- 1Department de Comunicació, Universitat Pompeu Fabra, Barcelona, Spain
- 2Eurecat—Centre Tecnològic de Catalunya, Barcelona, Spain
The online encyclopedia Wikipedia is the largest general information repository created through collaborative efforts from all over the globe. Despite the project's goal being to achieve the sum of human knowledge, there are strong content imbalances across the language editions. In order to quantify and investigate these imbalances, we study the impact of cultural context in 40 language editions. To this purpose, we developed a computational method to identify articles that can be related to the editors' cultural context associated to each Wikipedia language edition. We employed a combination of strategies taking into account geolocated articles, specific keywords and categories, as well as links between articles. We verified the method's quality with manual assessment and found an average precision of 0.92 and an average recall of 0.95. The results show that about a quarter of each Wikipedia language edition is dedicated to represent the corresponding cultural context. Although a considerable part of this content was created during the first years of the project, its creation is sustained over time. An analysis of cross-language coverage of this content shows that most of it is unique in its original language, and reveals special links between cultural contexts; at the same time, it highlights gaps where the encyclopedia could extend its content. The approach and findings presented in this study can help to foster participation and inter-cultural enrichment of Wikipedias. The datasets produced are made available for further research.
Introduction
Wikipedia's most striking characteristic is the fact that it is a collaborative project: everybody can become a volunteer contributor and join the community. At present, there are 288 Wikipedia language editions, English being the largest with more than 5 million articles (and a total of 40 million articles counting all the languages). Wikipedia's goal is to provide the “sum of human knowledge,” available to everyone for free, and at the moment it is already one of the most successful collaborative efforts in the Internet. Even though there is no central authority dictating the content to be created, the system is based on the following content rules. Probably the most important rule is the “Neutral Point of View” (NPOV), which roughly means “representing fairly, proportionately, and, as far as possible, without editorial bias, all of the significant views that have been published by reliable sources on a topic”1 “Notability”2, another core content rule, defines the criteria through which editors judge whether a specific topic deserves an article.
Although the above-mentioned norms exist in all Wikipedia language editions, their application and interpretation are constantly negotiated by the editors from each community. The fact that policies neither encourage or discourage languages' cultural differences and idiosyncrasies being reflected into content, results in a spontaneous creation of content. Moreover, each Wikipedia language edition is created in a decentralized way; as a result, editors themselves may not always be aware of the global product. In fact, each language edition has proven to be diverse in terms of both article content and absolute number of articles, up to the point that diversity has been often called “Systemic bias,” which is referred to as “an imbalanced coverage of subjects and perspectives on the encyclopedia.” This imbalance is often attributed to the lack of editors or resources in a particular language background. Among the reasons that explain why some languages do not have a Wikipedia language edition or have it underdeveloped, Van Dijk [1] and Ensslin [2] mention, among others, the reduced number of speakers, the digital divide, and the low online reputation of their language.
Cultural Contextualization
In general, differences in the content of language editions are attributed by the current literature to contextual factors or to a process named by Hecht ([3], p. 47) as cultural contextualization, which “is the cause of some of the content diversity in multilingual Wikipedia.” Cultural contextualization is also present in other user-generated projects such as OpenStreet Maps, Twitter or Flickr [3]. The explanation of how it influences the final characteristics of content is rooted in the fields of Linguistics, and Cultural and Social Psychology. For instance, according to Clark [4], the members of a cultural community usually share “facts, beliefs, procedures, norms, and assumptions.” Hence, it is likely that the editors of each language community (and subcommunities, especially considering those languages with large geographical extension) may reflect in their articles the meanings they implicitly agree on, resulting in a great deal of diversity in such a worldwide project. Cultural contextualization occurs when there is a certain degree of freedom in content-based projects.
In Wikipedia, there is extensive literature on how cultural contextualization has shaped each language edition. Depending on whether the emphasis is put on the articles' text or on the Wikipedia's overall structure, effects can be classified into two main groups: Discourse and Structure.
Discourse effects are based on the idea that since each language edition constitutes a community (and perhaps few subcommunities), their editors tend to hold a shared cultural background and this ultimately limits the points of view adopted in the articles within one and the same language edition. (In the literature, the editor's point of view is referred to as: “linguistic point of view,” “national point of view,” or “cultural bias”). In different language editions, the differences in the editors' point of view become more prominent, especially when it comes to controversial topics, where history and politics are seen from opposite positions [5, 6]. For instance, Rogers and Sendijarevic [7] compared an article dedicated to “The Srebrenica Massacre” throughout different Wikipedia language editions, including English and Balkan languages. The study shows how the same article in different language editions adopts a different point of view to illustrate facts; such points of view are sometimes unified, other times in total disagreement when it comes to the terminology employed and its political connotations.
Likewise, in order to explore how contextualized Wikipedia language editions are, Bao et al. [8] developed a website which allows to explore similarities and differences in points of view of an article whose concept exists across languages. Pentzold et al. [9] showed that topics related to cultural heritage such as “Bullfighting” are framed differently in Catalan, Spanish, and English language editions, and have different focuses of controversies. Other studies point out that editors' geographical closeness to the subject of their articles impacts on the level of article exhaustiveness. Callahan and Herring [10] explored in the English and Polish Wikipedia how the biographical articles of well-known people are more complete (in terms of features such as the number of pictures, education, political ideology, controversies mentioned, or family members names) in the language editions associated to the territories where the person is from.
Structural effects are based on the idea that context and culture are relevant factors that affect editor interests and consequently content coverage. Ronen et al. [11] explored the relationships between Wikipedia language editions by creating a network with all languages (global language network) articles' edits and assessed their centrality with eigenvector centrality. They found that English acts as an influential central hub, followed by other well-spread languages such as French, Spanish, German, among others. However, besides attributing it to visibility, they do not explain the factors which influence each other. In this sense, Saimolenko et al. [12], in order to explore to understand cultural similarity understood as the significant interest of communities in contributing to articles about similar topics, analyzed both edits in articles existing in various language editions and several cultural factors. They found that cultural similarity is due to various factors affecting topic choices such as shared language family, number of bilinguals, geographical proximity, among others.
In another study on common editing interests, Karimi et al. [13] gathered all the editors' edits from English Wikipedia and analyzed their relationships in order to determine how close their affinities were. Results showed that editors from close locations tend to have a higher coincidence in the articles they edit than editors from distant geographical locations. The geographical factor was also used to explain that Wikipedia language editions whose language-related territories are far from each other tend to have less articles in common (i.e., their articles have no equivalence) than those whose territories that are geographically close [14].
Other studies show that editors tend to focus on their territories, either because geolocated articles are edited by nearby editors or because they give them a higher visibility in the overall Wikipedia network of articles. For instance, Hecht B.J. and Gergle [15] computed the location of each anonymous edit in geolocated articles and discovered that many of the contributions were made from close distances. Another effect detected by Hecht and Gergle [16], called “Self-focus bias,” explains that the articles located in the countries local to each language edition are linked to many more articles (i.e., they have more inlinks) than the articles located in the other countries.
