- 1School of Business, East China University of Science and Technology, Shanghai, China
- 2Research Institute of Sports Economics, East China University of Science and Technology, Shanghai, China
- 3Department of Mathematics, East China University of Science and Technology, Shanghai, China
Nighttime lights (NTLs) have been used as a proxy for economic growth in recent years. To verify the effectiveness of NTL in measuring regional economies, this article studies the regional economic convergence phenomenon in China’s provinces by a comparative analysis of NTL data and GDP data from 1992 to 2013. It is found that there is a significant difference between the results of club convergence between NTL and GDP; GDP high-growth clubs are mainly concentrated in the east and central areas, while NTL’s high-growth clubs are mostly concentrated in the central and west areas. Besides, the growth rate gaps between GDP clubs are relatively flat, while the growth rate gaps between NTL clubs are large. From the perspective of influencing, factors of the regional convergence, technological innovation, and industrial structure have a significant impact on GDP and NTL, and industrial structure has opposite effects on GDP clubs and NTL clubs. Besides the above factors, for NTL convergence clubs, population growth rate, economic openness, and resource consumption are also significant.
1 Introduction
1.1 Outlining the Problem
The nighttime lights (this study is referred to as NTLs) data released by the National Oceanic and Atmospheric Administration (NOAA) show that NTL is a unique surface landscape phenomenon; it is representing the laws of human activities on the surface of the Earth and closely related to the socio-economic development of human beings. The advancement of technology and the development of NTL data resources have led to the more widespread use of NTL data for characterizing levels of economic growth and exploring the temporal and spatial characteristics and heterogeneity of economic development. This has become a trend in several related fields, and China is one of the most commonly researched regions in the literature on applying NTL to economic and social issues [1]. On the one hand, China’s high-speed economic growth over the past 40 years has been met with great interest by global economists; on the other hand, although China’s economy has maintained rapid growth as a whole, large gaps still exist in regional economic development. As China faces a period of critical strategic opportunity for development and potential social tensions, more attention has been given to balancing economic development between different regions. Also, China’s official GDP statistics have received a question for statistical difficulties, structural incomparable, and human manipulation [2].
For the above reasons, the practice of measuring economic development with the easily obtainable and more objective NTL data has been widely adopted in China, especially in the investigation of regional economic development issues. However, these studies have not thoroughly investigated the reliability of using NTL as an indicator of regional economic gaps. Questions remain over the difference between NTL data and traditional GDP statistics in measuring regional economic disparities, the difference between the two in reflecting objective economic facts and laws, and what kind of GDP economic information can and cannot be characterized by NTL. At the same time, previous studies also ignore China’s economy’s characteristics in a transition period, including the balanced growth of multiple regional economies and the heterogeneity of economic convergence, both of which directly affect the establishment of research perspectives and selection of research methods.
Given the above problems, as well as in consideration of the regional heterogeneity of development in China and the time-varying convergence rate, this article takes China’s provincial NTL data and GDP data as the research object, breaks the traditional eastern, central, and western division of economic zones, first using the
This work contributes to the literature in three aspects. First, the
1.2 Literature Review
Since NTL has been shown to have a significantly high correlation with regional economic activities (GDP, GRP, etc.) and social activities (population, energy, etc.) [3–9] and NTL can be used as a proxy variable for social and economic activities. An increasing number of studies have begun to use this data to characterize the economy and study the spatial and temporal patterns of regional economies and industries [10–14], urban development [15–21], human activities and their effects [22–24], and energy consumption [22, 25]. Of special interest is the research conducted in Refs. [26–29]. These studies propose a model, based on the proof of the correlation between NTL and GDP that can use the NTL data to correct GDP. This model provides estimates of economic growth for countries whose statistical agencies lack reliability and carry great significance for studying the economics of NTL. The above research enriches the surrogate indicators of economic variables, expands the research methods available for analyzing economic and social problems, opens up research areas in other related issues, and illustrates the great potential of using NTL data in economics [30].
Before introducing NTL, many scholars used GDP as the primary source of economic research data despite some skepticism toward China’s GDP. With the widespread use of NTL in economics research, many scholars have begun using NTL data to investigate China’s economic and social problems, particularly in research related to the correlation between NTL and economic and social indicators [31–33], and in studies that use NTL data to estimate economic and social indicators [34–37]. In a study on regional differences in economic development, Liu et al. conducted an empirical investigation on the spatial differences and spillover effects of urban economic growth in China and found that the economic development of urban agglomerations shows significant spatial nonequilibrium characteristics [38]. Liu and Du use NTL data to re-examine the regional disparity and stochastic convergence characteristics of China’s economic development and helping identify further the economic development of convergence clubs in China’s various regions [39]. Based on the convergence analysis framework of the growth theory, Wang et al. utilize a dynamic panel data model to assess the dynamic trend of China’s regional economic gap and find that between 1992 and 2012, China’s initial nighttime light intensity, whether at the provincial level or the prefecture-city level, regions that initially have lower nighttime light brightness levels exhibit faster growth rates [40]. This level of growth deviates somewhat from per capita GDP trends. Ding and Zhou found that the high concentration of regional NTL on the southeast coast is consistently stable from 1992 to 2013 and is self-reinforcing. They also discovered that population growth, regional surface area, and distance from the coastline are all negatively correlated with NTL [41]. Each of these studies either directly investigates the overall convergence trend of NTL or uses NTL to characterize economic development and investigate spatial differences, convergence characteristics, and development trends of China’s regional economies, and help to shed light on the nature and heterogeneity of China’s regional economic growth model.
