molecular Modeling: Principles and Applications. Upper Saddle River, NJ: Prentice Hall (2001).
192. Salsbury FR. Molecular dynamics simulations of protein dynamics and their relevance to drug discovery. Curr Opin Pharmacol. (2010) 10:738–44. doi: 10.1016/j.coph.2010.09.016
193. Yuan Y, Knaggs MH, Poole LB, Fetrow JS, Salsbury FR Jr. Conformational and oligomeric effects on the cysteine pKa of tryparedoxin peroxidase. J Biomol Struct Dyn. (2010) 28:51–70. doi: 10.1080/07391102.2010.10507343
194. Le L, Lee E, Truong T, Schulten K. Molecular modeling of swine influenza A/H1N1, Spanish H1N1, and avian H5N1 flu N1 neuraminidases bound to Tamiflu and Relenza. PLoS Curr. (2009) 1:RRN1015. doi: 10.1371/currents.RRN1015
195. Vasilyeva A, Clodfelter JE, Gorczynski MJ, Gerardi AR, King SB, Salsbury F, et al. Parameters of reserpine analogs that induce MSH2/MSH6-dependent cytotoxic response. J Nucleic Acids (2010) 2010:162018. doi: 10.4061/2010/162018
196. Law RJ, Forrest LR, Ranatunga KM, La Rocca P, Tieleman D, Sansom MS. Structure and dynamics of the pore-lining helix of the nicotinic receptor: MD simulations in water, lipid bilayers, and transbilayer bundles. Proteins (2000) 39:47–55. doi: 10.1002/(SICI)1097-0134(20000401)39:1<47::AID-PROT5>3.0.CO;2-A
197. Islam SM, Stein RA, McHaourab HS, Roux B. Structural refinement from restrained-ensemble simulations based on EPR/DEER data: application to T4 lysozyme. J Phys Chem B (2013) 117:4740–54. doi: 10.1021/jp311723a
198. Colquhoun D. Binding, gating, affinity efficacy: the interpretation of structure-activity relationships for agonists and of the effects of mutating receptors. Br J Pharmacol. (1998) 125:924–47. doi: 10.1038/sj.bjp.0702164
199. Zhang D, Weinstein H. Signal transduction by a 5-HT2 receptor: a mechanistic hypothesis from molecular dynamics simulations of the three-dimensional model of the receptor complexed to ligands. J Med Chem. (1993) 36:934–8. doi: 10.1021/jm00059a021
200. Huang X, Shen J, Cui M, Shen L, Luo X, Ling K, et al. Molecular dynamics simulations on SDF-1α: binding with CXCR4 receptor. Biophys J. (2003) 84:171–84. doi: 10.1016/S0006–3495(03)74840–1
201. Röhrig UF, Guidoni L, Rothlisberger U. Early steps of the intramolecular signal transduction in rhodopsin explored by molecular dynamics simulations. Biochemistry (2002) 41:10799–809. doi: 10.1021/bi026011h
202. Crozier PS, Stevens MJ, Forrest LR, Woolf TB. Molecular dynamics simulation of dark-adapted rhodopsin in an explicit membrane bilayer: coupling between local retinal larger scale conformational change. J Mol Biol. (2003) 333:493–514. doi: 10.1016/j.jmb.2003.08.045
203. Hallmen C, Wiese M. Molecular dynamics simulation of the human adenosine A3 receptor: agonist induced conformational changes of Trp243. J Comput Aided Mol Des. (2006) 20:673–84. doi: 10.1007/s10822-006-9088-5
204. Kiss R, Viskolcz B, Keseru GM. Activation mechanism of the human histamine H4 receptor-an explicit membrane molecular dynamics simulation study. J Chem Inf. Model. (2008) 48:1199–1210. doi: 10.1021/ci700450w
205. Dror RO, Pan AC, Arlow DH, Borhani DW, Maragakis P, Shan Y, et al. Pathway mechanism of drug binding to G-protein-coupled receptors. Proc Natl Acad Sci USA. (2011) 108:13118–123. doi: 10.1073/pnas.1104614108