All in all, this second group of effects shows that context has a key impact on Wikipedia content coverage and shows the relevance of geographical context to editors' activity.
However, in this research stream, one key perspective is missing. We argue that in order to estimate the impact of cultural context on content coverage, it would be necessary to know which articles relate to the cultural context of each language edition besides geolocated articles, including topics such as language, people, traditions, among others. This association would permit a more elucidated cartography on content coverage which would allow, first, to show whether the cultural context occupies a considerable part of each Wikipedia language edition, and second, to verify whether cultural context is at the base of the imbalances between Wikipedia language editions.
To do so, we advance the following three research questions about cultural context content:
• RQ1. What is the extent of cultural context content in each Wikipedia language edition?
• RQ2. How have cultural context content articles been created over time?
• RQ3. What is their availability across different language editions?
Therefore, in this work we aim to go one step further in the study of cultural contextualization, focusing on its structural effects and content coverage. We propose obtaining, for every Wikipedia language edition, a group of articles related to the editors' cultural context(s). In this way we are able to understand the relationship between the content imbalances and the representation of editors' cultural context in every Wikipedia language edition. We called culture gap the imbalances across language editions in content representing cultural context.
To the best of our knowledge, this is the first study that performs a perimetric analysis of the cultural context content. In particular, a valuable corpus is obtained to examine Wikipedia's cultural contextualization effects on content coverage more in depth than it has been done in previous studies. Moreover, the corpus also represents a valuable tool to understand the editors' culture and may be useful to both researchers and Wikipedia editors who want to increase cultural diversity.
In summary, our main contributions are the following:
• We provide a computational method to identify articles related to the cultural context of a given language community.
• We construct a dataset for 40 Wikipedia language editions comprising the articles representing their cultural contexts and make it publicly available for future research.
• We analyze the availability of the articles representing the cultural context of each language community across Wikipedia editions.
In this work we extend our previous study [17] adding a rigorous manual assessment of the accuracy of the method, an analysis of the creation of cultural context content over time, and a deeper analysis of cross-language coverage.
Methods
Dataset Construction: Cultural Context Content (CCC)
In this section, we describe the method employed to map Wikipedia articles to the cultural context(s) in every language edition with the aim of constructing a dataset. First, we report the selection of the list of languages to be included in the study. Second, we explain the criteria by which we include an article into the dataset. Third and finally, we propose a mechanism to manually assess the performance of the method.
List of Languages
For the study of cultural context, we consider that having a rich and diverse list of languages increases its value. The selection of languages includes the 30 largest Wikipedia language editions in terms of number of articles (as of July 20153 Arabic, Catalan, Cebuano, Chinese, Czech, Danish, Dutch, English, Finnish, French, German, Hungarian, Indonesian, Italian, Japanese, Korean, Malay, Norwegian, Persian, Polish, Portuguese, Romanian, Russian, Serbian, Spanish, Swedish, Turkish, Ukrainian, Vietnamese, and Waray. To cover diversity, we take into account different sociolinguistic factors and we decided to add 10 language editions to the initial list of 30; at least one language edition per continent, having various linguistic roots, various speaking community sizes, and various editing community sizes. The 10 added languages are: Afrikaans, Basque, Estonian, Guarani, Greek, Hebrew, Icelandic, Macedonian, Nepali, and Swahili. For the analysis of CCC article creation over time, we select a reduced subset of 15 language editions.
Cultural Context Content
Once languages are selected, it is necessary to map the content of each Wikipedia language edition to their cultural context concepts. The aim is to elaborate a method to collect a comprehensive set of Cultural Context Content articles (from now on referred to as CCC) for every Wikipedia language edition. The CCC encompasses a wide variety of topics to represent the shared concepts linked to the corresponding territories. We formalize that CCC articles deal with concepts that have been: (a) originated in the context, or (b) located in that context and have had a considerable influence there. In addition, in some contexts where two languages are spoken, their speakers may even share some of its concepts (they refer to the same objects or places), and at the same time, geographically widespread languages may be spoken in geographically distant contexts because of historical reasons. With this method, we created individual CCC datasets for every language, including all the cultural contexts of their speakers. This implies that languages that are official in several countries will conform a single dataset encompassing the diverse concepts of these contexts.
Language-Territory Mapping
After having taken all this into consideration, before being able to elaborate the method, we still need a first ground-truth with some reliable and central concepts for each language related cultural contexts. In this sense, we identify for each language: the language name, geographical entities (top political territories such as country and region names) where it is spoken, and its demonyms. To do so, it is necessary to use the ISO 639-2 and 639-3 codes already employed by Wikimedia Foundation to classify Wikipedia language editions (e.g., “ru” for the Russian language Wikipedia: ru.wikipedia.org), as well as the ISO 3166 and 3166-2 codes to identify each country and its subdivisions at a regional level. These codes are widely used on the Internet in geolocation services. In this way it is possible to pair each of the selected language editions with its native words to specify the territories where it is official or indigenous, their inhabitants' demonyms and the language names (e.g., eswiki españa mexico … español castellano) (see Wikipedia Cultural Diversity Observatory for the complete list4 This word list is generated by automatically crossing language ISO codes and the Ethnologue5 databases, which contain the territories where a language is spoken and their names in their corresponding language. This is especially relevant for those languages which are only spoken or official in a specific region of a country. The generated list is subsequently manually revised and extended (using information from the specific articles in the correspondent Wikipedia language edition) with second names for the same language and demonyms, which are introduced primarily in singular masculine, feminine, and plural when available, and with information from the Wikidata database property “demonym”6
Article Selection and Retrieval
Once the language-territory mapping keywords list is obtained, a computational implementation of the method is developed applying and integrating the three strategies described below. The method uses the databases of each Wikipedia language edition, which are updated in real time (we were granted access to them by the Wikimedia Foundation7 The first two strategies gather the articles considered totally reliable, while the third collects some undesired ones that need to be automatically filtered at a later stage.
The first strategy (i—geocoordinates) consists in examining the article location tags {{#coordinates}} and the information located in the geotags table, such as the geocoordinates and the ISO code, in order to obtain articles clearly located within the specified territories for each language edition. Articles satisfying this first criterion are directly retrieved from the databases of each Wikipedia language edition. Nonetheless, the implementation of geocoordinates is unequal throughout the different language editions and may contain errors. Therefore, articles with coordinates are verified using a reverse geocoder tool in Python8 Such tool returns an ISO code that needs to be verified in the ISO codes database to see whether the article is located in a territory associated to the language or not. As a last step, it is possible to add articles that are not tagged with coordinates and do not have a territory ISO code, but that can be matched to the corresponding articles in other language editions, where they are properly geolocated (e.g., an article about a city in Nepal which is not geolocated in the Nepali Wikipedia, but it is in the English Wikipedia).