We believe that there are still some shortcomings in the perspectives and methods that use NTL to characterize regional economic development in research. From a research perspective, they ignore the similarities and differences between the economy represented by NTL and the economy as defined by GDP. Most studies are based on a positive correlation between NTL and the economy, directly using NTL data as a substitute for GDP data, without giving proper attention to the information that is provided by GDP but absent in NTL data, and the new information provided by NTL data but absent in GDP data. Although a few scholars have mentioned this concern, none have carried out an in-depth investigation or argumentation on the topic. In terms of research methodology, the current mainstream convergence research methods are combing with statistical indicators such as the δ convergence index, using the conditional β convergence or club convergence methods to examine the regional convergence phenomenon, and to look for the influencing factors for the regional convergence. However, these methods ignore the fact that China is in a “transition period.” When the economy must converge, and there is heterogeneity in the rate of convergence, if the income level and convergence rate of the underdeveloped regions are lower than the developed regions, there is a period in which developed regions grow faster than underdeveloped regions, but this does not affect their ultimate convergence. This period is called the “transition period.” In 1986, Deng Xiaoping put forward the Reform and Opening Up strategy of “Let a part of the population get rich first, they will carry more people into wealth, and eventually everyone will enjoy common prosperity.” Since then, China has been in the transitional period of economic transformation. Compared with the traditional tools for measuring and analyzing economic trends, the nonlinear time-varying factor model proposed by Phillips and Sul based on the convergence club division method can more accurately evaluate the transitional period of the Chinese economy [42]. This method came to be widely used in the economic convergence analysis worldwide after its proposal [43–49].
2 Data and Method
2.1 Data Sets
The most widely used NTL in the academic community is acquired by the Optical Imaging Linear Scanning Service sensor of the United States Digital Defense Meteorological Satellite Program (DMSP/OLS). The device effectively monitors radiation information such as city lights, fishing fires, and forest fires. It provides the world’s most extended time series (1992–2013) of nighttime light-to-earth observation data provided by the NOAA’s National Geophysical Data Center, including average visible lights, stable lights, and cloud-free coverage. In the stable lights images, unstable light sources such as aurora and wildfire and the interference of moonlight and clouds have been removed. The final data value is the annual average gray value of cloudless stable light, which provides a unique research perspective for human activity detection. Although the data has a low resolution (approximately l km) and lacks calibration and urban center saturation [50], the corrected DMSP/OLS data have become the most commonly used data source for NTL remote sensing research.
This article uses DMSP/OLS stable lights data from 34 provincial-level administrative regions in China from 1992 to 2013. It combines the NTL data with the GDP data to explore differences between the use of NTL and GDP in regional economic convergence research. The NTL data have been prepared and corrected by Cao et al. [51], based on well-established methods [25, 52, 53].
The average growth rates of NTL and GDP are then taken as research variables. The NTL growth rate of each region is calculated from the average value of the NTL from 1992 to 2013, so the growth rate data for a total of 21 years from 1993 to 2013. In order to remove the inflation effect, the real GDP growth rates from 1993 to 2013 are adopted in our analysis. The real GDP growth rates are retrieved from the National Bureau of Statistics and the Compilation of 60 Years of Statistics of the People’s Republic of China. The descriptive statistics for each variable can be found in Table 1.
Between 1993 and 2013, both GDP and NTL growth rates experienced several fluctuations, and the magnitude and extent of the changes did not show significant consistency. The regions with high GDP growth rates are Inner Mongolia, Guangdong, and Tianjin. The high-growth areas with NTL are Tibet, Yunnan, and Xinjiang. Whether it is GDP or NTL, the regions with lower growth rates are concentrated in Macao, Hong Kong, and Taiwan.
2.2 Club Convergence Test
The convergence club division method proposed by Phillips and Sul is to judge the convergence of economic growth and price index changes in a transitional economy at a given level of significance and to identify and divide the convergence clubs 42. The advantage of using this method lies in that it can fully consider the heterogeneity of observed entities without the need for more stringent economic assumptions about model parameters or convergence clubs, enabling the analytic framework to be applied to a broader range of complete data that characterizes economic development.
The club convergence test method is for a nonlinear time-varying factor model:
where
A semiparametric model is then constructed as follows:
where
which verifies the original hypothesis of convergence:
In Eq. 4,
The above test method is called the
2.3 The Ordered Logit Regression
In order to search out the various factors affecting club membership and evaluate their importance in determining club membership, this article employs the ordered logit model, based on the club convergence results, to identify the influencing factors [45]. This method can estimate how the explanatory variable variation changes the probability that a given region belongs to the affiliated club while fixing all other variables to its sample mean. In addition to the indicators of population growth rate, technological innovation covered by the neoclassical growth theory model, and human capital emphasized by the endogenous growth model, other factors that are mainly involved in the convergence of regional economic growth include the degree of opening up, marketization, employment rate, geographical factors, policy factors, and industries structures [54–60]. Considering the characteristics of China’s economic development and the results of club convergence, combined with factors that may affect economic activity and NTL, this article examines the influencing factors of club formation from six dimensions: capital investment, technological innovation, industrial structure, market vitality, economic openness, and resource consumption. Capital investment is divided into physical capital and human capital, where the physical capital is measured by fixed capital investment and the human capital is divided into the number of employees and population growth rate. The technological innovation is measured by the number of patents granted. The industrial structure is divided into industrial proportion and service industry proportion, which are respectively measured by the secondary industry output and the tertiary industry output for GDP proportion. Market vitality is measured by the total retail sales of social consumer goods. Economic openness is measured by the amount of foreign direct investment. Resource consumption is measured by the consumption of coal. In order to eliminate inflation, all nominal data are deflated based on 1993. We use the fixed asset investment price index to deflate fixed capital investment, the consumer price index to deflate consumer goods’ total retail sales, and the U.S. GDP deflation index to deflate foreign direct investment.
