- 1PoreLab, Department of Physics, Norwegian University of Science and Technology, Trondheim, Norway
- 2Beijing Computational Sciences Research Center (CSRC), Beijing, China
- 3PoreLab, Department of Physics, University of Oslo, Oslo, Norway
- 4PoreLab, Department of Chemistry, Norwegian University of Science and Technology, Trondheim, Norway
We explore the anomalous diffusion that may arise as a result of a concentration dependent diffusivity. The diffusivity is taken to be a power law in the concentration, and from exact analytical solutions we show that the diffusion may be anomalous, or not, depending on the nature of the initial condition. The diffusion exponent has the value of normal diffusion when the initial condition is a step profile, but takes on anomalous values when the initial condition is a spike. Depending on the sign of the exponent in the diffusivity the diffusive behavior will then be either sub-diffusive or super-diffusive. We introduce a particle model that behaves according to the non-linear diffusion equation in the macroscopic limit. This correspondence is demonstrated via kinetic theory, i.e. by means of Chapman-Kolmogorov equation, as well as by direct simulations.
Introduction
A wide range of interesting physical systems are described by a diffusion equation where the diffusivity depends on the concentration of the diffusing quantity. That is, we have a concentration
where
Here
We e.g., find such behavior when compressible gases flow through porous media [1, 2] or in filtration processes [3]. Another example is heat diffusion at high temperature [4, 5]. Population dynamics gives rise to this kind of behavior [6–8], as does water ingress in zeolites as studied by Azevedo et al. [9, 10] and Fischer et al. [11]. The diffusion of grains in granular media considered by Christov and Stone [12] is yet another example. Pritchard et al. [13] studied gravity-driven fluid flow in layered porous media finding that the fluid motion could be described by a concentration-dependent diffusivity as did Hansen et al. [14] for the spreading of wetting films in wedges.
Common to all of these examples is that γ is negative, ranging from
where
There are two classes of examples in the list above: systems where there is a physical quantity that is diffusing due to random motion, and systems that are only described by the diffusion equation. The diffusion of excess porosity in the packing of beads is an example of the first class. On the other hand, the motion of a fluid-fluid interface under gravity in a porous layer [13] is an example of a system where there is no random diffusion.
In either case, there being physical diffusion or the system may be mapped onto equations having the form of a diffusion equation, we may consider an equivalent system consisting of diffusing particles. The concentration-dependent diffusivity may then be interpreted as mean-field type interaction between the particles, where the behavior of each particle is determined by the number of neighbors it has.
A key quantity to characterize diffusive behavior is the root mean square (rms) displacement traveled by the particles — i.e., random walkers — as a function of time. If a random walker in one dimension starts at position
When the diffusion exponent
There are several possible ways the behavior of the diffusivity in the diffusion equation may lead to anomalous diffusion. It may do so by having a position dependent diffusivity, see e.g., Refs. 21–24. The diffusivity we are considering in this work also depends on the position, but only through the concentration as in Eq. 3.
There is a relation between the exponent γ defined in Eq. 3 and the random walk exponent τ in Eq. 5,
This equation was first worked out by Pattle [25] for negative γ indicating sub diffusion. We will in this paper extend this equation to positive γ, using the self-similarity approach [26–28]. We find that Eq. 6 is valid for the range
In spite of the relation Eq. 6 between γ and the exponent that defines anomalous diffusion, τ, it is sometimes believed that the Boltzmann transformation [29] demonstrates that there is no anomalous diffusion, even when the diffusivity depends on the concentration. This is, e.g., demonstrated in the famous textbook by Crank [30]. However, the random walk exponent τ, depends on the initial condition Eq. 2.
We support our analytical work by numerical simulations through the introduction of a particle model where random walkers move in discrete steps that depend on the local concentration. We show that the average description of this model, that is given by the Chapman-Kolmogorov equation, reduces to Eq. 7. Hence, we are able to reproduce the analytical results by particle simulations. These particle simulations are easily generalized to higher dimensions and different kinds of non-linearities in the diffusion equation that describes them.