The second strategy (ii—keywords) implies examining the articles that contain in their title keywords related to the language or to the corresponding territories (e.g., “England National football team,” “English law,” etc.). These two criteria ensure a high reliability, but unfortunately, they cannot guarantee that all the articles which should belong to CCC are actually included.
The third strategy (iii—categories) aims at retrieving the articles more generally related to the identified keywords. Wikipedia articles are classified into categories that are named according to the topics developed in the articles. These categories are organized in a hierarchical tree structure. As the category hierarchy is manually curated and maintained by each community, it tends to be very rich, although it may contain some noise. The hierarchical structure can be leveraged to identify subareas of the encyclopedia related to a particular topic: starting from some category, it is possible to crawl down the classification structure, and gather all the articles belonging to the category and recursively to its subcategories. In a similar way to the second strategy, we start from the list of keywords associated to a language and the corresponding territories, and retrieve all the categories that include such keywords in their titles; for example: “Performing Arts in England” or “Disputes in English Grammar” in the English Wikipedia. These categories contain articles and other categories which contain in turn more specific articles (see Figure 1), until at a certain level the process of crawling and gathering articles finishes. The precise point where the process ends depends on how the category structures have been constructed (smaller Wikipedia language editions in number of articles also tend to have a less developed category graph).
The main advantage of this strategy is that it allows to obtain articles related to some keywords. However, the distance to the top also matters: while the category “Films directed by Charlie Chaplin,” is part of the category “Performing Arts in England,” its content will be considerably more specific. The further from the top category containing the keyword, the more specific and less related to the original top category topic the articles will be. The drawback of this category crawling is that sometimes the categorization includes circular references or does not follow a specialization path (e.g., occasionally a more general category appears under a more specific one, other times a category appears to be related to the immediately preceding one, but totally unrelated to the preceding ones). Such phenomena may produce interferences in the collection (e.g., the category “Wars involving the United States” includes the category “World War II,” which in turn leads to articles about the German army and makes them appear as related to the English Wikipedia cultural contexts). Because of this interference issue, when we use this method on the English Wikipedia we set a limit of five levels of iteration, i.e., when moving down toward more and more specific categories, we stop at the fifth level. As the category trees are simpler and less entangled in the other Wikipedias, in the rest of language editions we complete the iterations until the down category graph goes extinct.
Filtering
Considering that most, but not all of the articles collected using this third strategy can be considered CCC, we tackle possible interferences with a filter. In order to be effective, the filter has to discriminate whether the article is related to the editors' cultural contexts; as a proxy for assessing this thematic coherence, we look at the extent to which the links contained in the text of an article point to other CCC articles. As a starting point, we rely on the articles identified with the first two strategies: the geolocated articles and those including the keywords in their title, which we take as an initial reliable set of CCC articles. We then iteratively add to this set the articles from the bulk category crawling selection that have at least 15% of their links pointing out to these articles. While the algorithm usually converges and stops adding more articles after the third iteration, in large Wikipedia language editions such as the English it is necessary to limit the algorithm before too many articles, including false positives, start to be included; we decided to stop the algorithm after the fifth iteration. Using this procedure, we obtain a final CCC slightly smaller than we would obtain taking all the articles from the category crawling selection, and we are able to avoid most of the false positives.
Method Assessment
In order to validate the method, there has to be agreement over the nature of CCC articles (i.e., it is valid) and whether this is a stable construct that the method can identify in a consistent way (i.e., it is reliable). To examine the method's validity and reliability, we select German and Japanese, and propose an inter-rater reliability test between 3 raters and the algorithm, calculating the Cohen's Kappa coefficient [18]. In this way we can assess the agreement between human raters and test the accuracy of the automatic method as compared to expert human judgement.
We randomly selected 100 articles classified as CCC, and 100 non CCC articles from the German language edition. The same process was applied to the Japanese edition. We relied on Google translator to translate the text of each article into English, for the raters to understand the article content. Subsequently, the three raters manually classified the articles as positive or negative, i.e., as belonging or not to CCC.
The results of the inter-rater assessment are shown in Table 1, which reports, for the two language editions, the agreement between the algorithm and the raters, as well as the interrater agreement. Overall, the degree of agreement between human raters is beyond the 95% in all cases, and with a Kappa coefficient over 0.9, which confirms that there is agreement over what makes an article belong to the CCC category. The agreement between the human raters and the algorithm is slightly lower than between humans, but still satisfactory (nearly 90% agreement and a Kappa coefficient of 0.76 in average), confirming the reliability of the automatic method.
Non-CCC articles given as positive by the algorithm are mostly articles about specific topics from adjacent countries, or articles related through incidental relationships, for instance a basketball player who competed for one of the countries associated to the language. The cases of disagreement between raters concerned articles partially related to a particular territory or language, which lend themselves to different interpretations. For instance, there was disagreement about the article “Bronvaux,” a French municipality in the region of Lorraine, close to the German border and historically disputed between the two countries. The article in the German Wikipedia is categorized as “Historical Territory (Germany),” and this is why also the algorithm considered it as part of CCC for the German Wikipedia. One rater however considered that being located in France, the article should be part of CCC only for the French Wikipedia. In another case, there was disagreement on the article “ECN-T002” which refers to a mobile phone model released in 2009 by Toshiba, a Japanese company. While two raters considered this kind of creation to be part of the cultural context, the other one argued that technological products on the global market should not be associated with a cultural context. The algorithm did not assign the article to CCC. As the borders of cultural contexts are fuzzy, this kind of disagreements may be inevitable. Instead of confronting imported and original concepts, we argue that the selection of CCC articles should be seen as a continuum going from central to peripheral relevant concepts.
At this point, in order to evaluate the overall accuracy of the method we repeated the manual assessment procedure with one human rater for the rest of the Wikipedia language editions and computed the F1 Score. The results are presented in Table 2, which details the percentage of false positives (FP) and false negatives (FN) with the resulting F1 score for each language edition. We observe that false positives are on average the 8.1%, and false negatives the 5.9%. The average value of F1 is 0.92. The selections with more false positives are Korean and Serbian (19 and 23%, respectively). The results for these two language editions appear to be affected by categorization issues. In the former case, the Korean Wikipedia has a category tree were subcategory relationships do not always reflect a strict hierarchical structure, so that the algorithm gets to include unrelated concepts; at the same time, the 15% threshold on the outlinks is not always sufficient to filter out noise in this case due to the high presence of very short articles that only include very few links. In the latter case, the Serbian Wikipedia still employs “Yugoslavia” as a label for its categories and tends to encompass also non-Serbian territories, therefore the false positives detected through the method assessment actually reflect an inconsistency in the data due to a geopolitical conflict.