Considering that the club is divided according to the growth rate, all variables with the exceptions of population growth rate, industrial proportion, and service industry proportion use the increment data.
The data comes from the China Statistical Yearbook, the regional statistical yearbooks, and the China Energy Statistics Yearbook. Due to the lack of relevant data in Hong Kong, Macao, and Taiwan, convergence factor testing excludes these three regions, and only uses relevant data from the mainland’s 31 areas for the impact factor analysis.
3 Results
3.1 Club Convergence in GDP Growth Rates
According to Phillips and Sul’s recommendation 42, this article takes a value of
It can be seen that
Next, clubs are divided among Chinese provinces to identify economic convergence in the country. In accordance with the recommendations of Phillips and Sul, during the initial sorting process, the last
After excluding Inner Mongolia, Tianjin, Macao, and Guangdong, the
The trend of each region’s transition path is shown in the top panel of Figure 1. The club members have prominent convergence characteristics. In the first few years, Club 1 members’ transition paths are quite different, but in the subsequent years, it shows a characteristic of convergence. Club 2 members’ transition paths gradually narrowed in 2004 and 2005, and then the gap gradually widened, but it still converged to one club. The transition paths of Club 3 members are relatively consistent. The transition paths of Hong Kong and Taiwan included in Club 4 show a significant trend of convergence. The initial level of their transition paths is low, and the level of transition paths in 2013 is also low, which is significantly lower than the previous three clubs. It is worth noting that Macao, which has the lowest initial level, surpassed the transition paths of most regions and became a member of Club 1, reflecting the rapid growth of Macao’s GDP.
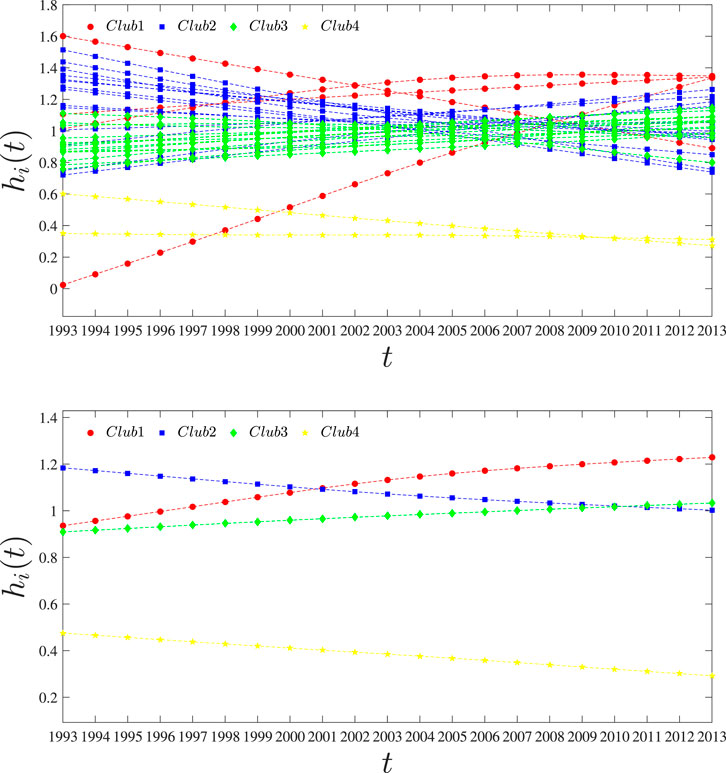
FIGURE 1. Top: transition path of GDP growth rate. Bottom: GDP convergence club average transition path.
At the same time, the club convergence results also showed obvious geographic effects. Both Club 1 and Club 2 are mainly concentrated in central and eastern China, except Tibet and Qinghai. While Club 3 is mostly concentrated in the western and northeast areas of China, the convergence result of the
Each club’s transition paths were averaged, and the transition trend of each club’s overall transition path is shown in the bottom panel of Figure 1. It can be seen from the figure that in the initial years of the time series, the transition paths of Club 1 and Club 3 is relatively concentrated, but then the gap gradually widened. Club 2’s transition path was the highest at first, but eventually lags behind Club 1, especially in 2001; it was a key point in reversing the transition paths of Club 1 and 2. Overall, clubs 1, 3, and 4’s transition paths show an upward trend, while the transition path of Club 2 shows a downward trend. That is to say, the GDP transition paths of the northeast and part of the central and western regions have an upward trend. Although the eastern region took the lead at first, it has subsequently shown a downward trend. One reason for the existence of this catching-up effect may be the “Western Development” and the “Rise of the Central China” strategies introduced by the Chinese government in the early 20th century; as investment in the central and western regions continued to increase, the economic growth rate also continued to grow and even surpassed that of the eastern area.