Analytic Solutions
The analytic results are most conveniently obtained by rewriting Eq. 1 in the form [31]
Hence, we see that we need
Boltzmann Transformation and the Step
In order for the Boltzmann transformation to be applicable we take the initial conditions to be
When the t and x derivatives are transformed to y-derivatives the diffusion Eq. 7 becomes the ordinary differential equation,
with the x and t dependence through y only. Now, the initial condition too may be written in terms of y alone: For
for some function q that satisfies Eq. 9. This immediately leads to the conclusion
i.e., that the diffusion is normal with
Exact Solutions From Delta-Function Initial Condition
Solving Eq. 7 with a point source initial condition turns out to change the diffusion exponent τ. Hence, we start with
where
There is no intrinsic length or time-scale in Eq. 7 since the diffusivity depends on C through the power law of Eq. 3. This means that as long as boundary- or initial conditions do not introduce such scales either, the solutions
This ensures that the normalization of Eq. 13 remains constant with time. We now choose λ so that
Combined with Eq. 14, this gives
where we have set
We introduce the reduced variable
and so get
and
Equation 7 may then be transformed into
This means that for some dimensionless separation constant c
and
The property expressed by Eq. 14 is that the right‐hand side is independent of λ. For this reason replacing
Our solution thus has the scaling form
for some function g and with τ given by Eq. 6. Note that this form immediately gives
This is in contrast to the Boltzmann transformation, which assumes step-like initial conditions, thus leading to
We now turn to finding an expression for the probability density
which may be integrated to
where K is an integration constant.
On physical grounds we require that Fick’s law be valid throughout the domain, and so C must be continuously differentiable. By symmetry it must also be symmetric around
which is integrable. Rewriting it as
it may be integrated to yield
where k is yet an integration constant. For
The constant k is determined by the normalization condition
which follows from Eq. 13. Since the two cases of positive and negative γ-values give either finite or infinite support for p , they give rise to different functions
where the integrals
These integrals may be calculated numerically, or evaluated through the analytic expressions
and
where
We may now reconstruct the normalized concentration field
where
and for
We now calculate
where for
For
For
Derivation of the Diffusion Equation From Particle Dynamics
We now turn to stochastic modeling of the process we so far have described using the non-linear diffusion Eq. 7. Following the discussion in the van Kampen book Stochastic Processes in Physics and Chemistry [32], we derive the non-linear diffusion equation, which is an example of a Fokker-Planck equation.
Particle Model and the Fokker-Planck Equation
A population of
where
is a Wiener process and η is a random variable with
where
where
according to Eq. 43. Setting
and requiring equivalence with Eq. 7 thus implies that
Itô-Stratonovitch Dilemma
However, the presence of a C-dependence in the diffusivity D introduces an ambiguity in the implementation of Eq. 42, since now
Stratonovitch read Eq. 42 as [32]
while Itô read it as
It turns out that it is the choice opted for by Itô that gives Eq. 45, while the Stratonovitch choice gives
see the van Kampen book [32], Chapter VI.4 for a derivation of this result. By setting
and equivalence with Eq. 7 now implies that
It is also possible to read read Eq. 42 as
This is known as the Hänggi-Klimontovich interpretation [22–24, 33, 34] Setting
and equivalence with Eq. 7 now implies that
This means that the only difference between the different implementations of Eq. 42 is the magnitude of the random step. In the Itô case the step length has to be a bit smaller than in the Stratonovitch case, which in turn is smaller than in the Hänggi-Klimontovich case, in order to correspond to the same macroscopic descriptions for
The other implementations are complicated by the fact that the step length of the particles depends on the local concentration after the steps are taken. Numerically, this problem is similar to that of implicit solvers for partial differential equations.