Results
RQ1. Extent of the Cultural Context Content Articles
Before answering the first research question, it is worth introducing four prototypical articles from English Wikipedia that represent the various types of content selected: (1) CCC keywords, (2) CCC geolocated, (3) the rest of CCC as part of the constructed datasets, (4) the rest of Wikipedia (Figure 2). For instance, for the English language edition, a good example of CCC Keyword is “English Literature,” because it perfectly explains the content of the article. The articles from the category CCC keywords are often a synthesis of a topic aggregated by the demonym or the territory name (e.g., English writers' biographies and works). An example of the CCC geolocated articles, the “Times Square” article, contains the name of a geographical territory associated to the English Wikipedia. Even though this is a very iconic place, in CCC geolocated there are articles with all levels of notability—from small towns to nationally renowned companies and famous monuments. A good example of the rest of CCC articles is the “Banbury Cake” article. After the CCC geolocated and CCC keywords articles, the rest of CCC articles dedicated to specific themes of local scope represent the majority in CCC. An example of an article from the rest of Wikipedia could be for instance the article “Sun,” an article containing universal knowledge not related to any cultural context in particular.
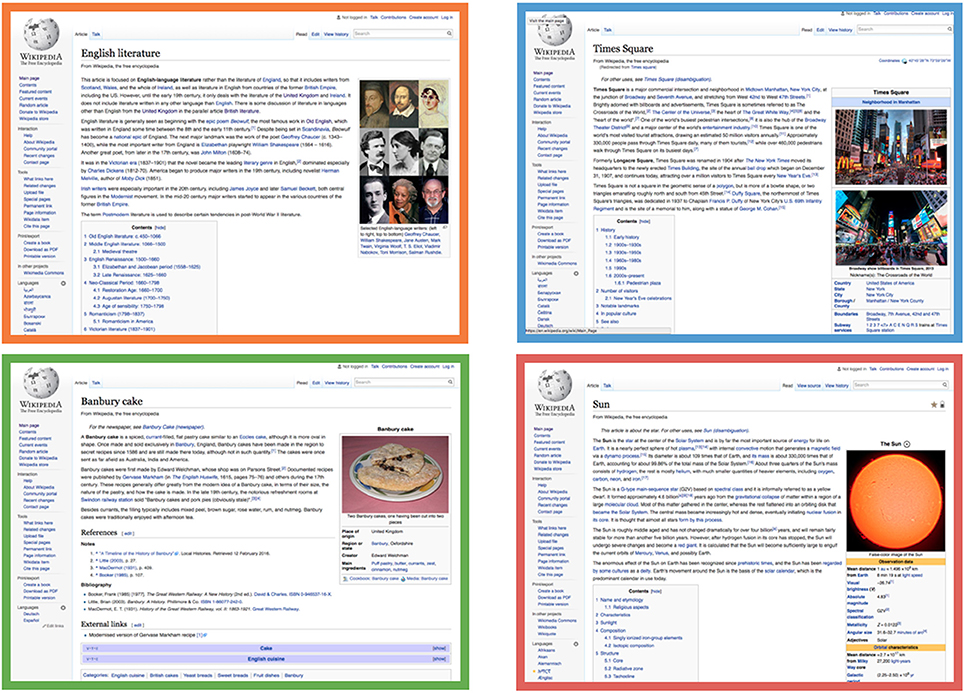
Figure 2. Examples of articles from English Wikipedia. CCC keywords (English Literature), CCC geolocated (Times Square), the rest of CCC (Banbury cake), and the rest of Wikipedia (Sun).
Results
The Venn diagram shown in Figure 3 presents the average proportion of CCC articles in the 40 considered language editions, and the breakdown into articles identified via the first (geolocation) and second (keywords in the title) strategies. We observe that about 1 out of 5 CCC articles were identified via geo-coordinates, and only about one out of 20 via keywords in the title. The intersection between the two subgroups is rather small. The proportion of articles identified through the third strategy (category structure) are omitted, as they represent almost the totality of CCC (29.5% on average).
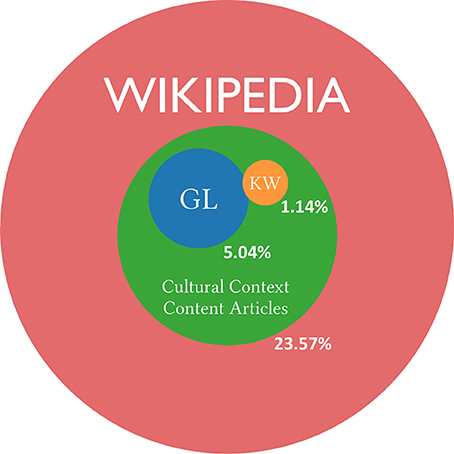
Figure 3. Average proportion of CCC, and of CCC detected through geolocation and keywords. Sizes are in scale according to their proportion.
As shown in Table 2, almost a quarter of each Wikipedia language edition (mean 23.2%, median 24.2%, standard deviation 11.1%) belongs to CCC articles (RQ1). These results indicate that a non-negligible percentage of each Wikipedia is dedicated to concepts representing the cultural contexts associated with it. Table 2 shows the total number of articles and the percentage of articles classified as CCC for each of the 40 language editions considered. Furthermore, the table shows the breakdown according to the different strategies through which CCC articles have been identified, i.e., through Strategy 1 (through geolocation tags) or Strategy 2 (keywords in the title). As above, the percentage for Strategy 3 (category crawling) is not reported, as for most language editions it is very near or almost equal to the final percentage of articles included in the CCC set.
The comparison of CCC percentages across languages shows that there is no obvious pattern. While for its role as an international reference one could expect the English Wikipedia to have a lower proportion of articles associated with its specific cultural context, the results actually show that it has the second highest percentage of CCC articles (46.8%), exceeded only by the Japanese version (49.2%), while the proportion is below 40% for the rest of language editions.
The extremely low percentage (0.1%) for Cebuano and Waray–Waray reflects that these language editions have a high number of articles but are mostly made by bots that automatically translate articles from other languages versions. This observation points out that the creation of CCC articles is inherently connected to the presence of an active and engaged community.
RQ2. Cultural Context Content Articles Over Time
The considerable extent of CCC shows that editors engage in contributing with content related articles to their context. One could think that topics about one's very near context may be finite or would stop being notable, especially if compared to the amount of universal content which deserves being included into an encyclopedia. However, we hypothesize that editing CCC could be an activity sustained over time, as editors may feel attached to their cultural context and keep enriching it in their language edition. To verify this, we propose an analysis of how CCC has been created over time. Such analysis may explain the most productive period and predict future scenarios. To investigate whether the creation of cultural context content is consistent over time, we count the number of CCC articles created every year in a Wikipedia language edition, since its creation until January 2016, and compare it to the overall number of articles created every year.