206. Miao Y, Nichols SE, Gasper PM, Metzger VT, McCammon JA. Activation dynamic network of the M2 muscarinic receptor. Proc Natl Acad Sci USA. (2013) 110:10982–7. doi: 10.1073/pnas.1309755110
207. Johnston JM, Filizola M. Showcasing modern molecular dynamics simulations of membrane proteins through G protein-coupled receptors. Curr Opin Struct Biol. (2011) 21:552–8. doi: 10.1016/j.sbi.2011.06.008
208. Grossfield A. Recent progress in the study of G protein-coupled receptors with molecular dynamics computer simulations. Biochim Biophys Acta Biomemb. (2011) 1808:1868–78. doi: 10.1016/j.bbamem.2011.03.010
209. Monod J, Wyman J, Changeux JP: On the nature of allosteric transitions: a plausible model. J Mol Biol. (1965) 12:88–118. doi: 10.1016/S0022–2836(65)80285–6
210. Koshland DE Jr, Nemethy G. Filmer D: Comparison of experimental binding data and theoretical models in proteins containing subunits. Biochemistry (1966) 5:365–385. doi: 10.1021/bi00865a047
211. Hilser VJ, Wrabl JO, Motlagh HN. Structural and energetic basis of allostery.Annu Rev Biophys. (2012) 41:585–609. doi: 10.1146/annurev-biophys-050511–102319
212. Nussinov R, Tsai CJ. The different ways through which specificity works in orthosteric and allosteric drugs. Curr Pharm Des. (2012) 18:1311–6. doi: 10.2174/138161212799436377
213. Ma B, Nussinov R. Druggable orthosteric and allosteric hot spots to target protein-protein interactions. Curr Pharm Des. (2014) 20:1293–1301. doi: 10.2174/13816128113199990073
214. Gunasekaran K, Ma B, Nussinov R. Is allostery an intrinsic property of all dynamic proteins? Proteins (2004). 57:433–43. doi: 10.1002/prot.20232
215. Kumar S, Ma B, Tsai CJ, Sinha N, Nussinov R. Folding and binding cascades: Dynamic landscapes and population shifts. Protein Sci. (2000) 9:10–19. doi: 10.1110/ps.9.1.10
216. Kern D, Zuiderweg ER. The role of dynamics in allosteric regulation. Curr Opin Struct Biol. (2003) 13:748–57. doi: 10.1016/j.sbi.2003.10.008
217. Boehr DD, Nussinov R, Wright PE. The role of dynamic conformational ensembles in biomolecular recognition. Nat Chem Biol. (2009) 5: 789–96. doi: 10.1038/nchembio.232
218. Motlagh HN, Wrabl JO, Li J, Hilser VJ. The ensemble nature of allostery. Nature (2014) 508:331–9. doi: 10.1038/nature13001
219. Tsai CJ, del Sol A, Nussinov R. Protein allostery, signal transmission and dynamics: a classification scheme of allosteric mechanisms. Mol Biosyst. (2009) 5:207–16. doi: 10.1039/b819720b
220. Wagner JR, Lee CT, Durrant JD, Malmstrom RD, Feher VA, Amaro RE. Emerging computational methods for the rational discovery of allosteric drugs. Chem Rev. (2016) 116:6370–90. doi: 10.1021/acs.chemrev.5b00631
221. Greener JG, Sternberg MJ. Structure-based prediction of protein allostery. Curr Opin Struct Biol. (2017) 50:1–8. doi: 10.1016/j.sbi.2017.10.002
222. Lotz SD, Dickson A. Unbiased molecular dynamics of 11 min timescale drug unbinding reveals transition state stabilizing interactions. J Am Chem Soc. (2018) 140:618–28. doi: 10.1021/jacs.7b08572
223. Lu S, Ji M, Ni D, Zhang J. Discovery of hidden allosteric sites as novel targets for allosteric drug design. Drug Discov Today (2018). 23:359–65. doi: 10.1016/j.drudis.2017.10.001
224. Hamelberg D., Mongan J., McCammon JA. Accelerated molecular dynamics: a promising and efficient simulation method for biomolecules. J Chem Phys. (2004) 120:11919–29. doi: 10.1063/1.1755656