Simulations
The numerical simulations are carried out using a population of
The Boltzmann Case
We start by considering the step initial conditions in the simulations by setting
Figure 1A shows the concentration profile
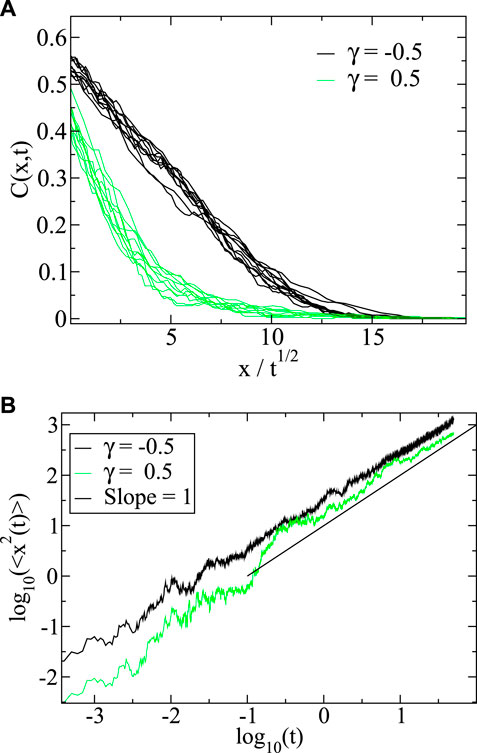
FIGURE 1. (A) Evolution of the step profile when
In Figure 1B we show the mean square displacement
The Initial Delta-Function
In this case all the particles start at the origin for
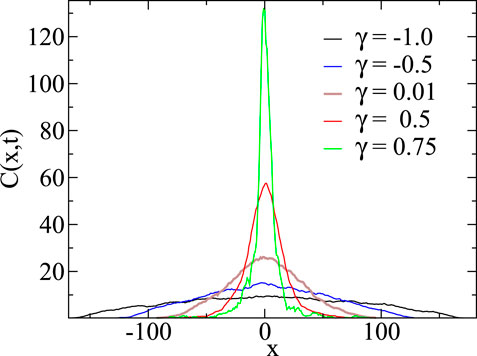
FIGURE 2. Concentration resulting from an initial δ-profile as a function of position for negative and positive γ-values after a time t = 50. For
Figure 3 show simulations compared to the analytic prediction of Eq. 30. We plot
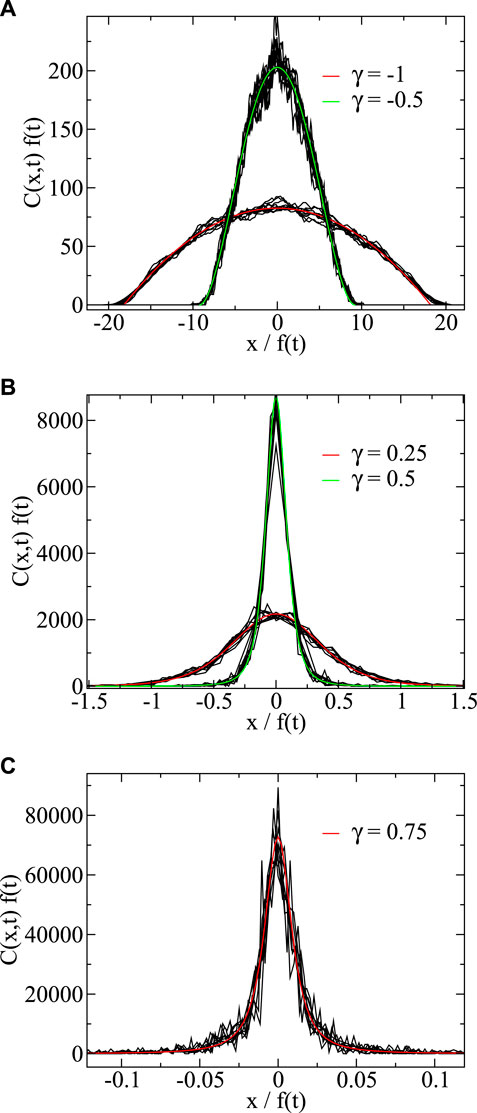
FIGURE 3. Scaled concentration as a function of scaled position for negative (A) and positive (B,C) γ-values. For the negative γ-values
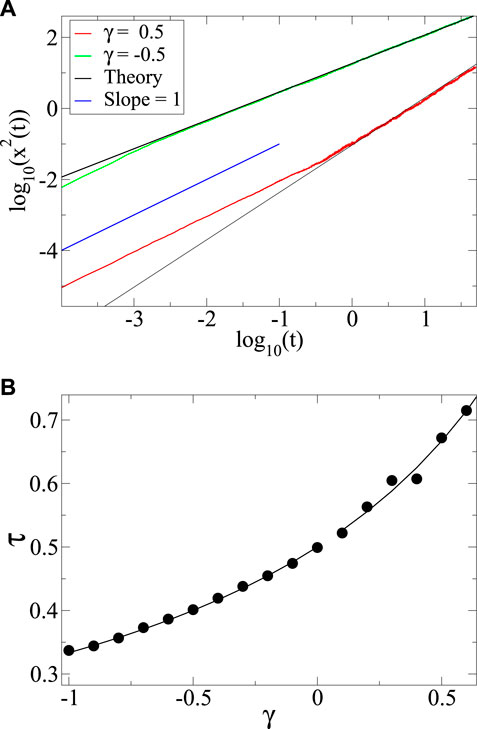
FIGURE 4. (A) Mean square displacement
Summary and Conclusion
With a power law diffusivity and a delta-function initial condition, there is no intrinsic length scale in the problem. In this case normalizability leads to the scaling form of Eq. 24 leading to the exponent relation Eq. 6 which is the defining characteristic of anomalous diffusion. With a step function initial condition however, as first studied by Boltzmann [26] the solution extends to
We have reviewed the Boltzmann result and demonstrated, as did Pattle 60 years ago [25], that when
We have also constructed a stochastic particle dynamics that satisfies the non-linear diffusion equation at hand, and implemented it computationally. Using this approach, we were able to verify the central results that we were derived analytically, and shown agreement between simulations and theory. In future applications, the particle model may also serve as a stepping stone for generalizations of our present model, that are not analytically tractable.
Both Küntz and Lavallée [15] and Gosh et al. [16] report sub-diffusion when the diffusivity decreases with increasing concentration. Figure 2, where we show the concentration profile