Results
Figure 4 shows the growth of each Wikipedia language edition in terms of number of articles. CCC articles are depicted in green, while the rest of the articles in gray. Figure 4 also represents the percentage of CCC created every year (green and red indicate, respectively values above and below the overall percentage). In general, CCC creation tends to remain as a stable part of the activity over the years, although some general patterns can be noticed (RQ2). The most prolific period tends to be located between 2005 and 2010, when Wikipedia language editions experienced their most important growth. It is the same period when the highest percentages of CCC for most languages occurred, which suggests that the most important bursts in content creation have been dominated by the local cultural context.
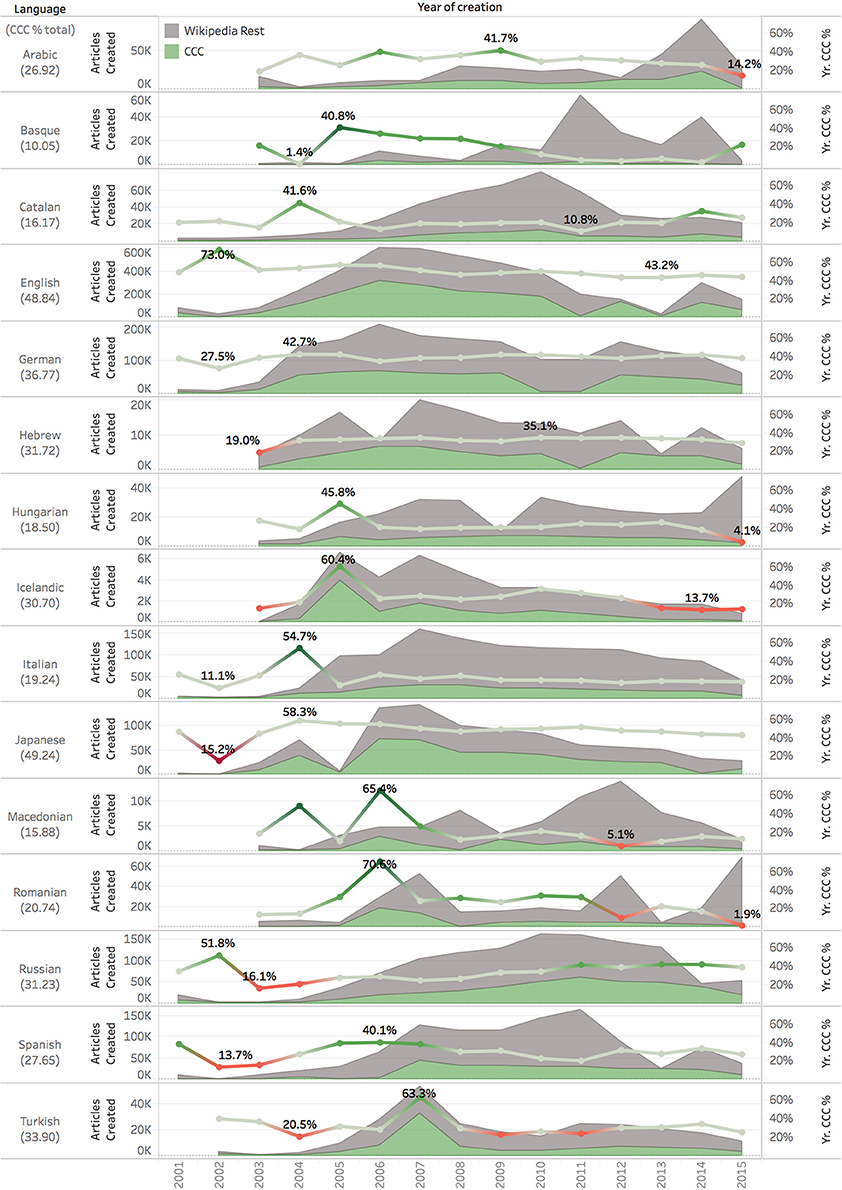
Figure 4. CCC creation over the 15 years of Wikipedia. For each language edition, the green area represents the absolute number of CCC articles created over years, and the gray area the rest of the articles created. The line shows the percentage of CCC over the total number of articles created during each year; it is depicted in gray when it is in line (<10% variation) with the final overall percentage of CCC in the encyclopedia, in green or red when it is higher or lower.
Usually CCC has grown parallel to Wikipedia, but in those years, it also grew more proportionally, occupying an important percentage of the entire amount of Wikipedia articles. After the years of “content boom,” the proportion of CCC tends to get stabilized for most of the languages and does not decrease. Generally, large Wikipedia language editions with strong communities, such as the English and the German ones, exhibit a more balanced growth, less affected by spikes in the creation of content, as it happens for instance in the Icelandic or the Macedonian Wikipedia.
RQ3. Cross-Language Coverage of Cultural Context Content
To address our third research question concerning cross-language coverage of CCC, we look at the Interlanguage links (ILL), i.e., links that connect the same article in two different languages. Interlanguage links may be created either by editors or by automatic bots and allow one to map content coverage between language editions. Previous work has shown that many articles tend to be created first in large language editions, and then translated and re-adapted into smaller language editions [14]. In our case however we expect to find different patterns; as we focus on content that is specific to each cultural context, the presence or absence of interlanguage links is an indicator of the degree of uniqueness, while the interwiki links toward specific language versions show the coverage that each cultural context receives from other language communities.
Interlanguage Links Analysis
Results
As seen in Table 2, the average number of ILLs per article is variable across languages, both in CCC articles and in the entire Wikipedia. The average for CCC articles is 4.15 times lower than the overall average (RQ3). Therefore, CCC is less shared across languages, and part of the language gap is due to the fact that the content representing the cultural context is not shared across languages. Namely, we can affirm that in the language gap there is a culture gap (where by culture gap is intended the CCC articles not shared across languages). Even though in most cases, the average number of ILLs in CCC is lower than in the entire Wikipedia, the ratio (avg. ILLs CCC/avg. ILLs WP) is also variable across languages. In fact, minor language editions like Icelandic, Afrikaans, Estonian and Swahili have between 7 and 11 times less ILLs in CCC than in their entire language edition. On the contrary, languages like English, French, Korean, German, and Italian, which represent the largest Wikipedia language editions, show smaller differences between ILLs in CCC and overall. This suggests that both language status and development degree of a Wikipedia language edition may strongly influence whether its CCC articles are created into other languages.
In order to further investigate the culture gap in each language edition, we measure the percentage of articles with no ILLs in CCC and the entire WP. This allows us to observe the degree to which CCC articles are responsible for the differences in content imbalance between Wikipedia language editions. Results show that languages with a high percentage of articles with no Interlanguage Links (WP NO ILLs) also tend to have a high percentage of CCC articles. In fact, CCC articles with no ILLs account for the majority of Wikipedia content with no ILLs in most languages (mean 62.83%, median 63.25%, and standard deviation 12.31%, without taking into account the results for languages such as Vietnamese, Waray–Waray, and Cebuano, where the automatic program bot had a major contribution in the creation and translation of articles from other language editions). This confirms again that to a great extent the culture gap is responsible for the language gap between Wikipedia language editions described by Warncke-Wang et al. [14].