225. Bucher D, Grant BJ, Markwick PR, McCammon JA. Accessing a hidden conformation of the maltose binding protein using accelerated molecular dynamics. PLoS Comput Biol. (2011) 7:e1002034. doi: 10.1371/journal.pcbi.1002034
226. Hastings WK. Monte Carlo sampling methods using Markov chains and their applications. Biometrika (1970) 57:97–109. doi: 10.1093/biomet/57.1.97
227. Pande VS, Beauchamp K, Bowman GR. Everything you wanted to know about Markov State Models but were afraid to ask. Methods (2010) 52:99–105. doi: 10.1016/j.ymeth.2010.06.002
228. Prinz JH, Wu H, Sarich M, Keller B, Senne M, Held M, et al. Markov models of molecular kinetics: generation and validation. J Chem Phys. (2011) 134:174105. doi: 10.1063/1.3565032
229. Chodera JD, Noe' F. Markov state models of biomolecular conformational dynamics. Curr Opin Struct Biol. (2014) 25:135–44. doi: 10.1016/j.sbi.2014.04.002
230. Kohlhoff KJ, Shukla D, Lawrenz M, Bowman GR, Konerding DE, Belov D, et al. Cloud-based simulations on Google Exacycle reveal ligand modulation of GPCR activation pathways. Nat Chem. (2013) 6:15–21. doi: 10.1038/nchem.1821
231. Bahar I, Rader A. Coarse-grained normal mode analysis in structural biology. Curr Opin Struct Biol. (2005) 15:586–92. doi: 10.1016/j.sbi.2005.08.007
232. Brooks B, Karplus M. Harmonic dynamics of proteins: normal modes and fluctuations in bovine pancreatic trypsin inhibitor. Proc Natl Acad Sci USA. (1983) 80:6571–5. doi: 10.1073/pnas.80.21.6571
233. Tirion MM. Large amplitude elastic motions in proteins from a single-parameter, atomic analysis. Phys Rev Lett. (1996) 77:1905–8. doi: 10.1103/PhysRevLett.77.1905
234. Yu X, Kokh DB, Nandekar P, Mustafa G, Richter S, Wade RC. Dynathor: dynamics of the complex of cytochrome p450 and cytochrome P450 reductase in a phospholipid bilayer. In: Nagel W., Kröner D., Resch M, editors. High Performance Computing in Science and Engineering. Cham: Springer (2016). p. 255–64.
235. Mustafa G, Nandekar PP, Yu X, Wade RC. On the application of the MARTINI coarse-grained model to immersion of a protein in a phospholipid bilayer. J Chem Phys. (2015) 143:243139. doi: 10.1063/1.4936909
236. Bond PJ, Holyoake J, Ivetac A, Khalid S, Sansom MS. Coarse-grained molecular dynamics simulations of membrane proteins and peptides. J Struct Biol. (2007) 157:593–605. doi: 10.1016/j.jsb.2006.10.004
237. Scott KA, Bond PJ, Ivetac A, Chetwynd AP, Khalid S, Sansom MS. Coarse-grained MD simulations of membrane protein-bilayer self-assembly. Structure (2008) 16:621–30. doi: 10.1016/j.str.2008.01.014
238. Tieleman DP, Biggin PC, Smith GR, Sansom MS. Simulation approaches to ion channel structure–function relationships. Q Rev Biophys. (2001) 34:473–561. doi: 10.1017/S0033583501003729
239. Tembe BL, Mccammon JA. Ligand receptor interactions. Comput Chem. (1984) 8:281–3. doi: 10.1016/0097–8485(84)85020–2
240. Durrant JD, McCammon JA. Molecular dynamics simulations and drug discovery. BMC Biol. (2011) 9:71. doi: 10.1186/1741–7007-9–71
241. Michel J, Essex JW. Hit identification and binding mode predictions by rigorous free energy simulations. J Med Chem. (2008) 51:6654–64. doi: 10.1021/jm800524s
242. McCammon JA. Computer-aided drug discovery: physics-based simulations from the molecular to the cellular level. In Zewail AH editor. Physical Biology: From Atoms to Medicine. London: Imperial College Press (2008). p. 401–10.