The transition path of Club 4 has continued to decline since 1992. It also markedly differs from the other three clubs and is the club with the lowest growth rate; this is due to the social system of Hong Kong and Taiwan differing from the mainland and can be regarded as exceptional cases.
In summary, there is evidence that the GDP growth rates of China’s 34 regions exhibited club convergence from 1992 to 2013. The club identification results reflect significant geographical features and are consistent with China’s regional economic development characteristics.
3.2 Club Convergence in Nighttime Light Growth Rates
In the convergence test of nighttime lights growth rate, we also take
It can be seen that
For the initial sorting process, the last 2/3 of the entire period’s data was selected for ranking. Based on this ranking, Tibet ranked first and was used as the reference region. Tibet, Jiangxi, Chongqing, Qinghai, Inner Mongolia, Sichuan, Guizhou, Gansu, Ningxia, Shaanxi, Hunan, and Yunnan were then added to carry out the
After excluding the members of the first club, the above test method was repeated to determine the second convergence club (Zhejiang, Jiangsu, Guangxi, Hainan, Heilongjiang, Hubei, Jilin, Fujian, Henan, Shandong, Liaoning, and Tianjin), the third convergence club (Shanxi, Guangdong, and Shanghai), the fourth convergence club (Hong Kong and Macao) and separate regions not belonging to any convergence clubs (Hebei, Beijing, and Taiwan). See Tables 4,5 for details. The
The trend of each region’s transition path is shown in the top panel of Figure 2. Except for Tibet, the growth rate of nighttime lights in all regions began to stabilize. From 2004, the growth rate gap between them has gradually opened, but there are still four clubs that are converging. Geographical effects still exist. Club 1 is mainly concentrated in the central and western China. Club 2 is mainly concentrated in the eastern and northeast areas, indicating that the convergence result also captures the spatial effect of NTL growth to a certain extent.
The average transition path of each club is averaged, and the transition trend of each club’s overall transition path is shown in the bottom panel of Figure 2. It can be seen from the graph that in the initial years of the time series, the transition paths of Clubs 1, 2, and 3 are relatively concentrated, and the initial growth rate of Club 4 (Hong Kongand Macao) is the lowest, even lower than the areas that do not converge. Subsequently, the transition path of Club 1 gradually increased, Club 2 had a slight downward trend, and Clubs 3 and 4 had significant downward trends. The difference in NTL growth rates between clubs has gradually increased.
In summary, there is evidence that the NTL growth rates of China’s 34 regions did not have an overall convergence during 1992–2013 but exhibited club convergence. The growth rates gap between different clubs increases with the year.
3.3 Robustness
In order to avoid the influence of the slow change function
The robustness tests for nighttime lights are shown in Table 7. The club size and members are exactly the same for
3.4 Convergence Factor Testing
Due to the lack of relevant data in Hong Kong, Macao, and Taiwan, Club 4 is not included in discussing the club convergence factors for GDP and NTL. According to the principles of club classification, as the club group rank increases, the growth rate of clubs in the corresponding region is lower; on the contrary, the lower the club group ranking, the higher the growth rate of clubs in the corresponding region. The overall fit of the GDP clubs gives
Excluding Hong Kong, Macao, Taiwan and nonconvergent regions, the overall fitting result of the NTL club gives
We further calculated the marginal effects of explanatory variables on GDP club division. The results are shown in columns 2, 3, and 4 of Table 8. Clubs 1 and 2 have the same direction for each variable, which is precisely the opposite of Club 3. Among these variables, the coefficients of patents increment, secondary industry proportion, and tertiary industry proportion are significant. If the patents increment increases by a mean of 1%, the likelihood that the region belongs to Clubs 1 and 2 will increase 0.046% and 0.077%, respectively, while the likelihood of belonging to Club 3 will decrease 0.124%. If the secondary industry proportion increases by a mean of 1%, the likelihood that the region belongs to Clubs 1 and 2 will increase 0.007% and 0.012%, respectively, while the likelihood of belonging to Club 3 will decrease 0.019%. If the mean tertiary industry proportion increases by 1%, the likelihood of the region belonging to Clubs 1 and 2 will increase 0.003% and 0.004%, respectively, while the likelihood of belonging to Club 3 will decrease 0.007%. These results indicate that technological innovation and industrial structure played an essential role in promoting economic growth. In fact, the results of marginal effects are consistent with the realities of economic development. The high GDP growth clubs are mainly concentrated in the eastern regions, which have an economic development level faster than the western regions. High-tech industries are mainly concentrated in the east developed areas, and the industrial and service industries have developed rapidly since China’s economic transformation, which was initially put forward in 2001. This is an important reason why the eastern regions’ economic growth is higher than that of the central and western regions.