CCC Cross-Language Availability
Results
Taking a closer look at CCC's Interlanguage links, it is possible to obtain a better understanding of the proximity between language communities, as indicated by the availability or expansion of CCC across Wikipedia language editions. To study such proximity, we compute the proportion of CCC articles from a particular language edition that can be found in other language editions (e.g., the proportion of the Italian CCC articles in the Catalan Wikipedia). In Figure 5 we depict a network of languages and show which ones have a higher proportion of CCC articles represented in other language editions. In order to create this graph, for each CCC we select the three languages where it is represented in the highest proportion and draw the corresponding edges. The network is therefore directed, as a link from language A to language B implies that B is one of the three language editions with better coverage of A's CCC articles, and this relationship is not necessarily reciprocal as A could have a poor coverage of B's CCC. Following a standard convention in graph representation, edges are curved and drawn in clockwise direction. Colors are assigned according to the clusters identified by the Louvain community detection algorithm [19] to highlight groups of language editions that are closer to each other.
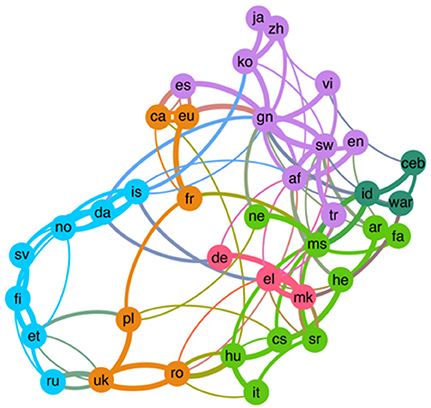
Figure 5. Network graph of language proximity in terms of shared CCC articles. Each node represents a Wikipedia language edition, and has three outgoing links to the three language editions in which its CCC represents the highest percentage of the articles. Links are represented in clockwise direction. Colors represent clusters of language editions identified through the Louvain algorithm for community detection.
Nordic languages form a cluster which includes Russian, while languages of the Iberian Peninsula are tightly connected to each other, as well as languages of Asia and Middle East. These results point out the relevance of geographic proximity and seem to confirm Tobler Law's idea according to which things near tend to be similar. These finding is in line with the comparison of biographical articles' availability in different languages [20, 21]. However, some less expected relationships also become apparent, such as the relevance of Italian CCC articles in the Hungarian Wikipedia.
Mapping the Culture Gap
Results
To see how well each Wikipedia language edition covers the CCC articles of other languages, we created Figure 6. The entire table allows to see the culture gap of each language edition, and how this also depends on linguistic and geographical proximity. However, it seems the factor of scale is more important, since wide language editions (in number of articles and created by large communities such as English, German, French, etc.) cover a higher percentage of the CCC articles of language editions that are significantly smaller.
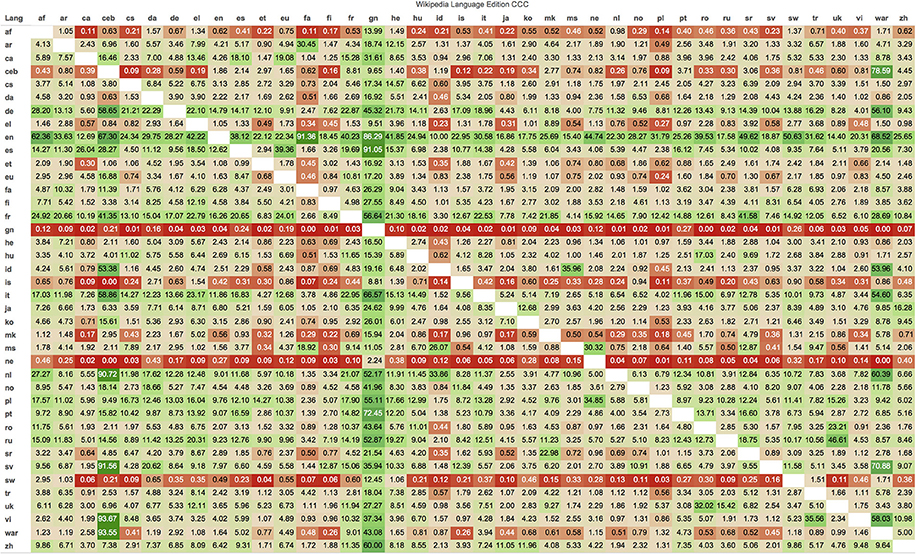
Figure 6. Culture gap: 40 Wikipedia language editions coverage (% articles) of 40 Wikipedia language editions CCC. Each row shows the coverage of other language editions' CCC. The coverage is calculated as the percentage of a Wikipedia language edition CCC (column) which exists in another Wikipedia language edition (row). For an easy identification of values, cells are colored in a scale of colors from red (to indicate a percentage lower than 1%), to green in a continuum until 93.67% (the highest value).
Generally, the culture gap highlights a common difficulty in achieving a representation of cultural diversity, indicating that editors are often not able to cover concepts from other cultures. Few languages cover a good percentage of the CCC of other languages. English is one of them, but still it only covers on average a 33.71% of the CCC articles of other languages (median 28.27%, standard deviation 19.36%). Conversely the CCC of large language editions such as English or German are poorly represented in the other language editions (barely 5% of the English and German CCC articles are found in other language editions). Considering the dimensions of the English and German editions as well as the difficulty of translating a large percentage of relevant articles for their culture into other languages, such a gap is not surprising.
Conclusions
In this paper we have presented a study of the content imbalances in Wikipedia language editions as a result of the impact of their cultural contexts. To this aim, we have proposed a method to analyze Wikipedia content and select articles that specifically relate to the cultural context of each Wikipedia language edition. We named such articles Cultural Context Content (CCC), whether they are about geography, people, language, traditions, among other topics. The method relies on a combination of different strategies in order to retrieve articles, leveraging characteristics such as geolocation, specific keywords in the titles, associated categories or links to other articles. We applied it to 40 language editions selected according to a diversity criterion. The method accuracy has been assessed manually resulting in an average of 8.1% of false positives, 5.9% of false negatives, and an accuracy of F1 = 0.92.
Limitations
Our work is not exempt of limitations, some of which are intrinsic to the same nature of cultural context, while other are more related to the constraints set by the Wikipedia data structure and the method proposed. Although we established a language-territory mapping for each Wikipedia language edition, the method aggregates all the articles from the different territories into a single generated CCC dataset, despite in some cases they may be geographically distant and share few elements in common but the language. We could consider this a limitation of the current dataset, since it would be much better to have a more fine grained collection which would allow further investigation and applications. For instance, the cross-language analysis proposed could be developed into more depth to bring new insights on particular cultural contexts within a language (e.g., British or US with respect to the English language edition) or even across different ones in the same territory (e.g., assessing differences and similarities between Ireland CCC in the English and in the Gaelic Wikipedia).