As mentioned in the introduction, anomalous diffusion originating from a concentration dependent diffusivity may have been seen in diffusion in granular media [11, 12]. These observations are based on rotating a bi-disperse composition of smaller and large glass beads in a horizontal cylindrical mixer. The mixer is filled with the larger beads except for a small disk of smaller beads. As the cylinder turns, the smaller beads diffuse into the larger beads and the concentration of smaller beads as a function of time and position along the cylinder is recorded. This setup mimics closely the initial conditions that we have studied here, except for Section 2.1, where we assumed a step initially. The connection with the present work is the proposal that the diffusivity of the smaller beads is larger when they are surrounded by other smaller beads than when they are surrounded by the larger beads; the higher the concentration of smaller beads, the larger their diffusivity is. We propose here to prepare the packing in a different way initially. Fill (say) the left half of the cylinder with the smaller beads and the right half with the larger beads. The system is therefore initiated with a step function in the concentration. One would then expect normal diffusion where the front evolves as
Data Availability Statement
The datasets generated for this study are available on request to the corresponding author.
Author Contributions
AH derived the solution to the diffusion equation and EF designed the numerical simulations and derived the macroscopic description via a Fokker-Planck equation. BB expanded the analytic solution to the positive gamma case.
Funding
This work was partly supported by the Research Council of Norway through its Centers of Excellence funding scheme, project number 262644.
Conflict of Interest
The authors declare that the research was conducted in the absence of any commercial or financial relationships that could be constructed as a potential conflict of interest.