The marginal effect of explanatory variables on NTL club division can be seen in Table 8. Clubs 2 and 3 have the same direction of change for each variable, which is precisely the opposite of Club 1. When these data are combined with the NTL transition paths (bottom panel of Figure 2), it can be found that the transition path of Club 1 is gradually increasing, that is, the NTL growth rates increase with time and regarded as a high growth rates club. The transition paths of Clubs 2 and 3 have a downward trend, that is, the NTL growth rates decline with time and regarded as a low growth rates clubs. From the results presented in Table 8, it can be found that, with the increase of fixed capital investment, population growth rate, patents increment, foreign direct investment, and coal consumption increment, the probability that a region has a high growth rate of NTL will increase. Increases in employee increment, the secondary industrial proportion, the tertiary industrial proportion, and retail sales of social consumer goods will increase the probability that a region will have a low growth rate of NTL. The marginal effect of the population growth rate, patents increment, secondary industrial proportion, tertiary industrial proportion, foreign direct investment, and coal consumption increment is significant. If the population growth rate mean increases by 1%, the probability of region belonging to Club 1 will increase 0.023%, while the probability of belonging to Clubs 2 and 3 will decrease 0.014% and 0.009%, respectively. If the patent increment mean increases by 1%, the probability of belonging to Club 1 will increase 0.185%, while the probability of belonging to Clubs 2 and 3 will decrease 0.109% and 0.076%, respectively. If the secondary industrial proportion mean increases by 1%, the probability of belonging to Club 1 will decrease 0.017%, while the probability of belonging to Clubs 2 and 3 will increase 0.01% and 0.007%. If the mean tertiary industrial proportion is increased by 1%, the probability of belonging to Club 1 will decrease 0.017%, while the probability of belonging to Clubs 2 and 3 will increase by 0.01% and 0.007%, respectively. The influence of foreign direct investment and coal consumption increment can also be observed from Table 8.
3.5 Comparison of GDP and NTL Growth Rate Convergence Characteristics
From the perspective of club membership, we identified the common regions where the club divisions’ results coexist. We refer to these common areas as groups. Group A includes six regions (Tibet, Chongqing, Qinghai, Guizhou, Shaanxi, and Anhui), Group B contains seven regions (Jiangxi, Sichuan, Gansu, Ningxia, Hunan, Yunnan, and Xinjiang), Group C includes six regions (Jiangsu, Shandong, Fujian, Hubei, Henan, and Zhejiang), and Group D includes five regions (Guangxi, Hainan, Heilongjiang, Jilin, and Liaoning). These four groups and their corresponding clubs all appear in the two different club division results, as shown in Table 9. It can be clearly seen that in terms of the order of regional growth rate, the results of the two clubs show certain degree of difference.
From the perspective of regional distribution, the maps in Figure 3 show that in GDP convergence clubs, the regions in higher ranked clubs are mainly concentrated in the central and eastern areas, that is, these regions have higher GDP growth rates. The western and northeast regions belong to clubs with a relatively low ranking and these regions have relatively low GDP growth rates. Simultaneously, Tibet and Qinghai are located in the west but are divided into the same club as the eastern coastal areas, indicating that Tibet and Qinghai’s GDP growth rates are higher than those of other western regions. In the NTL convergence clubs, the regions in higher ranked clubs are mainly concentrated in the west and central areas, which means these regions have higher NTL growth rates. The eastern and northeast regions belong to clubs with a relatively low ranking, which means these regions have relatively low NTL growth rates.
Judging from the gap in transition paths between clubs, the difference between the two types of data is significant. Due to the particularity of the data in Hong Kong, Macao, and Taiwan, only the 31 regions in the mainland are considered. The transition paths of GDP Clubs 1, 2, and 3 show a catching-up effect. The transition paths between clubs first converge, then diverge, with a relatively flat path of divergence. The transition paths of each club are in line with the patterns of linear development. The transition paths of Clubs 1 and 3 are on rise, while Club 2 is on decline. The transition paths of NTL Clubs 1, 2, and 3 do not show a catching-up effect. With the annual increase, the transition path of each club grew intenser, showing a nonlinear development pattern. The transition path of Club 1 is on the rise, and the transition paths of Clubs 2, 3, and 4 show a downward trend.
From the perspective of influencing factors of club division, fixed capital investment increment, population growth rate, patents increment, foreign direct investment increment, and coal consumption increment have the same direction of influence on the GDP growth rate and NTL growth rate, but there are subtle differences in the significance. For the GDP growth rate, the most significant factor is patents increment, followed by industrial proportion and industrial proportion. For the NTL growth rate, in addition to the above three variables, population growth rate, foreign direct investment increment, and coal consumption increment are also significant.
4 Conclusion
With the development of science and technology, the easily accessible and more objective NTL data is increasingly valued and used as a surrogate variable for economic growth. Besides, China is undergoing a transitional period characterized by strategic developmental opportunities and social tensions. The consideration of regional economic convergence where convergence speed is heterogeneous is crucial for the coordinated development of regional economies. To explore the reliability of economic variables in NTL data, from the perspective of regional economic convergence, the
This article further explored the club convergence mechanisms. We have found that patents increment, industrial proportion, and service industry proportion have a significant impact on both GDP and NTL. Among them, the increase in the proportion of industry and service industry has opposite effects on GDP clubs and NTL clubs, while the increase in patent increment has the same impact on GDP clubs and NTL clubs. This confirms that the industrial structure of eastern coastal areas has been upgraded faster than central and western regions. In addition, we found that, for NTL clubs, population growth rate, foreign direct investment, and coal consumption are also significant factors. An increase in population growth rate, foreign direct investment, and coal consumption will increase the probability that a region belongs to a high-growth club. It explains that there are also certain differences between GDP clubs and NTL clubs in terms of influencing factors.