243. Wermuth CG, Ganellin CR, Lindberg P, Mitscher LA. Glossary of terms used in medicinal chemistry (IUPAC Recommendations 1998). Pure Appl Chem. (1998) 70:1129–43. doi: 10.1351/pac199870051129
244. Langer T, Wolber G. Pharmacophore definition and 3D searches. Drug Discov Today (2004) 1:203–7. doi: 10.1016/j.ddtec.2004.11.015
245. Reddy AS, Pati SP, Kumar PP, Pradeep HN, Sastry GN. Virtual screening in drug discovery – a computational perspective. Curr Protein Pept Sci. (2007) 8:329–51. doi: 10.2174/138920307781369427
246. Mitra S, Banerjee TS, Hota SK, Bhattacharya D, Das S, Chattopadhyay P. Synthesis and biological evaluation of dibenz[b,f][1,5]oxazocine derivatives for agonist activity at κ-opioid receptor. Eur J Med Chem. (2011) 46:1713–20. doi: 10.1016/j.ejmech.2011.02.024
247. Bera I, Ghoshal N. Positively charged nitrogen is not indispensable requirement for binding of nitrogenous κ-opioid agonists: insights from docking studies. Lett Drug Des Discov. (2014) 11:809–13. doi: 10.2174/1570180811666140220004853
248. Bera I, Marathe MV, Payghan PV, Ghoshal N. Identification of novel hits as highly prospective dual agonists for mu and kappa opioid receptors: an integrated in silico approach. J Biomol Struct Dyn. (2018) 36:279–301. doi: 10.1080/07391102.2016.1275810
249. Goddard WA, Abrol R. 3-Dimensional structures of G protein-coupled receptors and binding sites of agonists and antagonists. J Nutr. (2007) 137:1528S−38S. doi: 10.1093/jn/137.6.1528S
250. Floriano WB, Vaidehi N, Singer M, Shepherd G, Goddard WA III. Molecular mechanisms underlying differential odor responses of a mouse olfactory receptor. Proc Natl Acad Sci USA. (2000) 97:10712–6. doi: 10.1073/pnas.97.20.10712
251. Cho A, Wendel JA, Vaidehi N, Kekenes-Huskey PM, Floriano WB, Maiti PK, et al. The MPSim-Dock Hierarchical Docking Algorithm: application to the eight trypsin inhibitor co-crystals. J Comput Chem. (2005) 26:48–71. doi: 10.1002/jcc.20118
252. Evers A, Klabunde T. Structure-based drug discovery using GPCR homology modeling: successful virtual screening for antagonists of the alpha1A adrenergic receptor. J Med Chem. (2005) 48:1088–97. doi: 10.1021/jm0491804
253. Chen JZ, Wang J, Xie XQ. GPCR structure-based virtual screening approach for CB2 antagonist search J Chem Inf Model. (2007) 47:1626–37. doi: 10.1021/ci7000814
254. De Graaf C, Rognan D. Selective structure-based virtual screening for full partial agonists of the β2 adrenergic receptor. J Med Chem. (2008) 51:4978–85. doi: 10.1021/jm800710x
255. Varady J, Wu X, Fang X, Min J, Hu Z, Levant B, Wang S. Molecular modeling of the three-dimensional structure of dopamine 3 (D3) subtype receptor: discovery of novel potent D3 ligands through a hybrid pharmacophore- structure-based database searching approach. J Med Chem. (2003) 46:4377–92. doi: 10.1021/jm030085p
256. Negri A, Rives ML, Caspers MJ, Prisinzano TE, Javitch JA, Filizola M. Discovery of a novel selective kappa-opioid receptor agonist using crystal structure-based virtual screening. J Chem Inf Model. (2013) 53:521–6. doi: 10.1021/ci400019t