In regards to the method, even though the results from the manual assessment can be considered satisfactory, we want to acknowledge several observations. First, we need to be cautious that the generated list of keywords may not be as extensive as it would be desirable. Even though articles usually employ the territory names and demonyms, there may exist specific cases which employ other forms which our keywords do not capture. Even though the lack of these words may not imply missing a significant number of articles, it would be necessary to obtain a systematic source for them, either a database or a collaboratively created space in the same Wikipedia, especially when considering to extend the method from 40 to all the 288 available language editions. Second, we are aware that using the category crawling strategy in order to retrieve articles may not be as reliable when the categorization is not exhaustive or precise. This is likely to be the main issue behind the lower accuracy obtained for some languages such as Korean and Waray. In future developments of the method we plan to introduce strategies that take into account Wikidata9, a rich complementary structured database which contains a variety of properties and relationships between items. Other strategies to diminish interference include using articles solidly included as CCC for another language as a negative ground-truth. Machine learning approaches could also be used to improve accuracy.
As a final general consideration, in this study we have pursued a quantitative approach, in order to be able to delimit and quantify the content specific to the context associated to each linguistic version of Wikipedia; as we deal with cultural contexts, boundaries are of course not always straightforward, and the manual assessment demonstrated that, although in few cases, even human experts may disagree on the definition of this task. While a comprehensive qualitative inspection of the results would help to more deeply grasp and interpret the findings of this work, such kind of analysis is out of the scope of this work and would be unviable at the scale of the whole dataset, which includes millions of articles in 40 different languages. However, we believe that making our method and the resulting datasets available has the potential to open up to more focused studies including qualitative methods and delving into specific aspects of the complex phenomenon of which we have here offered a first quantitative overview.
Main Findings
Our analyses offer new insights into how the cultural context impacts Wikipedia content coverage and imbalances across languages. In first place, the analysis of cultural context content in 40 language editions shows that its extent ranges from 7 to 49% of the total number of articles, with an average value of 23.53% (RQ1). This is a considerable extent, especially considering that CCC articles have generally been produced with no specific policy or guideline recommending it, but as an effect of editors' preferences. In second place, an analysis of the creation of CCC over time shows that this content grew constantly along with Wikipedia (RQ2). Even though certain relevant geographical places for the editors (cities, towns, rivers, etc.) can be finite, the degree of specificity that CCC can reach through very different topics implies that new content can continually appear. In third place the analysis based on ILLs in the 40 languages unveils and quantifies the culture gap: CCC articles are 4.15 times less shared between languages than the average content of each language edition (RQ3), and almost the half of CCC articles do not exist in any other language. This shows that the lack of correspondence of content between languages found by previous research [14, 22] is due largely to CCC articles. The graphs provided to illustrate this culture gap can be useful to show editors from every language edition which cultural context content of other languages should be priorly imported or extended.
Theoretical Implications
Our study makes a contribution to the online communities and cultural contextualization literature. The imbalance of content across languages has been seen as a negative issue, since it hinders the goal of achieving “the sum of human knowledge,” and is often explained by several demographic and territory factors. Some authors have demonstrated how editors tend to edit about geographically close territories [15], or how the overall article link structure in each Wikipedia language edition revolves around the countries where the language is spoken [16]. Proving also the centrality of geographical context, other authors have shown that editors' editing interests are similar when languages are geographically near [12–14].
Differently from these studies, we wondered whether obtaining all the content associated with a language cultural context could explain in a more thorough way the impact of cultural context on the content coverage and imbalances across languages. In fact, the results from the interlanguage analysis of Cultural Context Content are in in line with the results from previous literature that language communities share common interests [12]. The fact that large part of the language gap is due to the CCC articles confirms that cultural and geographical context influences communities' common interests. At the same time, the same constitution of the CCC dataset and the filtering process showed us that the collection is a continuum, in which there are articles that are prominently at the core of the dataset, while others are less related to the collection central meanings and could even belong to other collections related to neighbor contexts. The CCC datasets allow for further investigations to analyze the content similarities between contexts and their relationships.
As a final consideration, it is important to note that these imbalances in content should not be only considered as a bias to be corrected, but also as a natural expression of cultural diversity, which represents a richness of the Wikipedia project. In this sense, our work can be seen as a first effort to quantify and describe such diversity, facing the delicate challenge of tracing the boundaries between cultural contexts.
Implications for Practice
As an important contribution of this paper, we make available both the code we used to process the Wikipedia language editions, the language-territories mapping with the keywords and ISO codes, as well as the generated datasets, in a website (Wikipedia Cultural Diversity Observatory10) aimed to both the academia and the Wikipedia communities. We believe this can encourage and motivate new research on cultural context content at the same time as it helps the Wikipedia language communities to bridge the culture gap. At the same time, we believe that increasing the coverage of each other's' cultural context content may be an important goal for fostering inter-cultural dialogue and enrichment. Therefore, we suggest that the translator and the article recommendation tool developed by the Wikimedia Foundation could include CCC from each language, or subparts of it (e.g., articles including keywords in their title) as preferential content to be translated and exported to other languages. Making editors more aware of the culture gap and offering them tools to bridge it will likely encourage them to enrich their Wikipedia language editions with inter-cultural content, enlightening their readers and helping to build a world more open to diversity.
Future Lines of Research
The datasets and methods proposed in this paper suggest several lines for future research. On the one hand, it would be interesting to investigate the overlap between CCC and other relevant groups of articles for a particular reason or metric, e.g., the ones receiving higher attention (in terms of edits and page views), or related to breaking news or to controversial topics. The CCC dataset can be also used as a basis for cross-cultural studies in the field of Digital Humanities. On the other hand, analogous strategies to the ones proposed here to collect the CCC datasets could be applied to identify other kinds of content, and therefore are relevant to studies aimed at providing a topical coverage of Wikipedia or other knowledge repositories.
Data Availability Statement
The datasets generated/analyzed for this study can be found in the “Wikipedia Cultural Diversity Observatory Repository”: https://github.com/marcmiquel/WCDO.
Author Contributions
MM-R contributed with the original idea, the methodology planning and implementation and the first draft. DL contributed with the result analysis and the writing.
Funding
This work has been partially funded by a Project Grant from Wikimedia Foundation and the Catalan Agency for Business Competitiveness, ACCIÓ.
Conflict of Interest Statement
The authors declare that the research was conducted in the absence of any commercial or financial relationships that could be construed as a potential conflict of interest.