Acknowledgments
We thank M. R. Geiker, P. McDonald, and members of the ERICA network for interesting discussions. AH thanks Hai-Qing Lin and the CSRC for friendly hospitality.
References
1. Muskat M. The flow of fluids through porous media. J Appl Phys (1937) 8:274–82. doi:10.1063/1.1710292
2. Barenblatt GI, Entov VM, Ryzhik VM. Theory of fluid flows through natural rocks. Dordrecht, Netherlands: Kluwer (1990).
3. Kamenomostskaya SK. Similar solutions and the asymptotics of filtration equations. Arch Rational Mech Anal (1976) 60:171–83. doi:10.1007/BF00250678
4. Zeldovich IB, Kompaneez AS. On the theory of heat propagation with heat conduction depending on temperature. Lectures dedicated on the 70th Anniversary of A. F. Joffe. Moscow, Russia: Akad Nauk SSSR (1950).
5. Zeldovich Y, Raizer YP. Physics of shock waves and high-temperature hydrodynamic phenomena, Vol. 2. New York, NY: Academic Press (1968).
6. Gurtin ME, MacCamy RC. On the diffusion of biological populations. Math Biosci (1977) 33:35–49. doi:10.1016/0025-5564(77)90062-1
7. Newman WI. Some exact solutions to a non-linear diffusion problem in population genetics and combustion. J Theor Biol (1980) 85:325–34. doi:10.1016/0022-5193(80)90024-7
9. de Azevedo EN, de Sousa PL, de Souza RE, Engelsberg M, Miranda MdeN, Silva MA. Concentration-dependent diffusivity and anomalous diffusion: a magnetic resonance imaging study of water ingress in porous zeolite. Phys Rev E (2006) 73:011204. doi:10.1103/PhysRevE.73.011204
10. de Azevedo EN, da Silva DV, de Souza RE, Engelsberg M. Water ingress in Y-type zeolite: anomalous moisture-dependent transport diffusivity. Phys Rev E (2006) 74:041108. doi:10.1103/PhysRevE.74.041108
11. Fischer D, Finger T, Angenstein F, Stannarius R. Diffusive and subdiffusive axial transport of granular material in rotating mixers. Phys Rev E (2009) 80:061302. doi:10.1103/PhysRevE.80.061302
12. Christov IC, Stone HA. Resolving a paradox of anomalous scalings in the diffusion of granular materials. Proc Natl Acad Sci USA (2012) 109:16012–7. doi:10.1073/pnas.1211110109
13. Pritchard D, Woods AW, Hogg AJ. On the slow draining of a gravity current moving through a layered permeable medium. J Fluid Mech (2001) 444:23–47. doi:10.1017/S002211200100516X
14. Hansen A, Skagerstam B-S, Tørå G. Anomalous scaling and solitary waves in systems with nonlinear diffusion. Phys Rev E (2011) 83:056314. doi:10.1103/PhysRevE.83.056314
15. Küntz M, Lavallée P. Anomalous diffusion is the rule in concentration-dependent diffusion processes. J Phys D Appl Phys (2004) 37:L5. doi:10.1088/0022-3727/37/1/L02
16. Gosh SK, Cherstvy AG, Grebenkov DS, Metzler R. Anomalous non-Gaussian tracer diffusion in crowded two-dimensional environments. New J Phys (2016) 18:013027. doi:10.1088/1367-2630/18/1/013027
17. Bouchaud J-P, Georges A. Anomalous diffusion in disordered media: statistical mechanisms, models and physical applications. Phys Rep (1990) 195:127–293. doi:10.1016/0370-1573(90)90099-N
18. Metzler R, Klafter J. The random walk’s guide to anomalous diffusion: a fractional dynamics approach. Phys Rep (2000) 339:1–77. doi:10.1016/S0370-1573(00)00070-3
19. Levi L, Krivolapov Y, Fishman S, Segev M. Hyper-transport of light and stochastic acceleration by evolving disorder. Nature Phys (2012) 8:912–7. doi:10.1038/nphys2463