257. Bisignano P, Burford NT, Shang Y, Marlow B, Livingston KE, et al. Ligand-based discovery of a new scaffold for allosteric modulation of the μ-opioid receptor. J Chem Inf Model. (2015) 55:1836–43. doi: 10.1021/acs.jcim.5b00388
258. Basith S, Cui M, Macalino SJ, Park J, Clavio NA, Kang S, Choi S. Exploring G protein-coupled receptors (GPCRs) ligand space via cheminformatics approaches: impact on rational drug design. Front Pharmacol. (2018) 9:128. doi: 10.3389/fphar.2018.00128
259. Weiss DR, Ahn S, Sassano MF, Kleist A, Zhu X, Strachan R., et al. Conformation guides molecular efficacy in docking screens of activated beta-2 adrenergic G protein coupled receptor. ACS Chem Biol. (2013) 8:1018–1026. doi: 10.1021/cb400103f
260. Kooistra AJ, Vischer HF, McNaught-Flores D, Leurs R, de Esch IJP, de Graaf C. Function-specific virtual screening for GPCR ligands using a combined scoring method. Sci Rep. (2016) 6:282–88. doi: 10.1038/srep28288
261. Fourches D, Muratov E, Tropsha A. Trust, but verify: on the importance of chemical structure curation in cheminformatics and QSAR modeling research. J Chem Inf Model. (2010) 50:1189–1204. doi: 10.1021/ci100176x
262. Fourches D, Muratov E, Tropsha A. Trust, but verify II: a practical guide to chemogenomics data curation. J Chem Inf Model. (2016) 56:1243–1252. doi: 10.1021/acs.jcim.6b00129
263. Olah M, Mracec M, Ostopovici L, Rad R, Bora A, Hadaruga N, et al. Chapter 9: WOMBAT: world of molecular bioactivity. In Oprea TI, editor. Chemoinformatics in Drug Discovery. Weinheim, DE: WILEY-VCH Verlag GmbH & Co. (2005). p. 221–39.
Keywords: pLGIC, GPCRs, GABA A receptor, structure based drug design, MD simulations, CNS drug discovery, molecular docking, virtual screening
Citation: Payghan PV, Bera I, Bhattacharyya D and Ghoshal N (2018) Computational Studies for Structure-Based Drug Designing Against Transmembrane Receptors: pLGICs and Class A GPCRs. Front. Phys. 6:52. doi: 10.3389/fphy.2018.00052
Received: 05 January 2018; Accepted: 14 May 2018;
Published: 25 September 2018.
Edited by:
Francisco J. Barrantes, Instituto de Investigaciones Biomédicas (BIOMED UCA-CONICET), ArgentinaReviewed by:
Jesus Perez-Gil, Complutense University of Madrid, SpainKarim Nagi, Qatar University, Qatar
Copyright © 2018 Payghan, Bera, Bhattacharyya and Ghoshal. This is an open-access article distributed under the terms of the Creative Commons Attribution License (CC BY). The use, distribution or reproduction in other forums is permitted, provided the original author(s) and the copyright owner(s) are credited and that the original publication in this journal is cited, in accordance with accepted academic practice. No use, distribution or reproduction is permitted which does not comply with these terms.
*Correspondence: Dhananjay Bhattacharyya, dhananjay.bhattacharyya@saha.ac.in
Nanda Ghoshal, nghoshal@iicb.res.in
†These authors have contributed equally to this work
‡Present Address: Indrani Bera, Department of Chemical and Biomolecular Engineering, University of Maryland, College Park, MD, United States