Acknowledgments
We want to thank Andreas Kaltenbrunner for his wise critical comments that helped to strengthen the methodology, and Laura Vincze for her precious help to improve style and clarity of the manuscript. We would also like to acknowledge all those who supported the study in a direct or indirect way, including the Wikimedia Foundation and all the Wikimedians who endorsed the Wikipedia Cultural Diversity Observatory project. We hope the methods and datasets presented here as part of this open source project may be of help for all who are working hard to improve Wikipedia's cultural diversity coverage.
Footnotes
1. ^https://en.wikipedia.org/wiki/Wikipedia:Neutral_point_of_view.
2. ^https://en.wikipedia.org/wiki/Wikipedia:Notability
3. ^https://meta.wikimedia.org/wiki/List_of_Wikipedias):
4. ^https://github.com/marcmiquel/WCDO).
5. ^https://www.ethnologue.com.
6. ^https://www.wikidata.org/wiki/Property:P1549.
7. ^http://wikitech.wikimedia.org).
8. ^https://pypi.python.org/pypi/pygeocoder.
9. ^wikidata.org
10. ^https://meta.wikimedia.org/wiki/Grants:Project/Wikipedia_Cultural_Diversity_Observatory_(WCDO)
References
1. Van Dijk Z. Wikipedia and lesser-resourced languages. Lang. Prob. Lang. Plann. (2009) 33:234–50. doi: 10.1075/lplp.33.3.03van
2. Ensslin A. What an un-wiki way of Wikipedia's multilingual policy and metalinguistic practice. J Lang Polit (2011) 10:535–61. doi: 10.1075/jlp.10.4.04ens
3. Hecht BJ. The Mining and Application of Diverse Cultural Perspectives in User-Generated Content. Dissertation, Northwestern University (2013).
5. Massa P, Scrinzi F. Exploring linguistic points of view of Wikipedia. In: Presented at the WikiSym '09: Proceedings of the 5th International Symposium on Wikis and Open Collaboration. New York, NY: ACM Press (2011). p. 213–4.
6. Apic G, Betts MJ, Russell RB. Content disputes in Wikipedia reflect geopolitical instability. PLoS ONE (2011) 6:e20902. doi: 10.1371/journal.pone.0020902
7. Rogers R, Sendijarevic E. Neutral or national point of view? A comparison of srebrenica articles across Wikipedia's language versions. In: Proceedings of the Wikipedia Academy Conference 2012. Berlin (2012).
8. Bao P, Hecht B, Carton S, Quaderi M, Horn M, Gergle D. Omnipedia: bridging the wikipedia language gap. In: Proceedings of the SIGCHI Conference on Human Factors in Computing Systems. Austin, TX: ACM (2012, May). p. 1075–84.
9. Pentzold C, Weltevrede E, Mauri M, Laniado D, Kaltenbrunner A, Borra E. Digging Wikipedia. J Comput Cult Herit. (2017) 10:1–19. doi: 10.1145/3012285
10. Callahan ES, Herring SC. Cultural bias in Wikipedia content on famous persons. J Am Soc Inform Sci Technol. (2011) 62:1899–915. doi: 10.1002/asi.21577
11. Ronen S, Gonçalves B, Hu KZ, Vespignani A, Pinker S, Hidalgo CA. Links that speak: the global language network and its association with global fame. Proc Natl Acad Sci USA. (2014) 111:E5616–22. doi: 10.1073/pnas.1410931111
12. Samoilenko A, Karimi F, Edler D, Kunegis J, Strohmaier M. Linguistic neighbourhoods: explaining cultural borders on Wikipedia through multilingual co-editing activity. EPJ Data Sci. (2016) 5:9. doi: 10.1140/epjds/s13688-016-0070-8
13. Karimi F, Bohlin L, Samoilenko A, Rosvall M, Lancichinetti A. Quantifying national information interests using the activity of Wikipedia editors. ArXiv Abs/1312.0976 (2015) 1503:5522. doi: 10.1057/palcomms.2015.41
14. Warncke-Wang M, Uduwage A, Dong Z, Riedl J. In search of the ur-Wikipedia: universality, similarity, and translation in the Wikipedia inter-language link network. In: Proceedings of the Eighth Annual International Symposium on Wikis and Open Collaboration. ACM (2012). p. 20.
15. Hecht BJ, Gergle D. On the localness of user-generated content. In: Proceedings of the 2010 ACM Conference on Computer Supported Cooperative Work. ACM (2010). p. 229–232.
16. Hecht B, Gergle D. Measuring self-focus bias in community-maintained knowledge repositories. In: Proceedings of the Fourth International Conference on Communities and Technologies. ACM (2009). p. 11–20.
17. Miquel-Ribé M, Laniado D. Cultural identities in wikipedias. In: Proceedings of the 7th 2016 International Conference on Social Media and Society. ACM (2016). p. 24.
18. Cohen J. A coefficient of agreement for nominal scales. Educ Psychol Meas. (2016) 20:37–46. doi: 10.1177/001316446002000104
19. Blondel VD, Guillaume JL, Lambiotte R, Lefebvre E. Fast unfolding of communities in large networks. J Stat Mech. (2008) 10:P10008. doi: 10.1088/1742-5468/2008/10/P10008
20. Aragón P, Laniado D, Kaltenbrunner A, Volkovich Y. Biographical social networks on Wikipedia: a cross-cultural study of links that made history. In: Presented at the WikiSym '09: Proceedings of the 5th International Symposium on Wikis and Open Collaboration. New York, NY: ACM Press (2012). p. 19.
21. Eom YH, Aragón P, Laniado D, Kaltenbrunner A, Vigna S, Shepelyansky DL. Interactions of cultures and top people of Wikipedia from ranking of 24 language editions. PLoS ONE (2015) 10:e0114825. doi: 10.1371/journal.pone.0114825
Keywords: content imbalance, cross-cultural studies, cultural diversity, online communities, wikipedia, digital humanities, data mining, big data
Citation: Miquel-Ribé M and Laniado D (2018) Wikipedia Culture Gap: Quantifying Content Imbalances Across 40 Language Editions. Front. Phys. 6:54. doi: 10.3389/fphy.2018.00054
Received: 01 February 2018; Accepted: 17 May 2018;
Published: 06 June 2018.
Edited by:
Marija Mitrovic Dankulov, University of Belgrade, SerbiaReviewed by:
Ann Samoilenko, Universität Koblenz Landau, GermanyJuyong Park, Korea Advanced Institute of Science & Technology (KAIST), South Korea
Copyright © 2018 Miquel-Ribé and Laniado. This is an open-access article distributed under the terms of the Creative Commons Attribution License (CC BY). The use, distribution or reproduction in other forums is permitted, provided the original author(s) and the copyright owner are credited and that the original publication in this journal is cited, in accordance with accepted academic practice. No use, distribution or reproduction is permitted which does not comply with these terms.
*Correspondence: Marc Miquel-Ribé, bWFyY21pcXVlbEBnbWFpbC5jb20=