On the whole, from 1992 to 2013, there were certain differences in the results of club convergence between China’s provincial NTL and GDP growth. The difference between NTL and GDP growth is mainly reflected in regions and influencing factors. High GDP growth regions correspond to low NTL growth regions, while low GDP growth regions correspond to high NTL growth regions. GDP growth is mainly influenced by technological innovation and industrial structure, while NTL growth is mainly affected by the population growth rate, technological innovation, industrial structure, opening to the international world, and resource consumption. The impact of industrial structure on GDP and NTL is the opposite. At present, when GDP statistics are difficult to obtain or are of low quality, a large number of studies use NTL brightness as a representative of the level of economic development. But judging from the results of this work, NTL is not a good substitute for GDP. Our results suggest that by distinguishing the connotations and differences between GDP and NTL and combining the characteristics of the two, a more reasonable alternative indicator of economic development can be constructed to make it more in line with the specific facts and laws of economic activities.
Data Availability Statement
Publicly available datasets were analyzed in this study. This data can be found here: https://ngdc.noaa.gov/eog/download.html.
Author Contributions
WZ conceived the research. QX analyzed the data and prepared the figures. WZ and QX analyzed the results and wrote the paper. All authors discussed and reviewed the manuscript.
Funding
This work was partly supported by the Fundamental Research Funds for the Central Universities.
Conflict of Interest
The authors declare that the research was conducted in the absence of any commercial or financial relationships that could be construed as a potential conflict of interest.
References
1. Bennett MM, Smith LC Advances in using multi-temporal night-time lights satellite imagery to detect, estimate, and monitor socioeconomic dynamics. Remote Sens Environ (2017) 192:176–97. doi:10.1016/j.rse.2017.01.005
2. Rawski TG Chinese industrial production, 1952-1971. Rev Econ Stat (1973) 55:169–81. doi:10.2307/1926991
3. Elvidge CD, Baugh KE, Kihn EA, Kroehl HW, Davis ER, Davis CW Relation between satellite observed visible-near infrared emissions, population, economic activity and electric power consumption. Int J Remote Sens (1997) 18:1373–9. doi:10.1080/014311697218485
4. Doll CNH, Muller JP, Elvidge CD Night-time imagery as a tool for global mapping of socioeconomic parameters and greenhouse gas emissions. Ambio: J Human Environ (2000) 29:157–62. doi:10.1579/0044-7447-29.3.157
5. Sutton PC, Costanza R Global estimates of market and non-market values derived from nighttime satellite imagery, land cover, and ecosystem service valuation. Ecol Econ (2002) 41:509–27. doi:10.1016/S0921-8009(02)00097-6
6. Sutton PC, Elvidge CD, Ghosh T Estimation of gross domestic product at sub-national scales using nighttime satellite imagery. Int J Ecol Econ Stat (2007) 8:5–21.
7. Ghosh T, Anderson S, Powell R, Sutton PC, Elvidge CD Estimation of Mexico’s informal economy and remittances using nighttime imagery. Remote Sens (2009) 1:418–44. doi:10.3390/rs1030418
8. Ghosh T, Powell RL, Elvidge CD, Baugh KE, Sutton PC, Anderson S Shedding light on the global distribution of economic activity. Open Geogr J (2010) 3:147–60. doi:10.2174/1874923201003010147
9. Elvidge CD, Baugh KE, Zhizhin M, Hsu FC Why VIIRS data are superior to DMSP for mapping nighttime lights. Proc Asia-Pacific Adv Netw (2013) 35:62–9. doi:10.7125/APAN.35.7
10. Ebener S, Murray C, Tandon A, Elvidge CC From wealth to health: modelling the distribution of income per capita at the sub-national level using night-time light imagery. Int J Health Geogr (2005) 4:5. doi:10.1186/1476-072X-4-5
11. Doll CNH, Muller JP, Morley JG Mapping regional economic activity from night-time light satellite imagery. Ecol Econ (2006) 57:75–92. doi:10.1016/j.ecolecon.2005.03.007
12. Blumenstock JE Fighting poverty with data. Science (2016) 353:753–4. doi:10.1126/science.aah5217
13. Li D, Li X Applications of night-time light remote sensing in eveluation of socioeconomic development. J Macro-quality Res (in Chinese) (2015) 3:1–8. doi:10.13948/j.cnki.hgzlyj.2015.04.001
14. Mellander C, Lobo J, Stolarick K, Matheson Z Night-time light data: a good proxy measure for economic activity?. PLoS ONE (2015) 10:e0139779. doi:10.1371/journal.pone.0139779
15. Imhoff ML, Lawrence WT, Elvidge CD, Paul T, Levine E, Privalsky MV, et al. Using nighttime DMSP/OLS images of city lights to estimate the impact of urban land use on soil resources in the United States. Remote Sens Environ (1997) 59:105–17. doi:10.1016/s0034-4257(96)00110-1