21. Cherstvy AG, Chechkin AV, Metzler R. Anomalous diffusion and ergodicity breaking in heterogeneous diffusion processes. New J Phys (2013) 15:083039. doi:10.1088/1367-2630/15/8/083039
22. Cherstvy AG, Chechkin AV, Metzler R. Particle invasion, survival, and non-ergodicity in 2D diffusion processes with space-dependent diffusivity. Soft Matter (2014) 10:1591–601. doi:10.1039/C3SM52846D
23. Regev S, Grønbech-Jensen N, Farago O. Isothermal Langevin dynamics in systems with power-law spatially dependent friction. Phys Rev E (2016) 94:012116. doi:10.1103/PhysRevE.94.012116
24. Leibovich N, Barkai E. Infinite ergodic theory for heterogeneous diffusion processes. Phys Rev E (2019) 99:042138. doi:10.1103/PhysRevE.99.042138
25. Pattle RE. Diffusion from an instantaneous point source with a concentration-dependent coefficient. Q J Mechanics Appl Math (1959) 12:407–9. doi:10.1093/qjmam/12.4.407
26. Barenblatt GI. Scaling, self-similarity, and intermediate asymptotics. Cambridge, UK: Cambridge University Press (1996). doi:10.1017/CBO9781107050242
27. Witelski TP, Bernoff AJ. Self-similar asymptotics for linear and nonlinear diffusion equations. Stud Appl Math (1998) 100:153–93. doi:10.1111/1467-9590.00074
28. Nabokov R. An inverse problem for the porous medium equation: identification of the permeability. In: J Gottlieb, and P DuChateau, editors Parameter identification and Inverse problems in hydrology, geology and ecology, water science and technology library. Vol. 23. Dordrecht, Netherlands: Springer (1996). doi:10.1007/978-94-009-1704-0_9
29. Boltzmann L. Zur Integration der Diffusionsgleichung bei variabeln Diffusionscoefficienten. Ann Phys (1894) 289:959–64. doi:10.1002/andp.18942891315
31. Hristov J. Integral solutions to transient nonlinear heat (mass) diffusion with a power-law diffusivity: a semi-infinite medium with fixed boundary conditions. Heat Mass Tran (2016) 52:635–55. doi:10.1007/s00231-015-1579-2
32. van Kampen NG. Stochastic processes in physics and chemistry. 3rd ed. Amsterdam, Netherlands: North-Holland (2007).
33. Volpe G, Wehr J. Effective drifts in dynamical systems with multiplicative noise: a review of recent progress. Rep Prog Phys (2016) 79:053901. doi:10.1088/0034-4885/79/5/053901
Keywords: anomalous diffusion, concentration-dependent diffusivity, non-linear diffusion equation, non-linear diffusivity, Boltzmann transformation
Citation: Hansen A, Flekkøy EG and Baldelli B (2020) Anomalous Diffusion in Systems with Concentration-Dependent Diffusivity: Exact Solutions and Particle Simulations. Front. Phys. 8:519624. doi: 10.3389/fphy.2020.519624
Received: 12 December 2019; Accepted: 16 October 2020;
Published: 14 December 2020.
Edited by:
Víctor M. Eguíluz, Institute of Interdisciplinary Physics and Complex Systems (IFISC), SpainReviewed by:
Jordan Yankov Hristov, University of Chemical Technology and Metallurgy, BulgariaRalf Metzler, University of Potsdam, Germany
Copyright © 2020 Hansen, Flekkøy and Baldelli. This is an open-access article distributed under the terms of the Creative Commons Attribution License (CC BY). The use, distribution or reproduction in other forums is permitted, provided the original author(s) and the copyright owner(s) are credited and that the original publication in this journal is cited, in accordance with accepted academic practice. No use, distribution or reproduction is permitted which does not comply with these terms.
*Correspondence: Alex Hansen, YWxleC5oYW5zZW5AbnRudS5ubw==