16. Chen J, Zhuo L, Shi PJ, Ichinose T The study on urbanization process in China based on DMSP/OLS data :Development of a light index for urbanization level estimation. J Remote Sens (In Chinese) (2003) 7:168–75. doi:10.11834/jrs.20030302
17. Small C, Pozzi F, Elvidge CD Spatial analysis of global urban extent from DMSP-OLS night lights. Remote Sens Environ (2005) 96:277–91. doi:10.1016/j.rse.2005.02.002
18. Noor AM, Alegana VA, Gething PW, Tatem AJ, Snow RW Using remotely sensed night-time light as a proxy for poverty in Africa. Popul Health Metrics (2008) 6:1–13. doi:10.1186/1478-7954-6-5
19. Zhang QL, Seto KC Mapping urbanization dynamics at regional and global scales using multi-temporal DMSP/OLS nighttime light data. Remote Sens Environ (2011) 115:2320–9. doi:10.1016/j.rse.2011.04.032
20. Stathakis D, Tselios V, Faraslis I Urbanization in European regions based on night lights. Remote Sensing Appl Soc Environ (2015) 2:26–34. doi:10.1016/j.rsase.2015.10.001
21. Ma T, Zhou YK, Zhou CH, Haynie S, Pei T, Xu T Night-time light derived estimation of spatio-temporal characteristics of urbanization dynamics using DMSP/OLS satellite data. Remote Sens Environ (2015) 158:453–64. doi:10.1016/j.rse.2014.11.022
22. Amaral S, Camara G, Monteiro AMV, Quintanilha JA, Elvidge CD Estimating population and energy consumption in Brazilian Amazonia using DMSP night-time satellite data. Comput Environ Urban Syst (2005) 29:179–95. doi:10.1016/j.compenvurbsys.2003.09.004
23. Amaral S, Monteiro AMV, Camara G, Quintanilha JA DMSP/OLS night-time light imagery for urban population estimates in the Brazilian Amazon. Int J Remote Sens (2006) 27:855–70. doi:10.1080/01431160500181861
24. Axbard S Income opportunities and sea piracy in Indonesia: evidence from satellite data. Appl Econ (2016) 8:154–94. doi:10.1257/app.20140404
25. Elvidge CD, Ziskin D, Baugh KE, Tuttle BT, Ghosh T, Pack DW, et al. A fifteen year record of global natural gas flaring derived from satellite data. Energies (2009) 2:595–622. doi:10.3390/en20300595
26. Chen X, Nordhaus WD Using luminosity data as a proxy for economic statistics. Proc Natl Acad Sci U.S.A (2011) 108:8589–94. doi:10.1073/pnas.1017031108
27. Henderson V, Storeygard A, Weil DN A bright idea for measuring economic growth. Amer Econ Rev (2011) 101:194–9. doi:10.1257/aer.101.3.194
28. Henderson JV, Storeygard A, Weil DV Measuring economic growth from outer space. Amer Econ Rev (2012) 102:994–1028. doi:10.1257/aer.102.2.994
29. Chen X, Nordhaus W A test of the new VIIRS lights data set: population and economic output in Africa. Remote Sens (2015) 7:4937–47. doi:10.3390/rs70404937
30. Donaldson D, Storeygard A The view from above: applications of satellite data in economics. J Econ Persp (2016) 30:171–98. doi:10.1257/jep.30.4.171
31. Ma T, Zhou CH, Pei T, Haynie S, Fan JF Quantitative estimation of urbanization dynamics using time series of DMSP/OLS nighttime light data: a comparative case study from China’s cities. Remote Sens Environ (2012) 124:99–107. doi:10.1016/j.rse.2012.04.018
32. Yu B, Shi K, Hu Y, Huang C, Chen Z, Wu J Poverty evaluation using NPP-VIIRS nighttime light composite data at the county level in China. IEEE J Sel Top Appl Earth (2015) 8:1217–29. doi:10.1109/JSTARS.2015.2399416
33. Shi K, Yu B, Huang Y, Hu Y, Yin B, Chen Z, et al. Evaluating the ability of NPP-VIIRS nighttime light data to estimate the gross domestic product and the electric power consumption of China at multiple scales: a comparison with DMSP-OLS data. Remote Sens (2014) 6:1705–24. doi:10.3390/rs6021705
34. Xu K, Chen F, Liu X The truth of China economic growth: evidence from global night-time light data. Econ Res J (2015) 50:17–29. [in Chinese].
35. Li X, Xu HM, Chen XL, Li C Potential of NPP-VIIRS nighttime light imagery for modeling the regional economy of China. Remote Sens (2013) 5:3057–81. doi:10.3390/rs5063057
36. Huang X, Schneider A, Friedl MA Mapping sub-pixel urban expansion in China using MODIS and DMSP/OLS nighttime lights. Remote Sens Environ (2016) 175:92–108. doi:10.1016/j.rse.2015.12.042
37. He C, Ma Q, Li T, Yang Y, Liu Z Spatiotemporal dynamics of electric power consumption in Chinese Mainland from 1995 to 2008 modeled using DMSP/OLS stable nighttime lights data. J Geogr Sci (2012) 22:125–36. doi:10.1007/s11442-012-0916-3
38. Liu HJ, Pei YF, Jia WX Spatial differences and spillover effects of economic development of urban agglomerations in China: on DMSP/OLS nighttime light data from 1992 to 2013. Finance Trade Res (in Chinese) (2017) 28:1–12. doi:10.19337/j.cnki.34-093/f.2017.11.001
39. Liu HJ, Du GJ Regional inequality and stochastic convergence in China. J Quant Tech Econ (2017) 2017:43–59. [in Chinese]. doi:10.13653/j.cnki.jqte.2017.10.003
40. Wang XB, Huang LX, Xu XX, Li X Revisit China’s regional economic convergence: evidence from the DMSP/OLS night light data. Chin Econ Q (in Chinese) (2017) 16:877–96.
41. Ding HF, Zhou YX Viewing the spatial and temporal pattern of regional economic development in China from night light. Macroeconomics (2017) 2017:130–8.[in Chinese]. doi:10.16304/j.cnki.11-3952/f.2017.03.011
42. Phillips PCB, Sul D Transition modeling and econometric convergence tests. Econometrica (2007) 75:1771–855. doi:10.1111/j.1468-0262.2007.00811.x
43. He Y Economic convergence in transition economy of China: an empirical study with a nonlinear time-varying factor model. Econ Res J (2008) 2008:39–51.[in Chinese].
44. Fischer C Price convergence in the EMU? Evidence from micro data. Eur Econ Rev (2012) 56:757–76. doi:10.1016/j.euroecorev.2012.01.008
45. Bartkowska M, Riedl A Regional convergence clubs in Europe: identification and conditioning factors. Econ Model (2012) 29:22–31. doi:10.1016/j.econmod.2011.01.013
46. Meng H, Xie WJ, Zhou WX Club convergence of house prices: evidence from China’s ten key cities. Int J Mod Phys B (2015) 29:1550181. doi:10.1142/S0217979215501817
47. Tian X, Zhang XH, Zhou YH, Yu XH Regional income inequality in China revisited: a perspective from club convergence. Econ Model (2016) 56:50–8. doi:10.1016/j.econmod.2016.02.028
48. Lyncker KV, Thoennessen R Regional club convergence in the EU: evidence from a panel data analysis. Empir Econ (2017) 52:525–53. doi:10.1007/s00181-016-1096-2
49. Sun XH, Cao Y Convergence of clubs for urban economic growth:Identification methods and convergence mechanisms–An empirical test from 347 administrative regions in China. Mod Econ Sci (in Chinese) (2018) 40:14–25.
50. Ma L, Wu J, Li W, Peng J, Liu H Evaluating saturation correction methods for DMSP/OLS nighttime light data: a case study from China’s cities. Remote Sens (2014) 6:9853–72. doi:10.3390/rs6109853
51. Cao ZY, Wu ZF, Kuang YQ, Huang NS Correction of DMSP/OLS night-time light images and it’s application in China. J Geo-Information Sci (in Chinese) (2015) 17:1092–102. doi:10.3724/SP.J.1047.2015.01092
52. Wu J, He S, Peng J, Li W, Zhong X Intercalibration of DMSP-OLS night-time light data by the invariant region method. Int J Remote Sens (2013) 34:7356–68. doi:10.1080/01431161.2013.820365
53. Liu Z, He C, Zhang Q, Huang Q, Yang Y Extracting the dynamics of urban expansion in China using DMSP-OLS nighttime light data from 1992 to 2008. Landsc Urban Plan (2012) 106:62–72. doi:10.1016/j.landurbplan.2012.02.013
54. Cai F, Du Y Convergence and divergence of regional economic growth in China. Econ Res J (2000) 2000:30–7. [in Chinese].
55. Shen KR, Ma J The characteristics of “Club Convergence” of China’s economic growth and it’s cause. Econ Res J (2002) 2002:33–9. [in Chinese].
56. Lin YF, Liu PL Chinese development strategy and economic convergence. Econ Res J (2003) 2003:19–25. [in Chinese].
57. Tang XB, Chen XS Analysis of economic convergence and its influence factors in eight major regions of China. J Renmin Univ China (2007) 2007:106–13. [in Chinese].
58. Pan XF, Yang Y Rsearch on regional innovation club convergence and influencing factors in China. Stud Sci Sci (in Chinese) (2014) 32:130–8. doi:10.16192/j.cnki.1003-2053.2014.02.015
59. Zhu GZ, Qiao KY, Yu JH Is provincial economic growth convergence in China?. Chin Econ Q (in Chinese) (2014) 13:1171–94. doi:10.13821/j.cnki.ceq.2014.03.016
Keywords: nighttime lights, GDP, regional economies, club convergence, log t test
Citation: Xiao Q-L, Wang Y and Zhou W-X (2021) Regional Economic Convergence in China: A Comparative Study of Nighttime Light and GDP. Front. Phys. 9:525162. doi: 10.3389/fphy.2021.525162
Received: 08 January 2020; Accepted: 04 February 2021;
Published: 23 April 2021.
Edited by:
Valerio Capraro, Middlesex University, United KingdomReviewed by:
Gholamreza Jafari, Shahid Beheshti University, IranXu Tian, Nanjing Agricultural University, China
Copyright © 2021 Xiao, Wang and Zhou. This is an open-access article distributed under the terms of the Creative Commons Attribution License (CC BY). The use, distribution or reproduction in other forums is permitted, provided the original author(s) and the copyright owner(s) are credited and that the original publication in this journal is cited, in accordance with accepted academic practice. No use, distribution or reproduction is permitted which does not comply with these terms.
*Correspondence: Wei-Xing Zhou, d3h6aG91QGVjdXN0LmVkdS5jbg==